the Creative Commons Attribution 4.0 License.
the Creative Commons Attribution 4.0 License.
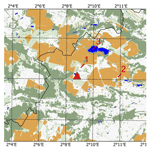
Eddy covariance with slow-response greenhouse gas analysers on tall towers: bridging atmospheric and ecosystem greenhouse gas networks
Pedro Henrique Herig Coimbra
Benjamin Loubet
Olivier Laurent
Laura Bignotti
Mathis Lozano
Michel Ramonet
Greenhouse gas monitoring is important to ensure climate goals are being achieved. This study unveils the potential of using atmospheric tall towers in direct flux measurements, bridging the gap between atmospheric and ecosystem monitoring networks. The ICOS Cities (PAUL) project aims to monitor CO2 emissions in urban areas, where concentrated emissions make them key targets for climate change mitigation. This study explores the synergy between ICOS atmospheric and ecosystem networks by utilizing slow-response analysers (∼ 3 s) on tall atmospheric towers for ecosystem studies using the eddy covariance method. A standard setup with an ultrasonic anemometer and an infrared (IR) fast-response CO2 analyser was installed and compared with measurements from an existing cavity ring-down spectroscopy (CRDS) analyser measuring CO2, CO, and CH4. Deployed on the 100 m Saclay tower near Paris, covering a 43.9 km2 80 % footprint with heavy traffic roads, a nearby heating plant, and a forest, the setup addressed technical challenges and height-induced complexities. Corrections for flux attenuation by high-frequency losses were limited to < 20 % on average for all stabilities and around 11 % for unstable conditions. Elevated mean fluxes for CO2 (10 ) and CH4 (200 ) were observed from the heating plant wind direction during December and January. Conversely, the forest direction exhibited the strongest sink among all wind directions, with −4 during July and August. Storage and vertical advection were estimated using the routine three-level profile measurements done in ICOS atmospheric towers. Storage term was of the same magnitude as turbulent flux, increasing at night and de-stocking during the first half of the day. Vertical advection averaged zero on a monthly basis. These results demonstrate the feasibility and versatility of utilizing atmospheric towers for urban emission monitoring, offering valuable insights for emission monitoring strategies worldwide.
- Article
(4362 KB) - Full-text XML
-
Supplement
(1354 KB) - BibTeX
- EndNote
Global surface temperature is 1.6 °C warmer on land compared with the pre-industrial era (IPCC, 2021), and projections show more than 2 °C warming in 2100 (IPCC, 2022). Warming results from the increase in greenhouse gas (GHG) concentration in the atmosphere, mainly driven by anthropogenic emissions (IPCC, 2021), of which 86 % comes from fossil fuel CO2 (Canadell et al., 2023). Agriculture, forestry, and other land use (AFOLU) are a significant source of GHG (12.0 ± 2.9 Gt CO2-eq yr−1) while concurrently possessing the potential to remove CO2 from the atmosphere (Jia et al., 2022).
Urban areas concentrate human activities and represent a significant source of GHG emissions, consequently making it one of the targets for mitigating climate change. Many northern countries' cities have ambitious GHG emission reduction plans over the next 2 decades that consist of electrifying the energy grid, implementing car-free zones, and investing in insulation improvement. Consequently, there arises an imperative for robust monitoring of urban areas' emissions reduction. Several works have tried to decompose eddy covariance measurements in (sub-)urban setups with different degrees of uncertainty (Velasco et al., 2009; Bergeron and Strachan, 2011; Ueyama and Takano, 2022). Currently, in Europe, the ICOS Cities (PAUL) project aims to advance technologies for monitoring CO2 concentrations in urban areas of three different-sized pilot cities (Munich, Paris, and Zurich).
Monitoring GHGs in the atmosphere, ocean, and ecosystem is the objective of world-distributed research infrastructures such as ICOS in Europe (Heiskanen et al., 2022). For this purpose, different methods are used on terrestrial sites. Ecosystem sites focus on local flux monitoring using high-frequency measurements. These sites measure the different terms of the surface fluxes often representing a specific biome determined by the tower's footprint. In contrast, atmospheric towers precisely measure the concentrations as an imprint of larger-scale fluxes. These sites have a footprint spanning several hundreds of square kilometres and may be used to identify anomalies in CO2 surface fluxes based on concentration (Ramonet et al., 2020) or retrieve surface flux by inverse modelling (Ciais et al., 2011).
At local scale, eddy covariance (EC) is the reference method for GHG monitoring. The method is praised for directly and continuously measuring surface turbulent flux and has largely been applied since early measurements of different gases, including water vapour, CO2, CH4, and N2O (Valentini et al., 1996; Moncrieff et al., 1996; Fowler et al., 1995). Standard measurements require fast-response instruments, which is a technical limitation in measuring certain compounds' concentrations. Long-term measurement sites are equipped with CO2 and H2O gas analysers and in some wet or agricultural sites with N2O or CH4 analysers (Nemitz et al., 2018). At larger scales, atmospheric concentration measurements are often used alongside mesoscale–continental-scale transport models to solve surface flux (Lauvaux et al., 2012). This top-down approach is often validated locally by direct EC measurements (Vuichard et al., 2016).
The differences between typical atmospheric and flux tower monitoring setups are the following:
-
Atmospheric towers are generally taller, exceeding 100 m, while flux towers range from 2 to 40 m. This height difference is due to atmospheric measurements being designed to capture seasonal and annual trends in regional atmospheric background concentrations, necessitating the minimization of local source impacts (El Yazidi et al., 2018), while flux towers focus on those local sources.
-
Atmospheric towers feature more precise but slower measurements, not cadenced, with sampling intervals spanning several seconds, in contrast to the eddy covariance method used by flux towers, which samples at constant rates between 5 and 20 Hz.
-
Ecosystem stations include monitoring of vegetation and soil, which is absent in atmospheric stations, while atmospheric stations measure additional gaseous compounds such as CH4, N2O, and CO (Hazan et al., 2016).
Being able to use slow-response analysers to calculate flux by eddy covariance has been identified as a useful strategy to expand the flux networks to other compounds (Wohlfahrt et al., 2009). Atmospheric towers have high-precision analysers, which, if we can use them to compute eddy covariance fluxes, would provide multi-species flux measurements that would expand the flux network. This would require a fast 3D anemometer and continuous data logging at these sites. Not all towers may be suitable though, as flux towers have more constraints related to the topography and surrounding obstacles.
Moreover, in atmospheric towers, ICOS focuses on measuring not only CO2 but also CO and CH4 concentrations routinely. Therefore, measuring fluxes on these towers potentially enables the measurement of CO and CH4 fluxes in the surrounding areas of each tower. These gases can bring relevant information on the site greenhouse gas budget, directly as CH4 is a greenhouse gas and indirectly by revealing processes otherwise mixed in the CO2 flux. Carbon monoxide (CO) emissions come in the most part from direct fuel combustion in the Northern Hemisphere mid-latitudes (Zheng et al., 2019). These emissions are likely to increase during winter due to reduced combustion efficiency at lower temperatures (Helfter et al., 2016). Methane (CH4) emissions are primarily sourced from natural wetlands, enteric fermentation, and fossil fuels (Saunois et al., 2020). Locally, lakes may be a significant contributor (e.g. 0.1–0.3 in Iwata et al., 2020), but their contribution depends on the amount of sediments (Delwiche et al., 2021). Soils act primarily as sinks for both CO (Inman et al., 1971; Conrad and Seiler, 1980; Conrad, 1996) and CH4 (Canadell et al., 2023; Dutaur and Verchot, 2007) through microbial oxidation processes.
In this study, we evaluate the capability of using an atmospheric monitoring tower with a slow-response analyser to compute turbulent fluxes of CO2, CH4, and CO by adding a fast-response sonic anemometer. For this purpose we installed a standard eddy covariance setup for CO2 at 100 m at the ICOS FR-SAC atmospheric tower in the south of Paris, collecting 4 months of data from July 2023 until October 2023. The chosen site is located in a sub-urban region surrounded by a mix of agriculture, forest, wetlands, roads, and areas with buildings. We computed net CO2 flux for slow- and fast-response analysers and compared them. The high-frequency loss was determined and the correction procedure evaluated. Recommendations are made for sites interested in measuring fluxes with the background concentration setup. The storage flux was computed using three-point profile concentrations routinely measured at the ICOS tower. The seasonal variations and variations with wind directions of the CO2, CH4, and CO fluxes were then briefly discussed.
The benefits of expanding geographical coverage and increasing the range of measurable gases using slow-response analysers are clear. The benefits of using existing networks for background concentration measurements are clear as well. However, the constraints of concentration and flux measurements are not the same, and so not all towers may be suitable. For any atmospheric tower, a couple of adversities must be addressed first. Discarding atmospheric stations on mountains that have unsuitable conditions for flux measurements, we focus on tall towers over reasonably flat landscapes.
2.1 Time response and high-frequency attenuation
Measuring flux with a 3 s response time analyser is challenging. High-frequency measurements contain information that is overlooked when measuring at slower frequencies. Fast-response analysers, typically with 100 ms response time, are needed for flux measurements to capture the small and fast eddies (turbulent fluctuations) that carry most of the flux signal in the surface layer (Kaimal and Finnigan, 1994). Using slow-response analysers on short towers would mean losing most of the signal. Fortunately, the contribution of higher frequencies to the EC flux is inversely proportional to height (Horst, 1997).
For instance, using a simple estimator for the attenuation (Horst, 1997), we find that using a slow analyser with a time response of 3 s would attenuate the flux by 60 % in unstable conditions with wind speed of 4 m s−1 for a 4 m tower, decreasing to 27 % on a 30 m tower and further decreasing to 8 % on a 100 m tower. Under stable conditions, the attenuation may increase to more than 90 %, 60 %, and 25 % respectively. Thus, at 100 m we expect only a limited amount of correction despite using a slow-response gas analyser.
High-frequency (HF) corrections based on predefined or experimental cospectra profiles are well established and routinely applied to correct for tube attenuations in ICOS and other flux networks (Horst, 1997; Massman and Lee, 2002; Ibrom et al., 2007; Fratini et al., 2012). We therefore expect sampling with slow-response analysers at tall towers may be suitable because the peak of the covariance cospectrum would be caught well and could be corrected with standardized approaches (Massman, 2000).
2.2 On the consequences of the height
Firstly, measurement height can impact footprint heterogeneity and non-stationarity. Height affects the source area of flux measurements, with taller towers having larger footprints. Data points are comparable only if their footprints are similar, which is less of an issue in homogeneous ecosystems. However, the ever-changing footprints due to wind conditions have a significant impact on the measured flux in heterogeneous environments. Furthermore, when these shifts happen during the averaging period, typically 30 min, they can lead to data being flagged as non-stationary and unusable with the standard eddy covariance method. This issue may be more pronounced for certain gases.
Secondly, height can have consequences for the terms of surface flux. Surface flux includes three terms: storage, advection, and turbulent transport (Finnigan et al., 2003). Storage flux arises from the accumulation or release of the compound below the measurement height. Advection is the transport by an organized flow, and it can occur when there is a non-zero mean wind velocity. Turbulent transport involves mixing caused by the eddies near the surface. While for short towers of only a few metres, the turbulent term predominates, taller towers require storage and vertical advection to be accounted for (Aubinet et al., 2005). As the measurement height increases, so does the volume below the measuring point, allowing bigger storage fluxes, and frictional effects decrease, allowing faster wind speeds. Larger-scale atmospheric circulation patterns also become more pronounced with height. While boundary layer dynamics may impact the surface fluxes, entrainment from the top of the atmospheric boundary layer may only have significant impact on lower frequencies (Asanuma et al., 2007). Here, we assume entrainment plays a negligible role in the turbulent fluxes.
