the Creative Commons Attribution 4.0 License.
the Creative Commons Attribution 4.0 License.
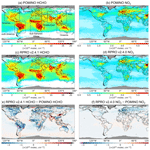
Global retrieval of TROPOMI tropospheric HCHO and NO2 columns with improved consistency based on the updated Peking University OMI NO2 algorithm
Yuhang Zhang
Isabelle De Smedt
Nicolas Theys
Michel Van Roozendael
Gaia Pinardi
Steven Compernolle
Ruijing Ni
Fangxuan Ren
Sijie Wang
Lulu Chen
Jos Van Geffen
Mengyao Liu
Alexander M. Cede
Martin Tiefengraber
Alexis Merlaud
Martina M. Friedrich
Andreas Richter
Ankie Piters
Vinod Kumar
Vinayak Sinha
Thomas Wagner
Yongjoo Choi
Hisahiro Takashima
Yugo Kanaya
Hitoshi Irie
Robert Spurr
Wenfu Sun
Lorenzo Fabris
The TROPOspheric Monitoring Instrument (TROPOMI), aboard the Sentinel-5 Precursor (S5P) satellite launched in October 2017, is dedicated to monitoring the atmospheric composition associated with air quality and climate change. This paper presents the global retrieval of TROPOMI tropospheric formaldehyde (HCHO) and nitrogen dioxide (NO2) vertical columns using an updated version of the Peking University OMI NO2 (POMINO) algorithm, which focuses on improving the calculation of air mass factors (AMFs). The algorithm features explicit corrections for the surface reflectance anisotropy and aerosol optical effects, and it uses daily high-resolution (0.25°×0.25°) a priori HCHO and NO2 profiles from the Global Earth Observing System Composition Forecast (GEOS-CF) dataset. For cloud correction, a consistent approach is used for both HCHO and NO2 retrievals, where (1) the cloud fraction is recalculated at 440 nm using the same ancillary parameters as those used in the NO2 AMF calculation, and (2) the cloud-top pressure is taken from the operational FRESCO-S cloud product.
The comparison between POMINO and reprocessed (RPRO) operational products in April, July and October 2021 as well as January 2022 exhibits high spatial agreement, but RPRO tropospheric HCHO and NO2 columns are lower by 10 % to 20 % over polluted regions. Sensitivity tests with POMINO show that the HCHO retrieval differences are mainly caused by different aerosol correction methods (implicit versus explicit), prior information on vertical profile shapes and background corrections, while the NO2 retrieval discrepancies result from different aerosol corrections, surface reflectances and a priori vertical profile shapes as well as their nonlinear interactions. With explicit aerosol corrections, the HCHO structural uncertainty due to the cloud correction using different cloud parameters is within ±20 %, mainly caused by cloud height differences. Validation against ground-based measurements from global Multi-Axis Differential Optical Absorption Spectroscopy (MAX-DOAS) observations and the Pandonia Global Network (PGN) shows that in April, July and October 2021 as well as in January 2022 POMINO retrievals present a comparable day-to-day correlation but a reduced bias (normalized mean bias, NMB) compared to the RPRO products (HCHO: R=0.62, versus R=0.68, ; NO2: R=0.84, versus R=0.85, ). An improved agreement of the ratio (FNR, formaldehyde to nitrogen dioxide ratio) with MAX-DOAS and PGN measurements based on POMINO retrievals is also found (NMB: −14.8 % versus −21.1 %). Our POMINO retrieval provides a useful source of information, particularly for studies combining HCHO and NO2.
- Article
(21468 KB) - Full-text XML
-
Supplement
(4110 KB) - BibTeX
- EndNote
Formaldehyde (HCHO) and nitrogen dioxide (NO2) are important trace gases in the troposphere. They play a critical role in the processes of tropospheric ozone (O3) and aerosol formation, and they have significant influences on air quality, climate and human health (Beelen et al., 2014; Crutzen, 1970; Shindell et al., 2009). Methods to retrieve tropospheric HCHO and NO2 vertical column densities (VCDs) in the ultraviolet (UV) and visible (VIS) spectral ranges, respectively, have rapidly developed in the last decades, based on sensors mounted on both sun-synchronous and geostationary satellites such as the Global Ozone Monitoring Experiment (GOME; Burrows et al., 1999), SCanning Imaging Absorption spectroMeter for Atmospheric CHartographY (SCIAMACHY; Bovensmann et al., 1999), Ozone Monitoring Instrument (OMI; Levelt et al., 2006), Global Ozone Monitoring Experiment-2 (GOME-2; Callies et al., 2000), Ozone Mapping and Profiling Suite Nadir Mapper (OMPS-NM; Dittman et al., 2002), TROPOspheric Monitoring Instrument (TROPOMI; Veefkind et al., 2012), Environmental Trace Gases Monitoring Instrument (EMI; Zhang et al., 2020), Geostationary Environment Monitoring Spectrometer (GEMS; Kim et al., 2020) and Tropospheric Emissions: Monitoring of Pollution (TEMPO; Zoogman et al., 2017). Such satellite observations have been extensively used in studies related to long-term trends and variabilities (De Smedt et al., 2010; Jiang et al., 2022; Richter et al., 2005), estimation of surface-level concentrations (Cooper et al., 2022; Wei et al., 2022), constraining emissions of non-methane volatile organic compounds (NMVOCs) and nitrogen oxides () (Kong et al., 2022; Lin, 2012; Stavrakou et al., 2018), nonlinear ozone chemistry (Jin et al., 2017, 2023; Jin and Holloway, 2015), and impacts on the environment and human health (Chen et al., 2022; Li et al., 2023).
The retrieval algorithms of tropospheric HCHO and NO2 VCDs based on observations from spaceborne instruments share many retrieval concepts. First, the slant column density (SCD), representing the trace gas concentration integrated along the average light path, is obtained by performing a spectral fit from backscattered radiance and irradiance spectra. Second, the SCD is converted to a VCD using air mass factors (AMFs) obtained from radiative transfer (RT) calculations, which are a function of the observation geometry, cloud information, aerosol properties, surface conditions and the shape of a priori vertical profiles. The main intrinsic differences between HCHO and NO2 retrievals are that (1) different wavelength ranges are used for each retrieval, and (2) the final tropospheric HCHO VCDs are determined with an additional background correction based on modeled HCHO columns in the reference region in the field of regard (FOR) of satellite instruments, while for NO2 a stratosphere–troposphere separation is performed in order to obtain tropospheric columns.
Many studies have focused on improving or developing retrieval algorithms to generate scientific HCHO or NO2 products for comparison with operational products and for applications. For example, Liu et al. (2021) presented an improved tropospheric NO2 retrieval algorithm from TROPOMI measurements over Europe, which employs a new stratosphere–troposphere separation and updated auxiliary parameters, including a more realistic cloud treatment, for air mass factor (AMF) calculation. Over East Asia, Liu et al. (2020) released a new TROPOMI product for tropospheric NO2 columns that features explicit aerosol corrections in the AMF calculation, and Su et al. (2020) improved the TROPOMI tropospheric HCHO retrieval by optimizing the spectral fitting and optimizing the AMF calculation by using a priori profiles from a higher-resolution regional chemistry transport model.
However, little attention has been paid to fixing the systematic differences in ancillary parameters between HCHO and NO2 AMF calculations. For instance, the TROPOMI reprocessed (RPRO) HCHO version 2.4.1 and NO2 version 2.4.0 operational products make use of cloud information from different sources: the Optical Cloud Recognition Algorithm and Retrieval of Cloud Information using Neural Networks (OCRA/ROCINN) – the Cloud as Reflecting Boundaries (CRB) product is used for HCHO, while the Fast Retrieval Scheme for Clouds from the Oxygen Absorptions for Sentinels (FRESCO-S) product is used for NO2. Besides, the surface albedo used in the current HCHO retrieval is the OMI-based monthly minimum Lambertian-equivalent reflectivity (MLER) at 340 nm with a spatial resolution of 0.5°×0.5° (lat.×long.), whereas the one used in the NO2 retrieval has been updated with the KNMI TROPOMI directionally dependent Lambertian-equivalent reflectivity (DLER) v1.0 database at 440 nm with a spatial resolution of 0.125°×0.125°. Finally, the radiative transfer model used for HCHO AMF calculation is the linearized pseudo-spherical scalar and vector discrete ordinate radiative transfer code (VLIDORT) version 2.6, whereas that used for NO2 AMF calculation is the Double-Adding KNMI (DAK) polarized radiative transfer code version 3.2. Such inconsistencies are an important limitation for studies combining satellite HCHO and NO2 products, such as analysis of ozone chemistry and wildfires (Jin et al., 2020, 2023). Therefore, there is a need for consistent retrievals of tropospheric HCHO and NO2 VCDs. Moreover, the TROPOMI operational HCHO and NO2 products do not explicitly account for the optical effect of aerosols, but they use a priori profile shapes from the massively parallel version of the Tracer Model 5 (TM5-MP; Williams et al., 2017) with a relatively coarse spatial resolution (1°×1°).
