the Creative Commons Attribution 4.0 License.
the Creative Commons Attribution 4.0 License.
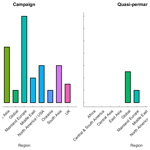
An overview of outdoor low-cost gas-phase air quality sensor deployments: current efforts, trends, and limitations
Laura T. Iraci
We reviewed 60 sensor networks and 17 related efforts (sensor review papers and data accessibility projects) to better understand the landscape of stationary low-cost gas-phase sensor networks deployed in outdoor environments worldwide. This study is not exhaustive of every gas-phase sensor network on the globe but rather exists to categorize types of sensor networks by their key characteristics and explore general trends. This also exposes gaps in monitoring efforts to date, especially regarding the availability of gas-phase measurements compared to particulate matter (PM) and geographic coverage gaps (the Global South, rural areas). We categorize ground-based networks that measure gas-phase air pollutants into two main subsets based on their deployment type – quasi-permanent (long term) and campaign (short to medium term) – and explore commonplace practices, strengths, and weaknesses of stationary monitoring networks. We conclude with a summary of cross-network unification and quality control efforts. This work aims to help scientists looking to build a sensor network explore best practices and common pathways and aid end users in finding low-cost sensor datasets that meet their needs.
- Article
(1760 KB) - Full-text XML
- BibTeX
- EndNote
1.1 A brief history of low-cost gas sensors: context, development, and growth
In the past few decades, the emergence, growth, and solidification of low-cost air quality sensor efforts have coincided with myriad policy decisions and social movements. In this section, we reference those in the United States (US) as examples of possible drivers behind the expansion of low-cost sensor use. The Clean Air Act, which serves as the US's primary set of air quality laws, was first enacted in 1963. It calls for the monitoring of six “criteria” pollutants, which are harmful to both human health and the environment (U.S. EPA, 2014a). The criteria pollutants are particulate matter (PM), ozone (O3), nitrogen oxides (NO, NO2, NOx), sulfur oxides, carbon monoxide, and lead. For each of these pollutants, the current guidelines were established between 2010 and 2015 (California Air Resources Board, 2023). Following the public awareness and federal regulations of these pollutants, general air quality monitoring efforts in the US increased and have remained relevant to date. In 2022, the US Environmental Protection Agency (U.S. EPA) allocated over USD 54 million to fund 143 air monitoring projects in 37 states, many of which include low-cost sensor networks, including USD 20 million in grants for ambient air monitoring in underserved communities (U.S. EPA, 2022). The affordability and ease of use of low-cost sensors continue to answer the need for more air quality monitoring, especially in historically underserved areas.
Environmental justice (EJ) refers to the unequal exposure to pollution based on factors such as race and class (Čapek, 1993), and even the placement of monitoring stations can be inequitable (Miranda et al., 2011). In the US, the National Association for the Advancement of Colored People (NAACP) is largely credited with formalizing the movement with their protests in the 1980s (U.S. Department of Energy, 2024). While a variety of other special interest groups, non-profit, and community organizations have been actively involved in democratizing air pollution monitoring (National Association of Clean Air Agencies, 2023; Coalition for Clean Air, 2023), formal government recognition and action on these issues may have encouraged further monitoring efforts, including low-cost sensors. Notably, in 1992 the Environmental Equity Working Group was established, followed by Executive Order 12898 issued in 1994, titled Federal Actions to Address Environmental Justice in Minority Populations and Low-Income Populations (U.S. Department of Energy, 2024). Environmental justice and air quality monitoring in underserved regions have remained focus areas of air sensor use to date (Oyola et al., 2022).
The rise of sensor-based exposure monitoring follows a similar timeline. In their paper, Sherwood and Greenhalgh (1960) introduced a “personal air sampler” that used a filter to collect PM. While a few other studies throughout the 1960s and 1970s began to broach the idea of personal air quality monitoring (Taylor, 1987; Ott, 1982; Morgan and Morris, 1976; National Research Council (US) Committee on Indoor Pollutants, 1981), modern-day air quality sensor technology began to emerge in the 1980s, with several studies noting the use of electrochemical (Khan and Meranger, 1983; Ramakrishna et al., 1989; Weppner, 1987) and non-dispersive infrared (NDIR) gas sensors (Shibata et al., 1987), which were new at the time. During the 1990s, other commonly used sensor technologies such as thin films (Korotchenkov et al., 1999; Meixner et al., 1995) and metal oxides (Papadopoulos et al., 1996; Sberveglieri et al., 1997; Meixner et al., 1996) began to emerge. The early 2000s brought the first few noteworthy sensor network studies (Arnold et al., 2002; Zampolli et al., 2004; Mahfuz and Ahmed, 2005).
The mid-aughts and early 2010s brought further advancements in sensor technology (Chang et al., 2006), major improvements to sensor calibration methods (Piedrahita et al., 2014; Spinelle et al., 2013), and a huge influx of sensor applications, including the advent of large-scale sensor networks (Postolache et al., 2009; Ma et al., 2008) and science-grade research projects (Mead et al., 2013; Bales et al., 2012; Sivaraman et al., 2013). Government programs began endorsing and funding low-cost sensors as a supplement to traditional air quality monitoring, contributing to the burst of sensor deployments during this period. For instance, the U.S. EPA (2024) published the first version of its Air Sensor Guidebook in 2014, targeted as a resource for individuals interested in low-cost sensors and sensor data.
During the most recent era of low-cost air quality sensing, from the mid 2010s to present, on which this review focuses, sensor projects have continued to expand with no sign of slowing down. Each of the 60 sensor networks reviewed in Sects. 2 and 3 originated or found widespread usage during this period, which includes the growth of low-cost sensors in university and other research groups and the advent of large-scale sensor network companies such as PurpleAir. This era represents an exciting time, as sensor networks continue to grow as a solution to gaps in monitoring and gain legitimacy as monitoring tools. Figure 1 shows the number of Google Scholar search results by the decadal ranges referenced in this section for “low-cost air quality sensors outdoor stationary”. While this search term is imperfect and related but irrelevant papers may still appear, it serves as a rough proxy for the explosion of low-cost sensor studies in the 2000s that is continuing into the present day.
1.2 The need for gas-phase sensor networks
One of the main reasons sensor networks have experienced this major growth in popularity in the twenty-first century is their ability to fill gaps in existing air quality monitoring efforts. Since low-cost sensors are a fraction of the cost of traditional air quality monitoring instruments, a fleet of them can be deployed at the same price point as one regulatory-grade monitor. Thus, low-cost sensors have been a boon in areas that have been overlooked by traditional monitoring efforts and alike in areas with high spatial variability of pollution where a single air quality monitor is not indicative of trends across the region.
While the findings of this study and others (Carotenuto et al., 2023) show that PM-based sensor networks are among the largest and most frequently deployed, there is a great need for more gas-based measurements. PM has traditionally been targeted in monitoring efforts because of the adverse health effects associated with it (Donaldson et al., 2005; Wyzga and Rohr, 2015; Pope and Dockery, 2006; Chen and Lippmann, 2009). PM measurements are particularly relevant to dust storms (Khader and Martin, 2019) and widespread cookstove usage (Alexander et al., 2018) in certain parts of the world and in studying wildfires worldwide. However, even in regions where PM is the primary air quality concern, gas-phase chemistry has been shown to drive PM emissions (Ren et al., 2017; Quevedo et al., 2024), necessitating data from both to understand the bigger picture.
Several volatile organic compounds (VOCs) have been shown to have severe health effects of their own, including leukemia (N. Liu et al., 2022), lung cancer (Kampa and Castanas, 2008), heart disease (Kampa and Castanas, 2008), asthma (Alford and Kumar, 2021; N. Liu et al., 2022; Ware et al., 1993), and a variety of other acute and chronic health conditions (Fiedler et al., 2005; Cheng et al., 2019; Johnston et al., 2021). For these pollutants, both the concentration and the length of exposure are crucial for understanding potential risks (Hu et al., 2023), making it doubly important to have highly localized datasets to both assess personal exposure and set regulations relevant to health risks. Trace gases have been shown to vary widely in concentrations on opposite sides of the same building (Collier-Oxandale et al., 2018b), demonstrating that personal exposure can vary widely even within a small radius. Again, the gas-phase data that sensors can supply on highly localized spatial and temporal scales are crucial to understanding and preventing health risks from air quality. VOCs can also contribute to ground-level ozone production, which is associated with a variety of health risks, including a variety of respiratory issues (Soares and Silva, 2022). Additionally, PM-centric sensor networks miss out on measuring greenhouse gas emissions, including methane (CH4) and carbon dioxide (CO2), which the present review found to be among the most studied with gas-phase sensor networks. Since sensors are valuable tools for filling monitoring gaps, these data can be influential in designing mitigation strategies or setting emission targets in areas lacking more widespread air pollution monitoring.
1.3 Nomenclature
In this work, a “sensor” refers to an individual component that typically measures one specific pollutant of interest. A “sensor package” refers to the complete measurement instrument, including one or more sensors, a circuit board, some form of data collection, and a means of pulling the ambient sample across the sensors (e.g., a fan or pump). A “sensor network” refers to a cluster of several sensor packages deployed in some predetermined location. A “node” refers to an individual sensor package that is part of a sensor network.
1.4 Criteria for inclusion in the review
For a low-cost sensor network or associated study to be included in our review, it had to meet the following criteria:
-
The cost of an individual sensor package could not exceed USD 1000 or an equivalent amount.
-
The sensor package must measure at least one gas-phase compound.
-
The sensor network must be ground based.
-
The sensor network must be deployed outdoors.
-
The sensors must be deployed in a stationary location; mobile and wearable devices were excluded.
We focused on application-based sensor deployments rather than reports on sensor development and calibration alone. However, in regions with few gas-phase monitoring efforts, we included deployments focusing on calibration or data quality, so long as the sensors were still deployed in a stationary outdoor location.
1.5 Literature review methods
We began our literature review with broad Google Scholar searches and relevant conference proceedings, including NASA Health & Air Quality Applied Sciences Team (HAQAST) 2017–2023 Proceedings (NASA HAQAST, 2023), the European Geosciences Union (EGU) General Assembly 2023 Monitoring Networks Session (EGU, 2023), Air Sensors International Conference (ASIC) 2022 Proceedings (Hemingway, 2022), the American Geophysical Union (AGU) Thriving Earth Exchange projects portal (Thriving Earth Exchange, 2017), and the AGU Ground-Based Atmospheric Monitoring Networks Session 2022 (AGU, 2022).
Most resulting networks originated or were deployed in English-speaking or western European countries, regions that we acknowledge our search was heavily biased towards, as these tend to be the areas with the most low-cost monitor coverage. For regions that were underrepresented in our initial search, we used targeted Google Scholar searches to find field deployments in these areas, aiming to include multiple networks from each region. While these methods are not exhaustive, we are confident that the trends in low-cost sensor practices have been illuminated with 60 sensor networks reviewed. This review was concluded in September 2023, with a few additional references added during the review process in 2024.
Several air quality sensor review papers were consulted in the writing of this paper, which targeted key aspects of the use of low-cost sensors and directed us towards relevant papers. These included the use of low-cost sensors to measure VOCs (Spinelle et al., 2017) and particulate matter (Engel-Cox et al., 2013), deployments in sub-Saharan Africa (Amegah, 2018), sensor types and their calibration (Narayana et al., 2022), two summaries of performance targets for low-cost sensors (Williams et al., 2019; Karagulian et al., 2019), a review of campaign-style temporary sensor networks (Carotenuto et al., 2023), and broad reviews of the use of low-cost sensors in the 2010s (Morawska et al., 2018). Additionally, government-sponsored initiatives to compare low-cost sensors were utilized, including the World Meteorological Organization's 2021 report (World Health Organization, 2021), and sensor comparison initiatives published by the European Network on New Sensing Technologies for Air-Pollution Control and Environmental Sustainability (EuNetAir) (Borrego et al., 2016), the South Coast Air Quality Management District (AQMD) Air Quality Sensor Performance Evaluation Center (AQ-SPEC) program (Collier-Oxandale et al., 2020a), and the Community Air Sensor Network (CAIRSENSE) project (Jiao et al., 2016).
1.6 Format
The format of each subsequent section and subsection is as follows. First, we define the section or subsection, explaining the characteristics of low-cost monitoring networks included within it. Following this, we dive into multiple examples of each, highlighting the characteristics mentioned in the introductory paragraph and providing more specific details and context. We conclude by summarizing the common threads in each category, exploring emerging sensor directions, and making recommendations for sensor usage and data management based on our findings.
Quasi-permanent networks are deployed in stationary locations with the intent of measuring for at least 2 years or the remainder of the sensor's useful life. These often feature countrywide or global calibration schemes and are the most likely to include a live dashboard for end users to visualize the data. Most sensor packages that we reviewed which fell into this category were operated by local or regional governments or consisted of widely deployed sensor packages developed by private companies. Many of these do not list specific calibration procedures, some from a lack of standardized calibration but others for proprietary reasons as data are part of their product. A list of the 12 quasi-permanent networks considered in this review and their key characteristics can be found in Table 1.
2.1 Quasi-permanent, operated by private companies
Most sensor networks in this category are operated by for-profit companies. The typical framework is as follows: the company will manufacture and sell the sensor packages widely for profit. Each of the individually owned sensor packages becomes part of a publicly available data map, which anyone can visit to visualize the air quality in their area. These maps sometimes include data from regulatory monitors or other sensor networks and typically focus on air quality index (AQI) as a key metric. While live or semi-live data are often accessible, historical data may not be available for download or may require the use of an application programming interface (API), which may present a barrier to non-scientific users and prevent them from downloading it for further inspection. Seven such companies are reviewed in this section, with the largest, PurpleAir, discussed in the “Case study: PurpleAir” section.
A case study of a company born out of a university research-grade project is TELLUS and its AirU sensors. The project was originally started at the University of Utah under a National Science Foundation (NSF) grant to bring higher spatial coverage air quality monitoring to the Wasatch Front region of Utah (AIRU, 2024). Since then, it has evolved into a product line of indoor and outdoor sensor packages measuring CO, NO2, and PM. As is typical of university research projects, the sensors are robustly calibrated via chamber tests (Sayahi et al., 2019; Kelly et al., 2017), colocation with reference-grade instruments (Becnel et al., 2019a; Sayahi et al., 2020; Kelly et al., 2017), and the development of machine-learning-based calibration algorithms (Becnel et al., 2019b; Becnel and Gaillardon, 2021). TELLUS' website includes its own live data map dubbed AirView, which not only includes data from the TELLUS sensor packages, but also incorporates data from two larger quasi-permanent monitoring networks: PurpleAir, discussed in the “Case study: PurpleAir” section, and the U.S. EPA's AirNow, discussed in Sect. 2.2 (TELLUS, 2023). This dashboard represents a recurring approach of incorporating data from other networks to fill in gaps in spatial distribution but does not feature a historical data archive for download. The sensors have been utilized in at least one end user's peer-reviewed study (Mullen et al., 2023).
IQAir, a company based in Switzerland, produces a line of low-cost sensor packages measuring CO2 and PM (IQAir, 2023). Their live data map has high spatial coverage in North America, Europe, East and South Asia, and Oceania. Their map also includes data from third-party sensors such as PurpleAir and Clarity (IQAir, 2024b). Historical data and other tools can be accessed via API. Their AirVisual app uses real-time weather and global satellite data in addition to their low-cost sensor data on the map to better predict hourly air quality. Their website describes using machine learning to validate and calibrate data based on the environmental conditions and estimating the AQI where monitoring gaps exist (IQAir, 2024a).
Clarity is a privately owned company that likewise sells sensor packages meant for long-term deployments, targeting NO2, O3, and PM (Clarity Movement Co., 2024a). It also features a live data dashboard called OpenMap, which includes data from both the Clarity sensor nodes and the government reference stations alike. Clarity also produces a data tool, which allows the user to view and plot historical data from individual nodes (Clarity Movement Co., 2024b). The map has worldwide coverage, with the highest sensor density in North America and Europe. Asia and the Global South receive considerably less spatial coverage. One end-user-led deployment saw the sensors colocated with reference monitoring stations to develop calibration algorithms (Zaidan et al., 2020).
AirGradient is another privately held company with sensors measuring CO2, NOx, PM, and VOCs (AirGradient, 2024). Different versions of the platform are available for indoor and outdoor monitoring. It also features a live data map, where near-real-time data and data up to 2 weeks old can be visualized. The map has worldwide coverage, with the highest sensor density in North America and Europe. An interesting feature is that the AirGradient platform uses open-source hardware, offering both completed sensor packages and ready-to-build kits for project-oriented customers. The sensors have been colocated in more than 20 different locations globally as of 2024, and calibration fits have been derived to assess goodness of fit, although the model specifications are not explicitly stated on its website. While the first few scientific studies using AirGradient sensors pertain to indoor air quality (Chatzidiakou et al., 2023) and outdoor PM (Hodoli and the Network for Atmospheric and Air Quality Research, 2024), the network has potential for future outdoor gas-phase studies.
Case study: PurpleAir
PurpleAir is the largest low-cost air quality sensor network across the globe, with over 22 000 PM sensors deployed across approximately 100 countries as of 2023 (PurpleAir, 2023). It offers a live global map where users can toggle between raw sensor data and several different air quality index (AQI) calculations based on the data at hand (PurpleAir, 2024). While there is no easy click-and-download option, an API can be used to access historical data. As of 2024, fees may be incurred for downloading historical data depending on the amount of data requested.
For quality assurance, PurpleAir relies on the sensors' dual-channel laser configuration. If the two laser channels read similarly, the data are deemed reliable. If not, the sensor will appear semi-translucent on the map with a highlighted warning if a user selects the sensor online. The confidence score between the two channels is also displayed accordingly with either a check mark or an X. Even with this feature, various agencies have reported that the raw PurpleAir data may exceed actual values by a factor of 2 in experiments compared with reference-grade PM instruments (Puget Sound Clean Air Agency, 2023). Likewise, the Plantower sensors used in the PurpleAir nodes have a functional life of approximately 2 years (PurpleAir Community, 2024), but as of 2023 there is no mechanism to remove sensor data from the map based on sensor age alone.
In recent years, PurpleAir has launched an add-on VOC sensor (Bosch BME688) to its existing PM sensor packages, but they have yet to reach the same widespread use as the original PurpleAir monitors. As seen in Fig. 2, there are far fewer VOC-enabled PurpleAir sensors worldwide than their standalone PM sensors. The AQI and calibration procedures for the VOC sensor have yet to be formalized, with only the raw sensor signal available on PurpleAir's interactive map rather than a calibrated mixing ratio estimate. Even within wealthier countries, socioeconomic disparities affect the spatial distribution of PurpleAir monitors (Sun et al., 2022).
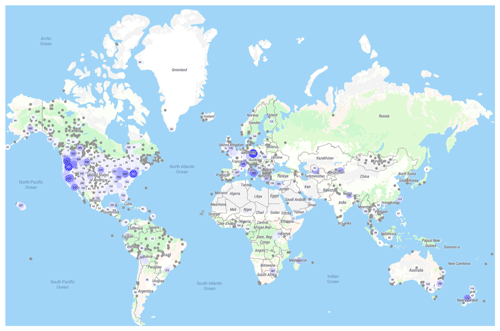
Figure 2Usage of outdoor PurpleAir VOC sensors (colored in shades of purple based on concentration) with additional PurpleAir PM-only sensors marked in gray. The map shows that global coverage for VOCs is sparser than for PM, with the Global South and rural areas lacking gas-phase coverage. PM coverage is also significantly less dense in the Global South. (© PurpleAir, source: https://map.purpleair.com/, last access: 18 September 2023).
Since PurpleAir is an affordable, user-friendly sensor with built-in mapping capabilities, many research groups utilize their products to collect air quality data. However, since the data quality is not necessarily science grade, many research groups have conducted pilot deployments to generate more reliable calibration algorithms, rendering the PurpleAir data more useful. We encountered many cases of research groups using the PurpleAir monitors as their low-cost sensing platform, often opting to develop their own calibration algorithms to ensure accuracy. Studies concerning the PurpleAir VOC sensor and its calibration are few as of 2023; although the VOC sensor is a newer addition to the PurpleAir product line, it appears to be underutilized in addition to being under-deployed. We reviewed one study which compared the VOC sensor data to an air quality dispersion model of VOC mixtures emanating from an industrial facility in the northeastern US (Rosmarin et al., 2023). Another study in the Ohio River valley in the US utilized both PurpleAir and Airviz monitors to measure PM and VOCs. While regression-based calibration algorithms were derived for PM, raw VOC outputs were used for qualitative analysis only (Raheja et al., 2022a).
Most other studies concerning PurpleAir have focused on PM sensing. Many have focused on improving calibration for monitoring during fire and smoke events (Barkjohn et al., 2021, 2022; Sablan et al., 2022), while others have used them in community-level monitoring and citizen science applications (Lu et al., 2022; AirWatchSTL, 2024; Jing et al., 2022; Byrne et al., 2023). Additional US-based studies have utilized the PurpleAir monitors and developed their own calibration algorithms for general usage (Malings et al., 2020; Tryner et al., 2020; Wallace et al., 2022). Internationally, PurpleAir PM data have also been utilized extensively, especially in the Global South where gas-phase measurements and air quality measurements generally are sparse compared to other regions (Washington University in St. Louis, 2024; Awokola et al., 2020, 2022; Velásquez et al., 2022; Coker et al., 2022; Campmier et al., 2023).
A host of other air quality monitoring tools incorporate data from PurpleAir into their interface, including the U.S. EPA's AirNow Fire and Smoke Map (AirNow, 2024b), AirView by TELLUS (2023), AirVisual by IQAir (IQAir, 2024b), Air Matters (2024; mobile app), Windy (2024; mobile app), Paku for PurpleAir (2024; mobile app), Local Haze (2023; mobile app), and AirCare (2024; mobile app).