Typically, positive storage flux may result from the decoupling of surface and atmospheric dynamics. Such a decoupling may arise especially under a stably stratified surface layer, occurring at night above canopies, especially under radiative cooling conditions (Kaimal and Finnigan, 1994). Negative storage fluxes arise during the early morning when the atmospheric boundary layer rises and the stably stratified layer breaks down (Aubinet et al., 2005). At tall towers, the storage can be high and remain large in the morning when the vegetation starts photosynthesizing but the turbulence is still low (Haszpra et al., 2005). At very tall towers (300 m), the storage dominates the flux dynamics (up to 95 % of the total flux, Winderlich et al., 2014). Under ideal surface homogeneous conditions, the storage term is expected to tend to zero when averaged over a day and hence only affect the surface flux dynamics but not the integrated fluxes. Over other conditions, this might be violated. The atmospheric boundary layer expansion and contraction may contribute to vertical wind dynamics and so to vertical advection as well. In some cases, including vertical advection was sufficient to reach a closed mass balance (Mammarella et al., 2007). Horizontal advection, on the other hand, is hard to measure with the current setups that focus on single-tower measurements. During decoupling it can have consequences by moving air parcels away from the measuring point, effectively renewing the air below measuring point with air from outside the area of interest.
For the turbulent fluxes the impact is less significant. In general, fluxes which would be measured as turbulent at a short tower may first appear as storage at a taller tower while being released as turbulent flux at a later stage. In addition to this, as we go farther from the sources and sinks, the concentration gradient, and thus the instant deviation, gets smaller. Consequently, this requires more accurate instruments.
3.1 Site description
The study uses data from a 100 m tall tower in the French Alternative Energies and Atomic Energy Commission (CEA) at a research campus in Saclay, 20 km southwest of Paris (Fig. 1). The tower is part of the ICOS atmospheric network (FR-Sac) and takes part in the ICOS Cities, Pilot Applications in Urban Landscapes (PAUL), project, focused on integrated city observatories for greenhouse gases. Climatically, the area is under oceanic influence, with mild temperatures (11.5 °C annual mean) and moderate precipitation (677–700 mm annual). The surrounded landscape is dominated by artificial (buildings, roads), agriculture (mainly cereal), and forest sources. The region serves as a pathway for urban-to-suburban daily mobility, with more than 60 000 vehicles every day in 2022 according to SIREDO on the national (N118) and regional (D306, D36, D128) roads.
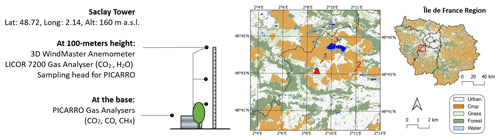
Figure 1Site diagram showing tower and acquisition house and tree for scale. In the right panel, the site map and the localization in the region are shown. Land use type is indicated in the colour legend. For reference, (1) heating plant, (2) manure/composting plant, and (3) lake.
Since 2011 the site has been equipped with high-precision cavity ring-down spectroscopy (CRDS) gas analysers (CO2, CO, CH4, G2401; Picarro Inc., Santa Clara, CA, USA) with a varying time response of a few seconds. The analyser is placed in a ground level hut, connected to three sampling lines, 12.7 mm of diameter, collecting air at the three different heights of the tower (15, 60, 100 m above ground level, a.g.l.) alternatively every 10 min. Since 2017, a second multi-gas analyser has been measuring continuously through a parallel sampling line connected to the top of the tower (100 m a.g.l.).
The flow rate through the sampling lines is set around 12 L min−1 but with no control. At the bottom of all lines connected to the CRDS analyser, the air is dried with Nafion (PermaPure, model MD-070-144S-4). The CRDS gas analysers followed the ICOS calibration procedure, aiming for a precision higher than 50, 1, and 2 ppb for CO2, CO, and CH4 (ICOS RI, 2020). From June to October 2023, we set up a full eddy covariance system at 100 m, consisting of a closed-path infrared (IR) gas analyser (LI-7200; Li-Cor Inc., Lincoln, NE, USA), a 0.7 m heated tube with a flow rate set to 15 L min−1, and a three-dimensional sonic anemometer (Gill WindMaster; Gill Instruments Ltd, Lymington, Hampshire, UK). The anemometer kept running after October 2023. Given the thin shape of the tower and the setup position on top, no major disturbances are expected from wind measurements. The tower is also equipped with pressure (Vaisala PTB200), humidity, and temperature sensors (Vaisala HMP155) at 1.5, 60, and 100 m.
Half-hourly average dry CO2 mixing ratio showed a high degree of comparability between instruments (R2 0.97) and no bias (slope = 1) (Fig. 2). Nonetheless we found an offset of 7.25 ppm and an average drift of −11 ppm yr−1, which has no impact on eddy covariance flux.
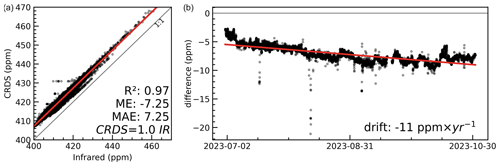
Figure 2Comparison between dry CO2 mixing ratio measured by the IR (LI-7200) and the CRDS (Picarro G2401) analysers. (a) Scatter plot. (b) Mixing ratio difference (IR-CRDS) as a function of time. Dots are observations, the red line is the linear fit, and the grey line is the 1:1 line. The correlation coefficient (R2), the mean error (ME; ppm), the mean absolute error (MAE; ppm), the linear fit, and the drift are shown.
Data were not available for most of November due to maintenance in the instruments. The IR (LI-7200) was set up unconventionally, with the analyser placed horizontally and the sampling tube vertically with a U-shaped head and a rain cap turned downwards. The choice was made based on the safety for maintenance on top of the tower. Unfortunately, the IR malfunctioned during the measurement campaign and prevented all the analysers from running at the same time for the whole period.
3.2 Data processing
The mass balance equation to compute surface flux includes three terms: storage, advection, and turbulent transport (Foken et al., 2012). With the setup of this work, horizontal advection was not addressable. The vertical component of the wind (w) and the mixing ratio of a scalar s (χs) can then be used to calculate flux at the surface Feco based on the turbulent covariance () measured at a certain height (hm), the storage term (, where t is time and z is the height) and vertical advection. Here overbars stand for time averaging. The surface flux Feco () can then be expressed as a function of the molar volume of dry air , where P is atmospheric pressure (Pa), e is vapour pressure (Pa), R is the ideal gas law constant (8.31 J kg−1 K−1), and Ta is air temperature (K):
3.2.1 Turbulent flux calculation
The turbulent flux was calculated based on covariance, hence the name of the eddy covariance (EC) method. Pre-processing is required and was done using EddyPro 7.0.9, applying de-spiking (Mauder et al., 2013), covariance maximization for time lag, and planar fit (Wilczak et al., 2001). Note that planar fit is required to be able to estimate vertical advection. Time lag relates to the delay from sampling and measurement, and the maximization can lose reliability under noisy measurements (Langford et al., 2015). Typically, a default value and bounds are set individually for each gas and gas analyser. If an optimal value falls within the bounds, it is retained; otherwise, the default is chosen. For the Li-Cor 7200 analyser the lag time was set to 0.09 ± 0.35 s based on tube dimensions and flow rate. For the Picarro analysers, which had a 100 m line, the lag time was set to 60 ± 2 s based on comparison with the Li-Cor 7200 CO2 concentration. This lag time is compatible with a flow rate ∼ 12.6 L min−1. The ±2 s tolerance was included to account for the uncertainty over the precise travel time and possible seasonal changes linked to air viscosity dependency on temperature and filter dirtiness.
Turbulent flux was calculated using EddyPro 7.0.9. For EC flux calculation, the slow-response analyser (Picarro) was resampled to 10 Hz to synchronize with the sonic anemometer sampling rate. This was achieved by repeating each measured value until it changed.
3.2.2 Storage and vertical advection flux computation
The storage and vertical advection were calculated using the three levels of measurements done by the same instrument, alternating between the three heights by periods of 10 min. The scalars CO2, CO, and CH4 were measured at 15, 60, and 100 m with the CRDS analysers. The dry air volume ratio was computed at each height based on measured air relative humidity and temperature. The 10 min average measurements were linearly interpolated, and 30 min averages were calculated.
The storage flux was computed as in Aubinet et al. (2005) as the derivative over time of scalar s contained in the column below the measurement height (100 m):
where Δt is 30 min, index i stands for the three layers (0–15, 15–60, and 60–100 m), and Δzi is the layer depth.
The vertical advection flux was computed using the derivative over the height of scalar s and integrated over the measurement height (100 m). Calculations were done in Python using NumPy (Harris et al., 2020) and SciPy (Virtanen et al., 2020).
3.2.3 Quality flags and stability classes
Quality flags were assigned using the standard 0–1–2 flag system from FLUXNET (Mauder and Foken, 2011), involving tests for stationarity and fully developed turbulence (Foken and Wichura, 1996). The stationarity test (STA) measures the absolute relative deviation between 5 and 30 min covariances, while the integral turbulence test (ITC) assesses the deviation between measured and modelled integral turbulent characteristics. Data are considered to be of high (< 30 %), medium (30 %–100 %), or low (> 100 %) quality, based on deviation percentages for each test (worst applicable result prevails). A detailed description of the quality flags can be found in Foken and Wichura (1996).
Stability classes were defined using the stability parameter , where z is the measurement height, d the zero-plane displacement height, and L the Obukhov length. We classified stability as unstable (ζ < −0.2), near-neutral (−0.2 0.2), and stable (ζ > 0.2).
3.3 High-frequency corrections on noisy measurements
Instruments have measurement limitations, which decreases their ability to produce a true value. Closed-path gas analysers require a gas sample to pass through a tube system including filters. Longer tube lengths typically result in increased time lag and reduced high-frequency signal. The signal degradation can be represented by a transfer function, TF, which attenuates the high frequency (Ibrom et al., 2007) of the true cospectrum of w and a compound s:
where f is the frequency (Hz), and Sps is the spectrum between w and a scalar s. Note that we can consider the transfer function equal for the spectrum and cospectrum, as we neglect the w transfer function and spatial sensor separation for the case of this tall tower (Massman, 2000). We assume the true covariance can be estimated by multiplying the measured covariance by a correction factor, CF:
Acknowledging that the covariance is the integral over all frequencies of the cospectra, the correction factor (CF) can be calculated from the transfer function (TF) and a true cospectrum, which is usually taken to be wTs (where Ts is the ultrasonic temperature). Indeed, we assume similarity of scalars in the atmospheric boundary layer and use the (co)spectrum of Ts as a proxy of the unattenuated cospectrum, as Ts is collocated to w (Ibrom et al., 2007). For CF, this yields
where TF can be calculated in different forms and can account for both low- and high-frequency attenuation. Experimental methods are recommended for high-frequency spectral correction (Ibrom et al., 2007; Fratini et al., 2012). We can approximate an empirical TF, explained further down, using a first-order system, as the product of a transfer function H accounting for a first-order filter's time constant, τc, representing the system response time (s), and a transfer function Hp, accounting for a generic phase shift φ as (Massman, 2000)
Note that the cut-off frequency, fc, equals . Ideally H would be the measured-to-true spectra ratio for the scalar of interest. However, only the measured spectrum is known, and so Eq. (7) is fitted using the sonic temperature Ts as a proxy of the unattenuated spectrum (Ibrom et al., 2007; Fratini et al., 2012; Peltola et al., 2021):
where Fn is a normalization factor to account for any inaccuracies in the variance.
Sometimes TF=H is used, and Hp is not considered (Ibrom et al., 2007). However, not accounting for the phase shift (e.g. using cross-covariance maximization for lag correction and solely H for cospectra correction) can bias CF (Peltola et al., 2021). Fortunately, , which leads to (Peltola et al., 2021). In this work we use Fratini et al. (2012), where .
The spectra and cospectra were calculated using EddyPro 7.0.9, following Fratini et al. (2012) described here in Eqs. (5), (7), and (9). The transfer function H, accounting for the first-order filter's time constant τc, was estimated for each analyser and each compound through a least-squares minimization approach of the spectra (Fig. 3).