The Peking University OMI NO2 (POMINO) algorithm offers a potential tool to address these limitations. Founded by Lin et al. (2014), POMINO has been continuously developed and applied to the OMI, TROPOMI and GEMS instruments (Lin et al., 2014, 2015; Liu et al., 2019, 2020; Zhang et al., 2023). POMINO features an explicit treatment of aerosol optical effects and surface reflectance anisotropy, as well as a recalculation of cloud information using ancillary parameters consistent with those used for NO2 AMF calculation. A smaller bias of POMINO NO2 data than the operational products has been reported from validation against independent ground-based measurements (Liu et al., 2019, 2020; Zhang et al., 2023). However, the previous POMINO-TROPOMI algorithm was limited to Asia, and its potential for HCHO retrieval remained unexplored.
In this paper, we present the global retrieval of TROPOMI tropospheric HCHO and NO2 VCDs with much improved consistency, based on an updated version of the POMINO algorithm. After describing the methods and data in Sect. 2, we present the quantitative comparison of tropospheric HCHO and NO2 columns between POMINO and RPRO products (Sect. 3). We then discuss the structural uncertainty of HCHO and NO2 retrievals based on the POMINO algorithm, by conducting a series of sensitivity tests on cloud correction, aerosol correction, surface reflectance and a priori profile shapes (Sect. 4). Tentative estimates of POMINO retrieval uncertainty are given in Sect. 5. Finally, we use independent ground-based measurements from a global network of Multi-Axis Differential Optical Absorption Spectroscopy (MAX-DOAS) instruments and the Pandonia Global Network (PGN) to validate the tropospheric HCHO and NO2 columns from the POMINO and RPRO products (Sect. 6).
2.1 TROPOMI instrument and operational algorithms for HCHO and NO2 retrieval
TROPOMI is an imaging spectrometer aboard the European Space Agency (ESA) Copernicus Sentinel-5 Precursor (S5P) satellite launched on 13 October 2017, crossing the Equator at around 13:30 local time (LT) (Veefkind et al., 2012). Its wide spectral range includes the ultraviolet (UV), visible (VIS), near-infrared (NIR) and shortwave infrared (SWIR), allowing for monitoring of atmospheric trace gases, aerosols, clouds and surface properties. The original spatial resolution of about 7 km×3.5 km (along-track × across-track) at nadir was refined to about 5.5 km×3.5 km on the 6 August 2019 by means of a reduction of the along-track integration time. The wide swath of about 2600 km in the across-track direction enables global coverage on a daily basis, except for narrow strips between orbits of about 0.5° wide at the Equator.
The TROPOMI operational HCHO and NO2 retrieval algorithms have been fully described in De Smedt (2022) and Van Geffen et al. (2022b), respectively. The first common step is to derive slant columns by performing a spectral fit using the Differential Optical Absorption Spectroscopy (DOAS) method. Specifics for the SCD retrieval are provided in Table S1 in the Supplement. After the DOAS spectral fitting, a two-step normalization of the HCHO slant columns is performed to remove any remaining global offset and possible stripes. Then the corrected differential SCDs (dSCDs) are converted to vertical columns using AMFs at 340 nm. The AMFs are derived from a precalculated look-up table (LUT) storing altitude-dependent AMFs calculated with the VLIDORT v2.6 radiative transfer model. This approach implements implicit aerosol corrections by assuming that aerosols can be simply treated as “effective clouds”, and it uses the OMI-based monthly MLER dataset for surface reflectance. The HCHO vertical profile shape is specified from TM5-MP daily analyses. For pixels with partly cloudy scenes, a cloud correction is applied based on the independent pixel approximation (IPA) (Martin et al., 2002), using cloud fraction (CF), cloud-top pressure (CP) and cloud albedo information from the OCRA/ROCINN-CRB product:
In Eq. (1), w is the cloud radiance fraction (CRF), Mcld the cloudy-sky AMF and Mclr the clear-sky AMF. In the OCRA/ROCINN-CRB cloud retrieval, OCRA first computes the cloud fraction using a broadband UV–VIS color-space approach with two colors: green (405–495 nm) and blue (350–395 nm); then ROCINN-CRB calculates the cloud height and cloud albedo using data in and around the oxygen (O2) A-band (∼760 nm). In the final step, TM5-MP HCHO vertical columns in the reference region are added as compensation for the background HCHO from methane (CH4) oxidation in the equatorial Pacific. The final tropospheric HCHO VCD, NV, can be written as follows:
with () being the corrected HCHO differential slant column; M the HCHO AMF; Mclear,0 the HCHO clear-sky AMF in the reference region (90° S, 90° N), (180° W, 120° W); and the HCHO vertical column from a daily latitude-dependent polynomial, which is fitted through 5° latitude bin means of TM5-MP HCHO vertical columns in the reference region (De Smedt, 2022).
For NO2, a destriping is also applied to the fitted slant columns even though the systematic across-track features are very small (Van Geffen et al., 2020). The second step is the stratosphere–troposphere separation, where TM5-MP is used to assimilate TROPOMI total NO2 SCDs, determine the stratospheric NO2 SCDs and (by subtraction) infer the tropospheric NO2 SCDs. To calculate tropospheric NO2 AMFs, the operational algorithm applies implicit aerosol corrections, uses NO2 a priori profile shapes from TM5-MP daily analyses and adopts a DLER at 440 nm from the KNMI TROPOMI DLER v1.0 surface reflectance database. For the cloud correction, it takes the cloud-top pressure from the FRESCO-S product (using the O2 A-band at ∼760 nm) and retrieves an effective cloud fraction (ECF) by fitting the observed continuum reflectance to a simulated reflectance at 440 nm, assuming an optically thick Lambertian cloud with a fixed cloud albedo of 0.8. The tropospheric NO2 VCD, , can be written as follows:
with () the tropospheric NO2 slant column and M the tropospheric NO2 AMF.
2.2 Improved POMINO-TROPOMI algorithm for global HCHO and NO2 AMF calculations
Focusing on the improvement of global HCHO and NO2 AMF calculations as well as their consistency, we use an updated POMINO-TROPOMI parallelized AMFv6 package (Fig. S1 in the Supplement) driven by the LInearized Discrete Ordinate Radiative Transfer code (LIDORT) version 3.6 inherited from previous POMINO products (Liu et al., 2020). The DOAS spectral fit, HCHO dSCD background correction and NO2 stratosphere–troposphere separation are not included in this study, so corrected HCHO dSCDs and tropospheric NO2 SCDs are directly taken from the RPRO HCHO v2.4.1 product and RPRO NO2 v2.4.0 product, respectively. Compared to the previous HCHO v2.3.0 processor, the HCHO v2.4.1 processor uses new and improved Level 1b v2.1.0 data products as input, and it has been applied for a full mission reprocessing starting from 7 May 2018. For NO2, the improvements to the v2.4.0 processor include the use of a DLER climatology derived from TROPOMI observations and new and improved Level 1b v2.1.0 data products as input, which have also been used for a full mission reprocessing from 1 May 2018. Detailed information on the S5P TROPOMI L2 HCHO and NO2 processing baseline, including the processor version, in-operation period and relevant improvements, can be found at https://sentiwiki.copernicus.eu/web/s5p-processing (last access: 31 March 2025).
Table 1 lists the main improvements in the POMINO AMF algorithm compared to the RPRO algorithms. POMINO calculates the AMFs with online pixel-by-pixel RT simulations rather than using the LUT. Explicit aerosol corrections are implemented at the corresponding wavelengths of HCHO and NO2, respectively, based on the aerosol information from Global Earth Observing System Composition Forecast (GEOS-CF; Keller et al., 2021) v1.0 and Moderate Resolution Imaging Spectroradiometer (MODIS) satellite data. We convert GEOS-CF vertical volume mixing ratio profiles to optical depth profiles for each aerosol type, i.e., dust, sulfate–nitrate–ammonium (SNA), organic carbon (OC), black carbon (BC) and sea salt, by using high-spectral-resolution aerosol optical parameters from the GEOS-Chem website (http://ftp.as.harvard.edu/gcgrid/data/aerosol_optics/hi_spectral_res/v9-02/, last access: 23 July 2024). We then convert component-specific aerosol information to vertical profiles of aerosol extinction coefficient, single scattering albedo and phase function. We further use monthly aerosol optical depth (AOD) data from the MODIS/Aqua Collection 6.1 MYD04_L2 dataset, with spatial and temporal interpolation for missing values, to constrain the model AOD (Lin et al., 2014). Daily a priori HCHO and NO2 profile shapes at TROPOMI overpass times are also obtained from GEOS-CF v1.0 at the spatial resolution of 0.25°×0.25°. A detailed comparison of the specifications between GEOS-CF and TM5-MP is provided in Table S2 in the Supplement.
