the Creative Commons Attribution 4.0 License.
the Creative Commons Attribution 4.0 License.
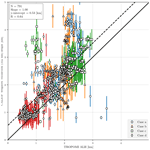
A first comparison of TROPOMI aerosol layer height (ALH) to CALIOP data
Swadhin Nanda
Martin de Graaf
J. Pepijn Veefkind
Maarten Sneep
Mark ter Linden
Jiyunting Sun
Pieternel F. Levelt
The TROPOspheric Monitoring Instrument (TROPOMI) level-2 aerosol layer height (ALH) product has now been released to the general public. This product is retrieved using TROPOMI's measurements of the oxygen A-band, radiative transfer model (RTM) calculations augmented by neural networks and an iterative optimal estimation technique. The TROPOMI ALH product will deliver ALH estimates over cloud-free scenes over the ocean and land that contain aerosols above a certain threshold of the measured UV aerosol index (UVAI) in the ultraviolet region. This paper provides background for the ALH product and explores its quality by comparing ALH estimates to similar quantities derived from spaceborne lidars observing the same scene. The spaceborne lidar chosen for this study is the Cloud-Aerosol LIdar with Orthogonal Polarization (CALIOP) on the Cloud-Aerosol Lidar and Infrared Pathfinder Satellite Observation (CALIPSO) mission, which flies in formation with NASA's A-train constellation since 2006 and is a proven source of data for studying ALHs. The influence of the surface and clouds is discussed, and the aspects of the TROPOMI ALH algorithm that will require future development efforts are highlighted.
A case-by-case analysis of the data from the four selected cases (mostly around the Saharan region with approximately 800 co-located TROPOMI pixels and CALIOP profiles in June and December 2018) shows that ALHs retrieved from TROPOMI using the operational Sentinel-5 Precursor Level-2 ALH algorithm is lower than CALIOP aerosol extinction heights by approximately 0.5 km. Looking at data beyond these cases, it is clear that there is a significant difference when it comes to retrievals over land, where these differences can easily go over 1 km on average.
- Article
(19658 KB) - Companion paper 1
- Companion paper 2
- BibTeX
- EndNote
Aerosols are an important component of the Earth system, which provide the means for the formation of clouds by acting as cloud condensation nuclei, affecting the Earth's radiation budget by absorbing or scattering incoming solar radiation (Twomey, 1974), and even nurturing forests from across oceans (Yu et al., 2015; Barkley et al., 2019). A significant source of aerosols to the Earth's atmosphere is natural, followed by the anthropogenic contribution. The IPCC (2014) report has made it clear that the current scientific consensus acknowledges the impact of aerosols on the Earth's radiation budget via direct, indirect and semi-direct effects. What makes monitoring aerosols difficult is the high spatial and temporal variability of aerosol micro- and macrophysical properties (Li et al., 2009). To that extent, there are several spaceborne, ground-based and airborne missions extensively monitoring these aerosol micro- and macrophysical properties. These missions aim to reduce the gaps in our knowledge of aerosol radiative effects by accurately measuring aerosol properties at a high spatial and temporal resolution. This paper specifically discusses retrieving information on the vertical distribution of aerosol layers in the atmosphere, which has significant relevance in deriving auxiliary aerosol properties and subsequently understanding aerosol radiative effects (ARE), primarily absorption of radiation by aerosols. Torres et al. (1998) explicitly mention the importance of knowledge about the aerosol vertical distribution, which can be used in tandem with the UV aerosol index (UVAI) to compute aerosol properties such as aerosol optical thickness (AOT) and effective single scattering albedo over cloud-free and snow-free scenes. The paper by de Graaf (2005) provides several sensitivity analyses that detail the importance of the aerosol height in interpreting the UVAI. Sun et al. (2018) explicitly mention in their study the requirement of accurate aerosol layer height (ALH) estimates in order to derive aerosol absorption from the UVAI.
The global monitoring of aerosol properties can only be done using remote sensing techniques from space. The space-based techniques currently utilised by the scientific community to retrieve aerosol vertical information are divided into two categories – active and passive techniques; active remote sensing techniques monitor aerosol properties by measuring the interaction of energy generated by the instrument with aerosols in the atmosphere, whereas passive techniques do the same by measuring the interaction of natural light with aerosol particles. There are several differences in the sensing principles between active and passive remote sensing of aerosols, specifically in terms of vertical resolution. Active sensors such as the Cloud-Aerosol LIdar with Orthogonal Polarization (CALIOP) instrument provide attenuated backscatter profiles resolved vertically at a vertical resolution as fine as 30 m for different channels over a spatial resolution as fine as 0.33 km (see Table 2 of Winker et al., 2009). While these measured backscatter profiles provide detailed quantitative information on the scattering components present in the atmosphere, spaceborne atmospheric profiling lidars have limited spatial coverage due to their limited beam width. Owing to this particular feature of active remote sensing, spaceborne lidars currently do not revisit a specific point on Earth several times a day or even on a daily basis. On the other hand, passive spaceborne remote sensing has the ability to measure a specific point on Earth once a day for polar-orbiting satellite missions and several times a day for geostationary satellite missions. Currently, however, the retrieved information on aerosol vertical distribution from passive remote sensing techniques is much more limited when compared to active techniques such as orbiting lidars.
Several passive retrieval strategies that are either currently in their operational phase or are upcoming remote sensing missions utilise the interaction of incoming solar radiation with the aerosol particles to retrieve height information. Some notable mentions of missions that retrieve ALH are the Multi-angle Imaging SpectroRadiometer (MISR) on board the NASA Terra satellite (Nelson et al., 2013), which measures aerosol height using geometric optics; the Deep Space Climate Observatory (DSCOVR) mission with its Earth Polychromatic Imaging Camera (EPIC) (Xu et al., 2017, 2019); the Ozone Monitoring Instrument (OMI) on board the NASA Aura satellite (Chimot et al., 2017, 2018; Choi et al., 2019); and finally the TROPOspheric Monitoring Instrument (TROPOMI) instrument on board the Sentinel-5 Precursor satellite (Veefkind et al., 2012). Xu et al. (2017, 2019) are the first studies to demonstrate that the diurnal cycle of aerosol height is retrievable. In the near future, missions like the upcoming Multi-Angle Imager for Aerosols (MAIA) mission (Davis et al., 2017), the Geostationary Environment Monitoring Spectrometer (GEMS) (Kim et al., 2019), Sentinel-4, Sentinel-5 (Ingmann et al., 2012) and the Tropospheric Emissions: Monitoring Pollution mission (TEMPO) (Zoogman et al., 2017) are expected to provide aerosol height retrievals as well (Kim et al., 2018; Park et al., 2016; Zoogman et al., 2017). These instruments are examples of missions demonstrably more (some theoretically, others practically) capable of retrieving ALH. Except for TROPOMI, however, there are currently no passive remote sensing missions that provide an operational stream of retrieved ALHs. In the fourth quarter of 2019, an operational data stream of retrieved ALHs derived from measured oxygen A-band spectra by TROPOMI was made available to the general public; the TROPOMI operational UVAI product augmented by the TROPOMI ALH product has the potential to further the operational monitoring of aerosol properties globally. This paper discusses some key features of the product and its limitations by comparing it with co-located CALIOP profiles, and it paints a future outlook of the evolution of the TROPOMI ALH algorithm. This paper looks into more than 2 million co-locations between TROPOMI ground pixels and CALIOP profiles over an extended period of time covering several months from May 2018 until March 2019 in order to draw conclusions on the accuracy of the TROPOMI ALH retrievals. Further on, the paper also discusses four selected cases in and around West Africa for a deeper analysis of the comparison with CALIOP data; the choice of using Africa as a study area arises from the fact that a significant majority of co-locations between TROPOMI and CALIOP are concentrated around the West African region.
In Sect. 2 of this paper, we discuss the data and methods used in this paper; Sect. 2.1 describes the retrieval algorithm and highlights different diagnostic parameters available for assessing the product's quality. Following this, the comparison between CALIOP and TROPOMI estimates of aerosol heights is presented in Sect. 3. Section 3.1 presents an overall analysis of a large number of TROPOMI–CALIOP co-locations, followed by Sect. 3.2 which discusses selected cases for a deeper dive into the TROPOMI product. The paper concludes with Sect. 4, highlighting important areas of potential improvement in the current TROPOMI ALH product.
