the Creative Commons Attribution 4.0 License.
the Creative Commons Attribution 4.0 License.
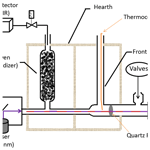
Highly time-resolved characterization of carbonaceous aerosols using a two-wavelength Sunset thermal–optical carbon analyzer
Mengying Bao
Fang Cao
Yu-Chi Lin
Yuhang Wang
Xiaoyan Liu
Wenqi Zhang
Meiyi Fan
Feng Xie
Robert Cary
Joshua Dixon
Lihua Zhou
Carbonaceous aerosols have great influence on the air quality, human health and climate change. Except for organic carbon (OC) and elemental carbon (EC), brown carbon (BrC) mainly originates from biomass burning as a group of OC, with strong absorption from the visible to near-ultraviolet wavelengths, and makes a considerable contribution to global warming. Large numbers of studies have reported long-term observation of OC and EC concentrations throughout the world, but studies of BrC based on long-term observations are rather limited. In this study, we established a two-wavelength method (658 and 405 nm) applied in the Sunset thermal–optical carbon analyzer. Based on a 1-year observation, we firstly investigated the characteristics, meteorological impact and transport process of OC and EC. Since BrC absorbs light at 405 nm more effectively than 658 nm, we defined the enhanced concentrations (dEC = EC405 nm − EC658 nm) and gave the possibility of providing an indicator of BrC. The receptor model and MODIS fire information were used to identify the presence of BrC aerosols. Our results showed that the carbonaceous aerosol concentrations were the highest in winter and lowest in summer. Traffic emission was an important source of carbonaceous aerosols in Nanjing. Receptor model results showed that strong local emissions were found for OC and EC; however, dEC was significantly affected by regional or long-range transport. The and ratios showed similar diurnal patterns, and the increased when the ratios increased, indicating strong secondary sources or biomass burning contributions to dEC. A total of two biomass burning events both in summer and winter were analyzed, and the results showed that the dEC concentrations were obviously higher on biomass burning days; however, no similar levels of the OC and EC concentrations were found both in biomass burning days and normal days in summer, suggesting that biomass burning emissions made a great contribution to dEC, and the sources of OC and EC were more complicated. Large number of open fire counts from the northwestern and southwestern areas of the study site were observed in winter and significantly contributed to OC, EC and dEC. In addition, the nearby Yangtze River Delta area was one of the main potential source areas of dEC, suggesting that anthropogenic emissions could also be important sources of dEC. The results proved that dEC can be an indicator of BrC on biomass burning days. Our modified two-wavelength instrument provided more information than the traditional single-wavelength thermal–optical carbon analyzer and gave a new idea about the measurement of BrC; the application of dEC data needs to be further investigated.
- Article
(9444 KB) - Full-text XML
-
Supplement
(575 KB) - BibTeX
- EndNote
Carbonaceous aerosols, including organic carbon (OC) and elemental carbon (EC), which have significant influence on the global radiative transfer, human health and atmospheric visibility, have been the focus of research in the atmospheric environment field for many years (Lelieveld et al., 2015; Wu and Yu, 2016; Wang et al., 2018; Zhang et al., 2017, 2019; Liu et al., 2019). EC mainly originates from fossil fuel and biomass combustion and is estimated to be the second-largest warming factor behind CO2 contributing to climate change (Liu et al., 2015; Zhang and Kang, 2019; Cao and Zhang, 2015). OC originates both from primary emissions and gas-to-particle conversion as secondary organic carbon (SOC) and can scatter the solar radiation, which causes negative forcing globally (Zhou et al., 2014; Huang et al., 2014).
In recent decades, brown carbon (BrC), as a kind of light-absorbing organic carbon which can absorb light especially from near-UV to visible wavelength, has caused global concern due to its positive climate effect (Andreae and Gelencsér, 2006; Zhang et al., 2020). BrC is mainly emitted from anthropogenic and biogenic emissions (Zhang et al., 2011). Previous studies have proved that biomass burning and biofuel combustion are the most important sources of primary BrC (Saleh et al., 2014; Wu et al., 2020; Lei et al., 2018). Recent researches reported that, in developing countries such as China and India, the contribution of fossil fuel combustion to BrC cannot be ignored (Satish et al., 2017; Yan et al., 2017; Kirillova et al., 2014). Secondary BrC is mainly produced by heterogeneous photo-oxidation reactions or aqueous reactions of anthropogenic and biogenic precursors (Zhang et al., 2011, 2020; Li et al., 2020). However, due to the lack of understanding of BrC at the molecular level and in situ BrC data, there are still large uncertainties in the estimates of the distribution and the magnitude of the BrC climate effect in both remote sensing and modeling (Arola et al., 2011; Feng et al., 2013).
The thermal–optical analysis (TOA) method is one of the most widely used quantitative methods for OC and EC making use of the difference between the thermal–optical properties of OC and EC (Birch and Cary, 1996; Chow et al., 2004). OC and EC will be volatilized at different heating protocols. The reflectance or transmittance of one laser source (near-infrared wavelength) through the sample filter is continuously monitored, and the return of the reflectance or transmittance to its initial value on the thermograph is taken as the split point between OC and EC. In this way, the formation of pyrolyzed carbon, which can also absorb the light and make the sample darker, is corrected. This method has been widely used in studies employing the National Institute for Occupational Safety and Health (NIOSH) protocol or IMPROVE_A protocol (Ji et al., 2016; Chow et al., 2007). However, the thermal–optical approach assumed that EC is the only light-absorbing species, and the presence of BrC, which is part of OC but also a light-absorbing component, shifts this separation towards EC, resulting in overestimated EC values and underestimated OC values (Chen et al., 2015; Birch and Cary, 1996).
Sandradewi et al. (2008) pointed out that light absorption measurements at different wavelengths by the Aethalometer can be used to quantify the contributions of wood combustion and traffic emissions to aerosols since wood smoke contains organic compounds which enhance the light absorption in the ultraviolet wavelength. But traffic emissions produce more black carbon (BC), which dominates the light absorption in the near-infrared wavelength. They used Aethalometer data measured at 470 and 950 nm to quantify the BC distinction between wood burning and traffic emission. With a similar principle, Wang et al. (2011) used a two-wavelength Aethalometer (370 and 880 nm) to identify the presence of residential wood combustion (RWC) particles which were closely associated with BrC. Organic components of wood smoke particles absorb light at 370 nm – more effectively than 880 nm in two-wavelength Aethalometer measurements. They believed that the enhanced absorption (Δ-C = BC370 nm − BC880 nm) can serve as an indicator of RWC particles. This method was further used by Wang et al. (2012a, b). Chen et al. (2015) used a modified seven-wavelength thermal–optical transmittance/thermal–optical reflectance (TOT/TOR) instrument (Thermal Spectral Analysis – TSA) allowing the determination of the OC–EC split at different wavelengths and light absorption measurements to be made with wavelength-specific loading corrections, providing additional information including the optical properties of BC and BrC from the infrared (IR) to the ultraviolet (UV) parts of the solar spectrum and their contributions. Massabò et al. (2016) further corrected the split point using the multi-wavelength absorbance analyzer (MWAA), which provides the aerosol absorbance values at five wavelengths from IR to UV, together with a Sunset analyzer to achieve the BrC concentration. With a set of samples collected during wintertime in the Ligurian Apennines in Italy, clear correlations were found between the BrC and levoglucosan mass concentration. A further step in BrC quantification, taking the use of TSA, was reported by Chow et al. (2018), further proving that the use of seven wavelengths in thermal–optical carbon analysis allows contributions from biomass burning and secondary organic aerosols to be estimated. Their results clearly demonstrated the role of BrC in the thermal–optical analysis. However, these techniques focus on the light absorption measurement of BrC and are still reported in a limited way in previous researches, though they provide quartz fiber filter samples that are currently being characterized for OC and EC by thermal–optical analysis. These abovementioned methods still cannot achieve the observation of long-term, real-time BrC mass concentrations.
