the Creative Commons Attribution 4.0 License.
the Creative Commons Attribution 4.0 License.
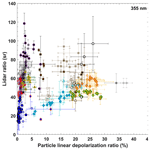
DeLiAn – a growing collection of depolarization ratio, lidar ratio and Ångström exponent for different aerosol types and mixtures from ground-based lidar observations
Holger Baars
Ronny Engelmann
Dietrich Althausen
Albert Ansmann
Stephanie Bohlmann
Birgit Heese
Julian Hofer
Thomas Kanitz
Moritz Haarig
Kevin Ohneiser
Martin Radenz
Patric Seifert
Annett Skupin
Zhenping Yin
Sabur F. Abdullaev
Mika Komppula
Maria Filioglou
Elina Giannakaki
Iwona S. Stachlewska
Lucja Janicka
Daniele Bortoli
Eleni Marinou
Vassilis Amiridis
Anna Gialitaki
Rodanthi-Elisavet Mamouri
Boris Barja
Ulla Wandinger
This paper presents a collection of lidar-derived aerosol intensive optical properties for several aerosol types, namely the particle linear depolarization ratio, the extinction-to-backscatter ratio (lidar ratio) and the Ångström exponent. The data collection, named DeLiAn, is based on globally distributed, long-term, ground-based, multiwavelength, Raman and polarization lidar measurements, conducted mainly with lidars that have been developed at the Leibniz Institute for Tropospheric Research. The intensive optical properties are presented at two wavelengths, 355 and 532 nm, for 13 aerosol categories. The categories cover the basic aerosol types (i.e., marine, pollution, continental European background, volcanic ash, smoke, mineral dust), as well as the most frequently observed mixtures they form. This extensive collection also incorporates more peculiar aerosol categories, including dried marine aerosol that, compared to marine aerosol, exhibits a significantly enhanced depolarization ratio (up to 15 %). Besides Saharan dust, additional mineral dust types related to their source region were identified due to their lower lidar ratios (Central Asian and Middle Eastern dust). In addition, extreme wildfire events (such as in north America and Australia) emitted smoke into the stratosphere showing significantly different optical properties, i.e., high depolarization values (up to 25 %), compared to tropospheric smoke. The data collection reflects and underlines the variety of aerosol mixtures in the atmosphere and can be used for the development of aerosol-typing schemes. The paper contains the most up-to-date and comprehensive overview of optical properties from aerosol lidar measurements and, therefore, provides a solid basis for future aerosol retrievals in the frame of both spaceborne and ground-based lidars. Furthermore, DeLiAn can assist the efforts for the harmonization of satellite records of aerosol properties performed at different wavelengths.
- Article
(8156 KB) - Full-text XML
- BibTeX
- EndNote
Aerosol typing is intertwined with the scientific efforts for the quantification of the direct and indirect aerosol radiative effects; the identification of the main aerosol sources; and the improvement of the aerosol measurements, retrievals and models. All these efforts have a common baseline: the reduction of the aerosol-induced uncertainties in the Earth’s radiative budget (Boucher et al., 2013). The uncertainties are directly linked to the physicochemical properties and the spatiotemporal variability of the aerosol particles. Apart from the impact on the environment, aerosols impact human health as well, and therefore, aerosol typing is necessary for air quality monitoring and assessment (Fuzzi et al., 2015).
The climate response to aerosols is not only type- but also altitude-dependent, and therefore, the vertical aerosol distribution is a key factor for the evaluation of the direct aerosol radiative effect (Hansen et al., 1997). Lidars are the only instruments that can accurately characterize complex aerosol mixtures as they provide information on the optical and microphysical properties of different aerosol types along with their vertical distribution (Ansmann and Müller, 2005). Lidar-derived intensive optical parameters can be used effectively for aerosol-typing purposes because, in contrast to extensive properties, they are concentration-independent. The extinction-to-backscatter ratio (lidar ratio), the particle linear depolarization ratio and the Ångström exponent (backscatter- and extinction-related) reveal information about the size, shape and absorption efficiency of the aerosol particles. The combination of different lidar-derived intensive optical properties is proven to be a sophisticated way for the classification of the different aerosol types and their mixtures (e.g., Sasano and Browell, 1989; Sugimoto et al., 2002; Ansmann et al., 2002a; Müller et al., 2002, 2003, 2005; Mattis et al., 2002b, 2004; Tesche et al., 2009b, 2011a; Groß et al., 2011, 2013; Weinzierl et al., 2011; Burton et al., 2012; Papagiannopoulos et al., 2018; Nicolae et al., 2018). Especially the combination of the particle linear depolarization ratio and the lidar ratio is highly effective for classification purposes, since those parameters exhibit the highest discrimination power among the lidar-derived intensive optical parameters (Burton et al., 2012).
Due to the high capabilities of the lidar instruments with respect to aerosol monitoring and characterization, several lidar networks have emerged around the globe in the last decades. The Micro-Pulse Lidar Network (MPLNET) of the National Aeronautics and Space Administration (NASA) has sites mainly across North America (Welton et al., 2002), the Asian Dust and Aerosol Lidar Observation Network (AD-Net) is expanding in East Asia (Sugimoto et al., 2014), the Latin American Lidar Network (LALINET) over Latin America (Antuña-Marrero et al., 2017), and most parts of Europe are covered by the European Aerosol Research Lidar Network (EARLINET; Pappalardo et al., 2014). These networks operate different lidar systems and therefore have different capabilities. For instance, MPLNET is equipped with an elastic-backscatter lidar that operates at 532 nm. AD-Net is mostly equipped with Raman lidars operational mainly at 532 and 1064 nm. Similarly, EARLINET stations are mostly equipped with multiwavelength (355, 532 and 1064 nm) Raman lidars. Elastic lidar systems have limited capabilities (e.g., with respect to aerosol typing) compared to Raman lidar systems, since two physical quantities, the particle backscatter and extinction coefficients, need to be determined by one measured quantity, i.e., the elastic lidar return (Ansmann and Müller, 2005). On the other hand, with the Raman lidar method (Ansmann et al., 1992) the backscatter and extinction coefficients can be determined independently. In addition, spectral information provided by multiwavelength lidars advance aerosol typing and can be used to derive microphysical particle properties (via inverse modeling).
Since the 1990s, the Leibniz Institute for Tropospheric Research (TROPOS) has conducted extensive research on the topic of lidar and aerosols. Two complex lidar systems named MARTHA (Multiwavelength Atmospheric Raman Lidar for Temperature, Humidity, and Aerosol) and BERTHA (Backscatter Extinction lidar-Ratio Temperature Humidity profiling Apparatus) have been developed using several different lidar-specific techniques (Raman, polarization, multi-wavelength, high-spectral-resolution, etc.) throughout the years. The mobile container-based BERTHA (Althausen et al., 2000) has been deployed in several field campaigns since the end of the 1990s (e.g., LACE98: Ansmann et al., 2002b; ACE-2: Ansmann et al., 2002a; INDOEX: Ansmann et al., 2000; COPS: Herold et al., 2011; SAMUM-1 and SAMUM-2: Ansmann et al., 2011; SALTRACE: Haarig et al., 2017a), while the lab-based MARTHA (Mattis et al., 2002a) was used for EARLINET observations (Mattis et al., 2004, 2008) and testing new methodologies (e.g., Mattis et al., 2002a; Schmidt et al., 2013; Jimenez et al., 2020a). In parallel, novel data retrieval techniques have been developed and steadily improved, which have become state-of-the-art for active aerosol profiling (Ansmann and Müller, 2005), e.g., inversion techniques (Müller et al., 1998), separation of aerosol components with polarization (POLIPHON; Mamouri and Ansmann, 2016), and automatic and unsupervised data retrievals (e.g., Baars et al., 2008, 2016; D'Amico et al., 2015; Baars and Yin, 2020; Yin and Baars, 2021). The intensive work on the inversion technique methodology (e.g., Müller et al., 1999a, b, 2000, 2011) led finally to the conclusion that 3+2 lidars (backscatter coefficient at three wavelengths, extinction coefficient at two wavelengths) are needed as the ideal setup for the remote sensing of aerosol microphysical properties – a conclusion which is presently also applied in EARLINET and ACTRIS (Aerosol, Clouds and Trace Gases Research Infrastructure). The introduction and intense use of the polarization technique led to the possibilities of separating dust and non-dust components (Tesche et al., 2011a) and fine and coarse modes of dust (Mamouri and Ansmann, 2014), as well as even the retrieval of CCN (cloud condensation nuclei) and INP (ice nucleating particle) properties (Mamouri and Ansmann, 2015, 2016) – important parameters to investigate aerosol–cloud interactions. Also, these techniques are currently widely standardized and applied in ACTRIS and beyond. Based on the experience and expertise gathered in the 1990s and with the upcoming need for automated observations, in 2002, the first portable, remotely controlled, multiwavelength Raman polarization lidar system (Polly) was developed at TROPOS (Althausen et al., 2009; Engelmann et al., 2016). Since then, more than a dozen lidars of Polly/PollyXT type (more details in Sect. 2.2; Engelmann et al., 2016) have been constructed and are operating within the framework of a voluntary, scientific network called PollyNET (Baars et al., 2016). The mobility of PollyXT systems, as well as their automated and continuous observational capabilities, makes them ideal for deployment in remote places during measurement campaigns (e.g., the Amazon: Baars et al., 2012; China: Hänel et al., 2012; Heese et al., 2016; South Africa: Giannakaki et al., 2016; India: Komppula et al., 2012; and more recently in Cyprus: Ansmann et al., 2019; the Arctic: Engelmann et al., 2021; and Punta Arenas, Chile: Radenz et al., 2021a) and on research vessels, such as Sonne, Meteor (Rittmeister et al., 2017) and Polarstern (Kanitz et al., 2013a; Bohlmann et al., 2018).
Lidars have also been successfully deployed in space, aiming to contribute to the scientific efforts for atmospheric measurements on a global scale. For a remarkable duration of 16 years, CALIOP (Cloud-Aerosol Lidar with Orthogonal Polarization) on board NASA's CALIPSO (Cloud-Aerosol Lidar and Infrared Pathfinder Satellite Observations) has measured vertical profiles of attenuated backscatter at visible and near-infrared wavelengths, along with depolarization in the visible channel (Winker et al., 2009). However, as an elastic-backscatter lidar, CALIOP is not able to perform direct extinction measurements. To enable the retrieval of the backscatter and extinction coefficients from the attenuated backscatter signals, the lidar ratio needs to be assumed. Since the lidar ratio depends on the aerosol types present in the atmosphere, an aerosol-typing scheme was developed for CALIPSO (Omar et al., 2005, 2009; Kim et al., 2018).
In 2018, the European Space Agency (ESA) launched the wind lidar mission Aeolus (Stoffelen et al., 2005). The satellite is equipped with a 355 nm high-spectral-resolution lidar (HSRL), the Atmospheric Laser Doppler Instrument (ALADIN). ALADIN is the first wind lidar in space, and its HSRL capabilities provide also the first directly measured extinction profiles and aerosol optical properties from space as a spin-off product (Flament et al., 2021), as already successfully demonstrated in Baars et al. (2021). This is a great step towards the harmonization of multiple satellite instruments, especially in view of the upcoming Cloud, Aerosol and Radiation Explorer (EarthCARE) joint mission of ESA and the Japanese Aerospace Exploration Agency (JAXA), scheduled for launch in 2024.
EarthCARE's payload consists of four instruments: an atmospheric lidar (ATLID), a cloud profiling radar (CPR), a multi-spectral imager (MSI) and a broad-band radiometer (BBR) (Illingworth et al., 2015). ATLID is a 355 nm HSRL that will provide direct cloud and aerosol profile measurements of backscatter and extinction coefficients. Furthermore, ATLID is able to measure the depolarization ratio of the atmospheric particles – an ideal parameter for aerosol typing – as well as ice particle characteristics (Illingworth et al., 2015; do Carmo et al., 2021). The primary goal of EarthCARE is radiative closure, which is aimed to be achieved in a synergistic approach from the two active and two passive instruments. One key element for this goal is a proper aerosol-typing scheme to calculate the aerosol's radiative properties. For this purpose, the Hybrid End-To-End Aerosol Classification (HETEAC) model has been developed (Wandinger et al., 2016a, 2022). As the name indicates, the HETEAC model delivers the required theoretical description of aerosol microphysics that is consistent with experimentally derived optical properties (hybrid approach) to close the loop from observations and aerosol microphysics to radiative properties (end-to-end approach).
It is evident that global, vertically resolved observations from ground-based and spaceborne lidars need to be harmonized (i.e., spectral harmonization). The harmonization of, e.g., lidar-derived intensive optical properties would improve the consistency of the lidar data obtained by different lidar systems and would allow for comprehensive studies on the statistical relations between those properties. Harmonized datasets of intensive optical parameters would not only lead to the creation of robust aerosol-typing algorithms but also improve already existing ones.
Groß et al. (2012)Sicard et al. (2012)Kanitz (2012)Groß et al. (2011)Preißler et al. (2011)Kanitz et al. (2013a)Baars et al. (2016)Rittmeister et al. (2017)Kaduk (2017)Haarig et al. (2017a)Urbanneck (2018)Bohlmann et al. (2018)Szczepanik et al. (2021)Haarig et al. (2022)Hofer et al. (2020)Müller et al. (2007)Kaduk (2017)Urbanneck (2018)Filioglou et al. (2020)Müller et al. (2007)Baars (2011)Tesche (2011)Pereira et al. (2014)Giannakaki et al. (2016)Janicka et al. (2016)Haarig et al. (2018)Floutsi et al. (2021)Ohneiser et al. (2021)Haarig et al. (2018)Ohneiser et al. (2020)Groß et al. (2011)Tesche (2011)Kanitz et al. (2013a)Giannakaki et al. (2016)Kanitz et al. (2014b)Kaduk (2017)Rittmeister et al. (2017)Ansmann et al. (2005)Müller et al. (2007)Tesche et al. (2007)Komppula et al. (2012)Preißler et al. (2013)Hänel et al. (2012)Giannakaki et al. (2016)Heese et al. (2017)Kaduk (2017)Preißler et al. (2013)Janicka et al. (2016)Kaduk (2017)Rittmeister et al. (2017)Haarig et al. (2017b)Bohlmann et al. (2018)Groß et al. (2011)Kaduk (2017)Bohlmann et al. (2018)Rittmeister et al. (2017)Groß et al. (2011)Kaduk (2017)Bohlmann et al. (2018)Rittmeister et al. (2017)Müller et al. (2007)Table 1Overview of the lidar-derived optical properties of different aerosol types. The lidar ratio S is expressed in steradians (sr) and the particle linear depolarization δ in percent (mean values) along with the mean observational errors. References for each category are given in the right column. Measurements conducted in a field campaign are indicated with a number at the reference and explained in the footnote of the table and during a Polarstern or Meteor cruise with a bullet symbol (•), while the rest are from PollyNET/EARLINET stations. Measurements conducted with a non-TROPOS lidar are accompanied with a star symbol (⋆).
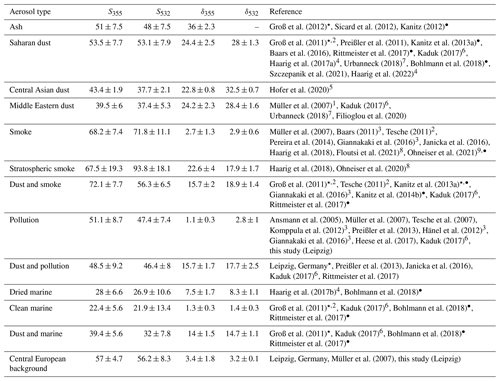
1 INDOEX, 2 SAMUM, 3 EUCAARI, 4 SALTRACE, 5 CADEX, 6 BACCHUS, 7 CyCARE and A-LIFE, 8 DACAPO-PESO, 9 MOSAiC.
Given the need for the development and improvement of aerosol-typing schemes, such as HETEAC, and data harmonization among lidar networks (i.e., MPLNET, AD-Net, LALINET, EARLINET and PollyNET) and satellites (e.g., CALIPSO and EarthCARE), in this paper, we present an experimental data collection of aerosol-type-dependent optical properties. The optical properties are the particle linear depolarization ratio, the lidar ratio and the Ångström exponent, and hence, the data collection is named DeLiAn. The optical properties have been obtained either by lidar systems that have been developed at TROPOS (such as MARTHA, BERTHA and PollyXT; more information in Sect. 2) or by other accompanying lidar systems (explicitly mentioned in Sect. 2) during different field campaigns and at different locations over many years (see Table 1 and Fig.1). In addition to the well-known aerosol types (marine, dust, pollution, etc.), DeLiAn features new findings and aerosol types (e.g., Central Asian dust: Hofer et al., 2020; dried marine: Haarig et al., 2017b; stratospheric smoke: Haarig et al., 2018; Ohneiser et al., 2020) that were identified during recent measurement campaigns. The aim is to provide additional knowledge on the intensive aerosol properties for different aerosol types and mixtures, which in turn can be used to constrain and enhance aerosol retrievals.
