the Creative Commons Attribution 4.0 License.
the Creative Commons Attribution 4.0 License.
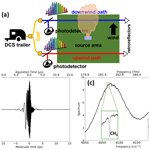
Using open-path dual-comb spectroscopy to monitor methane emissions from simulated grazing cattle
Chinthaka Weerasekara
Lindsay C. Morris
Nathan A. Malarich
Fabrizio R. Giorgetta
Daniel I. Herman
Kevin C. Cossel
Nathan R. Newbury
Clenton E. Owensby
Stephen M. Welch
Cosmin Blaga
Brett D. DePaola
Ian Coddington
Brian R. Washburn
Eduardo A. Santos
Accurate whole-farm or herd-level measurements of livestock methane emissions are necessary for anthropogenic greenhouse gas inventories and to evaluate mitigation strategies. A controlled methane (CH4) release experiment was performed to determine if dual-comb spectroscopy (DCS) can detect CH4 concentration enhancements produced by a typical herd of beef cattle in an extensive grazing system. Open-path DCS was used to measure downwind and upwind CH4 concentrations from 10 point sources of methane simulating cattle emissions. The CH4 mole fractions and wind velocity data were used to calculate CH4 flux using an inverse dispersion model, and the simulated fluxes were then compared to the actual CH4 release rate. For a source located 60 m from the downwind path, the DCS system detected 10 nmol mol−1 CH4 horizontal concentration gradient above the atmospheric background concentration with a precision of 6 nmol mol−1 in 15 min interval. A CH4 release of 3970 g d−1 was performed, resulting in an average concentration enhancement of 24 nmol mol−1 of CH4. The calculated CH4 flux was 4002 g d−1, showing good agreement with the actual CH4 release rate. Periodically altering the downwind path, which may be needed to track moving cattle, did not adversely affect the ability of the instruments to determine the CH4 flux. These results give us confidence that CH4 flux can be determined by grazing cattle with low disturbance and direct field-scale measurements.
- Article
(3228 KB) - Full-text XML
- BibTeX
- EndNote
Methane (CH4) emissions from enteric fermentation in domestic ruminants is the largest anthropogenic source of CH4 in the United States, with the dairy and beef industries being responsible for most of these emissions (EPA, 2023). Previous life cycle analyses indicate that 70 % to 80 % of the total greenhouse gas (GHG) emissions from the beef sector occur during the grazing phase (Alemu et al., 2017; Rotz et al., 2015; Thompson and Rowntree, 2020). However, direct herd-scale CH4 emission data in grazing systems are scarce. The low animal density and high animal mobility commonly found in most grazing systems makes herd-scale measurements quite challenging (Dengel et al., 2011; Felber et al., 2015; Flesch et al., 2018; Laubach et al., 2016; Stoy et al., 2021). Accurate whole-farm and herd-level measurements of livestock methane emissions are necessary to evaluate mitigation strategies to reduce GHG emissions; improve current GHG national inventories; and assist governments, industries, and other organizations in fulfilling commitments to reducing anthropogenic GHG emissions.
Methane emissions from individual animals have been measured using face masks (Place et al., 2011), head hood chambers (Hill et al., 2016), whole-animal respiration chambers (Pinares-Patiño et al., 2011), tunnels (Lockyer and Jarvis, 1995), automated spot head box measurements (Hristov et al., 2015), and tracer methods (Grainger et al., 2007; Johnson et al., 1994). The respiration chamber is considered the standard technique for measuring livestock CH4 emissions. Results from chamber studies have been used to develop predictive models and equations for national GHG inventories (Danielsson et al., 2017; Ramin and Huhtanen, 2013). However, chambers can create measurement artifacts by affecting animal behavior and are not practical for measuring CH4 emissions from many animals (Storm et al., 2012).
Micrometeorological techniques have been applied for measuring ammonia, carbon dioxide, nitrous oxide, and CH4 emissions from livestock systems (Laubach et al., 2024; McGinn and Flesch, 2018b; Phillips et al., 2007; Prajapati and Santos, 2018b; Sun et al., 2015) and have the advantages of being non-intrusive, being able to integrate fluxes from large areas or herds of cattle reducing measurement uncertainties due to animal-to-animal variability, and providing high temporal resolution (<1 h) flux measurements (McGinn, 2013). The widely used eddy covariance technique has been combined with flux footprint models to estimate methane emissions from ruminant herds (Coates et al., 2017; Dengel et al., 2011; Prajapati and Santos, 2018a; Stoy et al., 2021). However, this approach requires the presence of animals in the flux tower footprint, which makes its implementation challenging in extensive grazing systems where cattle often do not remain for long periods in the area sampled by the flux tower.
Lagrangian stochastic models, which are the basis for several inverse dispersion models (IDMs), have been used to infer emissions of gases such as ammonia and CH4 from agricultural systems (Flesch et al., 2005; Laubach and Kelliher, 2005; McGinn and Flesch, 2018b). Unlike traditional micrometeorological methods, such as the eddy covariance and flux gradient methods, they can handle source areas of different sizes and complex source geometries (Flesch et al., 2005). The IDM proposed by Flesch et al. (1995) has been used to quantify CH4 emissions from ruminants (Flesch et al., 2018; Laubach and Kelliher, 2005; McGinn et al., 2011; Prajapati and Santos, 2018a). In typical IDM applications, open-path line-averaged concentration sensors are placed upwind and downwind of the source of interest. The gas emission rates are then inferred based on the increase in gas concentration downwind of the source and turbulence statistics obtained from wind velocity measurements. McGinn et al. (2011) used IDM to estimate methane emissions from 18 animals grazing in a 1 ha paddock. They measured the area with five different paths ranging from 80 to 128 m in length so that at least one laser path was close enough to the cattle for their open-path system to be able to detect an enhancement in concentration. The main goal of this study is to determine if the dual-comb spectroscopy (DCS) combined with an IDM can precisely infer CH4 flux from a typical herd of cattle grazing on an extensive pasture.
2.1 Dual-comb spectroscopy
Dual-comb spectroscopy is a spectroscopic technique that uses two coherent frequency combs to get molecular concentrations through absorption (Coddington et al., 2016). A frequency comb is a laser spectrum composed of many (106) regularly spaced (MHz) spectral lines known as comb teeth with spectral coverage of multiple terahertz. Two frequency combs with slightly different repetition rates pass through a gas. Atmospheric molecular absorption lines, such as those due to CH4, have gigahertz-wide absorption features and will absorb multiple comb teeth. After passing through the gas, the light from the combs is incident onto a square-law photodetector generating a radio frequency (RF) comb composed of heterodyne beats between pairs of optical comb teeth. From this an electrical interferogram (IGM) is generated, and its Fourier transform provides both the gas absorption and laser spectra. DCS is a sensing tool that combines and enhances the most desirable traits of Fourier transform infrared spectroscopy (FTIR) and tunable diode laser absorption spectroscopy to measure entire absorption bands of multiple gas species at high speed with fine spectral resolution. In particular, DCS offers the unique ability to interrogate kilometer-scale paths and reliably measure very small changes in gas concentration, making DCS potentially valuable for quantifying fluxes of agriculturally significant gases in the field scale.