Since the establishment of the TOT method by Sunset Laboratory, the Sunset instrument, as part of the Chemical Speciation Network (CSN), with over 100 monitors across the United States over 15 years, offering long-term measurement of OC and EC concentrations, has been widely used in the United States and throughout the world, providing important in situ data of OC and EC (US EPA, 2019; Birch and Cary, 1996). This instrument had been designed with a tuned diode laser (red – 660 nm) to correct the formation of pyrolyzed carbon. In this study, we modified the Sunset instrument to a two-wavelength (658 and 405 nm) Sunset carbon analyzer by adding one more violet diode laser at λ=405 nm. The violet diode laser together with the red diode laser focus through the sample chamber, and then the laser beam passes through the filter to correct for the pyrolysis-induced error. Previous work reported by Chen et al. (2015), as mentioned above, was integrating the optical instrument like the Aethalometer to the traditional analyzer; in this way, they provided the light absorption contributions of BC and BrC. The enhanced carbon analyzer provided new insight into more accurate OC and EC measurements. Their work was conducted in offline mode; based on their work, our instrument can obtain the real-time OC and EC mass concentrations at both 658 and 405 nm. BrC particles absorb light at 405 nm, more effectively than 658 nm in the two-wavelength Sunset carbon measurements. We define dEC = EC405 nm − EC658 nm and hope it can be an indicator of BrC aerosols so that we can divide real-time BrC mass concentration measurement from the two-wavelength measurement.
Nanjing, as one of the largest cities in the Yangzi River Delta region, represents a heavy industry area with a dense population. In addition, due to its topography, Nanjing is very sensitive to regional transport of air masses from its surrounding areas. OC, EC and dEC were measured from June 2015 to July 2016 at Nanjing University of Information Science and Technology (NUIST). Based on the abundant data, together with MODIS fire information, we can analyze the temporal variation, transport processes and sources of carbonaceous aerosols in northern Nanjing and evaluate the biomass burning impact on dEC, which can be the scientific basis of pollution control policy.
2.1 Study site
In this study, the sampling site is located at Nanjing University of Information Science and Technology (NUIST) in the northern suburb of Nanjing (32∘207′ N, 118∘717′ E). The study site is surrounded by housing and industrial areas. Many chemical enterprises, for example, Yangzi Petrochemical, Nanjing Chemical Industry and Nanjing Iron and Steel Group are located at the northeast of the study region, which produces exhaust fumes with large amounts of aerosol particles. The study site is adjacent to a heavily trafficked road (Ningliu road) located near the site and approximately 600 m to the east. Therefore, this region has intense human activities, industrial emissions and heavy traffic flow.
2.2 A two-wavelength TOT measurement
Hourly concentrations of OC and EC in PM2.5 were sampled and measured by a semi-continuous carbon analyzer (Model-4; Sunset Laboratory, USA). Air samples were collected continuously with a sample flow of ∼8 L min−1 through a PM2.5 cyclone. The collection time was set at 45 min for each cycle. The airstream passed through a parallel plate organic denuder to reduce the effect of volatile organic compounds and, finally, deposited on a quartz filter with a diameter of ∼17 mm.
After a sample was collected, OC and EC were determined using the TOT method by applying a slightly modified NIOSH 5040 protocol. The details of the heating setup are shown in Table S1 in the Supplement. Figure 1 shows the structure and operational principle of the instrument. Briefly, it consists of the following two-stages: the oven is first purged with helium, and the oven temperature increased in a stepped ramp to 840 ∘C; OC is volatilized in this stage. Then the oven temperature is kept at 840 ∘C for a while and goes down to 550 ∘C. In the second stage, EC is volatilized in a second temperature ramp to 850 ∘C, while purging the oven with a mixture containing 2 % oxygen and 98 % helium. The pyrolysis products are converted to carbon dioxide (CO2), which is quantified using a self-contained nondispersive infrared (NDIR) system.
Also, in this study, we used a two-diode laser-equipped (658 and 405 nm) Sunset analyzer; thus, mass concentrations of OC and EC at different wavelengths can be measured with the two-laser system. The split point between OC and EC is detected automatically by the RTCalc731 software provided by Sunset Laboratory. The principle is the same as for the traditional Sunset carbon analyzer (Birch and Cary, 1996). An example thermogram of sample analysis using the two-wavelength Sunset semi-continuous carbon analyzer is shown in Fig. 2. During the sample analysis, the laser beam at 658 and 405 nm are both sent through the filter, and the transmitted light signal is monitored to correct the undesired formation of pyrolyzed carbon (PyrC) and then to determine the split point of OC and EC at both wavelengths. BrC aerosols absorb light at 405 nm more significantly than 658 nm in the two-laser system. Due to the strong absorption of BrC at the near-ultraviolet wavelength, the enhanced absorption at 405 nm can serve as an indicator of BrC aerosols (Liu et al., 2015). We define dEC data as the difference in EC concentrations at two wavelengths (dEC = EC405 nm − EC658 nm) to identify the presence of BrC aerosols. Our study provides a 1-year measurement of dEC mass concentrations. Additionally, OC and EC represent the OC and EC concentrations at 658 nm in this paper without a special explanation.
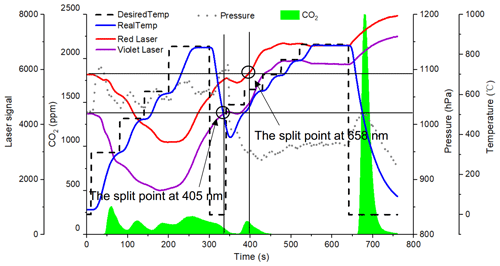
Figure 2Example thermogram of sample analysis using the two-wavelength Sunset semi-continuous carbon analyzer.
At the end of each analysis, a fixed volume of an internal standard, containing 5 % methane and 95 % helium, is injected, and thus, a known carbon mass can be derived. The external sucrose standard (4.207 µg µL−1) calibration was conducted every week to insure repeatable quantification. Calibration with an instrument blank was conducted every day. The detection limit for both OC and EC of the instrument was 0.5 µg m−3. We also did the measurements of OC and EC in PM2.5 filter samples using the same method followed by the NIOSH protocol. All data were corrected to blank measurement before comparison. Figure S1 in the Supplement shows the correlations between the real-time OC and EC concentrations and sampling OC and EC concentrations at the same time. The results showed that the online and offline data during the corresponding periods had good correlations, with R2 of 0.8 for OC, R2 of 0.4 for EC and R2 of 0.8 for total carbon (TC). In order to evaluate the impact of PyrC, we calculated the PyrC at 658 nm fraction of dEC, and the average PyrC/dEC was 4.4 %, indicating the little influence of PyrC.