2.1 TROPOMI ALH
The TROPOMI ALH product is derived from measurements of the oxygen A-band in the near-infrared region between 758 and 770 nm. Within this spectral range, TROPOMI measures top-of-atmosphere radiances and solar irradiances with a spectral resolution between 0.34 and 0.35 nm and a spectral sampling of 0.126 nm. The retrieval algorithm exploits the absorption characteristics of molecular oxygen, which varies with the photon path length – the photon path length for an aerosol layer closer to the surface is longer, which appears as deeper oxygen absorption lines in the measured spectrum (see Fig. 1 of Nanda et al., 2018a).
The reported ALH is the height of a single aerosol layer for the entire atmospheric column within the scene measured by TROPOMI; in reality, however, there can be several cases where distinctly separated elevated and boundary layer aerosols are present in the same scene. In such cases, the retrieval algorithm is expected to retrieve an optical centroid pressure or height of the two (or more) aerosol layers, depending on the atmospheric level of the aerosol layer from which most of the photons are scattered back. For instance, if the elevated aerosol layer contributes significantly more than the boundary layer aerosols to the top-of-atmosphere measured spectra, the ALH retrieval algorithm is expected to retrieve values closer to the elevated layer.
The technique for retrieving ALH is based on optimal estimation (Rodgers, 2000), where a radiative transfer model (RTM) that calculates the top-of-atmosphere oxygen A-band spectra is fitted to TROPOMI measured oxygen A-band spectra. The cost function that is minimised in this estimation step, χ2, is defined as
where y is the reflectance spectra calculated from measured radiances and irradiances for the oxygen A-band; F(x,b) is the modelled reflectance for input parameters b, of which the state vector x containing ALH zaer and AOT τ is a part; xa is the a priori state vector; and and are the measurement error covariance and the a priori error covariance matrices. Optimal estimation is an iterative process, requiring several iterations to minimise the cost function described in Eq. (1). The approach is Gauss–Newton, with a maximum number of iterations set at 10. If the optimal estimation does not converge within these iterations, the ALH field in the final level-2 product is filled with a fill value. For a given measurement, optimal estimation is said to have converged to a final solution if the update to the state vector for the next iteration is less than the expected precision.
The χ2 is a measure of how close the modelled sun-normalised radiances are to the observations, with smaller values representing a better fit. The consequence of the many assumptions in the model (described in Sect. 2.2 of Nanda et al., 2019) result in a large χ2 (of the order of 1×104 to 1×107), with larger χ2 representing a larger departure between the model and the observation. There are several reasons for these departures, the more important ones being the presence of undetected clouds in the scene, incorrect surface reflectance information and multiple aerosol layers. These attributes are not parameterised into the RTM, and they can be a source of discrepancies between the measured and the modelled reflectances. The RTM in this case is a neural network model that has learnt parts of a full physics RTM derived from de Haan et al. (1987) called Determining Instrument Specifications and Analyzing Methods for Atmospheric Retrieval (DISAMAR; described in Sect. 3 of Nanda et al., 2019), which is 3 orders of magnitude faster than DISAMAR. In short, the atmosphere is simplified by DISAMAR in order to reduce computational burden, and the neural network forward model is implemented for a further performance boost in an operational environment; for instance, DISAMAR ignores rotational Raman scattering even though the literature has shown that the oxygen A-band ring effects are sensitive to ALH (Vasilkov et al., 2013; Wagner et al., 2010). These decisions have been made in order to speed up line-by-line calculations of DISAMAR, which are the basis of the training data for its neural network counterpart. This decision is motivated by preliminary sensitivity analyses conducted by Sanders and de Haan (2016) which conclude that the effect of ignoring rotational Raman scattering (RRS) is not significant enough to venture into its implementation in the forward model.
The surface reflectance model used in the algorithm is derived from Tilstra et al. (2017), which is a Lambertian equivalent reflectance (LER) database with a spatial resolution of ∘. In contrast to TROPOMI's ALH product, which is reported at 7.2 km×3.6 km until 6 August 2019 and 5.6 km×3.6 km thereafter, the LER database is much coarser spatially. This can lead to several artefacts in the final product, discussed further on in this paper in Sect. 3.2. Another issue to note is in the influence of bright surfaces on the retrieval. The oxygen A-band lies beyond the red edge, a wavelength region in which vegetation has high reflectance values. This poses several challenges; a significant portion of the measured signal over land might be contributions from the surface reflectance (see Fig. 3 from Nanda et al., 2018a). If the AOT of the measured scene is low, the contribution of the surface to the top-of-atmosphere radiance dominates over the contribution from scattering by aerosols – there are more photons that get scattered back from the surface than the aerosol layer. In such cases, the retrieval algorithm will tend to retrieve an aerosol layer closer to the surface. Generally we find that if the contribution to the top-of-atmosphere reflectance from aerosols is significantly larger than the same from the surface (i.e. the aerosol layer appears brighter than the surface), the retrieval algorithm will tend to retrieve a height closer to the aerosol layer. (Sect. 5.2 and Fig. 10 from Nanda et al., 2018b, discusses this observation explicitly.)
The forward model parameterises aerosols with a Henyey–Greenstein scattering phase function (Henyey and Greenstein, 1941) with an asymmetry factor of 0.7, a single scattering albedo of 0.95 and a fixed AOT for an aerosol layer parameterised by a single atmospheric layer with a 50 hPa thickness. These assumptions have to be made since very little a priori information about aerosols in a scene is known. While more complex scattering models exist, the Henyey–Greenstein model has been used for retrieving ALH when the forward model was of a line-by-line nature as the number of calculations it requires is far less than a scattering model such as the Mie model (de Rooij and van der Stap, 1984). Sensitivity analyses have shown that this assumption has few ramifications (Sanders et al., 2015). Fixing the single scattering albedo is a much bigger concern; while retrievals over the ocean do not suffer from a priori errors in the single scattering albedo, retrievals over land do have large errors and non-convergences which reduce as the viewing zenith angle increases (Nanda et al., 2018a). The choice of using 0.95 as a fixed value arises from average values derived by Dubovik et al. (2002) from long-term observations using the AErosol RObotic NETwork (AERONET; Holben et al., 1998). The algorithm assumes a single aerosol layer for the entire atmosphere, within which aerosols are uniformly distributed and the aerosol volume extinction coefficient is constant. This is an important simplification to note when comparing with CALIOP profiles, since these lidar profiles have the capability to detect multiple aerosol layers. The simplicity in the aerosol profile parameterisation arises from the fact that it is impossible to know, without prior information, whether the scene consists of a single layer or multiple aerosol layers. While fitting of the aerosol layer pressure thickness along with the aerosol layer mid pressure does not result in large errors in the retrieved ALH, the precision of the retrieved aerosol layer mid pressure significantly deteriorates with increasing errors in the surface albedo (Sanders et al., 2015). More research has to be done before more information on the aerosol profile is retrieved from the oxygen A-band alone.
Finally, the ALH retrieval algorithm implements a pixel selection scheme before committing to retrieving ALH estimates. This pixel selection scheme involves auxiliary data products from TROPOMI such as the UVAI (http://www.tropomi.eu/document/atbd-uv-aerosol-index, last access: 8 June 2020) and cloud fraction estimates from the TROPOMI Fast Retrieval Scheme for Clouds from Oxygen absorption bands (FRESCO) algorithm (Wang et al., 2008) and the cirrus reflectances derived from the Visible Infrared Imaging Radiometer Suite (VIIRS) on the Suomi National Polar-orbiting Partnership (Suomi NPP) satellite.
-
The maximum solar zenith angle allowed is 75∘. If the pixel does not meet this criterion, it is removed from the processing and a flag is raised.
-
If the pixel over water lies in the sun-glint region (a maximum sun-glint angle of 18∘), it is processed but a sun-glint warning flag is recorded in the level-2 product.
-
If the standard deviation of the surface elevation within the pixel is beyond 1000 m, the pixel is not processed and a flag is raised. If it is beyond 300 m, a warning flag is raised and the pixel is processed.