2.2 Obtaining CH4 mole fractions using spectral line fitting
DCS is commonly used in an open-path differential measurement geometry to measure gas mole fraction on two beam paths to determine CH4 flux from a source area. As seen in Fig. 1a, comb light generated from the DCS system in a trailer is split and sent on upwind and downwind paths. A sample IGM (Fig. 1b), from each path is recorded, and its Fourier transform provides both the gas absorption and laser spectra (Fig. 1c). In order to obtain gas mole fraction, the spectral absorption is fit using a nonlinear curve-fitting routine (Newville et al., 2014) using molecular information from the HITRAN spectral database (Gordon et al., 2017; Rothman et al., 2009). The open-path DCS system used for this study has spectral coverage from 179.8 to 188.9 THz (6000 to 6300 cm−1) and with a spectral resolution of 200.005 MHz (0.00667 cm−1). The system is designed to target CH4, CO2, and water vapor with laboratory-level precision while operating in the field. It is based on all-polarization-maintaining, mode-locked erbium-doped fiber lasers with repetition frequencies of 200 005 000 Hz and 200 005 000 + 208.88 Hz (Sinclair et al., 2015). Mutual comb coherence is established by phase-locking each comb to the same free-running continuous-wave laser at 192.175 THz and by phase-locking the carrier–envelope offset frequency of each comb using an in-line f–2f interferometer (Truong et al., 2016). To tailor the comb spectrum to cover the CH4 absorption band at 181.97 THz, light for each comb is amplified in an erbium-doped fiber amplifier and sent through a short piece of highly nonlinear fiber. For the DCS measurement, the filtered outputs are combined using a fiber combiner generating two outputs that are directed over two open-air paths.
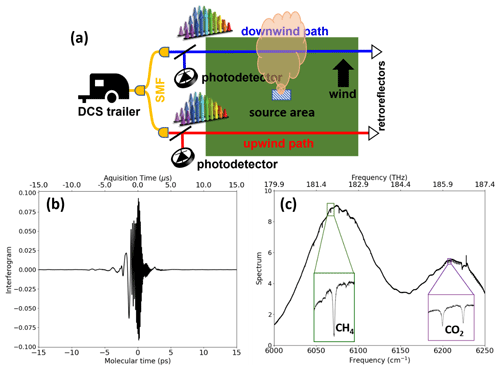
Figure 1(a) Schematic of the dual-comb spectrometer gas concentration measurements on two paths from a CH4 source area. Yellow lines indicate single-mode fiber (SMF) transmitting dual-comb light to an upwind (red) and downwind (blue) open-air paths. CH4 is emitted from an area between the two paths under proper wind directions. RF signals from two photodetectors are sent back to the trailer, and two interferograms (IGM) containing gas concentration information for each path are digitized. (b) A dual-comb spectroscopy phase-corrected IGM after 5 min acquisition time on the upwind path. “Acquisition time” is the microsecond timescale of the measured RF voltage. “Molecular time” is the timescale associated with the period of molecular oscillations, which is typically picoseconds. (c) The Fourier transform of the IGM with insets showing CH4 and CO2 absorption lines and the laser baseline.
Each IGM is digitally sampled with 14 bits and contains 957 500 points. The IGMs are generated at a rate of 208.88 Hz, so streaming and storing these data to a computer would require terabits of storage. To reduce data storage requirements during the course of the measurement 28 IGMs are co-added by a field-programmable gate array (FPGA) to produce a hardware-averaged IGM. These IGMs are streamed to a computer, which performs phase correction and additional averaging using a methodology similar to techniques used in FTIR (Griffiths and de Haseth, 2006). The computer calculates a phase-corrected IGM every 5 min and stores it in the hard drive. For the best case, 2238 hardware-averaged IGMs are used to generate a phase-corrected IGM every 5 min. Hardware-averaged IGMs with poor return power, mostly due to poor alignment between transceiver and the retroreflector, are rejected and not used in the phase correction. Under moderate windy conditions IGM rejection is less than 10 %.
2.3 Lagrangian stochastic model (WindTrax) simulations
2.3.1 Sensitivity and precision required for grazing measurements
A forward Lagrangian stochastic model (WindTrax, Thunderbeach Sci.; Crenna, 2006) was used to simulate the concentration field downwind of a hypothetical herd of 20 head of beef cattle grazing in an area of 25 ha, which is a typical stocking density (animal area) in the Flint Hills region, Kansas (Fig. 2). Wind orthogonal components and temperature data were measured at 10 Hz using a sonic anemometer (CSAT3, Campbell Sci, Logan, UT) deployed 5 m above a grazing unit on the Rannells' Flint Hills Prairie Preserve (full site description below) near Manhattan, Kansas, USA. The wind dataset selected for these simulations consisted of about 30 d in June 2021 during the grazing season. The raw wind data files were processed using the software Eddy Pro (LI-COR, Lincoln, NE), and means, variances, and covariances for wind velocity and sonic temperature data were calculated for 30 min intervals to be used as input variables for the WindTrax simulations. To investigate if the DCS system can resolve the expected increase in CH4 concentration due to the presence of cattle above the typical CH4 atmospheric background level (2000 nmol mol−1), two expected CH4 emission scenarios were evaluated: 100 and 300 g per head per day. These values were selected based on the reported IPCC Tier 1 emission values for grazing cattle in North America of 208 g per head per day (Eggleston et al., 2006). The simulated herd consisted of a fixed grid of point sources spaced 20 m apart (Fig. 2). The height of gas release was set to 1 m above the ground to mimic the height of the animal mouth, and a total of 50 000 particles were released for each point source. Three beam lines were used in this simulation located at 45, 160, and 310 m from the geometric center of the herd.
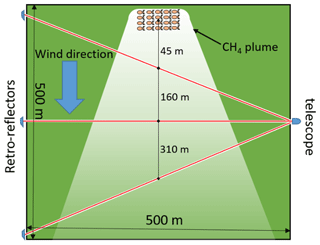
Figure 2Schematic diagram showing the location of the hypothetical herd of cattle, transceiver, retroreflectors, and three possible downwind paths used for the forward WindTrax simulations. A constant background of 2000 nmol mol−1 was assumed so no upwind path was used in the simulation and not shown in the figure.
The forward model predicted that a herd of 20 cattle grazing in an area of 25 ha would produce a CH4 enhancement of 16 nmol mol−1 above a 2000 nmol mol−1 background for a beamline 45 m away from the herd of cattle assuming an emission rate of 300 g per head per day of CH4. The enhancement drops to 2 nmol mol−1 for a beam path 310 m away assuming the same emission rate. For a low-emission scenario (100 g per head per day), the CH4 enhancements ranged from 5 to 1 nmol mol−1 for a beam line located at 45 and 310 m away from the center of the herd.
Table 1Grazing system methane emission WindTrax simulation results showing the expected average and standard deviation (SD) of CH4 concentration measured by line sensors positioned downwind of a cattle herd with two CH4 emission rates. The CH4 background level was assumed to be constant at 2000 nmol mol−1.