2.2 Quasi-permanent, operated by government
A small number of quasi-permanent low-cost sensor networks operated by various government entities were reviewed to understand how sensors are being used to complement traditional regulatory tools. These government-operated networks typically make use of commercially available sensors (although not always), include a live data dashboard for easy data visualization, and incorporate regulatory monitoring station data for comparison on the data dashboard or elsewhere on their site.
The most widely recognized usage of low-cost air quality sensors by the US government is the Environmental Protection Agency (EPA) AirNow program. While the main AirNow interactive map only displays regulatory-grade ozone and PM data from government agencies (AirNow, 2024a), the AirNow Fire and Smoke Map also includes data from PurpleAir's low-cost PM sensors. The inclusion of these sensors greatly improves spatial coverage but reduces confidence in the data as the PurpleAir sensors are not held to the same rigorous standards as the government-run analyzers. However, the Fire and Smoke Map is targeted towards the public for real-time decision-making during wildfire and other emission events, with the locations of fires also shown on the map and resources about the fires and safety available (AirNow, 2024c). Data from the main AirNow site have been used in scientific applications (Chai et al., 2017) in addition to individual- and community-level decision-making.
Several municipalities and programs in the US use Lunar Outpost's Canary sensor packages for local and regional monitoring, including the US Forest Service, U.S. EPA, and the Denver Department of Public Health and Environment's Love My Air program (Outpost Environmental, 2024). Love My Air has measured PM at schools in the Denver metropolitan area using the Canary modules since 2018 and now includes a few sites measuring a broader range of pollutants, including O3, CO, NO, NO2, H2S, SO2, and VOCs. It includes its own data visualization map with data from both the sensors (marked with circles) and nearby regulatory monitors (notated with squares) (Love My Air, 2024). The program aims to raise community awareness about local air quality issues and includes a downloadable education outreach curriculum for teachers to share with their students. In a similar vein, the Love My Air Wisconsin program began in the fall of 2023 (Children's Health Alliance of Wisconsin, 2024).
Government-run air quality monitoring utilizing low-cost sensors is most prevalent in the US and western Europe. The Dutch National Institute for Public Health and the Environment (RIVM) has incorporated low-cost sensors measuring NO2 and PM into its monitoring and citizen science projects, with approximately 30 sensors being operated directly by RIVM as of 2019. Outdoor colocation with a reference measurement and multivariate linear regression are used to calibrate the NO2 sensors, and the humidity dependence of the PM sensors has also been studied (Wesseling et al., 2019). RIVM has also created an interactive map (Measuring Together) to merge data from a variety of sensors – both their own and those deployed by various citizen scientists – with other public information, including regulatory-grade air quality measurements, real-time traffic, and satellite data (RIVM Data Portal, 2024). For individuals to add their data to the site, they must include information on the type of sensor and how it was calibrated to ensure data quality. As of 2019, RIVM has been working on an API to allow end users to download data from the site in bulk (Wesseling et al., 2019).
2.3 Quasi-permanent, operated by research institution or university
Few long-term monitoring networks are operated by research institutions or universities; most networks in this category are utilized for short-term deployments (see Sect. 3.1) or as commercial products (e.g., TELLUS, described in Sect. 2.1). However, one notable sensor network in this category is the Berkeley Environmental Air-quality and CO2 Network (BEACO2N) (BEACO2N, 2024). The BEACO2N nodes are widely deployed in the San Francisco Bay Area, with a few nodes in other select cities worldwide. BEACO2N focuses on CO2 measurements but also includes sensors for a variety of other pollutants (O3, NO2, CO, PM), making them broadly applicable in various monitoring efforts. Their website features both a live map of sensor readings and an easy-to-use data selection tool, which allows users to download historical data for selected date and location ranges. Their data also feature robust calibrations based on comparison with reference-grade instruments (Shusterman et al., 2016) and field calibration and evaluation (Kim et al., 2018). While the global coverage of this network is not as dense as many of the commercialized networks discussed in Sect. 2.1, BEACO2N's data quality is high due to their data management practices, as compared to others which leave quality assurance up to the end users.
Another project with long-term goals is Smart Citizen, developed by Fab Lab Barcelona in Spain. Offerings include two different varieties of sensor packages: a build-it-yourself kit, targeted at citizen scientists and educators, and a more robust sensor platform designed for long-term outdoor use, with more flexibility of sensors to be included and higher accuracy (Smart Citizen Kit, 2024). The latter can accommodate sensors for CO, CO2, NO2, O3, VOCs, and PM (Camprodon et al., 2019). It also features a global map with sensors deployed on each continent, which is still live as of 2024, although few nodes still appear to be logging live data (Smart Citizen, 2024). While the environmental dependencies of the sensors and field validation efforts are offered, there is no explicit scheme to correct the sensor readings, instead utilizing the raw signals from each (Camprodon et al., 2019).
2.4 Quasi-permanent, operated by non-profit
Here we discuss two different models of non-profit sensor networks in the quasi-permanent category: sub-networks that employ monitors purchased from the large, privatized sensor manufacturers and non-profit sensor networks that build their own sensor packages. Most serve a single community or region facing air quality issues. These networks tend to exist on smaller scales than their for-profit counterparts, with a smaller number of nodes per network and smaller spatial reach. While each of the networks we encountered in this category featured a live data map, we did not encounter any with a historical data archive or download option, nor did we find any obvious explanation of data quality or calibration procedures on their websites. However, even if the data are not science grade, these networks can be crucial to building community interest and understanding of local air quality issues.
One example of a non-profit utilizing commercially purchased sensors is MKE FreshAir Collective (2024), a community-led non-profit organization based in Milwaukee, Wisconsin, US. It aims to provide neighborhood-scale monitoring and address environmental racism through community action. It utilizes sensors purchased via IQAir, and the data can be viewed by zooming in on Milwaukee on the main IQAir map. This is an attractive model for community groups and other organizations or individuals with low technical skills and/or limited time, as it allows them to utilize pre-existing resources rather than having to develop their own. However, this also often means that the non-profit is limited to the calibration and visualization tools of the host network, which may be inadequate for some user needs.
A small number of non-profit organizations develop their own sensor packages and make them available for sale, typically on a much smaller scale than the for-profit networks. One example is Ribbit Network (2024), a non-profit organization that targets teachers and citizen scientists to deploy their CO2 sensors. Their offerings include a prefabricated sensor package or the materials for the end user to build it themselves, which are targeted towards K–12 education outreach efforts. Ribbit likewise has developed its own global map to view CO2 levels from its sensors worldwide and offers publicly available short-term data downloads. While calibrations are underway, there are no peer-reviewed studies using this network as of early 2024.
The remainder of the sensor networks reviewed are campaign based, deploying sensors for a limited time frame to study a point source or phenomenon local to the geographic area. The data quality in this category varies widely, with government and university groups typically producing the highest-quality data, while non-profit or smaller commercial networks may include less quality assurance. A few examples from each subcategory are described in detail here, with at least one example from a country outside the US or western Europe for each. Nearly 50 campaign-based networks are summarized here, with myriad others that fall slightly outside the scope of our review cited for further reading but not discussed in detail. All of the former can be found in Table 2.
3.1 Campaign, operated by research institution or university
We encountered more research-grade campaign-based studies using low-cost air quality sensors than all other categories combined. In this section, we select a few key projects from each global region to review in detail. The US, western Europe, and parts of southeast Asia are more thoroughly covered in these studies than any other region, and a portion of studies concerning the Global South were conducted by American or western European institutions, albeit usually with local collaborators. Research-grade projects tend to have the highest data quality and most robust calibration procedures, including an ambient colocation with a reference-grade instrument and an algorithm to relate the signals of each. These also tend to include the highest number of pollutants being measured by each sensor package. However, these often lack a central data portal for stakeholders to visualize or access data easily. Note that at least one example deployment is included for each campaign but is not intended to be an exhaustive list of every use case of each sensor network. Regional boundaries have been defined for illustrative purposes only; regions do not necessarily represent similarly sized populations or an equal number of countries. While most of the sensor packages utilized in this category are built in-house by the research institution, we encountered a small number of research institutions that made use of sensor packages built by commercial manufacturers. In these cases, we list the name of the manufacturer and also cite the research group responsible for deploying them.
3.1.1 Campaign, operated by research institution or university in North America
Campaign-based low-cost gas-phase sensor networks are abundant in North America, especially the US. In Canada, we found that PM studies were more common, including the measurement of wildfire smoke (Si et al., 2020; Lee et al., 2024; Jaafar et al., 2024). A research team at Carnegie Mellon University developed the Real-time Affordable Multi-Pollutant (RAMP) air quality sensor packages, measuring CO, NO2, O3, SO2, and PM. A dense sensor network was established near Pittsburgh, Pennsylvania, US, to better understand urban emissions (Tanzer et al., 2019; Li et al., 2019; Tanzer-Gruener et al., 2020), and a shorter-term campaign was conducted in San Juan, Puerto Rico, following Hurricane Maria in 2017 (Subramanian et al., 2018). Another set of sensors was deployed in British Columbia, Canada, to characterize emissions inside of a parking garage (Liu and Zimmerman, 2021). These sensors have also been deployed in various African countries (Bahino et al., 2021). Calibration efforts include colocation with a reference instrument and subsequent modeling, including linear and quadratic regression, clustering, neural networks, Gaussian processes, and hybrid random forest models (Malings et al., 2019a; Zimmerman et al., 2018; Jain et al., 2021). Their data are hosted on Google Drive for greater public access.
Another noteworthy US-based project is the University of Colorado Boulder's “pods”. The in-house-built nodes contain sensors for CO, CO2, NO2, O3, VOCs, and PM (Piedrahita et al., 2014). Several studies detail the calibration and validation of their sensors, which typically utilize an outdoor colocation with a reference-grade instrument and the use of machine learning techniques such as artificial neural networks (Casey et al., 2019) and multivariate linear regression (Collier-Oxandale et al., 2018a). Additional techniques have rendered its VOC sensors applicable for speciated hydrocarbons such as methane and formaldehyde (Okorn and Hannigan, 2021) in oil and gas monitoring applications (Cheadle et al., 2017; Collier-Oxandale et al., 2020b; Okorn et al., 2021). An offshoot of the network, Inexpensive Network Sensor Technology for Exploring Pollution (INSTEP), uses the same sensors in applications primarily concerning comparison with remote sensing instruments (NASA, 2024). Neither iteration of the project features a public-facing data dashboard or download option.
While the ARISense low-cost monitors for CO, NO, NO2, O3, and PM are primarily US based, they have also been deployed in Malawi in East Africa. The sensor packages were built in-house by Cross et al. (2017) and have since been used by researchers with a variety of affiliations, including North Carolina State University. After colocation with a reference-grade instrument, a series of different machine learning models were tested to best fit the data: hybrid k-nearest neighbors, random forest hybrid, high-dimensional model representation, quadratic regression, and multivariate linear regression (Bittner et al., 2022). Deployments in both Massachusetts, US, and Malawi were effective (Cross et al., 2017), although the authors note the lack of reference monitoring stations in Malawi hindered their ability to regularly recalibrate the sensor nodes.
3.1.2 Campaign, operated by research institution or university in Europe
Spatial coverage of low-cost sensors in Europe is among the highest of all the regions, but this is dominated by sensor usage in western European cities; rural areas and eastern Europe lack coverage. A recent ozone sensor calibration study in Poland (Badura et al., 2022) suggests that outdoor sensor usage is beginning to gain traction, but we only encountered a few application-based studies in eastern Europe.
One eastern European example is the Czech Technical University in Prague's Trafficsensnet project. It involved deploying approximately 20 in-house-built sensor packages measuring CO, NO2, SO2, VOCs, and PM in Prague to better understand the air quality effects of traffic in the urban area. Colocation with reference-grade instruments was used to validate the sensor readings, although no additional calibration algorithms were fit (Brynda et al., 2015). The project was later expanded to include an online data portal and map-style viewer (Brynda et al., 2016), which is unusual for university research-grade projects. In later iterations of the project, however, the gas-phase sensors were removed to focus on PM (Brynda et al., 2020).
We also include studies conducted in Siberia or Russia with the eastern European countries as they exhibit a similar lack of coverage, albeit for a much larger geographic area. We encountered one PM study in the region (Lin et al., 2020) and one study using spatially distributed passive sampling (Khuriganova et al., 2019) but no true gas-phase low-cost sensor studies, highlighting the need for more monitoring and availability of data in this region. A related 2023 paper (Labzovskii et al., 2023) explains the challenges of maintaining air quality networks in this region despite the high demand for localized air quality data, citing issues such as data access and decentralization. As of August 2024, there were two PM nodes logging data in Russia on PurpleAir's live map (PurpleAir, 2024).
The remaining sensor networks reviewed in this section hail from western European countries, which are generally wealthier (Brzeziński et al., 2020) and see much higher low-cost sensor usage. A recent project that served several European countries was Barcelona Technical University's in-house-built CAPTOR box measuring ozone in Spain, Italy, and Austria (Barcelo-Ordinas et al., 2021). Outdoor colocations with a reference instrument were conducted in each country, and different linear regression models were used to calibrate each set of sensor packages. This CAPTOR deployment was one of the few that serviced urban, suburban, and rural sites in one deployment to examine differences among them (Barcelo-Ordinas et al., 2019).
The Carbosense project out of ETH Zurich, Switzerland, deployed over 300 sensor nodes measuring CO2 throughout Switzerland, with many of them concentrated in the city of Zurich. The sensors were manufactured by Decentlab GmbH and calibrated in-lab and via ambient colocation with a regulatory-grade instrument. The subsequent calibration algorithms were based on the Beer–Lambert law and included corrections for abnormal humidity readings and drift correction over time. The sensors showed high accuracy during the approximately 2-year-long deployment but were insufficient in capturing small regional gradients (Müller et al., 2020).
The AirQino platform was developed by the Italian National Research Council as a sensor package to measure CO, CO2, NO2, O3, and PM. While it has primarily been used in mobile (Zaldei et al., 2015) and PM-focused studies (Brilli et al., 2020, 2021), one recent application tested its effectiveness for measuring CO2 in the Arctic in several locations in Svalbard, Norway, an island in the Arctic Circle. The sensors were colocated outdoors with a reference instrument, and although no further calibration algorithms were derived, the AirQino sensors showed strong agreement with the reference signals, even in the extreme cold (Carotenuto et al., 2020).
Several low-cost monitoring projects have sprung up in France in recent years, including the CairBox monitoring platform, which has been used to monitor reduced sulfur compounds H2S and CH3SH at and around a wastewater treatment plant. This study was a collaboration between researchers from IMT Mines Alès, a university in France, and the sensor manufacturer, Cairpol. The sensors showed strong agreement with theoretical concentrations, but no calibration factors were applied (Pereira-Rodrigues et al., 2010). Despite the lack of further studies with this sensor platform, we have opted to include it here as it is one of the few sulfur compound monitoring efforts we encountered.
Dutch research organization TNO (formerly known as ECN) developed a sensor platform designed to be deployed on lampposts in the early 2010s. Their “airboxes” (not to be confused with the RES-NOVAE “AIRBOXES” discussed in Sect. 3.3) are designed to measure PM, O3, and NO2 in urban areas (van Bree, 2024). A web portal dubbed AiREAS allows users to visualize and download the sensor data, although the TNO sensor data available as of 2024 seem to focus on PM rather than any gas-phase pollutants (AiREAS, 2024). Preliminary comparisons between the NO2 sensors and a reference-grade NO2 instrument show strong agreement, but any subsequent calibration algorithms are not disclosed (van Bree, 2024). Recently, TCN's airboxes were used in a dissertation study at the University of Twente Department of Earth Observation Science in the Netherlands. The study focused on factors affecting NO2 calibration of the sensors, including temperature, humidity, and transferability of models among nodes. Applications included health risk mapping, where the pollutant concentrations were combined with epidemiological data to estimate disease risk at the city scale (van Zoest, 2020).
The UK is a hotbed for low-cost sensor studies, including some high-profile deployments. A network of sensor packages known as SNAQ boxes (Sensor boxes for Air Quality measurements) designed and built by the University of Cambridge measure CO, CO2, NO, NO2, O3, SO2, VOCs, and PM. In-laboratory calibrations were conducted and further validated via colocation with an outdoor regulatory-grade instrument (Popoola et al., 2018). The SNAQ boxes have been utilized in applications ranging from a high-density network in and around London Heathrow Airport (Popoola et al., 2018) to international deployments in Nigeria (Omokungbe et al., 2023), China (Ouyang et al., 2016; Shi et al., 2019), and Portugal (Borrego et al., 2016).
Elsewhere in the UK, researchers at the University of Sheffield have made use of Envirowatch Ltd.'s E-MOTE sensor packages to measure CO, NO, and NO2 throughout the city. Note that although the complete sensor packages were purchased from a manufacturer, this study is included under university efforts because the manufacturer does not appear to provide recommendations or resources for calibration, data storage, or analysis as of 2024 (Envirowatch Ltd., 2024). For this study, the sensor packages were calibrated by the university team via colocation with a regulatory-grade instrument and subsequent supervised machine learning approaches, including linear regression and generalized additive models. Overall, the sensor network was able to demonstrate the diurnal, weekly, and annual pollutant variation in Sheffield (Munir et al., 2019).
3.1.3 Campaign, operated by research institution or university in the Middle East
While very few sensor network studies within our criteria for inclusion have been conducted in the Middle East to date, a few related studies suggest that sensor use in the region may be gaining momentum. We came across several PM-focused studies in the region (Khader and Martin, 2019; Saleh and Khader, 2022; Karaoghlanian et al., 2022; Yavus et al., 2021; Ozler, 2018) and one using air quality sensors on board an uncrewed aerial system (UAS; Al-Hajjaji et al., 2017). A recent study from the Cyprus Institute performed a yearlong outdoor colocation of low-cost CO, NO2, O3, and SO2 sensors with reference-grade instruments (Papaconstantinou et al., 2023); while this study lacked a wider field component, it marks an important step for sensor measurements in the region.
A notable study comes from the Israel Institute of Technology, where CanarIT sensor packages were deployed to measure NO2, O3, VOCs, and PM at sub-neighborhood scale. Linear regressions were used to improve data quality after the sensor packages were colocated with reference-grade instruments. A “rolling-forward field calibration procedure” was also introduced, in which calibration coefficients for each subsequent day were based on reference data from the previous 3 d, but would require further testing to be considered a viable method. Overall, the deployment identified hotspots within the neighborhood (Moltchanov et al., 2015).
In Doha, Qatar, researchers from the Qatar Mobility Innovations Center developed a low-cost sensor platform dubbed MGMSs, multi-gas monitoring stations. Each node included in the initial study measured O3, NO2, H2S, and CO, while additional nodes added later measured each of these in addition to SO2, NO, and PM. The team used the sensors to estimate the AQI around various locales of the city rather than precise concentrations, so robust calibrations were not used in this aim (Yaacoub et al., 2013).
3.1.4 Campaign, operated by research institution or university in South Asia
While there are several notable monitoring efforts in India, overall monitor density is low (Brauer et al., 2019), and monitoring efforts largely focus on urban areas (Agrawal et al., 2021). Other monitoring efforts in India focus on PM (Sahu et al., 2020) but are not described in detail here as they lie outside the scope of gas-phase monitoring. Recently, a US-based research group deployed their multi-pollutant air quality sensor (MPAQS) packages in Delhi, India, measuring CO, NO2, O3, SO2, and PM. Although colocations with research-grade instruments took place, calibration algorithms were not developed in this application, and factor analysis was later used to relate these measurements to local sources (Hagan et al., 2019).
At Lahore University of Management Sciences in Pakistan, the Volunteer Internet-based Environment Watch (VIEW) project aimed to monitor urban emissions. The in-house-built sensor packages measured CO, O3, and SO2 and were deployed around Lahore. Detailed information on their calibration and deployment results has yet to be formally released, but this project still marks a rare low-cost monitoring network in Pakistan. Another sensor network measuring CO and PM performed its pilot study in Vadodara, India. Preliminary results from this study further demonstrate the need for more gas-based monitoring in the country (Barot et al., 2020).
Elsewhere in India, the Streaming Analytics over Temporal Variables from Air quality Monitoring (SATVAM) initiative tested various calibration schemes and proposed a non-parametric algorithm as the most robust to sensors located in vastly different environments: Delhi and Mumbai. These in-house-built sensor packages built by the Indian Institute of Technology measured NO2 and O3. The proposed calibration algorithm consisted of a distance-weighted k-nearest-neighbor approach with a learned metric, which proved effective when deploying the monitors across cities and seasons (Sahu et al., 2021).
3.1.5 Campaign, operated by research institution or university in East Asia
Air quality issues in East Asia are complex (Jeong and Park, 2013), and in recent years low-cost gas-phase sensors have been able to help fill gaps in monitoring throughout the region, though most studies have concerned urban areas. Most East Asian studies we encountered were based in China.
A research team from the Chinese Academy of Sciences used in-house-built sensor packages to measure CO, NO2, O3, and SO2 in Beijing, China. Throughout the yearlong study, the sensors were robustly calibrated using single and multiple linear regression, random forest regression, and neural networks after outdoor colocation with nearby reference-grade instruments. The sensors were tested under different pollution levels and environmental conditions, although the resulting data appeared to be biased based on the calibration model (Han et al., 2021).
Another study in Beijing, China, was carried out by Tsinghua University and collaborators at two universities based in the US. In-house-built sensor packages were deployed on rooftops throughout Beijing to measure CO2, NO, NO2, O3, and PM. The sensors were calibrated for ozone only via colocation with a reference instrument, and best-fit linear regressions were generated. Sizable variation was seen in outdoor ozone concentrations across the city (M. Liu et al., 2020).