-
If the surface covered by the pixel comprises both land and water, a warning indicating mixed surface type is raised and the pixel is processed regardless.
-
If the pixel contains snow or ice, the pixel is not processed and a flag is raised.
-
If the TROPOMI level-2 UV aerosol index product reports a value below 0.0, the pixel is not processed and a flag is raised. If the value is less than 1.0, a low UVAI flag is raised.
-
If the reported cloud fraction values from the TROPOMI FRESCO product for the pixel is beyond 0.6, the pixel is not processed and a flag is raised.
-
If the VIIRS average cirrus reflectance for the pixel is beyond 0.4, the pixel is not processed and a flag is raised. If it is beyond 0.01, a warning for possible cirrus clouds is indicated.
-
If the difference between the scene albedo (calculated using a look-up table) from the level-2 UVAI product and the surface albedo from the Tilstra et al. (2017) database at 380 nm is beyond 0.4, the pixel is removed from the processing pool and a flag is raised for possible cloud contamination. If this value is beyond 0.2, a warning flag is raised.
-
The nominal TROPOMI pixels also contain radiances at a subpixel level, which are called small pixel radiances. If the standard deviation of the small pixel radiances is larger than 1×107, the scene is deemed to be non-homogeneous (possibly containing clouds), and it is removed from the processing pool.
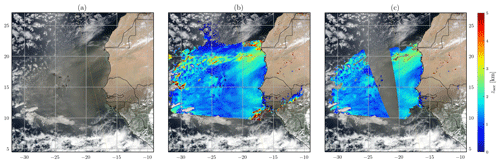
Figure 1(a) A VIIRS corrected reflectance image over the West African coast on 8 June 2018. (b) All successful TROPOMI retrievals are within a certain bounding box. (c) Same as (b) but with all pixels that possibly fall within the sun-glint region or are cloud contaminated removed (using the cloud_warning flag and sun_glint_warning flag from Table 1).
These relevant flags are reported in Table 1 and are available in the level-2 data products; the values for each of these flags can be accessed with bitwise operations for each pixel with the value of each processing quality flag. For cloud filtering, the “cloud_warning” flag is the preferred flag for removing possibly cloudy pixels. This flag is a combination of FRESCO cloud fraction retrievals, VIIRS cirrus reflectance retrievals, and the difference between the surface albedo and the scene albedo at 380 nm. An example of applying the cloud_warning flags to filter out possibly cloudy pixels is provided in Fig. 1.
2.2 CALIOP weighted extinction height
The Cloud-Aerosol lidar with Orthogonal Polarisation (CALIOP) instrument is a part of the payload for the Cloud-Aerosol Lidar and Infrared Pathfinder Satellite Observation (CALIPSO) mission (Winker et al., 2009), which orbits the Earth in a sun-synchronous orbit. The CALIOP instrument has three backscatter receiver channels: two channels for the orthogonal measurement of received backscatter signal at 532 nm and one channel for backscatter at 1064 nm. Lidar profiles from the CALIPSO mission are a good source of data for validating retrieved ALHs from TROPOMI because of their ability to map the vertical structure of the atmosphere. The data from the CALIOP instrument relevant for validating TROPOMI ALH are the level-1 backscatter profiles and the level-2 aerosol extinction profiles, which are used at the same time.
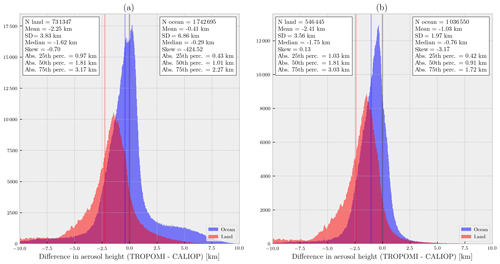
Figure 2Histogram of differences between CALIOP ALHext (Eq. 2) and TROPOMI ALH from co-located data between 1 May 2018 and 28 February 2019. Blue histogram represents TROPOMI pixels over the ocean, whereas the red histogram is for TROPOMI pixels over land. The blue line represents the mean difference between TROPOMI ALH and CALIOP ALHext for TROPOMI pixels over the ocean, whereas the red line represents the same for TROPOMI pixels over land. The black line at 0.0 km difference on the x axis is plotted to aid the reader in their interpretation of this figure. (a) All co-locations except TROPOMI pixels falling in the sun-glint region. TROPOMI pixels with retrieved AOT greater than 5.0 are discarded. For pixels over land, if the Global Ozone Monitoring Experiment-2 (GOME-2) surface albedo values are less than 0.1 or greater than 0.4, they are discarded. Similarly, over the ocean all TROPOMI pixels that have GOME-2 surface albedo values greater than 0.05 are discarded. (b) Same, except only TROPOMI ALH retrievals that are cloud screened using the cloud_warning flag from Table 1 are included.
In this paper, the level-1 total backscatter profiles from the 532 nm channel are used as curtain plots to visualise the vertical structure of the atmosphere. Level-2 aerosol extinction profiles from the 532 nm channel are then used to compute an aerosol weighted extinction height, ALHext, following the definition provided by Eq. (1) in Koffi et al. (2012),
where Zi is the height from sea level in the ith lidar vertical level i (in km), and βext,i is the aerosol extinction coefficient (in km−1) at the same level. The level-2 aerosol extinction profile product from CALIOP only includes atmospheric levels where aerosols are detected. In the case when aerosols are present over clouds, ALHext will be situated to the centre of the aerosol layer, with any possibly undetected aerosol layers below the cloud layer not included in the calculations due to attenuation of the signal beyond the cloud layer. This is an important detail as the TROPOMI ALH algorithm cannot separate cloud and aerosol signals from the measured radiances, and cloud contamination will affect the retrieved product. In this paper, the CALIOP 532 nm channel observations are chosen for analysis as the conclusions from the analysis of the results do not change when the 1064 nm channel observations are used instead. Appendix A explains the co-location technique used in this paper. The CALIOP aerosol product might be cloud contaminated as well, but this is difficult to ascertain. Plotting ALHext over curtain plots of level-1 total backscatter profiles can be used to visually discern possibly cloud-contaminated CALIOP level-2 aerosol data.
3.1 Comparison of TROPOMI ALH and CALIOP ALHext
TROPOMI–CALIOP co-locations between 1 May 2018 to the 28 February 2019 are selected. Two sets of overall comparisons are done between CALIOP ALHext and TROPOMI ALH, one with all co-locations (Fig. 2a) regardless of cloud filtering and the other with a smaller subset of the dataset constrained by the cloud_warning flag from Table 1 (Fig. 2b). The contrast between retrievals over land and ocean is apparent in Fig. 3 (clouds flagged) – a majority of the negative differences with values lower than −2 km occur over land.
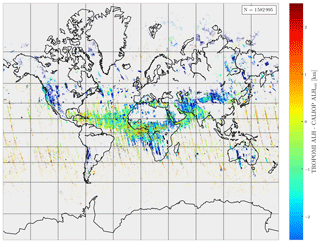
Figure 3A map of cloud-filtered and sun-glint-filtered differences between co-located TROPOMI ALH and CALIOP ALHext considered for Fig. 2b.
From Fig. 2a, what is immediately clear is that the CALIOP ALHext values are higher than the TROPOMI ALH. With an average difference of −2.25 km, median difference of −1.62 km and a standard deviation of 3.83 km, the retrieved ALH from TROPOMI over land is reported to be systematically closer to the surface than CALIOP ALHext than in comparison with retrievals over the ocean, which has a mean difference of −0.41 km, a median difference of −0.29 km and a very high standard deviation of 6.86 km. There are several cases over the ocean where TROPOMI ALH is significantly higher than the CALIOP ALHext, which could be due to cloud contamination. The comparison of the cloud-screened retrievals (Fig. 2b) reveals that the retrieved ALH from TROPOMI over the ocean differs from CALIOP ALHext by −1.03 km on average, a median difference of −0.76 km and a standard deviation of 1.97 km. More than 50 % of the TROPOMI ALH retrievals over the ocean have an absolute difference with ALHext less than 1.0 km. Retrievals over land have a larger difference, with −2.41 km on average and a median of −1.75 km. The results are very skewed over land, with very large negative values dictating the average – this is indicated by the very large standard deviation of 3.56 km. Thus, 50 % of the selected co-locations over land have an absolute difference with ALHext less than approximately 1.8 km.