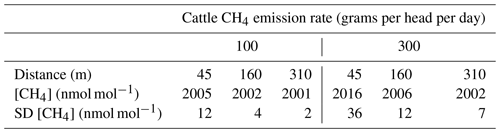
Figure 3a shows spectral data and the results of a H2O, CH4, and CO2 fit. The DCS concentration measurement precision under field conditions was determined using Allen–Werle analysis (Werle, 2011), which includes effects of field-condition-induced misalignment on the retroreflectors that cause fluctuations in the signal-to-noise ratio (SNR). The result of an Allen–Werle analysis on a dataset taken for 24 h on 18 December 2022 is shown in Fig. 3b, showing a precision of 6 nmol mol−1 CH4 in 900 s (15 min) for 200 m paths. This result is consistent with results of (Herman et al., 2021) where data were taken with a SNR of 1000 and a precision of 25 nmol mol−1 in 5 min.
2.3.2 Computing CH4 flux using an inverse dispersion model
WindTrax was also used for computing CH4 fluxes using upwind and downwind CH4 dry mole fractions (Sect. 3.1), and wind velocity data as described in Sect. 2.3.1. As WindTrax flux estimates are more precise for 15 min or longer timescales (Flesch et al., 2004), we averaged the 5 min DCS mole fraction data to 15 min. WindTrax requires appropriate weather conditions to provide accurate estimate of fluxes, so the data were screened based on the following acceptance criteria: wind friction velocity (u*) > 0.1 m s−1 and absolute Monin–Obukhov length values > 10 m (Flesch et al., 2005; Todd et al., 2014). The source area (Fig. 4) used by WindTrax to infer fluxes was set to match the 12.5 m2 area of the CH4 point sources, and the source level was set to 0.7 m above the ground, which is the same height as the manifold outlets. In WindTrax all DCS measurement paths were modeled as line concentration sensors consisting of 60 particle “release” points along the path, starting at the transceiver and ending at the retroreflector. A total of 50 000 particles were released from each of those points for each WindTrax simulation.
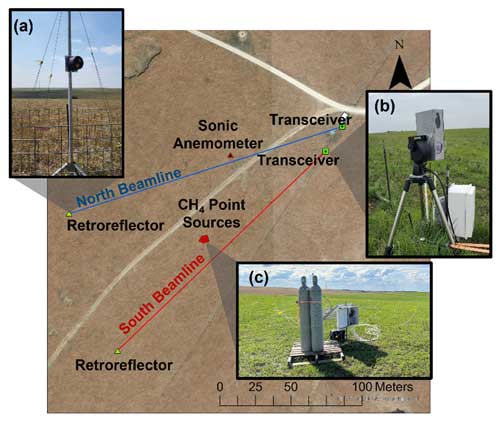
Figure 4Layout of the experimental site at the Rannells' Flint Hills Prairie Preserve. Insets show (a) hollow gold retroreflectors, (b) optical transceiver on a tip–tilt gimbal, and (c) gas manifold and point sources used to release CH4 at a known rate. The one-way path distances were 202 m for the north beamline and 203 m for the south beamline. Image credit ArcGIS® (software by Esri). ArcGIS® and ArcMap™ are the intellectual property of Esri and are used herein under license. Copyright ©Esri.
One of the principal sources of uncertainty in the IDM estimates arises from the errors in the gas concentration measurements themselves. The flux is dependent on the difference between downwind (rd) and upwind (ru) dry mole fractions which are measured along the north and south beamlines (Fig. 4). The fractional uncertainty in the flux is given by (Herman et al., 2021)
where F is the flux, σF is flux error, is downwind (background) dry mole fraction error, is upwind dry mole fraction, cov(d,u) is the covariance of the downwind and upwind errors. A covariance term was added to the quadrature error following previous studies (Bai et al., 2022; Herman et al., 2021) to account for small correlations in the different path errors. The errors in the dry mole fractions ( and ) and the covariance were determined from the recorded 5 min measured SNR assuming that the mole fraction error is inversely proportional to the SNR. The fractional uncertainty ignores errors due to measurement dead time, wind field measurements, and IDM inherent uncertainties (Flesch et al., 2004). Typical values of fractional uncertainty in the flux vary from 20 % to 30 %.
3.1 Description
Controlled CH4 release field experiments were conducted on the Rannells' Flint Hills Prairie Preserve (hereafter Rannells' ranch) near Manhattan, Kansas USA (39°08′ 28′′ N, 96°31′31′′ W, 324 m a.s.l.). The dominant steer grazing system in the Kansas Flint Hills is Intensive Early Stocking (IES) (Smith and Owensby, 1978). IES is a grazing system that takes advantage of the early summer high-quality forage by stocking at twice the normal season-long stocking rate (1.25 steers per hectare) for the first half of the growing season (∼ 1 May–∼ 15 July) with no grazing during the last half. The grazing unit used in the study has 31 ha and has been annually burned in late April each year. This grazing unit was selected because its topography is suitable for micrometeorological measurements and because it allows unobstructed paths for the DCS system for most of its extension.
Previous work (Alden et al., 2019; Coburn et al., 2018) used DCS to measure simulated CH4 leaks from oil and gas production at the level of 1400 g d−1 from a distance of 1 km. Here we seek to provide a similar verification of the technology but with two important changes in the measurement configuration appropriate for livestock-based methane sources. The sources will be distributed rather than concentrated to single point source, and the sources are further from the measurement paths. This larger separation will be necessary to accommodate the fact that the herd will wander over time. The measurement paths might be adjusted to accommodate the cattle movement, but there will be a limit to how close the measurement paths can be kept from the source.
The DCS system was housed in a temperature-controlled trailer at the Rannells' Ranch, as seen in Fig. 4. Single-mode telecommunication fibers (Corning SMF-28) with lengths of 10 and 40 m carried the dual-comb laser output light to two telescope transceivers (Fig. 4b) that were used to send comb light across the north (blue) and south (red) beamlines. The transceiver consisted of a fixed-connection angled physical contact (FC/APC) fiber termination followed by a collimating 179 mm focal length, 102 mm diameter, 45° off-axis parabolic mirror, resulting in a collimated beam of ∼ 35 mm diameter. Eye-safe (< 10 mW) collimated dual-comb light was directed with a 127 mm clear aperture, 5 arcsec gold retroreflector (Edmund Optics1) positioned 200 m away (Fig. 4a), and the reflected signal was focused onto a 150 MHz bandwidth photodetector (PDA10CF, Thorlabs, Newton, NJ) in the transceivers. RF signals from photodetectors were transmitted to the trailer through RF cables (RG58, Pasternack Irvine, CA) and digitized using a 14-bit digitizer (FMC104, Abaco Systems, Huntsville, AL). To remove any concentration bias due to digitizer nonlinearities we added a dither signal to the received DCS interferogram (Malarich et al., 2023). The dither improved the individual channel precision by 5 % and reduced the differences between channels to below 3 nmol mol−1.