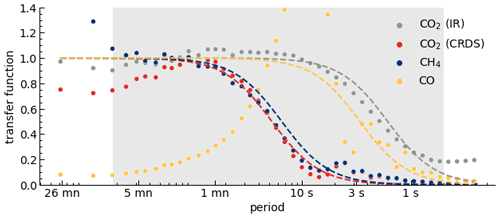
Figure 3Transfer functions H (dotted lines) for each compound and analyser. The transfer function was fitted to the ratio of each compound's spectra to the sonic temperature spectra. All spectra are ensemble-averaged taken from EddyPro outputs, filtered for significant fluxes. Dots show the mean spectra per frequency band. The grey-shaded area shows the frequency range (2–0.0018 Hz) over which transfer functions were fitted. See Fig. S1 in the Supplement for more detailed power spectra.
From the H, TF was computed as , and CF was calculated with Eq. (5). For both TF optimization and CF calculation, only frequencies between 2 and 0.0018 Hz were used (see Fig. 3).
3.4 Footprint and spatial tools
For an analysis of the fluxes' footprint as a function of wind direction, we used a simple parameterization of a backward Lagrangian stochastic particle dispersion model (LPDM-B) for the footprint (Kljun et al., 2015) and computed vegetation indexes based on Sentinel 2 (ESA/Copernicus Data) and a French land use map (Thierion et al., 2022). Note that a single roughness parameter was used for the footprint calculations, while in reality the covers and thus the roughness vary according to land use and wind direction. This source of uncertainty is reduced by taking measurements at 100 m a.g.l., farther away from the surface than typical flux towers.
3.5 Performance measurements
Comparisons between instruments were carried out using mean bias and absolute error, defined as
where N equals the amount of data, and X is the variable measured with instrument a and b at a time n.
In figures, 95 % confidence interval bands were calculated using the Seaborn module in Python. It uses a random sampling method with replacement strategy, bootstrapping, to construct a confidence interval (Dragicevic, 2016).
For linear fits, if not declared otherwise, the squared loss, also named ordinary least-squares method, is used. The method consists of minimizing the sum of the squares of the difference between the observed and predicted values. When robust or Huber loss is mentioned, we use a linear fit, which minimizes the squared loss for the samples where the absolute difference between the observed, y, and predicted, f(x), values is smaller than δ and the absolute loss, sum of the absolute difference, otherwise. This feature makes it less sensitive to outliers than the squared error.
By default, we arbitrarily chose δ = 5; note that very low δ values may increase the number of values considered outliers.
4.1 Mixing ratios of CO2, CO, and CH4
In Fig. 4, the diel pattern shows a peak in during the morning (07:00 CET (UTC+1)) in July and moving towards 09:00 in October and a clear valley around 15:00. The pattern disappears when moving towards winter months. CO and CH4 both show a similar peak in the morning in autumn, although less marked. Only CO shows an afternoon peak in September, which is also the month with the clearer morning peaks for CH4 and CO. Seasonally, CO2, CO, and CH4 mixing ratios are the highest in winter. This difference may be explained by a larger biogenic CO2 sink during the daytime in summer and a higher anthropogenic CO2 emission in winter (heating on). The difference may also be explained by larger (smaller) boundary layer thickness during the summer (winter) which can effectively dilute (concentrate) the molecules emitted at the ground.
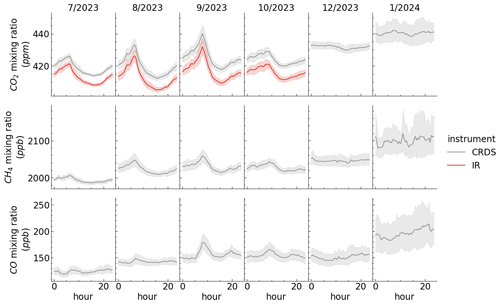
Figure 4Monthly dry mixing ratio diel pattern for all measured gases (CO2, CH4, and CO) for IR (LI-7200) and CRDS (Picarro G2401). Solid line indicates median, and the shaded region shows 95 % confidence interval.
A look into how the mixing ratios vary with wind direction reveals some spatial patterns (Fig. 5). During warmer months (July to October), the west-wind CO2 mixing ratio was smaller than the median value, while for CH4 and CO we can notice a value that is higher than the median for the northeast sector, especially clear for CO. In colder months (December and January), all mixing ratios were higher (also seen in Fig. 4), with easterly winds (0–180°) showing larger mixing ratios than in other directions. A peak in mixing ratios is observed for all three gases for winds coming from around 20° N, the direction from the heating plant. Interestingly, a smaller peak can be seen in the northwest direction from the lake (100 m away), bare soil fields (around 500 m away), and a regional road roundabout (around 1 km away).
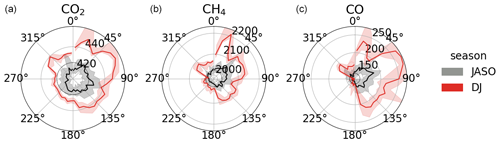
Figure 5Median dry mixing ratios by wind direction; the band shows the interquartile (25th–75th percentiles). Warmer months (JASO, July to October) in grey and colder months (DJ, December and January) in red. Extreme values in the left and right 0.1 % tails were removed. See Fig. S2 for monthly observations. CO2 is in parts per million (ppm), while CH4 and CO are in parts per billion (ppb). All data are shown, including non-stationary and underdeveloped turbulence.
4.2 Footprint and stationarity
4.2.1 Footprint analysis
A characterization of the site's flux footprint (Fig. 6) shows a heterogeneous landscape composition, comprising 25 % urban, 23 % agriculture, 21 % forest, and 21 % grassland areas inside the 90 % level source area. In the western part of the site (42 % forest), there is a relatively dense woodland primarily featuring deciduous trees. To the south (41 % grassland), the landscape includes a nearby golf club in the vicinity of the CEA campus. In all directions there are croplands, predominantly cultivated cereal crops (winter wheat, barley, maize) and oilseeds (rapeseed), typical of the region. In the northeast (45 % urban), the landscape aligns with the location of the CEA campus, which includes a heating plant aligned to 20° N. The 43.9 km2 80 % footprint encompasses a national road (N118) and several regional roads (D306, D36, D128), with weekly traffic of 60 000 vehicles on average (in 2022 according to SIREDO). Water ponds have a small contribution for northwest to northeast sectors (2.3 %–2.6 %). Two ponds are situated in these sectors, one approximately 100 m from the tower (northwest) and a second larger farther away (around 2.4 km northeast, visible on the map in Fig. 6a).
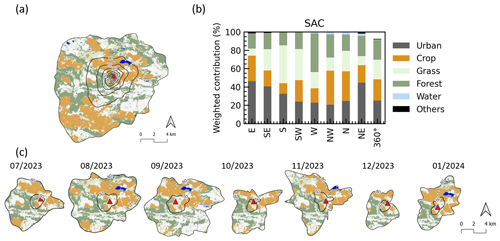
Figure 6Flux footprint by land use group. (a) Footprint for the whole period, where lines indicate 10 % to 90 % (border) level source area. (b) Contribution of each land use weighted by footprint density inside 90 % level source area. (c) Monthly footprint, where lines indicate 50 % and 80 % (border) level source area. Note that for visual purposes, the urban area is coloured white-grey in the map. Footprints estimated using model in Kljun et al. (2015).
In Fig. 6c, we can see the monthly changes in composition and shape of the area contributing to the fluxes measured at the tower (the flux footprint). Some months have larger footprints (e.g. August and September), while others have smaller ones (e.g. December and January), related to changes in the dispersion conditions. This difference is explained by the largest occurrence of stable conditions during the summer nights, which leads to larger footprints than during the winter, which mainly has neutral conditions (shown by the stability ratio ζ in Fig. 7b) driven by stronger winter winds, elevated friction velocity, and cloudy conditions (Fig. 7). Note as well that the landscape is not homogeneous (Fig. 6b), and so wind direction can also change the effective profile of sources and sinks contributing to each compound flux measured at the tower. December, for instance, was the month with the least contribution from the most urban northeast sector.
The mixing layer height, and similarly the atmospheric boundary layer height, shows a clear diurnal and seasonal cycles (Fig. 7). Warmer hours of the day and months show taller boundary layer heights, implying a larger volume of developed layer in which the compounds can be diluted. The mixed-layer heights were on average several times higher than the measuring height and so limiting any effect from entrainment. During these warmer periods, the conditions are often unstable ( < 0.2), and friction velocity is high (> 0.4 m s−1). This indicates well-mixed layer and bigger eddy sizes. Conversely, colder months (December and January) showed a relatively flat diel pattern, mostly due to a shorter photoperiod, leading to a much lower boundary layer height. We also noted on-site fog was frequently observed during these periods. Concurrently, friction velocity increased on average during winter. We note that horizontal winds, over heterogeneous terrain in particular in stable and neutral conditions, would favour horizontal advection. In the scope of the present work, however, it was not quantified due to a lack of measurements (horizontal gradients of concentration and fluxes).
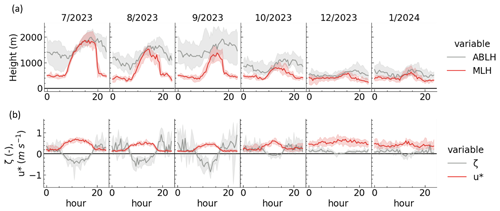
Figure 7Boundary layer conditions. (a) Heights of the atmospheric boundary layer (ABLH) and the mixing layer (MLH) measured at the SIRTA in Palaiseau, 4.8 km away from the tower. Data available online (Kotthaus et al., 2023). (b) Stability parameter ( and friction velocity (u∗) measured at the FR-Sac tower. Absolute values of ζ bigger than 2 were ignored.
4.2.2 Stationarity and well-developed turbulence
Most of the data collected were under well-developed turbulence, 73 % if only considering high-quality (flag 0) data based on the ITC test and 99 % including medium-quality (flag 1) data. Around half of the data (42 %), with an ITC flag of 0, were also considered stationary (Stationarity flag 0), increasing to 82 % if we include a flag of 1 on both tests. The stationarity test is required for standard EC; thus the use of the latter increases the data amount by 34 % in the case that only high-quality observations are used and 55 % in the case that medium-quality data are included (Fig. 8). This saving happens more often during the day due to a higher coincidence of both flags during night. The percentages given are for the Li-Cor (IR) fast analyser but are of the same order of magnitude as for the Picarro analyser (CRDS).
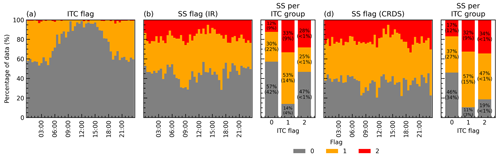
Figure 8Quality control flags for turbulence (ITC) and CO2 stationarity (SS). Flags follow a 0–1–2 system for high, medium, and low quality. Percentage of (a) turbulence flagged data by hour of the day. Stationarity flagged CO2 data by hour of the day for (b) the Li-Cor instrument (IR) and (d) the Picarro instrument (CRDS). Stationarity flags per ITC group are also given for the IR (b) and CRDS instrument (c). Percentages are summed to 100 % in each group and over all data (in parentheses).
4.3 Comparison of CO2 flux between slow- and fast-response analysers
4.3.1 High-frequency spectral correction
The CRDS analysers showed significantly more high-frequency attenuation of the flux than IR analysers (Table 2 and Fig. 9), as expected, due to the much longer sampling tube of the CRDS analyser (115 m) than the IR analyser (0.7 m), as well as the slower CRDS acquisition frequency (∼ 3 s) compared to the IR (0.1 s). The difference was greater in (very) stable conditions, when higher frequencies contribute more to the flux, than in (very) unstable conditions (Fig. 9). In (very) unstable conditions, the contribution of low frequencies to the flux increased, as shown by the fact that none of the ogive levelled to 1 towards 30 min integration time (ogive slope > 0). Surprisingly CO (measured by CRDS) showed an atypical curve with stronger contribution from high frequencies, which after analysis was attributable to amplitude resolution from a signal with weak variance and a less sensitive instrument. This shape explains the higher cut-off frequency of the spectral corrections in comparison with CO2 and CH4 (Table 1).