For NO2 AMF calculations, to account for the surface reflectance anisotropy over land and coastal ocean regions, we use bidirectional reflectance distribution function (BRDF) coefficients around 470 nm (band 3; bandwidth: 459–479 nm) from the MODIS MCD43C2.061 dataset. The reason for the choice of MODIS BRDF over KNMI TROPOMI DLER is that the operational MODIS BRDF algorithm fully characterizes the dependence of surface reflectance on the solar zenith angle (SZA), viewing zenith angle (VZA) and relative azimuth angle (RAA) by a linear combination of an isotropic parameter plus the volumetric and geometric scattering kernels (Roujean et al., 1992; Zhou et al., 2010), while the DLER model only considers the satellite viewing angle (Tilstra et al., 2024). For HCHO, given that the UV spectral band is not included in the MODIS instrument, we decided to use the climatological DLER at 340 nm from the KNMI TROPOMI DLER v2.0 database.
To allow for a consistent cloud correction, we use the same cloud information for both HCHO and NO2 AMF calculations. For each pixel, we acquire the cloud parameters by (1) taking the cloud-top pressure from the FRESCO-S cloud product and (2) recalculating the cloud fraction at 440 nm in a similar way as used in the operational NO2 algorithm. To simulate the top-of-atmosphere (TOA) reflectance at 440 nm to derive cloud fraction, we use the ancillary parameters that are fully consistent with those used in the NO2 AMF calculation, i.e., a surface reflectance derived from MODIS BRDF coefficients and explicit aerosol information. Previous studies have demonstrated that, in most cases, explicit aerosol corrections lead to reduced cloud (radiance) fractions, especially over regions with heavy aerosol loads such as the North China Plain in winter (Lin et al., 2015), while over regions where frequent aerosol-cloud overlap occurs such as southeast China in spring, the explicit corrections for absorbing aerosols overlying the cloud deck lead to increased cloud fraction (Jethva et al., 2018). Such differences are because the optical effects of aerosols are separated from those of clouds.
Based on the POMINO structure, we implemented a series of sensitivity tests to assess the importance of structural uncertainties that arise when different ancillary parameters or methodologies are applied to the same data. For HCHO, we first conducted the Fst_ORcp test (Case F1) by (1) recalculating the cloud fraction at 340 nm based on the reflectance derived using the TROPOMI L1B radiance dataset version 2.1 in TROPOMI spectral band 3 (305–400 nm) and irradiance dataset version 2.1 for the ultra-violet, visible, and near-infrared (UVN) module post-processed by BIRA-IASB and (2) using the cloud-top pressure from the OCRA/ROCINN-CRB product. Therefore, the differences between POMINO HCHO columns (Case F0) and those of the Fst_ORcp test represent the structural uncertainty from the cloud correction using different cloud products. Based on the Fst_ORcp test, we separately evaluated the effect of aerosol correction, surface reflectance and a priori profile shapes by conducting the Fst_imaer (Case F2), Fst_mler (Case F3) and Fst_tm5 (Case F4) tests, respectively. Note that in all sensitivity tests, only HCHO AMFs are changed accordingly, while we keep using GEOS-CF HCHO columns for background correction.
Similarly, for NO2 AMF calculations, based on POMINO NO2 retrievals as the reference (Case N0), Nst_imaer (Case N1), Nst_dler (Case N2) and Nst_tm5 (Case N3) tests were used to quantify the individual effects of aerosol correction, surface reflectance and a priori profile shapes. However, we noticed that the NO2 differences between POMINO and RPRO products can hardly be explained by the linear combination of the individual effects of each ancillary parameter as in the HCHO analysis. Therefore, we further conducted an additional Nst_joint (Case N4) test to mimic the AMF calculation in the RPRO algorithm, quantifying the joint effects of implicit aerosol corrections, KNMI TROPOMI DLER and TM5-MP a priori NO2 profile shapes.
Table 1Comparison of ancillary parameters between POMINO and RPRO operational products and sensitivity tests on the corresponding ancillary parameters (S.A.P. means same as POMINO).
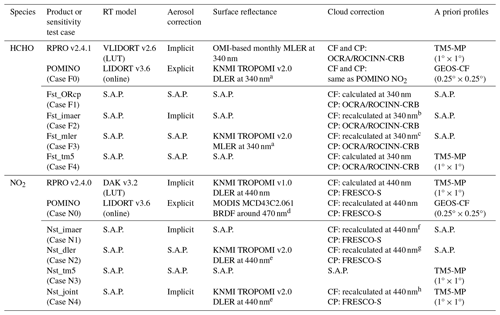
a KNMI TROPOMI v2.0 DLER at 340 nm over land and coastal ocean regions, as well as MLER at 340 nm over open ocean.
b Fst_imaer (Case F2) cloud fraction is recalculated with implicit aerosol corrections and is different from that of Case F1.
c Fst_mler (Case F3) cloud fraction is recalculated with KNMI TROPOMI v2.0 MLER and is different from that of Case F1.
d MODIS MCD43C2.061 BRDF around 470 nm over land and coastal ocean regions, as well as KNMI TROPOMI v2.0 MLER at 440 nm over open ocean.
e KNMI TROPOMI v2.0 DLER at 440 nm over land and coastal ocean regions, as well as MLER at 440 nm over open ocean.
f Nst_imaer (Case N1) cloud fraction is recalculated with implicit aerosol corrections and is different from that of Case N0.
g Nst_dler (Case N2) cloud fraction is recalculated with KNMI TROPOMI v2.0 DLER and is different from that of Case N0.
h Nst_joint (Case N4) cloud fraction is recalculated with implicit aerosol corrections and KNMI TROPOMI v2.0 DLER and is different from that of Case N0.
2.3 Ground-based MAX-DOAS datasets
Ground-based MAX-DOAS instruments can provide vertical columns and profiles of trace gases from the surface up to the lower free troposphere (around 4 km). The measurement sensitivity is the highest near the surface and decreases at higher altitudes. Information on ground-based MAX-DOAS measurements used in this study is summarized in Table 2 with locations specified in Fig. S2 in the Supplement. For each site, we use Fiducial Reference Measurements for Ground-based DOAS Air-Quality Observations (FRM4DOAS; https://frm4doas.aeronomie.be/, last access: 31 March 2025, Van Roozendael et al., 2024) version 01.01 harmonized HCHO and NO2 data if available; otherwise, we use data generated by principal investigators of each instrument using non-harmonized retrieval settings. The aim of the FRM4DOAS project is to minimize inhomogeneities in the current MAX-DOAS network to provide reference datasets for satellite data validation. So far, many MAX-DOAS sites have been used for validation (De Smedt et al., 2021; Pinardi et al., 2020; Verhoelst et al., 2021; Yombo Phaka et al., 2023), but this is the starting point of the FRM4DOAS project, and many more sites will join the centralized processing facility.
According to previous studies, the total estimated uncertainty of ground-based MAX-DOAS measurements in polluted conditions is about 30 % for HCHO and NO2 VCDs (De Smedt et al., 2021; Verhoelst et al., 2021). The mean bias is due mainly to systematic uncertainties related to AMF calculations. The uncertainty may also vary when different report strategies are used. Routine validation results show an overall bias of −37 % for HCHO and −28 % for NO2 in the operational TROPOMI products compared to MAX-DOAS measurements in the validation report (available at https://mpc-vdaf.tropomi.eu/, last access: 31 March 2025).
Table 2MAX-DOAS datasets used for the validation. The sites are listed in alphabetical order based on the first letter of the site name.
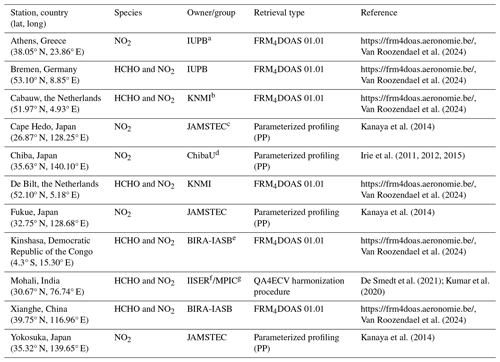
a Institute of Environmental Physics, University of Bremen.
b Royal Netherlands Meteorological Institute.
c Japan Agency for Marine-Earth Science and Technology.
d Chiba University.
e Royal Belgian Institute for Space Aeronomy.
f Indian Institute of Science Education and Research.
g Max Planck Institute for Chemistry.