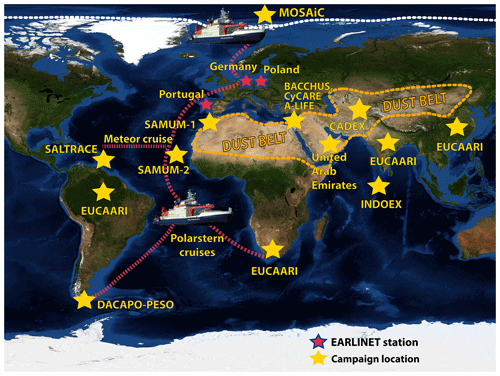
Figure 1Locations of measurement campaigns (yellow stars) or permanent EARLINET stations (red and blue stars) from which data for the current study were used. Map source: NASA Earth Observatory.
In the following section, we briefly present the lidar systems used and information about the data handling, and we provide an overview of the locations where the relevant lidar systems have been operated. In Sect. 3, the collection of the lidar-derived intensive optical parameters (DeLiAn) is presented and discussed in detail with respect to the different aerosol types and mixtures. The statistical analysis performed is also presented in the same section along with comparisons between the optical properties used for the CALIPSO aerosol-typing scheme and the respective ground-based ones that were obtained from the present study. The conclusions and an outlook finalize the paper.
2.1 Intensive optical properties
Multiwavelength polarization Raman lidars emit polarized light at different wavelengths (typically at 355, 532 and 1064 nm) into the atmosphere. The fraction of the light that gets backscattered by molecules and particles is detected by the instrument's receiver with a high temporal resolution. After the appropriate corrections (e.g., background subtraction, temporal averaging, overlap correction), the retrieved profiles of backscatter (β) and extinction (α) coefficients (typically at 355, 532 and 1064 nm for β and 355 and 532 nm for α) can be used to calculate the intensive, i.e., concentration-independent, particle optical properties. These parameters are sensitive to the size, shape and refractive index of the atmospheric particles and therefore can be used effectively for aerosol characterization (e.g., Ansmann and Müller, 2005; Müller et al., 2007; Freudenthaler et al., 2009; Groß et al., 2011).
2.1.1 Lidar ratio
The particle lidar ratio or simply lidar ratio (S), defined as the extinction-to-backscatter ratio,
is a valuable quantity for aerosol characterization. The lidar ratio reveals information regarding the size, shape and the absorption efficiency of the aerosol particles. The particle size and the lidar ratio are usually inversely related, while the particle absorption efficiency and the lidar ratio are directly related (i.e, S increases with particle absorption efficiency). When spherical and non-spherical particles have the same size range, the non-spherical ones tend to exhibit higher lidar ratio values (Müller et al., 2007). However, all these different effects could counterbalance each other, leading to similar lidar ratios for different aerosol types.
2.1.2 Particle linear depolarization ratio
As mentioned earlier, lidars emit polarized light, and during the backscatter process, parts of this light get unpolarized. The depolarization ratio (Schotland et al., 1971) usually refers to the ratio of signals measured in the perpendicular and parallel receiver channels (cross-polarized and co-polarized, respectively). Cross-polarized (⟂) refers to light with a state of polarization that is perpendicular to the one of the emitted laser light, and co-polarized (∥) refers to light that has the same state of polarization as the emitted laser light. The linear volume depolarization ratio (δvol) comprises contributions of molecules and aerosol particles (subscripted as m and p, respectively) and is defined as
The molecules and particles can be considered independently, leading to the molecular (δm) and particle (δp) linear depolarization ratios, respectively:
The particle linear depolarization ratio (δp or often denoted as δ) is an intensive property of the ensemble of scattering particles and can be used for aerosol typing because spherical particles do not alter the state of polarization of the emitted light (δp≈0), while non-spherical particles do (δp>0).
2.1.3 Ångström exponent
The Ångström exponent (Å) is a parameter that contains information regarding the spectral dependency of the aerosol optical properties (Ansmann and Müller, 2005) and can be obtained from, e.g., multiwavelength lidars. An Ångström exponent of zero denotes the wavelength independence of the investigated quantity. The Ångström exponent can be calculated for the backscatter coefficient (β), the extinction coefficient (α) and the lidar ratio (S) when they are available at least for two distinctive wavelengths λ1 and λ2:
where σ is an observable (Ansmann et al., 2002a). In general, the extinction-related Ångström exponent is around zero for large particles, while scattering by small particles exhibits a strong wavelength dependence and causes Å values greater than unity (Eck et al., 1999). The extinction- and backscatter-related Ångström exponents act as a proxy for the size of the particles, and thus, a separation between large and small particles can be achieved.
2.2 Lidar systems
2.2.1 MARTHA
The Multiwavelength Atmospheric Raman Lidar for Temperature, Humidity, and Aerosol (MARTHA) profiling is a lab-based lidar of TROPOS that has been used not only for acquiring cloud and aerosol measurements but also for testing new methodologies (Mattis et al., 2002a; Schmidt et al., 2013; Jimenez et al., 2019, 2020a). MARTHA's powerful laser, together with its large prime mirror (80 cm in diameter), makes it ideal for tropospheric and stratospheric aerosol observations, and it has recently been upgraded to a dual-receiver-field-of-view lidar (RFOV; Jimenez et al., 2020b). The operational setup has been steadily changed but covers at least (three backscatter coefficients at 355, 532 and 1064 nm; two extinction coefficients at 355 and 532 nm; and one depolarization ratio at 532 nm). MARTHA is part of EARLINET/ACTRIS (Pappalardo et al., 2014) but not yet automatized.
2.2.2 BERTHA
The Backscatter Extinction lidar-Ratio Temperature Humidity profiling Apparatus (BERTHA) is the oldest mobile, container-based multiwavelength polarization Raman lidar of TROPOS (Althausen et al., 2000). For 25 years, BERTHA has been providing extensive and intensive aerosol optical properties and has been deployed in numerous field campaigns (most notably in both the SAMUM (Saharan Mineral Dust Experiment) and SALTRACE (Saharan Aerosol Long-range Transport and Aerosol–Cloud interaction Experiment) field experiments; more details in Sect. 2.3). Manual operation is needed for this lidar. A distinctive feature of this lidar system is that, since 2012, it has enabled simultaneous measurements of the depolarization ratio at three wavelengths (355, 532 and 1064 nm). In 2015 the setup was extended by the first measurements of the extinction coefficient at 1064 nm, leading to a setup (three backscatter coefficients, three extinction coefficients and three depolarization ratios). The system includes a water vapor and a HSRL channel (407 and 532 nm, respectively). For a more detailed description of the latest setup of the lidar system, readers may refer to Haarig et al. (2016, 2017a).
2.2.3 PollyXT lidar systems
Since the first Polly system (Althausen et al., 2009) was assembled in 2002, more than a dozen lidars of the Polly/PollyXT type have been constructed at TROPOS, with continuous upgrading efforts (Engelmann et al., 2016), and deployed either permanently or temporarily in measurement campaigns and on research vessels (for visualization see the online map at https://polly.tropos.de/, last access: 20 March 2023). Some PollyXT lidars are part of EARLINET/ACTRIS (Pappalardo et al., 2014). PollyXT Raman lidars emit light at three different wavelengths, 355, 532 and 1064 nm. The lidars are nowadays equipped with a far-range receiver that consists of 12 or more channels which enable measurements of the elastically (355, 532 and 1064 nm) and Raman-scattered light (387 and 607 nm from nitrogen for aerosol extinction measurements and 407 nm from water vapor), as well as the depolarization state of the backscatter light (at 355 and 532 nm). A second near-range receiver allows the detection of scattered light (at 355, 387, 532 and 607 nm) from a lower altitude of around 60–80 m above ground level (a.g.l.) due to the laser-beam overlap with the receiving telescope. The lidar signals are recorded up to 46 km, but depending on the instrument performance (laser power, background light, etc.) the maximum height of useful signals varies, usually reaching at least up to 20 km. Data from all channels are acquired with a vertical resolution of 7.5 m and a temporal resolution of 30 s (for more details refer to Engelmann et al., 2016). The operational setup might vary from instrument to instrument but covers at least (three backscatter coefficients at 355, 532 and 1064 nm; two extinction coefficients at 355 and 532 nm; and two depolarization ratios at 355 and 532 nm). Quality assurance procedures are a key aspect for lidars (e.g., Bravo-Aranda et al., 2016; Wandinger et al., 2016b; Freudenthaler, 2016; Belegante et al., 2018; Freudenthaler et al., 2018). The PollyXT lidar systems and data processing follow the EARLINET standards even when the lidars are operated at non-stationary sites (e.g., on research vessels). Near-real-time (NRT) quicklooks can be found at https://polly.tropos.de/.
2.3 Lidar data analysis, locations and sources
The data collection presented here comprises various measurements from several locations, and an overview is given in Fig. 1. We have considered data that are already published (layer and observational mean values) and added additionally analyzed data for specific aerosol types. The evaluation of the published lidar data has been performed by the authors of the corresponding papers. Along with the determination of the intensive optical properties, which play a crucial role in the categorization of the observed particles, other tools such as back trajectories are also widely considered. Trajectory and particle dispersion models (e.g., HYSPLIT, FLEXPART; Stein et al., 2015; Pisso et al., 2019) provide valuable information about the source, the distance traveled and the destination of an air mass for a specific transport time (simulation performed either backward or forward in time). Recently, an automated air-mass source attribution tool which combines backward trajectories (or particle positions from a dispersion model) with geographical information (land cover classification), TRACE (Radenz et al., 2021b), was developed at TROPOS. The full list of the respective references can be found in Table 1, while a brief description of the major field campaigns mentioned in the same table is provided below.
Table 1 provides an overview of all the aerosol types that were considered during the creation of DeLiAn. It corroborates previous findings and provides new insights regarding aerosol types based on recent measurement campaigns and studies. Furthermore, the data collection is regularly updated, aiming to provide a comprehensive up-to-date collection of the optical properties of the different aerosol types at the typical lidar wavelengths.
The portable TROPOS lidar systems BERTHA and PollyXT have been deployed in numerous campaigns, on different research platforms and at various locations (Fig. 1). In the following, a brief introduction of major field campaigns and research vessels where TROPOS lidars have operated is presented. Measurements from the field campaigns listed below have contributed greatly to the collection presented here. Campaigns that contributed to the collection with a few measurements only are not listed here. In addition, the locations of all the campaigns relevant for this study and permanent measuring stations are indicated in Fig. 1.
2.3.1 Research cruises
Two German research vessels (RVs), namely Meteor and Polarstern, serve as research platforms on which PollyXT lidars have been deployed. The OCEANET-Atmosphere observatory, which includes a PollyXT lidar among other instruments, has been frequently operated on board Polarstern for the transects from the Northern Hemisphere to the Southern Hemisphere and vice versa (e.g., Kanitz et al., 2013b; Bohlmann et al., 2018; Yin et al., 2019), as well as in the polar regions (e.g., Griesche et al., 2020; Engelmann et al., 2021). Pure marine aerosol conditions, as well as complex mixtures typically including sea salt, i.e., dust and marine aerosol mixtures, are observed frequently. Data acquired during the transatlantic ship cruises and MOSAiC (Multidisciplinary drifting Observatory for the Study of Arctic Climate; more about that campaign in Sect. 2.3.9) have contributed greatly to the scientific efforts for aerosol monitoring by reaching places where no permanent stations can be established. Table 3 shows the identification sequences for the cruises relevant to this study.
2.3.2 SAMUM
The Saharan Mineral Dust Experiment (SAMUM) was focused on the investigation of the relationship between chemical composition, shape morphology, size distribution and optical effects of the dust particles originating from the Saharan desert (Ansmann et al., 2011). Two field campaigns were conducted in southern Morocco in 2006 and in Cabo Verde in 2008 (SAMUM-1 and SAMUM-2, respectively). The combination of the ground-based multiwavelength Raman lidar BERTHA, two lidar systems from the University of Munich (the portable lidar system – POLIS (Groß et al., 2008) and MULIS – multiple wavelength lidar system) and the airborne HSRL measurements (Falcon-20 research aircraft of the German Aerospace Center, DLR) allowed the profiling of pure dust optical properties, as well as mixed aerosol plumes, and provided a unique dataset that has been extensively used for radiation closure studies, the development of appropriate dust parameterizations for large-scale and regional weather and climate models, and in situ comparison studies (Tesche et al., 2009a, b, 2011a, b).
2.3.3 EUCAARI campaigns
The European Integrated Project on Aerosol Cloud Climate and Air Quality Interactions (EUCAARI; Kulmala et al., 2011) was a multidisciplinary project focused on the interactions between climate and air pollution. EUCAARI lasted for 3 years (2007–2010) and resulted in comprehensive datasets of aerosol properties from Europe and from four non-European countries (China, India, Brazil and South Africa). In the present study, measurements from all the aforementioned countries are included with a specific focus on measurements that were conducted in two EUCAARI campaigns in Amazonia and South Africa. A PollyXT lidar was deployed for the first time near Manaus, Brazil, from January to November 2008. The long-term lidar observations obtained during that campaign have advanced our knowledge on the vertical aerosol distribution of Saharan dust, biomass-burning aerosol (BBA) and their mixtures, which get advected from Africa, and helped us determine the aerosol conditions of Amazonia (Baars, 2011). In addition, a clear distinction between the prevailing aerosol conditions during the wet (January–June) and dry seasons (July–November) was achieved (Baars, 2011). A PollyXT lidar was also operated in Elandsfontein, South Africa, from 30 January 2010 to 31 January 2011. During that period, biomass-burning aerosol from natural phenomena (lightning) and human-induced activities and urban and industrial aerosol of anthropogenic origin were observed (Giannakaki et al., 2016). Measurements from the EUCAARI stations in China (Hänel et al., 2012) and India (Komppula et al., 2012) were heavily influenced by pollution-related aerosol. Complex mixtures of aged desert dust, biomass-burning smoke and industrial pollution were frequently observed.
2.3.4 SALTRACE
The Saharan Aerosol Long-range Transport and Aerosol–Cloud interaction Experiment (SALTRACE) was conducted from spring 2013 to summer 2014 in Barbados (Weinzierl et al., 2017). The campaign involved ground-based and airborne in situ and remote-sensing observations. The main goal of SALTRACE was the characterization of Saharan dust particles after long-range transport (Groß et al., 2015; Haarig et al., 2017a) and their interaction with clouds (Haarig et al., 2019) as a follow-on to the SAMUM field campaigns. TROPOS contributed with the deployment of the multiwavelength Raman lidar BERTHA (see Sect. 2) during the dusty summer conditions and the rather clear marine conditions in winter (Haarig et al., 2017a, b).
2.3.5 CADEX
The Central Asian Dust Experiment (CADEX; Hofer et al., 2017, 2020) was focused on long-term observations of the optical and microphysical properties of Central Asian mineral dust. It was the first time that ground-based lidar observations (performed with a PollyXT system in Dushanbe, Tajikistan) were conducted in Central Asia. CADEX lasted for 2 years (2014–2016, measurements conducted between 2015 and 2016) and resulted in major findings on optical properties of Central Asian dust, thus establishing it as a separate aerosol type. In addition, the campaign pointed out the necessity of a permanent ground-based station in the area, which was later on established as part of EARLINET. It is obvious that campaigns aiming at monitoring a specific aerosol type in a specific location where it is found in abundance are very important in the global efforts on aerosol-type standardization.
2.3.6 BACCHUS
BACCHUS (Impact of Biogenic versus Anthropogenic emissions on Clouds and Climate: towards a Holistic UnderStanding) was a European collaborative project led by ETH Zurich (https://www.bacchus-env.eu/, last access: 20 March 2023). In the framework of BACCHUS, a PollyXT lidar system performed measurements in Nicosia, Cyprus, in spring 2015. Pure aerosol types such as dust (Saharan and Middle Eastern), marine and pollution, as well as complex mixtures including smoke, were regularly observed (Kaduk, 2017).
2.3.7 CyCARE and A-LIFE
The Cyprus Clouds Aerosol and Rain Experiment (CyCARE; Ansmann et al., 2019) took place in Limassol, Cyprus, from October 2016 to March 2018 with its main focus on the complex aerosol mixtures, vertical aerosol layering, and their influence on cloud evolution and precipitation processes. PollyXT, part of LACROS (Leipzig Aerosol and Cloud Remote Observations System, the ground-based remote-sensing supersite of TROPOS; Bühl et al., 2013), was deployed in Limassol in 2017. During the deployment, the A-LIFE (absorbing aerosol layers in a changing climate: aging, lifetime and dynamics) campaign took place (April 2017), which was led by the University of Vienna. A-LIFE aimed to investigate the properties of absorbing aerosol and in particular those of mineral dust, black carbon and their mixtures (https://www.a-life.at/, last access: 20 March 2023). In parallel to the A-LIFE campaign, the PRE-TECT campaign took place at the Greek atmospheric observatory of Finokalia in Crete, Greece. The campaign was led by the National Observatory of Athens (NOA) and aimed to improve the desert dust characterization from remote-sensing measurements. For that reason, the PollyXT lidar system of the NOA was deployed in Finokalia.