2.3 Test of the new dEC data
To evaluate the new dEC data, parallel BC concentrations were measured with a seven-wavelength Aethalometer with dEC concentrations in December 2019. Radiation attenuation of an aerosol deposition on a filter (ATNλ) is determined by the Beer–Lambert law as follows:
where I0,λ and Iλ are the measured wavelength-specific laser reflectance signals. ATNλ is used to calculate the attenuation coefficient with Eq. (2) as follows:
where A is the filter area, and V is the sampled air volume. Then a simplified two-component model is used to calculate the contribution of light attenuation to both BC and BrC as follows (Chow et al., 2018; Chen et al., 2015; Sandradewi et al., 2008; Hareley et al., 2008):
where qBC and qBrC are fitting coefficients, and AAE is the absorption Ångström exponent, which represents the wavelength-dependent characteristics of light absorption capability of aerosols. The AAE of BC was assumed to be 1. Fitting coefficients in Eq. (3) were obtained for potential AAEBrC between 1 and 8 by a least squares linear regression, and the AAEBrC leading to the overall best fit in terms of r2 is selected as the effective AAEBrC. Using these fitting coefficients, the bATN due to BC and BrC are calculated at each wavelength. Figure S2 shows that the fitted bATN at 405 nm are within ±5 % of the measured values for bATN>0.01. Figure 3 shows the relationship between the bATN due to BrC at 405 nm and the dEC. Good correlation between them is found with R2 of 0.64, indicating that dEC was associated with BrC.
2.4 Sampling
2.4.1 Real-time PM2.5 observation
The real-time PM2.5 concentrations were measured through the tapered element oscillating microbalance (TEOM) method (TEOM 1405-DF; Thermo Scientific, USA) from August 2015 to July 2016. The resolution of the measured data was 6 min. The instrumental operation maintenance, data assurance and quality control were performed according to the Chinese Ministry of Environmental Protection Standards for PM10 and PM2.5, which was named “HJ 653-2013” (Zhang and Cao, 2015b).
2.4.2 Sample collections
PM2.5 in the atmosphere was collected on 8⋅10 inch (0.2⋅0.25 m prebaked quartz fiber filters (QFFs; Pall Corporation, USA) by a high-volume air sampler (KC-1000; Qingdao, China) at a flow rate of 999 L min−1 in 4 months, namely 4 to 18 June, 6 October to 2 November, 10 to 31 December 2015 and 10 to 31 May 2016. Sampling started and ended at around 08:00 and 20:00 LT (local time), respectively, every day; each sample was collected for 12 h. A total of 148 samples were collected, including four field blanks in the four seasons using 10 min exposure to ambient air without active sampling.
All QFFs were prebaked at 450 ∘C for 6 h before sampling to remove residual carbon. Before and after sampling, all QFFs were weighed with an electronic balance (0.1 mg; Sartorius, Germany). After weighting, the filters were wrapped in aluminum foil, packed in air-tight polyethylene bags and stored at −20 ∘C until further analysis. All procedures during handling of filters were strictly quality controlled to avoid any possible contamination.
2.5 Identification of potential regional sources
The Hybrid Single-Particle Lagrangian Integrated Trajectory (HYSPLIT 4.8) model, provided by the National Oceanic and Atmospheric Administration (NOAA), was used to investigate the air mass origins of carbonaceous aerosols. The 48 h back trajectories at Nanjing (32.2∘ N, 118.7∘ E) were calculated every hour (Draxler and Hess, 1998; Rolph et al., 2017; Cohen et al., 2015). In order to evaluate the behavior of the air mass circulation in the planetary boundary layer (PBL), the trajectories at 500 m, corresponding to the upper-middle height of the PBL, were calculated, representing a well-mixed convective boundary layer for regional transport investigation (Xu and Akhtar, 2010). The National Center for Environmental Prediction Global Data Assimilation System (NCEP GDAS) data obtained from NOAA, with a spatial resolution of and 24 levels of the vertical resolution, were used as meteorological data input to the model. The potential source contribution function (PSCF) model was usually applied to localize the potential sources of pollutants. The details about the setup of the model can be found in Bao et al. (2017).
3.1 Characteristics of carbonaceous aerosols
3.1.1 Concentrations of carbonaceous aerosols
The statistics for the PM2.5, OC, EC and dEC mass concentrations at the NUIST site are summarized in Table 1. The hourly OC concentrations ranged from 0.5 to 45.8 µg m−3 (average of 8.9±5.5 µg m−3), and the EC concentrations ranged from 0.0 to 17.6 µg m−3 (average of 3.1±2.0 µg m−3). The results are comparable to those reported by Chen et al. (2017) in the Xianlin Campus of Nanjing University (5.7 µg m−3 for OC and 3.2 µg m−3 for EC), for which the site was located in the southeastern suburb of Nanjing and close to the G25 highway and was also affected by traffic sources. The higher OC concentrations in this study are probably due to the chemical enterprise emissions. The average contributions of OC and EC to the total measured PM2.5 mass were 12.8 % and 4.3 %, respectively, suggesting that carbonaceous fraction made an important contribution to fine particulate matter. The average dEC mass concentration was 0.8 µg m−3, contributing 10.0 % to OC, 22.3 % to EC and 1.3 % to the PM2.5 concentrations, with a maximum concentration of 8.1 µg m−3 contributing 48.2 % to OC, 97.8 % to EC and 17.6 % to total PM2.5 concentrations. This information can be further applied in the positive matrix factorization (PMF) analysis to evaluate the sources of the carbonaceous aerosols (Zhu et al., 2014; Sahu et al., 2011; Yan et al., 2019).
Compared with carbonaceous aerosol levels in other cities (Table S2), the OC and EC concentrations in Nanjing were generally lower than those observed in urban sites, such as Beijing and Shanghai, and inland cities, like Chengdu and Chongqing, which are affected by the basin terrain characteristics, with static wind and unfavorable diffusion conditions, but higher than those observed in the southern coastal cities such as Guangzhou, which is a megacity in China. The reason for this could be that the site in Guangzhou is a rural site. In general, the level of carbonaceous aerosol concentrations in China is higher than that in developed countries, such as the United States and countries in Europe, and lower than that in developing countries, like India, though the sampling period in India was from late autumn to winter, and the much higher concentrations in India indicated the heavy pollution level. The average ratio in this study was 3.6, which is lower than most of those reported in other studies, indicating the important impact of vehicle emissions at our study site.
Figure 4 shows the mass fractions of hourly carbonaceous aerosols and ratios at different PM2.5 concentration intervals during the study period. During that period, 84.2 % of the PM2.5 samples exceeded the daily averaged Chinese National Ambient Air Quality Standard (NAAQS) of 35.0,µg m−3 for the first grade and 40.1 % of the total samples exceeded the NAAQS of 75.0 µg m−3 for the second grade, reflecting heavy aerosol pollution in the study area. Generally, the fractions of carbonaceous components decreased with increasing PM2.5 pollution level. A larger mass fraction (about 32.3 %) of carbonaceous aerosols in PM2.5 was found for relatively lower PM2.5 levels (0–20 µg m−3) compared to high PM2.5 levels (300–500 µg m−3), with a carbonaceous aerosol mass fraction of 5.2 %. The results indicate that other components, like secondary inorganic aerosol (SIA), contribute more significantly to heavy haze events in Nanjing, which was also found in other cities in the Yangtze River Delta area (Yang et al., 2011; Zhang and Zhang, 2019). The contribution of dEC to OC decreased with the increase in PM2.5 concentrations between 0–200 µg m−3 and then increased with the increase in PM2.5 concentrations between 200–500 µg m−3. The dEC contributed most significantly to OC of 14.3 % for PM2.5 concentrations below 20 µg m−3. A similar trend was found for the ratios, which showed a sharp increase along with enhanced PM2.5 level above 150 µg m−3. Previous studies have reported that high ratios were related to SOC formation or biomass burning emissions, whereas low ratios were related to vehicle exhaust (Wang et al., 2015). We divided the at different intervals of ratios and found that the increased when the ratios increased in the four seasons, indicating strong secondary sources or biomass burning contributions to dEC during heavy pollution periods (Fig. S3).