A research group at the City University of Hong Kong developed their own mini air system (MAS) to monitor ambient CO, NO2, and PM in Beijing, China. The sensors were calibrated in both laboratory and field settings. After an outdoor colocation with reference-grade instruments, linear corrections were applied to the gas-phase sensor outputs. One notable application included the use of the sensor network to measure pollution at stationary locations along the Hong Kong Marathon route (Sun et al., 2016).
Another study sought to characterize ozone in Hong Kong Special Administrative Region's greater bay area using portable air stations (PASs) sourced from Sapiens, a private company. A team from the Hong Kong University of Science and Technology deployed the PAS monitors to measure NO, NO2, and O3. An outdoor calibration was conducted with a government-run air quality monitoring station prior to deployment, and the sensor measurements were later used as inputs in a chemical transport model (the Nested Air Quality Prediction Modeling System, NAQPMS) to better characterize a high-ozone event that occurred in 2016 (Chen et al., 2024).
Outside of China, a Tokyo Institute of Technology study measured NO2 concentrations in the university's surrounding area using in-house-built sensor platforms. An in-lab calibration was utilized to correct the sensor response for different temperature and humidity conditions. Through the sensor's wireless network, measured NO2 concentrations were frequently compared with other sensors and nearby regulatory-grade monitors for auto-calibration purposes. Overall, the network proved effective in discerning air quality differences throughout the city, including anomalies in NO2 (Tsujita et al., 2005).
At the National Taiwan University, in-house-built CO sensor packages were deployed throughout Taipei near major roadways to determine the air quality effects of traffic in the city. The sensors were colocated at an EPA reference station, and a segmented linear regression approach was used to calibrate the sensors, since there only appeared to be a linear relationship between sensor voltage and reference concentration above a certain threshold. The study provided street-level insights into pollution from traffic, with tangible differences between weekdays and weekends as well as between rush hour and regular traffic (Wen et al., 2013).
Researchers at Seoul National University employed a network of self-built CO2 monitors dubbed Seoul National University CO2 Measurement (SNUCO2M) to better understand CO2 fluctuations in Seoul, South Korea. The sensors were colocated with reference-grade instruments to ensure reliable functioning before full deployment in Seoul. The study demonstrated the characteristics of urban CO2 throughout the city (Park et al., 2021).
3.1.6 Campaign, operated by research institution or university in Oceania
One uncommon instance of a campaign-based sensor network with a live data dashboard is the Knowing Our Ambient Local Air-quality (KOALA) project at Queensland University of Technology in Australia (Queensland University of Technology, 2024). KOALA offers measurements of CO and PM in a variety of locations, including deployments in Australia, China, Vietnam, and Sri Lanka and on several islands in Oceania. Since this network is deployment based, not all regions of the map are active, and there is no historical archive for end users to download data from. However, as is typical of university-grade research projects, the KOALA monitors are calibrated both indoors and outdoors with a reference-grade instrument to ensure proper performance (Jayaratne et al., 2021; X. Liu et al., 2020; Kuhn et al., 2021).
Another sensor campaign in the Oceania region used Aeroqual ozone sensors in Tāmaki Makaurau / Auckland, Aotearoa / New Zealand, conducted by the University of Auckland (Miskell et al., 2017). The same general framework and ozone sensors were also used in a study in British Columbia, Canada. An in-lab calibration scheme was utilized to apply simple linear corrections to the ozone data (Bart et al., 2014) as well as an outdoor colocation with a reference-grade instrument and field comparisons of individual sensors. Both studies aimed to establish best practices for deploying networked sensors, including the examination of intra- and inter-site differences and the effects of local topography and meteorological conditions on sensor readings.
3.1.7 Campaign, operated by research institution or university in Africa
In Africa, we encountered numerous studies using low-cost sensors to monitor PM (Singh et al., 2021; Abera et al., 2020; Tékouabou et al., 2022; Pope et al., 2018; Raheja et al., 2022b; Hodoli et al., 2023; Lassman et al., 2020; Giordano et al., 2021; Omokungbe et al., 2020; Gryech et al., 2020; Gnamien et al., 2021; Ngo et al., 2019; Bainomugisha et al., 2023; Ndamuzi et al., 2023) but far fewer targeting gas-phase compounds. One reason for this is the prevalence of cookstoves in certain African countries, for which PM is a greater concern than any gaseous pollutant (Alexander et al., 2018). The gas-phase sensor studies we reviewed were less likely to include robust calibration techniques than those of higher-income regions.
Carnegie Mellon University's RAMP sensor network, described in more detail in Sect. 3.1.1, has deployed five sensors in Côte d'Ivoire and another four in Accra, Ghana, as part of their Improving Air Quality in West Africa project (Bahino et al., 2021). These ongoing studies include colocation of several sensors with reference monitors for improved calibration.
In Nairobi, a team of researchers from the Massachusetts Institute of Technology and their collaborators deployed a network of in-house-built sensors measuring NO, NO2, SO2, and PM. This study represents the first time that air quality at schools was studied in Nairobi; thus student and community engagement played a large role in the study. Since the gas sensors exhibited temperature dependencies, a temperature correction was used to ensure data quality. Despite the technical limitations of the sensors, in this context their data were sufficient to begin understanding the differences in air quality at the different schools studied (deSouza et al., 2017).
In Nigeria, one study deployed five low-cost sensors in a semi-urban environment to measure ambient NO, NO2, CO, CO2, O3, and PM. Researchers from Obafemi Awolowo University did not specify any true calibration procedures in their paper but did note previous colocations of the same brand of sensor used with reference-grade instruments in the UK by another research group. Overall, the study was able to examine spatial differences among the five test locations (Owoade et al., 2021). In another Nigerian study, researchers from the Department of Meteorology of the Federal University of Technology Nigeria used portable CO loggers to compare urban and rural pollution. Since a prefabricated monitor was used, no additional calibration or quality assurance was completed (Balogun et al., 2014).
A Moroccan team from Abdelmalek Essaâdi University created and deployed their own low-cost sensor node measuring CO2, NO2, and meteorological data with the goal of creating an algorithm that estimated the air quality index (AQI) from the sensor inputs alone. No gas sensor calibrations were conducted as exact concentrations were not required for their intended purpose. The initial sensor node was successful in predicting the AQI, although the project does not appear to have been scaled up to include more nodes yet (Fahim et al., 2023).
Due to the lack of gas-phase stationary outdoor monitoring studies in Africa, here we list a few related studies outside the scope of our review for further reading. We encountered a mobile monitoring low-cost sensor study of CO, CO2, and PM in Senegal (Ngom et al., 2018). Another study used low-cost sensors measuring CO2, NO2, O3, and PM indoors in Nigeria (Adamu et al., 2023). Although these studies do not fit precisely into our review, they still represent lower-cost methods for improving the understanding of gas-phase compounds in Africa, which is severely understudied.
3.1.8 Campaign, operated by research institution or university in Central and South America
Similar to Africa, the extent of low-cost sensors in Central and South America is extremely limited compared to other regions. Since PM is typically a greater concern than gases in the Global South (Carvalho, 2016), we encountered more sensor networks measuring PM in these areas (Tagle et al., 2020; Jang and Jung, 2023; Lopez-Restrepo et al., 2021; Gramsch et al., 2020, 2021; Candia et al., 2018; Connerton et al., 2023; Dirienzo et al., 2023; Quintero et al., 2023) than those measuring gas-phase compounds, although overall coverage is still sparse. A few mobile sensor studies are available for further reading on gas-phase coverage in Central and South America (Guevara et al., 2010; Alvear-Puertas et al., 2022).
Researchers at the Institute of Geosciences of the University of Brasilia performed in-lab calibrations and applied linear regressions to ensure the quality of their low-cost CO sensors before deploying them in the local area, including during the FIFA World Cup. Data were stored in WebGIS via ArcGIS software, allowing geographic data to be integrated with the CO data (Aguiar et al., 2015). In Argentina, low-cost sensors measuring VOCs were deployed for agricultural monitoring by a team including several researchers from the Universidad Nacional de San Luis (Valenzuela et al., 2018). While this preliminary study focused more on the creation of the sensor platform rather than its calibration or results, it shows promise for the growth of low-cost sensor networks in South America in the coming years.
AQMesh sensors, which are described in more detail in Sect. 3.2, were deployed by the University of Leeds and local collaborators in Nicaragua for 6 months in 2017 downwind of the active Masaya volcano to monitor SO2 and PM. Outdoor colocation with a reference-grade instrument was used to calibrate the sensor signals. Some nodes were more accurate than others in detecting volcanic emissions but were suitable for identifying PM enhancements nonetheless (Whitty et al., 2022). Other studies in Nicaragua and neighboring countries like Costa Rica have employed low-cost sensors on board UASs (Xi et al., 2016; Granados-Bolaños et al., 2021) or other low-cost techniques (Aguilera et al., 2020) to monitor volcanic plumes.
In Peru, sensors measuring CO, NO2, SO2, H2S, O3, CO2, and PM were deployed at four outdoor locations by the Pontifical Catholic University of Peru. The research group built geographic data visualization into their interface and compared measured concentrations among sensors as well as with a nearby government-run air quality monitoring station (David et al., 2015).
In Cienfuegos, Cuba, a low-cost sensor measuring NO2, SO2, O3, CO, and PM was deployed by researchers from Universidad Central “Marta Abreu” de Las Villas and collaborators, primarily for calibration and quality assessment purposes, including two laboratory calibrations and a month-long field deployment near a reference-grade monitor. We have included this calibration-focused study here due to the lack of gas-focused studies in this region, as well as the relevant local sources surrounding the monitor placement, including an oil refinery, thermoelectric plant, charcoal production plant, and diesel-related sources (González Rivero et al., 2023; Martinez et al., 2023).
We also encountered two low-cost sensor network pilot studies in Ecuador by researchers at Universidad San Francisco de Quito USFQ and Universidad Técnica de Cotopaxi. One measured CO, CO2, and PM in three distinct locations. A previous study by the same group conducted a chamber calibration test for the sensors (Guanochanga et al., 2018). Researchers found that for their urban site in Quito, Ecuador, the average CO value was below the target given by the local government but exceeded World Health Organization guidelines (Fuertes et al., 2015).
3.2 Campaign, operated by private companies
Several sensor networks operated by private companies operate using campaign-based deployments. Typically, these companies have their own portals for data visualization and/or analysis, but unlike the quasi-permanent sensor network companies, these data are only available to users who purchase a sensor and a data management plan. These companies focus on targeted air quality solutions for their clients rather than widely available public data or citizen science. Since their full data portals are typically only available on a subscription basis, we have opted to include them in the campaign category because widespread long-term stationary data were not publicly available at the time of writing. The data analysis included with the subscriptions is generally more advanced than that offered by public networks, including advanced calibration procedures and real-time modeling. MODULAIR and Bettair are the only two companies reviewed that directly address the effects of age on their sensors' performance. Peer-reviewed studies concerning the use of the sensor packages in this category tend to be short-term deployments focusing on a specific point source or local phenomenon. These companies are usually headquartered in the US or Europe.
Airly requires its users to purchase a subscription to fully utilize their sensor packages. It is dually headquartered in both Poland and California, US. Their target pollutants include CO, NO2, O3, SO2, and PM (Airly, 2024a). Airly's live map includes data from government-run monitoring stations in addition to its own sensors, notated by different shapes on the map. Historical data can be viewed on the map but only represent the past 24 h, and the end user needs to utilize the API to download it. The map also includes a forecast extending forward 24 h. Airly's sensor calibration procedures are regularly performed, hence incentivizing customers to maintain their subscriptions. Sensors are calibrated in a laboratory setting followed by an outdoor colocation with a reference-grade instrument. Routine monitoring of the sensor package once deployed is a unique aspect of Airly's model, including consideration of local environmental conditions and relevant satellite data to ensure sensor packages are performing adequately during deployment (Airly, 2024b).
The MODULAIR sensor packages by QuantAQ measure CO, CO2, NO, NO2, O3, and PM. Similar to Airly, users need to purchase access to the QuantAQ cloud to access their data and the API. Different pricing plans are offered for “basic”, “pro”, and “enterprise” users, the latter two of which include an annual inspection and recalibration of the sensor, ensuring proper functioning and accuracy of the data. The details of this calibration process are not specified on their public-facing website as of 2023, but it appears that data transparency and calibration models are available to paid-subscription users. Likewise, data visualization is available within the cloud for paid users but has yet to be made publicly available for citizen science use (QuantAQ, 2024). The MODULAIR sensors have been utilized in several peer-reviewed publications (Yang et al., 2022; Prathibha et al., 2020).
Another company attempting to address the limited longevity of its sensors is Barcelona-based Bettair. Rather than reeling its devices in yearly for recalibration, the company sells sensor “cartridges” that are meant to be repurchased every 2 years, ensuring that the sensors are easily replaced at the end of their useful lifetime without having to purchase an entirely new node. Each sensor package measures CO, CO2, NO, NO2, O3, SO2, H2S, and PM (Bettair Cities, 2024). Colocation with reference-grade monitors shows strong agreement for NO, NO2, and O3 (Perello, 2018). Bettair offers a software platform for its users, which includes mapping and other data visualization, but the data are not made publicly available. While the Bettair nodes can be implemented as stationary devices in future studies, to date, the only peer-reviewed studies utilizing them have adapted them as wearables (Kotzagianni et al., 2023; Vrijheid et al., 2021).
Kunak AIR, a company based in Spain, has deployed their sensor packages for CO, O3, NOx, SO2, and PM in short-term campaigns globally, with notable deployments including Ethiopia, Germany, India, Belgium, and Spain (Ibarrola, 2022; Hofman et al., 2022; Saúco et al., 2022). By opting into a paid software package called Kunak AIR Cloud, users unlock access to air quality reports, unspecified calibration and supervision of their sensors, and data visualization and analysis tools, including mapping and basic plotting features (Kunak AIR Cloud, 2024). This company shares many characteristics of the quasi-permanent networks described previously, but we did not encounter any publicized long-term (2+ years) stationary deployments of Kunak sensors in our review.
AQMesh is a UK-based sensor package manufacturer which targets many common pollutants, CO, CO2, NO, NO2, O3, SO2, VOC, and PM, in addition to less commonly studied H2S. Data portal and API access are only available to subscribers (AQMesh, 2024). The AQMesh sensor packages have been used in a wide array of campaign-based research studies (Castell et al., 2018; Jiao et al., 2015; Rodríguez et al., 2020). One study reported in 2017 that the sensors were not yet ready for research-grade applications (Castell et al., 2017), zeroing in on the importance of proper calibration.
Ellona's WT1 electronic nose platform is one of the few sensor nodes studied here which is customizable from a long list of sensors, many of which are less frequently studied (including H2S, NH3, HCl, H2O2, HCHO, CH4, and H2) in addition to the typical pollutant list: CO, CO2, NO, NO2, O3, SO2, VOCs, and PM. Similar to the other campaign-based sensor companies, its data processing, calibration, and visualization procedures are hidden behind a paywall for users only (Ellona, 2024a). At least one peer-reviewed study has utilized the WT1 sensors for campaign-style research efforts as of September 2023 (Panzitta et al., 2022). Ellona is based in France, and their website boasts usage in countries that appeared infrequently elsewhere in our review, including Estonia and Canada (Ellona, 2024b).
India-based Prana Air builds sensor packages offering a similar sensor range: CO, CO2, NO, NO2, O3, SO2, VOC, H2S, NH3, and PM. Sensor packages can be added to their AQI mobile app, which also includes data from regulatory monitoring stations. According to their website, the sensor packages are calibrated “distinctively” (Prana Air, 2024). Studies using the Prana Air monitors seem to focus on PM (Patwardhan et al., 2021; Kumar and Doss, 2023).
Oizom is another privately owned sensor package company with offices in India and France. Their Polludrone platform measures CO, CO2, VOCs, and PM. The sensors include unspecified built-in calibrations and a software package, including live data monitoring and API options. Oizom sensors appear to be gaining international traction, with their website boasting usage of their products in more than 50 countries (Oizom, 2024); peer-reviewed outdoor applications include traffic monitoring in Germany (Kurtenbach et al., 2022) and emissions from fireworks in India (Shankar et al., 2023).
Serbian company ekoNET manufactures sensors that measure CO, CO2, NO, NO2, O3, SO2, and PM. Like most of the companies in this category, web- and app-based data visualization is limited to subscribers, with three different packages available: basic, standard, and advanced (ekoNET, 2024). The ekoNET sensors have been utilized in several peer-reviewed publications to date (Vajs et al., 2021; Jovašević-Stojanović et al., 2015; Drajic and Gligoric, 2020).
3.3 Campaign, operated by government
The two examples of campaign-style low-cost sensor deployments organized by government agencies listed in this section both consist of in-house-built sensor packages deployed for the governing body to measure key pollutants in certain regions and to better understand the use of low-cost sensors themselves. These reports include calibration and data validation efforts such that the governing agency learned best practices to pass on to their constituents.
The U.S. EPA has sponsored various low-cost sensor projects over the years. Its Village Green project focused on community outreach using low-cost sensor packages measuring O3 and PM, later expanding to NO2, VOCs, and black carbon. The project deployed these sensor packages campaign style in a handful of US cities, including Durham, NC; Houston, TX; Washington, DC; Kansas City, KS; Philadelphia, PA; Oklahoma City, OK; Hartford, CT; and Chicago, IL (U.S. EPA, 2014b). Sensor data were compared with those of nearby regulatory-grade monitors to ensure data quality (Jiao et al., 2015).
The Italian government's ENEA (translated, Italian National Agency for New Technologies, Energy and Sustainable Economic Development) has likewise sponsored various network sensor efforts in recent years. During the RES-NOVAE (translated, Networks Buildings Streets: New Challenging Objectives for Environment and Energy) national project, sensor packages designed and built by ENEA called AIRBOX were deployed in Bari, Italy, to measure CO, CO2, NO2, O3, SO2, VOCs, and PM, focusing on roadside emissions (Penza et al., 2017). Early results comparing the sensors with nearby reference monitors were promising (Suriano et al., 2016). ENEA's AIRBOX sensors, not to be confused with ECN's “airboxes” discussed in Sect. 3.1.2, have been used in a small number of other deployments (Borrego et al., 2016). Another ENEA-built sensor package, NASUS, was designed to measure CO, NO2, SO2, H2S, and PM in outdoor deployments and proved effective in lab calibrations (Suriano et al., 2014), in outdoor colocations with reference instruments (Penza et al., 2014), and during a deployment near a landfill (Penza et al., 2015).
The most commonly occurring gas-phase compounds to appear in our review of stationary gas-phase monitoring networks varied by deployment type. Even though we selected for gas-phase networks only, networks that also included PM dominated the quasi-permanent pollutant counts and were sizable in campaign-style deployments as well. NO2, O3, and CO were the most measured gas-phase pollutants for campaign networks. For quasi-permanent networks, O3 was measured more than any other gas-phase compound, followed by CO2 and VOCs. Total and speciated VOCs are lumped into one category, increasing their counts, but the low counts of VOCs overall highlight the need for more observations of these compounds, which are important in both human health and climate change contexts.
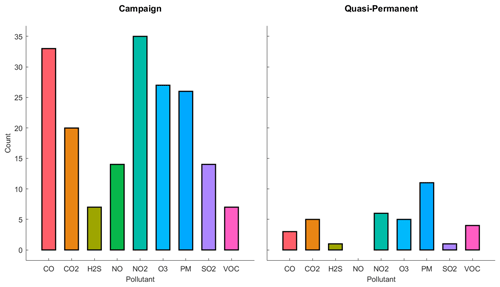
Figure 3Bar chart of pollutants in each selected sensor package. Note that a sensor package must measure at least one gas-phase compound to be included; no standalone PM sensors are listed here.
We also sought to characterize global coverage and biases in low-cost sensor placement by mapping the locations of each of the sensor networks studied. Note that for the campaign-based studies, we sought to include at least two networks from each geographic area, although some regions (North America and Europe) were much easier to find information on than others (the Middle East, Central and South America, Oceania), which is reflected in Fig. 4. In the regions with sparser sensor sampling, the networks also tended to be smaller in scale with less longevity compared to more commonly sampled regions; we encountered networks with few nodes and more standalone studies as opposed to multiple deployments of the same sensor packages.
For the quasi-permanent networks, the vast majority either were US based or featured global coverage, although most of the networks with global coverage had their headquarters based in the US. Overall, we found that monitors are concentrated in the Northern Hemisphere mid-latitudes, in high- and some middle-income countries, and in urban areas. Ironically, low-cost sensors are an attractive solution to this bias in monitoring locations, but clearly more needs to be done, perhaps by local governments or even sensor companies themselves, to distribute the technology into less commonly studied areas.
Since one of the biggest issues plaguing low-cost sensor usage is data quality, we also sorted each of the networks we reviewed by their calibration method, as shown in Fig. 5. Most sensor networks reviewed relied on colocation of the sensors with a reference-grade instrument, making this the gold standard for sensor calibration. However, it is important to note that this category is extremely broad and that variations in the implementation of colocations can still affect sensor data quality considerably.
For instance, some networks may only colocate one of their sensors with the reference instrument (e.g., Love My Air Denver), which does not consider variations among individual sensors. Others that colocate their sensors with a reference instrument may do so as more of a quality check than a true calibration; a sensor is considered “good enough” if concentrations between the sensor and reference instrument do not deviate beyond a set range. The most robust in this category are sensor networks that use this colocation period to derive calibration algorithms, ensuring that the sensor data are as close to the reference data as possible. Even within the algorithm category, some networks may use a single correction factor, while others will use machine learning or other more sophisticated methods to achieve the best fit possible (e.g., Y-Pods).
There is also a time component to sensor calibrations due to both sensor age and seasonality. Sensor performance tends to decline as they age (Rai et al., 2017), with most sensors' useful life hovering around approximately 2 years (Okorn et al., 2021). Beyond this point, their performance may become less reliable, and drift may become evident in the signals. Likewise, low-cost sensors can be very sensitive to environmental conditions, including temperature, pressure, and humidity (Okorn et al., 2021; Jayaratne et al., 2021), so a calibration conducted in hot, humid summer weather may not translate well to cold, dry winter conditions experienced at the same location during different times of the year. Thus, these broad-brush categories do not control for the frequency of calibration or the robustness of the comparison or algorithm.