2.3.8 DACAPO-PESO
The Dynamics, Aerosol, Clouds, And Precipitation Observations in the Pristine Environment of the Southern Ocean (DACAPO-PESO) field campaign took place in Punta Arenas, Chile, and it was focused on the investigation of cloud formation and aerosol–cloud interaction in environments of contrasting aerosol conditions (Radenz et al., 2021a). LACROS (Bühl et al., 2013) was measuring continuously for a period of 3 years (2018–2021), and with respect to aerosol, lofted aerosol layers were observed occasionally in the troposphere of Punta Arenas (Floutsi et al., 2021). Furthermore, stratospheric smoke originating from the record-breaking Australian bushfires in January 2020 was fully captured by the lidar observations (Ohneiser et al., 2020). However, the observations confirmed that in the general clean environment of Punta Arenas the aerosol backscatter is on average more than 30 % below the mean of the backscatter observed in Europe (Limassol and Leipzig) (Radenz et al., 2021a). In addition, it was found that in Punta Arenas most aerosol is confined in the boundary layer with pristine conditions dominating aloft (Radenz et al., 2021a).
2.3.9 MOSAiC
During the MOSAiC (Multidisciplinary drifting Observatory for the Study of Arctic Climate) expedition, a PollyXT multiwavelength polarization Raman lidar was operated on board the RV Polarstern from October 2019 to October 2020. For the first time, PollyXT conducted continuous measurements of aerosols and clouds (up to 30 km altitude) in the central Arctic (Engelmann et al., 2021). This unique dataset provided new insights about smoke trapped in the upper troposphere–lower stratosphere (UTLS) of the High Arctic in the winter of 2019–2020 (Ohneiser et al., 2021) and improved our knowledge on aerosol–cloud interactions (Engelmann et al., 2021).
2.3.10 EARLINET stations
Measurements from four permanent EARLINET stations have also significantly contributed to this study. The stations are mainly located in Europe: Leipzig (Germany), Évora (Portugal) and Warsaw (Poland). Pure aerosol types such as smoke, Saharan dust and pollution, as well as their complex mixtures, are frequently observed above the aforementioned stations. A non-European station located in Dushanbe (Tajikistan; see Sect. 2.3.5) was also considered.
3.1 Intensive optical properties at 355 and 532 nm
Two intensive aerosol optical properties from the collection of ground-based observations described above (Sect. 2.3), namely the lidar ratio and the particle linear depolarization ratio at 355 nm, are contrasted against each other for several aerosol types and mixtures in Fig. 2. The aforementioned intensive properties exhibit the highest discriminatory power (e.g., as demonstrated in Burton et al., 2012). The dataset used for the conceptualization of the aerosol-typing-related activities of EarthCARE (Wandinger et al., 2016a; Illingworth et al., 2015) has been merged with the present dataset and is shown as semi-transparent symbols. In addition to the intensive optical properties at 355 nm, in Fig. 3 we present the values for the same aerosol types at 532 nm. Furthermore, we show the wavelength dependence, i.e., the Ångström exponent contrasted with the lidar ratio and particle linear depolarization ratio, as presented in Fig. 4. Having the intensive properties at 355 and 532 nm not only allows the use of the spectral dependence for typing purposes but also facilitates the bridging of datasets obtained at one of these wavelengths only as, e.g., in the case of the spaceborne lidars CALIOP (532 nm) and ALADIN and ATLID (355 nm) or in the case of spaceborne and ground-based lidars (Amiridis et al., 2015).
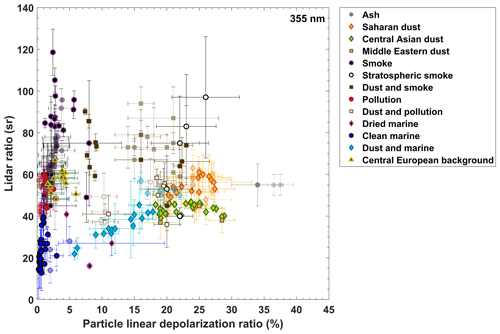
Figure 2Intensive optical properties of different aerosol types, measured at 355 nm. The dataset used for the conceptualization of EarthCARE's typing scheme is shown with faded markers (see Fig. 8 in Illingworth et al., 2015).
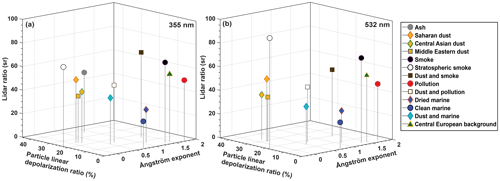
Figure 4Mean lidar ratio and particle linear depolarization ratio at (a) 355 nm and (b) 532 nm versus the mean extinction-related Ångström exponent for the 13 aerosol categories.
3.1.1 Ash
The volcanic ash category contains measurements of fresh mineral particles from the Eyjafjallajökull eruption (April 2010) observed at various locations, including Maisach, Germany, with POLIS (faded grey circles in Fig. 2; Groß et al., 2012); near Bremerhaven, Germany, conducted on board the RV Polarstern (at 532 nm; Kanitz, 2012); and Évora, Portugal (no depolarization information available; Sicard et al., 2012). The mean lidar ratio and particle linear depolarization ratio at 355 and 532 nm and the mean Ångström exponents are reported in Tables 1 and 2, respectively.
Table 2Mean values of the extinction- () and backscatter-related ( and ) Ångström exponents along with the mean observational error for the aerosol categories listed in Table 1.
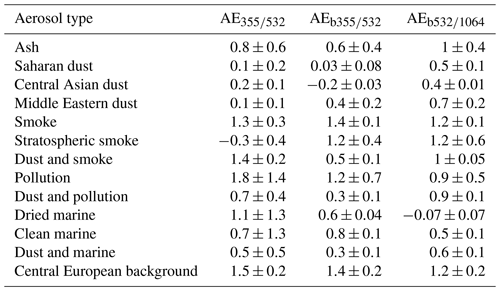
3.1.2 Desert dust
Mineral dust is an important constituent of the atmospheric aerosol load, and in DeLiAn three aerosol categories have been dedicated for this specific aerosol type. Saharan dust has been targeted in many field campaigns and is the first of the three aforementioned categories (orange and red rhombuses in Fig. 2). Saharan dust is frequently advected above Europe, and observations over Portugal (Preißler et al., 2011), Warsaw (Szczepanik et al., 2021) and Leipzig (Baars et al., 2016) have been included in the data collection. Long-range transported Saharan dust observed over Barbados during SALTRACE in 2013 and 2014 was also considered in the collection (Haarig et al., 2017a, 2022). The island of Cyprus, located in the eastern part of the Mediterranean Basin, is frequently affected by Saharan dust plumes, and therefore, dust measurements from Limassol (CyCARE and A-LIFE; Urbanneck, 2018) and from Nicosia (BACCHUS; Kaduk, 2017) were used. Saharan dust has also been observed from on board the RV Polarstern over the Atlantic Ocean (faded orange and red rhombuses in Fig. 2; Kanitz et al., 2013a) and near the Canary Islands (Bohlmann et al., 2018), as well as from on board the RV Meteor during a cruise from Guadeloupe to Cabo Verde (Rittmeister et al., 2017). Measurements at 355 nm conducted with POLIS during the SAMUM-2 campaign in Cabo Verde in 2008 were also considered (faded orange and red rhombuses in Fig. 2; Groß et al., 2011). At the current state of the experimental data collection, the representative mean lidar ratio and particle linear depolarization values at 355 nm are 53.5±7.7 sr and 24.4±2.5 %, respectively. Optical information at wavelengths beyond EarthCARE's wavelength (i.e., 355 nm) is presented in Fig. 3 (532 nm), and the Ångström exponents are shown in Fig. 4. At 532 nm, the mean lidar ratio is 53.1±7.9 sr and the mean particle linear depolarization ratio is 28±1.3 %. Mean values of the extinction-related Ångström exponent are 0.1±0.2, and the backscatter-related Ångström exponent values at both 355/532 and 532/1064 nm are around 0.03±0.08 and 0.5±0.1, respectively (Table 2 and Fig. 4).
In contrast to the findings from the major dust-targeting campaigns, SAMUM-1 and SAMUM-2 and SALTRACE, in recent years it was confirmed that Central Asian dust has different optical properties than Saharan dust. Mineral dust that originates from the Central Asian deserts typically exhibits lower lidar ratio values (35–45 sr) than Saharan dust (50–60 sr), as shown by Hofer et al. (2017) on the basis of long-term observations in Tajikistan, and, hence, has been introduced to DeLiAn as a separate aerosol type (green and yellow rhombuses in Fig. 2). The Central Asian dust measurements were conducted in the framework of the CADEX field campaign in Dushanbe, Tajikistan (Hofer et al., 2017, 2020). Mean lidar ratio values for this aerosol category are 43.4±1.9 and 37.7±2.1 sr at 355 and 532 nm, respectively. The mean particle linear depolarization ratios are 22.8±0.8 % and 32.5±0.7 % at 355 and 532 nm, respectively. Mean values of the extinction-related Ångström exponent are similar to those of Saharan dust (0.2±0.1), while the mean backscatter-related Ångström exponent values are and 0.4±0.01 at the wavelength pairs of 355/532 and 532/1064 nm, respectively.
Since the Eastern Mediterranean region has been characterized as a primary climate change “hot spot” (Lelieveld et al., 2012), atmospheric measurements and campaigns have been intensified over the region. Complex mixtures of desert dust, biomass-burning and pollution aerosol are usually encountered. Typically, dust particles observed above that region originate from either the Sahara or the Middle Eastern deserts. Therefore, in addition to the Saharan and Central Asian dust categories we have introduced a new aerosol type: Middle Eastern dust. At the moment, this aerosol category (brown and yellow squares in Fig. 2) comprises dust originating from Saudi Arabia (Müller et al., 2007) and was observed within the framework of the Indian Ocean Experiment (INDOEX; Ramanathan et al., 2001) from the United Arab Emirates (Filioglou et al., 2020) and mineral dust measurements from Cyprus, specifically in Limassol (CyCARE and A-LIFE; Urbanneck, 2018) and in Nicosia (BACCHUS; Kaduk, 2017). On average, the lidar ratios at 355 and 532 nm are 39.5±6 and 37.4±5.3 sr and the particle linear depolarization ratios are 24.2±2.3 % and 28.4±1.6 %, respectively. The extinction- and backscatter-related Ångström exponents are 0.1±0.1, 0.4±0.2 (355/532 nm) and 0.7±0.2 (532/1064 nm), respectively.
3.1.3 Smoke
The smoke category used for the conceptualization of EarthCARE's classification approach is based on measurements of smoke that were conducted during a EUCAARI campaign in the Amazon Basin in 2008 (Baars et al., 2012). At the time of the campaign, measurements of lidar ratio and particle linear depolarization ratio were usually conducted at 532 nm, while measurements at 355 nm (especially of particle linear depolarization ratio) were only occasionally performed (depicted with faded black and purple circles in Fig. 2). Since then, 355 nm lidar measurements have become much more available, and therefore, fresh and aged smoke observations from other locations and fire types (e.g., smoldering or flaming combustion) have been observed too (depicted by black and purple circles in Fig. 2). Smoke observations from another EUCAARI campaign, this time from Elandsfontein, South Africa (Giannakaki et al., 2016), have been included in the data collection. Similarly, smoke measurements performed in the framework of SAMUM-2 in Cabo Verde (Tesche, 2011) were added. Regional smoke and smoke from Australia were observed on the southern tip of South America (Punta Arenas, Chile) during the DACAPO-PESO field campaign (Floutsi et al., 2021). Smoke from Siberian wildfires, which was measured in the Arctic during the MOSAiC campaign, has also been added to the data collection (Ohneiser et al., 2021), which broadens even further the geographical coverage of the observations of that particular aerosol type. Smoke has also been observed above Europe frequently: from boreal forest fires in western Canada in Leipzig, Germany (aged; Haarig et al., 2018); from Siberia and Canada measured at multiple EARLINET stations (aged; Müller et al., 2007); from North America above Warsaw, Poland (aged; Janicka et al., 2016, 2017); and fresh, locally produced smoke above Portugal (Pereira et al., 2014). The 355 nm mean lidar ratio and particle linear depolarization ratio are 68.2±7.4 sr and 2.7±1.3 %, respectively. At 532 nm, the mean smoke lidar ratio is 71.8±11.1 sr and, thus, higher and more widely distributed compared to the 355 nm mean lidar ratio. The mean smoke particle linear depolarization ratio at the same wavelength is 2.9±0.6 %. Extinction-related Ångström exponent values are on average around 1.3±0.3, and backscatter-related Ångström exponent values are 1.4±0.1 and 1.2±0.1 for the wavelength pairs of 355/532 and 532/1064 nm.
3.1.4 Stratospheric smoke
The different properties of smoke in the troposphere and in the stratosphere resulting from pyrocumulonimbus convection were not realized before the intense Canadian wildfires of 2017 and were first studied by Haarig et al. (2018) and Ansmann et al. (2018), as well as by Baars et al. (2019) for the event's long-term evolution over Europe based on EARLINET measurements. In contrast to tropospheric smoke, which contains mainly spherical particles, stratospheric smoke consists of non-spherical soot particles, yielding high depolarization ratios (at both 355 and 532 nm), a feature that was first observed in an elevated aged smoke layer in the upper troposphere on the eastern seaboard of the United States with an HSRL on board the NASA B200 aircraft by Burton et al. (2015). The enhanced depolarization ratios, along with the characteristic lidar ratio wavelength dependence of the stratospheric smoke, were recently modeled by Gialitaki et al. (2020). In this data collection, the stratospheric smoke category (depicted by black and white circles in Fig. 2) includes the aforementioned Canadian wildfires' stratospheric smoke measurements (Haarig et al., 2018) and observations from the record-breaking Australian wildfires of January 2020 measured in Punta Arenas during DACAPO-PESO (Ohneiser et al., 2020). Mean lidar ratios for this aerosol category are 67.5±19.3 and 93.8±18.1 sr at 355 and 532 nm, respectively, and, thus, significantly higher, on average, than for tropospheric smoke. Mean particle linear depolarization ratios are 22.6±4 % and 17.9±1.7 %. This is a significant finding, as depolarizing aerosol particles in the stratosphere were usually attributed to volcanic origin (or generically classified as “stratospheric features” in the version 3 CALIPSO data; Kim et al., 2018). However, the classification of stratospheric smoke layers during nighttime appears to be improved after the introduction of the CALIPSO version 4.5 stratospheric aerosol subtyping algorithm (Tackett et al., 2023). With these new findings it might be possible to distinguish stratospheric aerosol in more detail, and new findings may resurface from historic datasets.
3.1.5 Pollution
The pollution category includes measurements that have been conducted in cities of Europe, Asia and Africa. In particular, the category contains pollution measurements from multiple EARLINET stations in Europe (Müller et al., 2007), including the urban EARLINET station in Leipzig, from Évora (Preißler et al., 2013), from Nicosia (BACCHUS; Kaduk, 2017), from Elandsfontein (EUCAARI; Giannakaki et al., 2016), from China (more specifically in Xinken: Ansmann et al., 2005; in Beijing: Tesche et al., 2007; in Shangdianzi: Hänel et al., 2012; and in Guangzhou, in the framework of the German project “Megacities–Megachallenges – Informal Dynamics of Global Change”: Heese et al., 2017) and from India (Gual Pahari near New Delhi; Komppula et al., 2012). The mean 355 nm lidar ratio and particle linear depolarization ratio are 51.1±8.7 sr and 1.1±0.3 %, respectively. The corresponding properties for 532 nm are 47.4±7.4 sr and 2.8±1 %. Ångström exponents are reported in Table 2 and reflect the small size of the specific aerosol type.
3.1.6 Marine
The clean marine category (depicted with blue and black circles in Fig. 2) contains measurements of marine particles (i.e., sea salt) that were conducted mostly on board a RV or at a coastal station. Measurements conducted during two Polarstern cruises (PS95 from Bremerhaven, Germany, to Cape Town, Republic of South Africa, and PS98 from Punta Arenas to Bremerhaven; Bohlmann et al., 2018); during a Meteor cruise (M96 from Guadeloupe to Cabo Verde; Rittmeister et al., 2017); in Nicosia, Cyprus (Kaduk, 2017); and in Cabo Verde (faded blue and black circles in Fig. 2; SAMUM-2; Groß et al., 2011) have been included in the collection. At 355 nm the mean lidar ratio for this aerosol category is 22.4±5.6 sr, while the mean particle linear depolarization ratio is 1.3±0.3 %. At 532 nm, the mean lidar ratio is 21.9±13.4 sr and the mean particle linear depolarization ratio is 1.4±0.3 %, both indicating a very weak wavelength dependency. Ångström exponent values are on average 0.7±1.3 for the extinction-related and 0.8±0.1 and 0.5±0.1 for the backscatter-related exponents (355/532 and 532/1064 nm, respectively).