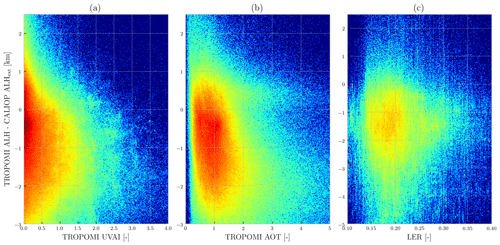
Figure 4Scatter density plots of the difference between TROPOMI ALH and CALIOP ALHext as a function of (a) TROPOMI UVAI, (b) TROPOMI AOT and (c) GOME-2 LER for the oxygen A-band used for the TROPOMI retrievals for cases over land (with a minimum surface albedo of 0.1). The colours represent density of plots. The y axis is optimised for each plot. The data are filtered in the same fashion as in Fig. 2, with data over the ocean and land combined for plots (a) and (b) and data only over land for plot (c).
The distribution of the differences between TROPOMI ALH and CALIOP ALHext as a function of the retrieved UVAI (Fig. 4a) shows that for most cases the UVAI is below 2.0. The spread of the differences in this UVAI regime is large, which reduces as the UVAI increases. The differences seem to be less often positive as the UVAI increases; if compared with the behaviour observed between Fig. 2a and b, where a majority of the positive differences vanish once the data are cloud screened, such a behaviour could be related to clouds. The distribution of the differences as a function of retrieved AOT in Fig. 4b shows that a majority of the co-locations have AOT values between 0 and 2. Finally, the distribution of these differences as a function of the GOME-2 LER values used for the retrievals for cases over land shows that the retrievals tend to have a lower difference as the LER value increases – this could be a consequence of the fact that so few retrievals converge in high LER regimes that, unless the aerosol layer has a significant contribution to the measured top-of-atmosphere radiance in comparison to the surface, the retrievals tend to fail.
Retrieved ALH over land (if successful) can be closer to the surface than where the aerosol layer actually is situated vertically. The TROPOMI ALH product, unlike the CALIOP ALHext, which only considers aerosol signatures in the recorded backscatter profile, is also influenced by the presence of undetected clouds. These are some of the several possible sources of departures between the observations of CALIOP and TROPOMI over the same scene. In the next section, the comparison between TROPOMI ALH and CALIOP ALHext is extended for a few selected scenes.
3.2 Analysis of selected cases
3.2.1 Selected cases
The analysis presented in the previous section alone is insufficient to fully quantify the quality of the retrieved TROPOMI ALHs, due to the manner in which clouds are handled by both aerosol heights; TROPOMI pixels are affected by the presence of undetected clouds, whereas CALIOP aerosol extinction profiles do not consider clouds. Another significant source of departure between TROPOMI and CALIOP is their different sensing principles. Making conclusions on the quality of the current TROPOMI ALH product requires case-by-case studies of selected scenes. In line with this, four cases are selected to represent a very good mix of scenes containing elevated aerosol layers as well as aerosol layers close to the surface, high and low UV aerosol index, clear and cloudy scenes, clouds over and below aerosol layers, multiple aerosol layers, and retrievals over land and the ocean.
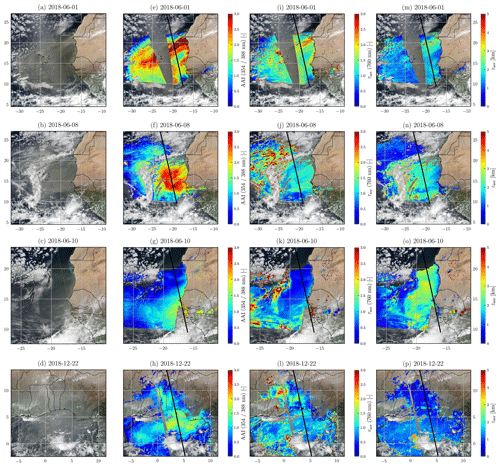
Figure 5First column: corrected reflectance for the four selected cases as measured by the Suomi NPP/VIIRS imager. The yellow line represents the CALIOP ground track. Second column: the TROPOMI level-2 UV aerosol index product. The black line passing through the TROPOMI level-2 retrievals on this plot represents the ground track of the CALIPSO mission. Third column: retrieved AOT from TROPOMI. Fourth column: operational TROPOMI ALH.
The cases selected are Saharan desert dust and biomass burning events: three off the west coast of Sahara (desert dust) in June 2018 and one off the southern Saharan coast (biomass burning) in December 2018. All four cases have very good co-locations between TROPOMI and CALIOP, with the CALIOP ground track over the aerosol plumes (plotted with a yellow line over the VIIRS images in Fig. 5, first column). The operational ALH level-2 algorithm operates on pixels falling within the sun-glint regime; however, they are excluded from the analyses presented in this paper. The retrieved UV aerosol index (UVAI) from the operational level-2 UVAI product gives an idea about the shape of the aerosol plumes in all these cases (Fig. 5, second column). The UVAI is influenced by many factors including the ALH, with lower UVAI values for aerosol layers closer to the surface (discussed further in Appendix B). Cases a and b contain several pixels with UVAI values greater than 3.0, whereas a majority of the TROPOMI pixels in cases c and d have TROPOMI UVAI values between 0.0 and 2.0. A significant majority of successful retrievals in these selected scenes are over a dark surface, owing to the bright surface albedo of the Sahara. The reader is referred to Griffin et al. (2020) for comparison of the TROPOMI ALH retrievals over land for biomass burning aerosol plumes with the same from several other instruments including CALIOP.
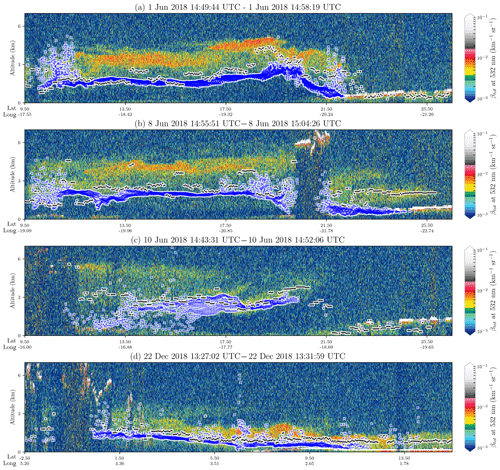
Figure 6CALIOP level-1 backscatter curtain plots for measurements in the 532 nm channel for the four selected cases in Fig. 5. The blue markers (crosses over a white box) represent co-located TROPOMI ALH retrievals within 100 km of each CALIOP profile present in this plot. The black markers (crosses over a white box) represent the CALIOP weighted aerosol heights as computed using Eq. (2). TROPOMI data that are either in the sun-glint region or cloud contaminated are removed (cloud detection is done using the cloud_warning flag from Table 1).
It is important to note that spaceborne lidars, while having the advantage of being able to map more than one vertical layer in the atmosphere, suffer from attenuation of the signal in the presence of strongly backscattering components such as clouds or aerosols with a large optical depth. In the presence of a primary strongly backscattering aerosol layer, the attenuation of the signal may lead to undetected secondary aerosol layers beneath the primary layer. These layers, not apparent in the CALIOP curtain plots of the measured attenuated backscatter profiles, may be detected by the level-2 aerosol extinction profile product from the CALIOP mission, using the formula described in Eq. (2). Some of these discussed situations are observed in the CALIOP curtain plots of the selected cases in Fig. 6, especially for cases a and b where the attenuated signal does not detect possibly lower aerosol or cloud layers and in case d where the attenuation of the signal due to a thick aerosol plume can hide the surface from the received backscatter signal. TROPOMI, on the other hand, will tend to report an ALH between these two layers as it will be influenced by photons scattered back from both layers.