2.4 PGN/Pandora datasets
The Pandonia Global Network (PGN) is a large-scale global network providing ground-based observations of multiple atmospheric reactive trace gases, including HCHO and NO2, and associated uncertainty values for satellite validation and other scientific activities. It is based on ground-based passive spectrometer systems called Pandora that can perform sun, moon and sky observations. The datasets have been widely used to validate HCHO and NO2 measurements from satellite instruments and field campaigns (Herman et al., 2019; Kai-Sikhakhane et al., 2024; Li et al., 2021; Liu et al., 2024a; Verhoelst et al., 2021).
Herman et al. (2009) reported that the nominal estimated uncertainty of total NO2 columns is for the random part and for the systematic part, and an uncertainty of 20 % is reported by comparisons with in situ measurements (Verhoelst et al., 2021). However, the newer PGN NO2 rnvs3p1-8 data, which are employed in this study, have considerably lower uncertainties due to changes in (1) the optical setup, (2) the gas-calibration approach and (3) a more accurate NO2 effective temperature estimation. As reported in the PGN data products README file (https://publications.pandonia-global-network.org/manuals/PGN_DataProducts_Readme.pdf, last access: 31 March 2025), the combined uncertainty increases with decreasing SZA, reaching for NO2 rnvs3p1-8 data and for HCHO rfus5p1-8 data at SZA=10° (median uncertainty over 137 datasets). The report uncertainty does not yet include the impact of spectral fitting quality and is therefore a lower limit. This uncertainty component will be included in a future PGN release; at the Izana site, it is estimated to increase the reported uncertainty at SZA=10° to for NO2 and for HCHO.
In this work, we use HCHO rfus5p1-8 and NO2 rnvs3p1-8 direct-sun total column measurements only from the ESA Validation Data Centre (EVDC) (https://evdc.esa.int, last access: 31 March 2025), because the PGN sub-dataset submitted to EVDC undergoes a more thorough quality check, in which the issues in PGN HCHO retrievals are mostly mitigated. A total of 22 sites across the globe have valid measurements for HCHO and NO2 validation in the period of study (Fig. S2).
2.5 Data use and validation statistics
For comparison between satellite HCHO data, we filter out the retrieved data based on the following criteria: we exclude pixels with RPRO quality assurance values (QA) ≤0.5, which includes SZA or VZA>70° or an activated snow/ice flag. We also exclude pixels with POMINO-derived CRFs at 440 nm greater than 0.5, to minimize the impact of cloud contamination. The same criteria are applied to the NO2 comparison as well. To examine the spatial distribution, gridded tropospheric HCHO and NO2 VCDs in April, July and October 2021 as well as January 2022 at a resolution of 0.25°×0.25° are calculated using an area-weighted oversampling technique (Zhang et al., 2023).
For comparisons between satellite and ground-based HCHO data, we take two successive steps for data processing. First, we calculate the daily average HCHO columns from ground-based MAX-DOAS and PGN measurements within the time window between 11:00 and 16:00 LT. For PGN data, we only use those with the flag “assured high quality” (data quality flag of 0) or “not-assured high quality” (data quality flag of 10) (https://www.pandonia-global-network.org/wp-content/uploads/2024/11/PGN_DataProducts_Readme_v1-8-9.pdf, last access: 31 March 2025). Then we calculate daily average satellite HCHO columns based on pixels selected using the cloud information from the POMINO retrieval, with the pixel center located within a radius of 20 km from the instruments. The daily colocated data pair is considered valid only if 10 satellite pixels or more are used for calculation. The processing for NO2 data is different from that of HCHO in three aspects: (1) the time window for NO2 is between 13:00 to 14:00 LT, as the diurnal variation of NO2 is much stronger than that of HCHO; (2) the radius between the satellite pixel center and the instrument is 5 km, considering the much larger spatial gradient of the NO2 distribution and less noise in the NO2 retrieval; and (3) we derive PGN tropospheric NO2 columns each day by subtracting stratospheric NO2 columns from the RPRO NO2 v2.4.0 L2 product over the instrument from the total NO2 columns, in order to make them comparable with satellite tropospheric NO2 columns (Pinardi et al., 2020). Based on colocated HCHO and NO2 columns, we further compare the daily tropospheric column ratio of formaldehyde to nitrogen dioxide (FNR) derived from satellite products and ground-based MAX-DOAS and PGN measurements.
To quantify the performance of satellite products relative to ground-based measurements, we derive slope, offset and correlation of the linear regression using the robust Theil–Sen estimator (Sen, 1968), which is insensitive to occasional outliers. In a relative sense, we use normalized mean bias (NMB) to quantify the deviation between satellite and ground-based measurements:
with Ω being the HCHO or NO2 vertical column given in Sects. 6.1 and 6.2 and FNR in Sect. 6.3.
Figures 1a and c illustrate the global distribution of tropospheric HCHO VCDs averaged over April, July and October 2021 as well as over January 2022 from POMINO and RPRO retrievals, respectively. High levels of tropospheric HCHO columns () are evident over the Amazon rainforest, sub-Saharan Africa, South Asia and East Asia, and northern Australia. Enhanced HCHO concentrations are also noticeable in the southeastern United States of America (USA) and Mexico, while localized hotspots with lower magnitudes are evident in the Middle East and Europe. Over the remote background regions, HCHO is primarily from CH4 oxidation, and the abundance is about at maximum. Similarly, Fig. 1b and d show the POMINO and RPRO tropospheric NO2 VCDs in April, July and October 2021 as well as in January 2022. High NO2 columns are visible over three well-known polluted regions, i.e., the North China Plain, western Europe and eastern USA, with strong hotspot signals over megacities and metropolitan areas across the globe. Low NO2 content in the remote atmosphere comes from aviation and ship emissions, natural biogenic emissions, lightning, and oxidation of long-lifetime species such as peroxyacetyl nitrate (PAN).
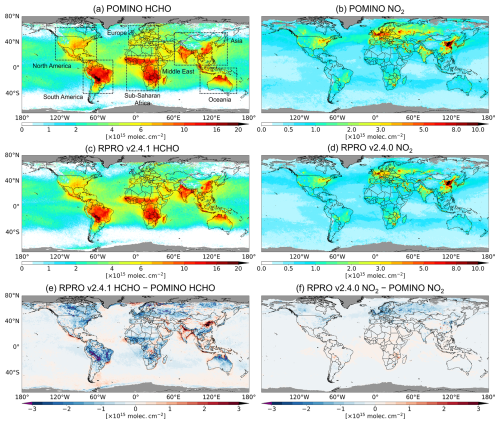
Figure 1Spatial distribution of POMINO tropospheric HCHO and NO2 VCDs (a, b), RPRO tropospheric HCHO and NO2 VCDs (c, d), and respective absolute differences (e, f) at a spatial resolution of 0.25°×0.25° averaged in April, July and October 2021 as well as in January 2022. The dashed black rectangles illustrate the spatial range of the regions used for comparison. The regions in gray mean that there are no valid observations.
A high qualitative agreement is observed for both HCHO and NO2 VCDs between RPRO and POMINO retrievals, as the same HCHO dSCDs and tropospheric NO2 SCDs are used. However, as shown in Fig. 1e, RPRO HCHO tropospheric columns are lower by or more over almost all regions with elevated HCHO columns except northern India and the North China Plain; RPRO NO2 columns are also lower than those of POMINO over most of eastern China, India, Europe and North America by up to about 20 % in a relative sense, despite the positive differences over sub-Saharan Africa and some cities such as Xi'an, Teheran, and Los Angeles (Fig. 1f).
We further make the comparison in seven specific regions (bounded by black rectangles in Fig. 1a): North America (125–60° W, 10–65° N), South America (85–35° W, 40° S–10° N), Europe (10° W–35° E, 35–60° N), sub-Saharan Africa (15° W–35° E, 35° S–20° N), the Middle East (30–60° E, 10–40° N), Asia (60–145° E, 5–55° N) and Oceania (100–160° E, 40° S–0°). Figure 2 shows the comparison results over the most polluted areas in each region, defined as where the POMINO tropospheric HCHO or NO2 VCDs averaged over April, July and October 2021 as well as over January 2022 exceed their 99th percentiles; results for regional mean comparisons are shown in Fig. S3 in the Supplement. For HCHO, RPRO data are consistently lower than POMINO by around 15 % over polluted areas in five regions, although the difference is small over the Middle East and Asia because of the cancellation between positive and negative differences on the finer spatial scale. For NO2, RPRO is smaller than POMINO by −19.4 % for North America and −23.3 % for Europe. Detailed comparisons for each month are shown in Figs. S4 and S5 in the Supplement.