The networks included in the “not reported” calibration category did not provide specific information on their calibration techniques in publications or on their websites at the time of this review. Note that the researchers may have chosen to withhold details for proprietary reasons, in the case of private companies, or may not have performed any calibration on their sensors. In either case, we did not have sufficient information to categorize their techniques into any other category. The “varies by end user” category implies just that: we found several groups employing the sensors in both campaign and quasi-permanent structures, with each group choosing their own calibration scheme for the same sensor. Likewise, several groups in each category pointed back to materials on the sensor's effectiveness as reported by the manufacturer in lieu of conducting their own calibration. Again, due to the environmental dependence of low-cost sensors, outdoor colocations are crucial for understanding how the sensor will respond in its deployment environment, which all other calibration methods are deficient in.
On a related note, data quality does not necessitate data availability. The highest-quality sensor data, calibrated via colocation with subsequent fitting algorithms, are most likely to hail from university or research groups, which are also the least likely to ensure public data access. While most data collected by these groups are available upon request, end users outside the scientific community are less likely to know where to look for data that are not available in a public repository.
In the wake of the abundance of sensor networks springing up worldwide, several websites and databases have emerged in attempts to highlight noteworthy efforts in the space and make it easier for sensor users and potential data end users to find sensor networks and data that match their needs. Most of the sensor unification efforts we studied included lists of sensor networks with links to their individual websites. Several others include an interface for data visualization – typically a map with pins for each sensor node, with different colors corresponding to different concentration ranges. These may also include a data download or API option, but none of the sensor network unification efforts we studied include specifics on data quality broken down by each sensor type.
As discussed in the previous sections, calibrations are not created equal among sensor networks; while some include vigorous, frequent calibration procedures, others are seldom calibrated, if at all. Sensor synthesis efforts could be made stronger with more emphasis on data quality, ensuring that the end user understands the uncertainty in the reported values. Furthermore, having a standard data format and download option would make it easier for users outside the scientific community to access, combine, and analyze data that might be relevant to them. Below, we describe several examples of data unification projects, highlighting both their strengths and their limitations.
Several of the long-term monitoring networks discussed in Sect. 2 operate de facto data unification products, with their data maps including data from multiple low-cost sensor networks and regulatory monitors (e.g., AirNow's Fire and Smoke Map, Clarity, TELLUS). These data unification efforts to date have broadly overlooked the low-cost sensor networks established by university and other small-scale research groups, focusing instead on other companies or non-profit organizations. However, it is precisely this smaller scale that allows sensor researchers in this space to develop state-of-the-art calibration procedures; academic institutions tend to have the highest degree of certainty and calibration in their data (see Sects. 2.3 and 3.1).
Beyond these, there are a small number of projects that aim to highlight data from a variety of different sensor networks. The Clean Air Monitoring and Solutions Network (CAMS-Net) is an NSF-funded project which aims to create a “network of networks”, making air quality data from a variety of sources publicly accessible in one digital space, with a focus on low-cost sensors. As of 2023, their website hosts an extensive list of participating networks but does not yet have a large, publicly available data repository (CAMS-Net, 2024). OpenAQ is a non-profit organization that similarly aims to unify air quality sensor data, including an interactive map and API for data downloads (OpenAQ, 2024). RIVM, a government division in the Netherlands, has created a data portal dubbed Measuring Together, where citizen scientists can upload their low-cost sensor data (Wesseling et al., 2019).
Another major player in the sensor network unification space is the World Air Quality Index, which operates as a non-profit organization (Real-time Air Quality Sensor Networks, 2024). The site's strengths include live mapping, basic plotting, a link to the network's own website, and an option to download historical data. For users wanting to add their own data to the site, it encourages the use of GAIA sensors, which are PM focused, with the option to add a CO2 sensor only for an additional cost. However, it also supports data from other sensor manufacturers and networks. Like the other sensor unification projects, end users wanting more detailed information about each network will need to follow links, which sometimes direct the user to information on the deployment location rather than the sensor network itself. For instance, a sensor deployed on the roof of an embassy might link to the embassy's website regardless of whether the embassy or a third party was responsible for the deployment and upkeep of the sensor, leaving the user with little additional information. Likewise, multiple different sensor networks may be represented on an individual sub-map, which can make determining the source of data difficult. For users who are merely interested in the data at face value or want to understand how many monitors are already present in a certain area, tools like this are immensely helpful in mapping current sensor efforts. However, more advanced end users who want to understand the measurement and calibration techniques behind each sensor should expect to do a lot more digging to find this information.
We categorize these projects combining sensor data from a variety of sources in a list or map format as sensor unification focused, making it possible for end users to locate real-time or historical data that may be personally relevant. While these projects can be useful, sensor harmonization should be the next step in increasing the usefulness of sensor data from a variety of networks. Data “harmonization” as used here follows the intent of Zeb et al. (2021) of “reconciling various types, levels and sources of data in formats that are compatible and comparable, and thus useful for better decision-making” and the principles of Cheng et al. (2024). In the context of low-cost sensors, harmonization includes taking additional steps to ensure the seamless use of data: simple enough for citizen scientists to access them, yet quality-assured for scientists and other advanced users. This is no small task, but steps in this direction might include a common download format for sensor data, availability of both live and historical data, and information on data quality or calibration procedures. Sensor data harmonization projects could take sensor unification efforts to the next level by making sensor data more practical for scientific use. One recent example of a call for data harmonization is Schneider et al.'s (2019) scientific opinion piece, which aims to create standard terminology for processed low-cost sensor data, ranging from raw measurements (level 0) to data that have been corrected using nearby reference stations and/or measured environmental parameters (level 2A–B). The highest prescribed level (4) describes spatially continuous maps derived from sensor data, including assimilation into models. The authors state that these levels will provide end users with improved data integrity and transparency without requiring researchers to disclose proprietary calibration algorithms.
In the most recent low-cost sensor studies (spanning approximately 2020 to present), an emerging trend is incorporating sensors into larger-scale modeling. Many of the application-based projects included in our review tend to view each sensor data point as individual, e.g., for discerning air quality at a specific point in space and time, typically for comparative purposes with other sensor nodes. In more recent studies, however, we encountered many more applications in geospatial interpolation models such as kriging across sensor nodes to estimate pollutant concentrations not only at sensor locations, but also between them in an even finer grid (Lee et al., 2024; J. Liu et al., 2022; Nori-Sarma et al., 2020).
While low-cost sensors have long been implemented as a solution to gaps in traditional air quality monitoring techniques, only more recently have they been used to complement other monitoring efforts via data fusion techniques. In these kinds of studies, low-cost sensor measurements are typically coupled with a combination of regulatory monitoring, satellite data, and model outputs, with different weights assigned to each data stream according to the estimated data quality (Yoo et al., 2020; Bobbia et al., 2022; Gressent et al., 2020; Liang et al., 2023; Li et al., 2020; Dewage et al., 2024). Including low-cost sensor data in these models can improve forecasting (Malings et al., 2021).
A smaller number of studies we found noteworthy utilize low-cost sensors to estimate pollutant flux across a region, since finely spaced sensors can essentially act as a grid to estimate across (Turner et al., 2020). Low-cost gas sensors have also been used in flux chambers to aid evaluation efforts (Bastviken et al., 2020). Overall, we expect to see the point-source and general monitoring efforts described in Sects. 2 and 3 continue to flourish in the next decade. Further evolution of these approaches and advanced modeling-based studies will require improvement in data quality and spatial coverage of trace gas sensing networks.
Current and emerging sensor directions for non-stationary deployments include the increased use of air quality sensors on UASs, which can afford air quality monitoring in previously inaccessible areas. Common applications in this area include measuring emissions from wildfires (Bushnaq et al., 2021; Afghah et al., 2019; Mo et al., 2021), measuring emissions from volcanoes (Villa et al., 2016; Granados-Bolaños et al., 2021; Xi et al., 2016), and gas leak detection (Pochwala et al., 2020; S. Liu et al., 2020; Sonkar et al., 2022). Mobile sensors have also been deployed indoors on ships (Schalm et al., 2022; Metreveli, 2021), and complementary stationary deployments at ports are helping to assess emissions and the influence of maritime meteorology on air quality (Jayaratne et al., 2020; Zhou et al., 2022; Gagic et al., 2022).
Particulate matter is the most frequently studied pollutant using low-cost sensors. In regions with sparse gas-phase sensor data, we could always find several examples reporting particulate matter. Even among the gas-phase networks studied here, most multi-pollutant sensor packages also included a PM sensor, especially among the largest networks. Despite the surge in low-cost sensor networks in the past decade, more monitoring is needed to better address air quality concerns and disparities on global and regional scales. At present, Central and South America, the Middle East, and Africa are especially lacking. Although larger networks such as PurpleAir service these areas, coverage is significantly less dense compared to the US and western Europe. Even in countries with higher spatial coverage of sensors, rural areas lack the same coverage as their urban counterparts, and countries with higher gross domestic product tend to have more sensors – the differences between western and eastern Europe are a prime example. Less wealthy and more rural areas tend to have more regulatory monitoring gaps; closing the gap with well-calibrated low-cost sensors is an important first step towards adequate monitoring in underserved areas. Likewise, satellite- and model-derived projections of climate-related impacts will be improved by well-calibrated, globally distributed, high-density surface observations of trace gases, particularly in regions with regulatory monitoring gaps. Forward progress on complicated issues of human health and environmental justice will require significant expansion of the spatial coverage of hydrocarbon trace gas sensors, particularly with attention to detection of specific toxic compounds.
Data quality and availability also plague the use of low-cost sensors. This review found that the highest-quality sensor data are obtained by utilizing an outdoor colocation with a reference instrument and subsequent algorithm development to ensure a proper fit. While most campaign and quasi-permanent sensor networks alike rely on a colocation approach, university-based and other research groups typically remain the highest quality. One reason for this is their “gold-standard” post-colocation algorithms, which bring the response of the sensors closer to the true value observed during the colocation period. Another strength of university-based projects is the smaller number of nodes usually deployed; the larger the network and the more area covered, the less likely that every node has been properly calibrated.
However, data from research-focused networks are typically the hardest to access, with very few publicly available data portals. Most data are available by request only and lack a standard format, making them inaccessible for most citizen science or education outreach purposes. In contrast, many privately owned sensor companies, particularly in the quasi-permanent category, make their dataset publicly available, despite lacking these rigorous calibration procedures. Research-grade end users have largely deemed global or country-wide calibrations inadequate, especially during wildfires or other extreme weather events. Addressing sensor deployment gaps in trace gases and global spatial coverage and sensor data gaps in quality and availability will ensure that low-cost sensors remain a valuable tool for decades to come.
The figures in this paper were created using the MATLAB Gramm package (Morel, 2018; https://doi.org/10.21105/joss.00568); contact the corresponding author for access.
For additional sensor network metrics, contact the corresponding author or the network directly.
KO: conceptualization, data curation, formal analysis, writing (original draft preparation). LTI: conceptualization, writing (review and editing).
The contact author has declared that neither of the authors has any competing interests.
Publisher’s note: Copernicus Publications remains neutral with regard to jurisdictional claims made in the text, published maps, institutional affiliations, or any other geographical representation in this paper. While Copernicus Publications makes every effort to include appropriate place names, the final responsibility lies with the authors.
Kristen Okorn was supported by an appointment to the NASA Postdoctoral Program at NASA Ames Research Center, administered by Oak Ridge Associated Universities under contract with NASA. Laura T. Iraci acknowledges support from the NASA Earth Science Research and Analysis Program.
This research has been supported by NASA under the Postdoctoral Program (grant no. 80HQTR21CA005), the Early Career Research Program, and the GLOBE Program (award no. 23-ECIPES23-0202).
This paper was edited by Haichao Wang and reviewed by two anonymous referees.
Abera, A., Mattisson, K., Eriksson, A., Ahlberg, E., Sahilu, G., Mengistie, B., Bayih, A. G., Aseffaa, A., Malmqvist, E., and Isaxon, C: Air pollution measurements and land-use regression in urban sub-Saharan Africa using low-cost sensors – Possibilities and pitfalls, Atmosphere, 11, 1357, https://doi.org/10.3390/atmos11121357, 2020.
Adamu, A., Arifalo, K. M., and Abulude, F. O.: Indoor air quality assessment using a low-cost sensor: A case study in ikere-ekiti, nigeria, Engineering Proceedings, 58, 42, https://doi.org/10.3390/ecsa-10-16021, 2023.
Afghah, F., Razi, A., Chakareski, J., and Ashdown, J.: Wildfire Monitoring in Remote Areas using Autonomous Unmanned Aerial Vehicles, in: IEEE INFOCOM 2019 - IEEE Conference on Computer Communications Workshops, Paris, France, 29 April–2 May 2019, IEEE, 835–840, https://doi.org/10.1109/INFCOMW.2019.8845309, 2019.
Agrawal, G., Mohan, D., and Rahman, H.: Ambient air pollution in selected small cities in India: Observed trends and future challenges, IATSS Research, 45, 19–30, https://doi.org/10.1016/j.iatssr.2021.03.004, 2021.
AGU: AGU Fall Meeting 2022, Chicago, IL, 12–16 December 2022, https://www.agu.org/fall-meeting-2022 (last access: 17 September 2023), 2022.
Aguiar, E. F. K., Roig, H. L., Mancini, L. H., and and de Carvalho, E. N. C. B.: Low-cost sensors calibration for monitoring air quality in the federal district – Brazil, Journal of Environmental Protection, 6, 173–189, https://doi.org/10.4236/jep.2015.62019, 2015.
Aguilera, F., Layana, S., Rojas, F., Arratia, P., Wilkes, T. C., González, C., Inostroza, M., McGonigle, A. J. S., Pering, T. D., and Ureta, G.: First measurements of gas flux with a low-cost smartphone sensor-based UV camera on the volcanoes of northern Chile, Remote Sens.-Basel, 12, 2122, https://doi.org/10.3390/rs12132122, 2020.
AirCare: Free air quality and pollen tracking app, https://getaircare.com/, last access: 10 March 2024.
AirGradient: https://www.airgradient.com/, last access: 26 August 2024.
Airly: Data platform and monitors, https://airly.org/en/, last access: 10 March 2024a.
Airly: Quality & certificates, https://airly.org/en/features/quality- and-certificates/, last access: 10 March 2024b.
Air Matters: https://air-matters.com/, last access: 10 March 2024.
AirNow: https://www.airnow.gov/, last access: 10 March 2024a.
AirNow: Fire and Smoke Map, https://fire.airnow.gov/, last access: 10 March 2024b.
AirNow: Fire and Smoke Map – Getting Started, https://document.airnow.gov/airnow-fire-and-smoke-map-getting-started.pdf, last access: 10 March 2024c.
AiREAS: Real time measurements, https://aireas.com/en/real- time-measurements/, last access: 25 October 2024.
AIRU: https://airu.coe.utah.edu/, last access: 9 March 2024.
AirWatchSTL: https://airwatchstl.mcustlouis.org/, last access: 9 March 2024.
Alexander, D. A., Northcross, A., Karrison, T., Morhasson-Bello, O., Wilson, N., Atalabi, O. M., Dutta, A., Adu, D., Ibigbami, T., Olamijulo, J., Adepoju, D., Ojengbede, O., and Olopade, C. O.: Pregnancy outcomes and ethanol cook stove intervention: A randomized-controlled trial in Ibadan, Nigeria, Environ. Int., 111, 152–163, https://doi.org/10.1016/j.envint.2017.11.021, 2018.
Alford, K. L. and Kumar, N.: Pulmonary health effects of indoor volatile organic compounds – A meta-analysis, Int. J. Env. Res. Pub. He., 18, 1578, https://doi.org/10.3390/ijerph18041578, 2021.
Al-Hajjaji, K., Ezzin, M., Khamdan, H., Hassani, A. E., and Zorba, N.: Design, development and evaluation of a UAV to study air quality in Qatar, arXiv [preprint], https://doi.org/10.48550/arXiv.1709.05628, 17 September 2017.
Alvear-Puertas, V. E., Burbano-Prado, Y. A., Rosero-Montalvo, P. D., Tözün, P., Marcillo, F., and Hernandez, W.: Smart and portable air-quality monitoring IOT low-cost devices in Ibarra city, Ecuador, Sensors, 22, 7015, https://doi.org/10.3390/s22187015, 2022.
Amegah, A. K.: Proliferation of low-cost sensors. What prospects for air pollution epidemiologic research in Sub-Saharan Africa?, Environ. Pollut., 241, 1132–1137, https://doi.org/10.1016/j.envpol.2018.06.044, 2018.
AQMesh: Outdoor air quality monitoring system, https://www.aqmesh.com/, last access: 1 September 2024.
Arnold, C., Harms, M., and Goschnick, J.: Air quality monitoring and fire detection with the Karlsruhe electronic micronose KAMINA, IEEE Sens. J., 2, 179–188, https://doi.org/10.1109/JSEN.2002.800681, 2002.
Awokola, B., Okello, G., Johnson, O., Dobson, R., Ouédraogo, A. R., Dibba, B., Ngahane, M., Ndukwu, C., Agunwa, C., Marangu, D., Lawin, H., Ogugua, I., Eze, J., Nwosu, N., Ofiaeli, O., Ubuane, P., Osman, R., Awokola, E., Erhart, A., Mortimer, K., Jewell, C., and Semple, S.: Longitudinal ambient PM2.5 measurement at fifteen locations in eight sub-Saharan African countries using low-cost sensors, Atmosphere, 13, 1593, https://doi.org/10.3390/atmos13101593, 2022.
Awokola, B. I., Okello, G., Mortimer, K. J., Jewell, C. P., Erhart, A., and Semple, S.: Measuring air quality for advocacy in africa (MA3): Feasibility and practicality of longitudinal ambient PM2.5 measurement using low-cost sensors, Int. J. Env. Res. Pub. He., 17, 7243, https://doi.org/10.3390/ijerph17197243, 2020.
Badura, M., Batog, P., Drzeniecka-Osiadacz, A., and Modzel, P.: Low- and medium-cost sensors for tropospheric ozone monitoring – Results of an evaluation study in Wrocław, Poland, Atmosphere, 13, 542, https://doi.org/10.3390/atmos13040542, 2022.
Bahino, J., Giordano, M., Yoboué, V., Ochou, A., Galy-Lacaux, C., Liousse, C., Amegah, K., Hugues, A., Nimo, J., Beekmann, M., and Subramanian, R.: MOPGA/Improving Air Quality in West Africa: Low-cost sensors as a solution to improve the understanding of spatial and temporal variability in urban air pollution, EGU General Assembly 2021, online, 19–30 Apr 2021, EGU21-8210, https://doi.org/10.5194/egusphere-egu21-8210, 2021.
Bainomugisha, E., Ssematimba, J., and Okure, D.: Design considerations for a distributed low-cost air quality sensing system for urban environments in low-resource settings, Atmosphere, 14, 354, https://doi.org/10.3390/atmos14020354, 2023.
Bales, E., Nikzad, N., Quick, N., Ziftci, C., Patrick, K., and Griswold, W.: CitiSense: Mobile air quality sensing for individuals and communities. Design and deployment of the CitiSense mobile air-quality system, in: Proceedings of the 6th International Conference on Pervasive Computing Technologies for Healthcare, 6th International Conference on Pervasive Computing Technologies for Healthcare, San Diego, United States, 21–24 May 2012, IEEE, https://doi.org/10.4108/icst.pervasivehealth.2012.248724, 2012.
Balogun, I. A., Balogun, A. A., and Adegoke, J.: Carbon monoxide concentration monitoring in Akure – A comparison between urban and rural environment, Journal of Environmental Protection, 5, 266–273, https://doi.org/10.4236/jep.2014.54030, 2014.
Barcelo-Ordinas, J. M., Ferrer-Cid, P., Garcia-Vidal, J., Ripoll, A., and Viana, M.: Distributed multi-scale calibration of low-cost ozone sensors in wireless sensor networks, Sensors, 19, 2503, https://doi.org/10.3390/s19112503, 2019.
Barcelo-Ordinas, J. M., Ferrer-Cid, P., Garcia-Vidal, J., Viana, M., and Ripoll, A.: H2020 project CAPTOR dataset: Raw data collected by low-cost MOX ozone sensors in a real air pollution monitoring network, Data in Brief, 36, 107127, https://doi.org/10.1016/j.dib.2021.107127, 2021.
Barkjohn, K. K., Gantt, B., and Clements, A. L.: Development and application of a United States-wide correction for PM2.5 data collected with the PurpleAir sensor, Atmos. Meas. Tech., 14, 4617–4637, https://doi.org/10.5194/amt-14-4617-2021, 2021.
Barkjohn, K. K., Holder, A. L., Frederick, S. G., and Clements, A. L.: Correction and accuracy of PurpleAir PM2.5 measurements for extreme wildfire smoke, Sensors, 22, 9669, https://doi.org/10.3390/s22249669, 2022.
Barot, V., Kapadia, V., and Pandya, S.: QOS enabled IOT based low cost air quality monitoring system with power consumption optimization, Cybernetics and Information Technologies, 20, 122–140, https://doi.org/10.2478/cait-2020-0021, 2020.
Bart, M., Williams, D. E., Ainslie, B., McKendry, I., Salmond, J., Grange, S. K., Alavi-Shoshtari, M., Steyn, D., and Henshaw, G. S.: High density ozone monitoring using gas sensitive semi-conductor sensors in the lower Fraser Valley, British Columbia, Environm. Sci. Technol., 48, 3970–3977, https://doi.org/10.1021/es404610t, 2014.