The optical properties of aerosol of marine origin do not show variability with respect to the source, since they are usually observed in environments of high relative humidity (typically 60 %–80 %), and the particles have a spherical shape due to water uptake (Haarig et al., 2017b; Thomas et al., 2022). However, in rarer cases, when marine particles are exposed to very dry atmospheric conditions, typically with relative humidity lower than 45 %, they adopt a cubic-like shape due to the sodium chloride contained in the sea salt aerosol (Zieger et al., 2017). The cubic-like shape of the particles causes significantly higher particle linear depolarization ratios (Haarig et al., 2017b; Bohlmann et al., 2018). This fact was not realized earlier, e.g., in Illingworth et al. (2015) or in aerosol-typing schemes such as the one of CALIPSO (Omar et al., 2005; Kim et al., 2018), and may have led to the misclassification of this specific type, e.g., as mixture containing depolarizing mineral dust particles. Therefore, the introduction of the dried marine aerosol as a separate category was essential. The category includes measurements conducted in Barbados (2014) during SALTRACE (Haarig et al., 2017b) and above the Atlantic Ocean with the RV Polarstern (Bohlmann et al., 2018), and dried marine aerosol is always observed on top of the local marine boundary layer. Measurements of dried marine particles are sparse, leading to only three measurements in this category. Therefore, the statistics presented here should be interpreted with caution. Dried marine particles exhibit on average lidar ratios of 28±6.6 and 26.9±10.6 sr and particle linear depolarization ratios of 7.5±1.7 % and 8.3±1.1 % at 355 and 532 nm, respectively. The Ångström exponent values are presented in Table 2.
3.1.7 Central European background
While optically similar to the pollution aerosol category, the “Central European background” aerosol type has been introduced to the collection, given the plethora of permanent ground-based stations in the indicated geographical area and its resemblance to the more generalized aerosol category of clean continental in the CALIPSO typing scheme. An aerosol layer must follow certain criteria to be categorized as Central European background aerosol, which include the absence of advection of aerosol, the confinement of the particles within the planetary boundary layer and an optical thickness of less than 0.2. In this way, both Central European background and pollution categories can be separated, even though they both contain mainly aerosol of anthropogenic origin. The Central European background category (depicted with yellow and green triangles in Fig. 2) includes measurements of the background aerosol load in a typical Central European region (background aerosol measurements performed in Leipzig and from multiple EARLINET stations, as adapted from Müller et al., 2007). Overall, this aerosol category exhibits slightly higher lidar ratios and particle linear depolarization ratios than pollution (Tables 1 and 2).
3.1.8 Mixtures
Apart from pure aerosol types, aerosol mixtures of dust particles with smoke, pollution and marine particles have been considered in DeLiAn. The determination of the main aerosol types present in an aerosol mixture (performed by the authors of the respective studies) was based on combined information on the intensive optical properties of the aerosol layers and air-mass analysis with the help of trajectory or particle dispersion modeling.
Dust and smoke mixtures from Cabo Verde (SAMUM-2; Groß et al., 2011) and from measurements above the Atlantic Ocean (RV Polarstern; Kanitz et al., 2013a) served as the starting point for the conceptualization of HETEAC (faded brown squares in Fig. 2). Additional measurements from Cabo Verde (SAMUM-2; Tesche, 2011), from the Atlantic (conducted while on board the RV Polarstern, near Cabo Verde and in the Caribbean: Kanitz et al., 2014b; while on board the RV Meteor: Rittmeister et al., 2017), in Elandsfontein (EUCAARI; Giannakaki et al., 2016) and in Nicosia (BACCHUS, Kaduk, 2017) were added to the data collection. The mean lidar ratio and particle linear depolarization ratio for dust-and-smoke mixtures at 355 nm are 72.1±7.7 sr and 15.7±2 %, respectively. The same parameters for the newly added 532 nm wavelength are 56.3±6.5 sr and 18.9±1.4 %, respectively. Extinction- and backscatter-related Ångström exponents vary with wavelength, reflecting the versatile particle size range of the mixture (exact values in Table 2).
Mixtures of dust and marine particles (cyan and blue rhombuses in Fig. 2) are frequently observed in the lowermost atmosphere, especially in coastal stations such as in Cabo Verde (faded cyan and blue rhombuses in Fig. 2; SAMUM-2; Groß et al., 2011) and in Nicosia, Cyprus (Kaduk, 2017). Dust and marine mixtures are also frequently observed with the RV Polarstern (Bohlmann et al., 2018) and the RV Meteor (Rittmeister et al., 2017). The 355 nm mean lidar ratio and particle linear depolarization ratio are 39.4±5.6 sr and 14±1.5 %, respectively. Accordingly, they are 32±7.8 sr and 14.7±1.1 % for the 532 nm wavelength. The mean extinction-related Ångström exponent is 0.5±0.5, and the mean backscatter-related Ångström exponents are 0.3±0.1 and 0.6±0.1 for the wavelength pairs of 355/532 and 532/1064 nm, respectively.
Mixtures of dust and pollution (brown and white squares in Fig. 2) have been observed at Évora (Preißler et al., 2013), Warsaw (Janicka et al., 2016, 2017), Nicosia (Kaduk, 2017) and while on board the RV Meteor above the Atlantic Ocean (Rittmeister et al., 2017). The mean lidar ratios for this category are 48.5±9.2 and 46.4±8 sr, and the particle linear depolarization ratios are 15.7±1.7 % and 17.7±2.5 % at 355 and 532 nm, respectively. The mean extinction- and backscatter-related (355/532 and 532/1064 nm) Ångström exponents are 0.7±0.4, 0.3±0.1 and 0.9±0.1, respectively.
3.2 Statistical analysis of intensive optical properties
The main findings of this study are summarized in Fig. 4, which depicts the mean values of lidar ratio and particle linear depolarization ratio at (a) 355 nm and (b) 532 nm, respectively, against the mean extinction-related Ångström exponent for the different aerosol categories of Table 1. Incorporating the Ångström exponent as a third dimension results in more distinctive aerosol categories (in comparison to Figs. 2 and 3). For instance, the “Central European background” aerosol category can be now clearly distinguished from the pollution category as it exhibits higher extinction-related Ångström exponent values. The respective 2D plots, including the backscatter-related Ångström exponents, are shown in Appendix A (Figs. A1 and A2).
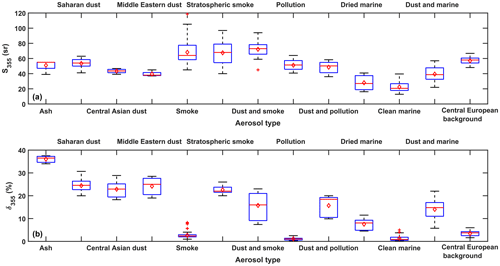
Figure 5Statistics of the lidar ratio (a) and the particle linear depolarization ratio (b) at 355 nm for the 13 aerosol categories. The minimum and maximum values are indicated by the lower and upper whiskers and median and mean values by the red lines and rhombuses, respectively. The lower part of each box indicates the 25 % percentile and the upper part the 75 % percentile. Red crosses represent the outliers.
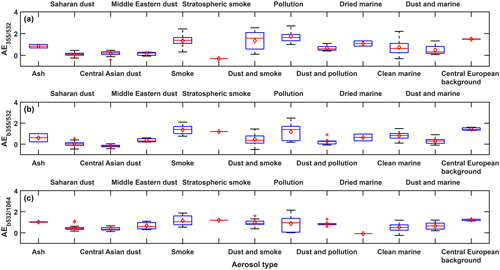
Figure 7Statistics of the extinction-related (a), the 355/532 nm backscatter-related (b) and the 532/1064 nm backscatter-related (c) Ångström exponents for the 13 aerosol categories.
A statistical analysis of the intensive optical properties was performed for the 13 different aerosol categories. It should be noted that, since the data for each aerosol category have been collected from various sources (see Table 1), they naturally exhibit a variation in the number of data points per aerosol category. In addition, the measurements were performed by lidar systems with different capabilities, and therefore, not all intensive optical parameters were always available for all the observations (e.g., lidar ratio available only at one wavelength or information on depolarization completely missing). Nevertheless, all available measurements were considered for the creation of DeLiAn and the statistical analysis presented here, and the number of data points used are listed in Table B1. The statistics for the lidar ratio and the particle linear depolarization ratio are presented in the form of boxplots in Figs. 5 and 6 for the 355 nm and 532 nm wavelengths, respectively. The statistics for all the Ångström exponents are presented in Fig. 7. The minimum and maximum values are indicated by the lower and upper whiskers and the median and mean values by the red lines and rhombuses, respectively. The lower part of each box indicates the 25 % percentile and the upper part the 75 % percentile. Red crosses represent the outliers (values greater than 1.5 times the interquartile range).
Comparison with CALIPSO aerosol subtypes
Statistics on the aerosol-type-separated optical properties can be used not only for the development of typing schemes but also to consolidate already existing aerosol classification schemes, such as the typing scheme of CALIPSO (Omar et al., 2009; Kim et al., 2018). In Fig. 8, the lidar ratio (532 nm) assumed for the different aerosol types in the CALIPSO scheme is contrasted with the observations from the different ground-based lidars (Table 1). The CALIPSO lidar ratios for the different aerosol subtypes are adapted from Table 2 of Kim et al. (2018) (version 4). The stratospheric aerosol subtypes have been adapted from the latest version (4.5) (Tackett et al., 2023). The version 4.5 stratospheric aerosol subtypes of “polar stratospheric aerosol”, “sulfate” and “unclassified” are not included in the comparison, as there is no respective category in DeLiAn yet.
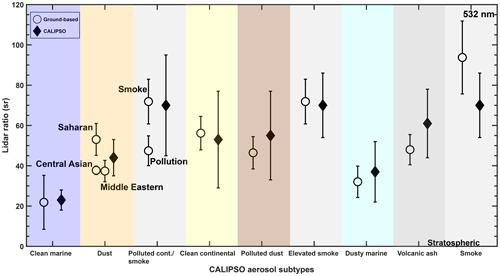
Figure 8Comparison of the 532 nm lidar ratio as derived from ground-based lidars (white circles; this study) and from the CALIPSO aerosol subtypes (black rhombuses; Kim et al., 2018). The newest CALIPSO data version (4.5) was used for the stratospheric aerosol subtypes (Tackett et al., 2023).
The assumptions of the lidar ratio of several aerosol subtypes appear to be in good agreement with the ground-based observations, e.g., for “clean marine” mainly due to an overlap in the data sources (Kim et al., 2018). Other aerosol subtype categories such as “clean continental”, “polluted dust”, “elevated smoke”, “volcanic ash” and “dusty marine” agree well within the allowed variability, which is rather large for the CALIPSO data. The large variability in the assigned lidar ratio values in the aforementioned aerosol subtypes can potentially lead to large uncertainties in the retrieved extinction profiles. Kanitz et al. (2014a) had shown that for the version 3 CALIPSO data, the surface-dependent aerosol typing was not allowing for a correct classification of marine aerosol over land, and recently Ansmann et al. (2021) showed that stratospheric smoke layers are misclassified as sulfate aerosol layers (version 4 CALIPSO data). The updated stratospheric lidar ratios in version 4.5 CALIPSO data appear to improve the classification accuracy (Tackett et al., 2023). The present collection could therefore be utilized to reduce the aerosol-subtype-related variability linked to the 532 nm lidar ratio. Three CALIPSO subtypes, namely “dust”, “polluted continental/smoke” and “smoke” (stratospheric), seem to be problematic with respect to the present ground-based data collection. “Dust” has a lidar ratio of 44±9 sr, which is lower than what we observed for Saharan dust and higher than Central Asian and Middle Eastern dust. An increase in the lidar ratio variability in the specific aerosol subtype or a geographical-specific constraint would therefore be suggested to cover all the observations. The “polluted continental/smoke” subtype has the highest variability, and the nomenclature itself indicates that several aerosol types can be assigned under that category. With the current allowed variability, this category includes both the smoke and pollution categories of the present study and is therefore correct. However, we would suggest the splitting of this subtype to allow a better discrimination in the radiative effects. Finally, the stratospheric smoke observations (see Table 1) suggest that the 532 nm lidar ratio of stratospheric smoke is higher than the one considered in the CALIPSO version 4.5 “smoke” subtype (stratospheric).
A collection of intensive optical properties (DeLiAn) for various aerosol types based mainly on ground-based lidar observations was presented in this paper. DeLiAn merges measurements from old and more recent campaigns (large temporal coverage) and from various locations (large spatial coverage) and brings together aerosol types that were previously disregarded (e.g., dried marine particles, stratospheric smoke). The optical properties are presented at two wavelengths, 355 and 532 nm, and therefore can be widely used for aerosol-typing purposes, covering spaceborne lidars (CALIOP, ATLID) and ground-based lidar networks (e.g., MPLNET, EARLINET, AD-NET).
The presented statistics cover the most frequently observed aerosol types, i.e., smoke, marine, pollution, dust and complex mixtures that they create, as well as occasionally observed aerosol types, e.g., dried marine particles. Such statistics can have multiple usages and are needed for aerosol classification purposes and aerosol-typing schemes since they can be utilized as thresholds separating the different aerosol categories or as a priori information. DeLiAn has been used for the development of both HETEAC (Wandinger et al., 2016a, 2022) and HETEAC-Flex, a novel aerosol-typing approach (Floutsi et al., 2019) which will homogenize aerosol typing from EarthCARE but also ground-based lidar systems with different capabilities and which will serve as a ground-based validation scheme for EarthCARE. The data collection of the different intensive optical properties can also be used for further improvement of already existing aerosol classification schemes, for instance as shown in the case of CALIPSO, where more accurate lidar ratios can lead to better extinction retrievals. Thus, DeLiAn could be considered by the MIRA (Models, In situ, and Remote sensing of Aerosols) working group in their efforts for the creation of an updated CALIPSO lidar ratio climatology (version 5; Schuster et al., 2022).
The presented data collection is only the beginning of a bigger effort for creating an aerosol climatology of intensive optical properties based on ground-based lidar measurements. Measurements from other permanent PollyNET stations are planned to be incorporated in DeLiAn to include geographical regions with interesting aerosol conditions which are currently underrepresented. For instance, measurements from the Eastern Mediterranean region would significantly enrich many aerosol categories such as marine, pollution, dust and their complex mixtures. Heese et al. (2022) recently presented a 2-year-long lidar dataset from the coastal city of Haifa, Israel. It was found that most of the observed aerosol layers were aerosol mixtures. Even though a seasonal air-mass source attribution was performed by the authors using TRACE (Radenz et al., 2021b), a more detailed case-by-case air-mass source attribution needs to be performed before the data are included in the data collection.
Data from recent measurement campaigns are also planned to be included in DeLiAn, after data collection and processing is complete. Since the beginning of the ASKOS experiment (Amiridis and the ASKOS team, 2022) in the summer of 2021, a PollyXT Raman lidar has been fully operational in Mindelo, Cabo Verde. Clean marine, mineral dust and the complex mixtures that they form have been observed regularly. Dried marine particles have also been observed often, typically on the top of the marine boundary layer.
In a recent study, Haarig et al. (2022) provided the first-ever lidar measurements of the lidar ratio and particle linear depolarization ratio for desert dust particles at all three lidar wavelengths (355, 532 and 1064 nm). As these measurements become more and more available, DeLiAn ought to be updated accordingly, since spectrally resolved information including 1064 nm is important for aerosol-typing purposes.
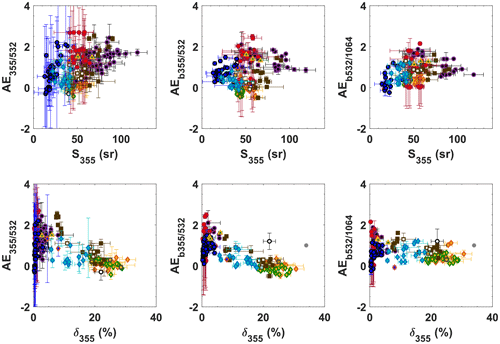
Figure A1The 355 nm lidar ratio (S) and particle linear depolarization ratio (δ) against the extinction- () and backscatter-related () Ångström exponent. The figure legend is the same as in Fig. 2.
The data collection is available at https://doi.org/10.5281/zenodo.7751752 (Floutsi et al., 2023).
The paper was conceptualized and written by AAF and HB. AAF collected and visualized the data and drafted the manuscript. UW provided guidance throughout the study. DA, JH and SFA were involved in the CADEX campaign; AA in the SAMUM-1 and SAMUM-2 campaigns; PS, MR and BB in the DACAPO-PESO campaign; MH in the SALTRACE campaign; and HB, EG and MK in the EUCAARI campaign. MH and REM were involved in the CyCARE and A-LIFE campaigns; EM and VA in the BACCHUS, CyCARE, A-LIFE and PRE-TECT campaigns; and AG in the A-LIFE and PRE-TECT campaigns. MF and MK were responsible for the lidar observations in the United Arab Emirates. TK, SB, KO and MR were involved in Polarstern cruises. Instruments on board Polarstern have been regularly taken care of by RE, HB and ZY. ISS, LJ, DB and HB are responsible for the PollyNET/EARLINET stations mentioned in this study. DA, BH, AS and RE have continuously contributed to the development and upgrade of PollyXT lidar systems.
At least one of the (co-)authors is a member of the editorial board of Atmospheric Measurement Techniques. The peer-review process was guided by an independent editor, and the authors also have no other competing interests to declare.