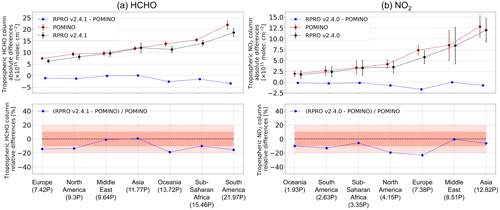
Figure 2Absolute and relative differences between POMINO and RPRO (a) HCHO and (b) NO2 tropospheric columns averaged in April, July and October 2021 as well as in January 2022 over polluted areas (defined as where POMINO mean HCHO or NO2 columns exceed their 99 percentiles) in seven regions. Regions are sorted as a function of POMINO mean HCHO or NO2 columns, with values (in the unit of “P” as ) shown in the brackets in the bottom axis. Mean POMINO (red) and RPRO (black) columns are also plotted with the absolute differences in the upper panel. Error bars represent the standard deviations of the columns. Pink areas indicate 10 % and 20 % relative differences.
Overall, POMINO and RPRO HCHO and NO2 retrievals show excellent agreement in a qualitative sense, but the column values differ by 10 % to 20 % on average over polluted areas around the world. Such differences result from the different cloud correction, aerosol correction, surface reflectance and vertical profile shapes used in AMF calculations, which will be further discussed in Sect. 4.
As listed in Table 1, we implement a series of sensitivity tests to quantify the structural uncertainty from either individual or joint effects of using different ancillary parameters in the HCHO and NO2 AMF calculations. The time period selected for the sensitivity analysis is July 2021 and January 2022, representing the summer and winter, respectively. Note that one of the most important features of the POMINO HCHO and NO2 retrievals is that they use the same cloud parameters for consistent cloud correction. Therefore, besides discussing the effect of cloud correction based on POMINO cloud parameters, we also compare the differences between HCHO columns retrieved using different cloud parameters, especially the cloud-top pressures. The influences of aerosol correction, surface reflectance, a priori profile shapes and their joint effects are discussed in the subsequent subsections.
4.1 Cloud correction
4.1.1 Effect of cloud correction based on POMINO cloud parameters
When calculating tropospheric AMFs, it is important to account for the influence of clouds on the radiative transfer process in the atmosphere (Boersma et al., 2011; De Smedt et al., 2021; Lorente et al., 2017; Martin et al., 2002). Clouds can either enhance or reduce the sensitivity to the trace gas molecules depending on their height relative to the trace gas layers (the so-called albedo or shielding effect, respectively). Despite the relatively large uncertainty of retrieved cloud parameters in the near-cloud-free scenario (defined here as CF≤0.1 or CRF≤0.4) (Richter and Burrows, 2002), most HCHO and NO2 AMF algorithms make use of the IPA method (Sect. 2.1) to explicitly account for the cloud effect.
Figure 3 shows the differences between clear-sky AMF and total AMF of all pixels with HCHO or NO2 QA>0.5 in July 2021 and January 2022, based on the FRESCO-S cloud-top pressures and POMINO recalculated cloud fractions at 440 nm with explicit aerosol corrections. For both HCHO and NO2, the differences between clear-sky AMF and total AMF are negative when cloud-top pressures are higher than 700 hPa, and their magnitudes continue to increase along with the cloud-top pressures. The negative differences can be as large as −30 % for HCHO and −20 % for NO2 when the CRFs are in the interval of 0.45 to 0.5 and cloud-top pressures are higher than 900 hPa. This illustrates the albedo effect of low clouds by increasing the contribution of photons from near-surface layers to the ensemble of photons received at the satellite instrument and thus leading to higher total AMF.
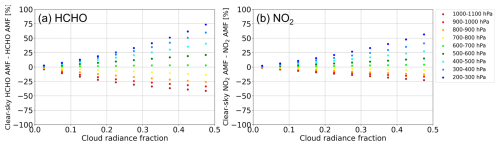
Figure 3Differences in (a) HCHO and (b) NO2 clear-sky AMF to total AMF for different cloud radiance fractions with an interval of 0.05 in different cloud-top pressure ranges (shown in different colors). All pixels with HCHO or NO2 QA>0.5 in July 2021 and January 2022 are included.
On the contrary, clouds with cloud-top pressure lower than 700 hPa reflect most photons back to the top of the atmosphere as a shield before they reach the HCHO- or NO2-abundant layers. As a result, positive differences in clear-sky AMF to total AMF occur, and they increase as the cloud-top pressures decrease, reaching 50 % or more when CRFs are in the interval of 0.4 to 0.5 and cloud-top pressures are lower than 400 hPa. This result is also in line with the previous study by Lorente et al. (2017).
In the global view (Fig. 4), for both HCHO and NO2 columns, the difference due to cloud correction (i.e., using clear-sky AMF versus total AMF) is ±10 % on average over high-value regions and can reach 40 % over specific areas. Note that all these comparisons are based on HCHO and NO2 a priori profile shapes from GEOS-CF. The signs and values of the differences might be different when using the profile shapes from another model, along with the structural uncertainty discussed in Sect. 4.1.2.
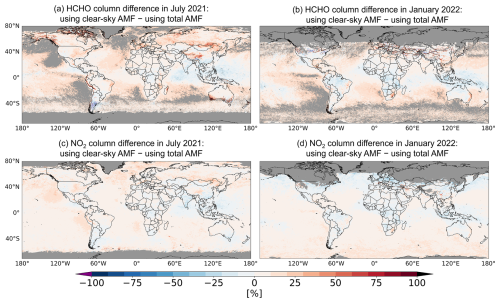
Figure 4Relative differences in tropospheric HCHO (a, b) and NO2 (c, d) columns derived using clear-sky POMINO AMF compared to those using total POMINO AMF in July 2021 and January 2022. The regions in gray mean that there are no valid observations.
One issue existing in the process of cloud correction in the POMINO retrieval is that only the cloud fraction is recalculated with explicit aerosol corrections, while the cloud-top pressure is taken from the external dataset, i.e., the FRESCO-S cloud product, in which the aerosols are implicitly accounted for. As a result, this step introduces presumably an aerosol overcorrection issue in the cloud-top pressures of partly cloudy pixels and therefore brings in additional uncertainties in the AMF calculations. Lin et al. (2015) reported that excluding aerosols leads to an increase of O2–O2-based cloud-top pressures (from 700–900 to 750–950 hPa) over eastern China, but it is difficult to clarify the mechanism due to its complexity (Lin et al., 2014). Currently there is no direct way to estimate the effect of aerosol correction on the FRESCO-S cloud height retrieval without doing O2 A-band cloud retrieval tests, which is beyond the scope of this study. However, below we give an estimation of the uncertainty in POMINO HCHO and NO2 vertical columns caused by this issue.
Given the fact that, in the retrieval algorithm, the cloud is assumed to be an optically thick Lambertian reflector with a high albedo of 0.8, the cloudy-sky AMF (and hence tropospheric AMF) is very sensitive to the accuracy of the cloud height when the cloud is low and vertically mixed with the aerosols and trace gases. In these cases, we can assume that the retrieved cloud height is primarily influenced by aerosols (Van Geffen et al., 2022a); therefore, the aerosol overcorrection issue becomes non-negligible. Focusing on valid pixels for which the difference between the surface pressure and the FRESCO-S cloud-top pressure is equal to 100 hPa or less (∼17.5 % and ∼19.9 % of total pixels in July 2021 and January 2022, respectively), the aerosol overcorrection uncertainty can be roughly estimated from the difference in HCHO and NO2 vertical columns retrieved using either aerosol-corrected clear-sky AMFs (aerosol correction applied; cloud correction not applied) or aerosol-corrected total AMFs (both aerosol and cloud corrections applied). Based on the results shown in Fig. S6 in the Supplement, we tentatively estimate the uncertainty to be in the range from 10 % to 15 % for HCHO, and within 10 % for NO2. The estimated NO2 uncertainty level is also supported by the sensitivity test results in Liu et al. (2020). They implemented a semi-explicit aerosol correction approach, in which aerosol optical effects are explicitly corrected for clear-sky AMFs, but are excluded for the cloudy-sky portion of partly cloudy pixels, and they found the NO2 differences due to the aerosol correction choice for cloudy-sky AMFs to vary from 3.1 % to 11.2 % over eastern China in July 2018. The tentatively estimated uncertainty range above is comparable to or less than that from other ancillary parameters (Sect. 5), and it only needs to be taken into account for partly cloudy pixels with low clouds.