3.2.2 Analysis
The retrieved TROPOMI ALH in Fig. 5 (fourth column) represent successful retrievals for each of the selected cases. Beyond the sun-glint warning, the cloud_warning flag in Table 1 is applied to remove possibly cloud-contaminated data. The retrieved aerosol optical thickness (AOT), which is a part of the state vector, for each of the scenes are plotted over the VIIRS image of the scene in Fig. 5 (third column). The retrieved AOT (τaer) can act as a diagnostic tool to indicate the influence of the surface (over bright surfaces) or the presence of undetected clouds (both over bright and dark surfaces) – in these cases, the retrieved AOT of the scene can be uncharacteristically high with values much greater than 3.0. All retrieved TROPOMI AOT values beyond 5.0 are discarded as the neural network forward models are trained with AOT values less than or equal to 5.0.
A visual inspection of Fig. 5 shows that the retrieved UVAI, AOT and ALH values need not be spatially correlated, as they are separate properties of the observed aerosol plumes – for instance, if the retrieved UVAI and AOT are low (case c), the retrieved ALH need not necessarily be low. An inspection of the retrieved AOT values for cases c (between latitudes 10 and 15∘ and longitude ) and d reveals square structures, both over the ocean and land. These square-shaped spatial artefacts are the surface albedo grids derived from the database provided by Tilstra et al. (2017), which is the current source for surface reflectance in the ALH retrieval algorithm. In case c, the retrieved AOT contains surface information influenced by the assumed albedo in the database. These spatial features are not as apparent in cases a and b (Fig. 5, first and second rows) as a majority of the signal in the measured top-of-atmosphere radiances come from aerosols and the minority from the surface. Another major observation is the lack of retrievals over the desert. This is within expectation, as measurements of the top-of-atmosphere radiances over a cloud-free desert scene tend to contain more photons scattered back from the surface than the aerosol layer. As a result, retrievals over bright scenes are sensitive to the assumed errors in the surface albedo, thereby reducing sensitivity to the assumed ALH (Sanders et al., 2015, Sect. 2, Fig. 2).
While scenes not contaminated with clouds show a smooth spatial distribution of the retrieved ALH, the presence of clouds may or may not add spatial variability in the ALH product. For instance, the presence of low clouds is clear in case b (Fig. 5b) beyond latitude 21.0∘, but the retrieved ALH is spatially homogeneous with values less than 1.0 km. For each of the selected cases, co-located CALIOP profiles in Fig. 6 give additional information about the scene. These TROPOMI–CALIOP co-locations are done via the method discussed in Appendix A. The CALIOP curtain plot for case b reveals the influence of low clouds as well as high clouds on the cloud-screened ALH. An example of cloud-contaminated heterogeneous vertical distribution of TROPOMI ALH in Fig. 6a can be observed between latitudes 9.5 and 11.0∘. The cloud filtering following the cloud_warning flag in Table 1 does not detect these low clouds (for instance above latitude 21.5∘; see Fig. 6a, b). These are manually removed for comparison further on.
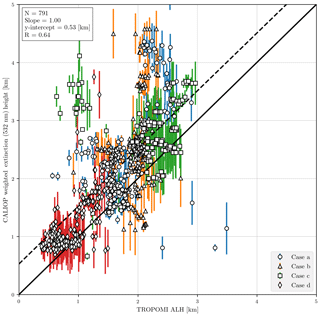
Figure 7Comparison between the CALIOP weighted extinction heights (y axis) calculated using Eq. (2) and plotted in Fig. 6 against averaged TROPOMI ALH (x axis). The blue lines represent the standard deviation of the TROPOMI heights in the averaging pool, and the markers represent the mean TROPOMI ALH for each CALIOP ALHext. The dashed black line marks the fit between CALIOP ALHext and TROPOMI ALH. The solid black line is a neutral line between the x and the y axes. The legend in the bottom right corner describes the different markers used for the different cases. The colours represent the cases – blue for case a, yellow for case b, green for case c and red for case d.
From Fig. 2b, TROPOMI retrievals of ALH over bright surfaces are expected to differ from CALIOP ALHext, wherein the TROPOMI ALH product may report ALH estimates closer to the surface than CALIOP will. This is observed in case d (Fig. 5, bottom row), wherein the CALIOP curtain plot for Fig. 6d indicates that the plume is close to the surface, with a maximum height less than 3 km; TROPOMI ALH for biomass burning aerosol plume that extends from land to the ocean is slightly closer to the surface in the case of land when compared to CALIOP ALHext, whereas over the ocean both height estimates are more or less in agreement.
For cases a and b, retrieved TROPOMI ALH does not seem to coincide with large values of the received backscatter signal in the level-1 data, whereas it does for case c and to a certain extent for case d (over land it tends to be closer to the surface). Parts of the CALIOP curtain plots for cases a, b and c suggest the existence of a possible second layer beneath the layer that is visually obvious or that the desert dust layer extends deeper to the surface and the CALIOP signal is simply too attenuated to detect it.
A direct comparison of the CALIOP ALHext and TROPOMI ALH for these four selected cases is presented in Fig. 7. For this comparison, every cloud-filtered and sun-glint-filtered TROPOMI pixel with ALH information co-located to a specific CALIOP level-2 aerosol extinction profile in Fig. 6 is averaged and a standard deviation is also computed. These averaged TROPOMI ALH values are then compared to the CALIOP ALHext and show that TROPOMI ALH differ from CALIOP ALHext by 0.53 km, with a Pearson correlation coefficient of 0.64 and a slope of 1.0; CALIOP ALHext are systematically higher than TROPOMI ALH (indicated by a y intercept of the fit at 0.53 km). The CALIOP ALHext is also higher than TROPOMI ALH almost consistently in most cases. This could possibly be due to CALIOP possibly underestimating the aerosol layer thickness due to strong attenuation of the lidar signal at the top of the aerosol layer (Rajapakshe et al., 2017), whereas TROPOMI ALH product does not suffer from such attenuation.
This paper discusses the quality of the soon to be released TROPOMI ALH product by comparing it with CALIOP data of co-located measurements of scenes containing aerosols between the two instruments. In order to do so, CALIOP weighted extinction heights from the 532 nm channel were calculated following Eq. (2) and then directly compared to TROPOMI ALH. Further on, four individual cases of Saharan desert dust and biomass burning aerosol events in 2018 were selected for a deeper analysis of the product's quality.
From the analysis presented in this paper, TROPOMI's neural network ALH retrieval algorithm retrieves ALH values that compare well with CALIOP weighted extinction heights in cloud-screened cases following the cloud screening strategy using the TROPOMI ALH level-2 processing quality flags discussed in Table 1. For more than 1 million co-locations between CALIOP and TROPOMI over the ocean, the TROPOMI ALH differs from CALIOP ALHext by approximately −1 km on average and −0.76 km median, with the TROPOMI ALH values being lower than the CALIOP ALHext. Over land, the same values are −2.41 km on average and −1.75 km as the median. To get a better understanding of the differences between TROPOMI and CALIOP retrieved aerosol heights, this paper compared the ALH for selected cases among the more than 1 million co-locations that were better understood. The four selected scenes were chosen around the western part of the Sahara, where a majority of the TROPOMI–CALIOP co-locations were found. For the selected cases, largely over the ocean within a portion of the data over land, the averaged retrieved ALH from TROPOMI differed from CALIOP ALHext by 0.53 km, with CALIOP ALHext being higher than TROPOMI ALH. These numbers are indicative that TROPOMI ALH performs well, especially considering the many simplifications made by the retrieval algorithm in order to optimise the computational speed; future improvements to the forward model may only improve the product further.
There is a clear distinction between TROPOMI ALH retrievals over land and the ocean as photons scattering back from bright surfaces tend to influence ALH estimates closer towards the surface than an elevated aerosol layer. Retrieved ALH over land, if successful, can be closer to the surface if measured signal in the top of atmosphere contains more photons scattered back from the deepest atmospheric layer, which is the surface, in comparison to elevated aerosol layers which are higher up in the atmosphere. This, however, can change depending on the amount of aerosol information available in the spectrum compared to the same from the surface. Any attempt at retrieving ALH over the desert generally fails, with very few exceptions. There are several challenges that will need further development.