Publisher’s note: Copernicus Publications remains neutral with regard to jurisdictional claims in published maps and institutional affiliations.
The authors would like to acknowledge the support, which allowed the realization of the experimental work described in this paper, through the following projects and research programs:
-
ACTRIS under grant agreement no. 262 254 of the European Union Seventh Framework Programme (FP7/2007–2013)
-
ACTRIS-2 under grant agreement no. 654109 from the European Union’s Horizon 2020 research and innovation program
-
EUCAARI funded by the European Union Sixth Framework Programme (FP6) under grant no. 036 833-2
-
CADEX funded by the German Federal Ministry of Education and Research (BMBF) under the grant no. 01DK14014
-
the Gottfried Willhelm Leibniz Association (OCEANET project in the framework of PAKT)
-
BEYOND (funded under: FP7-REGPOT-2012-2013-1) under grant agreement no. 316210
-
“Megacities–Megachallenge – Informal Dynamics of Global Change” (SPP 1233) funded by the German Research Foundation (DFG)
-
Foundation of Science and Technology of Poland (FNiTP) grant no. 519/FNITP/115/2010
-
National Science Centre of Poland (NCN, DAINA-2) grant no. 2020/38/L/ST10/00480
-
Polarstern expeditions ANT-XXVI/1, ANT-XXVI/4, ANT-XXVII/1, AWI_PS75_00, AWI_PS77_00, AWI_PS81_00, AWI_PS83_00, AWI_PS95_00, AWI_PS98_00, AWI_PS122_00 and MOSAiC20192020
-
SAMUM funded by the Deutsche Forschungsgemeinschaft (DFG) under grant number FOR 539
-
BACCHUS funded by the European Union's 7th Framework Programme (FP7/2007-2013) under grant agreement no. 603445
-
A–LIFE funded by the European Research Council (grant no. 640458)
-
EVAA funded by the German Federal Ministry for Economic Affairs and Energy (BMWi) under grant no. 50EE1721C
-
European Research Council (ERC) under the European Community's Horizon 2020 research and innovation framework programme – ERC grant agreement no. 725698 (D-TECT)
-
PANhellenic Geophysical Observatory of Antikythera (PANGEA) of the National Observatory of Athens, Greece
-
“EXCELSIOR”: ERATOSTHENES: EXcellence Research Centre for Earth Surveillance and Space-Based Monitoring of the Environment H2020 Widespread Teaming project (http://www.excelsior2020.eu, last access: 20 March 2023), funded by the European Union's Horizon 2020 research and innovation programme under grant agreement no. 857510, from the Government of the Republic of Cyprus through the Directorate General for the European Programmes, Coordination and Development, and the Cyprus University of Technology
-
PoLiCyTa project “PollyXT-CYP” funded by the German Federal Ministry of Education and Research (BMBF)
-
The National Fund for Scientific and Technological Development of Chile, FONDECYT, through grant agreement no. 11181335
-
the Portuguese national funds through FCT (Fundação para a Ciência e Tecnologia, I.P.) in the framework of the ICT project with the references UIDB/04683/2020 and UIDP/04683/2020
-
funding received by joint Lithuanian–Polish research project DAINA-2 grant “Importance of long range transport of BIOmass-burning emissions to local Smog events in Urban Environments” (BIOSURE) supported by Narodowe Centrum Nauki (grant no. 2020/38/L/ST10/00480) and the Research Council of Lithuania (Lietuvos mokslo taryba) (grant no. S-LL-21-7).
Furthermore, the authors would like to acknowledge and thank all the scientists and technical personnel involved in the realization of all the measurement campaigns and maintenance of the lidar stations, the RV Meteor team for their support during the M96 cruise, the Alfred Wegener Institute, and the RV Polarstern crew during the ship cruises. Many improvements, both in terms of hardware and software, were triggered by the fruitful discussions and network activities within EARLINET and ACTRIS. The authors would like to thank Edward P. Nowottnick for serving as an editor and gratefully acknowledge the constructive comments of Ali H. Omar and one anonymous referee.
This paper was edited by Edward Nowottnick and reviewed by Ali Omar and one anonymous referee.
Althausen, D., Müller, D., Ansmann, A., Wandinger, U., Hube, H., Clauder, E., and Zörner, S.: Scanning 6-Wavelength 11-Channel Aerosol Lidar, J. Atmos. Ocean. Tech., 17, 1469–1482, https://doi.org/10.1175/1520-0426(2000)017<1469:SWCAL>2.0.CO;2, 2000. a, b
Althausen, D., Engelmann, R., Baars, H., Heese, B., Ansmann, A., Müller, D., and Komppula, M.: Portable Raman Lidar Polly(XT) for Automated Profiling of Aerosol Backscatter, Extinction, and Depolarization, J. Atmos. Ocean. Tech., 26, 2366–2378, https://doi.org/10.1175/2009jtecha1304.1, 2009. a, b
Amiridis, V. and the ASKOS team: The ASKOS experiment for desert dust science applications, EGU General Assembly 2022, Vienna, Austria, 23–27 May 2022, EGU22-3633, https://doi.org/10.5194/egusphere-egu22-3633, 2022. a
Amiridis, V., Marinou, E., Tsekeri, A., Wandinger, U., Schwarz, A., Giannakaki, E., Mamouri, R., Kokkalis, P., Binietoglou, I., Solomos, S., Herekakis, T., Kazadzis, S., Gerasopoulos, E., Proestakis, E., Kottas, M., Balis, D., Papayannis, A., Kontoes, C., Kourtidis, K., Papagiannopoulos, N., Mona, L., Pappalardo, G., Le Rille, O., and Ansmann, A.: LIVAS: a 3-D multi-wavelength aerosol/cloud database based on CALIPSO and EARLINET, Atmos. Chem. Phys., 15, 7127–7153, https://doi.org/10.5194/acp-15-7127-2015, 2015. a
Ansmann, A. and Müller, D.: Lidar and Atmospheric Aerosol Particles, in: Lidar: Range-Resolved Optical Remote Sensing of the Atmosphere, 1st edn., edited by: Weitkamp, C., vol. 102 of Springer Series in Optical Sciences, 105–141, Springer, New York, New York, USA, https://doi.org/10.1007/b106786, 2005. a, b, c, d, e
Ansmann, A., Wandinger, U., Riebesell, M., Weitkamp, C., and Michaelis, W.: Independent measurement of extinction and backscatter profiles in cirrus clouds by using a combined Raman elastic-backscatter lidar, Appl. Optics, 31, 7113–7131, https://doi.org/10.1364/AO.31.007113, 1992. a
Ansmann, A., Althausen, D., Wandinger, U., Franke, K., Müller, D., Wagner, F., and Heintzenberg, J.: Vertical profiling of the Indian aerosol plume with six-wavelength lidar during INDOEX: A first case study, Geophys. Res. Lett., 27, 963–966, https://doi.org/10.1029/1999GL010902, 2000. a
Ansmann, A., Wagner, F., Müller, D., Althausen, D., Herber, A., von Hoyningen-Huene, W., and Wandinger, U.: European pollution outbreaks during ACE 2: Optical particle properties inferred from multiwavelength lidar and star-Sun photometry, J. Geophys. Res.-Atmos., 107, AAC 8-1–AAC 8-14, https://doi.org/10.1029/2001JD001109, 2002a. a, b, c
Ansmann, A., Wandinger, U., Wiedensohler, A., and Leiterer, U.: Lindenberg Aerosol Characterization Experiment 1998 (LACE 98): Overview, J. Geophys. Res.-Atmos., 107, LAC 11-1–LAC 11-12, https://doi.org/10.1029/2000JD000233, 2002b. a
Ansmann, A., Engelmann, R., Althausen, D., Wandinger, U., Hu, M., Zhang, Y. H., and He, Q. S.: High aerosol load over the Pearl River Delta, China, observed with Raman lidar and Sun photometer, Geophys. Res. Lett., 32, L13815, https://doi.org/10.1029/2005gl023094, 2005. a, b
Ansmann, A., Petzold, A., Kandler, K., Tegen, I., Wendisch, M., Müller, D., Weinzierl, B., Müller, T., and Heintzenberg, J.: Saharan Mineral Dust Experiments SAMUM-1 and SAMUM-2: what have we learned?, Tellus B, 63, 403–429, https://doi.org/10.1111/j.1600-0889.2011.00555.x, 2011. a, b
Ansmann, A., Baars, H., Chudnovsky, A., Mattis, I., Veselovskii, I., Haarig, M., Seifert, P., Engelmann, R., and Wandinger, U.: Extreme levels of Canadian wildfire smoke in the stratosphere over central Europe on 21–22 August 2017, Atmos. Chem. Phys., 18, 11831–11845, https://doi.org/10.5194/acp-18-11831-2018, 2018. a
Ansmann, A., Mamouri, R.-E., Bühl, J., Seifert, P., Engelmann, R., Hofer, J., Nisantzi, A., Atkinson, J. D., Kanji, Z. A., Sierau, B., Vrekoussis, M., and Sciare, J.: Ice-nucleating particle versus ice crystal number concentrationin altocumulus and cirrus layers embedded in Saharan dust:a closure study, Atmos. Chem. Phys., 19, 15087–15115, https://doi.org/10.5194/acp-19-15087-2019, 2019. a, b
Ansmann, A., Ohneiser, K., Chudnovsky, A., Baars, H., and Engelmann, R.: CALIPSO Aerosol-Typing Scheme Misclassified Stratospheric Fire Smoke: Case Study From the 2019 Siberian Wildfire Season, Front. Environ. Sci., 9, 769852, https://doi.org/10.3389/fenvs.2021.769852, 2021. a
Antuña-Marrero, J. C., Landulfo, E., Estevan, R., Barja, B., Robock, A., Wolfram, E., Ristori, P., Clemesha, B., Zaratti, F., Forno, R., Armandillo, E., Bastidas, Á. E., de Frutos Baraja, Á. M., Whiteman, D. N., Quel, E., Barbosa, H. M. J., Lopes, F., Montilla-Rosero, E., and Guerrero-Rascado, J. L.: LALINET: The First Latin American-Born Regional Atmospheric Observational Network, B. Am. Meteorol. Soc., 98, 1255–1275, https://doi.org/10.1175/BAMS-D-15-00228.1, 2017. a
Baars, H.: Aerosol profiling with lidar in the Amazon Basin during wet and dry season, PhD dissertation, Leipzig University, https://nbn-resolving.org/urn:nbn:de:bsz:15-qucosa-98757 (last access: 20 March 2023), 2011. a, b, c
Baars, H. and Yin, Z.: PollyNET/Pollynet_Processing_Chain: Version 2.0, Zenodo [code], https://doi.org/10.5281/zenodo.3774689, 2020. a
Baars, H., Ansmann, A., Engelmann, R., and Althausen, D.: Continuous monitoring of the boundary-layer top with lidar, Atmos. Chem. Phys., 8, 7281–7296, https://doi.org/10.5194/acp-8-7281-2008, 2008. a
Baars, H., Ansmann, A., Althausen, D., Engelmann, R., Heese, B., Müller, D., Artaxo, P., Paixao, M., Pauliquevis, T., and Souza, R.: Aerosol profiling with lidar in the Amazon Basin during the wet and dry season, J. Geophys. Res.-Atmos., 117, D21201, https://doi.org/10.1029/2012jd018338, 2012. a, b
Baars, H., Kanitz, T., Engelmann, R., Althausen, D., Heese, B., Komppula, M., Preißler, J., Tesche, M., Ansmann, A., Wandinger, U., Lim, J.-H., Ahn, J. Y., Stachlewska, I. S., Amiridis, V., Marinou, E., Seifert, P., Hofer, J., Skupin, A., Schneider, F., Bohlmann, S., Foth, A., Bley, S., Pfüller, A., Giannakaki, E., Lihavainen, H., Viisanen, Y., Hooda, R. K., Pereira, S. N., Bortoli, D., Wagner, F., Mattis, I., Janicka, L., Markowicz, K. M., Achtert, P., Artaxo, P., Pauliquevis, T., Souza, R. A. F., Sharma, V. P., van Zyl, P. G., Beukes, J. P., Sun, J., Rohwer, E. G., Deng, R., Mamouri, R.-E., and Zamorano, F.: An overview of the first decade of PollyNET: an emerging network of automated Raman-polarization lidars for continuous aerosol profiling, Atmos. Chem. Phys., 16, 5111–5137, https://doi.org/10.5194/acp-16-5111-2016, 2016. a, b, c, d
Baars, H., Ansmann, A., Ohneiser, K., Haarig, M., Engelmann, R., Althausen, D., Hanssen, I., Gausa, M., Pietruczuk, A., Szkop, A., Stachlewska, I. S., Wang, D., Reichardt, J., Skupin, A., Mattis, I., Trickl, T., Vogelmann, H., Navas-Guzmán, F., Haefele, A., Acheson, K., Ruth, A. A., Tatarov, B., Müller, D., Hu, Q., Podvin, T., Goloub, P., Veselovskii, I., Pietras, C., Haeffelin, M., Fréville, P., Sicard, M., Comerón, A., Fernández García, A. J., Molero Menéndez, F., Córdoba-Jabonero, C., Guerrero-Rascado, J. L., Alados-Arboledas, L., Bortoli, D., Costa, M. J., Dionisi, D., Liberti, G. L., Wang, X., Sannino, A., Papagiannopoulos, N., Boselli, A., Mona, L., D'Amico, G., Romano, S., Perrone, M. R., Belegante, L., Nicolae, D., Grigorov, I., Gialitaki, A., Amiridis, V., Soupiona, O., Papayannis, A., Mamouri, R.-E., Nisantzi, A., Heese, B., Hofer, J., Schechner, Y. Y., Wandinger, U., and Pappalardo, G.: The unprecedented 2017–2018 stratospheric smoke event: decay phase and aerosol properties observed with the EARLINET, Atmos. Chem. Phys., 19, 15183–15198, https://doi.org/10.5194/acp-19-15183-2019, 2019. a
Baars, H., Radenz, M., Floutsi, A. A., Engelmann, R., Althausen, D., Heese, B., Ansmann, A., Flament, T., Dabas, A., Trapon, D., Reitebuch, O., Bley, S., and Wandinger, U.: Californian Wildfire Smoke Over Europe: A First Example of the Aerosol Observing Capabilities of Aeolus Compared to Ground-Based Lidar, Geophys. Res. Lett., 48, e2020GL092194, https://doi.org/10.1029/2020GL092194, 2021. a
Belegante, L., Bravo-Aranda, J. A., Freudenthaler, V., Nicolae, D., Nemuc, A., Ene, D., Alados-Arboledas, L., Amodeo, A., Pappalardo, G., D'Amico, G., Amato, F., Engelmann, R., Baars, H., Wandinger, U., Papayannis, A., Kokkalis, P., and Pereira, S. N.: Experimental techniques for the calibration of lidar depolarization channels in EARLINET, Atmos. Meas. Tech., 11, 1119–1141, https://doi.org/10.5194/amt-11-1119-2018, 2018. a
Bohlmann, S., Baars, H., Radenz, M., Engelmann, R., and Macke, A.: Ship-borne aerosol profiling with lidar over the Atlantic Ocean: from pure marine conditions to complex dust–smoke mixtures, Atmos. Chem. Phys., 18, 9661–9679, https://doi.org/10.5194/acp-18-9661-2018, 2018. a, b, c, d, e, f, g, h, i, j, k, l
Boucher, O., Randall, D., Artaxo, P., Bretherton, C., Feingold, G., Forster, P., Kerminen, V.-M., Kondo, Y., Liao, H., and Lohmann, U.: Clouds and aerosols, in: Climate change 2013: the physical science basis. Contribution of Working Group I to the Fifth Assessment Report of the Intergovernmental Panel on Climate Change, edited by: Stocker, T. F., Qin, D., Plattner, G.-K., Tignor, M., Allen, S. K., Boschung, J., Nauels, A., Xia, Y., Bex, V., and Midgley, P. M., Cambridge University Press, Cambridge, United Kingdom and New York, NY, USA, 571–657, https://www.ipcc.ch/site/assets/uploads/2018/02/WG1AR5_Chapter07_FINAL-1.pdf (last access: 20 March 2023), 2013. a
Bravo-Aranda, J. A., Belegante, L., Freudenthaler, V., Alados-Arboledas, L., Nicolae, D., Granados-Muñoz, M. J., Guerrero-Rascado, J. L., Amodeo, A., D'Amico, G., Engelmann, R., Pappalardo, G., Kokkalis, P., Mamouri, R., Papayannis, A., Navas-Guzmán, F., Olmo, F. J., Wandinger, U., Amato, F., and Haeffelin, M.: Assessment of lidar depolarization uncertainty by means of a polarimetric lidar simulator, Atmos. Meas. Tech., 9, 4935–4953, https://doi.org/10.5194/amt-9-4935-2016, 2016. a