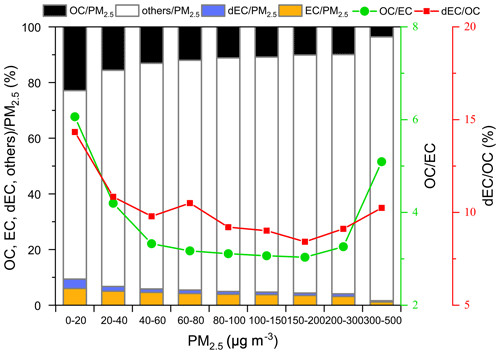
Figure 4Carbonaceous species fractions of PM2.5 and ratios at different PM2.5 concentration intervals at NUIST from June 2015 to August 2016.
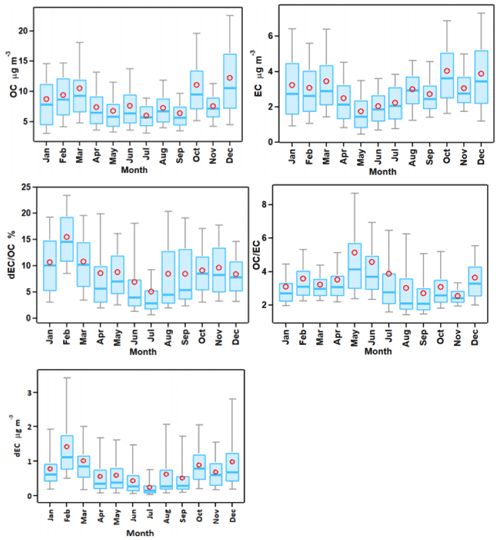
Figure 5Monthly variations in OC, EC and dEC and and ratios at NUIST from June 2015 to August 2016. The boundary of the box indicates the 25 % and 75 % percentile, respectively. The lower and upper whiskers indicate the 10 % and 90 % percentile, respectively. The red circle within the box marks the average, while the line within the box marks the median.
3.1.2 Seasonal variations in carbonaceous aerosols
As shown in Fig. 5, the OC, EC and dEC concentrations and ratios showed similar variations, with the highest in winter and lowest in summer. The average OC and EC concentration in winter was ∼1.4 times and 1.5 times higher than that in summer, and the average dEC concentrations and in winter were approximately 1.4 and 1.6 times higher than those in summer (Table 1). High was found in January and February in winter, indicating the strong influence of anthropogenic sources on dEC, such as coal combustion. In addition, we found strong biomass burning activities in February, which significantly contributed to the high concentrations of dEC in February; more details can be found in Sect. 3.3. The seasonality of carbonaceous species in PM2.5 was strongly influenced by the seasonal variations in emission intensities and meteorological parameters. Table S3 summarizes the meteorological parameters in the four seasons during the study period. The high carbonaceous aerosol concentrations in winter were mainly a result of relatively stable atmospheric conditions with low temperature, relative humidity and boundary layer on the one hand and, on the other hand, increasing emissions from fossil fuel combustion for heating from the chemical enterprises nearby. In summer, the higher boundary layer resulted in the dispersion of aerosols in the atmosphere, and the higher temperature promoted the partitioning of semi-volatile organic compounds (SVOCs) into the gaseous phase (Yang et al., 2011). In addition, heavy precipitation in summer (586 mm in total) favored the wet scavenging processes of aerosols.
The average ratios in spring, summer, autumn and winter were 3.9, 4.0, 2.8 and 3.4, respectively (Table 1). The ratio could give some information about primary and secondary organic carbon (Turpin and Huntzicker, 1995; Lim and Turpin, 2002). In summer, strong convective activities in the atmospheric boundary layer and solar radiation, high temperature and plenty of moisture in the atmosphere were favorable for the formation of SOC. On the other hand, the high ratios in June in this study were also strongly related to biomass burning, which will be discussed in Sect. 3.3. The lower ratios of OC to EC in autumn and winter indicate strong primary sources in these two seasons. It should be noted that the ratios were a rough indicator to estimate the primary and secondary organic carbon; further analysis of the formation of SOC needs to be conducted in the future (Pio et al., 2011; Wu and Yu, 2016).
3.1.3 Diurnal variation in carbonaceous aerosols
The diurnal pattern of carbonaceous aerosols can be affected by both meteorological parameters and sources (Ji et al., 2016). Figure 6 depicts the diurnal variation in OC, EC and dEC and and ratios during the study period. Clear diurnal variations were observed in OC and EC. Both the OC and EC concentrations kept high levels at night and low levels in the daytime, indicating the strong influence of the atmospheric boundary layer on air quality in northern Nanjing. The peak occurred in the morning for both OC and EC, indicating the significant impact of traffic sources on the OC and EC concentrations. The and ratios showed similar trends in the daytime, with a gradual increase from morning till afternoon, indicating the importance of the contribution of secondary sources to dEC. Similar, though not so obvious, diurnal variations were found in dEC. It should be noted that the vehicle emissions and the boundary layer height had no significant effect on the diurnal variation in , suggesting there were no significant local sources of dEC. There was a small peak in at 03:00 LT, which might be related to the aqueous secondary organic aerosol formation during nighttime (Sullivan et al., 2016).
The relative humidity (RH) and temperature (T)-dependent distributions of OC, EC mass concentrations and and throughout the study period are shown in Fig. 7. High (>30 %) can be found in three areas; the first is shown in the right area, with relatively high T at 25–40 ∘C and RH at 40 %–60 %, which were usually found in the summer afternoon, which was closely related to the strong formation of SOC. This distribution was also seen in . The second area is in the upper region, with RH over 80 % and T at 10–20 ∘C, and the third area appears for RH below 30 % and T at about 10 ∘C, corresponding to nighttime and winter afternoon. In general, dEC had no strong dependence on the RH and T distribution, indicating the complex formation mechanism of dEC. OC and EC show similar distributions with the highest mass loading (OC is >20 µg m−3; EC is >8 µg m−3) at relatively high RH at 60 %–80 %, which usually occurred at night with relatively low boundary layer height, leading to the accumulation of aerosols. However, the corresponding ratios were low, suggesting the importance of primary sources to OC and EC in northern Nanjing, which will be verified in the wind rose of OC and EC (Fig. 8).
3.2 Air mass transport
3.2.1 Wind rose of carbonaceous aerosols
To investigate the influence of air mass transport on the study site, the wind rose of OC, EC and , using hourly data in the four seasons, is shown in Fig. 8 (Carslaw and Ropkins, 2012). There are two points that should be noted. First, high OC and EC mass concentrations were found near the field site (indicated by wind speed (WS) < 1 m s−1), suggesting that local and primary emissions (e.g., industrial and vehicle emissions) were stable and important sources contributing to atmospheric OC and EC mass concentrations in northern Nanjing. The OC mass concentrations from the southwest increased with the increase of WS in summer, indicating that the sources of OC are complicated in summer, including secondary reaction during long-range or regional transport. Second, compared with OC and EC, dEC showed no significant local sources. The increased with increasing WS, and the highest were found for WS over 3 m s−1. Long-range or regional transport were highly likely the main sources contributing to the dEC mass concentrations.