Bastviken, D., Nygren, J., Schenk, J., Parellada Massana, R., and Duc, N. T.: Technical note: Facilitating the use of low-cost methane (CH4) sensors in flux chambers – calibration, data processing, and an open-source make-it-yourself logger, Biogeosciences, 17, 3659–3667, https://doi.org/10.5194/bg-17-3659-2020, 2020.
BEACO2N: http://beacon.berkeley.edu/, last access: 10 March 2024.
Becnel, T. and Gaillardon, P.-E.: A deep learning approach to sensor fusion inference at the edge, in: 2021 Design, Automation and Test in Europe Conference and Exhibition (DATE), Grenoble, France, 1–5 February 2021, IEEE, 1420–1425, https://doi.org/10.23919/DATE51398.2021.9474178, 2021.
Becnel, T., Sayahi, T., Kelly, K., and Gaillardon, P.-E.: A recursive approach to partially blind calibration of a pollution sensor network, in: 2019 IEEE International Conference on Embedded Software and Systems (ICESS), Las Vegas, NV, USA, 2–3 June 2019, IEEE, 1–8, https://doi.org/10.1109/ICESS.2019.8782523, 2019a.
Becnel, T., Tingey, K., Whitaker, J., Sayahi, T., Lê, K., Goffin, P., Butterfield, A., Kelly, K., and Gaillardon, P.-E.: A distributed low-cost pollution monitoring platform, IEEE Internet Things, 6, 10738–10748, https://doi.org/10.1109/JIOT.2019.2941374, 2019b.
Bettair cities: https://bettaircities.com/, last access: 10 March 2024.
Bittner, A. S., Cross, E. S., Hagan, D. H., Malings, C., Lipsky, E., and Grieshop, A. P.: Performance characterization of low-cost air quality sensors for off-grid deployment in rural Malawi, Atmos. Meas. Tech., 15, 3353–3376, https://doi.org/10.5194/amt-15-3353-2022, 2022.
Bobbia, M., Poggi, J., and Portier, B.: Spatial correction of low-cost sensors observations for fusion of air quality measurements, Appl. Stoch. Model. Bus., 38, 766–786, https://doi.org/10.1002/asmb.2713, 2022.
Borrego, C., Costa, A. M., Ginja, J., Amorim, M., Coutinho, M., Karatzas, K., Sioumis, Th., Katsifarakis, N., Konstantinidis, K., De Vito, S., Esposito, E., Smith, P., André, N., Gérard, P., Francis, L. A., Castell, N., Schneider, P., Viana, M., Minguillón, M. C., Reimringer, W., Otjes, R. P., von Sicard, O., Pohle, R., Elen, B., Suriano, D., Pfister, V., Prato, M., Dipinto, S., and Penza, M.: Assessment of air quality microsensors versus reference methods: The EuNetAir joint exercise, Atmos. Environ., 147, 246–263, https://doi.org/10.1016/j.atmosenv.2016.09.050, 2016.
Brauer, M., Guttikunda, S. K., K A, Nishad, Dey, S., Tripathi, S. N., Weagle, C., and Martin, R. V.: Examination of monitoring approaches for ambient air pollution: A case study for India, Atmos. Environ., 216, 116940, https://doi.org/10.1016/j.atmosenv.2019.116940, 2019.
Brilli, L., Berton, A., Carotenuto, F., Gioli, B., Gualtieri, G., Martelli, F., Profeti, S., Trombi, G., Dibari, C., Moriondo, M., Vagnoli, C., and Zaldei, A.: Innovative low-cost air quality stations as a supporting means for road traffic regulations in urban areas, IOP Conf. Ser.-Earth Environ. Sci., 489, 012023, https://doi.org/10.1088/1755-1315/489/1/012023, 2020.
Brilli, L., Carotenuto, F., Gioli, B., Berton, A., Profeti, S., Gualtieri, G., Andreini, B. P., Stefanelli, M., Martelli, F., Vagnoli, C., and Zaldei, A.: An integrated low-cost monitoring platform to assess air quality over large areas, in: Proceedings of the Future Technologies Conference (FTC) 2020, edited by: Arai, K., Kapoor, S., and Bhatia, R., Springer International Publishing, 2, 965–975, https://doi.org/10.1007/978-3-030-63089-8_63, 2021.
Brynda, P., Kopřiva, J., and Horák, M.: Trafficsensnet sensor network for measuring emissions from transportation, Procedia Engineer., 120, 902–907, https://doi.org/10.1016/j.proeng.2015.08.781, 2015.
Brynda, P., Kosová, Z., and Kopřiva, J.: Mobile sensor unit for online air quality monitoring, in: 2016 Smart Cities Symposium Prague (SCSP), Prague, Czech Republic, 26–27 May 2016, IEEE, 1–4, https://doi.org/10.1109/SCSP.2016.7501028, 2016.
Brynda, P., Honzík, P., Kosová, Z., and Šimonová, K.: Experience from the pilot project of the air quality sensor network in Litoměřice, in: 2020 Smart City Symposium Prague (SCSP), Prague, Czech Republic, 25–25 June 2020, IEEE, 1–6, https://doi.org/10.1109/SCSP49987.2020.9133785, 2020.
Brzeziński, M., Sałach, K., and Wroński, M.: Wealth inequality in Central and Eastern Europe: Evidence from household survey and rich lists' data combined∗, Economics of Transition and Institutional Change, 28, 637–660, https://doi.org/10.1111/ecot.12257, 2020.
Bushnaq, O. M., Chaaban, A., and Al-Naffouri, T.: The Role of UAV-IoT Networks in Future Wildfire Detection, IEEE Internet Things, 8, 23, https://doi.org/10.1109/JIOT.2021.3077593, 2021.
Byrne, R., Ryan, K., Venables, D. S., Wenger, J. C., and Hellebust, S.: Highly local sources and large spatial variations in PM2.5 across a city: evidence from a city-wide sensor network in Cork, Ireland, Environmental Science: Atmospheres, 3, 919–930, https://doi.org/10.1039/D2EA00177B, 2023.
California Air Resources Board: National Ambient Air Quality Standards, https://ww2.arb.ca.gov/resources/national-ambient-air-quality-standards, last access: 17 September 2023.
Campmier, M. J., Gingrich, J., Singh, S., Baig, N., Gani, S., Upadhya, A., Agrawal, P., Kushwaha, M., Mishra, H. R., Pillarisetti, A., Vakacherla, S., Pathak, R. K., and Apte, J. S.: Seasonally optimized calibrations improve low-cost sensor performance: long-term field evaluation of PurpleAir sensors in urban and rural India, Atmos. Meas. Tech., 16, 4357–4374, https://doi.org/10.5194/amt-16-4357-2023, 2023.
Camprodon, G., González, Ó., Barberán, V., Pérez, M., Smári, V., de Heras, M. Á., and Bizzotto, A.: Smart Citizen Kit and Station: An open environmental monitoring system for citizen participation and scientific experimentation, HardwareX, 6, e00070, https://doi.org/10.1016/j.ohx.2019.e00070, 2019.
CAMS-Net (Clean Air Monitoring and Solutions Network): https://camsnet.org/, last access: 10 March 2024.
Candia, A., Represa, S. N., Giuliani, D., Luengo, M. Á., Porta, A. A., and Marrone, L. A.: Solutions for SmartCities: Proposal of a monitoring system of air quality based on a LoRaWAN network with low-cost sensors, in: 2018 Congreso Argentino de Ciencias de La Informática y Desarrollos de Investigación (CACIDI), Buenos Aires, Argentina, 28–30 November 2018, IEEE, 1–6, https://doi.org/10.1109/CACIDI.2018.8584183, 2018.
Čapek, S. M.: The “environmental justice” frame: A conceptual discussion and an application, Soc. Probl., 40, 5–24, https://doi.org/10.2307/3097023, 1993.
Carotenuto, F., Brilli, L., Gioli, B., Gualtieri, G., Vagnoli, C., Mazzola, M., Viola, A. P., Vitale, V., Severi, M., Traversi, R., and Zaldei, A.: Long-term performance assessment of low-cost atmospheric sensors in the arctic environment, Sensors, 20, 1919, https://doi.org/10.3390/s20071919, 2020.
Carotenuto, F., Bisignano, A., Brilli, L., Gualtieri, G., and Giovannini, L.: Low-cost air quality monitoring networks for long-term field campaigns: A review, Meteorol. Appl., 30, e2161, https://doi.org/10.1002/met.2161, 2023.
Carvalho, H.: The air we breathe: Differentials in global air quality monitoring, Lancet Resp. Med., 4, 603–605, https://doi.org/10.1016/S2213-2600(16)30180-1, 2016.
Casey, J. G., Collier-Oxandale, A., and Hannigan, M.: Performance of artificial neural networks and linear models to quantify 4 trace gas species in an oil and gas production region with low-cost sensors, Sensor. Actuat. B-Chem., 283, 504–514, https://doi.org/10.1016/j.snb.2018.12.049, 2019.
Castell, N., Dauge, F. R., Schneider, P., Vogt, M., Lerner, U., Fishbain, B., Broday, D., and Bartonova, A.: Can commercial low-cost sensor platforms contribute to air quality monitoring and exposure estimates?, Environ. Int., 99, 293–302, https://doi.org/10.1016/j.envint.2016.12.007, 2017.
Castell, N., Schneider, P., Grossberndt, S., Fredriksen, Mirjam. F., Sousa-Santos, G., Vogt, M., and Bartonova, A.: Localized real-time information on outdoor air quality at kindergartens in Oslo, Norway using low-cost sensor nodes, Environ. Res., 165, 410–419, https://doi.org/10.1016/j.envres.2017.10.019, 2018.
Chai, T., Kim, H., Pan, L., Lee, P., and Tong, D.: Impact of moderate resolution imaging spectroradiometer aerosol optical depth and AirNow PM2.5 assimilation on community multi-scale air quality aerosol predictions over the contiguous united states, J. Geophys. Res.-Atmos., 122, 5399–5415, https://doi.org/10.1002/2016JD026295, 2017.
Chang, J. B., Liu, V., Subramanian, V., Sivula, K., Luscombe, C., Murphy, A., Liu, J., and Fréchet, J. M. J.: Printable polythiophene gas sensor array for low-cost electronic noses, J. Appl. Phys., 100, 014506, https://doi.org/10.1063/1.2208743, 2006.
Chatzidiakou, L., Archer, R., Beale, V., Bland, S., Carter, H., Castro-Faccetti, C., Edwards, H., Finneran, J., Hama, S., Jones, R. L., Kumar, P., Linden, P. F., Rawat, N., Roberts, K., Symons, C., Vouriot, C., Wang, D., Way, L., West, S., Weston, D., Williams, N., Wood, S., and Burridge, H. C.: Schools' air quality monitoring for health and education: Methods and protocols of the SAMHE initiative and project, Developments in the Built Environment, 16, 100266, https://doi.org/10.1016/j.dibe.2023.100266, 2023.
Cheadle, L., Deanes, L., Sadighi, K., Gordon Casey, J., Collier-Oxandale, A., and Hannigan, M.: Quantifying neighborhood-scale spatial variations of ozone at open space and urban sites in Boulder, Colorado using low-cost sensor technology, Sensors, 17, 2072, https://doi.org/10.3390/s17092072, 2017.
Chen, L. C. and Lippmann, M.: Effects of metals within ambient air particulate matter (PM) on human health, Inhal. Toxicol., 21, 1–31, https://doi.org/10.1080/08958370802105405, 2009.
Chen, W., Yang, Y., Mei, H., Sun, H., Louie, P. K. K., Jiang, S. Y., and Ning, Z.: Analysis of an ozone episode in the Greater Bay Area based on low-cost sensor network, Atmos. Environ., 322, 120367, https://doi.org/10.1016/j.atmosenv.2024.120367, 2024.
Cheng, C., Messerschmidt, L., Bravo, I., Waldbauer, M., Bhavikatti, R., Schenk, C., Grujic, V., Model, T., Kubinec, R., and Barceló, J.: A general primer for data harmonization, Scientific Data, 11, 152, https://doi.org/10.1038/s41597-024-02956-3, 2024.
Cheng, S., Chang-Chien, G.-P., Huang, Q., Zhang, Y.-B., Yan, P., Zhang, J., Wang, Y., Zhang, D., and Teng, G.: Global research trends in health effects of volatile organic compounds during the last 16 years: A bibliometric analysis, Aerosol Air Qual. Res., 19, 1834–1843, https://doi.org/10.4209/aaqr.2019.06.0327, 2019.
Children's Health Alliance of Wisconsin: Love my air Wisconsin, https://www.chawisconsin.org/initiatives/environmental-health/air-quality/, last access: 10 March 2024.
Clarity Movement Co.: https://www.clarity.io/, last access: 10 March 2024a.
Clarity Movement Co.: Open map, https://openmap.clarity.io/, last access: 9 March 2024b.
Coalition for Clean Air: https://www.ccair.org/, last access: 17 September 2023.
Coker, E. S., Buralli, R., Manrique, A. F., Kanai, C. M., Amegah, A. K., and Gouveia, N.: Association between PM2.5 and respiratory hospitalization in Rio Branco, Brazil: Demonstrating the potential of low-cost air quality sensor for epidemiologic research, Environ. Res., 214, 113738, https://doi.org/10.1016/j.envres.2022.113738, 2022.
Collier-Oxandale, A., Casey, J. G., Piedrahita, R., Ortega, J., Halliday, H., Johnston, J., and Hannigan, M. P.: Assessing a low-cost methane sensor quantification system for use in complex rural and urban environments, Atmos. Meas. Tech., 11, 3569–3594, https://doi.org/10.5194/amt-11-3569-2018, 2018a.
Collier-Oxandale, A., Coffey, E., Thorson, J., Johnston, J., and Hannigan, M.: Comparing building and neighborhood-scale variability of CO2 and O3 to inform deployment considerations for low-cost sensor system use, Sensors, 18, 1349, https://doi.org/10.3390/s18051349, 2018b.
Collier-Oxandale, A., Feenstra, B., Papapostolou, V., Zhang, H., Kuang, M., Der Boghossian, B., and Polidori, A.: Field and laboratory performance evaluations of 28 gas-phase air quality sensors by the AQ-SPEC program, Atmos. Environ., 220, 117092, https://doi.org/10.1016/j.atmosenv.2019.117092, 2020a.
Collier-Oxandale, A., Wong, N., Navarro, S., Johnston, J., and Hannigan, M.: Using gas-phase air quality sensors to disentangle potential sources in a Los Angeles neighborhood, Atmos. Environ., 233, 117519, https://doi.org/10.1016/j.atmosenv.2020.117519, 2020b.
Connerton, P., Nogueira, T., Kumar, P., and Ribeiro, H.: Use of low-cost sensors for environmental health surveillance: Wildfire-related particulate matter detection in Brasília, Brazil, Atmosphere, 14, 1796, https://doi.org/10.3390/atmos14121796, 2023.
Cross, E. S., Williams, L. R., Lewis, D. K., Magoon, G. R., Onasch, T. B., Kaminsky, M. L., Worsnop, D. R., and Jayne, J. T.: Use of electrochemical sensors for measurement of air pollution: correcting interference response and validating measurements, Atmos. Meas. Tech., 10, 3575–3588, https://doi.org/10.5194/amt-10-3575-2017, 2017.
David, C. M., River, Q. T., Jorge, R. M., Andres, J. K., and Guillermo, G. L.: A low-cost, rapid-deployment and energy-autonomous wireless sensor network for air quality monitoring, in: 2015 9th International Conference on Sensing Technology (ICST), Auckland, New Zealand, 8–10 December 2015, 122–127, https://doi.org/10.1109/ICSensT.2015.7438376, 2015.
deSouza, P., Nthusi, V., Klopp, J., Shaw, B., Ho, W., Saffell, J., Jones, R., and Ratti, C.: A Nairobi experiment in using low cost air quality monitors, Clean Air Journal, 27, https://doi.org/10.17159/2410-972X/2017/v27n2a6, 2017.
Dewage, P. M. H., Wijeratne, L. O. H., Yu, X., Iqbal, M., Balagopal, G., Waczak, J., Fernando, A., Lary, M. D., Ruwali, S., and Lary, D. J.: Providing Fine Temporal and Spatial Resolution Analyses of Airborne Particulate Matter Utilizing Complimentary In Situ IoT Sensor Network and Remote Sensing Approaches, Remote Sens.-Basel, 16, 2454, https://doi.org/10.3390/rs16132454, 2024.
Dirienzo, N., Mitchell, K., Forde, M., Rainham, D., and Villeneuve, P. J.: Temporal trends in ambient fine particulate matter and the impacts of COVID-19 on this pollutant in Grenada, West Indies, J. Air Waste Manage., 73, 97–108, https://doi.org/10.1080/10962247.2022.2126555, 2023.
Donaldson, K., Mills, N., MacNee, W., Robinson, S., and Newby, D.: Role of inflammation in cardiopulmonary health effects of PM, Toxicol. Appl. Pharm., 207, 483–488, https://doi.org/10.1016/j.taap.2005.02.020, 2005.
Drajic, D. D. and Gligoric, N. R.: Reliable low-cost air quality monitoring using off-the-shelf sensors and statistical calibration, Elektron. Elektrotech., 26, 32–41, https://doi.org/10.5755/j01.eie.26.2.25734, 2020.
EGU: Monitoring Networks, Session GI1.3, EGU General Assembly, Vienna, Austria, 23–28 April 2023, https://meetingorganizer.copernicus.org/EGU23/session/45206, last access: 18 September 2023.
ekoNET: https://ekonet.solutions/air-monitoring, last access: 9 March 2024.
Ellona: https://www.ellona.io/, last access: 10 March 2024a.
Ellona: Ellona customer cases: https://www.ellona.io/customer- case/, last access: 10 March 2024b.
Engel-Cox, J., Kim Oanh, N. T., van Donkelaar, A., Martin, R. V., and Zell, E.: Toward the next generation of air quality monitoring: Particulate Matter, Atmos. Environ., 80, 584–590, https://doi.org/10.1016/j.atmosenv.2013.08.016, 2013.
Envirowatch Ltd.: E-MOTE, http://www.envirowatch.ltd.uk/e-mote/, last access: 10 March 2024.
Fahim, M., El Mhouti, A., Boudaa, T., and Jakimi, A.: Modeling and implementation of a low-cost IoT-smart weather monitoring station and air quality assessment based on fuzzy inference model and MQTT protocol, Modeling Earth Systems and Environment, 9, 4085–4102, https://doi.org/10.1007/s40808-023-01701-w, 2023.
Fiedler, N., Laumbach, R., Kelly-McNeil, K., Lioy, P., Fan, Z.-H., Zhang, J., Ottenweller, J., Ohman-Strickland, P., and Kipen, H.: Health effects of a mixture of indoor air volatile organics, their ozone oxidation products, and stress, Environ. Health Persp., 113, 1542–1548, https://doi.org/10.1289/ehp.8132, 2005.
Fuertes, W., Carrera, D., Villacís, C., Toulkeridis, T., Galárraga, F., Torres, E., and Aules, H.: Distributed system as internet of things for a new low-cost, air pollution wireless monitoring on real time, in: 2015 IEEE/ACM 19th International Symposium on Distributed Simulation and Real Time Applications (DS-RT), Chengdu, China, 14–16 October 2015, IEEE, 58–67, https://doi.org/10.1109/DS-RT.2015.28, 2015.
Gagic, R., Skuric, M., Djukanovic, G., and Nikolic, D.: Establishing Correlation between Cruise Ship Activities and Ambient PM Concentrations in the Kotor Bay Area Using a Low-Cost Sensor Network, Atmosphere, 13, 1819, https://doi.org/10.3390/atmos13111819, 2022.
Giordano, M. R., Malings, C., Pandis, S. N., Presto, A. A., McNeill, V. F., Westervelt, D. M., Beekmann, M., and Subramanian, R.: From low-cost sensors to high-quality data: A summary of challenges and best practices for effectively calibrating low-cost particulate matter mass sensors, J. Aerosol Sci., 158, 105833, https://doi.org/10.1016/j.jaerosci.2021.105833, 2021.
Gnamien, S., Yoboué, V., Liousse, C., Ossohou, M., Keita, S., Bahino, J., Siélé, S., and Diaby, L.: Particulate pollution in korhogo and abidjan (Cote d'Ivoire) during the dry season, Aerosol Air Qual. Res., 21, 200201, https://doi.org/10.4209/aaqr.2020.05.0201, 2021.
González Rivero, R. A., Schalm, O., Alvarez Cruz, A., Hernández Rodríguez, E., Morales Pérez, M. C., Alejo Sánchez, D., Martinez Laguardia, A., Jacobs, W., and Hernández Santana, L.: Relevance and reliability of outdoor SO2 monitoring in low-income countries using low-cost sensors, Atmosphere, 14, 912, https://doi.org/10.3390/atmos14060912, 2023.
Gramsch, E., Morales, L., Baeza, M., Ayala, C., Soto, C., Neira, J., Pérez, P., and Moreno, F.: Citizens' surveillance micro-network for the mapping of PM2.5 in the city of Concón, Chile, Aerosol Air Qual. Res., 20, 358–368, https://doi.org/10.4209/aaqr.2019.04.0179, 2020.
Gramsch, E., Oyola, P., Reyes, F., Vásquez, Y., Rubio, M. A., Soto, C., Pérez, P., Moreno, F., and Gutiérrez, N.: Influence of particle composition and size on the accuracy of low cost PM sensors: Findings from field campaigns, Frontiers in Environmental Science, 9, 751267, https://doi.org/10.3389/fenvs.2021.751267, 2021.
Granados-Bolaños, S., Quesada-Román, A., and Alvarado, G. E.: Low-cost applications in dynamic tropical volcanic landforms, J. Volcanol. Geoth. Res., 410, 107143, https://doi.org/10.1016/j.jvolgeores.2020.107143, 2021.