Bühl, J., Seifert, P., Wandinger, U., Baars, H., Kanitz, T., Schmidt, J., Myagkov, A., Engelmann, R., Skupin, A., Heese, B., Klepel, A., Althausen, D., and Ansmann, A.: LACROS: the Leipzig Aerosol and Cloud Remote Observations System, SPIE Remote Sensing, 8890, 889002, https://doi.org/10.1117/12.2030911, 2013. a, b
Burton, S. P., Ferrare, R. A., Hostetler, C. A., Hair, J. W., Rogers, R. R., Obland, M. D., Butler, C. F., Cook, A. L., Harper, D. B., and Froyd, K. D.: Aerosol classification using airborne High Spectral Resolution Lidar measurements – methodology and examples, Atmos. Meas. Tech., 5, 73–98, https://doi.org/10.5194/amt-5-73-2012, 2012. a, b, c
Burton, S. P., Hair, J. W., Kahnert, M., Ferrare, R. A., Hostetler, C. A., Cook, A. L., Harper, D. B., Berkoff, T. A., Seaman, S. T., Collins, J. E., Fenn, M. A., and Rogers, R. R.: Observations of the spectral dependence of linear particle depolarization ratio of aerosols using NASA Langley airborne High Spectral Resolution Lidar, Atmos. Chem. Phys., 15, 13453–13473, https://doi.org/10.5194/acp-15-13453-2015, 2015. a
D'Amico, G., Amodeo, A., Baars, H., Binietoglou, I., Freudenthaler, V., Mattis, I., Wandinger, U., and Pappalardo, G.: EARLINET Single Calculus Chain – overview on methodology and strategy, Atmos. Meas. Tech., 8, 4891–4916, https://doi.org/10.5194/amt-8-4891-2015, 2015. a
do Carmo, J. P., de Villele, G., Wallace, K., Lefebvre, A., Ghose, K., Kanitz, T., Chassat, F., Corselle, B., Belhadj, T., and Bravetti, P.: ATmospheric LIDar (ATLID): Pre-Launch Testing and Calibration of the European Space Agency Instrument That Will Measure Aerosols and Thin Clouds in the Atmosphere, Atmosphere, 12, 76, https://doi.org/10.3390/atmos12010076, 2021. a
Eck, T. F., Holben, B. N., Reid, J. S., Dubovik, O., Smirnov, A., O'Neill, N. T., Slutsker, I., and Kinne, S.: Wavelength dependence of the optical depth of biomass burning, urban, and desert dust aerosols, J. Geophys. Res.-Atmos., 104, 31333–31349, https://doi.org/10.1029/1999JD900923, 1999. a
Engelmann, R., Kanitz, T., Baars, H., Heese, B., Althausen, D., Skupin, A., Wandinger, U., Komppula, M., Stachlewska, I. S., Amiridis, V., Marinou, E., Mattis, I., Linné, H., and Ansmann, A.: The automated multiwavelength Raman polarization and water-vapor lidar PollyXT: the neXT generation, Atmos. Meas. Tech., 9, 1767–1784, https://doi.org/10.5194/amt-9-1767-2016, 2016. a, b, c, d
Engelmann, R., Ansmann, A., Ohneiser, K., Griesche, H., Radenz, M., Hofer, J., Althausen, D., Dahlke, S., Maturilli, M., Veselovskii, I., Jimenez, C., Wiesen, R., Baars, H., Bühl, J., Gebauer, H., Haarig, M., Seifert, P., Wandinger, U., and Macke, A.: Wildfire smoke, Arctic haze, and aerosol effects on mixed-phase and cirrus clouds over the North Pole region during MOSAiC: an introduction, Atmos. Chem. Phys., 21, 13397–13423, https://doi.org/10.5194/acp-21-13397-2021, 2021. a, b, c, d
Filioglou, M., Giannakaki, E., Backman, J., Kesti, J., Hirsikko, A., Engelmann, R., O'Connor, E., Leskinen, J. T. T., Shang, X., Korhonen, H., Lihavainen, H., Romakkaniemi, S., and Komppula, M.: Optical and geometrical aerosol particle properties over the United Arab Emirates, Atmos. Chem. Phys., 20, 8909–8922, https://doi.org/10.5194/acp-20-8909-2020, 2020. a, b
Flament, T., Trapon, D., Lacour, A., Dabas, A., Ehlers, F., and Huber, D.: Aeolus L2A aerosol optical properties product: standard correct algorithm and Mie correct algorithm, Atmos. Meas. Tech., 14, 7851–7871, https://doi.org/10.5194/amt-14-7851-2021, 2021. a
Floutsi, A. A., Baars, H., and Wandinger, U.: Towards an automatic aerosol typing algorithm applicable for ground-based and spaceborne lidars, Living Planet Symposium, 13–17 May 2019, Milan, Italy, ResearchGate, https://doi.org/10.13140/RG.2.2.14147.45604, 2019. a
Floutsi, A. A., Baars, H., Radenz, M., Haarig, M., Yin, Z., Seifert, P., Jimenez, C., Ansmann, A., Engelmann, R., Barja, B., Zamorano, F., and Wandinger, U.: Advection of Biomass Burning Aerosols towards the Southern Hemispheric Mid-Latitude Station of Punta Arenas as Observed with Multiwavelength Polarization Raman Lidar, Remote Sens., 13, 138, https://doi.org/10.3390/rs13010138, 2021. a, b, c
Floutsi, A. A., Baars, H., Engelmann, R., Althausen, D., Ansmann, A., Bohlmann, S., Heese, B., Hofer, J., Kanitz, T., Haarig, M., Ohneiser, K., Radenz, M., Seifert, P., Skupin, A., Yin, Z., Abdullaev, S. F., Komppula, M., Filioglou, M., Giannakaki, E., Stachlewska, I. S., Janicka, L., Bortoli, D., Marinou, E., Amiridis, V., Gialitaki, A., Mamouri, R.-E., Barja, B., and Wandinger, U.: DeLiAn – a growing collection of depolarization ratio, lidar ratio and Ångström exponent for different aerosol types and mixtures from ground-based lidar observations, Zenodo [data set], https://doi.org/10.5281/zenodo.7751752, 2023. a
Freudenthaler, V.: About the effects of polarising optics on lidar signals and the Δ90 calibration, Atmos. Meas. Tech., 9, 4181–4255, https://doi.org/10.5194/amt-9-4181-2016, 2016. a
Freudenthaler, V., Esselborn, M., Wiegner, M., Heese, B., Tesche, M., Ansmann, A., Müller, D., Althausen, D., Wirth, M., Fix, A., Ehret, G., Knippertz, P., Toledano, C., Gasteiger, J., Garhammer, M., and Seefeldner, M.: Depolarization ratio profiling at several wavelengths in pure Saharan dust during SAMUM 2006, Tellus B, 61, 165–179, https://doi.org/10.1111/j.1600-0889.2008.00396.x, 2009. a
Freudenthaler, V., Linné, H., Chaikovski, A., Rabus, D., and Groß, S.: EARLINET lidar quality assurance tools, Atmos. Meas. Tech. Discuss. [preprint], https://doi.org/10.5194/amt-2017-395, in review, 2018. a
Fuzzi, S., Baltensperger, U., Carslaw, K., Decesari, S., Denier van der Gon, H., Facchini, M. C., Fowler, D., Koren, I., Langford, B., Lohmann, U., Nemitz, E., Pandis, S., Riipinen, I., Rudich, Y., Schaap, M., Slowik, J. G., Spracklen, D. V., Vignati, E., Wild, M., Williams, M., and Gilardoni, S.: Particulate matter, air quality and climate: lessons learned and future needs, Atmos. Chem. Phys., 15, 8217–8299, https://doi.org/10.5194/acp-15-8217-2015, 2015. a
Gialitaki, A., Tsekeri, A., Amiridis, V., Ceolato, R., Paulien, L., Kampouri, A., Gkikas, A., Solomos, S., Marinou, E., Haarig, M., Baars, H., Ansmann, A., Lapyonok, T., Lopatin, A., Dubovik, O., Groß, S., Wirth, M., Tsichla, M., Tsikoudi, I., and Balis, D.: Is the near-spherical shape the “new black” for smoke?, Atmos. Chem. Phys., 20, 14005–14021, https://doi.org/10.5194/acp-20-14005-2020, 2020. a
Giannakaki, E., van Zyl, P. G., Müller, D., Balis, D., and Komppula, M.: Optical and microphysical characterization of aerosol layers over South Africa by means of multi-wavelength depolarization and Raman lidar measurements, Atmos. Chem. Phys., 16, 8109–8123, https://doi.org/10.5194/acp-16-8109-2016, 2016. a, b, c, d, e, f, g, h
Griesche, H. J., Seifert, P., Ansmann, A., Baars, H., Barrientos Velasco, C., Bühl, J., Engelmann, R., Radenz, M., Zhenping, Y., and Macke, A.: Application of the shipborne remote sensing supersite OCEANET for profiling of Arctic aerosols and clouds during Polarstern cruise PS106, Atmos. Meas. Tech., 13, 5335–5358, https://doi.org/10.5194/amt-13-5335-2020, 2020. a
Groß, S., Freudenthaler, V., Toledano, C., Seefeldner, M., and Wiegner, M.: Mini-lidar measurements of particle depolarization and Raman scattering of Saharan-dust and biomass burning at 355 nm during SAMUM 2, Proceedings of 24th International Laser Radar Conference (ILRC 24), 23–27 June 2008, Boulder, Colorado, USA, International Coordination Group on Laser Atmospheric Studies (ICLAS), ISBN 9781617826061, 2008. a
Groß, S., Tesche, M., Freudenthaler, V., Toledano, C., Wiegner, M., Ansmann, A., Althausen, D., and Seefeldner, M.: Characterization of Saharan dust, marine aerosols and mixtures of biomass-burning aerosols and dust by means of multi-wavelength depolarization and Raman lidar measurements during SAMUM-2, Tellus B, 63, 706–724, https://doi.org/10.1111/j.1600-0889.2011.00556.x, 2011. a, b, c, d, e, f, g, h, i, j
Groß, S., Freudenthaler, V., Wiegner, M., Gasteiger, J., Geiß, A., and Schnell, F.: Dual-wavelength linear depolarization ratio of volcanic aerosols: Lidar measurements of the Eyjafjallajökull plume over Maisach, Germany, Atmos. Environ., 48, 85–96, https://doi.org/10.1016/j.atmosenv.2011.06.017, 2012. a, b
Groß, S., Esselborn, M., Weinzierl, B., Wirth, M., Fix, A., and Petzold, A.: Aerosol classification by airborne high spectral resolution lidar observations, Atmos. Chem. Phys., 13, 2487–2505, https://doi.org/10.5194/acp-13-2487-2013, 2013. a
Groß, S., Freudenthaler, V., Schepanski, K., Toledano, C., Schäfler, A., Ansmann, A., and Weinzierl, B.: Optical properties of long-range transported Saharan dust over Barbados as measured by dual-wavelength depolarization Raman lidar measurements, Atmos. Chem. Phys., 15, 11067–11080, https://doi.org/10.5194/acp-15-11067-2015, 2015. a
Haarig, M., Engelmann, R., Ansmann, A., Veselovskii, I., Whiteman, D. N., and Althausen, D.: 1064 nm rotational Raman lidar for particle extinction and lidar-ratio profiling: cirrus case study, Atmos. Meas. Tech., 9, 4269–4278, https://doi.org/10.5194/amt-9-4269-2016, 2016. a
Haarig, M., Ansmann, A., Althausen, D., Klepel, A., Groß, S., Freudenthaler, V., Toledano, C., Mamouri, R.-E., Farrell, D. A., Prescod, D. A., Marinou, E., Burton, S. P., Gasteiger, J., Engelmann, R., and Baars, H.: Triple-wavelength depolarization-ratio profiling of Saharan dust over Barbados during SALTRACE in 2013 and 2014, Atmos. Chem. Phys., 17, 10767–10794, https://doi.org/10.5194/acp-17-10767-2017, 2017a. a, b, c, d, e, f
Haarig, M., Ansmann, A., Gasteiger, J., Kandler, K., Althausen, D., Baars, H., Radenz, M., and Farrell, D. A.: Dry versus wet marine particle optical properties: RH dependence of depolarization ratio, backscatter, and extinction from multiwavelength lidar measurements during SALTRACE, Atmos. Chem. Phys., 17, 14199–14217, https://doi.org/10.5194/acp-17-14199-2017, 2017b. a, b, c, d, e, f
Haarig, M., Ansmann, A., Baars, H., Jimenez, C., Veselovskii, I., Engelmann, R., and Althausen, D.: Depolarization and lidar ratios at 355, 532, and 1064 nm and microphysical properties of aged tropospheric and stratospheric Canadian wildfire smoke, Atmos. Chem. Phys., 18, 11847–11861, https://doi.org/10.5194/acp-18-11847-2018, 2018. a, b, c, d, e, f
Haarig, M., Walser, A., Ansmann, A., Dollner, M., Althausen, D., Sauer, D., Farrell, D., and Weinzierl, B.: Profiles of cloud condensation nuclei, dust mass concentration, and ice-nucleating-particle-relevant aerosol properties in the Saharan Air Layer over Barbados from polarization lidar and airborne in situ measurements, Atmos. Chem. Phys., 19, 13773–13788, https://doi.org/10.5194/acp-19-13773-2019, 2019. a
Haarig, M., Ansmann, A., Engelmann, R., Baars, H., Toledano, C., Torres, B., Althausen, D., Radenz, M., and Wandinger, U.: First triple-wavelength lidar observations of depolarization and extinction-to-backscatter ratios of Saharan dust, Atmos. Chem. Phys., 22, 355–369, https://doi.org/10.5194/acp-22-355-2022, 2022. a, b, c
Hänel, A., Baars, H., Althausen, D., Ansmann, A., Engelmann, R., and Sun, J. Y.: One-year aerosol profiling with EUCAARI Raman lidar at Shangdianzi GAW station: Beijing plume and seasonal variations, J. Geophys. Res.-Atmos., 117, D13201, https://doi.org/10.1029/2012JD017577, 2012. a, b, c, d
Hansen, J., Sato, M., and Ruedy, R.: Radiative forcing and climate response, J. Geophys. Res.-Atmos., 102, 6831–6864, https://doi.org/10.1029/96JD03436, 1997. a
Heese, B., Althausen, D., Baars, H., Bohlmann, S., and Deng, R.: Aerosol Properties over Southeastern China from Multi-Wavelength Raman and Depolarization Lidar Measurements, Proceedings of the 27th International Laser Radar Conference (ILRC 27), 5–10 July 2015, New York City, USA, EPJ Web Conf., 119, 23018, https://doi.org/10.1051/epjconf/201611923018, 2016. a
Heese, B., Baars, H., Bohlmann, S., Althausen, D., and Deng, R.: Continuous vertical aerosol profiling with a multi-wavelength Raman polarization lidar over the Pearl River Delta, China, Atmos. Chem. Phys., 17, 6679–6691, https://doi.org/10.5194/acp-17-6679-2017, 2017. a, b
Heese, B., Floutsi, A. A., Baars, H., Althausen, D., Hofer, J., Herzog, A., Mewes, S., Radenz, M., and Schechner, Y. Y.: The vertical aerosol type distribution above Israel – 2 years of lidar observations at the coastal city of Haifa, Atmos. Chem. Phys., 22, 1633–1648, https://doi.org/10.5194/acp-22-1633-2022, 2022. a
Herold, C., Althausen, D., Müller, D., Tesche, M., Seifert, P., Engelmann, R., Flamant, C., Bhawar, R., and Girolamo, P. D.: Comparison of Raman Lidar Observations of Water Vapor with COSMO-DE Forecasts during COPS 2007, Weather Forcast., 26, 1056–1066, https://doi.org/10.1175/2011WAF2222448.1, 2011. a
Hofer, J., Althausen, D., Abdullaev, S. F., Makhmudov, A. N., Nazarov, B. I., Schettler, G., Engelmann, R., Baars, H., Fomba, K. W., Müller, K., Heinold, B., Kandler, K., and Ansmann, A.: Long-term profiling of mineral dust and pollution aerosol with multiwavelength polarization Raman lidar at the Central Asian site of Dushanbe, Tajikistan: case studies, Atmos. Chem. Phys., 17, 14559–14577, https://doi.org/10.5194/acp-17-14559-2017, 2017. a, b, c
Hofer, J., Ansmann, A., Althausen, D., Engelmann, R., Baars, H., Fomba, K. W., Wandinger, U., Abdullaev, S. F., and Makhmudov, A. N.: Optical properties of Central Asian aerosol relevant for spaceborne lidar applications and aerosol typing at 355 and 532 nm, Atmos. Chem. Phys., 20, 9265–9280, https://doi.org/10.5194/acp-20-9265-2020, 2020. a, b, c, d