3.2.2 The potential source areas of carbonaceous aerosols
The possible source contributions were evaluated using the PSCF model, and the PSCF maps are shown in Fig. 9 (Petit et al., 2017). The areas with high PSCF values were highly likely the potential pollution source areas. As shown in Fig. 9, the PSCF results further proved the strong regional transport contribution to dEC and local contributions to OC and EC. In spring, the potential source areas of OC and EC were mainly from the southwest of Nanjing; however, the potential source areas of dEC were from the east of Nanjing, indicating obvious different sources between OC, EC and dEC. In summer, local areas were the main source areas of EC, and the nearby Yangtze River Delta City Group from the southeast of Nanjing, including developed cities like Shanghai, were the main sources areas of OC and dEC. The anthropogenic emissions from these areas might be important sources of OC and dEC. Besides, both the potential source areas of dEC and EC were in the northwest of Nanjing in summer, suggesting strong primary sources of dEC from this area, which were very likely associated with biomass burning; more details are given in Sect. 3.3. In autumn, local sources from the study site were strongest for OC and EC. However, dEC mainly originated from regional transport from the northwestern and southeastern areas of Nanjing. Biomass burning has been proved to be an important source of air pollutants in the Yangtze River Delta (YRD) area, especially in the wheat harvest seasons (e.g., June and October; Cheng et al., 2014; Zhang and Cao, 2015a). In addition, the YRD area is the most economically developed region in China and has lots of industrial cities, which means that industrial emissions and anthropogenic sources contribute to high carbonaceous aerosol pollution levels. In winter, dEC was mainly from long-range transport from northern cities and regional transport from the southwestern areas of Nanjing, while both long-range transport and local sources were found in OC and EC concentrations.
Table 2Statistics of OC, EC, , dEC and during biomass burning days and normal days. The values represent the average ( ± ) standard deviation.
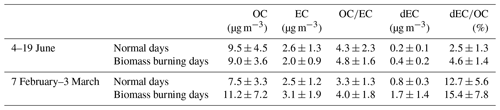
3.3 The characteristics of carbonaceous aerosols during biomass burning periods
Biomass burning emissions have been proved to be an important source of BrC on a global scale; they are consistently observed in large-scale forest fire events (Laskin et al., 2015). Based on the Fire Information for Resource Management System (FIRMS) derived from the Moderate Resolution Imaging Spectroradiometer (MODIS), we found that the fire points amounted to 2028, 1773 and 967 on 11 June 2015, 7 February 2016 and 2 March 2016, respectively, in the areas around our study site, suggesting there were strong biomass burning events on these days (Fig. S4). To further investigate the biomass burning impact on dEC, we analyzed the temporal trends of carbonaceous aerosols from 4 to 19 June 2015 and 7 February to 3 March 2016, respectively. Combining the observed aerosol concentrations and fire information, we divided the periods into normal days and biomass burning days. It should be noted that the biomass burning days are not determined based only on fire points. We also considered the 48 h backward trajectories and open biomass burning areas. For example, we found lots of fire points from 11 to 12 June 2015 and from 7 to 10 February 2016, respectively, and the 48 h back trajectories passed over these biomass burning areas (Fig. S5b and c). However, although there were large amounts of fire points in the northwest of Nanjing from 8 to 9 June 2015, the backward trajectories showed that the air masses during the periods came from the southeastern areas where no open fire points were found (Fig. S5a). In contrast, there were only a few fire points found near the study site from 26 to 27 February 2016; the 48 h backward trajectories showed the air masses came from the area (Fig. S5d).
As shown in Figs. 10 and 11, we found that dEC concentrations and and ratios showed peaks during each biomass burning period which were not that obvious in OC and EC concentrations, suggesting that the unique biomass burning impact on dEC and the sources of OC and EC were more complicated. It should be noted that there were peaks of dEC on 9 June 2015 and 13 February 2016, which were not biomass burning days, suggesting that biomass burning was not the only source of dEC. As mentioned in Sect. 3.1 and 3.2, anthropogenic emissions could be the sources of dEC, and the secondary sources cannot be ignored, either. Summarized in Table 2 are the average and standard deviation values of OC, EC, , dEC and during biomass burning and normal days. The , dEC concentrations and were obviously higher on biomass burning days than in normal days, but similar levels of the OC and EC concentrations were found on both biomass burning days and normal days in summer, suggesting the great contribution of biomass burning emissions to dEC, and there were other sources of OC and EC in summer. All the carbonaceous aerosols were higher on biomass burning days in winter; in addition, the locations of open-fire counts were mainly in the northwestern and southwestern area of the study site (Fig. S5c and d), which were the potential source areas of OC, EC and dEC in winter, as discussed in Sect. 3.2.2, indicating strong contributions of biomass burning emissions to all the carbonaceous aerosols in winter.
In this study, the characteristics and sources of carbonaceous aerosols in northern Nanjing were investigated, and we introduced a two-wavelength method by modifying the Sunset carbon analyzer. We incorporated a new diode laser at λ=405 nm in the instrument, making it possible to detect the laser beam passing through the filter, at both wavelength λ=658 nm and λ=405 nm, so that we can obtain the dEC concentrations. Our study illustrated the feasibility of using dEC to characterize the BrC aerosols, providing a new idea about the measurement of BrC. The results showed that high (low) OC, EC and dEC concentrations were found in winter (summer), indicating the significant impact of the increase in various emission sources in winter and wet scavenging by rain in summer. Similar diurnal cycles for OC and EC concentrations were found to be high at night and low in the daytime and strongly affected by the boundary layer. Traffic emissions were found to have a significant influence on the concentrations of OC and EC. Similar trends were found in the diurnal cycle of and , and the increased when the ratio increased, indicating strong secondary sources or biomass burning impact on dEC. The wind rose and receptor model results showed that strong local emissions were found for OC and EC; however, dEC was significantly affected by regional or long-range transport. The nearby YRD area was one of the main potential source areas of dEC, suggesting that anthropogenic emissions could be the sources of dEC. Together with the back trajectories analysis and MODIS fire information, we analyzed two biomass burning events in both summer and winter. The results showed that the sources of OC and EC were more complicated than those of dEC in summer. Biomass burning emissions made a great contribution to dEC concentrations in summer. A large number of open-fire counts from the northwestern and southwestern areas of the study site was observed; these fires significantly contributed to the carbonaceous aerosol pollution.
Our modified two-wavelength instrument provided more information than the traditional single-wavelength thermal–optical carbon analyzer. The results proved that dEC can be an indicator of BrC on biomass burning days. It should be noted that the sources of dEC were complicated, and the anthropogenic emissions and secondary formations of dEC aerosols could not be ignored; further chemical analysis needs to be conducted in the future. The evaluation of SOC formation and the relationship between dEC and SOC can be conducted. In addition, more chemical analysis, such as the analysis for ions, organic matter or sugars in PM2.5 can be made; thus, we can obtain some information of the tracers of different sources, and more accurate and quantitative source apportionment can be done (Bhattaraia et al., 2019; Wu et al., 2018, 2019). We also hope that the dEC data can be further applied in more research.
All the original research data used in this paper are available from the Open Science Framework (https://doi.org/10.17605/OSF.IO/MA8CU; Bao, 2021).
The supplement related to this article is available online at: https://doi.org/10.5194/amt-14-4053-2021-supplement.
YLZ designed the study. MB, YLZ and FC contributed to the data collection and analysis. MB and YLZ prepared the article with contributions from the other authors.
The authors declare that they have no conflict of interest.