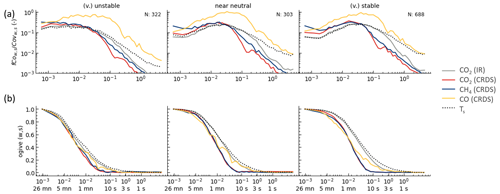
Figure 9Normalized cospectra (a) and ogives (b) of w and CO2, CH4, and CO covariances, for gases measured by IR and CRDS and the reference sonic temperature, Ts. Median values from July to October 2023 grouped by stability classes: ζ < −0.2 (unstable), −0.2 > ζ > 0.2 (near-neutral), and ζ > 0.2 (stable). N indicates the amount of half hourly data in each class.
Table 1The transfer function parameters for each instrument accounting for high-frequency attenuation. Here , where τc is the first-order filter's time constant. The cut-off frequency, fc, equals . Fn is a normalization factor. The optimized values correspond to optimizations as shown in Fig. 3. See Eqs. (5)–(9) and text for details.
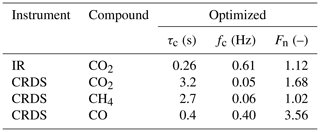
The high-frequency attenuation was around 2 %–3 % for the fast instrument (IR), while for the CRDS instruments sampling at 100 m, it ranged from 11 % to 19 % (Table 2). We can expect larger corrections in stable conditions, characterized by a larger contribution of high frequencies to the flux, as observed for IR; contrarily CRDS shows a decrease compared to near-neutral. It is worth noting that despite the 10 Hz acquisition frequency and 100 m height, the attenuation of the IR instrument was non-negligible. Additionally, the time response of the slow CRDS analysers, estimated based on the transfer function (3.62 s), matches the acquisition frequency (ranging between 3 and 4 s), but it also matches the expected attenuation for a long tube (Fig. 13).
Table 2Percentage of high-frequency corrections of the CO2, CH4, and CO fluxes per stability class for each instrument.
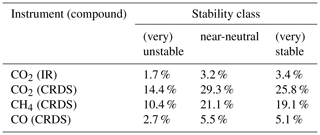
The median random uncertainty for CO2 was approximately 11 % using the fast setup (IR), comparable to the slow setup (CRDS). In contrast, uncertainties were higher for CH4 and CO, reaching 23 % and 36 % respectively. The higher uncertainty is expected due to smaller fluxes and lower instrument sensitivity.
4.3.2 Comparing CO2 flux measured by slow- and fast-response analysers
The CO2 fluxes computed from the IR (LI-7200) and the CRDS (Picarro) analysers were well correlated with an underestimation of 18 % of the CRDS for uncorrected fluxes that was diminished to 2 % after high-frequency corrections (Fig. 10). High-frequency correction decreased the bias, ME, by 0.18 and the mean absolute error (MAE) by 0.11 , with no effect on the correlation coefficient (R2). Moreover, there was a tendency of the CRDS corrected fluxes to slightly underestimate the CO2 fluxes under stable conditions (Fig. S3).
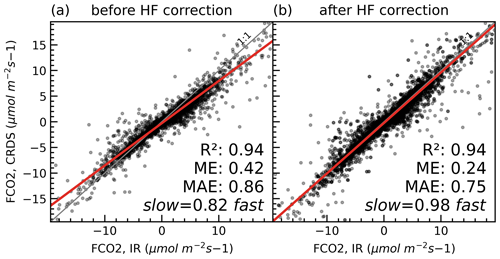
Figure 10Comparison of CO2 flux computed with the IR (LI-7200) and the CRDS (Picarro G2401) analysers, (a) before and (b) after high-frequency loss corrections. Dots are observations, red line is a robust linear relation and grey line is the 1:1 line. The correlation coefficient (R2), the mean error (ME, ), the mean absolute error (MAE, ). Statistics are calculated ignoring outliers from robust linear regression.
4.4 Turbulent flux dynamics
4.4.1 Diel pattern over the months
We observed a well-defined summer pattern for the CO2 flux, with emissions during the night and sequestration during the day (Fig. 11). From summer to winter, the sink shortens in time and decreases in magnitude up to the point that during winter, the site behaves on average as a source all along the day. We note that the concentration morning peak observed in Fig. 4 does not correspond to a peak in the flux. Following the seasonal pattern of CO2, satellite data show less green leaves from September on (Fig. S5).
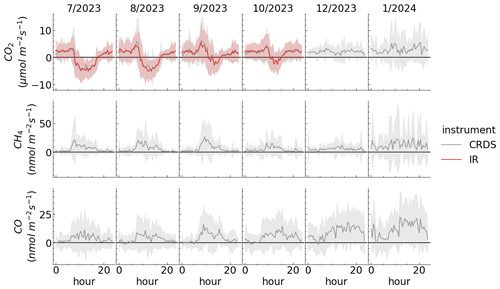
Figure 11Monthly mean turbulent flux diel pattern of CO2, CH4, and CO for IR (LI-7200, only CO2) and CRDS (Picarro G2401) gas analysers. Fluxes shown after spectral correction. Data points falling within the extreme 1 % tail of the distribution were removed. Shaded area refers to standard deviation.
4.4.2 Flux by wind direction
The CH4 fluxes showed a marked daily pattern from July to September, with higher emissions in the morning than in the afternoon, while daily emissions were overall higher in January. Looking at the CO flux, we see a marked increase in November and January but not in December, despite similar temperatures and traffic. In January, winds were relatively well distributed, while in December the most urban NE sector was rarely in the footprint, which may explain the difference between the 3 months.
Daytime and night-time turbulent fluxes reveal differences depending on wind direction and season (Fig. 12). During warmer months (January to October), the site was a daytime net sink of CO2 when the wind was coming from the forest in the west. Nocturnal wind coming from the SW (∼ 210°) was an important source. No sector was identified as a sink for CH4 or CO, and emissions were higher for both gases from eastern winds with a peak at around 40°.
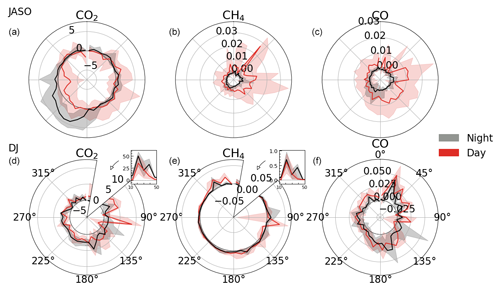
Figure 12CO2, CH4, and CO turbulent fluxes by wind direction. Warmer months (JASO) in panels (a), (b), and (c) and colder months (DJ) in panels (d), (e), and (f). The values are the median with the interquartile range in the shaded area; 0.1 % extreme values were removed. Wind direction bins with fewer than 10 observations were added to the next bin clockwise. CO2 fluxes are in , and fluxes of CH4 and CO are in nmol m−2 s−1. Note that 10–50° for CO2 and CH4 in December and January was plotted separately for visual purposes. See Fig. S4 for monthly values. Only stationary periods and those with well-developed turbulence are considered.
During colder months, daytime and night-time fluxes become similar. During these months when the leaves have fallen, the CO2 sink becomes a source. CO emissions in the northeast sector increase, possibly due to the alignment with the national road, N118. Additionally, the wind direction spanning 10–45°, the direction of the local heating plant, exhibited CO2 and CH4 emissions significantly higher than those observed in other directions, during both daytime and night-time periods. CO also increases at around 20° but with a much smaller magnitude.
5.1 Challenges of measuring on a tall tower with slow-response analysers
5.1.1 High-frequency loss corrections on the atmospheric tower configuration
Our findings revealed that an ICOS atmospheric tower configuration utilizing a CRDS gas analyser with an acquisition frequency of approximately 0.3 Hz and a tube length of 100 m exhibited a high-frequency loss correction of approximately 20 %. This correction was around 3 times more than the conventional ecosystem flux measurement setup, which employed an IR gas analyser with a 10 Hz acquisition frequency and a tube length of 0.7 m, positioned at the top of the tower. The observed transfer function (TF) for the CRDS setup closely matched the theoretical attenuation expected, as depicted in Fig. 13. Indeed, the tube and sensor attenuation together lead to a first-order time constant around 3 s, as we observed for the CRDS setup (Table 1). This outcome suggests that even with a faster measurement system or a smaller tube attenuation, only a limited reduction of the attenuation can be expected. In order to substantially decrease the high-frequency attenuation of the flux, both an increase of the acquisition frequency and a decrease in tube attenuation (decrease in tube length or increase in flow rate) would be required.
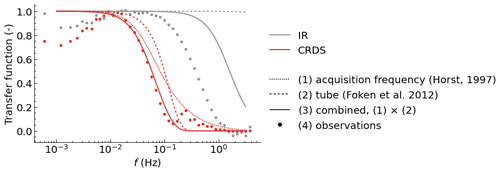
Figure 13Transfer functions computed for (1) the sensors' acquisition frequencies (TFacq, Horst, 1997), (2) the tube attenuation (TFtube, Leuning and Moncrieff, 1990; Foken et al., 2012), (3) the combination of (1) and (2), and (4) the CO2 observations, for the two setups at the tower. The Saclay atmospheric setup (CRDS) consists of a 100 m sampling line with a 9.5 mm diameter, a sampling frequency of ∼ 0.3 Hz, and a flow rate of 12.7 L min−1. The conventional ecosystem setup (IR) consists of a 0.7 m sampling line with a 5.33 mm diameter, a sampling frequency of 10 Hz, and a flow rate of 15 L min−1. Note that for the IR setup, the curves (1) and (3) are superposed.
We note that since the tube attenuation is higher when flow inside the tube is laminar (Lenschow and Raupach, 1991), ensuring a Reynolds number larger than ∼ 2300 is key to minimizing attenuation. We can define , where Re is the Reynolds number (–), r is the tube radius (m), Q is the volumetric flow rate (m3 s−1), and v is the kinematic viscosity of air. We find that for the tube in place with 9.5 mm of internal diameter, pumping ∼ 14–17 L min−1 is necessary to achieve a turbulent flow. Under these conditions, the cut-off frequency would increase to more than 0.6 Hz, but the pressure would also drop from −6 to −47 mbar.
We can also notice a high-frequency peak around 10−0.85 Hz (7 s) in the observations, which might come from resampling the CRDS concentrations to 10 Hz. The resampling by repeating the same value 30 times creates sharp corners in the time series, which might create noise and systematic problems in a Fourier transform. In the future, other high-frequency gap filling methods can be tried. However, problems related to the resampling happen at frequencies higher than setup attenuation and have limited effect on the transfer functions and corrections. It is worth mentioning that we used a first-order filter fitted on in situ data as a transfer function, following Fratini et al. (2012) and shared by other studies (Ibrom et al., 2007; Peltola et al., 2021). In the atmospheric tower configuration, where the main attenuation arises from the tube length, the transfer function may take an exponential shape, as proposed by Leuning and Moncrieff (1990) and Foken et al. (2012), and the fitting may not be perfect, as depicted in Fig. 13. The effect was however evaluated to be negligible on the correction factor.
For the same transfer function, attenuation may change based on the cospectra dependence on measuring height, wind speed, and stability parameter (). Specifically, increases in wind speed and stability parameter, or decreases in measuring height, are expected to shift a cospectrum towards higher frequencies, thereby enhancing attenuation for a given transfer function (Horst, 1997). Theoretical expectations of the attenuation factor from Horst et al. (1997) based on empirical cospectra agree very well with our measurements under unstable to near-neutral conditions but do not entirely align with our observations for neutral and stable conditions (Fig. 14). Indeed, surprisingly, we found that the attenuation remained stable or slightly decreased for values over 0.2 in the case of IR and CRDS respectively. This contrasts with the prediction by Horst et al. (1997), which suggested an increase by a factor of 5 under very stable conditions. This difference needs further investigation.