Both transceivers were mounted on motorized tip–tilt gimbals (PT100, FLIR, Wilsonville, OR) that were automatically aligned using a datalogger (CR1000x, Campbell Sci.) or personal computer algorithms to the retroreflectors based on the return DC signal from the photodetector. The transceiver also housed a visible camera (BFLY-PGE-50A2M-CS, FLIR) to aid with alignment and a consumer 5 W 850 nm LED flashlight to allow the user to see the retroreflectors with the visible camera during nighttime. The datalogger-controlled alignment system was able to maintain sufficient power back from the retroreflector to the transceiver in moderate wind conditions for over 24 h. The wind velocity orthogonal components and temperature were measured using a sonic anemometer (CSAT3 Campbell Sci.) at 5 m above the ground. The sonic anemometer was connected to a datalogger (CR3000, Campbell Sci.), and the raw data were saved at 10 Hz. The positions of the retroreflectors, manifold, sonic anemometer, and transceiver were measured using a multi-band real-time kinematic positioning (RTK) receiver (Reach RS2+, Emlid, Budapest, Hungary) with 7 and 14 mm horizontal and vertical accuracies, respectively. The horizontal and vertical coordinates obtained for the transceivers and retroreflectors were then used to determine the path lengths shown in Fig. 4.
A custom-built gas manifold (Fig. 4c) was used to control the release of CH4 through 10 point sources located within the two DCS beam lines. Methane gas from a compressed tank (99.97 % purity) was delivered to a proportional solenoid valve (PVQ13, SMC, Noblesville, IN) using a two-stage pressure regulator and high-density polyethylene tubing (internal diameter 5.3 mm). The proportional valve was then connected to a multi-port aluminum manifold using high-density polyethylene tubing. The pressure inside the manifold was monitored using a pressure transducer (PX119-030GI, Omega, Norwalk, CT). The CH4 from the manifold flowed through 10 individual 0.254 mm precision orifice assemblies (K2-10-SS, O'Keefe Controls Co., Monroe, CT). The precision orifice assemblies were then connected to 8 m high-density polyethylene tubing lengths. The other extremity of these plastic tubes was then attached to metal rods at a height of 0.7 m above the ground. During CH4 controlled-release campaigns, the pressure inside the manifold was adjusted to provide the desired flow rate by controlling the voltage applied to the proportional valve using a datalogger (CR1000, Campbell Sci.). A feedback loop between the proportional valve and pressure transducer ensured a constant pressure inside the manifold during the control release campaigns. The CH4 tank was weighted in the beginning and end of the gas release campaigns, and the mass of gas released was determined gravimetrically using a scale (D125WQL, Ohaus, Parsippany, NJ). We used the mass given by the scale to determine the amount of gas released in each release campaign since it provides a more direct estimate of the release rate than the one obtained using the gas manifold. Previous gas release studies have successfully used scale data to verify the flow rate of mass flow controller (Coates et al., 2017).
Mole fractions (χ) of CO2, H2O, and CH4 were obtained from the measured interferogram using a fit model derived from a combination of the HITRAN databases (Rothman et al., 2013) and the cepstral domain technique (Cole et al., 2019). Temperature and pressure data used as an initial guess for the fit were provided by the sonic anemometer (CSAT3) and a pressure transducer (CS100, Campbell Sci.), respectively, which were both located on the same tower during the measurement campaign. The spectral band used in the cepstral domain fitting was from 6000 to 6300 cm−1 and contains CH4, H2O, and weak CO2 absorption lines. HITRAN 2008 (Rothman et al., 2009) molecular parameters for CH4 with a Voigt line shape were computed using the HITRAN Application Programming Interface (Kochanov et al., 2016). Line strengths greater than 10−22 cm−1 molec.−1 cm2 were used. A cepstral domain filter operates in the time domain and removes the broad comb baseline structure in the IGM at times shorter than 15 ps and an etalon feature from 30 to 40 ps. The conversion from CH4 mole fraction () to dry mole fraction () was calculated using the fit H2O mole fraction () and
3.2 Results from controlled CH4 release measurements
Data from a CH4 release at a rate of 3078 g d−1, equivalent to 15 head of grazing cattle, are shown in Fig. 5. The wind speed showed high variability, with minimum and maximum values equal to 0.7 and 7.6 m s−1, respectively (Fig. 5a). CH4 mole fraction was measured at 5 min intervals for the north and south laser beamlines, as seen in Fig. 4. The CH4 gas release started at 10:30 Central Time (CT, UTC−6) and ended at 18:00 CT on 4 February 2023. The enhancement is given by rd–ru (Fig. 5c). The small 10 nmol mol−1 average enhancement can be seen fluctuating around a 2026 nmol mol−1 average background concentration. However, the wind speed affected the ability of the DCS to measure these small concentration enhancements by diluting the methane plume, as can be seen when the wind speed values were high during the afternoon of 4 February 2023. The two-path DCS measurement was also capable of capturing the temporal dynamics of the CH4 background driven by changes in atmospheric boundary layer conditions.
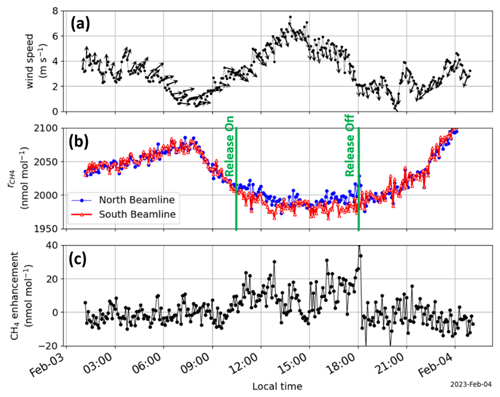
Figure 5Measured 5 min values of (a) wind speed and direction, (b) dry CH4 mole fraction (), and (c) enhancement during a controlled CH4 release of 3078 g d−1 equivalent to 15 head of cattle assuming a CH4 rate of 208 g per head per day. Wind arrows point in the direction from which the wind is blowing. During the release wind was mostly coming from the south, causing an enhancement on the north beamline.
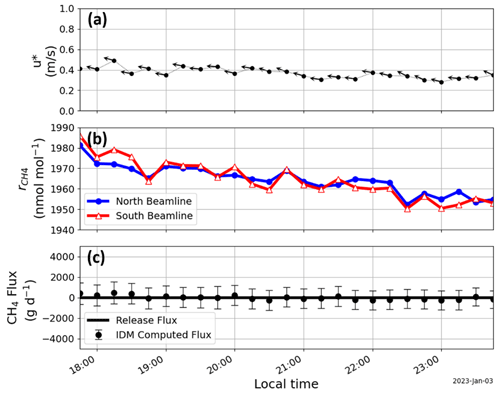
Figure 6Time series of (a) wind friction velocity and direction, (b) dry CH4 mole fraction (), and (c) release, where IDM computed a case with no released gas for CH4 flux. The error bars are uncertainties due to the DCS-measured concentrations calculated using Eq. (2). Wind arrows point in the direction from which the wind is blowing.
We used downwind and upwind DCS concentration measurements for a period with no gas release to determine if any concentration biases exist between the north and south beamlines that may lead to incorrect flux values and to estimate the precision of CH4 fluxes inferred using DCS and WindTrax. North and south measurements were taken over 6.25 h with no gas released and the wind from the west (Fig. 6). CH4 dry mole fraction and WindTrax were used to compute an average CH4 flux of 1.3 g d−1 and a standard deviation of ±217.5 g d−1. This standard deviation value is equivalent to approximately one head of cattle, assuming an emission rate of 200 g per head per day.