The TROPOMI level-2 UVAI product is currently an ingredient in selecting pixels containing aerosols for retrieving ALH. While this choice works quite well for cloud-free scenarios, it does not do a great job when a scene that contains both aerosols and clouds. These cloudy scenes do not seem to be detected by the current cloud-filtering schematic in the level-2 algorithm, and they will require a significant update in deciding whether a pixel is cloudy or not. For cases of scenes with a low aerosol load, square-shaped artefacts resulting from a surface albedo database with a resolution significantly lower than TROPOMI exist. Currently, the GOME-2 surface LER product derived from Tilstra et al. (2017) is used operationally and will eventually need to be updated with a higher-resolution version possibly derived from TROPOMI itself. To that extent, owing to the boost in the computational speed of the radiative transfer calculations, the algorithm can now incorporate more complex aerosol property and profile parameterisations. Such a step will benefit the TROPOMI ALH retrieval accuracy significantly.
Finally, space-based lidar (such as the CALIOP instrument on the CALIPSO mission) is a very good tool to retrieve aerosol vertical information to validate the TROPOMI ALH product. While the CALIOP level-1 backscatter profiles may be attenuated in cases of very strong signals from the top of the aerosol layer, the weighted extinction heights in conjunction with the backscatter profiles are sufficient for validation activities. These CALIOP profiles will be very important in assessing the impact of future development activities of the TROPOMI ALH product.
The co-location between TROPOMI and CALIOP ground pixels is done in the following manner. First, the geographic coordinates of CALIOP level-1 backscatter profiles and level-2 aerosol extinction profiles are converted into the Cartesian coordinate system. These CALIOP coordinates are fed into a k-dimensional tree, which is a fast algorithm developed by Maneewongvatana and Mount (1999) to quickly locate the nearest neighbour of a point a (TROPOMI ground pixel) to a k-dimensional tree of points (CALIOP ground pixels). The scipy.spatial.KDtree module (Maneewongvatana and Mount, 1999) in Python 3 is used to create the k-dimensional tree of the ground coordinates of CALIOP profiles (separate for level-1 and level-2 data). Second, all TROPOMI ground pixel coordinates are converted to Cartesian coordinates. For each of these TROPOMI pixels, the distance to the nearest CALIOP profile is queried using the scipy.spatial.KDtree.query function. This creates a list of TROPOMI pixels, their nearest CALIOP profile and a distance in metres. Finally, only co-locations with a maximum distance of 100 km and a maximum time difference of 5 h are selected. A map of all 2 474 042 co-locations (in Fig. A1) shows that most of the co-locations are close to the continent of Africa. After filtering out all co-locations in the TROPOMI sun-glint region, all retrieved AOTs greater than 5.0 (as the neural network is trained for all AOT less than 5.0), and ocean pixels with a surface albedo greater than 0.05 and land pixels with a surface albedo less than 0.1 and greater than 0.4, there are in total 731 347 TROPOMI pixels entirely over land and 1 742 695 pixels entirely over water (see Fig. 2a). After cloud screening using the cloud_warning flag in Table 1, a total of 546 445 pixels over land and 1 036 550 pixels over the ocean remain (see Fig. 2b).
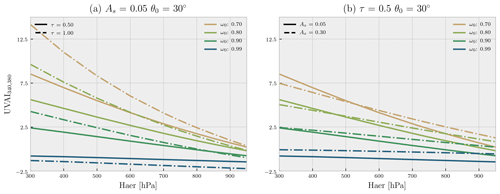
Figure A2Sensitivity analysis of UV aerosol index to show the influence of different aerosol properties on the UVAI. The aerosols in these analyses have a Henyey–Greenstein scattering phase function with an asymmetry factor of 0.7; an Ångström exponent of 1.0; viewing zenith angle of 0∘; and the solar azimuth angle and the viewing azimuth angle of 0 and 60∘, respectively; the surface pressure is 1013 hPa and, for this specific case, the solar zenith angle θ0 is 30∘. The y axis is the UVAI for 340 and 380 nm, whereas the x axis is the height of the geometric centroid of the aerosol layer (in hPa, Haer). The legends in each of the plots describe the different configurations chosen for these sensitivity analyses. Panel (a) looks into the sensitivity of UVAI with a fixed surface albedo of 0.05, and panel (b) does the same for a fixed AOT of 0.5.
It is well documented that the UVAI depends on ALH (Herman et al., 1997; Torres et al., 1998; de Graaf, 2005; Sun et al., 2018). Absorbing aerosols mainly interact with molecular scattered radiation beneath the aerosol layer. The higher the layer, the more Rayleigh scattering underneath is shielded, leading to a high UVAI value (Fig. A2a). This altitude dependence increases with aerosol single scattering albedo and aerosol loading (i.e. AOT), whereas it becomes weaker over brighter surfaces where the importance of molecular scattering reduces significantly (Fig. A2b). On the other hand, little altitude dependence is found for non-absorbing aerosols (i.e. SSA =0.99, single scattering albedo). The conclusions from this synthetic experiment are reproduced with real TROPOMI data in a separate article, where for retrieved ALH for pixels with a UVAI greater than 1 for measurements from TROPOMI showed an increase in the correlation as well as the slope between ALH and UVAI for an increase in MODIS AOT values for the same scenes. That article has been submitted to Atmospheric Chemistry and Physics and awaits review (Sun et al., 2020).
All TROPOMI data are publicly available. For the latest information on accessing these data, please check https://scihub.copernicus.eu/dhus/#/home (ESA, 2020). CALIOP data were acquired via iCARE (http://www.icare.univ-lille1.fr/, AERIS, 2020).
The research analysis was conducted by SN under the supervision of MdG, JPV and PFL. The operational level-2 algorithm on which the research is based was developed together by JdH, MS, MtL and SN under the supervision of JPV and PFL. JS provided valuable insights into the role of UV absorbing index and the aerosol layer height.
The authors declare that they have no conflict of interest.
TROPOMI on Sentinel-5 Precursor: first year in operation (AMT/ACP inter-journal SI) SI statement: This article is part of the special issue “TROPOMI on Sentinel-5 Precursor: first year in operation (AMT/ACP inter-journal SI)”. It is not associated with a conference.
This publication contains modified Copernicus Sentinel data. This research is partly funded by the European Space Agency (ESA) within the EU Copernicus programme. We acknowledge the use of VIIRS imagery from the NASA Worldview application (https://worldview.earthdata.nasa.gov/, last access: 8 June 2020), part of the NASA Earth Observing System Data and Information System (EOSDIS).
This research has been supported by the ESA (TROPOMI Science Contract).
This paper was edited by Jhoon Kim and reviewed by two anonymous referees.