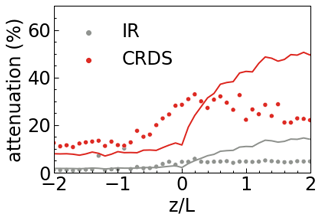
Figure 14Flux attenuation due to high-frequency losses, theoretical as lines and measured as points. The theoretical losses are computed from Horst (1997, Eq. 11), using the measured first-order time constant τc for the IR (0.5 s) and the CRDS (3 s).
More surprising is the HF losses found for CO2 measured by the IR (LI-7200). On this supposedly conventional ecosystem setup (0.7 m heated tube, 5.33 inner diameter, 15 L min−1 flow rate, 7 µm filter) attenuation and the transfer function were expected to be much smaller. The time constant of 0.5 s is equal to the cutting frequency (0.32 Hz) reported by Ibrom et al. (2007) for CO2 with 50 m long tube, 8 mm diameter, and a flow rate of 20 L min−1. We do not have strong evidence to explain this very large attenuation, and we are bound to speculate that this may be due to the inlet filter. Indeed, we observed a very large HF loss for H2O, with a τc ranging from 0.7 s for RH < 30 % to 5 s at 70 % RH and 50 s for RH larger than 80 % (data not shown). This is the sign that the inlet did accumulate water vapour, most probably on the filter holding hygroscopic aerosols. Since CO2 dissolves in water, the microscopic water accumulated in the tube may have buffered the CO2, leading to a large attenuation.
The CRDS setup, however, exhibited relatively small attenuation. In comparison, Wintjen et al. (2020) reported a damping factor of around 16 %–22 % for a 48 m tube with a 6 mm diameter, measuring reactive nitrogen at 10 Hz. Despite the longer tube length and slower analysers in our study compared to Wintjen et al. (2020), their slower flow rate (2.1 L min−1) and the expected stronger air–wall interactions for reactive nitrogen compounds may have contributed to the higher damping factor in their study. Correcting for high-frequency losses resulted in agreement between the IR and CRDS methods within 3 %, maintaining the elevated R2 of 0.94 (Fig. 10). This demonstrates that the high-frequency correction was able to correct for the losses.
We assumed all compounds (CO2, CH4, CO) measured by CRDS (Picarro G2401) suffered the same attenuation and used CO2, the best-defined curve for all three analysers (Table 1). This assumption is grounded on the fact that measurements are done by the same instrument at the same acquisition rate sampled through the same line and is backed by the proximity between CH4 and CO2 spectra, while the unexpected CO spectra can be explained by the CO signal noise due to the lower signal-to-noise ratio. Indeed, the noise was already larger than the signal at periods larger than 5 min (Fig. 3). Similarly, the small step increase around 4 s for the CRDS analysers corresponds to the actual measurement interval.
Furthermore, while this paper primarily examines the high-frequency aspect of the signal, it is important to note that the 30 min integration period did not allow the low frequencies of the fluxes to be captured entirely, especially under unstable conditions, as can be seen by the ogive slope being non-zero at the lowest frequencies (Fig. 9). A recent study on urban tall towers also reported low-frequency contribution for kinematic heat and CO2, indicating the importance of low-frequency corrections (Lan et al., 2024).
5.1.2 Storage and advection terms
The current paper focused on calculating turbulent flux using a setup dedicated to measure background concentration. To estimate the surface flux, storage and advection must be considered. The typical atmospheric setup also performs profile measurements, which can provide data to estimate both storage and vertical advection terms. The profile setup is not ideal since measurements at each height are not taken simultaneously, and the 20 min delay between measurements at each height can induce errors. A typical error can happen when for instance an urban plume rich in CO2 and CO is passing through the tower and both the asynchronism and delay in measurements can introduce artefacts in the fluxes. However, the estimations are still helpful and may give an order of magnitude and general patterns when aggregated.
The CO2 storage flux amplitude was of the same order of magnitude as the turbulent fluxes (Fig. 15). This agrees with observations in Haszpra et al. (2005) and means that the storage term is important when looking into the diel surface flux pattern. In particular we observe a strong emission during sunset not captured by the turbulent flux and a clear negative storage flux in the morning in July. A similar diel pattern can be seen in CH4 storage fluxes but not for CO. The early release in the case of CO2 may account for both the early onset of photosynthesis and an artefact from the expansion of the mixed layer (Fig. 7). The difference between this early negative storage flux between CO2 and CH4 may indicate the weight of these two causes, as CH4 is independent of photosynthesis. Atmospheric dilution results in both negative storage and positive vertical advection; however it is not a surface emission nor removal and cancels out if all conservation equation terms are considered.
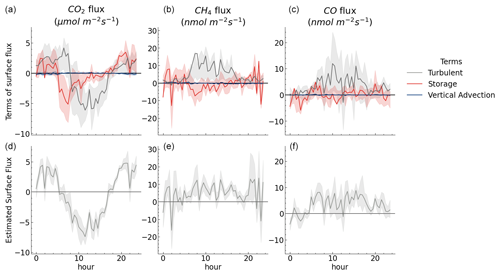
Figure 15Diel mean for CO2, CH4, and CO turbulent, storage, and vertical advection fluxes in panels (a), (b), and (c) and their sum, i.e. estimated surface flux, in panels (d), (e), and (f). Data for July 2023. Values for the CRDS (Picarro G2401). The band shows the interquartile (25th–75th percentiles). See Fig. S9 for all months.
Vertical advection depends on the mean vertical component of the wind (w) and thus on the quality of the tilt correction applied. On average, w was positive during unstable and near-neutral conditions ( < 0) and near zero for stable conditions (Fig. S8). For vertical advection, however, monthly averages were near zero for all measured gases (Figs. 15 and S9). Indeed, in the long term, vertical advection could be neglected for very tall towers based on the assumption that synoptic-scale processes should counterbalance vertical advection (Davis et al., 2003).
In this work horizontal advection was assumed negligible, but in reality, it may not be (Aubinet et al., 2005). Indeed, daily mean storage in the site gravitates around zero during July but shows a non-negligible variability for CO2 in August and September and for all gases in January (Fig. S6). In ideal conditions, the storage flux should average 0 over 24 h since what is stored at night should be de-stocked during the day. A non-zero daily storage may indicate advection.
Additionally, the chimney of the heating plant, ∼ 600 m away from the tower, situated at a certain height, may bias the storage fluxes, which rely on gradient profiles along the tower. Indeed, if the plume from the heating plant emissions is measured intermittently due to changes in wind directions, we may attribute or ignore storage fluxes where lateral advection is happening. Properly identifying such a process would require tracking the chimney plume with a 3D dispersion model and half-hourly resolution which was out of the scope of the present work.
5.2 Wind directional interpretations
Monitoring fluxes over a heterogeneous landscape demands spatial awareness. Turbulent fluxes were shown to vary depending on wind direction and season (Fig. 12). Notably the heating plant direction could be easily detected by the higher levels of CO2 and CH4 flux. Furthermore, winds originating from the deciduous forest (west) went from a daytime CO2 sink in warmer months to a source in winter when leaves had fallen.
Comparison with a mixed deciduous forest site at 50 km SE (FR-Fon ICOS site, e.g. Delpierre et al., 2016) shows a similar seasonality of the CO2 fluxes, storage terms being slightly larger in FR-Sac than in FR-Fon, and a turbulent term notably smaller in FR-Sac (Fig. S7). Differences may arise mainly from the proportion of the forest on the respective site's footprints. The forest represents around 40 % of the FR-Sac Western footprint and is expected to be higher during the day when unstable conditions shorten the footprint range. The remaining source area includes urban and traffic, which may add a positive component to the flux, offsetting the net flux during the day (Fig. 6). Although the footprint model does not account for variations in surface roughness, the results are still expected to remain valid despite this limitation.
Interpretations based on wind direction and comparisons with other sites serve the purpose of enlightening the potential of recovering spatially explicit fluxes from tower measurements. Nonetheless, accurate spatialized surface fluxes at finer scales remain a scientific challenge. The more complex discussions on relating these tall tower turbulence measurements to surface emissions and removals, as well as the intricate footprint analyses, should be further explored. Recent developments in the literature have shown promising results in addressing spatial variability. Indeed, research has been done on mapping fluxes from single-tower measurements by means of regressions (Crawford and Christen, 2015), Bayesian methods (Levy et al., 2020), or machine learning algorithms (Metzger et al., 2013; Metzger, 2018; Xu et al., 2018, 2020). For those efforts, capturing low-frequency fluxes as done in the “virtual control volume” in Metzger et al. (2018) could benefit the spatialization as they may be important, at least in urban tall towers (Lan et al., 2024). At the same time, maintaining a process-based approach could be advantageous for upscaling and understanding the ecophysiological processes in play. For flux attribution, a defensible partitioning method to decompose net fluxes under heterogeneous landscape could help map gases with considerable sources and sinks, such as CO2.
5.3 Recommendations for atmospheric sites concerned by such a method
As of now, the ICOS network comprises 47 atmospheric sites, with 39 labelled class 1 or 2 and the remainder as on track for labelling. The ecosystem centre is more extensive, encompassing 103 sites, including 77 labelled class 1 or 2, and the remaining associated sites. This count may further rise when other regional networks are taken into consideration.
Not all atmospheric sites are adapted for flux measurements. EC towers prioritize flat surfaces, slim towers, and homogeneity, whilst atmospheric towers may prioritize locations based on grid redundancy to improve atmospheric inversions. Slim towers with limited topography around them are recommended for reliable measurements. Atmospheric measurements conducted in close proximity to large structures (e.g. just above domes) or in mountainous regions can introduce disturbances in the turbulence signal. This can lead to unreliable tilt angle correction and surface flux assessments. In some cases, flagging wind sectors that are not appropriate for EC measurements can be a straightforward solution. With that said, eddy covariance has successfully been used in mountainous landscapes using appropriate tilt corrections (Matthews et al., 2017).
For atmospheric tower research candidates interested in measuring flux, we recommend the following:
-
Select at least one height for calculating fluxes through eddy covariance. For this decision, a footprint estimation (Kljun et al., 2015) may be relevant.
-
Include a high-frequency 3D anemometer on the chosen height(s).
-
Evaluate the first-order filter time for tube and sensors, as shown here, to verify that high-frequency attenuation is below an acceptable threshold, ∼ 20 %.
-
Perform continuous mixing ratio measurements on the chosen height(s), either limiting profile measurements to specific hours or with a separate set of instruments. This ensures that low-frequency signal for eddy covariance and profile measurements can prioritize transition periods when fluxes may exhibit non-stationary behaviour or low turbulence.
-
When performing profile measurements, alternate between levels every 5 min can improve the storage estimation.
-
Evaluate the flow regime in the sampling tube, if possible increasing flow rate to guarantee turbulence (Re > 2300).
-
Additional meteorological data (e.g. precipitation, short- and longwave incoming and outgoing radiation) and metadata (e.g. forest type, crops, transport counting) pertinent for flux interpretation should also be collected.
Note that adding a high-frequency 3D anemometer is enough for reanalysis. Ensuring continuous measurements is important for having a better-defined turbulent cospectrum and keeping low-frequency information. Certainly, profile measurements are often contemplated, and doubling the instruments may not be feasible. Therefore, we recommended restricting profile measurements to specific hours when the development of the boundary layer may overshadow the relevance of measuring flux close to the tower. These moments, typically during sunrise and sunset, provide valuable insights from both atmospheric and ecosystem (storage) perspectives. Furthermore, during these moments, standard covariance would typically flag and disregard measurements, as depicted in Fig. 8.
This study serves as a proof of concept for leveraging existing atmospheric towers to measure fluxes by simply adding a 3D anemometer. While eddy covariance on tall towers introduces challenges related to heterogeneity and storage effects, it mitigates concerns such as high-frequency attenuation. Comparing slow-response analysers with fast-response ones revealed very similar net fluxes across all stability conditions (R2 > 0.94), indicating the viability of using slower instruments in this case.