This research was financially supported by the National Natural Science Foundation of China (grant no. 41977305), the Provincial Natural Science Foundation of Jiangsu (grant no. BK20180040) and the Postgraduate Research and Practice Innovation Program of Jiangsu Province (grant no. KYCX18_1014). This study was supported by the funding of Jiangsu Innovation and Entrepreneurship Team. The authors would also like to thank the China Scholarship Council for the support of Mengying Bao. We would also like to express our gratitude to Yuanyuan Zhang, Zufei Xu and Tianran Zhang, for their assistance in the instrument maintenance throughout the observation period. Additionally, we are grateful for Yunhua Chang, who made considerable comments and suggestions for this paper.
This research has been supported by the National Natural Science Foundation of China (grant no. 41977305), the Provincial Natural Science Foundation of Jiangsu (grant no. BK20180040), and the Postgraduate Research and Practice Innovation Program of Jiangsu Province (grant no. KYCX18_1014).
This paper was edited by Willy Maenhaut and reviewed by three anonymous referees.
Andreae, M. O. and Gelencsér, A.: Black carbon or brown carbon? The nature of light-absorbing carbonaceous aerosols, Atmos. Chem. Phys., 6, 3131–3148, https://doi.org/10.5194/acp-6-3131-2006, 2006.
Arola, A., Schuster, G., Myhre, G., Kazadzis, S., Dey, S., and Tripathi, S. N.: Inferring absorbing organic carbon content from AERONET data, Atmos. Chem. Phys., 11, 215–225, https://doi.org/10.5194/acp-11-215-2011, 2011.
Bao, M.: Highly time-resolved characterization of carbonaceous aerosols using a two-wavelength Sunset thermal-optical carbon analyzer, OSF, https://doi.org/10.17605/OSF.IO/MA8CU, 2021.
Bao, M., Cao, F., Chang, Y., Zhang, Y.-L., Gao, Y., Liu, X., Zhang, Y., Zhang, W., Tang, T., Xu, Z., Liu, S., Lee, X., Li, J., and Zhang, G.: Characteristics and origins of air pollutants and carbonaceous aerosols during wintertime haze episodes at a rural site in the Yangtze River Delta, China, Atmos. Pollut. Res., 8, 900–911, https://doi.org/10.1016/j.apr.2017.03.001, 2017.
Bhattaraia, H., Saikawac, E., Wana, X., Zhue, H., Ram, K., Gao, S., Kang, S., Zhanga, Q., Zhang, Y., Wu, G., Wang, X., Kawamura, K., Fui, P., and Cong, Z.: Levoglucosan as a tracer of biomass burning recent progress and perspectives, Atmos. Res., 220, 20–33, https://doi.org/10.1016/j.atmosres.2019.01.004, 2019.
Birch, M. E. and Cary, R. A.: Elemental carbon-based method for occupational monitoring of particulate diesel exhaust: methodology and exposure issues, Analyst, 121, 1183–1190, 1996.
Cao, F. and Zhang, Y.-L.: Principle, method development and application of radiocarbon (14C) – based source apportionment of carbonaceous aerosols: a review, Adv. Earth Sci., 30, 425–432, 2015.
Carslaw, D. C. and Ropkins, K.: openair – An R package for air quality data analysis, Environ. Model. Softw., 27–28, 52–61, https://doi.org/10.1016/j.envsoft.2011.09.008, 2012.
Chen, D., Cui, H., Zhao, Y., Yin, L., Lu, Y., and Wang, Q.: A two-year study of carbonaceous aerosols in ambient PM2.5 at a regional background site for western Yangtze River Delta, China, Atmos. Res., 183, 351–361, https://doi.org/10.1016/j.atmosres.2016.09.004, 2017. Chen, L. W. A., Chow, J. C., Wang, X. L., Robles, J. A., Sumlin, B. J., Lowenthal, D. H., Zimmermann, R., and Watson, J. G.: Multi-wavelength optical measurement to enhance thermal/optical analysis for carbonaceous aerosol, Atmos. Meas. Tech., 8, 451–461, https://doi.org/10.5194/amt-8-451-2015, 2015.
Cheng, Z., Wang, S., Fu, X., Watson, J. G., Jiang, J., Fu, Q., Chen, C., Xu, B., Yu, J., Chow, J. C., and Hao, J.: Impact of biomass burning on haze pollution in the Yangtze River delta, China: a case study in summer 2011, Atmos. Chem. Phys., 14, 4573–4585, https://doi.org/10.5194/acp-14-4573-2014, 2014.
Chow, J. C., Watson, J. G., Chen, L.-W. A., Arnott, W. P., Moosmüller, H., and Fung, K.: Equivalence of elemental carbon by thermal/optical reflectance and transmittance with different temperature protocols, Environ. Sci. Technol., 38, 4414–4422, https://doi.org/10.1021/es034936u, 2004.
Chow, J. C., Watson, J. G., Chen, L. W., Chang, M. C., Robinson, N. F., Trimble, D., and Kohl, S.: The IMPROVE_A temperature protocol for thermal/optical carbon analysis: maintaining consistency with a long-term database, J. Air Waste Manage. Assoc., 57, 1014–1023, https://doi.org/10.3155/1047-3289.57.9.1014, 2007.
Chow, J. C., Watson, J. G., Green, M. C., Wang, X., Chen, L. A., Trimble, D. L., Cropper, P. M., Kohl, S. D., and Gronstal, S. B.: Separation of brown carbon from black carbon for IMPROVE and Chemical Speciation Network PM2.5 samples, J. Air Waste Manage. Assoc., 68, 494–510, 2018.
Cohen, M. D., Stunder, B. J. B., Rolph, G. D., Draxler, R. R., Stein, A. F., and Ngan, F.: NOAA's HYSPLIT atmospheric transport and dispersion modeling system, B. Am. Meteorol. Soc., 96, 2059–2077, https://doi.org/10.1175/bams-d-14-00110.1, 2015.
Draxler, R. R. and Hess, G. D.: An overview of the HYSPLIT_4 modelling system for trajectories, dispersion, and deposition, Aust. Meteorol. Mag., 47, 295–308, 1998.
Feng, Y., Ramanathan, V., and Kotamarthi, V. R.: Brown carbon: a significant atmospheric absorber of solar radiation?, Atmos. Chem. Phys., 13, 8607–8621, https://doi.org/10.5194/acp-13-8607-2013, 2013.
Hareley, O. L., Corrigan, C. E., and Kirchstetter, T. W.: Modified thermal-optical analysis using spectral absorption selectivity to distinguish black carbon from pyrolized organic carbon, Environ. Sci. Technol., 42, 8459–8464, https://doi.org/10.1021/es800448n, 2008.
Huang, R. J., Zhang, Y., Bozzetti, C., Ho, K. F., Cao, J. J., Han, Y., Daellenbach, K. R., Slowik, J. G., Platt, S. M., Canonaco, F., Zotter, P., Wolf, R., Pieber, S. M., Bruns, E. A., Crippa, M., Ciarelli, G., Piazzalunga, A., Schwikowski, M., Abbaszade, G., Schnelle-Kreis, J., Zimmermann, R., An, Z., Szidat, S., Baltensperger, U., El Haddad, I., and Prevot, A. S.: High secondary aerosol contribution to particulate pollution during haze events in China, Nature, 514, 218–222, https://doi.org/10.1038/nature13774, 2014.
Ji, D. S., Zhang, J. K., He, J., Wang, X. J., Pang, B., Liu, Z. R., Wang, L. L., and Wang, Y. S.: Characteristics of atmospheric organic and elemental carbon aerosols in urban Beijing, China, Atmos. Environ., 306, 293–306, https://doi.org/10.1016/j.atmosenv.2015.11.020, 2016.