4.1.2 Structural uncertainty of cloud correction based on different cloud parameters
The structural uncertainty of the cloud correction can be evaluated using cloud parameters from different cloud products. Lorente et al. (2017) have demonstrated that the systematic differences in cloud-top pressure can lead to substantial differences in tropospheric NO2 AMFs and VCDs. Focusing on HCHO in this section, we first compare the effective cloud fractions and cloud-top pressures calculated either in different ways or from different products. As shown in the left column of Fig. S7 in the Supplement, POMINO-based ECF calculated at 440 and 340 nm and OCRA/ROCINN-CRB ECF show similar global patterns in July 2021. Despite the differences over certain areas, great agreement is exhibited between OCRA/ROCINN-CRB ECF and POMINO-based ECF calculated at 440 nm (linear regression slope of 0.92, offset of 0.02 and correlation coefficient of 0.80) and between POMINO-based ECF calculated at 340 and 440 nm (linear regression slope of 0.93, offset of 0.01 and correlation coefficient of 0.93). However, the OCRA/ROCINN-CRB cloud-top pressures are significantly higher than those of the FRESCO-S product over the Amazon rainforest, equatorial Africa and eastern China by 100–300 hPa, while the FRESCO-S cloud-top pressures tend to be higher over many other places such as the Intertropical Convergence Zone (ITCZ) over the oceans (Fig. S6f). The comparison results over China are also qualitatively consistent with the findings by Latsch et al. (2022), in which the ROCINN CRB cloud heights differ significantly from those of FRESCO-S when considering low cloud fraction and lowest cloud height values that are critical for tropospheric trace gas retrievals. Such differences are systematic and are caused by different methodologies and ancillary parameters used in each cloud retrieval (Loyola et al., 2018; Van Geffen et al., 2022a), which are also reported in recent validation exercises using independent cloud measurements (Compernolle et al., 2021).
As shown in Fig. 5, by comparing the result of POMINO to the Fst_ORcp test (Case F1, using the OCRA/ROCINN-CRB cloud-top pressures and the POMINO-based ECFs calculated at 340 nm), we find differences in HCHO columns by up to 20 % on average over highly polluted regions, as well as a positive increment over South America. Over remote background regions such as the Pacific Ocean, however, negative differences of 0.5– are found. We attribute these differences to different OCRA/ROCINN-CRB and FRESCO-S cloud-top pressures, as ECFs in POMINO and test case Fst_ORcp are very close. Note that this is a tentative estimate of HCHO column structural uncertainty from the choices of cloud parameters for cloud correction, because the results are dependent on the explicit aerosol corrections and HCHO a priori profile shapes used in the tests.
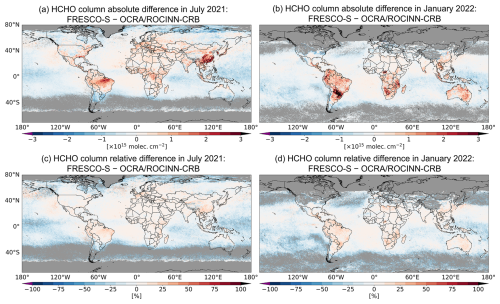
Figure 5Absolute (a, b) and relative differences (c, d) of tropospheric HCHO columns of POMINO (using FRESCO-S cloud-top pressures) to those of the Fst_ORcp sensitivity test (using OCRA/ROCINN-CRB cloud-top pressures) in July 2021 and January 2022. Different cloud-top pressures are emphasized in the title. The regions in gray mean that there are no valid observations.
In summary, the implementation of the cloud correction in HCHO and NO2 retrievals is necessary, and the structural uncertainty due to different cloud parameters needs be taken into consideration in product comparisons. On the other hand, given the different spectral ranges used for trace gas retrievals (HCHO: 340 nm; NO2: 440 nm) and cloud retrievals (OCRA/ROCINN-CRB: O2 A-band between 758 and 771 nm; FRESCO-S: O2 A-band around 760 nm), cloud parameters should always be used with caution, especially for low-cloud-fraction conditions. For example, in the ROCINN-CRB model, a priori OCRA cloud fractions smaller than 0.05 are set to zero, and the ROCINN retrieval is not activated under such clear-sky conditions. Instead of the NIR spectral range, the O2–O2 cloud algorithm uses the O2–O2 absorption window around 477 nm, but it is more sensitive to low clouds and aerosols. Therefore, further work is still needed to address such discrepancies.
4.2 Aerosol correction
The influence of aerosols on AMF calculations is very complicated as they depend on the type of aerosols (scattering or absorbing) and their height relative to the trace gases. The AMFs are generally increased when non-absorbing aerosols are vertically colocated with or lower than the trace gases, while an opposite effect arises when the non-absorbing aerosols reside vertically higher than the trace gases. On the other hand, absorbing aerosols (e.g., black carbon) always reduce the sensitivity of the satellite instruments to the trace gases (Leitão et al., 2010; Lin et al., 2014, 2015; Liu et al., 2024b). Figure S8 in the Supplement shows a global map of AOD at 340 and 440 nm used in POMINO retrievals. Areas with heavy aerosol loads in July 2021 include North America, equatorial Africa, the Middle East, India and eastern China due to biomass burning and/or anthropogenic activities, while in January 2022, the aerosol content is significant in equatorial Africa, northern India and the North China Plain. Different aerosol corrections can directly change the clear-sky AMF, affect the retrieval of cloud information (cloud fraction in particular) and modulate the AMF in the cloudy portion of the pixel. The latter two effects influence the total AMF in an indirect way, and the impact on cloud information is often more significant than on cloudy-sky AMF (Vasilkov et al., 2021).
Figure 6 shows that when using clear-sky AMFs to derive vertical columns, implicit aerosol corrections lead to higher HCHO columns by 10 % to 20 % over North America in July 2021, and the differences exceed 20 % over northern India and eastern China in January 2022. A similar pattern is shown in the NO2 comparison. This is because when aerosols that reside vertically lower than or are mixed with HCHO and NO2 molecules are excluded (i.e., in the case of implicit corrections), the calculated AMFs are lower than those with explicit aerosol corrections. On the other hand, for scenarios with strong anthropogenic emissions or biomass burning, where most HCHO and NO2 molecules are near the surface while aerosols reside above these trace gases, implicit aerosol corrections neglect the strong shielding effect of the scattering aerosols and the strong absorption of photons by the absorbing aerosols (e.g., BC), which leads to higher AMFs and lower vertical columns. The negative differences in HCHO columns over the Democratic Republic of the Congo in July 2021 (Fig. 6a) can be explained by the second case.
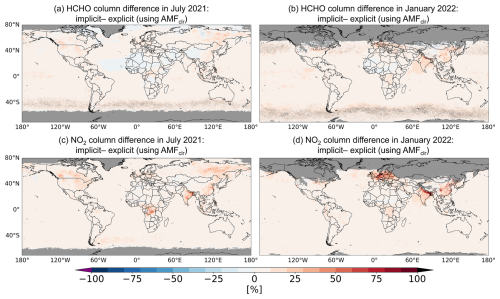
Figure 6Relative differences in tropospheric HCHO (a, b) and NO2 (c, d) columns retrieved using clear-sky AMF with implicit aerosol corrections to those with explicit aerosol corrections in July 2021 and January 2022. The regions in gray mean that there are no valid observations.
For cloudy-sky AMF, the impact of non-absorbing aerosols above a cloud is negligible since we assume the cloud to be an optically thick Lambertian reflectivity with a high albedo of 0.8 (Vasilkov et al., 2021). For absorbing aerosols above the clouds, they can reduce the backscattered radiance and hence affect the cloudy-sky AMF. However, Jethva et al. (2018) show that the occurrence of above-cloud absorbing aerosols is most frequent over coastal and oceanic regions because of the long-range transport of aerosols and low-level stratocumulus clouds. Over Southeast Asia during the springtime, the cloudy-sky frequency of occurrence of above-cloud absorbing aerosols is 20 % to 40 %, probably caused by biomass burning activities. Retrievals under these conditions are mostly discarded because the cloud fractions are too high to meet the filtering criteria for valid pixels (Sect. 2.5). Therefore, the overall influence of implicit aerosol corrections on the cloud-sky AMF can be neglected and the influence on the retrieval of cloud information, especially cloud fraction, is much more significant.