Illingworth, A. J., Barker, H. W., Beljaars, A., Ceccaldi, M., Chepfer, H., Clerbaux, N., Cole, J., Delanoe, J., Domenech, C., Donovan, D. P., Fukuda, S., Hirakata, M., Hogan, R. J., Huenerbein, A., Kollias, P., Kubota, T., Nakajima, T., Nakajima, T. Y., Nishizawa, T., Ohno, Y., Okamoto, H., Oki, R., Sato, K., Satoh, M., Shephard, M. W., Velazquez-Blazquez, A., Wandinger, U., Wehr, T., and van Zadelhoff, G. J.: THE EARTHCARE SATELLITE The Next Step Forward in Global Measurements of Clouds, Aerosols, Precipitation, and Radiation, B. Am. Meteorol. Soc., 96, 1311–1332, https://doi.org/10.1175/bams-d-12-00227.1, 2015. a, b, c, d, e
Janicka, L., Stachlewska, I. S., Markowicz, K. M., Baars, H., Engelmann, R., and Heese, B.: Lidar Measurements of Canadian Forest Fire Smoke Episode Observed in July 2013 over Warsaw, Poland, Proceedings of the 27th International Laser Radar Conference (ILRC 27), 5–10 July 2015, New York City, USA, EPJ Web Conf., 119, 18005, https://doi.org/10.1051/epjconf/201611918005, 2016. a, b, c, d
Janicka, L., Stachlewska, I. S., Veselovskii, I., and Baars, H.: Temporal variations in optical and microphysical properties of mineral dust and biomass burning aerosol derived from daytime Raman lidar observations over Warsaw, Poland, Atmos. Environ., 169, 162–174, https://doi.org/10.1016/j.atmosenv.2017.09.022, 2017. a, b
Jimenez, C., Ansmann, A., Engelmann, R., Haarig, M., Schmidt, J., and Wandinger, U.: Polarization lidar: an extended three-signal calibration approach, Atmos. Meas. Tech., 12, 1077–1093, https://doi.org/10.5194/amt-12-1077-2019, 2019. a
Jimenez, C., Ansmann, A., Engelmann, R., Donovan, D., Malinka, A., Schmidt, J., Seifert, P., and Wandinger, U.: The dual-field-of-view polarization lidar technique: a new concept in monitoring aerosol effects in liquid-water clouds – theoretical framework, Atmos. Chem. Phys., 20, 15247–15263, https://doi.org/10.5194/acp-20-15247-2020, 2020a. a, b
Jimenez, C., Ansmann, A., Engelmann, R., Donovan, D., Malinka, A., Seifert, P., Wiesen, R., Radenz, M., Yin, Z., Bühl, J., Schmidt, J., Barja, B., and Wandinger, U.: The dual-field-of-view polarization lidar technique: a new concept in monitoring aerosol effects in liquid-water clouds – case studies, Atmos. Chem. Phys., 20, 15265–15284, https://doi.org/10.5194/acp-20-15265-2020, 2020b. a
Kaduk, C.: Characterization of the optical properties of complex aerosol mixtures observed with a multiwavelength–Raman–polarization lidar during the 6-weeks BACCHUS campaign in Cyprus in spring 2015, MSc thesis, Leipzig University, https://www.tropos.de/fileadmin/user_upload/Institut/Abteilungen/Fernerkundung/Daten_PDF/MA_Clara_Kaduk.pdf (last access: 20 March 2023), 2017. a, b, c, d, e, f, g, h, i, j, k, l, m, n, o
Kanitz, T.: Vertical distribution of aerosols above the Atlantic Ocean, Punta Arenas (Chile), and Stellenbosch (South Africa), PhD dissertation, Technische Universität Berlin, https://doi.org/10.14279/depositonce-3386, 2012. a, b
Kanitz, T., Ansmann, A., Engelmann, R., and Althausen, D.: North-south cross sections of the vertical aerosol distribution over the Atlantic Ocean from multiwavelength Raman/polarization lidar during Polarstern cruises, J. Geophys. Res.-Atmos., 118, 2643–2655, https://doi.org/10.1002/jgrd.50273, 2013a. a, b, c, d, e, f
Kanitz, T., Ansmann, A., Seifert, P., Engelmann, R., Kalisch, J., and Althausen, D.: Radiative effect of aerosols above the northern and southern Atlantic Ocean as determined from shipborne lidar observations, J. Geophys. Res.-Atmos., 118, 12556–12565, https://doi.org/10.1002/2013jd019750, 2013b. a
Kanitz, T., Ansmann, A., Foth, A., Seifert, P., Wandinger, U., Engelmann, R., Baars, H., Althausen, D., Casiccia, C., and Zamorano, F.: Surface matters: limitations of CALIPSO V3 aerosol typing in coastal regions, Atmos. Meas. Tech., 7, 2061–2072, https://doi.org/10.5194/amt-7-2061-2014, 2014a. a
Kanitz, T., Engelmann, R., Heinold, B., Baars, H., Skupin, A., and Ansmann, A.: Tracking the Saharan Air Layer with shipborne lidar across the tropical Atlantic, Geophys. Res. Lett., 41, 1044–1050, https://doi.org/10.1002/2013GL058780, 2014b. a, b
Kim, M.-H., Omar, A. H., Tackett, J. L., Vaughan, M. A., Winker, D. M., Trepte, C. R., Hu, Y., Liu, Z., Poole, L. R., Pitts, M. C., Kar, J., and Magill, B. E.: The CALIPSO version 4 automated aerosol classification and lidar ratio selection algorithm, Atmos. Meas. Tech., 11, 6107–6135, https://doi.org/10.5194/amt-11-6107-2018, 2018. a, b, c, d, e, f, g
Komppula, M., Mielonen, T., Arola, A., Korhonen, K., Lihavainen, H., Hyvärinen, A.-P., Baars, H., Engelmann, R., Althausen, D., Ansmann, A., Müller, D., Panwar, T. S., Hooda, R. K., Sharma, V. P., Kerminen, V.-M., Lehtinen, K. E. J., and Viisanen, Y.: Technical Note: One year of Raman-lidar measurements in Gual Pahari EUCAARI site close to New Delhi in India – Seasonal characteristics of the aerosol vertical structure, Atmos. Chem. Phys., 12, 4513–4524, https://doi.org/10.5194/acp-12-4513-2012, 2012. a, b, c, d
Kulmala, M., Asmi, A., Lappalainen, H. K., Baltensperger, U., Brenguier, J.-L., Facchini, M. C., Hansson, H.-C., Hov, Ø., O'Dowd, C. D., Pöschl, U., Wiedensohler, A., Boers, R., Boucher, O., de Leeuw, G., Denier van der Gon, H. A. C., Feichter, J., Krejci, R., Laj, P., Lihavainen, H., Lohmann, U., McFiggans, G., Mentel, T., Pilinis, C., Riipinen, I., Schulz, M., Stohl, A., Swietlicki, E., Vignati, E., Alves, C., Amann, M., Ammann, M., Arabas, S., Artaxo, P., Baars, H., Beddows, D. C. S., Bergström, R., Beukes, J. P., Bilde, M., Burkhart, J. F., Canonaco, F., Clegg, S. L., Coe, H., Crumeyrolle, S., D'Anna, B., Decesari, S., Gilardoni, S., Fischer, M., Fjaeraa, A. M., Fountoukis, C., George, C., Gomes, L., Halloran, P., Hamburger, T., Harrison, R. M., Herrmann, H., Hoffmann, T., Hoose, C., Hu, M., Hyvärinen, A., Hõrrak, U., Iinuma, Y., Iversen, T., Josipovic, M., Kanakidou, M., Kiendler-Scharr, A., Kirkevåg, A., Kiss, G., Klimont, Z., Kolmonen, P., Komppula, M., Kristjánsson, J.-E., Laakso, L., Laaksonen, A., Labonnote, L., Lanz, V. A., Lehtinen, K. E. J., Rizzo, L. V., Makkonen, R., Manninen, H. E., McMeeking, G., Merikanto, J., Minikin, A., Mirme, S., Morgan, W. T., Nemitz, E., O'Donnell, D., Panwar, T. S., Pawlowska, H., Petzold, A., Pienaar, J. J., Pio, C., Plass-Duelmer, C., Prévôt, A. S. H., Pryor, S., Reddington, C. L., Roberts, G., Rosenfeld, D., Schwarz, J., Seland, Ø., Sellegri, K., Shen, X. J., Shiraiwa, M., Siebert, H., Sierau, B., Simpson, D., Sun, J. Y., Topping, D., Tunved, P., Vaattovaara, P., Vakkari, V., Veefkind, J. P., Visschedijk, A., Vuollekoski, H., Vuolo, R., Wehner, B., Wildt, J., Woodward, S., Worsnop, D. R., van Zadelhoff, G.-J., Zardini, A. A., Zhang, K., van Zyl, P. G., Kerminen, V.-M., S Carslaw, K., and Pandis, S. N.: General overview: European Integrated project on Aerosol Cloud Climate and Air Quality interactions (EUCAARI) – integrating aerosol research from nano to global scales, Atmos. Chem. Phys., 11, 13061–13143, https://doi.org/10.5194/acp-11-13061-2011, 2011. a
Lelieveld, J., Hadjinicolaou, P., Kostopoulou, E., Chenoweth, J., El Maayar, M., Giannakopoulos, C., Hannides, C., Lange, M. A., Tanarhte, M., Tyrlis, E., and Xoplaki, E.: Climate change and impacts in the Eastern Mediterranean and the Middle East, Clim. Change, 114, 667–687, https://doi.org/10.1007/s10584-012-0418-4, 2012. a
Mamouri, R. E. and Ansmann, A.: Fine and coarse dust separation with polarization lidar, Atmos. Meas. Tech., 7, 3717–3735, https://doi.org/10.5194/amt-7-3717-2014, 2014. a
Mamouri, R. E. and Ansmann, A.: Estimated desert-dust ice nuclei profiles from polarization lidar: methodology and case studies, Atmos. Chem. Phys., 15, 3463–3477, https://doi.org/10.5194/acp-15-3463-2015, 2015. a
Mamouri, R.-E. and Ansmann, A.: Potential of polarization lidar to provide profiles of CCN- and INP-relevant aerosol parameters, Atmos. Chem. Phys., 16, 5905–5931, https://doi.org/10.5194/acp-16-5905-2016, 2016. a, b
Mattis, I., Ansmann, A., Althausen, D., Jaenisch, V., Wandinger, U., Müller, D., Arshinov, Y. F., Bobrovnikov, S. M., and Serikov, I. B.: Relative-humidity profiling in the troposphere with a Raman lidar, Appl. Optics, 41, 6451–6462, https://doi.org/10.1364/AO.41.006451, 2002a. a, b, c
Mattis, I., Ansmann, A., Müller, D., Wandinger, U., and Althausen, D.: Dual-wavelength Raman lidar observations of the extinction-to-backscatter ratio of Saharan dust, Geophys. Res. Lett., 29, 1306, https://doi.org/10.1029/2002GL014721, 2002b. a
Mattis, I., Ansmann, A., Müller, D., Wandinger, U., and Althausen, D.: Multiyear aerosol observations with dual-wavelength Raman lidar in the framework of EARLINET, J. Geophys. Res.-Atmos., 109, D13203, https://doi.org/10.1029/2004JD004600, 2004. a, b
Mattis, I., Müller, D., Ansmann, A., Wandinger, U., Preißler, J., Seifert, P., and Tesche, M.: Ten years of multiwavelength Raman lidar observations of free-tropospheric aerosol layers over central Europe: Geometrical properties and annual cycle, J. Geophys. Res.-Atmos., 113, D20202, https://doi.org/10.1029/2007JD009636, 2008. a
Müller, D., Wandinger, U., Althausen, D., Mattis, I., and Ansmann, A.: Retrieval of physical particle properties from lidar observations of extinction and backscatter at multiple wavelengths, Appl. Optics, 37, 2260–2263, https://doi.org/10.1364/AO.37.002260, 1998. a
Müller, D., Wandinger, U., and Ansmann, A.: Microphysical particle parameters from extinction and backscatter lidar data by inversion with regularization: theory, Appl. Optics, 38, 2346–2357, https://doi.org/10.1364/AO.38.002346, 1999a. a
Müller, D., Wandinger, U., and Ansmann, A.: Microphysical particle parameters from extinction and backscatter lidar data by inversion with regularization: simulation, Appl. Optics, 38, 2358–2368, https://doi.org/10.1364/AO.38.002358, 1999b. a
Müller, D., Wagner, F., Wandinger, U., Ansmann, A., Wendisch, M., Althausen, D., and von Hoyningen-Huene, W.: Microphysical particle parameters from extinction and backscatter lidar data by inversion with regularization: experiment, Appl. Optics, 39, 1879–1892, https://doi.org/10.1364/AO.39.001879, 2000. a
Müller, D., Ansmann, A., Wagner, F., Franke, K., and Althausen, D.: European pollution outbreaks during ACE 2: Microphysical particle properties and single-scattering albedo inferred from multiwavelength lidar observations, J. Geophys. Res.-Atmos., 107, 4248, https://doi.org/10.1029/2001JD001110, 2002. a
Müller, D., Franke, K., Ansmann, A., Althausen, D., and Wagner, F.: Indo-Asian pollution during INDOEX: Microphysical particle properties and single-scattering albedo inferred from multiwavelength lidar observations, J. Geophys. Res.-Atmos., 108, 4600, https://doi.org/10.1029/2003JD003538, 2003. a
Müller, D., Mattis, I., Wandinger, U., Ansmann, A., Althausen, D., and Stohl, A.: Raman lidar observations of aged Siberian and Canadian forest fire smoke in the free troposphere over Germany in 2003: Microphysical particle characterization, J. Geophys. Res.-Atmos., 110, D17201, https://doi.org/10.1029/2004jd005756, 2005. a
Müller, D., Ansmann, A., Mattis, I., Tesche, M., Wandinger, U., Althausen, D., and Pisani, G.: Aerosol-type-dependent lidar ratios observed with Raman lidar, J. Geophys. Res.-Atmos., 112, D16202, https://doi.org/10.1029/2006jd008292, 2007. a, b, c, d, e, f, g, h, i, j
Müller, D., Kolgotin, A., Mattis, I., Petzold, A., and Stohl, A.: Vertical profiles of microphysical particle properties derived from inversion with two-dimensional regularization of multiwavelength Raman lidar data: experiment, Appl. Optics, 50, 2069–2079, https://doi.org/10.1364/AO.50.002069, 2011. a
Nicolae, D., Vasilescu, J., Talianu, C., Binietoglou, I., Nicolae, V., Andrei, S., and Antonescu, B.: A neural network aerosol-typing algorithm based on lidar data, Atmos. Chem. Phys., 18, 14511–14537, https://doi.org/10.5194/acp-18-14511-2018, 2018. a
Ohneiser, K., Ansmann, A., Baars, H., Seifert, P., Barja, B., Jimenez, C., Radenz, M., Teisseire, A., Floutsi, A., Haarig, M., Foth, A., Chudnovsky, A., Engelmann, R., Zamorano, F., Bühl, J., and Wandinger, U.: Smoke of extreme Australian bushfires observed in the stratosphere over Punta Arenas, Chile, in January 2020: optical thickness, lidar ratios, and depolarization ratios at 355 and 532 nm, Atmos. Chem. Phys., 20, 8003–8015, https://doi.org/10.5194/acp-20-8003-2020, 2020. a, b, c, d
Ohneiser, K., Ansmann, A., Chudnovsky, A., Engelmann, R., Ritter, C., Veselovskii, I., Baars, H., Gebauer, H., Griesche, H., Radenz, M., Hofer, J., Althausen, D., Dahlke, S., and Maturilli, M.: The unexpected smoke layer in the High Arctic winter stratosphere during MOSAiC 2019–2020 , Atmos. Chem. Phys., 21, 15783–15808, https://doi.org/10.5194/acp-21-15783-2021, 2021. a, b, c, d
Omar, A. H., Won, J.-G., Winker, D. M., Yoon, S.-C., Dubovik, O., and McCormick, M. P.: Development of global aerosol models using cluster analysis of Aerosol Robotic Network (AERONET) measurements, J. Geophys. Res.-Atmos., 110, D10S14, https://doi.org/10.1029/2004JD004874, 2005. a, b
Omar, A. H., Winker, D. M., Vaughan, M. A., Hu, Y., Trepte, C. R., Ferrare, R. A., Lee, K.-P., Hostetler, C. A., Kittaka, C., Rogers, R. R., Kuehn, R. E., and Liu, Z.: The CALIPSO Automated Aerosol Classification and Lidar Ratio Selection Algorithm, J. Atmos. Ocean. Tech., 26, 1994–2014, https://doi.org/10.1175/2009jtecha1231.1, 2009. a, b
Papagiannopoulos, N., Mona, L., Amodeo, A., D'Amico, G., Gumà Claramunt, P., Pappalardo, G., Alados-Arboledas, L., Guerrero-Rascado, J. L., Amiridis, V., Kokkalis, P., Apituley, A., Baars, H., Schwarz, A., Wandinger, U., Binietoglou, I., Nicolae, D., Bortoli, D., Comerón, A., Rodríguez-Gómez, A., Sicard, M., Papayannis, A., and Wiegner, M.: An automatic observation-based aerosol typing method for EARLINET, Atmos. Chem. Phys., 18, 15879–15901, https://doi.org/10.5194/acp-18-15879-2018, 2018. a