Kirillova, E. N., Andersson, A., Han, J., Lee, M., and Gustafsson, O.: Sources and light absorption of water-soluble organic carbon aerosols in the outflow from northern China, Atmos. Chem. Phys., 14, 1413–1422, https://doi.org/10.5194/acp-14-1413-2014, 2014.
Laskin, A., Laskin, J., and Nizkorodov, S. A.: Chemistry of atmospheric brown carbon, Chem. Rev., 115, 4335–4382, https://doi.org/10.1021/cr5006167, 2015.
Lei, Y., Shen, Z., Zhang, T., Zhang, Q., Wang, Q., Sun, J., Gong, X., Cao, J., Xu, H., Liu, S., and Yang, L.: Optical source profiles of brown carbon in size-resolved particulate matter from typical domestic biofuel burning over Guanzhong Plain, China, Sci. Total. Environ., 622–623, 244–251, https://doi.org/10.1016/j.scitotenv.2017.11.353, 2018.
Lelieveld, J., Evans, J. S., Fnais, M., Giannadaki, D., and Pozzer, A.: The contribution of outdoor air pollution sources to premature mortality on a global scale, Nature, 525, 367–371, https://doi.org/10.1038/nature15371, 2015.
Li, C., He, Q., Hettiyadura, A. P. S., Kafer, U., Shmul, G., Meidan, D., Zimmermann, R., Brown, S. S., George, C., Laskin, A., and Rudich, Y.: Formation of secondary brown carbon in biomass burning aerosol proxies through NO3 radical reactions, Environ. Sci. Technol., 54, 1395–1405, https://doi.org/10.1021/acs.est.9b05641, 2020.
Lim, H.-J. and Turpin, B. J.: Origins of primary and secondary organic aerosol in Atlanta: results of time-resolved measurements during the Atlanta supersite experiment, Environ. Sci. Technol., 36, 4489–4496, https://doi.org/10.1021/es0206487, 2002.
Liu, S., Aiken, A. C., Gorkowski, K., Dubey, M. K., Cappa, C. D., Williams, L. R., Herndon, S. C., Massoli, P., Fortner, E. C., Chhabra, P. S., Brooks, W. A., Onasch, T. B., Jayne, J. T., Worsnop, D. R., China, S., Sharma, N., Mazzoleni, C., Xu, L., Ng, N. L., Liu, D., Allan, J. D., Lee, J. D., Fleming, Z. L., Mohr, C., Zotter, P., Szidat, S., and Prevot, A. S. H.: Enhanced light absorption by mixed source black and brown carbon particles in UK winter, Nat. Commun., 6, 8435, https://doi.org/10.1038/ncomms9435, 2015.
Liu, X., Zhang, Y.-L., Peng, Y., Xu, L., Zhu, C., Cao, F., Zhai, X., Haque, M. M., Yang, C., Chang, Y., Huang, T., Xu, Z., Bao, M., Zhang, W., Fan, M., and Lee, X.: Chemical and optical properties of carbonaceous aerosols in Nanjing, eastern China: regionally transported biomass burning contribution, Atmos. Chem. Phys., 19, 11213–11233, https://doi.org/10.5194/acp-19-11213-2019, 2019.
Massabò, D., Caponi, L., Bove, M. C., and Prati, P.: Brown carbon and thermal–optical analysis: A correction based on optical multi-wavelength apportionment of atmospheric aerosols, Atmos. Environ., 125, 119–125, https://doi.org/10.1016/j.atmosenv.2015.11.011, 2016.
Petit, J. E., Favez, O., Albinet, A., and Canonaco, F.: A user-friendly tool for comprehensive evaluation of the geographical origins of atmospheric pollution: Wind and trajectory analyses, Environ. Model. Softw., 88, 183–187, https://doi.org/10.1016/j.envsoft.2016.11.022, 2017.
Pio, C., Cerqueira, M., Harrison, R. M., Nunes, T., Mirante, F., Alves, C., Oliveira, C., Sanchez de la Campa, A., Artíñano, B., and Matos, M.: ratio observations in Europe: Re-thinking the approach for apportionment between primary and secondary organic carbon, Atmos. Environ., 45, 6121–6132, https://doi.org/10.1016/j.atmosenv.2011.08.045, 2011.
Rolph, G., Stein, A., and Stunder, B.: Real-time environmental applications and display system: READY, Environ. Model. Softw., 95, 210–228, https://doi.org/10.1016/j.envsoft.2017.06.025, 2017.
Sahu, M., Hu, S., Ryan, P. H., Le Masters, G., Grinshpun, S. A., Chow, J. C., and Biswas, P.: Chemical compositions and source identification of PM2.5 aerosols for estimation of a diesel source surrogate, Sci. Total. Environ., 409, 2642–2651, https://doi.org/10.1016/j.scitotenv.2011.03.032, 2011.
Saleh, R., Robinson, E. S., Tkacik, D. S., Ahern, A. T., Liu, S., Aiken, A. C., Sullivan, R. C., Presto, A. A., Dubey, M. K., Yokelson, R. J., Donahue, N. M., and Robinson, A. L.: Brownness of organics in aerosols from biomass burning linked to their black carbon content, Nat. Geosci., 7, 647–650, https://doi.org/10.1038/ngeo2220, 2014.
Sandradewi, J., Prévôt, A. S. H., Szidat, S., Perron, N., Alfarra, M. R., Lanz, V. A., Weingartner, E., and Baltensperger, U.: Using aerosol light absorption measurements for the quantitative determination of wood burning and traffic emission contributions to particulate matter, Environ. Sci. Technol., 42, 3316–3323, https://doi.org/10.1021/es702253m, 2008.
Satish, R., Shamjad, P., Thamban, N., Tripathi, S., and Rastogi, N.: Temporal characteristics of brown carbon over the central Indo-Gangetic Plain, Environ. Sci. Technol., 51, 6765–6772, https://doi.org/10.1021/acs.est.7b00734, 2017.
Sullivan, A. P., Hodas, N., Turpin, B. J., Skog, K., Keutsch, F. N., Gilardoni, S., Paglione, M., Rinaldi, M., Decesari, S., Facchini, M. C., Poulain, L., Herrmann, H., Wiedensohler, A., Nemitz, E., Twigg, M. M., and Collett Jr., J. L.: Evidence for ambient dark aqueous SOA formation in the Po Valley, Italy, Atmos. Chem. Phys., 16, 8095–8108, https://doi.org/10.5194/acp-16-8095-2016, 2016.
Turpin, B. J. and Huntzicker, J.: Identification of secondary organic aerosol episodes and quantitation of frimary and secondary organic aerosol concentrations during SCAQS, Atmos. Environ., 29, 3527–3544, 1995.
US EPA: Review of sunset organic and elemental carbon (OC and EC) measurements during EPA's sunset carbon evaluation project, Sonoma Technology, Inc., CA, US Environmental Protection Agency, NC, 2019.
Wang, J., Nie, W., Cheng, Y., Shen, Y., Chi, X., Wang, J., Huang, X., Xie, Y., Sun, P., Xu, Z., Qi, X., Su, H., and Ding, A.: Light absorption of brown carbon in eastern China based on 3-year multi-wavelength aerosol optical property observations and an improved absorption Ångström exponent segregation method, Atmos. Chem. Phys., 18, 9061–9074, https://doi.org/10.5194/acp-18-9061-2018, 2018.
Wang, P., Cao, J. J., Shen, Z. X., Han, Y. M., Lee, S. C., Huang, Y., Zhu, C. S., Wang, Q. Y., Xu, H. M., and Huang, R. J.: Spatial and seasonal variations of PM2.5 mass and species during 2010 in Xi'an, China, Sci. Total. Environ., 508, 477–487, https://doi.org/10.1016/j.scitotenv.2014.11.007, 2015.