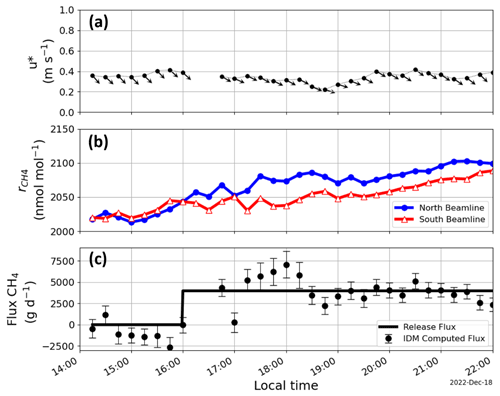
Figure 7Time series of (a) wind friction velocity and direction, (b) CH4 dry mole fraction (), and (c) release, where IDM computed the CH4 flux for a CH4 release of 3970 g d−1, which is equivalent to 19 head of cattle. Wind arrows point in the direction from which the wind is blowing.
To test if the DCS measurement can be used to correctly reproduce the release flux rate, a controlled CH4 release corresponding to 3970 g d−1, which simulates a 19-head cattle herd with an emission rate of 200 g per head per day, was performed, where DCS-measured concentrations and 3D wind statistics were measured for 6 h (Fig. 7). DCS dry mole fractions and wind data for 15 min intervals were then used to estimate CH4 fluxes using WindTrax. The DCS system was able to detect the small 24 nmol mol−1 average enhancement above the 2041 nmol mol−1 average background concentration. WindTrax computed average CH4 flux was 4002 g d−1 and the flux uncertainty due to DCS concentration errors (Eq. 2) ±1498 g d−1, showing a good agreement to the actual release CH4 flux of 3970 g d−1. As a point of comparison, Harper et al. (2010) summarized the accuracy of IDM in 13 controlled release studies. They expressed the IDM accuracy using a recovered rate given by () × 100 and found an average recovery rate of 95 % for all the studies. We estimated our recovery rate to be 100.8 () using the data shown in Fig. 7a. This is a noteworthy result indicating that the combination of DCS with IDM can produce flux estimates with high accuracy.
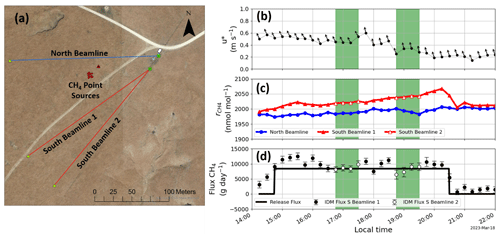
Figure 8(a) Layout of the experimental site the Rannells' Flint Hills Prairie Preserve used to alternate between two downwind south paths. Time series of (b) wind friction velocity and direction, (c) CH4 dry mole fraction and interferogram signal-to-noise ratio, and (d) release and IDM-computed CH4 flux for a release of 8396 g d−1. The red triangle indicates the position of the 3D sonic anemometer. Wind arrows point in the direction from which the wind is blowing. The downwind south beamline was changed during the release and focused on Retroreflector 2 from 16:45 to 17:30 CT and 18:45 to 19:30 CT (indicated by shaded green regions) and on Retroreflector 1 at all other times. Moving between the two downwind paths did not distort the concentration measurement or the computed IDM flux compared to the release rate. Image credit ArcGIS® (software by Esri). ArcGIS® and ArcMap™ are the intellectual property of Esri and are used herein under license. Copyright ©Esri.
Monitoring grazing cattle emissions in the field will require changing between laser paths to capture emissions from a moving herd. To investigate the effect of the distance between the herd and the beam paths, we alternated between two downwind south paths (Fig. 8). Here a release simulating 40 head was performed where the downwind south path was changed at hourly intervals during the release. Figure 8 shows the measured wind conditions (Fig. 8a), CH4 dry mole fraction (Fig. 8b), and release and IDM-computed CH4 flux (Fig. 8c). During the measurement, the downwind path altered between south beamline 1 and 2 as a function of time. The good comparison between the measured and calculated flux using both south beamlines demonstrated that altering the beam paths did not adversely impact our ability to determine a flux from the source area.
The agreement between the computed and actual CH4 fluxes in this study shows that DCS can precisely measure the small CH4 concentration enhancements due to a herd of beef cattle in the field at distances up to 100 m from the source area. Our ability to measure results shows that the DCS precision is limited to the ability to maintain sufficient laser alignment between transceiver and retroreflector. A robust transceiver design and housing and a fast-response datalogger-controlled gimbal alignment are critical to make continuous measurements under turbulent and varying environmental conditions.
In addition to the good precision, other important characteristics of the DCS measurement were highlighted in this study: (1) the use of inexpensive (USD 1.3 per meter) and robust telecommunication-grade fiber optics (SMF28) to transport the light from the DCS to outdoor transceivers over long distances (tens to hundreds of meters) with very low power losses (4.5 % loss per kilometer) and (2) its ability to measure multiple open atmospheric laser beamlines simultaneously with a single instrument. From a pure measurement standpoint, using a single instrument to measure gradients of concentration is desirable to eliminate measurement biases. For example, cross-calibrations are often necessary when using multiple line-average sensors to perform multi-path gas concentration measurements. The minimization of instrument biases is crucial when combining the DCS with existing micrometeorological techniques that utilize of vertical or horizontal gradients of concentration to infer fluxes (Flesch et al., 1995; McGinn and Flesch, 2018a). Expected CH4 horizontal gradients in grazing systems are often small, as demonstrated in this study, so small instrument biases can lead to large errors when inferring fluxes. Furthermore, the use of a single instrument to measure multiple source areas will also lead to a reduction in the cost necessary to evaluate multiple treatments. This is particularly important when assessing GHG mitigation strategies, which often require evaluation of multiple treatments and management practices simultaneously.
The driving rationale of this work is to quantify the net CH4 fluxes produced by cattle grazing system, which will require measuring wind velocity and CH4 concentration enhancements upwind and downwind of the animals over long periods. Although soil CH4 fluxes are expected to be smaller than animal emissions, they could be important for estimating whole-system CH4 budgets. Separating animal and soil contributions into the net CH4 fluxes will require a combination of measuring approaches, such as chamber and micrometeorological measurements (e.g., eddy covariance measurements). High animal mobility in extensive grazing systems will also pose additional challenges for the quantification of cattle emissions. The instrument's current inability to track cattle for laser-based greenhouse gas detection is an open and significant problem. Animal tracking using GPS collars (Felber et al., 2015) or digital photographs (Stoy et al., 2021) have been used to track ruminants in grazing systems. Both approaches have their own challenges, GPS collars need to provide high-accuracy and high-temporal-resolution spatial data while consuming low power to allow animals to be monitored during an entire grazing season. Wide-angle-camera images were used to determine the position of the cattle herd during summer 2023 with limited success (data not shown), since it was difficult to properly discern animal positions with the level of spatial resolution needed for the IDM. Ideally, real-time animal tracking using GPS collars, digital images, or a combination of both could be used to improve flux estimate accuracies. This could be done by subdividing the grazing system into smaller monitoring areas. The area monitored by the DCS system could be selected by aiming the laser beam at different retroreflectors installed at different points of the pasture. By monitoring these small areas, it would be possible to keep the downwind laser beam close to the animals, which would thus measure a larger CH4 concentration enhancement and reduce the uncertainties in concentration measurements. The ability of DCS to measure gases from a large area continuously will permit monitoring of CH4 emissions from a slow-moving herd of cattle, providing precise CH4 flux values to improve agricultural GHG inventories and management practices.