As explained in Sect. 2.2, explicit aerosol corrections affect the retrieved cloud (radiance) fraction due to the inclusion of aerosol radiative contribution. This is also confirmed in Fig. S9 in the Supplement that compares retrieved cloud radiance fractions for the implicit versus explicit aerosol correction settings, in both UV and visible bands. As shown in Fig. 7, when using cloud-corrected AMFs to consider both direct and indirect aerosol optical effects on the retrieval, the sign of HCHO relative differences over many regions is reversed from positive to negative compared to Fig. 6a and b, such as North America and South America. This reflects the enhanced cloud albedo effect that increases the calculated HCHO scattering weights over the areas where cloud layers are vertically near or below the HCHO layers. As for NO2, similar results due to an enhanced cloud albedo effect are found over North America and eastern Russia in July 2021 (Fig. 7c), but the overall pattern in January 2022 remains the same as that in Fig. 6d. Over the polluted regions in Asia and Europe, implicit aerosol corrections increase the retrieved NO2 columns by 20 % to 40 % on average. This is because most NO2 molecules over these polluted areas reside within 1 km above the ground and below the FRESCO-S cloud layers during wintertime, so the increased cloud fractions due to implicit aerosol corrections enhance the shielding effect on tropospheric NO2 AMF calculation and hence higher NO2 columns. The signs of the HCHO and NO2 differences over the North China Plain are not the same, probably because of the differences between HCHO and NO2 vertical profile shapes.
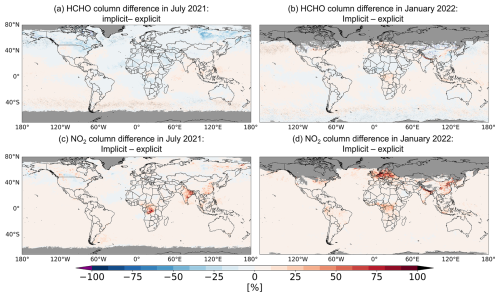
Figure 7Relative differences in tropospheric HCHO (a, b) and NO2 (c, d) columns retrieved using cloud-corrected total AMF with implicit aerosol corrections (Cases Fst_imaer and Nst_imaer) to those with explicit aerosol corrections (test case Fst_ORcp and POMINO NO2) in July 2021 and January 2022. The regions in gray mean that there are no valid observations.
4.3 Surface reflectance
Compared to the Lambertian-equivalent reflectivity (LER) model, which simply assumes the surface to be a Lambertian reflector, DLER partly accounts for the anisotropy of the surface reflectance by building a certain relationship between the reflectance and the satellite VZA, but its dependence on the SZA and RAA is still not included. The BRDF model fully considers the surface optical property as a function of SZA, VZA, RAA and wavelength. At 340 nm, the directionality of the surface reflectance is small over most regions (Kleipool et al., 2008). Figure S10 in the Supplement compares the MODIS BRDF-derived blue-sky albedo (BSA; Schaepman-Strub et al., 2006) around 470 nm and KNMI TROPOMI DLER at 440 nm over land and coastal ocean regions. In both months, DLER shows higher values than MODIS BSA except over desert and mountain regions, and the positive differences are larger than 0.1 over India in July 2021 and eastern Europe in January 2022. Reasons for these differences are not clear yet, but they are likely associated with different parameters and corrections for aerosols and snow/ice cover in the algorithm. The accuracy of the MODIS operational BRDF/albedo product (MCD43) is estimated to be 5 % to 10 % of the field data at most validation sites studied so far (https://modis-land.gsfc.nasa.gov/ValStatus.php?ProductID=MOD43, last access: 31 March 2025). Chong et al. (2024) also provide an estimation of random uncertainties in MODIS MCD43C1 surface reflectances for various surface types, which vary in the range of 0.01–0.03 for most cases.
Figures 8a and b present the influence of surface reflectance on HCHO retrievals. As it is well known that the directionality of surface reflectance plays a marginal role in the retrieval based on the UV band, nearly no difference is shown between HCHO columns retrieved using KNMI TROPOMI DLER and MLER at 340 nm. However, the systematic differences between different MLER products are a more important source of the structural uncertainty in HCHO AMFs. For example, KNMI TROPOMI MLER albedo at 340 nm is found to be consistently lower than OMI climatology monthly MLER albedo used in the RPRO product by 0.01–0.05 (Kleipool et al., 2008; Tilstra et al., 2024).
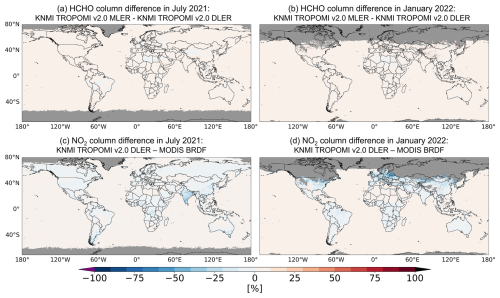
Figure 8Relative differences in tropospheric HCHO columns retrieved using KNMI TROPOMI v2.0 MLER at 340 nm (test case Fst_mler) to those using KNMI TROPOMI v2.0 DLER at 340 nm (test case Fst_ORcp) (a, b), and relative differences in tropospheric NO2 columns retrieved using KNMI TROPOMI v2.0 DLER at 440 nm (test case Nst_dler) to those using MODIS BRDF at 440 nm (POMINO NO2) (c, d) in July 2021 and January 2022. The regions in gray mean that there are no valid observations.
As for NO2, Fig. 8c and d show significantly lower tropospheric NO2 VCDs in the test case Nst_dler (Case N2) than those in the reference POMINO retrieval (Case N0) over most land areas. In January 2022, the NO2 columns retrieved using KNMI TROPOMI DLER are lower by 30 % on average over the polluted regions with NO2 columns larger than in Europe and North America. Like aerosols, the influence of surface reflectance on AMFs is also a combination of the direct effect on clear-sky AMF and the indirect effect through cloud correction (Boersma et al., 2011). As discussed by Tilstra (2024), DLER should not be considered the optimal replacement for the BRDF in the VIS wavelength range. If the directional surface reflection can be modeled in the RT calculation, it is better to use BRDF to derive surface reflectance for the tropospheric NO2 AMF calculation.
4.4 A priori profiles
In POMINO, we consistently use GEOS-CF HCHO and NO2 vertical profile shapes as the prior information for AMF calculations. Compared with the TM5-MP model of which the spatial resolution is 1°×1°, GEOS-CF features a much finer spatial resolution (0.25°×0.25°). The horizontal distributions of GEOS-CF and TM5-MP tropospheric HCHO and NO2 VCDs are shown in Fig. S11 in the Supplement, and comparisons of monthly mean HCHO and NO2 vertical profile shapes between the models and the ground-based MAX-DOAS measurements are shown in Fig. S12 in the Supplement. The colocation of model profiles and MAX-DOAS profiles follows the same methodology as described in Sect. 2.5. The differences between GEOS-CF, TM5-MP and MAX-DOAS profiles reflect the imperfections in these data yet to be fully characterized (Keller et al., 2021; Williams et al., 2017), and they are also an important source of structural uncertainty in HCHO and NO2 retrievals.
Figure 9 shows the differences in retrieved HCHO and NO2 VCDs caused by using different a priori vertical profile shapes. The HCHO and NO2 columns retrieved with the TM5-MP prior information are obtained using AMFs recalculated by combining interpolated POMINO averaging kernels (AKs) and TM5-MP a priori profile shapes. As shown in Fig. 9a and b, the spatial patterns of HCHO relative differences are variable over different places and in different months, and are they generally more significant than the individual effects of clouds, aerosols and surface reflectance changes (Figs. 4, 7 and 8). At the regional level, the HCHO structural uncertainty from a priori profile shapes is 20 % to 30 % over the clean background areas and 10 % to 20 % over the polluted areas. In contrast, the NO2 differences caused by different a priori profile shapes are around 10 % over the clean areas and reach 30 % or more over the polluted areas. Over eastern China, India and the Middle East, localized differences over cities and polluted regions are obvious (Fig. 9c and d), reflecting the significant differences between TM5-MP and GEOS-CF NO2 profile shapes. Besides, distinctive patterns along the coastal lines are visible, especially in the HCHO relative differences. This is caused by the relatively coarse horizontal resolution of TM5-MP, in which the large heterogeneity of the HCHO vertical distribution is smoothed in the 1°×1° grid.
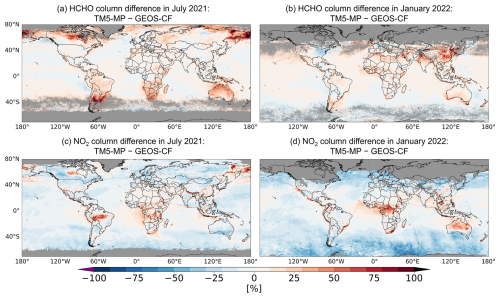
Figure 9Relative differences in tropospheric HCHO (a, b) and NO2 (c, d) columns retrieved with the TM5-MP a priori profiles (test cases Fst_tm5 and Nst_tm5) to those with GEOS-CF a priori profiles (test case Fst_ORcp and POMINO NO2) in July 2021 and January 2022. The regions in gray mean that there are no valid observations.