It is important to note that our results focus solely on passive gases, as water was not considered due to air drying before measurement in the CRDS. For water, we could expect greater attenuation linked to tube length, as air–wall interactions of absorption and desorption are much stronger in water vapour (Massman and Ibrom, 2008). Similarly, we would not recommend measuring reactive gases, as their residence time might be too long (∼ 60 s) for accurate eddy covariance measurements to be made.
While many of the variables affecting attenuation are not under the researcher's control, limited choices remain for measurement height, tube dimensions, flow rate, and acquisition frequency. Thus we recommend continuous gas measurements to be systematically done with a high-frequency 3D anemometer and a flow rate sufficiently large to ensure turbulent flows in the sampling tube.
To calculate the surface flux, we estimated the storage and vertical advection terms. Although the storage term calculated using three heights provided useful estimations, caution is warranted due to potential biases from not measuring height at the same time but also due to the limited number of heights sampled. Our results underscore the significance of the storage term, which was as large as the turbulent flux at the measurement height.
Analysing fluxes by wind direction revealed distinct patterns, particularly between the forest (W) and campus site (NE). Notably, emissions from a heating plant significantly influenced CO2 and CH4 fluxes in colder months, highlighting the importance of considering local sources. While our findings align with anticipated patterns across various land uses, accurately attributing fluxes to land uses would necessitate additional modelling efforts, which were beyond the scope of this study.
Overall, this study demonstrates the potential of expanding flux measurements through a relatively inexpensive instrumentation addition, offering valuable insights for both ecosystem and atmospheric research. It further shows that the eddy covariance method has sufficiently matured so that we can use less-than-ideal instrumentation.
The theoretical approach for high-frequency loss corrections requires defining a transfer function, TF, for each of the relevant origins of frequency losses, i, and multiplying them to find the total transfer function, TFtotal (Moore, 1986):
Note that TFs range between 0 and 1, and so the TFws is driven by the most restrictive function for each frequency. Considering only the attenuation from the air transport in the tube (TFtube, Leuning and Moncrieff, 1990; Foken et al., 2012) and acquisition rate (TFacq, Horst, 1997),
where Re is the Reynolds number (–); r is the tube radius (m); f is the frequency (Hz); L is the tube length (m); Q is the volumetric flow rate (m3 s−1); and τ is the first-order filter's time constant (s), where and facq is the acquisition frequency (Hz) for vertical wind speed or scalar. The Reynolds number is defined as , where v is the kinematic viscosity.
Attenuation also depends on the cospectra. A theoretical approach is proposed in Horst (1997, Eq. 11), where
where is the mean wind speed at height z; nmax is 0.085 in case < 0, else ; and α is for < 0, else 1.
Code used for resampling is available online and can be accessed at https://doi.org/10.5281/zenodo.13842150 (Herig Coimbra, 2024). The repository contains sample data.
ICOS data for FR-Sac can be downloaded from the carbon portal: https://data.icos-cp.eu/portal/ (ICOS data portal, 2024).
The supplement related to this article is available online at: https://doi.org/10.5194/amt-17-6625-2024-supplement.
PHHC, BL, and OL conceptualized the manuscript; PHHC, LB, and ML curated the data; PHHC and BL wrote the manuscript draft; and OL and MR reviewed and edited the manuscript.
The contact author has declared that none of the authors has any competing interests.
Publisher’s note: Copernicus Publications remains neutral with regard to jurisdictional claims made in the text, published maps, institutional affiliations, or any other geographical representation in this paper. While Copernicus Publications makes every effort to include appropriate place names, the final responsibility lies with the authors.
The authors would like to acknowledge the support of ICOS, ICOS France, and ICOS Atmosphere Thematic Centre. We thank Stefan Metzger and two anonymous reviewers for their valuable comments, which improved the manuscript's clarity and flow. We thank Rainer Hilland for the support in hosting the Saclay data and Daniel Berveiller and Nicolas Delpierre for maintaining the FR-Fon site. We also thank the SIRTA team, especially Simone Kotthaus, for comments on the lidar data. We acknowledge using artificial intelligence for language checks in earlier drafts of the manuscript.
This research has been supported by the EU Horizon 2020 (grant no. 101037319; PAUL ICOS-Cities project). The Environnement Ile De France doctoral school (ED129) of the University of Paris-Saclay provided Pedro Henrique Herig Coimbra's PhD grant.
This paper was edited by Christian Brümmer and reviewed by Stefan Metzger and two anonymous referees.
Asanuma, J., Tamagawa, I., Ishikawa, H., Ma, Y., Hayashi, T., Qi, Y., and Wang, J.: Spectral similarity between scalars at very low frequencies in the unstable atmospheric surface layer over the Tibetan plateau, Bound.-Lay. Meteorol., 122, 85–103, https://doi.org/10.1007/s10546-006-9096-y, 2007.
Aslan, T., Peltola, O., Ibrom, A., Nemitz, E., Rannik, Ü., and Mammarella, I.: The high-frequency response correction of eddy covariance fluxes – Part 2: An experimental approach for analysing noisy measurements of small fluxes, Atmos. Meas. Tech., 14, 5089–5106, https://doi.org/10.5194/amt-14-5089-2021, 2021.
Aubinet, M., Berbigier, P., Bernhofer, Ch., Cescatti, A., Feigenwinter, C., Granier, A., Grünwald, Th., Havrankova, K., Heinesch, B., Longdoz, B., Marcolla, B., Montagnani, L., and Sedlak, P.: Comparing CO2 Storage and Advection Conditions at Night at Different Carboeuroflux Sites, Bound.-Lay. Meteorol., 116, 63–93, https://doi.org/10.1007/s10546-004-7091-8, 2005.
Bergeron, O. and Strachan, I. B.: CO2 sources and sinks in urban and suburban areas of a northern mid-latitude city, Atmos. Environ., 45, 1564–1573, https://doi.org/10.1016/j.atmosenv.2010.12.043, 2011.
Canadell, J. G., Monteiro, P. M. S., Costa, M. H., Cotrim da Cunha, L., Cox, P. M., Eliseev, A. V., Henson, S., Ishii, M., Jaccard, S., Koven, C., Lohila, A., Patra, P. K., Piao, S., Rogelj, J., Syampungani, S., Zaehle, S., and Zickfeld, K.: Global Carbon and other Biogeochemical Cycles and Feedbacks, in: Climate Change 2021 – The Physical Science Basis: Working Group I Contribution to the Sixth Assessment Report of the Intergovernmental Panel on Climate Change, edited by: Masson-Delmotte, V., Zhai, P., Pirani, A., Connors, S. L., Péan, C., Berger, S., Caud, N., Chen, Y., Goldfarb, L., Gomis, M. I., Huang, M., Leitzell, K., Lonnoy, E., Matthews, J. B. R., Maycock, T. K., Waterfield, T., Yelekçi, O., Yu, R., and Zhou, B., Cambridge University Press, Chap. 5, 673–816, https://doi.org/10.1017/9781009157896.007, 2023.
Ciais, P., Rayner, P., Chevallier, F., Bousquet, P., Logan, M., Peylin, P., and Ramonet, M.: Atmospheric inversions for estimating CO2 fluxes: methods and perspectives, in: Greenhouse Gas Inventories: Dealing With Uncertainty, edited by: Jonas, M., Nahorski, Z., Nilsson, S., and Whiter, T., Springer Netherlands, Dordrecht, 69–92, https://doi.org/10.1007/978-94-007-1670-4_6, 2011.
Conrad, R.: Soil microorganisms as controllers of atmospheric trace gases (H2, CO, CH4, OCS, N2O, and NO), Microbiol. Rev., 60, 609–640, https://doi.org/10.1128/mr.60.4.609-640.1996, 1996.
Conrad, R. and Seiler, W.: Role of microorganisms in the consumption and production of atmospheric carbon monoxide by soil, Appl. Environ. Microb., 40, 437–445, https://doi.org/10.1128/aem.40.3.437-445.1980, 1980.
Crawford, B. and Christen, A.: Spatial source attribution of measured urban eddy covariance CO2 fluxes, Theor. Appl. Climatol., 119, 733–755, https://doi.org/10.1007/s00704-014-1124-0, 2015.
Davis, K. J., Bakwin, P. S., Yi, C., Berger, B. W., Zhao, C., Teclaw, R. M., and Isebrands, J. G.: The annual cycles of CO2 and H2O exchange over a northern mixed forest as observed from a very tall tower, Glob. Change Biol., 9, 1278–1293, https://doi.org/10.1046/j.1365-2486.2003.00672.x, 2023.
Delpierre, N., Berveiller, D., Granda, E., and Dufrêne, E.: Wood phenology, not carbon input, controls the interannual variability of wood growth in a temperate oak forest, New Phytol., 210, 459–470, https://doi.org/10.1111/nph.13771, 2016.
Delwiche, K. B., Knox, S. H., Malhotra, A., Fluet-Chouinard, E., McNicol, G., Feron, S., Ouyang, Z., Papale, D., Trotta, C., Canfora, E., Cheah, Y.-W., Christianson, D., Alberto, Ma. C. R., Alekseychik, P., Aurela, M., Baldocchi, D., Bansal, S., Billesbach, D. P., Bohrer, G., Bracho, R., Buchmann, N., Campbell, D. I., Celis, G., Chen, J., Chen, W., Chu, H., Dalmagro, H. J., Dengel, S., Desai, A. R., Detto, M., Dolman, H., Eichelmann, E., Euskirchen, E., Famulari, D., Fuchs, K., Goeckede, M., Gogo, S., Gondwe, M. J., Goodrich, J. P., Gottschalk, P., Graham, S. L., Heimann, M., Helbig, M., Helfter, C., Hemes, K. S., Hirano, T., Hollinger, D., Hörtnagl, L., Iwata, H., Jacotot, A., Jurasinski, G., Kang, M., Kasak, K., King, J., Klatt, J., Koebsch, F., Krauss, K. W., Lai, D. Y. F., Lohila, A., Mammarella, I., Belelli Marchesini, L., Manca, G., Matthes, J. H., Maximov, T., Merbold, L., Mitra, B., Morin, T. H., Nemitz, E., Nilsson, M. B., Niu, S., Oechel, W. C., Oikawa, P. Y., Ono, K., Peichl, M., Peltola, O., Reba, M. L., Richardson, A. D., Riley, W., Runkle, B. R. K., Ryu, Y., Sachs, T., Sakabe, A., Sanchez, C. R., Schuur, E. A., Schäfer, K. V. R., Sonnentag, O., Sparks, J. P., Stuart-Haëntjens, E., Sturtevant, C., Sullivan, R. C., Szutu, D. J., Thom, J. E., Torn, M. S., Tuittila, E.-S., Turner, J., Ueyama, M., Valach, A. C., Vargas, R., Varlagin, A., Vazquez-Lule, A., Verfaillie, J. G., Vesala, T., Vourlitis, G. L., Ward, E. J., Wille, C., Wohlfahrt, G., Wong, G. X., Zhang, Z., Zona, D., Windham-Myers, L., Poulter, B., and Jackson, R. B.: FLUXNET-CH4: a global, multi-ecosystem dataset and analysis of methane seasonality from freshwater wetlands, Earth Syst. Sci. Data, 13, 3607–3689, https://doi.org/10.5194/essd-13-3607-2021, 2021.
Dragicevic, P.: Fair Statistical Communication in HCI, in: Modern Statistical Methods for HCI, edited by: Robertson, J. and Kaptein, M., Springer International Publishing, Cham, 291–330, https://doi.org/10.1007/978-3-319-26633-6_13, 2016.
Dutaur, L. and Verchot, L. V.: A global inventory of the soil CH4 sink, Global Biogeochem. Cy., 21, GB4013, https://doi.org/10.1029/2006GB002734, 2007.