Data associated with this paper are publicly available at https://doi.org/10.18434/mds2-3139 (Washburn, 2024) or can be obtained from the authors upon a reasonable request.
IC, BDD, KCC, SMW, CB, CEO, NRN, EAS, and BRW conceived of and designed the experiments. EAS, DIH, NAM, and BRW built the DCS system. FRG and BRW wrote DCS data acquisition code. NAM and BRW wrote the code used for spectral line fitting. CW, LCM, EAS, and BRW performed the experiments and analyzed the data. CEO is the manager of the Rannells' Ranch. LCM, CW, KCC, EAS, and BRW performed the experiments and analyzed the DCS data. CW and EAS implemented the inverse dispersion model. BRW and EAS supervised the project. All authors contributed to the writing of this manuscript.
The contact author has declared that none of the authors has any competing interests.
Publisher's note: Copernicus Publications remains neutral with regard to jurisdictional claims made in the text, published maps, institutional affiliations, or any other geographical representation in this paper. While Copernicus Publications makes every effort to include appropriate place names, the final responsibility lies with the authors.
This work was partially funded by the NSF Division of Biological Infrastructure (award no. 1726304), the William and Joan Porter Endowment, the Habiger Heritage Fund, and NIST. This is contribution no. 25-069-J from the Kansas Agricultural Experiment Station.
This research has been supported by the National Science Foundation (grant no. 1726304), the William and Joan Porter Endowment, the Habiger Heritage Fund, and NIST.
This paper was edited by David Griffith and reviewed by two anonymous referees.
Alden, C. B., Coburn, S. C., Wright, R. J., Baumann, E., Cossel, K., Perez, E., Hoenig, E., Prasad, K., Coddington, I., and Rieker, G. B.: Single-Blind Quantification of Natural Gas Leaks from 1 km Distance Using Frequency Combs, Environ. Sci. Technol., 53, 2908–2917, https://doi.org/10.1021/acs.est.8b06259, 2019.
Alemu, A. W., Janzen, H., Little, S., Hao, X., Thompson, D. J., Baron, V., Iwaasa, A., Beauchemin, K. A., and Kröbel, R.: Assessment of grazing management on farm greenhouse gas intensity of beef production systems in the Canadian Prairies using life cycle assessment, Agr. Syst., 158, 1–13, https://doi.org/10.1016/j.agsy.2017.08.003, 2017.
Bai, M., Loh, Z., Griffith, D. W. T., Turner, D., Eckard, R., Edis, R., Denmead, O. T., Bryant, G. W., Paton-Walsh, C., Tonini, M., McGinn, S. M., and Chen, D.: Performance of open-path lasers and Fourier transform infrared spectroscopic systems in agriculture emissions research, Atmos. Meas. Tech., 15, 3593–3610, https://doi.org/10.5194/amt-15-3593-2022, 2022.
Coates, T. W., Flesch, T. K., McGinn, S. M., Charmley, E., and Chen, D.: Evaluating an eddy covariance technique to estimate point-source emissions and its potential application to grazing cattle, Agr. Forest Meteorol., 234, 164–171, 2017.
Coburn, S., Alden, C. B., Wright, R., Cossel, K., Baumann, E., Truong, G.-W., Giorgetta, F., Sweeney, C., Newbury, N. R., Prasad, K., Coddington, I., and Rieker, G. B.: Regional trace-gas source attribution using a field-deployed dual frequency comb spectrometer, Optica, 5, 320–327, https://doi.org/10.1364/OPTICA.5.000320, 2018.
Coddington, I., Newbury, N., and Swann, W.: Dual-comb spectroscopy, Optica, 3, 414–426, https://doi.org/10.1364/OPTICA.3.000414, 2016.
Cole, R. K., Makowiecki, A. S., Hoghooghi, N., and Rieker, G. B.: Baseline-free quantitative absorption spectroscopy based on cepstral analysis, Opt. Express, 27, 37920, https://doi.org/10.1364/OE.27.037920, 2019.
Crenna, B.: An introduction to WindTrax, University of Alberta, http://thunderbeachscientific.com/downloads/introduction.pdf (last access: 7 October 2024), 2006.
Danielsson, R., Ramin, M., Bertilsson, J., Lund, P., and Huhtanen, P.: Evaluation of a gas in vitro system for predicting methane production in vivo, J. Dairy Sci., 100, 8881–8894, 2017.
Dengel, S., Levy, P. E., Grace, J., Jones, S. K., and Skiba, U. M.: Methane emissions from sheep pasture, measured with an open-path eddy covariance system, Global Change Biol., 17, 3524–3533, https://doi.org/10.1111/j.1365-2486.2011.02466.x, 2011.
Eggleston, S., Buendia, L., Miwa, K., Mgara, T., and Tanabe, K.: Emissions from Livestock and Manure Management, in: 2006 IPCC Guidelines for National Greenhouse Gas Inventories, Vol. 4, Institute for Global Environmental Strategies, 87 pp., https://www.ipcc-nggip.iges.or.jp/public/2006gl/pdf/4_Volume4/V4_10_Ch10_Livestock.pdf (last access: 7 October 2024), 2006.
EPA: Inventory of U.S. greenhouse gas emissions and sinks: 1990–2021, 881 pp., https://www.epa.gov/ghgemissions/inventory-us-greenhouse-gas-emissions-and-sinks-1990-2021 (last access: 7 October 2024), 2023.
Felber, R., Münger, A., Neftel, A., and Ammann, C.: Eddy covariance methane flux measurements over a grazed pasture: effect of cows as moving point sources, Biogeosciences, 12, 3925–3940, https://doi.org/10.5194/bg-12-3925-2015, 2015.
Flesch, T., Wilson, J., Harper, L., and Crenna, B.: Estimating gas emissions from a farm with an inverse-dispersion technique, Atmos. Environ., 39, 4863–4874, https://doi.org/10.1016/j.atmosenv.2005.04.032, 2005.
Flesch, T. K., Wilson, J. D., and Yee, E.: Backward-time Lagrangian stochastic dispersion models and their application to estimate gaseous emissions, J. Appl. Meteorol. Climatol., 34, 1320–1332, 1995.
Flesch, T. K., Wilson, J. D., Harper, L. A., Crenna, B. P., and Sharpe, R. R.: Deducing Ground-to-Air Emissions from Observed Trace Gas Concentrations: A Field Trial with Wind Disturbance, J. Appl. Meteorol., 43, 475–484, https://doi.org/10.1175/jam2214.1, 2004.