AERIS: ICARE Data Services Center, available at: http://www.icare.univ-lille1.fr/calipso/products, last acces: 9 June 2020. a
Barkley, A. E., Prospero, J. M., Mahowald, N., Hamilton, D. S., Popendorf, K. J., Oehlert, A. M., Pourmand, A., Gatineau, A., Panechou-Pulcherie, K., Blackwelder, P., and Gaston, C. J.: African biomass burning is a substantial source of phosphorus deposition to the Amazon, Tropical Atlantic Ocean, and Southern Ocean, P. Natl. Acad. Sci. USA, 116, 16216–16221, https://doi.org/10.1073/pnas.1906091116, 2019. a
Chimot, J., Veefkind, J. P., Vlemmix, T., de Haan, J. F., Amiridis, V., Proestakis, E., Marinou, E., and Levelt, P. F.: An exploratory study on the aerosol height retrieval from OMI measurements of the 477 nm O2 − O2 spectral band using a neural network approach, Atmos. Meas. Tech., 10, 783–809, https://doi.org/10.5194/amt-10-783-2017, 2017. a
Chimot, J., Veefkind, J. P., Vlemmix, T., and Levelt, P. F.: Spatial distribution analysis of the OMI aerosol layer height: a pixel-by-pixel comparison to CALIOP observations, Atmos. Meas. Tech., 11, 2257–2277, https://doi.org/10.5194/amt-11-2257-2018, 2018. a
Choi, W., Lee, H., Kim, J., Ryu, J. Y., Park, S. S., Park, J., and Kang, H.: Effects of spatiotemporal O4 column densities and temperature-dependent O4 absorption cross-section on an aerosol effective height retrieval algorithm using the O4 air mass factor from the ozone monitoring instrument, Remote Sens. Environ., 229, 223–233, https://doi.org/10.1016/j.rse.2019.05.001, 2019. a
Davis, A. B., Kalashnikova, O. V., and Diner, D. J.: Aerosol Layer Height over Water from O2 A-Band: Mono-Angle Hyperspectral and/or Bi-Spectral Multi-Angle Observations, https://doi.org/10.20944/preprints201710.0055.v1, 2017. a
de Graaf, M.: Absorbing Aerosol Index: Sensitivity analysis, application to GOME and comparison with TOMS, J. Geophys. Res., 110, 110, https://doi.org/10.1029/2004JD005178, 2005. a, b
de Haan, J. F., Bosma, P. B., and Hovenier, J. W.: The adding method for multiple scattering calculations of polarized light, Astron. Astrophys., 183, 371–391, 1987. a
de Rooij, W. A. and van der Stap, C. C. A. H.: Expansion of Mie scattering matrices in generalized spherical functions, Astron. Astrophys., 131, 237–248, 1984. a
Dubovik, O., Holben, B., Eck, T. F., Smirnov, A., Kaufman, Y. J., King, M. D., Tanré, D., and Slutsker, I.: Variability of Absorption and Optical Properties of Key Aerosol Types Observed in Worldwide Locations, J. Atmos. Sci., 59, 590–608, https://doi.org/10.1175/1520-0469(2002)059<0590:VOAAOP>2.0.CO;2, 2002. a
European Space Agency (ESA): Copernicus Open Access Hub, available at: https://scihub.copernicus.eu/dhus/#/home, last access: 9 June 2020. a
Griffin, D., Sioris, C., Chen, J., Dickson, N., Kovachik, A., de Graaf, M., Nanda, S., Veefkind, P., Dammers, E., McLinden, C. A., Makar, P., and Akingunola, A.: The 2018 fire season in North America as seen by TROPOMI: aerosol layer height intercomparisons and evaluation of model-derived plume heights, Atmos. Meas. Tech., 13, 1427–1445, https://doi.org/10.5194/amt-13-1427-2020, 2020. a
Henyey, L. C. and Greenstein, J. L.: Diffuse radiation in the Galaxy, Astrophys. J., 93, 70–83, https://doi.org/10.1086/144246, 1941. a
Herman, J. R., Bhartia, P. K., Torres, O., Hsu, C., Seftor, C., and Celarier, E.: Global distribution of UV-absorbing aerosols from Nimbus 7/TOMS data, J. Geophys. Res.-Atmos., 102, 16911–16922, https://doi.org/10.1029/96JD03680, 1997. a
Holben, B. N., Eck, T. F., Slutsker, I., Tanré, D., Buis, J. P., Setzer, A., Vermote, E., Reagan, J. A., Kaufman, Y. J., Nakajima, T., Lavenu, F., Jankowiak, I., and Smirnov, A.: AERONET–A Federated Instrument Network and Data Archive for Aerosol Characterization, Remote Sens. Environ., 66, 1–16, https://doi.org/10.1016/S0034-4257(98)00031-5, 1998. a
Ingmann, P., Veihelmann, B., Langen, J., Lamarre, D., Stark, H., and Courrèges-Lacoste, G. B.: Requirements for the GMES Atmosphere Service and ESA's implementation concept: Sentinels-4/-5 and -5p, Remote Sens. Environ., 120, 58–69, https://doi.org/10.1016/j.rse.2012.01.023, 2012. a
IPCC: Clouds and Aerosols, in: Climate Change 2013 – The Physical Science Basis, 571–658, Cambridge University Press, Cambridge, https://doi.org/10.1017/CBO9781107415324.016, 2014. a
Kim, J., Jeong, U., Ahn, M.-H., Kim, J. H., Park, R. J., Lee, H., Song, C. H., Choi, Y.-S., Lee, K.-H., Yoo, J.-M., Jeong, M.-J., Park, S. K., Lee, K.-M., Song, C.-K., Kim, S.-W., Kim, Y. J., Kim, S.-W., Kim, M., Go, S., Liu, X., Chance, K., Chan Miller, C., Al-Saadi, J., Veihelmann, B., Bhartia, P. K., Torres, O., Abad, G. G., Haffner, D. P., Ko, D. H., Lee, S. H., Woo, J.-H., Chong, H., Park, S. S., Nicks, D., Choi, W. J., Moon, K.-J., Cho, A., Yoon, J., Kim, S.-K., Hong, H., Lee, K., Lee, H., Lee, S., Choi, M., Veefkind, P., Levelt, P. F., Edwards, D. P., Kang, M., Eo, M., Bak, J., Baek, K., Kwon, H.-A., Yang, J., Park, J., Han, K. M., Kim, B.-R., Shin, H.-W., Choi, H., Lee, E., Chong, J., Cha, Y., Koo, J.-H., Irie, H., Hayashida, S., Kasai, Y., Kanaya, Y., Liu, C., Lin, J., Crawford, J. H., Carmichael, G. R., Newchurch, M. J., Lefer, B. L., Herman, J. R., Swap, R. J., Lau, A. K. H., Kurosu, T. P., Jaross, G., Ahlers, B., Dobber, M., McElroy, C. T., and Choi, Y.: New Era of Air Quality Monitoring from Space: Geostationary Environment Monitoring Spectrometer (GEMS), B. Am. Meteorol. Soc., 101, E1–E22, https://doi.org/10.1175/BAMS-D-18-0013.1, 2019. a
Kim, M., Kim, J., Torres, O., Ahn, C., Kim, W., Jeong, U., Go, S., Liu, X., Moon, K. J., and Kim, D.-R.: Optimal Estimation-Based Algorithm to Retrieve Aerosol Optical Properties for GEMS Measurements over Asia, Remote Sens., 10, 162, https://doi.org/10.3390/rs10020162, 2018. a
Koffi, B., Schulz, M., Bréon, F.-M., Griesfeller, J., Winker, D., Balkanski, Y., Bauer, S., Berntsen, T., Chin, M., Collins, W. D., Dentener, F., Diehl, T., Easter, R., Ghan, S., Ginoux, P., Gong, S., Horowitz, L. W., Iversen, T., Kirkevåg, A., Koch, D., Krol, M., Myhre, G., Stier, P., and Takemura, T.: Application of the CALIOP layer product to evaluate the vertical distribution of aerosols estimated by global models: AeroCom phase I results, J. Geophys. Res.-Atmos., 117, D10, https://doi.org/10.1029/2011JD016858, 2012. a
Li, J., Carlson, B. E., and Lacis, A. A.: A study on the temporal and spatial variability of absorbing aerosols using Total Ozone Mapping Spectrometer and Ozone Monitoring Instrument Aerosol Index data, J. Geophys. Res.-Atmos., 114, D9, https://doi.org/10.1029/2008JD011278, 2009. a
Maneewongvatana, S. and Mount, D. M.: Analysis of approximate nearest neighbor searching with clustered point sets, arXiv [preprint], arXiv:9901013), 26 January 1999. a, b
Nanda, S., de Graaf, M., Sneep, M., de Haan, J. F., Stammes, P., Sanders, A. F. J., Tuinder, O., Veefkind, J. P., and Levelt, P. F.: Error sources in the retrieval of aerosol information over bright surfaces from satellite measurements in the oxygen A band, Atmos. Meas. Tech., 11, 161–175, https://doi.org/10.5194/amt-11-161-2018, 2018a. a, b, c