Gressent, A., Malherbe, L., Colette, A., Rollin, H., and Scimia, R.: Data fusion for air quality mapping using low-cost sensor observations: Feasibility and added-value, Environ. Int., 143, 105965, https://doi.org/10.1016/j.envint.2020.105965, 2020.
Gryech, I., Ben-Aboud, Y., Guermah, B., Sbihi, N., Ghogho, M., and Kobbane, A.: Moreair: A low-cost urban air pollution monitoring system, Sensors-Basel, 20, 998, https://doi.org/10.3390/s20040998, 2020.
Guanochanga, B., Cachipuendo, R., Fuertes, W., Benítez, D. S., Toulkeridis, T., Torres, J., Villacís, C., Tapia, F., and Meneses, F.: Towards a real-time air pollution monitoring systems implemented using wireless sensor networks: Preliminary results, in: 2018 IEEE Colombian Conference on Communications and Computing (COLCOM), Medellin, Colombia, 16–18 May 2017, IEEE, 1–4, https://doi.org/10.1109/ColComCon.2018.8466721, 2018.
Guevara, J., Vargas, E., Barrero, F., and Toral, S.: Ubiquitous architecture for environmental sensor networks in road traffic applications, in: 13th International IEEE Conference on Intelligent Transportation Systems, Funchal, Portugal, 19–22 September 2010, IEEE, 1227–1232, https://doi.org/10.1109/ITSC.2010.5625110, 2010.
Hagan, D. H., Gani, S., Bhandari, S., Patel, K., Habib, G., Apte, J. S., Hildebrandt Ruiz, L., and Kroll, J. H.: Inferring aerosol sources from low-cost air quality sensor measurements: A case study in Delhi, India, Environmental Science and Technology Letters, 6, 467–472, https://doi.org/10.1021/acs.estlett.9b00393, 2019.
Han, P., Mei, H., Liu, D., Zeng, N., Tang, X., Wang, Y., and Pan, Y.: Calibrations of low-cost air pollution monitoring sensors for CO, NO2, O3, and SO2, Sensors, 21, 256, https://doi.org/10.3390/s21010256, 2021.
Hemingway, S. E.: 2022 Air Sensors International Conference Program Topics, https://asic.aqrc.ucdavis.edu/2022-program-topics (last access: 8 March 2024), 2022.
Hodoli, C. G. and the Network for Atmospheric and Air Quality Research (NAAQR): Sensors as a component of urban air quality management planning: a case study with AirGradient OpenAir PM monitors from Accra, Ghana., EGU General Assembly 2024, Vienna, Austria, 14–19 Apr 2024, EGU24-20857, https://doi.org/10.5194/egusphere-egu24-20857, 2024.
Hodoli, C. G., Coulon, F., and Mead, M. I.: Source identification with high-temporal resolution data from low-cost sensors using bivariate polar plots in urban areas of Ghana, Environ. Pollut., 317, 120448, https://doi.org/10.1016/j.envpol.2022.120448, 2023.
Hofman, J., Peters, J., Stroobants, C., Elst, E., Baeyens, B., Van Laer, J., Spruyt, M., Van Essche, W., Delbare, E., Roels, B., Cochez, A., Gillijns, E., and Van Poppel, M.: Air quality sensor networks for evidence-based policy making: Best practices for actionable insights, Atmosphere, 13, 944, https://doi.org/10.3390/atmos13060944, 2022.
Hu, J., Wang, F., and Shen, H.: The influence of PM2.5 exposure duration and concentration on outpatient visits of urban hospital in a typical heavy industrial city, Environ. Sci. Pollut. R., 30, 115098–115110, https://doi.org/10.1007/s11356-023-30544-2, 2023.
Ibarrola, E.: Considerations when deploying a sensor-based air quality network, Air Sensors International Conference, https://asic.aqrc.ucdavis.edu/sites/g/files/dgvnsk3466/files/inline-files/Edurne%20Ibarolla%20-%20Considerations%20when%20deploying%20a%20sensor-based%20air%20quality%20network%20.pdf, 2022.
IQAir: First in air quality, https://www.iqair.com/, last access: 17 September 2023.
IQAir: AirVisual Platform, https://www.iqair.com/us/commercial- air-quality-monitors/airvisual-platform, last access: 9 March 2024a.
IQAir: Live animated air quality map, https://www.iqair.com/us/air-quality-map, last access: 9 March 2024b.
Jaafar, W., Xu, J., Farrar, E., Jeong, C., Ganji, A., Evans, G., and Hatzopoulou, M.: Challenges and opportunities of low-cost sensors in capturing the impacts of construction activities on neighborhood air quality, Build. Environ., 254, 111363, https://doi.org/10.1016/j.buildenv.2024.111363, 2024.
Jain, S., Presto, A. A., and Zimmerman, N.: Spatial modeling of daily PM2.5, NO2, and CO concentrations measured by a low-cost sensor network: Comparison of linear, machine learning, and hybrid land use models, Environ. Sci. Technol., 55, 8631–8641, https://doi.org/10.1021/acs.est.1c02653, 2021.
Jang, Y.-W. and Jung, G.-W.: Temporal characteristics and sources of PM2.5 in Porto Velho of Amazon region in Brazil from 2020 to 2022, Sustainability, 15, 14012, https://doi.org/10.3390/su151814012, 2023.
Jayaratne, R., Liu, X., Thai, P., Dunbabin, M., and Morawska, L.: The influence of humidity on the performance of a low-cost air particle mass sensor and the effect of atmospheric fog, Atmos. Meas. Tech., 11, 4883–4890, https://doi.org/10.5194/amt-11-4883-2018, 2018.
Jayaratne, R., Kuhn, T., Christensen, B., Liu, X., Zing, I., Lamont, R., Dunbabin, M., Maddox, J., Fisher, G., and Morawska, L.: Using a network of low-cost particle sensors to assess the impact of ship emissions on a residential community, Aerosol Air Qual. Res., 20, 2754, https://doi.org/10.4209/aaqr.2020.06.0280, 2020.
Jayaratne, R., Thai, P., Christensen, B., Liu, X., Zing, I., Lamont, R., Dunbabin, M., Dawkins, L., Bertrand, L., and Morawska, L.: The effect of cold-start emissions on the diurnal variation of carbon monoxide concentration in a city centre, Atmos. Environ., 245, 118035, https://doi.org/10.1016/j.atmosenv.2020.118035, 2021.
Jeong, J. I. and Park, R. J.: Effects of the meteorological variability on regional air quality in East Asia, Atmos. Environ., 69, 46–55, https://doi.org/10.1016/j.atmosenv.2012.11.061, 2013.
Jiao, W., Hagler, G. S. W., Williams, R. W., Sharpe, R. N., Weinstock, L., and Rice, J.: Field assessment of the village green project: An autonomous community air quality monitoring system, Environ. Sci. Technol., 49, 6085–6092, https://doi.org/10.1021/acs.est.5b01245, 2015.
Jiao, W., Hagler, G., Williams, R., Sharpe, R., Brown, R., Garver, D., Judge, R., Caudill, M., Rickard, J., Davis, M., Weinstock, L., Zimmer-Dauphinee, S., and Buckley, K.: Community Air Sensor Network (CAIRSENSE) project: evaluation of low-cost sensor performance in a suburban environment in the southeastern United States, Atmos. Meas. Tech., 9, 5281–5292, https://doi.org/10.5194/amt-9-5281-2016, 2016.
Jing, P., Schusler, T., Dahal, D., Zhang, B., Fischer, E., Pollack, I. B., and Sablan, O. M.: Community air research experience (Care): Engaging undergraduate students underrepresented in stem fields in research on air pollution in chicago communities, in: AGU Fall Meeting, Chicago, IL, 12–16 December 2022, ED42C-0608, https://ui.adsabs.harvard.edu/abs/2022AGUFMED42C0608J (last access: 8 March 2024), 2022.
Johnston, J. E., Enebish, T., Eckel, S. P., Navarro, S., and Shamasunder, B.: Respiratory health, pulmonary function and local engagement in urban communities near oil development, Environ. Res., 197, 111088, https://doi.org/10.1016/j.envres.2021.111088, 2021.
Jovašević-Stojanović, M., Bartonova, A., Topalović, D., Lazović, I., Pokrić, B., and Ristovski, Z.: On the use of small and cheaper sensors and devices for indicative citizen-based monitoring of respirable particulate matter, Environ. Pollut., 206, 696–704, https://doi.org/10.1016/j.envpol.2015.08.035, 2015.
Kampa, M. and Castanas, E.: Human health effects of air pollution, Environ. Pollut., 151, 362–367, https://doi.org/10.1016/j.envpol.2007.06.012, 2008.
Karagulian, F., Barbiere, M., Kotsev, A., Spinelle, L., Gerboles, M., Lagler, F., Redon, N., Crunaire, S., and Borowiak, A.: Review of the performance of low-cost sensors for air quality monitoring, Atmosphere, 10, 506, https://doi.org/10.3390/atmos10090506, 2019.
Karaoghlanian, N., Noureddine, B., Saliba, N., Shihadeh, A., and Lakkis, I.: Low cost air quality sensors “PurpleAir” calibration and inter-calibration dataset in the context of Beirut, Lebanon, Data in Brief, 41, 108008, https://doi.org/10.1016/j.dib.2022.108008, 2022.
Kelly, K. E., Whitaker, J., Petty, A., Widmer, C., Dybwad, A., Sleeth, D., Martin, R., and Butterfield, A.: Ambient and laboratory evaluation of a low-cost particulate matter sensor, Environ. Pollut., 221, 491–500, https://doi.org/10.1016/j.envpol.2016.12.039, 2017.
Khader, A. and Martin, R. S.: Use of low-cost ambient particulate sensors in Nablus, Palestine with application to the assessment of regional dust storms, Atmosphere, 10, 539, https://doi.org/10.3390/atmos10090539, 2019.
Khan, T. R. and Meranger, J. C.: Recent advances in SO2, NOx, and O3 personal monitoring, Environ. Int., 9, 195–206, https://doi.org/10.1016/0160-4120(83)90037-5, 1983.
Khuriganova, O. I., Obolkin, V. A., Golobokova, L. P., Bukin, Y. S., and Khodzher, T. V.: Passive sampling as a low-cost method for monitoring air pollutants in the Baikal region (Eastern Siberia), Atmosphere, 10, 470, https://doi.org/10.3390/atmos10080470, 2019.
Kim, J., Shusterman, A. A., Lieschke, K. J., Newman, C., and Cohen, R. C.: The BErkeley Atmospheric CO2 Observation Network: field calibration and evaluation of low-cost air quality sensors, Atmos. Meas. Tech., 11, 1937–1946, https://doi.org/10.5194/amt-11-1937-2018, 2018.
Korotchenkov, G. S., Dmitriev, S. V., and Brynzari, V. I.: Processes development for low cost and low power consuming SnO2 thin film gas sensors (Tfgs), Sensor. Actuat. B-Chem., 54, 202–209, https://doi.org/10.1016/S0925-4005(99)00017-9, 1999.
Kotzagianni, M., Hassani, A., Morresi, N., Udina, S., Kyfonidis, C., Roussos, A., Casaccia, S., Revel, G. M., and Noriega-Ortega, B.: Calibration strategies for low-cost compact field sensors in Citizen Science Air Quality measurements: Insights from SOCIO-BEE project, in: 2023 8th International Conference on Smart and Sustainable Technologies (SpliTech), Split/Bol, Croatia, 20–23 June 2023, IEEE, 1–6, https://doi.org/10.23919/SpliTech58164.2023.10193423, 2023.
Kuhn, T., Jayaratne, R., Thai, P. K., Christensen, B., Liu, X., Dunbabin, M., Lamont, R., Zing, I., Wainwright, D., Witte, C., Neale, D., and Morawska, L.: Air quality during and after the Commonwealth Games 2018 in Australia: Multiple benefits of monitoring, J. Aerosol Sci., 152, 105707, https://doi.org/10.1016/j.jaerosci.2020.105707, 2021.
Kumar, T. and Doss, A.: Airo: Development of an intelligent IOT-based air quality monitoring solution for urban areas, Procedia Comput. Sci., 218, 262–273, https://doi.org/10.1016/j.procs.2023.01.008, 2023.
Kunak AIR Cloud: Air quality software for data analysis, http://kunakair.com/air-quality-software/, last access: 10 March 2024.
Kurtenbach, R., Ulianova, K., Gibilisco, R. G., Villena, G., and Wiesen, P.: Validation of low cost sensors for gases and particulate matter in the city centre of Wuppertal, Germany, in: Proceedings of the 24th International Transport and Air Pollution (TAP) Conference, https://data.europa.eu/doi/10.2760/019404, 2022.
Labzovskii, L. D., Vande Hey, J., Romanov, A. A., Golovatina-Mora, P., Belikov, D. A., Lashkari, A., Takele Kenea, S., and Hekman, E.: Who should measure air quality in modern cities? The example of decentralization of urban air quality monitoring in Krasnoyarsk (Siberia, Russia), Environ. Sci. Policy, 140, 93–103, https://doi.org/10.1016/j.envsci.2022.11.016, 2023.
Lassman, W., Pierce, J. R., Bangs, E. J., Sullivan, A. P., Ford, B., Mengistu Tsidu, G., Sherman, J. P., Collett, J. L., and Bililign, S.: Using low-cost measurement systems to investigate air quality: A case study in Palapye, Botswana, Atmosphere, 11, 583, https://doi.org/10.3390/atmos11060583, 2020.
Lee, Y.-M., Lin, G.-Y., Le, T.-C., Hong, G.-H., Aggarwal, S. G., Yu, J.-Y., and Tsai, C.-J.: Characterization of spatial-temporal distribution and microenvironment source contribution of PM2.5 concentrations using a low-cost sensor network with artificial neural network/kriging techniques, Environ. Res., 244, 117906, https://doi.org/10.1016/j.envres.2023.117906, 2024.
Li, H. Z., Gu, P., Ye, Q., Zimmerman, N., Robinson, E. S., Subramanian, R., Apte, J. S., Robinson, A. L., and Presto, A. A.: Spatially dense air pollutant sampling: Implications of spatial variability on the representativeness of stationary air pollutant monitors, Atmospheric Environment: X, 2, 100012, https://doi.org/10.1016/j.aeaoa.2019.100012, 2019.
Li, J., Zhang, H., Chao, C.-Y., Chien, C.-H., Wu, C.-Y., Luo, C. H., Chen, L.-J., and Biswas, P.: Integrating low-cost air quality sensor networks with fixed and satellite monitoring systems to study ground-level PM2.5, Atmos. Environ., 223, 117293, https://doi.org/10.1016/j.atmosenv.2020.117293, 2020.
Liang, L., Daniels, J., Bailey, C., Hu, L., Phillips, R., and South, J.: Integrating low-cost sensor monitoring, satellite mapping, and geospatial artificial intelligence for intra-urban air pollution predictions, Environ. Pollut., 331, 121832, https://doi.org/10.1016/j.envpol.2023.121832, 2023.
Lin, C., Labzovskii, L. D., Leung Mak, H. W., Fung, J. C. H., Lau, A. K. H., Kenea, S. T., Bilal, M., Vande Hey, J. D., Lu, X., and Ma, J.: Observation of PM2.5 using a combination of satellite remote sensing and low-cost sensor network in Siberian urban areas with limited reference monitoring, Atmos. Environ., 227, 117410, https://doi.org/10.1016/j.atmosenv.2020.117410, 2020.
Liu, B. and Zimmerman, N.: Fleet-based vehicle emission factors using low-cost sensors: Case study in parking garages, Transportation Res. D-Tr. E., 91, 102635, https://doi.org/10.1016/j.trd.2020.102635, 2021.
Liu, J., Banerjee, S., Oroumiyeh, F., Shen, J., del Rosario, I., Lipsitt, J., Paulson, S., Ritz, B., Su, J., Weichenthal, S., Lakey, P., Shiraiwa, M., Zhu, Y., and Jerrett, M.: Co-kriging with a low-cost sensor network to estimate spatial variation of brake and tire-wear metals and oxidative stress potential in Southern California, Environ. Int., 168, 107481, https://doi.org/10.1016/j.envint.2022.107481, 2022.
Liu, M., Barkjohn, K., Norris, C., Schauer, J., Zhang, J., Zhang, Y., Hu, M., and Bergin, M.: Using low-cost sensors to monitor indoor, outdoor, and personal ozone concentrations in Beijing, China, Environ. Sci.-Proc. Imp., 22, 131–143, https://doi.org/10.1039/C9EM00377K, 2020.
Liu, N., Bu, Z., Liu, W., Kan, H., Zhao, Z., Deng, F., Huang, C., Zhao, B., Zeng, X., Sun, Y., Qian, H., Mo, J., Sun, C., Guo, J., Zheng, X., Weschler, L. B., and Zhang, Y.: Health effects of exposure to indoor volatile organic compounds from 1980 to 2017: A systematic review and meta-analysis, Indoor Air, 32, e13038, https://doi.org/10.1111/ina.13038, 2022.
Liu, S., Yang, X., and Zhou, X.: Development of a low-cost UAV-based system for CH4 monitoring over oil fields, Environ. Technol., 42, 3154–3163, https://doi.org/10.1080/09593330.2020.1724199, 2020.
Liu, X., Jayaratne, R., Thai, P., Kuhn, T., Zing, I., Christensen, B., Lamont, R., Dunbabin, M., Zhu, S., Gao, J., Wainwright, D., Neale, D., Kan, R., Kirkwood, J., and Morawska, L.: Low-cost sensors as an alternative for long-term air quality monitoring, Environ. Res., 185, 109438, https://doi.org/10.1016/j.envres.2020.109438, 2020.
Local Haze [Mobile App]: https://localhaze.humanlogic.com/, last access: 17 September 2023.
Lopez-Restrepo, S., Yarce, A., Pinel, N., Quintero, O. L., Segers, A., and Heemink, A. W.: Urban air quality modeling using low-cost sensor network and data assimilation in the Aburrá Valley, Colombia, Atmosphere, 12, 91, https://doi.org/10.3390/atmos12010091, 2021.
Love my air: https://denver.lovemyair.com, last access: 10 March 2024.
Lu, T., Liu, Y., Garcia, A., Wang, M., Li, Y., Bravo-Villasenor, G., Campos, K., Xu, J., and Han, B.: Leveraging citizen science and low-cost sensors to characterize air pollution exposure of disadvantaged communities in southern california, Int. J. Env. Res. Pub. He., 19, 8777, https://doi.org/10.3390/ijerph19148777, 2022.
Ma, Y., Richards, M., Ghanem, M., Guo, Y., and Hassard, J.: Air pollution monitoring and mining based on sensor grid in London, Sensors, 8, 3601–3623, https://doi.org/10.3390/s80603601, 2008.
Mahfuz, M. U. and Ahmed, K.: A review of micro-nano-scale wireless sensor networks for environmental protection: Prospects and challenges, Sci. Technol. Adv. Mat., 6, 302–306, https://doi.org/10.1016/j.stam.2005.02.008, 2005.
Malings, C., Tanzer, R., Hauryliuk, A., Kumar, S. P. N., Zimmerman, N., Kara, L. B., Presto, A. A., and R. Subramanian: Development of a general calibration model and long-term performance evaluation of low-cost sensors for air pollutant gas monitoring, Atmos. Meas. Tech., 12, 903–920, https://doi.org/10.5194/amt-12-903-2019, 2019a.
Malings, C., Tanzer, R., Hauryliuk, A., Saha, P. K., Robinson, A. L., Presto, A. A., and Subramanian, R.: Fine particle mass monitoring with low-cost sensors: Corrections and long-term performance evaluation, Aerosol Sci. Technol., 54, 160–174, https://doi.org/10.1080/02786826.2019.1623863, 2019b.
Malings, C., Knowland, K. E., Keller, C. A., and Cohn, S. E.: Sub-city scale hourly air quality forecasting by combining models, satellite observations, and ground measurements, Earth and Space Science, 8, e2021EA001743, https://doi.org/10.1029/2021EA001743, 2021.
Martinez, A., Hernandez-Rodríguez, E., Hernandez, L., Schalm, O., González-Rivero, R. A., and Alejo-Sánchez, D.: Design of a low-cost system for the measurement of variables associated with air quality, IEEE Embedded Systems Letters, 15, 105–108, https://doi.org/10.1109/LES.2022.3196543, 2023.
Mead, M. I., Popoola, O. A. M., Stewart, G. B., Landshoff, P., Calleja, M., Hayes, M., Baldovi, J. J., McLeod, M. W., Hodgson, T. F., Dicks, J., Lewis, A., Cohen, J., Baron, R., Saffell, J. R., and Jones, R. L.: The use of electrochemical sensors for monitoring urban air quality in low-cost, high-density networks, Atmos. Environ., 70, 186–203, https://doi.org/10.1016/j.atmosenv.2012.11.060, 2013.
Meixner, H. and Lampe, U.: Metal oxide sensors, Sensor. Actuat. B-Chem., 33, 198–202, https://doi.org/10.1016/0925-4005(96)80098-0, 1996.
Meixner, H., Gerblinger, J., Lampe, U., and Fleischer, M.: Thin-film gas sensors based on semiconducting metal oxides, Sensor. Actuat. B-Chem., 23, 119–125, https://doi.org/10.1016/0925-4005(94)01266-K, 1995.
Metreveli, Y. Y.: The Internet of things and indoor air quality on ship, IOP Conf. Ser.: Earth Environ. Sci., 872, 012015, https://doi.org/10.1088/1755-1315/872/1/012015, 2021.
Miranda, M. L., Edwards, S. E., Keating, M. H., and Paul, C. J.: Making the environmental justice grade: The relative burden of air pollution exposure in the united states, Int. J. Env. Res. Pub. He., 8, 1755–1771, https://doi.org/10.3390/ijerph8061755, 2011.