Flesch, T. K., Basarab, J. A., Baron, V. S., Wilson, J. D., Hu, N., Tomkins, N. W., and Ohama, A. J.: Methane emissions from cattle grazing under diverse conditions: An examination of field configurations appropriate for line-averaging sensors, Agr. Forest Meteorol., 258, 8–17, https://doi.org/10.1016/j.agrformet.2017.10.012, 2018.
Gordon, I. E., Rothman, L. S., Hill, C., Kochanov, R. V., Tan, Y., Bernath, P. F., Birk, M., Boudon, V., Campargue, A., Chance, K. V., Drouin, B. J., Flaud, J.-M., Gamache, R. R., Hodges, J. T., Jacquemart, D., Perevalov, V. I., Perrin, A., Shine, K. P., Smith, M.-A. H., Tennyson, J., Toon, G. C., Tran, H., Tyuterev, V. G., Barbe, A., Császár, A. G., Devi, V. M., Furtenbacher, T., Harrison, J. J., Hartmann, J.-M., Jolly, A., Johnson, T. J., Karman, T., Kleiner, I., Kyuberis, A. A., Loos, J., Lyulin, O. M., Massie, S. T., Mikhailenko, S. N., Moazzen-Ahmadi, N., Müller, H. S. P., Naumenko, O. V., Nikitin, A. V., Polyansky, O. L., Rey, M., Rotger, M., Sharpe, S. W., Sung, K., Starikova, E., Tashkun, S. A., Auwera, J. V., Wagner, G., Wilzewski, J., Wcisło, P., Yu, S., and Zak, E. J.: The HITRAN2016 molecular spectroscopic database, J. Quant. Spectrosc. Ra., 203, 3–69, https://doi.org/10.1016/j.jqsrt.2017.06.038, 2017.
Grainger, C., Clarke, T., McGinn, S. M., Auldist, M. J., Beauchemin, K. A., Hannah, M. C., Waghorn, G. C., Clark, H., and Eckard, R. J.: Methane emissions from dairy cows measured using the sulfur hexafluoride (SF6) tracer and chamber techniques, J. Dairy Sci., 90, 2755–2766, https://doi.org/10.3168/jds.2006-697, 2007.
Griffiths, P. and de Haseth, J.: Fourier Transform Infrared Spectrometry, John Wiley & Sons, Inc, 375–393, https://doi.org/10.1002/9780470106310.ch18, 2006.
Harper, L. A., Flesch, T. K., Weaver, K. H., and Wilson, J. D.: The Effect of Biofuel Production on Swine Farm Methane and Ammonia Emissions, J. Environ. Qual., 39, 1984–1992, https://doi.org/10.2134/jeq2010.0172, 2010.
Herman, D. I., Weerasekara, C., Hutcherson, L. C., Giorgetta, F. R., Cossel, K. C., Waxman, E. M., Colacion, G. M., Newbury, N. R., Welch, S. M., DePaola, B. D., Coddington, I., Santos, E. A., and Washburn, B. R.: Precise multispecies agricultural gas flux determined using broadband open-path dual-comb spectroscopy, Sci Adv, 7, eabe9765, https://doi.org/10.1126/sciadv.abe9765, 2021.
Hill, J., McSweeney, C., Wright, A.-D. G., Bishop-Hurley, G., and Kalantar-zadeh, K.: Measuring methane production from ruminants, Trend. Biotechnol., 34, 26–35, 2016.
Hristov, A. N., Oh, J., Giallongo, F., Frederick, T., Weeks, H., Zimmerman, P. R., Harper, M. T., Hristova, R. A., Zimmerman, R. S., and Branco, A. F.: The Use of an Automated System (GreenFeed) to Monitor Enteric Methane and Carbon Dioxide Emissions from Ruminant Animals, J. Vis. Exp., 103, 52904, https://doi.org/10.3791/52904, 2015.
Johnson, K., Huyler, M., Westberg, H., Lamb, B., and Zimmerman, P.: Measurement of methane emissions from ruminant livestock using a sulfur hexafluoride tracer technique, Environ. Sci. Technol., 28, 359–362, 1994.
Kochanov, R. V., Gordon, I. E., Rothman, L. S., Wcisło, P., Hill, C., and Wilzewski, J. S.: HITRAN Application Programming Interface (HAPI): A comprehensive approach to working with spectroscopic data, J. Quant. Spectrosc. Ra., 177, 15–30, https://doi.org/10.1016/j.jqsrt.2016.03.005, 2016.
Laubach, J. and Kelliher, F. M.: Methane emissions from dairy cows: Comparing open-path laser measurements to profile-based techniques, Agr. Forest Meteorol., 135, 340–345, https://doi.org/10.1016/j.agrformet.2005.11.014, 2005.
Laubach, J., Barthel, M., Fraser, A., Hunt, J. E., and Griffith, D. W. T.: Combining two complementary micrometeorological methods to measure CH4 and N2O fluxes over pasture, Biogeosciences, 13, 1309–1327, https://doi.org/10.5194/bg-13-1309-2016, 2016.
Laubach, J., Flesch, T. K., Ammann, C., Bai, M., Gao, Z., Merbold, L., Campbell, D. I., Goodrich, J. P., Graham, S. L., Hunt, J. E., Wall, A. M., and Schipper, L. A.: Methane emissions from animal agriculture: Micrometeorological solutions for challenging measurement situations, Agr. Forest Meteorol., 350, 109971, https://doi.org/10.1016/j.agrformet.2024.109971, 2024.
Lockyer, D. and Jarvis, S.: The measurement of methane losses from grazing animals, Environ. Pollut., 90, 383–390, 1995.
Malarich, N. A., Cossel, K. C., Deschenes, J.-D., Giorgetta, F. R., Washburn, B. R., Newbury, N. R., Genest, J., and Coddington, I.: Removing biases in dual frequency comb spectroscopy due to digitizer nonlinearity, Opt. Express, 31, 29074, https://doi.org/10.1364/OE.497497, 2023.
McGinn, S. and Flesch, T.: A Technique for Estimating Greenhouse Gas Exchange Adjacent Cattle Feedlots, Atmosphere, 9, 139, https://doi.org/10.3390/atmos9040139, 2018a.
McGinn, S. M.: Developments in micrometeorological methods for methane measurements, Animal, 7, 386–393, https://doi.org/10.1017/S1751731113000657, 2013.
McGinn, S. M. and Flesch, T. K.: Ammonia and greenhouse gas emissions at beef cattle feedlots in Alberta Canada, Agr. Forest Meteorol., 258, 43–49, https://doi.org/10.1016/j.agrformet.2018.01.024, 2018b.
McGinn, S. M., Turner, D., Tomkins, N., Charmley, E., Bishop-Hurley, G., and Chen, D.: Methane Emissions from Grazing Cattle Using Point-Source Dispersion, J. Environ. Qual., 40, 22–27, https://doi.org/10.2134/jeq2010.0239, 2011.
Newville, M., Stensitzki, T., Allen, D., and Ingargiola, A.: LMFIT: Non-Linear Least-Square Minimization and Curve-Fitting for Python, Zenodo [code], https://doi.org/10.5281/zenodo.11813, 2014.
Phillips, F., Leuning, R., Baigent, R., Kelly, K., and Denmead, O.: Nitrous oxide flux measurements from an intensively managed irrigated pasture using micrometeorological techniques, Agr. Forest Meteorol., 143, 92–105, 2007.