Wang, Y., Hopke, P. K., Rattigan, O. V., Xia, X., Chalupa, D. C., and Utell, M. J.: Characterization of residential wood combustion particles using the two-wavelength aethalometer, Environ. Sci. Technol., 45, 7387–7393, https://doi.org/10.1021/es2013984, 2011.
Wang, Y., Hopke, P. K., and Rattigan, O. V.: A new indicator of fireworks emissions in Rochester, New York, Environ. Monit. Assess., 184, 7293–7297, https://doi.org/10.1007/s10661-011-2497-5, 2012a.
Wang, Y., Hopke, P. K., Rattigan, O. V., Chalupa, D. C., and Utell, M. J.: Multiple-year black carbon measurements and source apportionment using delta-C in Rochester, New York, J. Air Waste Manage. Assoc., 62, 880–887, https://doi.org/10.1080/10962247.2012.671792, 2012b.
Wu, C. and Yu, J. Z.: Determination of primary combustion source organic carbon-to-elemental carbon () ratio using ambient OC and EC measurements: secondary OC–EC correlation minimization method, Atmos. Chem. Phys., 16, 5453–5465, https://doi.org/10.5194/acp-16-5453-2016, 2016.
Wu, G., Wan, X., Gao, S., Fu, P., Yin, Y., Li, G., Zhang, G., Kang, S., Ram, K., and Cong, Z.: Humic-like substances (HULIS) in aerosols of central Tibetan Plateau (Nam Co, 4730 m asl): Abundance, light absorption properties, and sources, Environ. Sci. Technol., 52, 7203–7211, https://doi.org/10.1021/acs.est.8b01251, 2018.
Wu, G., Ram, K., Fu, P., Wang, W., Zhang, Y., Liu, X., Stone, E. A., Pradhan, B. B., Dangol, P. M., Panday, A. K., Wan, X., Bai, Z., Kang, S., Zhang, Q., and Cong, Z.: Water-soluble brown carbon in atmospheric aerosols from Godavari (Nepal), a regional representative of South Asia, Environ. Sci. Technol., 53, 3471–3479, https://doi.org/10.1021/acs.est.9b00596, 2019.
Wu, G., Wan, X., Ram, K., Li, P., Liu, B., Yin, Y., Fu, P., Loewen, M., Gao, S., Kang, S., Kawamura, K., Wang, Y., and Cong, Z.: Light absorption, fluorescence properties and sources of brown carbon aerosols in the Southeast Tibetan Plateau, Environ. Pollut., 257, 113616, https://doi.org/10.1016/j.envpol.2019.113616, 2020.
Xu, X. and Akhtar, U. S.: Identification of potential regional sources of atmospheric total gaseous mercury in Windsor, Ontario, Canada using hybrid receptor modeling, Atmos. Chem. Phys., 10, 7073–7083, https://doi.org/10.5194/acp-10-7073-2010, 2010.
Yan, C., Zheng, M., Bosch, C., Andersson, A., Desyaterik, Y., Sullivan, A. P., Collett, J. L., Zhao, B., Wang, S., He, K., and Gustafsson, O.: Important fossil source contribution to brown carbon in Beijing during winter, Sci. Rep., 7, 43182, https://doi.org/10.1038/srep43182, 2017.
Yan, C., Zheng, M., Shen, G., Cheng, Y., Ma, S., Sun, J., Cui, M., Zhang, F., Han, Y., and Chen, Y.: Characterization of carbon fractions in carbonaceous aerosols from typical fossil fuel combustion sources, Fuel, 254, 115620, https://doi.org/10.1016/j.fuel.2019.115620, 2019.
Yang, F., Tan, J., Zhao, Q., Du, Z., He, K., Ma, Y., Duan, F., Chen, G., and Zhao, Q.: Characteristics of PM2.5 speciation in representative megacities and across China, Atmos. Chem. Phys., 11, 5207–5219, https://doi.org/10.5194/acp-11-5207-2011, 2011.
Zhang, Q., Shen, Z., Zhang, L., Zeng, Y., Ning, Z., Zhang, T., Lei, Y., Wang, Q., Li, G., Sun, J., Westerdahl, D., Xu, H., and Cao, J.: Investigation of primary and secondary particulate brown carbon in two Chinese cities of Xi'an and Hong Kong in wintertime, Environ. Sci. Technol., 54, 3803–3813, https://doi.org/10.1021/acs.est.9b05332, 2020.
Zhang, W. and Zhang, Y.: Oxygen isotope anomaly (Δ17O) in atmospheric nitrate: a review, Chinese Sci. Bull., 64, 649–662, https://doi.org/10.1360/n972018-01028, 2019.
Zhang, W., Zhang, Y.-L., Cao, F., Xiang, Y., Zhang, Y., Bao, M., Liu, X., and Lin, Y.-C.: High time-resolved measurement of stable carbon isotope composition in water-soluble organic aerosols: method optimization and a case study during winter haze in eastern China, Atmos. Chem. Phys., 19, 11071–11087, https://doi.org/10.5194/acp-19-11071-2019, 2019.
Zhang, X., Lin, Y.-H., Surratt, J. D., Zotter, P., Prevot, A. S. H., and Weber, R. J.: Light-absorbing soluble organic aerosol in Los Angeles and Atlanta: a contrast in secondary organic aerosol, Geophys. Res. Lett., 38, L21810, https://doi.org/10.1029/2011gl049385, 2011.
Zhang, Y. and Kang, S.: Characteristics of carbonaceous aerosols analyzed using a multiwavelength thermal/optical carbon analyzer: a case study in Lanzhou City, Sci. China Earth Sci., 62, 389–402, https://doi.org/10.1007/s11430-017-9245-9, 2019.
Zhang, Y., Ren, H., Sun, Y., Cao, F., Chang, Y., Liu, S., Lee, X., Agrios, K., Kawamura, K., Liu, D., Ren, L., Du, W., Wang, Z., Prevot, A. S. H., Szida, S., and Fu, P.: High contribution of nonfossil sources to submicrometer organic aerosols in Beijing, China, Environ. Sci. Technol., 51, 7842–7852, https://doi.org/10.1021/acs.est.7b01517, 2017.
Zhang, Y.-L. and Cao, F.: Is it time to tackle PM2.5 air pollutions in China from biomass-burning emissions?, Environ. Pollut., 202, 217-219, 10.1016/j.envpol.2015.02.005, 2015a.
Zhang, Y. L. and Cao, F.: Fine particulate matter (PM2.5) in China at a city level, Sci. Rep., 5, 14884, https://doi.org/10.1038/srep14884, 2015b.
Zhou, S., Wang, T., Wang, Z., Li, W., Xu, Z., Wang, X., Yuan, C., Poon, C. N., Louie, P. K. K., Luk, C. W. Y., and Wang, W.: Photochemical evolution of organic aerosols observed in urban plumes from Hong Kong and the Pearl River Delta of China, Atmos. Environ., 88, 219–229, https://doi.org/10.1016/j.atmosenv.2014.01.032, 2014.
Zhu, C. S., Cao, J. J., Tsai, C. J., Shen, Z. X., Han, Y. M., Liu, S. X., and Zhao, Z. Z.: Comparison and implications of PM2.5 carbon fractions in different environments, Sci. Total. Environ., 466–467, 203–209, https://doi.org/10.1016/j.scitotenv.2013.07.029, 2014.