Miskell, G., Salmond, J., and Williams, D. E.: Low-cost sensors and crowd-sourced data: Observations of siting impacts on a network of air-quality instruments, Sci. Total Environ., 575, 1119–1129, https://doi.org/10.1016/j.scitotenv.2016.09.177, 2017.
MKE FreshAir Collective: https://www.mkefreshair.com/, last access: 10 March 2024.
Mo, X., Peters, D., and Lei, C.: Low Cost Autonomous UAV Swarm Application in Wildfire Surveillance and Suppression, Proceedings of the 2021 6th International Conference on Machine Learning Technologies, Jeju Island, Republic of Korea, 23–25 April 2021, Association for Computing Machinery, 164–169, https://doi.org/10.1145/3468891.3468916, 2021.
Moltchanov, S., Levy, I., Etzion, Y., Lerner, U., Broday, D. M., and Fishbain, B.: On the feasibility of measuring urban air pollution by wireless distributed sensor networks, Sci. Total Environ., 502, 537–547, https://doi.org/10.1016/j.scitotenv.2014.09.059, 2015.
Morawska, L., Thai, P. K., Liu, X., Asumadu-Sakyi, A., Ayoko, G., Bartonova, A., Bedini, A., Chai, F., Christensen, B., Dunbabin, M., Gao, J., Hagler, G. S. W., Jayaratne, R., Kumar, P., Lau, A. K. H., Louie, P. K. K., Mazaheri, M., Ning, Z., Motta, N., Mullins, B., Rahman, M. M., Ristovski, Z., Shafiei, M., Tjondronegoro, D., Westerdahl, D., and Williams, R.: Applications of low-cost sensing technologies for air quality monitoring and exposure assessment: How far have they gone?, Environ. Int., 116, 286–299, https://doi.org/10.1016/j.envint.2018.04.018, 2018.
Morel, P.: Gramm: Grammar of graphics plotting in Matlab, Journal of Open Source Software, 3, 568, https://doi.org/10.21105/joss.00568, 2018.
Morgan, M. G. and Morris, S. C.: Individual air pollution monitors: An assessment of national research needs, Brookhaven National Laboratory, BNL-50482, https://doi.org/10.2172/7293144, 1976.
Mullen, C. J., Grineski, S. E., Collins, T. W., and Flores, A. B.: Air quality sensors and distributional environmental justice: A case study of Salt Lake County, Utah, Environmental Sociology, 10, 179–191, https://doi.org/10.1080/23251042.2023.2295099, 2023.
Müller, M., Graf, P., Meyer, J., Pentina, A., Brunner, D., Perez-Cruz, F., Hüglin, C., and Emmenegger, L.: Integration and calibration of non-dispersive infrared (NDIR) CO2 low-cost sensors and their operation in a sensor network covering Switzerland, Atmos. Meas. Tech., 13, 3815–3834, https://doi.org/10.5194/amt-13-3815-2020, 2020.
Munir, S., Mayfield, M., Coca, D., Jubb, S. A., and Osammor, O.: Analysing the performance of low-cost air quality sensors, their drivers, relative benefits and calibration in cities – A case study in Sheffield, Environ. Monit. Assess., 191, 94, https://doi.org/10.1007/s10661-019-7231-8, 2019.
Narayana, M. V., Jalihal, D., and Nagendra, S. M. S.: Establishing a sustainable low-cost air quality monitoring setup: A survey of the state-of-the-art, Sensors, 22, 394, https://doi.org/10.3390/s22010394, 2022.
NASA: Ames INSTEP, https://www.nasa.gov/inexpensive- network-sensor-technology-exploring-pollution-instep/, last access: 10 March 2024.
NASA HAQAST (NASA Health and Air Quality Applied Sciences Team): Past Meetings, https://haqast.org/get-involved/meetings/, last access: 17 September 2023.
National Research Council (US) Committee on Indoor Pollutants: VI Monitoring and modeling of indoor air pollution, in: Indoor Pollutants, National Academies Press, US, https://www.ncbi.nlm.nih.gov/books/NBK234059/ (last access: 8 March 2024), 1981.
National Association of Clean Air Agencies: https://www.4cleanair.org/, last access: 17 September 2023.
Ndamuzi, E., Akimana, R., Gahungu, P., and Bimenyimana, E.: Modelling and characterization of fine Particulate Matter dynamics in Bujumbura using low cost sensors, arXiv [preprint], https://doi.org/10.48550/arXiv.2312.12003, 19 December 2023.
Ngo, N. S., Asseko, S. V. J., Ebanega, M. O., Allo'o Allo'o, S. M., and Hystad, P.: The relationship among PM2.5, traffic emissions, and socioeconomic status: Evidence from Gabon using low-cost, portable air quality monitors, Transport. Res. D-Tr. E., 68, 2–9, https://doi.org/10.1016/j.trd.2018.01.029, 2019.
Ngom, B., Seye, M. R., Diallo, M., Gueye, B., and Drame, M. S.: A hybrid measurement kit for real-time air quality monitoring across senegal cities. 2018 1st International Conference on Smart Cities and Communities (SCCIC), Ouagadougou, Burkina Faso, 24–26 July 2018, IEEE, 1–6, https://doi.org/10.1109/SCCIC.2018.8584551, 2018.
Nori-Sarma, A., Thimmulappa, R. K., Venkataramana, G. V., Fauzie, A. K., Dey, S. K., Venkareddy, L. K., Berman, J. D., Lane, K. J., Fong, K. C., Warren, J. L., and Bell, M. L.: Low-cost NO2 monitoring and predictions of urban exposure using universal kriging and land-use regression modelling in Mysore, India, Atmos. Environ., 226, 117395, https://doi.org/10.1016/j.atmosenv.2020.117395, 2020.
Oizom: https://oizom.com/, last access: 10 March 2024.
Okorn, K. and Hannigan, M.: Applications and limitations of quantifying speciated and source-apportioned vocs with metal oxide sensors, Atmosphere, 12, 1383, https://doi.org/10.3390/atmos12111383, 2021.
Okorn, K., Jimenez, A., Collier-Oxandale, A., Johnston, J., and Hannigan, M.: Characterizing methane and total non-methane hydrocarbon levels in Los Angeles communities with oil and gas facilities using air quality monitors, Sci. Total Environ., 777, 146194, https://doi.org/10.1016/j.scitotenv.2021.146194, 2021.
Omokungbe, O. R., Fawole, O. G., Owoade, O. K., Popoola, O. A. M., Jones, R. L., Olise, F. S., Ayoola, M. A., Abiodun, P. O., Toyeje, A. B., Olufemi, A. P., Sunmonu, L. A., and Abiye, O. E.: Analysis of the variability of airborne particulate matter with prevailing meteorological conditions across a semi-urban environment using a network of low-cost air quality sensors, Heliyon, 6, e04207, https://doi.org/10.1016/j.heliyon.2020.e04207, 2020.
Omokungbe, O. R., Olufemi, A. P., Toyeje, A. B., and Abiodun, P. O.: Investigating the evolution of gaseous air pollutants with prevailing meteorology across selected sites within a pollution hotspot in Ile-Ife, Southwestern Nigeria, Discover Environment, 1, 5, https://doi.org/10.1007/s44274-023-00006-0, 2023.
OpenAQ: https://openaq.org/, last access: 10 March 2024.
Ott, W. R.: Concepts of human exposure to air pollution, Environ. Int., 7, 179–196, https://doi.org/10.1016/0160-4120(82)90104-0, 1982.
Outpost Environmental: https://outpostenvironmental.com/, last access: 10 March 2024.
Ouyang, B., Popoola, L., Jones, R., Li, C., and Chen, J.: Portable and low-cost sensors in monitoring air qualities in China, EGU General Assembly, Vienna, Austria, 17–22 April 2016, EGU2016-16213, 2016.
Owoade, O. K., Abiodun, P. O., Omokungbe, O. R., Fawole, O. G., Olise, F. S., Popoola, O. O. M., Jones, R. L., and Hopke, P. K.: Spatial-temporal variation and local source identification of air pollutants in a semi-urban settlement in Nigeria using low-cost sensors, Aerosol Air Qual. Res., 21, 200598, https://doi.org/10.4209/aaqr.200598, 2021.
Oyola, P., Carbone, S., Timonen, H., Torkmahalleh, M., and Lindén, J.: Editorial: Rise of low-cost sensors and citizen science in air quality studies, Frontiers in Environmental Science, 10, 868543, https://doi.org/10.3389/fenvs.2022.868543, 2022.
Ozler, S.: Field applications of low-cost air quality monitors for PM2.5 studies, MS thesis, https://minds.wisconsin.edu/handle/1793/78604 (last access: 8 March 2024), 2018.
Paku for PurpleAir: https://paku.app, last access: 9 March 2024.
Panzitta, A., Bax, C., Lotesoriere, B. J., Ratti, C., and Capelli, L.: Realisation of a multi-sensor system for real-tim emonitoring of odour emissions at a waste treatment plant, Chem. Engineer. Trans., 95, 139–144, https://doi.org/10.3303/CET2295024, 2022.
Papaconstantinou, R., Demosthenous, M., Bezantakos, S., Hadjigeorgiou, N., Costi, M., Stylianou, M., Symeou, E., Savvides, C., and Biskos, G.: Field evaluation of low-cost electrochemical air quality gas sensors under extreme temperature and relative humidity conditions, Atmos. Meas. Tech., 16, 3313–3329, https://doi.org/10.5194/amt-16-3313-2023, 2023.
Papadopoulos, C. A., Vlachos, D. S., and Avaritsiotis, J. N.: Comparative study of various metal-oxide-based gas-sensor architectures, Sensor. Actuat. B-Chem., 32, 61–69, https://doi.org/10.1016/0925-4005(96)80110-9, 1996.
Park, C., Jeong, S., Park, H., Woo, J.-H., Sim, S., Kim, J., Son, J., Park, H., Shin, Y., Shin, J., Kwon, S.-M., and Lee, W.: Challenges in monitoring atmospheric CO2 concentrations in Seoul using low-cost sensors, Asia-Pac. J. Atmos. Sci., 57, 547–553, https://doi.org/10.1007/s13143-020-00213-2, 2021.
Patwardhan, I., Sara, S., and Chaudhari, S.: Comparative evaluation of new low-cost particulate matter sensors, in: 2021 8th International Conference on Future Internet of Things and Cloud (FiCloud), Rome, Italy, 23–25 August 2021, 192–197, https://doi.org/10.1109/FiCloud49777.2021.00035, 2021.
Penza, M., Suriano, D., Villani, M. G., Spinelle, L., and Gerboles, M.: Towards air quality indices in smart cities by calibrated low-cost sensors applied to networks, 2014 IEEE SENSORS, Valencia, Spain, 2–5 November 2014, IEEE, 2012–2017, https://doi.org/10.1109/ICSENS.2014.6985429, 2014.
Penza, M., Suriano, D., Cassano, G., Pfister, V., Amodio, M., Trizio, L., Brattoli, M., and De Gennaro, G.: A case-study of microsensors for landfill air-pollution monitoring applications, Urban Climate, 14, 351–369, https://doi.org/10.1016/j.uclim.2014.09.002, 2015.
Penza, M., Suriano, D., Pfister, V., Prato, M., and Cassano, G.: Urban air quality monitoring with networked low-cost sensor-systems, Proceedings, 1, 573, https://doi.org/10.3390/proceedings1040573, 2017.
Pereira-Rodrigues, N., Guillot, J. M., Fanlo, J. L., Renner, C., and Aubert, B.: Miniature and low-cost devices for the precise and reliable monitoring of low concentrations of H2s in changing environments, Chem. Engineer. Trans., 23, 237–242, https://doi.org/10.3303/CET1023040, 2010.
Perello, J.: Bettair, https://www.miteco.gob.es/content/dam/miteco/es/calidad-y-evaluacion-ambiental/formacion/ateknea_bettair_jornada_sensores_20180605_tcm30-457716.pdf (last access: 8 March 2024), 2018.
Piedrahita, R., Xiang, Y., Masson, N., Ortega, J., Collier, A., Jiang, Y., Li, K., Dick, R. P., Lv, Q., Hannigan, M., and Shang, L.: The next generation of low-cost personal air quality sensors for quantitative exposure monitoring, Atmos. Meas. Tech., 7, 3325–3336, https://doi.org/10.5194/amt-7-3325-2014, 2014.
Pochwala, S., Gardecki, A., Lewandowski, P., Somogyi, V., Anweiler, S.: Developing of Low-Cost Air Pollution Sensor – Measurements with the Unmanned Aerial Vehicles in Poland, Sensors, 20, 3582, https://doi.org/10.3390/s20123582, 2020.
Pope, C. A. and Dockery, D. W.: Health effects of fine particulate air pollution: Lines that connect, J. Air Waste Manage., 56, 709–742, https://doi.org/10.1080/10473289.2006.10464485, 2006.
Pope, F. D., Gatari, M., Ng'ang'a, D., Poynter, A., and Blake, R.: Airborne particulate matter monitoring in Kenya using calibrated low-cost sensors, Atmos. Chem. Phys., 18, 15403–15418, https://doi.org/10.5194/acp-18-15403-2018, 2018.
Popoola, O. A. M., Carruthers, D., Lad, C., Bright, V. B., Mead, M. I., Stettler, M. E. J., Saffell, J. R., and Jones, R. L.: Use of networks of low cost air quality sensors to quantify air quality in urban settings, Atmos. Environ., 194, 58–70, https://doi.org/10.1016/j.atmosenv.2018.09.030, 2018.
Postolache, O. A., Pereira, J. M. D., and Girao, P. M. B. S.: Smart sensors network for air quality monitoring applications, IEEE T. Instrum. Meas., 58, 3253–3262, https://doi.org/10.1109/TIM.2009.2022372, 2009.
Prana Air: https://pranaair.com, last access: 9 March 2024.
Prathibha, P. S., Cross, E. S., Strehl, R. L., Yeager II, R. A., Bhatnagar, A., and Turner, J. R.: Green heart louisville: Community-level assessment of exposure to air pollution, AGU, A206-02, https://ui.adsabs.harvard.edu/abs/2020AGUFMA206...02P (last access: 8 March 2024), 2020.
Puget Sound Clean Air Agency: Air quality sensors: https://www.pscleanair.gov/539/Air-Quality-Sensors#:~:text=One%20of%20the%20most%20popular,regulatory%20monitors%20and%20comparing%20measurements, last access: 17 September 2023.
PurpleAir: New API dashboard and data download tool release, https://www2.purpleair.com/blogs/blog-home/purpleair-s-new-api-dashboard-data-download-tool-release, last access: 11 November 2023.
PurpleAir: Real-time air quality map, https://map.purpleair.com/, last access: 9 March 2024.
PurpleAir Community: Is there an Introductory guide on how to utilize the API to analyze historical data?, https://community.purpleair.com/t/is-there-an-introductory- guide-on-how-to-utilise-the-api-to-analyse-historical-data/3148/3, last access: 10 March 2024.
QuantAQ: https://quant-aq.com/, last access: 10 March 2024.
Queensland University of Technology: Koala sensors, https://research.qut.edu.au/ilaqh/projects/koala-sensors/, last access: 10 March 2024.
Quevedo, D., Gao, Z., Do, K., Bahreini, R., Collins, D., and Ivey, C.: Multidecadal analysis of meteorological and emissions regimes for PM2.5 across california, ACS ESandT Air, 1, 33–42, https://doi.org/10.1021/acsestair.3c00019, 2024.
Quintero, E., González, J., García, F., Sáez, Y., and Collado, E.: IoT-based system prototype for particulate matter monitoring in the city of Chitre, Panama, https://laccei.org/LACCEI2023-BuenosAires/meta/FP847.html (last access: 8 March 2024), 2023.
Raheja, G., Harper, L., Hoffman, A., Gorby, Y., Freese, L., O'Leary, B., Deron, N., Smith, S., Auch, T., Goodwin, M., and Westervelt, D. M.: Community-based participatory research for low-cost air pollution monitoring in the wake of unconventional oil and gas development in the Ohio River Valley: Empowering impacted residents through community science, Environ. Res. Lett., 17, 065006, https://doi.org/10.1088/1748-9326/ac6ad6, 2022a.
Raheja, G., Sabi, K., Sonla, H., Gbedjangni, E. K., McFarlane, C. M., Hodoli, C. G., and Westervelt, D. M.: A network of field-calibrated low-cost sensor measurements of PM2.5 in Lomé, Togo, over one to two years, ACS Earth Space Chem., 6, 1011–1021, https://doi.org/10.1021/acsearthspacechem.1c00391, 2022b.
Rai, A. C., Kumar, P., Pilla, F., Skouloudis, A. N., Di Sabatino, S., Ratti, C., Yasar, A., and Rickerby, D.: End-user perspective of low-cost sensors for outdoor air pollution monitoring, Sci. Total Environ., 607–608, 691–705, https://doi.org/10.1016/j.scitotenv.2017.06.266, 2017.
Ramakrishna, J., Durgaprasad, M. B., and Smith, K. R.: Cooking in India: The impact of improved stoves on indoor air quality, Environ. Int., 15, 341–352, https://doi.org/10.1016/0160-4120(89)90047-0, 1989.
Real-Time Air Quality Sensor Networks: The world air quality index project, https://aqicn.org/network/, last access: 10 March 2024.
Ren, Y., Qu, Z., Du, Y., Xu, R., Ma, D., Yang, G., Shi, Y., Fan, X., Tani, A., Guo, P., Ge, Y., and Chang, J.: Air quality and health effects of biogenic volatile organic compounds emissions from urban green spaces and the mitigation strategies, Environ. Pollut., 230, 849–861, https://doi.org/10.1016/j.envpol.2017.06.049, 2017.
Ribbit Network: https://www.ribbitnetwork.org/, last access: 10 March 2024.
RIVM data portal: https://samenmeten.rivm.nl/dataportaal/, last access: 10 March 2024.
Rodríguez, E. H., Schalm, O., and Martínez, A.: Development of a low-cost measuring system for the monitoring of environmental parameters that affect air quality for human health, ITEGAM-JETIA, 6, 22–27, https://itegam-jetia.org/journal/index.php/jetia/article/view/648 (last access: 8 March 2024), 2020.
Rosmarin, A., Curtis, L., and Brown, D. R.: Weather-based evaluation of exposure to airborne toxins to nearby residents, Environmental Advances, 13, 100415, https://doi.org/10.1016/j.envadv.2023.100415, 2023.
Sablan, O. M., Gargulinski, E., Slater, K., Rosen, Z., Henery, G., Ford, B., Magzamen, S., Soja, A. J., Pierce, J. R., and Fischer, E.: Monitoring smoke from landscape fires in the flint hills region of Kansas during the 2022 burning season, in: AGU Fall Meeting, Chicago, IL, 12–16 December 2022, GH42A-33, https://ui.adsabs.harvard.edu/abs/2022AGUFMGH42A..33S (last access: 8 March 2024), 2022.
Sahu, R., Dixit, K. K., Mishra, S., Kumar, P., Shukla, A. K., Sutaria, R., Tiwari, S., and Tripathi, S. N.: Validation of low-cost sensors in measuring real-time PM10 concentrations at two sites in Delhi national capital region, Sensors, 20, 1347, https://doi.org/10.3390/s20051347, 2020.
Sahu, R., Nagal, A., Dixit, K. K., Unnibhavi, H., Mantravadi, S., Nair, S., Simmhan, Y., Mishra, B., Zele, R., Sutaria, R., Motghare, V. M., Kar, P., and Tripathi, S. N.: Robust statistical calibration and characterization of portable low-cost air quality monitoring sensors to quantify real-time O3 and NO2 concentrations in diverse environments, Atmos. Meas. Tech., 14, 37–52, https://doi.org/10.5194/amt-14-37-2021, 2021.
Saleh, T. and Khader, A.: Urban particulate matter hazard mapping and monitoring site selection in Nablus, palestine, Atmosphere, 13, 1134, https://doi.org/10.3390/atmos13071134, 2022.
Saúco, L., Pradas, P., Ibarrola, E., Martín, J., and Lardín, C.: Early alert system for odour management in WWTP, in: 2022 IEEE International Symposium on Olfaction and Electronic Nose (ISOEN), Aveiro, Portugal, 29 May–1 June 2022, IEEE, 1–2, https://doi.org/10.1109/ISOEN54820.2022.9789637, 2022.
Sayahi, T., Kaufman, D., Becnel, T., Kaur, K., Butterfield, A. E., Collingwood, S., Zhang, Y., Gaillardon, P.-E., and Kelly, K. E.: Development of a calibration chamber to evaluate the performance of low-cost particulate matter sensors, Environ. Pollut., 255, 113131, https://doi.org/10.1016/j.envpol.2019.113131, 2019.
Sayahi, T., Garff, A., Quah, T., Lê, K., Becnel, T., Powell, K. M., Gaillardon, P.-E., Butterfield, A. E., and Kelly, K. E.: Long-term calibration models to estimate ozone concentrations with a metal oxide sensor, Environ. Pollut., 267, 115363, https://doi.org/10.1016/j.envpol.2020.115363, 2020.
Sberveglieri, G., Hellmich, W., and Müller, G.: Silicon hotplates for metal oxide gas sensor elements, Microsyst. Technol., 3, 183–190, https://doi.org/10.1007/s005420050078, 1997.
Schalm, O., Carro, G., Lazarov, B., Jacobs, W., and Stranger, M.: Reliability of Lower-Cost Sensors in the Analysis of Indoor Air Quality on Board Ships, Atmosphere, 13, 1579, https://doi.org/10.3390/atmos13101579, 2022.
Shankar, S., Abbas, G., Nithyaprakash, R., Naveenkumar, R., Rakesh Mohanty, S., Sabarinathan, A., and Karthick, S.: Study on the impact of firecrackers on atmospheric pollutants during Diwali festival in Tamil Nadu, India, E3S Web Conf., 453, 01004, https://doi.org/10.1051/e3sconf/202345301004, 2023.