El Yazidi, A., Ramonet, M., Ciais, P., Broquet, G., Pison, I., Abbaris, A., Brunner, D., Conil, S., Delmotte, M., Gheusi, F., Guerin, F., Hazan, L., Kachroudi, N., Kouvarakis, G., Mihalopoulos, N., Rivier, L., and Serça, D.: Identification of spikes associated with local sources in continuous time series of atmospheric CO, CO2 and CH4, Atmos. Meas. Tech., 11, 1599–1614, https://doi.org/10.5194/amt-11-1599-2018, 2018.
Finnigan, J. J., Clement, R., Malhi, Y., Leuning, R., and Cleugh, H. A.: A re-evaluation of long-term flux measurement techniques. Part 1: Averaging and coordinate rotation, Bound.-Lay. Meteorol., 107, 1–48, https://doi.org/10.1023/A:1021554900225, 2003.
Foken, T. and Wichura, B.: Tools for quality assessment of surface-based flux measurements, Agr. Forest Meteorol., 78, 83–105, https://doi.org/10.1016/0168-1923(95)02248-1, 1996.
Foken, T., Aubinet, M., and Leuning, R.: The Eddy Covariance Method, in: Eddy Covariance: A Practical Guide to Measurement and Data Analysis, edited by: Aubinet, M., Vesala, T., and Papale, D., Springer Netherlands, Dordrecht, 1–19, https://doi.org/10.1007/978-94-007-2351-1_1, 2012.
Fowler, D., Hargreaves, K., Skiba, U., Milne, R., Zahniser, M., Moncrieff, J., Beverland, I., and Gallagher, M.: Measurements of CH4 and N2O Fluxes at the Landscape Scale Using Micrometeorological Methods, Philos. T. Roy. Soc.-A, 351, 339–355, https://doi.org/10.1098/rsta.1995.0038, 1995.
Fratini, G., Ibrom, A., Arriga, N., Burba, G., and Papale, D.: Relative humidity effects on water vapour fluxes measured with closed-path eddy-covariance systems with short sampling lines, Agr. Forest Meteorol., 165, 53–63, https://doi.org/10.1016/j.agrformet.2012.05.018, 2012.
Harris, C. R., Millman, K. J., van der Walt, S. J., Gommers, R., Virtanen, P., Cournapeau, D., Wieser, E., Taylor, J., Berg, S., Smith, N. J., Kern, R., Picus, M., Hoyer, S., van Kerkwijk, M. H., Brett, M., Haldane, A., del Río, J. F., Wiebe, M., Peterson, P., Gérard-Marchant, P., Sheppard, K., Reddy, T., Weckesser, W., Abbasi, H., Gohlke, C., and Oliphant, T. E.: Array programming with NumPy, Nature, 585, 357–362, https://doi.org/10.1038/s41586-020-2649-2, 2020.
Haszpra, L., Barcza, Z., Davis, K. J., and Tarczay, K.: Long-term tall tower carbon dioxide flux monitoring over an area of mixed vegetation, Agr. Forest Meteorol., 132, 58–77, https://doi.org/10.1016/j.agrformet.2005.07.002, 2005.
Hazan, L., Tarniewicz, J., Ramonet, M., Laurent, O., and Abbaris, A.: Automatic processing of atmospheric CO2 and CH4 mole fractions at the ICOS Atmosphere Thematic Centre, Atmos. Meas. Tech., 9, 4719–4736, https://doi.org/10.5194/amt-9-4719-2016, 2016.
Heiskanen, J., Brümmer, C., Buchmann, N., Calfapietra, C., Chen, H., Gielen, B., Gkritzalis, T., Hammer, S., Hartman, S., Herbst, M., Janssens, I. A., Jordan, A., Juurola, E., Karstens, U., Kasurinen, V., Kruijt, B., Lankreijer, H., Levin, I., Linderson, M.-L., Loustau, D., Merbold, L., Myhre, C. L., Papale, D., Pavelka, M., Pilegaard, K., Ramonet, M., Rebmann, C., Rinne, J., Rivier, L., Saltikoff, E., Sanders, R., Steinbacher, M., Steinhoff, T., Watson, A., Vermeulen, A. T., Vesala, T., Vítková, G., and Kutsch, W.: The Integrated Carbon Observation System in Europe, B. Am. Meteorol. Soc., 103, E855–E872, https://doi.org/10.1175/BAMS-D-19-0364.1, 2022.
Helfter, C., Tremper, A. H., Halios, C. H., Kotthaus, S., Bjorkegren, A., Grimmond, C. S. B., Barlow, J. F., and Nemitz, E.: Spatial and temporal variability of urban fluxes of methane, carbon monoxide and carbon dioxide above London, UK, Atmos. Chem. Phys., 16, 10543–10557, https://doi.org/10.5194/acp-16-10543-2016, 2016.
Herig Coimbra, P. H.: Resampling code with sample data for: Eddy-covariance with slow-response greenhouse gas analyser on tall towers, Version v1, Zenodo [code/data set], https://doi.org/10.5281/zenodo.13842150, 2024.
Horst, T. W.: A simple formula for attenuation of eddy fluxes measured with first-order-response scalar sensors, Bound.-Lay. Meteorol., 82, 219–233, https://doi.org/10.1023/A:1000229130034, 1997.
Ibrom, A., Dellwik, E., Flyvbjerg, H., Jensen, N. O., and Pilegaard, K.: Strong low-pass filtering effects on water vapour flux measurements with closed-path eddy correlation systems, Agr. Forest Meteorol., 147, 140–156, https://doi.org/10.1016/j.agrformet.2007.07.007, 2007.
ICOS data portal: https://data.icos-cp.eu/portal/ last access: 13 November 2024.
ICOS RI: ICOS Atmosphere Station Specifications V2.0, edited by: Laurent, O., ICOS ERIC, https://doi.org/10.18160/GK28-2188, 2020.
Inman, R. E., Ingersoll, R. B., and Levy, E. A.: Soil: a natural sink for carbon monoxide, Science, 172, 1229–1231, https://doi.org/10.1126/science.172.3989.1229, 1971.
IPCC: Climate Change 2021: The Physical Science Basis. Contribution of Working Group I to the Sixth Assessment Report of the Intergovernmental Panel on Climate Change, Cambridge University Press, in press, https://doi.org/10.1017/9781009157896, 2021.
IPCC: Climate Change 2022: Mitigation of Climate Change, Cambridge University Press, https://doi.org/10.1017/9781009157926, 2022.
Iwata, H., Nakazawa, K., Sato, H., Itoh, M., Miyabara, Y., Hirata, R., Takahashi, Y., Tokida, T., and Endo, R.: Temporal and spatial variations in methane emissions from the littoral zone of a shallow mid-latitude lake with steady methane bubble emission areas, Agr. Forest Meteorol., 295, 108184, https://doi.org/10.1016/j.agrformet.2020.108184, 2020.
Jia, G., Shevliakova, E., Artaxo, P., De Noblet-Ducoudré, N., Houghton, R., House, J., Kitajima, K., Lennard, C., Popp, A., Sirin, A., Sukumar, R., and Verchot, L.: Land–climate interactions, in: Climate Change and Land: an IPCC special report on climate change, desertification, land degradation, sustainable land management, food security, and greenhouse gas fluxes in terrestrial ecosystems, edited by: Shukla, P. R., Skea, J., Calvo Buendia, E., Masson-Delmotte, V., Pörtner, H.-O., Roberts, D. C., Zhai, P., Slade, R., Connors, S., van Diemen, R., Ferrat, M., Haughey, E., Luz, S., Neogi, S., Pathak, M., Petzold, J., Portugal Pereira, J., Vyas, P., Huntley, E., Kissick, K., Belkacemi, M., and Malley, J., Cambridge, Cambridge University Press, 131–248, https://doi.org/10.1017/9781009157988.004, 2022.
Kaimal, J. C. and Finnigan, J. J.: Atmospheric Boundary Layer Flows: Their Structure and Measurement, Oxford University Press, 304 pp., https://doi.org/10.1093/oso/9780195062397.001.0001, 1994.
Kljun, N., Calanca, P., Rotach, M. W., and Schmid, H. P.: A simple two-dimensional parameterisation for Flux Footprint Prediction (FFP), Geosci. Model Dev., 8, 3695–3713, https://doi.org/10.5194/gmd-8-3695-2015, 2015.
Kotthaus, S., Bravo-Aranda, J. A., Collaud Coen, M., Guerrero-Rascado, J. L., Costa, M. J., Cimini, D., O'Connor, E. J., Hervo, M., Alados-Arboledas, L., Jiménez-Portaz, M., Mona, L., Ruffieux, D., Illingworth, A., and Haeffelin, M.: Atmospheric boundary layer height from ground-based remote sensing: a review of capabilities and limitations, Atmos. Meas. Tech., 16, 433–479, https://doi.org/10.5194/amt-16-433-2023, 2023.
Lan, C., Mauder, M., Stagakis, S., Loubet, B., D'Onofrio, C., Metzger, S., Durden, D., and Herig-Coimbra, P.-H.: Intercomparison of eddy-covariance software for urban tall-tower sites, Atmos. Meas. Tech., 17, 2649–2669, https://doi.org/10.5194/amt-17-2649-2024, 2024.
Langford, B., Acton, W., Ammann, C., Valach, A., and Nemitz, E.: Eddy-covariance data with low signal-to-noise ratio: time-lag determination, uncertainties and limit of detection, Atmos. Meas. Tech., 8, 4197–4213, https://doi.org/10.5194/amt-8-4197-2015, 2015.
Lauvaux, T., Schuh, A. E., Uliasz, M., Richardson, S., Miles, N., Andrews, A. E., Sweeney, C., Diaz, L. I., Martins, D., Shepson, P. B., and Davis, K. J.: Constraining the CO2 budget of the corn belt: exploring uncertainties from the assumptions in a mesoscale inverse system, Atmos. Chem. Phys., 12, 337–354, https://doi.org/10.5194/acp-12-337-2012, 2012.
Lenschow, D. H. and Raupach, M. R.: The attenuation of fluctuations in scalar concentrations through sampling tubes, J. Geophys. Res.-Atmos., 96, 15259–15268, https://doi.org/10.1029/91JD01437, 1991.
Leuning, R. and Moncrieff, J.: Eddy-covariance CO2 flux measurements using open- and closed-path CO2 analysers: Corrections for analyser water vapour sensitivity and damping of fluctuations in air sampling tubes, Bound.-Lay. Meteorol., 53, 63–76, https://doi.org/10.1007/BF00122463, 1990.
Levy, P., Drewer, J., Jammet, M., Leeson, S., Friborg, T., Skiba, U., and van Oijen, M.: Inference of spatial heterogeneity in surface fluxes from eddy covariance data: A case study from a subarctic mire ecosystem, Agr. Forest Meteorol., 280, 107783, https://doi.org/10.1016/j.agrformet.2019.107783, 2020.
Mammarella, I., Kolari, P., Rinne, J., Keronen, P., Pumpanen, J., and Vesala, T.: Determining the contribution of vertical advection to the net ecosystem exchange at Hyytiälä forest, Finland, Tellus B, 59, 900–909, 2007.
Massman, W. J.: A simple method for estimating frequency response corrections for eddy covariance systems, Agr. Forest Meteorol., 104, 185–198, https://doi.org/10.1016/S0168-1923(00)00164-7, 2000.
Massman, W. J. and Ibrom, A.: Attenuation of concentration fluctuations of water vapor and other trace gases in turbulent tube flow, Atmos. Chem. Phys., 8, 6245–6259, https://doi.org/10.5194/acp-8-6245-2008, 2008.
Massman, W. J. and Lee, X.: Eddy covariance flux corrections and uncertainties in long-term studies of carbon and energy exchanges, Agr. Forest Meteorol., 113, 121–144, https://doi.org/10.1016/S0168-1923(02)00105-3, 2002.
Matthews, B., Mayer, M., Katzensteiner, K., Godbold, D. L., and Schume, H.: Turbulent energy and carbon dioxide exchange along an early-successional windthrow chronosequence in the European Alps, Agr. Forest Meteorol., 232, 576–594, https://doi.org/10.1016/j.agrformet.2016.10.011, 2017.