Pinares-Patiño, C., Lassey, K., Martin, R., Molano, G., Fernandez, M., MacLean, S., Sandoval, E., Luo, D., and Clark, H.: Assessment of the sulphur hexafluoride (SF6) tracer technique using respiration chambers for estimation of methane emissions from sheep, Anim. Feed Sci. Tech., 166, 201–209, 2011.
Place, S. E., Pan, Y., Zhao, Y., and Mitloehner, F. M.: Construction and operation of a ventilated hood system for measuring greenhouse gas and volatile organic compound emissions from cattle, Animals, 1, 433–446, 2011.
Prajapati, P. and Santos, E. A.: Comparing methane emissions estimated using a backward-Lagrangian stochastic model and the eddy covariance technique in a beef cattle feedlot, Agr. Forest Meteorol., 256–257, 482–491, https://doi.org/10.1016/j.agrformet.2018.04.003, 2018a.
Prajapati, P. and Santos, E. A.: Estimating methane emissions from beef cattle in a feedlot using the eddy covariance technique and footprint analysis, Agr. Forest Meteorol., 258, 18–28, https://doi.org/10.1016/j.agrformet.2017.08.004, 2018b.
Ramin, M. and Huhtanen, P.: Development of equations for predicting methane emissions from ruminants, J. Dairy Sci., 96, 2476–2493, 2013.
Rothman, L. S., Gordon, I. E., Barbe, A., Benner, D. C., Bernath, P. E., Birk, M., Boudon, V., Brown, L. R., Campargue, A., Champion, J. P., Chance, K., Coudert, L. H., Dana, V., Devi, V. M., Fally, S., Flaud, J. M., Gamache, R. R., Goldman, A., Jacquemart, D., Kleiner, I., Lacome, N., Lafferty, W. J., Mandin, J. Y., Massie, S. T., Mikhailenko, S. N., Miller, C. E., Moazzen-Ahmadi, N., Naumenko, O. V., Nikitin, A. V., Orphal, J., Perevalov, V. I., Perrin, A., Predoi-Cross, A., Rinsland, C. P., Rotger, M., Simeckova, M., Smith, M. A. H., Sung, K., Tashkun, S. A., Tennyson, J., Toth, R. A., Vandaele, A. C., and Vander Auwera, J.: The HITRAN 2008 molecular spectroscopic database, J. Quant. Spectrosc. Ra., 110, 533–572, https://doi.org/10.1016/j.jqsrt.2009.02.013, 2009.
Rothman, L. S., Gordon, I. E., Babikov, Y., Barbe, A., Chris Benner, D., Bernath, P. F., Birk, M., Bizzocchi, L., Boudon, V., Brown, L. R., Campargue, A., Chance, K., Cohen, E. A., Coudert, L. H., Devi, V. M., Drouin, B. J., Fayt, A., Flaud, J.-M., Gamache, R. R., Harrison, J. J., Hartmann, J.-M., Hill, C., Hodges, J. T., Jacquemart, D., Jolly, A., Lamouroux, J., Le Roy, R. J., Li, G., Long, D. A., Lyulin, O. M., Mackie, C. J., Massie, S. T., Mikhailenko, S., Müller, H. S. P., Naumenko, O. V., Nikitin, A. V., Orphal, J., Perevalov, V., Perrin, A., Polovtseva, E. R., Richard, C., Smith, M. A. H., Starikova, E., Sung, K., Tashkun, S., Tennyson, J., Toon, G. C., Tyuterev, V. G., and Wagner, G.: The HITRAN 2012 molecular spectroscopic database, J. Quant. Spectrosc. Ra., 130, 4–50, https://doi.org/10.1016/j.jqsrt.2013.07.002, 2013.
Rotz, C. A., Asem-Hiablie, S., Dillon, J., and Bonifacio, H.: Cradle-to-farm gate environmental footprints of beef cattle production in Kansas, Oklahoma, and Texas, J. Anim. Sci., 93, 2509–2519, https://doi.org/10.2527/jas.2014-8809, 2015.
Sinclair, L. C., Deschênes, J.-D., Sonderhouse, L., Swann, W. C., Khader, I. H., Baumann, E., Newbury, N. R., and Coddington, I.: Invited Article: A compact optically coherent fiber frequency comb, Rev. Sci. Instrum., 86, 081301, https://doi.org/10.1063/1.4928163, 2015.
Smith, E. F. and Owensby, C. E.: Intensive-Early Stocking and Season-Long Stocking of Kansas Flint Hills Range, J. Range Manage., 31, 14, https://doi.org/10.2307/3897624, 1978.
Storm, I. M. L. D., Hellwing, A. L. F., Nielsen, N. I., and Madsen, J.: Methods for measuring and estimating methane emission from ruminants, Animals, 2, 160–183, https://doi.org/10.3390/ani2020160, 2012.
Stoy, P. C., Cook, A. A., Dore, J. E., Kljun, N., Kleindl, W., Brookshire, E. N. J., and Gerken, T.: Methane efflux from an American bison herd, Biogeosciences, 18, 961–975, https://doi.org/10.5194/bg-18-961-2021, 2021.
Sun, K., Tao, L., Miller, D. J., Zondlo, M. A., Shonkwiler, K. B., Nash, C., and Ham, J. M.: Open-path eddy covariance measurements of ammonia fluxes from a beef cattle feedlot, Agr. Forest Meteorol., 213, 193–202, https://doi.org/10.1016/j.agrformet.2015.06.007, 2015.
Thompson, L. R. and Rowntree, J. E.: Invited Review: Methane sources, quantification, and mitigation in grazing beef systems, Appl. Animal Sci., 36, 556–573, https://doi.org/10.15232/aas.2019-01951, 2020.
Todd, R. W., Altman, M. B., Cole, N. A., and Waldrip, H. M.: Methane Emissions from a Beef Cattle Feedyard during Winter and Summer on the Southern High Plains of Texas, J. Environ. Qual., 43, 1125–1130, https://doi.org/10.2134/jeq2013.09.0386, 2014.
Truong, G.-W., Waxman, E. M., Cossel, K. C., Baumann, E., Klose, A., Giorgetta, F. R., Swann, W. C., Newbury, N. R., and Coddington, I.: Accurate frequency referencing for fieldable dual-comb spectroscopy, Opt. Express, 24, 30495–30504, https://doi.org/10.1364/OE.24.030495, 2016.
Washburn, B.: Using Open-Path Dual-Comb Spectroscopy to Monitor Methane Emissions from Simulated Grazing Cattle, National Institute of Standards and Technology [data set], https://doi.org/10.18434/mds2-3139, 2024.
Werle, P.: Accuracy and precision of laser spectrometers for trace gas sensing in the presence of optical fringes and atmospheric turbulence, Appl. Phys. B, 102, 313–329, https://doi.org/10.1007/s00340-010-4165-9, 2011.
Certain equipment or instruments are identified in this paper in order to specify the experimental procedure adequately. Such identification is not intended to imply recommendation or endorsement of any product by NIST nor is it intended to imply that the equipment identified are necessarily the best available for the purpose.