Pappalardo, G., Amodeo, A., Apituley, A., Comeron, A., Freudenthaler, V., Linné, H., Ansmann, A., Bösenberg, J., D'Amico, G., Mattis, I., Mona, L., Wandinger, U., Amiridis, V., Alados-Arboledas, L., Nicolae, D., and Wiegner, M.: EARLINET: towards an advanced sustainable European aerosol lidar network, Atmos. Meas. Tech., 7, 2389–2409, https://doi.org/10.5194/amt-7-2389-2014, 2014. a, b, c
Pereira, N. S., Preißler, J., Guerrero-Rascado, J. L., Silva, A. M., and Wagner, F.: Forest Fire Smoke Layers Observed in the Free Troposphere over Portugal with a Multiwavelength Raman Lidar: Optical and Microphysical Properties, Sc. World J., 2014, 421838, https://doi.org/10.1155/2014/421838, 2014. a, b
Pisso, I., Sollum, E., Grythe, H., Kristiansen, N. I., Cassiani, M., Eckhardt, S., Arnold, D., Morton, D., Thompson, R. L., Groot Zwaaftink, C. D., Evangeliou, N., Sodemann, H., Haimberger, L., Henne, S., Brunner, D., Burkhart, J. F., Fouilloux, A., Brioude, J., Philipp, A., Seibert, P., and Stohl, A.: The Lagrangian particle dispersion model FLEXPART version 10.4, Geosci. Model Dev., 12, 4955–4997, https://doi.org/10.5194/gmd-12-4955-2019, 2019. a
Preißler, J., Wagner, F., Pereira, S. N., and Guerrero-Rascado, J. L.: Multi-instrumental observation of an exceptionally strong Saharan dust outbreak over Portugal, J. Geophys. Res.-Atmos., 116, D24204, https://doi.org/10.1029/2011JD016527, 2011. a, b
Preißler, J., Wagner, F., Guerrero-Rascado, J. L., and Silva, A. M.: Two years of free-tropospheric aerosol layers observed over Portugal by lidar, J. Geophys. Res.-Atmos., 118, 3676–3686, https://doi.org/10.1002/jgrd.50350, 2013. a, b, c, d
Radenz, M., Bühl, J., Seifert, P., Baars, H., Engelmann, R., Barja González, B., Mamouri, R.-E., Zamorano, F., and Ansmann, A.: Hemispheric contrasts in ice formation in stratiform mixed-phase clouds: disentangling the role of aerosol and dynamics with ground-based remote sensing, Atmos. Chem. Phys., 21, 17969–17994, https://doi.org/10.5194/acp-21-17969-2021, 2021a. a, b, c, d
Radenz, M., Seifert, P., Baars, H., Floutsi, A. A., Yin, Z., and Bühl, J.: Automated time–height-resolved air mass source attribution for profiling remote sensing applications, Atmos. Chem. Phys., 21, 3015–3033, https://doi.org/10.5194/acp-21-3015-2021, 2021b. a, b
Ramanathan, V., Crutzen, P. J., Lelieveld, J., Mitra, A. P., Althausen, D., Anderson, J., Andreae, M. O., Cantrell, W., Cass, G. R., Chung, C. E., Clarke, A. D., Coakley, J. A., Collins, W. D., Conant, W. C., Dulac, F., Heintzenberg, J., Heymsfield, A. J., Holben, B., Howell, S., Hudson, J., Jayaraman, A., Kiehl, J. T., Krishnamurti, T. N., Lubin, D., McFarquhar, G., Novakov, T., Ogren, J. A., Podgorny, I. A., Prather, K., Priestley, K., Prospero, J. M., Quinn, P. K., Rajeev, K., Rasch, P., Rupert, S., Sadourny, R., Satheesh, S. K., Shaw, G. E., Sheridan, P., and Valero, F. P. J.: Indian Ocean Experiment: An integrated analysis of the climate forcing and effects of the great Indo-Asian haze, J. Geophys. Res.-Atmos., 106, 28371–28398, https://doi.org/10.1029/2001JD900133, 2001. a
Rittmeister, F., Ansmann, A., Engelmann, R., Skupin, A., Baars, H., Kanitz, T., and Kinne, S.: Profiling of Saharan dust from the Caribbean to western Africa – Part 1: Layering structures and optical properties from shipborne polarization/Raman lidar observations, Atmos. Chem. Phys., 17, 12963–12983, https://doi.org/10.5194/acp-17-12963-2017, 2017. a, b, c, d, e, f, g, h, i, j, k, l
Sasano, Y. and Browell, E. V.: Light scattering characteristics of various aerosol types derived from multiple wavelength lidar observations, Appl. Optics, 28, 1670–1679, https://doi.org/10.1364/AO.28.001670, 1989. a
Schmidt, J., Wandinger, U., and Malinka, A.: Dual-field-of-view Raman lidar measurements for the retrieval of cloud microphysical properties, Appl. Optics, 52, 2235–2247, https://doi.org/10.1364/AO.52.002235, 2013. a, b
Schotland, R. M., Sassen, K., and Stone, R.: Observations by Lidar of Linear Depolarization Ratios for Hydrometeors, J. Appl. Meteorol. Clim., 10, 1011–1017, https://doi.org/10.1175/1520-0450(1971)010<1011:oblold>2.0.co;2, 1971. a
Schuster, G., Toth, T., Trepte, C., Chin, M., Bian, H., Kim, D., Kar, J., and Welton, E.: Mapping Modeled Aerosol Species to Measured Lidar Ratios for the MIRA Project, The 30th International Laser Radar Conference, 26 June–1 July 2022, Montana, USA, https://science.larc.nasa.gov/mira-wg/ (last access: 20 March 2023), 2022. a
Sicard, M., Guerrero-Rascado, J. L., Navas-Guzmán, F., Preißler, J., Molero, F., Tomás, S., Bravo-Aranda, J. A., Comerón, A., Rocadenbosch, F., Wagner, F., Pujadas, M., and Alados-Arboledas, L.: Monitoring of the Eyjafjallajökull volcanic aerosol plume over the Iberian Peninsula by means of four EARLINET lidar stations, Atmos. Chem. Phys., 12, 3115–3130, https://doi.org/10.5194/acp-12-3115-2012, 2012. a, b
Stein, A. F., Draxler, R. R., Rolph, G. D., Stunder, B. J. B., Cohen, M. D., and Ngan, F.: NOAA’s HYSPLIT Atmospheric Transport and Dispersion Modeling System, B. Am. Meteorol. Soc., 96, 2059–2077, https://doi.org/10.1175/bams-d-14-00110.1, 2015. a
Stoffelen, A., Pailleux, J., Källén, E., Vaughan, J. M., Isaksen, L., Flamant, P., Wergen, W., Andersson, E., Schyberg, H., Culoma, A., Meynart, R., Endemann, M., and Ingmann, P.: The Atmospheric Dynamics Mission For Global Wind Field Measurement, B. Am. Meteorol. Soc., 86, 73–88, https://doi.org/10.1175/BAMS-86-1-73, 2005. a
Sugimoto, N., Matsui, I., Shimizu, A., Uno, I., Asai, K., Endoh, T., and Nakajima, T.: Observation of dust and anthropogenic aerosol plumes in the Northwest Pacific with a two-wavelength polarization lidar on board the research vessel Mirai, Geophys. Res. Lett., 29, 7-1–7-4, https://doi.org/10.1029/2002GL015112, 2002. a
Sugimoto, N., Nishizawa, T., Shimizu, A., Matsui, I., and Jin, Y.: Characterization of aerosols in East Asia with the Asian Dust and Aerosol Lidar Observation Network (AD-Net), SPIE Asia-Pacific Remote Sensing, 9262, 74–82, https://doi.org/10.1117/12.2069892, 2014. a
Szczepanik, D. M., Stachlewska, I. S., Tetoni, E., and Althausen, D.: Properties of Saharan Dust Versus Local Urban Dust–A Case Study, Earth Space Sci., 8, e2021EA001816, https://doi.org/10.1029/2021EA001816, 2021. a, b
Tackett, J. L., Kar, J., Vaughan, M. A., Getzewich, B. J., Kim, M.-H., Vernier, J.-P., Omar, A. H., Magill, B. E., Pitts, M. C., and Winker, D. M.: The CALIPSO version 4.5 stratospheric aerosol subtyping algorithm, Atmos. Meas. Tech., 16, 745–768, https://doi.org/10.5194/amt-16-745-2023, 2023. a, b, c, d
Tesche, M.: Vertical profiling of aerosol optical properties with multiwavelength aerosol lidar during the Saharan Mineral Dust Experiments, PhD dissertation, University of Leipzig, https://nbn-resolving.org/urn:nbn:de:bsz:15-qucosa-71257, (last access: 20 March 2023), 2011. a, b, c, d
Tesche, M., Ansmann, A., Müller, D., Althausen, D., Engelmann, R., Hu, M., and Zhang, Y.: Particle backscatter, extinction, and lidar ratio profiling with Raman lidar in south and north China, Appl. Optics, 46, 6302–6308, https://doi.org/10.1364/AO.46.006302, 2007. a, b
Tesche, M., Ansmann, A., Müller, D., Althausen, D., Mattis, I., Heese, B., Freudenthaler, V., Wiegner, M., Esselborn, M., Pisani, G., and Knippertz, P.: Vertical profiling of Saharan dust with Raman lidars and airborne HSRL in southern Morocco during SAMUM, Tellus B, 61, 144–164, https://doi.org/10.1111/j.1600-0889.2008.00390.x, 2009a. a
Tesche, M., Ansmann, A., Müller, D., Althausen, D., Engelmann, R., Freudenthaler, V., and Groß, S.: Vertically resolved separation of dust and smoke over Cape Verde using multiwavelength Raman and polarization lidars during Saharan Mineral Dust Experiment 2008, J. Geophys. Res.-Atmos., 114, D13202, https://doi.org/10.1029/2009jd011862, 2009b. a, b
Tesche, M., Groß, S., Ansmann, A., Müller, D., Althausen, D., Freudenthaler, V., and Esselborn, M.: Profiling of Saharan dust and biomass-burning smoke with multiwavelength polarization Raman lidar at Cape Verde, Tellus B, 63, 649–676, https://doi.org/10.1111/j.1600-0889.2011.00548.x, 2011a. a, b, c
Tesche, M., Müller, D., Groß, S., Ansmann, A., Althausen, D., Freudenthaler, V., Weinzierl, B., Veira, A., and Petzold, A.: Optical and microphysical properties of smoke over Cape Verde inferred from multiwavelength lidar measurements, Tellus B, 63, 677–694, https://doi.org/10.1111/j.1600-0889.2011.00549.x, 2011b. a
Thomas, M. A., Devasthale, A., and Kahnert, M.: Marine aerosol properties over the Southern Ocean in relation to the wintertime meteorological conditions, Atmos. Chem. Phys., 22, 119–137, https://doi.org/10.5194/acp-22-119-2022, 2022. a
Urbanneck, C.: Retrieval of aerosol optical and microphysical properties in Cyprus during A-LIFE and CyCARE by lidar and closure studies with airborne in-situ measurements – Towards aerosol-cloud interaction investigations, MSc thesis, Leipzig University, https://www.tropos.de/fileadmin/user_upload/Institut/Abteilungen/Fernerkundung/Daten_PDF/MA_claudia_urbanneck.pdf, (last access: 20 March 2023), 2018. a, b, c, d
Wandinger, U., Baars, H., Engelmann, R., Hünerbein, A., Horn, S., Kanitz, T., Donovan, D., van Zadelhoff, G.-J., Daou, D., Fischer, J., von Bismarck, J., Filipitsch, F., Docter, N., Eisinger, M., Lajas, D., and Wehr, T.: HETEAC: The Aerosol Classification Model for EarthCARE, Proceedings of the 27th International Laser Radar Conference (ILRC 27), 5–10 July 2015, New York City, USA, EPJ Web Conf., 119, 01004, https://doi.org/10.1051/epjconf/201611901004, 2016a. a, b, c
Wandinger, U., Freudenthaler, V., Baars, H., Amodeo, A., Engelmann, R., Mattis, I., Groß, S., Pappalardo, G., Giunta, A., D'Amico, G., Chaikovsky, A., Osipenko, F., Slesar, A., Nicolae, D., Belegante, L., Talianu, C., Serikov, I., Linné, H., Jansen, F., Apituley, A., Wilson, K. M., de Graaf, M., Trickl, T., Giehl, H., Adam, M., Comerón, A., Muñoz-Porcar, C., Rocadenbosch, F., Sicard, M., Tomás, S., Lange, D., Kumar, D., Pujadas, M., Molero, F., Fernández, A. J., Alados-Arboledas, L., Bravo-Aranda, J. A., Navas-Guzmán, F., Guerrero-Rascado, J. L., Granados-Muñoz, M. J., Preißler, J., Wagner, F., Gausa, M., Grigorov, I., Stoyanov, D., Iarlori, M., Rizi, V., Spinelli, N., Boselli, A., Wang, X., Lo Feudo, T., Perrone, M. R., De Tomasi, F., and Burlizzi, P.: EARLINET instrument intercomparison campaigns: overview on strategy and results, Atmos. Meas. Tech., 9, 1001–1023, https://doi.org/10.5194/amt-9-1001-2016, 2016b. a
Wandinger, U., Floutsi, A. A., Baars, H., Haarig, M., Ansmann, A., Hünerbein, A., Docter, N., Donovan, D., van Zadelhoff, G.-J., Mason, S., and Cole, J.: HETEAC – The Hybrid End-To-End Aerosol Classification model for EarthCARE, EGUsphere [preprint], https://doi.org/10.5194/egusphere-2022-1241, 2022. a, b
Weinzierl, B., Sauer, D., Esselborn, M., Petzold, A., Veira, A., Rose, M., Mund, S., Wirth, M., Ansmann, A., Tesche, M., Groß, S., and Freudenthaler, V.: Microphysical and optical properties of dust and tropical biomass burning aerosol layers in the Cape Verde region – an overview of the airborne in situ and lidar measurements during SAMUM-2, Tellus B, 63, 589–618, https://doi.org/10.1111/j.1600-0889.2011.00566.x, 2011. a
Weinzierl, B., Ansmann, A., Prospero, J. M., Althausen, D., Benker, N., Chouza, F., Dollner, M., Farrell, D., Fomba, W. K., Freudenthaler, V., Gasteiger, J., Groß, S., Haarig, M., Heinold, B., Kandler, K., Kristensen, T. B., Mayol-Bracero, O. L., Müller, T., Reitebuch, O., Sauer, D., Schäfler, A., Schepanski, K., Spanu, A., Tegen, I., Toledano, C., and Walser, A.: The Saharan Aerosol Long-Range Transport and Aerosol–Cloud-Interaction Experiment: Overview and Selected Highlights, B. Am. Meteorol. Soc., 98, 1427–1451, https://doi.org/10.1175/bams-d-15-00142.1, 2017. a
Welton, E. J., Campbell, J. R., Berkoff, T. A., Spinhirne, J. D., Tsay, S.-C., Holben, B., Shiobara, M., and Starr, D. O.: The micro-pulse lidar network (MPLNET), 21st International Laser Radar Conference (ILRC21), Quebec, Canada, 8–12 July 2002, https://ams.confex.com/ams/pdfpapers/86086.pdf, (last access: 20 March 2023), 2002. a
Winker, D. M., Vaughan, M. A., Omar, A., Hu, Y., Powell, K. A., Liu, Z., Hunt, W. H., and Young, S. A.: Overview of the CALIPSO mission and CALIOP data processing algorithms, J. Atmos. Ocean. Tech., 26, 2310–2323, https://doi.org/10.1175/2009JTECHA1281.1, 2009. a
Yin, Z. and Baars, H.: PollyNET/Pollynet_Processing_Chain: Version 3.0, Zenodo [code], https://doi.org/10.5281/zenodo.5571289, 2021. a
Yin, Z., Ansmann, A., Baars, H., Seifert, P., Engelmann, R., Radenz, M., Jimenez, C., Herzog, A., Ohneiser, K., Hanbuch, K., Blarel, L., Goloub, P., Dubois, G., Victori, S., and Maupin, F.: Aerosol measurements with a shipborne Sun–sky–lunar photometer and collocated multiwavelength Raman polarization lidar over the Atlantic Ocean, Atmos. Meas. Tech., 12, 5685–5698, https://doi.org/10.5194/amt-12-5685-2019, 2019. a
Zieger, P., Väisänen, O., Corbin, J. C., Partridge, D. G., Bastelberger, S., Mousavi-Fard, M., Rosati, B., Gysel, M., Krieger, U. K., Leck, C., Nenes, A., Riipinen, I., Virtanen, A., and Salter, M. E.: Revising the hygroscopicity of inorganic sea salt particles, Nat. Comm., 8, 15883, https://doi.org/10.1038/ncomms15883, 2017. a
- Abstract
- Introduction
- Intensive optical properties, instruments, data analysis and sources
- DeLiAn
- Conclusions and outlook
- Appendix A: 2D spaces of intensive optical properties
- Appendix B: Number of data samples per aerosol category
- Data availability
- Author contributions
- Competing interests
- Disclaimer
- Acknowledgements
- Review statement
- References
- Abstract
- Introduction
- Intensive optical properties, instruments, data analysis and sources
- DeLiAn
- Conclusions and outlook
- Appendix A: 2D spaces of intensive optical properties
- Appendix B: Number of data samples per aerosol category
- Data availability
- Author contributions
- Competing interests
- Disclaimer
- Acknowledgements
- Review statement
- References