Schneider, P., Bartonova, A., Castell, N., Dauge, F. R., Gerboles, M., Hagler, G., Hüglin, C., Jones, R. L., Khan, S., Lewis, A. C., Mijling, B., Müller, M., Penza, M., Spinelle, L., Stacey, B., Vogt, M., Wesseling, J., and Williams, R. W.: Toward a Unified Terminology of Processing Levels for Low-Cost Air-Quality Sensors, Environ. Sci. Technol., 53, 8485–8487, https://doi.org/10.1021/acs.est.9b03950, 2019.
Sherwood, R. J. and Greenhalgh, D. M.: A personal air sampler, Ann. Occup. Hyg., 2, 127–132, https://doi.org/10.1093/annhyg/2.2.127, 1960.
Shi, Z., Vu, T., Kotthaus, S., Harrison, R. M., Grimmond, S., Yue, S., Zhu, T., Lee, J., Han, Y., Demuzere, M., Dunmore, R. E., Ren, L., Liu, D., Wang, Y., Wild, O., Allan, J., Acton, W. J., Barlow, J., Barratt, B., Beddows, D., Bloss, W. J., Calzolai, G., Carruthers, D., Carslaw, D. C., Chan, Q., Chatzidiakou, L., Chen, Y., Crilley, L., Coe, H., Dai, T., Doherty, R., Duan, F., Fu, P., Ge, B., Ge, M., Guan, D., Hamilton, J. F., He, K., Heal, M., Heard, D., Hewitt, C. N., Hollaway, M., Hu, M., Ji, D., Jiang, X., Jones, R., Kalberer, M., Kelly, F. J., Kramer, L., Langford, B., Lin, C., Lewis, A. C., Li, J., Li, W., Liu, H., Liu, J., Loh, M., Lu, K., Lucarelli, F., Mann, G., McFiggans, G., Miller, M. R., Mills, G., Monk, P., Nemitz, E., O'Connor, F., Ouyang, B., Palmer, P. I., Percival, C., Popoola, O., Reeves, C., Rickard, A. R., Shao, L., Shi, G., Spracklen, D., Stevenson, D., Sun, Y., Sun, Z., Tao, S., Tong, S., Wang, Q., Wang, W., Wang, X., Wang, X., Wang, Z., Wei, L., Whalley, L., Wu, X., Wu, Z., Xie, P., Yang, F., Zhang, Q., Zhang, Y., Zhang, Y., and Zheng, M.: Introduction to the special issue “In-depth study of air pollution sources and processes within Beijing and its surrounding region (APHH-Beijing)”, Atmos. Chem. Phys., 19, 7519–7546, https://doi.org/10.5194/acp-19-7519-2019, 2019.
Shibata, K., Yokoo, T., Takeuchi, K., Tanaka, T., Kamino, M., Nishikawa, S., Nakano, S., and Kuwano, Y.: A new-structure ir gas sensor, Jpn. J. Appl. Phys., 26, 1898, https://doi.org/10.1143/JJAP.26.1898, 1987.
Shusterman, A. A., Teige, V. E., Turner, A. J., Newman, C., Kim, J., and Cohen, R. C.: The BErkeley Atmospheric CO2 Observation Network: initial evaluation, Atmos. Chem. Phys., 16, 13449–13463, https://doi.org/10.5194/acp-16-13449-2016, 2016.
Si, M., Xiong, Y., Du, S., and Du, K.: Evaluation and calibration of a low-cost particle sensor in ambient conditions using machine-learning methods, Atmos. Meas. Tech., 13, 1693–1707, https://doi.org/10.5194/amt-13-1693-2020, 2020.
Singh, A., Ng'ang'a, D., Gatari, M. J., Kidane, A. W., Alemu, Z. A., Derrick, N., Webster, M. J., Bartington, S. E., Thomas, G. N., Avis, W., and Pope, F. D.: Air quality assessment in three East African cities using calibrated low-cost sensors with a focus on road-based hotspots, Environmental Research Communications, 3, 075007, https://doi.org/10.1088/2515-7620/ac0e0a, 2021.
Sivaraman, V., Carrapetta, J., Hu, K., and Luxan, B. G.: HazeWatch: A participatory sensor system for monitoring air pollution in Sydney, in: 38th Annual IEEE Conference on Local Computer Networks - Workshops, Sydney, NSW, Australia, 21–24 October 2013, IEEE, 56–64, https://doi.org/10.1109/LCNW.2013.6758498, 2013.
Smart Citizen: Map of smartcitizen kits, https://smartcitizen.me/kits, last access: 10 March 2024.
Smart Citizen Kit: https://www.seeedstudio.com/Smart-Citizen-Kit-p-2864.html, last access: 10 March 2024.
Soares, A. R. and Silva, C.: Review of ground-level ozone impact in respiratory health deterioration for the past two decades, Atmosphere, 13, 434, https://doi.org/10.3390/atmos13030434, 2022.
Sonkar, S. K., Kumar, P., George, R. C., Philip, D. and Ghosh, A. K.: Detection and Estimation of Natural Gas Leakage Using UAV by Machine Learning Algorithms, IEEE Sens. J., 22, 8041–8049, https://doi.org/10.1109/JSEN.2022.3157872, 2022.
Spinelle, L., Aleixandre, M., and Gerboles, M.: Protocol of evaluation and calibration of low-cost gas sensors for the monitoring of air pollution, JRC Publications Repository, https://doi.org/10.2788/9916, 2013.
Spinelle, L., Gerboles, M., Kok, G., Persijn, S., and Sauerwald, T.: Review of portable and low-cost sensors for the ambient air monitoring of benzene and other volatile organic compounds, Sensors, 17, 1520, https://doi.org/10.3390/s17071520, 2017.
Subramanian, R., Ellis, A., Torres-Delgado, E., Tanzer, R., Malings, C., Rivera, F., Morales, M., Baumgardner, D., Presto, A., and Mayol-Bracero, O. L.: Air quality in Puerto Rico in the aftermath of hurricane maria: A case study on the use of lower cost air quality monitors, ACS Earth and Space Chemistry, 2, 1179–1186, https://doi.org/10.1021/acsearthspacechem.8b00079, 2018.
Sun, L., Wong, K. C., Wei, P., Ye, S., Huang, H., Yang, F., Westerdahl, D., Louie, P. K. K., Luk, C. W. Y., and Ning, Z.: Development and application of a next generation air sensor network for the Hong Kong marathon 2015 air quality monitoring, Sensors, 16, 211, https://doi.org/10.3390/s16020211, 2016.
Sun, Y., Mousavi, A., Masri, S., and Wu, J. Socioeconomic disparities of low-cost air quality sensors in California, 2017–2020, Am. J. Public Health, 112, 434–442, https://doi.org/10.2105/AJPH.2021.306603, 2022.
Suriano, D., Cassano, G., and Penza, M.: A portable gas sensor system for air quality monitoring, in: Sensors and Microsystems, edited by: Di Natale, C., Ferrari, V., Ponzoni, A., Sberveglieri, G., and Ferrari, M., Springer International Publishing, 155–158, https://doi.org/10.1007/978-3-319-00684-0_29, 2014.
Suriano, D., Prato, M., Pfister, V., Cassano, G., Dipinto, S., and Penza, M.: 20 - the case-study of the res-novae national project: Low-cost sensor-systems for urban air quality monitoring, in: Sixth Scientific Meeting EuNetAir, Academy of Sciences, Prague, Czech Republic, 5–7 October 2016, Proceedings, 77–80, https://doi.org/10.5162/6EuNetAir2016/20, 2016.
Tagle, M., Rojas, F., Reyes, F., Vásquez, Y., Hallgren, F., Lindén, J., Kolev, D., Watne, Å. K., and Oyola, P.: Field performance of a low-cost sensor in the monitoring of particulate matter in Santiago, Chile, Environ. Monit. Assess., 192, 171, https://doi.org/10.1007/s10661-020-8118-4, 2020.
Tanzer, R., Malings, C., Hauryliuk, A., Subramanian, R., and Presto, A. A.: Demonstration of a low-cost multi-pollutant network to quantify intra-urban spatial variations in air pollutant source impacts and to evaluate environmental justice, Int. J. Env. Res. Pub. He., 16, 2523, https://doi.org/10.3390/ijerph16142523, 2019.
Tanzer-Gruener, R., Li, J., Eilenberg, S. R., Robinson, A. L., and Presto, A. A.: Impacts of modifiable factors on ambient air pollution: A case study of COVID-19 shutdowns, Environ. Sci. Tech. Let., 7, 554–559, https://doi.org/10.1021/acs.estlett.0c00365, 2020.
Taylor, J. K.: Sampling and calibration for atmospheric measurements: A symposium sponsored by ASTM committee d-22 on sampling and analysis of atmospheres. ASTM International, 1987.
Tékouabou, S. C. K., Chenal, J., Azmi, R., Diop, E. B., Toulni, H., and de Nsegbe, A. P.: Towards air quality particulate-matter monitoring using low-cost sensor data and visual exploration techniques: Case study of Kisumu, Kenya, Procedia Comput. Sci., 215, 963–972, https://doi.org/10.1016/j.procs.2022.12.099, 2022.
TELLUS: AirView Map, https://airview.tellusensors.com/, last access: 17 September 2023.
Thriving Earth Exchange: Projects Archive, https://thrivingearthexchange.org/projects/, last access: 17 September 2017.
Tryner, J., L'Orange, C., Mehaffy, J., Miller-Lionberg, D., Hofstetter, J. C., Wilson, A., and Volckens, J.: Laboratory evaluation of low-cost PurpleAir PM monitors and in-field correction using co-located portable filter samplers, Atmos. Environ., 220, 117067, https://doi.org/10.1016/j.atmosenv.2019.117067, 2020.
Tsujita, W., Yoshino, A., Ishida, H., and Moriizumi, T.: Gas sensor network for air-pollution monitoring, Sensor. Actuat. B-Chem., 110, 304–311, https://doi.org/10.1016/j.snb.2005.02.008, 2005.
Turner, A. J., Kim, J., Fitzmaurice, H., Newman, C., Worthington, K., Chan, K., Wooldridge, P. J., Köehler, P., Frankenberg, C., and Cohen, R. C.: Observed impacts of covid-19 on urban CO2 emissions, Geophys. Res. Lett., 47, e2020GL090037, https://doi.org/10.1029/2020GL090037, 2020.
U.S. Department of Energy: Environmental Justice History, https://www.energy.gov/lm/environmental-justice-history, last access: 7 March 2024.
U.S. EPA (Environmental Protection Agency): NAAQS Table [Other Policies and Guidance], https://www.epa.gov/criteria-air-pollutants/naaqs-table (last access: 17 September 2023), 2014a.
U.S. EPA (Environmental Protection Agency): Village Green Project – Overviews and Factsheets, https://www.epa.gov/air-research/village-green-project (last access: 17 September 2023), 2014b.
U.S. EPA (Environmental Protection Agency): Biden-Harris Administration Announces $53 Million for 132 Community Air Pollution Monitoring Projects Across the Nation, https://www.epa.gov/newsreleases/biden-harris-administration- announces-53-million-132-community-air-pollution (last access: 24 October 2024), 2022.
U.S. EPA (Environmental Protection Agency) Office of Research and Development: Air Sensor Guidebook, https://cfpub.epa.gov/si/si_public_record_Report.cfm?Lab=NERLanddirEntryId=277996, last access: 7 March 2024.
Vajs, I., Drajic, D., and Cica, Z.: Covid-19 lockdown in Belgrade: Impact on air pollution and evaluation of a neural network model for the correction of low-cost sensors' measurements, Appl. Sci., 11, 10563, https://doi.org/10.3390/app112210563, 2021.
Valenzuela, A. A., Schwab, M., Silnik, A. A., Debattista, A. F., and Kiessling, R. A.: Low power wireless sensor node platform for agriculture monitoring in Argentina, in: 2018 International Conference on Cyber-Enabled Distributed Computing and Knowledge Discovery (CyberC), Zhengzhou, China, 18–20 October 2018, IEEE, 101–1014, https://doi.org/10.1109/CyberC.2018.00029, 2018.
van Bree, B.: Innovative Monitoring Network - Ambient Urban Air Quality, Netherlands Organisation for Applied Scientific Research (TNO), https://resolver.tno.nl/uuid:7e12cbaa-0ad0-47f4-9bd6-2682992b8d2a, last access: 25 October 2024.
van Zoest, V. M.: Spatio-temporal modelling of urban sensor network data: Mapping air quality risks in Eindhoven, PhD thesis, Faculty of Geo-Information Science and Earth Observation (ITC), University of Twente, the Netherlands, ISBN 978-90-365-4929-5, https://library.itc.utwente.nl/papers_2020/phd/vanzoest.pdf (last access: 5 November 2024), 2020.
Velásquez, R. M. A., Acevedo, D. M. V., Paredes, M. G. S. P., and Vargas, C. Z.: Implementation and analysis of low-cost network for air pollution in Lima and Arequipa, in: 2022 IEEE XXIX International Conference on Electronics, Electrical Engineering and Computing (INTERCON), Lima, Peru, 11–13 August 2022, IEEE, 1–4, https://doi.org/10.1109/INTERCON55795.2022.9870052, 2022.
Villa, T. F., Gonzalez, F., Miljievic, B., Ristovski, Z. D., and Morawska, L.: An Overview of Small Unmanned Aerial Vehicles for Air Quality Measurements: Present Applications and Future Prospectives, Sensors-Basel, 16, 1072, https://doi.org/10.3390/s16071072, 2016.
Vrijheid, M., Basagaña, X., Gonzalez, J. R., et al.: Advancing tools for human early life course exposome research and translation, Environmental Epidemiology, 5, e166, https://doi.org/10.1097/EE9.0000000000000166, 2021.
Wallace, L., Zhao, T., and Klepeis, N. E.: Calibration of PurpleAir PA-I and PA-II monitors using daily mean PM2.5 concentrations measured in California, Washington, and Oregon from 2017 to 2021, Sensors-Basel, 22, 4741, https://doi.org/10.3390/s22134741, 2022.
Ware, J. H., Spengler, J. D., Neas, L. M., Samet, J. M., Wagner, G. R., Coultas, D., Ozkaynak, H., and Schwab, M.: Respiratory and irritant health effects of ambient volatile organic compounds, Am. J. Epidemiol., 137, 1287–1301, https://doi.org/10.1093/oxfordjournals.aje.a116639, 1993.
Washington University in St. Louis: BKH Outdoor Sensor Network, https://sites.wustl.edu/mongoliaproject/bkh-outdoor-network/, last access: 10 March 2024.
Wen, T.-H., Jiang, J.-A., Sun, C.-H., Juang, J.-Y., and Lin, T.-S.: Monitoring street-level spatial-temporal variations of carbon monoxide in urban settings using a wireless sensor network (Wsn) framework, Int. J. Env. Res. Pub. He., 10, 6380–6396, https://doi.org/10.3390/ijerph10126380, 2013.
Weppner, W.: Solid-state electrochemical gas sensors, Sensor. Actuator., 12, 107–119, https://doi.org/10.1016/0250-6874(87)85010-2, 1987.
Wesseling, J., de Ruiter, H., Blokhuis, C., Drukker, D., Weijers, E., Volten, H., Vonk, J., Gast, L., Voogt, M., Zandveld, P., van Ratingen, S., and Tielemans, E.: Development and implementation of a platform for public information on air quality, sensor measurements, and citizen science, Atmosphere, 10, 445, https://doi.org/10.3390/atmos10080445, 2019.
Whitty, R., Pfeffer, M., Ilyinskaya, E., Roberts, T., Schmidt, A., Barsotti, S., Strauch, W., Crilley, L., Pope, F., Bellanger, H., Mendoza, E., Mather, T., Liu, E., Peters, N., Taylor, I., Francis, H., Hernández Leiva, X., Lynch, D., Nobert, S., and Baxter, P.: Effectiveness of low-cost air quality monitors for identifying volcanic SO2 and PM downwind from Masaya volcano, Nicaragua, Volcanica, 5, 33–59, https://doi.org/10.30909/vol.05.01.3359, 2022.
Williams, R., Duvall, R., Kilaru, V., Hagler, G., Hassinger, L., Benedict, K., Rice, J., Kaufman, A., Judge, R., Pierce, G., Allen, G., Bergin, M., Cohen, R. C., Fransioli, P., Gerboles, M., Habre, R., Hannigan, M., Jack, D., Louie, P., Martin, N. A., Penza, M., Polidori, A., Subramanian, R., Ray, K., Schauer, J., Seto, E., Thurston, G., Turner, J., Wexler, A. S., and Ning, Z.: Deliberating performance targets workshop: Potential paths for emerging PM2.5 and O3 air sensor progress, Atmospheric Environment: X, 2, 100031, https://doi.org/10.1016/j.aeaoa.2019.100031, 2019.
Windy: https://www.windy.com/, last access: 10 March 2024.
Wyzga, R. E. and Rohr, A. C.: Long-term particulate matter exposure: Attributing health effects to individual PM components, J. Air Waste Manage., 65, 523–543, https://doi.org/10.1080/10962247.2015.1020396, 2015.
World Meteorological Organization: 2021 WMO Report No. 1215 – An update on low-cost sensors for the measurement of atmospheric composition, ISBN 978-92-63-11215-6, https://library.wmo.int/idurl/4/37465 (last access: 8 March 2024), 2021.
Xi, X., Johnson, M. S., Jeong, S., Fladeland, M., Pieri, D., Diaz, J. A., and Bland, G. L.: Constraining the sulfur dioxide degassing flux from Turrialba volcano, Costa Rica using unmanned aerial system measurements, J. Volcanol. Geoth. Res., 325, 110–118, https://doi.org/10.1016/j.jvolgeores.2016.06.023, 2016.
Yaacoub, E., Kadri, A., Mushtaha, M., and Abu-Dayya, A.: Air quality monitoring and analysis in Qatar using a wireless sensor network deployment, in: 2013 9th International Wireless Communications and Mobile Computing Conference (IWCMC), Sardinia, Italy, 1–5 July 2013, IEEE, 596–601, https://doi.org/10.1109/IWCMC.2013.6583625, 2013.
Yang, L. H., Hagan, D. H., Rivera-Rios, J. C., Kelp, M. M., Cross, E. S., Peng, Y., Kaiser, J., Williams, L. R., Croteau, P. L., Jayne, J. T., and Ng, N. L.: Investigating the sources of urban air pollution using low-cost air quality sensors at an urban Atlanta site, Environ. Sci. Technol., 56, 7063–7073, https://doi.org/10.1021/acs.est.1c07005, 2022.
Yavus, E., Kuzu, S. L., and Saral, A.: Investigation of vertical particulate matter distribution using low-cost sensors in Istanbul, Turkey, BL2ES2-21, BABES-21 and BISET-21, Barcelona, Spain, 13–15 October 2021, https://doi.org/10.17758/EIRAI10.F1021210, 2021.
Yoo, E.-H., Zammit-Mangion, A., and Chipeta, M. G.: Adaptive spatial sampling design for environmental field prediction using low-cost sensing technologies, Atmos. Environ., 221, 117091, https://doi.org/10.1016/j.atmosenv.2019.117091, 2020.
Zaidan, M. A., Hossein Motlagh, N., Fung, P. L., Lu, D., Timonen, H., Kuula, J., Niemi, J. V., Tarkoma, S., Petäjä, T., Kulmala, M., and Hussein, T.: Intelligent calibration and virtual sensing for integrated low-cost air quality sensors, IEEE Sens. J., 20, 13638–13652, https://doi.org/10.1109/JSEN.2020.3010316, 2020.
Zaldei, A., Vagnoli, C., Di Lonardo, S., Gioli, B., Gualtieri, G., Toscano, P., Martelli, F., and Matese, A.: AIRQino, a low-cost air quality mobile platform, EGU General Assembly, Vienna, Austria, 12–17 April 2015, EGU2015-6158, 2015.
Zampolli, S., Elmi, I., Ahmed, F., Passini, M., Cardinali, G. C., Nicoletti, S., and Dori, L.: An electronic nose based on solid state sensor arrays for low-cost indoor air quality monitoring applications, Sensor. Actuat. B-Chem., 101, 39–46, https://doi.org/10.1016/j.snb.2004.02.024, 2004.
Zeb, A., Soininen, J.-P., and Sozer, N.: Data harmonisation as a key to enable digitalisation of the food sector: A review, Food Bioprod. Process., 127, 360–370, https://doi.org/10.1016/j.fbp.2021.02.005, 2021.
Zhou, F., Liu, J., Zhu, H., Yang, X., and Fan, Y.: A Real-Time Measurement-Modeling System for Ship Air Pollution Emission Factors, J. Mar. Sci. Eng.-Basel, 10, 760, https://doi.org/10.3390/jmse10060760, 2022.
Zimmerman, N., Presto, A. A., Kumar, S. P. N., Gu, J., Hauryliuk, A., Robinson, E. S., Robinson, A. L., and R. Subramanian: A machine learning calibration model using random forests to improve sensor performance for lower-cost air quality monitoring, Atmos. Meas. Tech., 11, 291–313, https://doi.org/10.5194/amt-11-291-2018, 2018.
- Abstract
- Introduction
- Quasi-permanent low-cost sensor networks
- Campaign-based low-cost sensor networks
- Trends across stationary low-cost sensor networks
- Sensor network unification efforts
- Emerging and future sensor directions
- Conclusions
- Code availability
- Data availability
- Author contributions
- Competing interests
- Disclaimer
- Acknowledgements
- Financial support
- Review statement
- References
- Abstract
- Introduction
- Quasi-permanent low-cost sensor networks
- Campaign-based low-cost sensor networks
- Trends across stationary low-cost sensor networks
- Sensor network unification efforts
- Emerging and future sensor directions
- Conclusions
- Code availability
- Data availability
- Author contributions
- Competing interests
- Disclaimer
- Acknowledgements
- Financial support
- Review statement
- References