Nanda, S., Veefkind, J. P., de Graaf, M., Sneep, M., Stammes, P., de Haan, J. F., Sanders, A. F. J., Apituley, A., Tuinder, O., and Levelt, P. F.: A weighted least squares approach to retrieve aerosol layer height over bright surfaces applied to GOME-2 measurements of the oxygen A band for forest fire cases over Europe, Atmos. Meas. Tech., 11, 3263–3280, https://doi.org/10.5194/amt-11-3263-2018, 2018b. a
Nanda, S., de Graaf, M., Veefkind, J. P., ter Linden, M., Sneep, M., de Haan, J., and Levelt, P. F.: A neural network radiative transfer model approach applied to the Tropospheric Monitoring Instrument aerosol height algorithm, Atmos. Meas. Tech., 12, 6619–6634, https://doi.org/10.5194/amt-12-6619-2019, 2019. a, b
Nelson, D. L., Garay, M. J., Kahn, R. A., and Dunst, B. A.: Stereoscopic Height and Wind Retrievals for Aerosol Plumes with the MISR INteractive eXplorer (MINX), Remote Sens., 5, 4593–4628, https://doi.org/10.3390/rs5094593, 2013. a
Park, S. S., Kim, J., Lee, H., Torres, O., Lee, K.-M., and Lee, S. D.: Utilization of O4 slant column density to derive aerosol layer height from a space-borne UV–visible hyperspectral sensor: sensitivity and case study, Atmos. Chem. Phys., 16, 1987–2006, https://doi.org/10.5194/acp-16-1987-2016, 2016. a
Rajapakshe, C., Zhang, Z., Yorks, J. E., Yu, H., Tan, Q., Meyer, K., Platnick, S., and Winker, D. M.: Seasonally transported aerosol layers over southeast Atlantic are closer to underlying clouds than previously reported, Geophys. Res. Lett., 44, 5818–5825, https://doi.org/10.1002/2017GL073559, 2017. a
Rodgers, C. D.: Inverse methods for atmospheric sounding: theory and practice, vol. 2, World Scientific, 2000. a
Sanders, A. F. J. and de Haan, J. F.: TROPOMI ATBD of the Aerosol Layer Height product, available at: http://www.tropomi.eu/sites/default/files/files/S5P-KNMI-L2-0006-RP-TROPOMI_ATBD_Aerosol_Height-v1p0p0-20160129.pdf (last access: 8 June 2020), 2016. a
Sanders, A. F. J., de Haan, J. F., Sneep, M., Apituley, A., Stammes, P., Vieitez, M. O., Tilstra, L. G., Tuinder, O. N. E., Koning, C. E., and Veefkind, J. P.: Evaluation of the operational Aerosol Layer Height retrieval algorithm for Sentinel-5 Precursor: application to O2 A band observations from GOME-2A, Atmos. Meas. Tech., 8, 4947–4977, https://doi.org/10.5194/amt-8-4947-2015, 2015. a, b, c
Sun, J., Veefkind, J. P., van Velthoven, P., and Levelt, P. F.: Quantifying the single-scattering albedo for the January 2017 Chile wildfires from simulations of the OMI absorbing aerosol index, Atmos. Meas. Tech., 11, 5261–5277, https://doi.org/10.5194/amt-11-5261-2018, 2018. a, b
Sun, J., Veefkind, J. P., van Velthoven, P., Tilstra, L. G., Chimot, J., Nanda, S., and Levelt, P. F.: Defining aerosol layer height for UVAI interpretation using aerosol vertical distributions characterized by MERRA-2, Atmos. Chem. Phys. Discuss., https://doi.org/10.5194/acp-2020-39, in review, 2020 a
Tilstra, L. G., Tuinder, O. N. E., Wang, P., and Stammes, P.: Surface reflectivity climatologies from UV to NIR determined from Earth observations by GOME-2 and SCIAMACHY: GOME-2 and SCIAMACHY surface reflectivity climatologies, J. Geophys. Res.-Atmos., 7, 4048–4111, https://doi.org/10.1002/2016JD025940, 2017. a, b, c, d
Torres, O., Bhartia, P. K., Herman, J. R., Ahmad, Z., and Gleason, J.: Derivation of aerosol properties from satellite measurements of backscattered ultraviolet radiation: Theoretical basis, J. Geophys. Res.-Atmos., 103, 17099–17110, https://doi.org/10.1029/98JD00900, 1998. a, b
Twomey, S.: Pollution and the planetary albedo, Atmos. Environ., 8, 1251–1256, https://doi.org/10.1016/0004-6981(74)90004-3, 1974. a
Vasilkov, A., Joiner, J., and Spurr, R.: Note on rotational-Raman scattering in the O2 A- and B-bands, Atmos. Meas. Tech., 6, 981–990, https://doi.org/10.5194/amt-6-981-2013, 2013. a
Veefkind, J. P., Aben, I., McMullan, K., Förster, H., de Vries, J., Otter, G., Claas, J., Eskes, H. J., de Haan, J. F., Kleipool, Q., van Weele, M., Hasekamp, O., Hoogeveen, R., Landgraf, J., Snel, R., Tol, P., Ingmann, P., Voors, R., Kruizinga, B., Vink, R., Visser, H., and Levelt, P. F.: TROPOMI on the ESA Sentinel-5 Precursor: A GMES mission for global observations of the atmospheric composition for climate, air quality and ozone layer applications, Remote Sens. Environ., 120, 70–83, https://doi.org/10.1016/j.rse.2011.09.027, 2012. a
Wagner, T., Beirle, S., Deutschmann, T., and Penning de Vries, M.: A sensitivity analysis of Ring effect to aerosol properties and comparison to satellite observations, Atmos. Meas. Tech., 3, 1723–1751, https://doi.org/10.5194/amt-3-1723-2010, 2010. a
Wang, P., Stammes, P., van der A, R., Pinardi, G., and van Roozendael, M.: FRESCO+: an improved O2 A-band cloud retrieval algorithm for tropospheric trace gas retrievals, Atmos. Chem. Phys., 8, 6565–6576, https://doi.org/10.5194/acp-8-6565-2008, 2008. a
Winker, D. M., Vaughan, M. A., Omar, A., Hu, Y., Powell, K. A., Liu, Z., Hunt, W. H., and Young, S. A.: Overview of the CALIPSO Mission and CALIOP Data Processing Algorithms, J. Atmos. Ocean. Tech., 26, 2310–2323, https://doi.org/10.1175/2009JTECHA1281.1, 2009. a, b
Xu, X., Wang, J., Wang, Y., Zeng, J., Torres, O., Yang, Y., Marshak, A., Reid, J., and Miller, S.: Passive remote sensing of altitude and optical depth of dust plumes using the oxygen A and B bands: First results from EPIC/DSCOVR at Lagrange-1 point, Geophys. Res. Lett., 44, 2017GL073939, https://doi.org/10.1002/2017GL073939, 2017. a, b
Xu, X., Wang, J., Wang, Y., Zeng, J., Torres, O., Reid, J. S., Miller, S. D., Martins, J. V., and Remer, L. A.: Detecting layer height of smoke aerosols over vegetated land and water surfaces via oxygen absorption bands: hourly results from EPIC/DSCOVR in deep space, Atmos. Meas. Tech., 12, 3269–3288, https://doi.org/10.5194/amt-12-3269-2019, 2019. a, b
Yu, H., Chin, M., Yuan, T., Bian, H., Remer, L. A., Prospero, J. M., Omar, A., Winker, D., Yang, Y., Zhang, Y., Zhang, Z., and Zhao, C.: The fertilizing role of African dust in the Amazon rainforest: A first multiyear assessment based on data from Cloud-Aerosol Lidar and Infrared Pathfinder Satellite Observations, Geophys. Res. Lett., 42, 1984–1991, https://doi.org/10.1002/2015GL063040, 2015. a
Zoogman, P., Liu, X., Suleiman, R. M., Pennington, W. F., Flittner, D. E., Al-Saadi, J. A., Hilton, B. B., Nicks, D. K., Newchurch, M. J., Carr, J. L., Janz, S. J., Andraschko, M. R., Arola, A., Baker, B. D., Canova, B. P., Chan Miller, C., Cohen, R. C., Davis, J. E., Dussault, M. E., Edwards, D. P., Fishman, J., Ghulam, A., González Abad, G., Grutter, M., Herman, J. R., Houck, J., Jacob, D. J., Joiner, J., Kerridge, B. J., Kim, J., Krotkov, N. A., Lamsal, L., Li, C., Lindfors, A., Martin, R. V., McElroy, C. T., McLinden, C., Natraj, V., Neil, D. O., Nowlan, C. R., O'Sullivan, E. J., Palmer, P. I., Pierce, R. B., Pippin, M. R., Saiz-Lopez, A., Spurr, R. J. D., Szykman, J. J., Torres, O., Veefkind, J. P., Veihelmann, B., Wang, H., Wang, J., and Chance, K.: Tropospheric emissions: Monitoring of pollution (TEMPO), J. Quant. Spectrosc. Ra., 186, 17–39, https://doi.org/10.1016/j.jqsrt.2016.05.008, 2017. a, b