Mauder, M. and Foken, T.: Documentation and Instruction Manual of the Eddy-Covariance Software Package TK3, 60 pp., ISSN: 1614-8924, 2011.
Mauder, M., Cuntz, M., Drüe, C., Graf, A., Rebmann, C., Schmid, H. P., Schmidt, M., and Steinbrecher, R.: A strategy for quality and uncertainty assessment of long-term eddy-covariance measurements, Agr. Forest Meteorol., 169, 122–135, https://doi.org/10.1016/j.agrformet.2012.09.006, 2013.
Metzger, S.: Surface-atmosphere exchange in a box: Making the control volume a suitable representation for in-situ observations, Agr. Forest Meteorol., 255, 68–80, https://doi.org/10.1016/j.agrformet.2017.08.037, 2018.
Metzger, S., Junkermann, W., Mauder, M., Butterbach-Bahl, K., Trancón y Widemann, B., Neidl, F., Schäfer, K., Wieneke, S., Zheng, X. H., Schmid, H. P., and Foken, T.: Spatially explicit regionalization of airborne flux measurements using environmental response functions, Biogeosciences, 10, 2193–2217, https://doi.org/10.5194/bg-10-2193-2013, 2013.
Moncrieff, J. B., Malhi, Y., and Leuning, R.: The propagation of errors in long-term measurements of land-atmosphere fluxes of carbon and water, Glob. Change Biol., 2, 231–240, https://doi.org/10.1111/j.1365-2486.1996.tb00075.x, 1996.
Moore, C. J.: Frequency response corrections for eddy correlation systems, Bound.-Lay. Meteorol., 37, 17–35, https://doi.org/10.1007/BF00122754, 1986.
Nemitz, E., Mammarella, I., Ibrom, A., Aurela, M., Burba, G. G., Dengel, S., Gielen, B., Grelle, A., Heinesch, B., Herbst, M., Hörtnagl, L., Klemedtsson, L., Lindroth, A., Lohila, A., McDermitt, D. K., Meier, P., Merbold, L., Nelson, D., Nicolini, G., Nilsson, M. B., Peltola, O., Rinne, J., and Zahniser, M.: Standardisation of eddy-covariance flux measurements of methane and nitrous oxide, Int. Agrophys., 32, 517–549, https://doi.org/10.1515/intag-2017-0042, 2018.
Peltola, O., Aslan, T., Ibrom, A., Nemitz, E., Rannik, Ü., and Mammarella, I.: The high-frequency response correction of eddy covariance fluxes – Part 1: An experimental approach and its interdependence with the time-lag estimation, Atmos. Meas. Tech., 14, 5071–5088, https://doi.org/10.5194/amt-14-5071-2021, 2021.
Ramonet, M., Ciais, P., Apadula, F., Bartyzel, J., Bastos, A., Bergamaschi, P., Blanc, P. E., Brunner, D., Caracciolo di Torchiarolo, L., Calzolari, F., Chen, H., Chmura, L., Colomb, A., Conil, S., Cristofanelli, P., Cuevas, E., Curcoll, R., Delmotte, M., di Sarra, A., Emmenegger, L., Forster, G., Frumau, A., Gerbig, C., Gheusi, F., Hammer, S., Haszpra, L., Hatakka, J., Hazan, L., Heliasz, M., Henne, S., Hensen, A., Hermansen, O., Keronen, P., Kivi, R., Komínková, K., Kubistin, D., Laurent, O., Laurila, T., Lavric, J. V., Lehner, I., Lehtinen, K. E. J., Leskinen, A., Leuenberger, M., Levin, I., Lindauer, M., Lopez, M., Myhre, C. L., Mammarella, I., Manca, G., Manning, A., Marek, M. V., Marklund, P., Martin, D., Meinhardt, F., Mihalopoulos, N., Mölder, M., Morgui, J. A., Necki, J., O'Doherty, S., O'Dowd, C., Ottosson, M., Philippon, C., Piacentino, S., Pichon, J. M., Plass-Duelmer, C., Resovsky, A., Rivier, L., Rodó, X., Sha, M. K., Scheeren, H. A., Sferlazzo, D., Spain, T. G., Stanley, K. M., Steinbacher, M., Trisolino, P., Vermeulen, A., Vítková, G., Weyrauch, D., Xueref-Remy, I., Yala, K., and Yver Kwok, C.: The fingerprint of the summer 2018 drought in Europe on ground-based atmospheric CO2 measurements, Philos. T. Roy. Soc. B, 375, 20190513, https://doi.org/10.1098/rstb.2019.0513, 2020.
Saunois, M., Stavert, A. R., Poulter, B., Bousquet, P., Canadell, J. G., Jackson, R. B., Raymond, P. A., Dlugokencky, E. J., Houweling, S., Patra, P. K., Ciais, P., Arora, V. K., Bastviken, D., Bergamaschi, P., Blake, D. R., Brailsford, G., Bruhwiler, L., Carlson, K. M., Carrol, M., Castaldi, S., Chandra, N., Crevoisier, C., Crill, P. M., Covey, K., Curry, C. L., Etiope, G., Frankenberg, C., Gedney, N., Hegglin, M. I., Höglund-Isaksson, L., Hugelius, G., Ishizawa, M., Ito, A., Janssens-Maenhout, G., Jensen, K. M., Joos, F., Kleinen, T., Krummel, P. B., Langenfelds, R. L., Laruelle, G. G., Liu, L., Machida, T., Maksyutov, S., McDonald, K. C., McNorton, J., Miller, P. A., Melton, J. R., Morino, I., Müller, J., Murguia-Flores, F., Naik, V., Niwa, Y., Noce, S., O'Doherty, S., Parker, R. J., Peng, C., Peng, S., Peters, G. P., Prigent, C., Prinn, R., Ramonet, M., Regnier, P., Riley, W. J., Rosentreter, J. A., Segers, A., Simpson, I. J., Shi, H., Smith, S. J., Steele, L. P., Thornton, B. F., Tian, H., Tohjima, Y., Tubiello, F. N., Tsuruta, A., Viovy, N., Voulgarakis, A., Weber, T. S., van Weele, M., van der Werf, G. R., Weiss, R. F., Worthy, D., Wunch, D., Yin, Y., Yoshida, Y., Zhang, W., Zhang, Z., Zhao, Y., Zheng, B., Zhu, Q., Zhu, Q., and Zhuang, Q.: The Global Methane Budget 2000–2017, Earth Syst. Sci. Data, 12, 1561–1623, https://doi.org/10.5194/essd-12-1561-2020, 2020.
Thierion, V., Vincent, A., and Valero, S.: Theia OSO Land Cover Map 2021, Version 1, Zenodo [data set], https://doi.org/10.5281/zenodo.6538910, 2022.
Ueyama, M. and Takano, T.: A decade of CO2 flux measured by the eddy covariance method including the COVID-19 pandemic period in an urban center in Sakai, Japan, Environ. Pollut., 304, 119210, https://doi.org/10.1016/j.envpol.2022.119210, 2022.
Valentini, R., De Angelis, P., Matteucci, G., Monaco, R., Dore, S., and Mucnozza, G. E. S.: Seasonal net carbon dioxide exchange of a beech forest with the atmosphere, Glob. Change Biol., 2, 199–207, https://doi.org/10.1111/j.1365-2486.1996.tb00072.x, 1996.
Velasco, E., Pressley, S., Grivicke, R., Allwine, E., Coons, T., Foster, W., Jobson, B. T., Westberg, H., Ramos, R., Hernández, F., Molina, L. T., and Lamb, B.: Eddy covariance flux measurements of pollutant gases in urban Mexico City, Atmos. Chem. Phys., 9, 7325–7342, https://doi.org/10.5194/acp-9-7325-2009, 2009.
Virtanen, P., Gommers, R., Oliphant, T. E., Haberland, M., Reddy, T., Cournapeau, D., Burovski, E., Peterson, P., Weckesser, W., Bright, J., van der Walt, S. J., Brett, M., Wilson, J., Millman, K. J., Mayorov, N., Nelson, A. R. J., Jones, E., Kern, R., Larson, E., Carey, C. J., Polat, İ., Feng, Y., Moore, E. W., VanderPlas, J., Laxalde, D., Perktold, J., Cimrman, R., Henriksen, I., Quintero, E. A., Harris, C. R., Archibald, A. M., Ribeiro, A. H., Pedregosa, F., and van Mulbregt, P.: SciPy 1.0: fundamental algorithms for scientific computing in Python, Nat. Methods, 17, 261–272, https://doi.org/10.1038/s41592-019-0686-2, 2020.
Vuichard, N., Ciais, P., Viovy, N., Li, L., Ceschia, E., Wattenbach, M., Bernhofer, C., Emmel, C., Grünwald, T., Jans, W., Loubet, B., and Wu, X.: Simulating the net ecosystem CO2 exchange and its components over winter wheat cultivation sites across a large climate gradient in Europe using the ORCHIDEE-STICS generic model, Agr. Ecosyst. Environ., 226, 1–17, https://doi.org/10.1016/j.agee.2016.04.017, 2016.
Wilczak, J. M., Oncley, S. P., and Stage, S. A.: Sonic Anemometer Tilt Correction Algorithms, Bound.-Lay. Meteorol., 99, 127–150, https://doi.org/10.1023/A:1018966204465, 2001.
Winderlich, J., Gerbig, C., Kolle, O., and Heimann, M.: Inferences from CO2 and CH4 concentration profiles at the Zotino Tall Tower Observatory (ZOTTO) on regional summertime ecosystem fluxes, Biogeosciences, 11, 2055–2068, https://doi.org/10.5194/bg-11-2055-2014, 2014.
Wintjen, P., Ammann, C., Schrader, F., and Brümmer, C.: Correcting high-frequency losses of reactive nitrogen flux measurements, Atmos. Meas. Tech., 13, 2923–2948, https://doi.org/10.5194/amt-13-2923-2020, 2020.
Wohlfahrt, G., Hörtnagl, L., Hammerle, A., Graus, M., and Hansel, A.: Measuring eddy covariance fluxes of ozone with a slow-response analyser, Atmos. Environ., 43, 4570–4576, https://doi.org/10.1016/j.atmosenv.2009.06.031, 2009.
Xu, K., Metzger, S., and Desai, A. R.: Surface-atmosphere exchange in a box: Space-time resolved storage and net vertical fluxes from tower-based eddy covariance, Agr. Forest Meteorol., 255, 81–91, https://doi.org/10.1016/j.agrformet.2017.10.011, 2018.
Xu, K., Sühring, M., Metzger, S., Durden, D., and Desai, A. R.: Can data mining help eddy covariance see the landscape? A large-eddy simulation study, Bound.-Lay. Meteorol., 176, 85–103, https://doi.org/10.1007/s10546-020-00513-0, 2020.
Zheng, B., Chevallier, F., Yin, Y., Ciais, P., Fortems-Cheiney, A., Deeter, M. N., Parker, R. J., Wang, Y., Worden, H. M., and Zhao, Y.: Global atmospheric carbon monoxide budget 2000–2017 inferred from multi-species atmospheric inversions, Earth Syst. Sci. Data, 11, 1411–1436, https://doi.org/10.5194/essd-11-1411-2019, 2019.
- Abstract
- Introduction
- Requirements and constraints of slow- and fast-response analysers
- Material and methods
- Results
- Discussion
- Conclusions
- Appendix A
- Code availability
- Data availability
- Author contributions
- Competing interests
- Disclaimer
- Acknowledgements
- Financial support
- Review statement
- References
- Supplement
- Abstract
- Introduction
- Requirements and constraints of slow- and fast-response analysers
- Material and methods
- Results
- Discussion
- Conclusions
- Appendix A
- Code availability
- Data availability
- Author contributions
- Competing interests
- Disclaimer
- Acknowledgements
- Financial support
- Review statement
- References
- Supplement