4.5 Summarizing the impacts of input parameters
As shown in each panel of Fig. 10, the first three columns summarize the structural uncertainty of aerosol correction, surface reflectance and a priori profile shapes on the HCHO retrieval in the corresponding region and month. As noted in Sect. 2.2, we consistently use GEOS-CF HCHO columns for background correction in every HCHO sensitivity test case. The TM5-MP HCHO columns over background regions are systematically lower than those of GEOS-CF by about on average (Fig. S11), which strongly affects the comparisons over the low-HCHO regions.
Over clean areas (HCHO columns ), a priori profile shapes are the primary source of the HCHO structural uncertainty (third column in Fig. 10). However, the differences between Fst_tm5 and the reference case Fst_ORcp are not in alignment with those of RPRO to the reference case, as manifested in the consistent drop of the blue line from the third (Fst_tm5 minus reference) to the fourth column (RPRO minus reference). This drop can be attributed to the systematic issue in the background correction. Over most areas with HCHO columns larger than , relative to the same reference case, the HCHO differences caused by using implicit aerosol corrections and TM5-MP a priori profile shapes match well with those of the RPRO product (the fourth column). However, the lower values of RPRO compared to the reference case in Europe in January 2022 do not agree with the combined results of tests Fst_imaer and Fst_tm5. This indicates that the higher OMI-based climatology of monthly MLER used in the RPRO retrieval is probably the dominant factor. Furthermore, the influence of cloud correction using different cloud parameters, especially the cloud-top pressures, varies from −20 % to 20 % depending on the specific regions and seasons. This is also an important factor for the HCHO differences between the POMINO and RPRO retrievals.
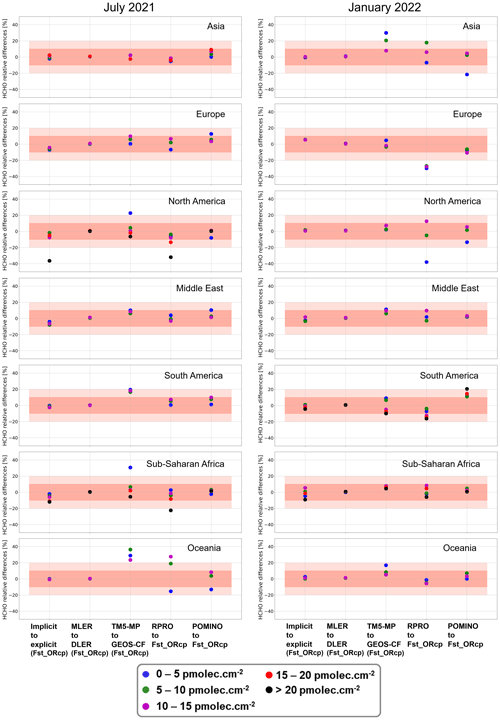
Figure 10HCHO relative differences in the sensitivity test cases Fst_imaer (Case F2, first column), Fst_mler (Case F3, second column), Fst_tm5 (Case F4, third column), the RPRO product (fourth column) and the POMINO product (fifth column) to the reference Fst_ORcp (Case F1) over seven regions in July 2021 and January 2022.
For NO2, the first three columns in Fig. 11 show the individual effects of each input parameter on the NO2 retrieval in each region. Apparently, the relative differences between RPRO and POMINO (the fifth column) show a discrepancy with the sum of the differences between each of the three cases (Nst_imaer, Nst_dler and Nst_tm5) and the reference POMINO retrieval, especially over polluted areas in North America, Europe and Asia in January 2022. However, the NO2 columns of the test case Nst_joint (Case N4) show high agreement with those of the RPRO product when compared to the POMINO retrieval (fourth column in Fig. 11); a similar result is shown for the spatial distribution in Fig. S13 in the Supplement. Therefore, the NO2 differences between POMINO and RPRO are the result of compensation effects between different aerosol corrections, on the one hand, and different surface reflectances as well as vertical profile shapes, on the other hand. These results demonstrate the nonlinear joint effects of aerosols, surface reflectance, clouds and a priori profiles in the AMF calculation, which are consistent with the previous findings (Lin et al., 2015; Liu et al., 2020). The remaining differences between Nst_joint and RPRO NO2 columns are caused by their different ways to obtain tropospheric NO2 AMFs, i.e., online pixel-specific RT calculation versus LUT-based interpolation (Lin et al., 2014).
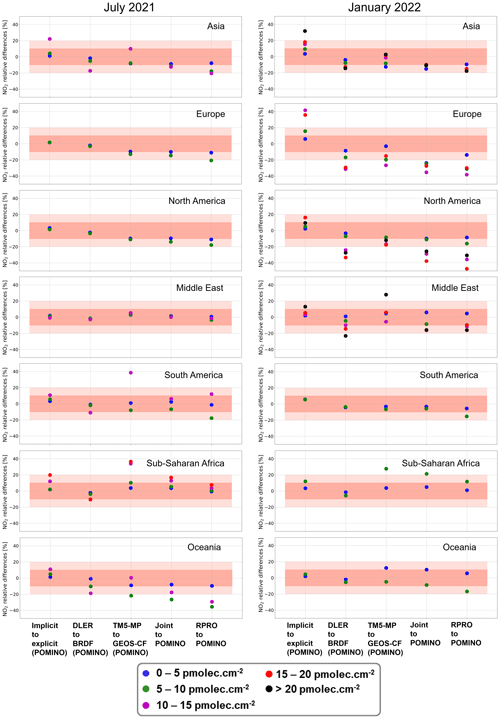
Figure 11NO2 relative differences in the sensitivity test cases Nst_imaer (Case N1, first column), Nst_dler (Case N2, second column), Nst_tm5 (Case N3, third column), Nst_joint (Case N4, fourth column) and the RPRO product (fifth column) to the POMINO product as the reference (Case N0) over seven regions in July 2021 and January 2022.
The theoretical uncertainties of the POMINO retrievals can be analytically derived by uncertainty propagation based on Eqs. (2) and (3) (Boersma et al., 2004). However, it is difficult to estimate the overall AMF uncertainty for each pixel, as one challenge is the computational cost of sensitivity calculations with the online pixel-by-pixel RT simulations. Nonetheless, random uncertainties in the observations can be reduced by spatial and temporal averaging, although the systematic uncertainties from the main retrieval steps remain. There remains a lack of information to separate random and systematic uncertainties accurately. Here we provide a preliminary estimate of the uncertainty budget for monthly averaged HCHO and NO2 columns from POMINO retrievals (Tables 3 and 4), based on our sensitivity tests and validations as well as previous work.
For HCHO, the systematic differential slant column uncertainty is 25 % for regions with low columns and 15 % for regions with elevated columns (De Smedt, 2022; De Smedt et al., 2018). The background correction uncertainty is significant for low columns (around 40 %), in which the systematic uncertainty from the dSCD normalization is estimated to be 0–, and the uncertainty from the model background is 0–. The AMF uncertainty, which is the largest contributor to the vertical column uncertainty, is mainly dependent on the errors of the ancillary parameters tested in Sect. 4. The AMF uncertainty induced by the error of a priori profile shapes is the largest with 30 % to 60 % over clean regions and around 20 % over polluted regions. The errors of cloud parameters and surface reflectance are assumed to contribute 10 % to 20 % to the AMF uncertainty, and the errors in the aerosol parameters contribute about 5 % to the AMF uncertainty for regions with low columns and 10 % for regions with elevated columns. In addition, the uncertainty due to the aerosol overcorrection issue for partly cloudy pixels with low cloud height is estimated to be 10 % to 15 % (Sect. 4.1.1). Overall, the HCHO AMF uncertainty is estimated to be about 70 % for clean regions and 30 % for polluted regions.
Table 3Estimated uncertainty budget of POMINO HCHO vertical columns for monthly mean low and elevated columns (higher than ).
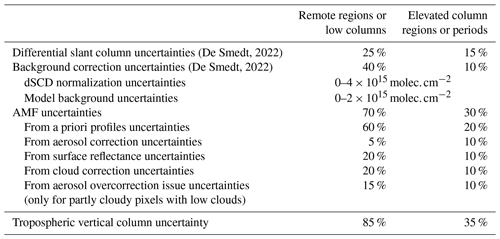
For NO2, the total SCD uncertainty is reported to be 0.5–, and a constant value of is assigned to the uncertainty of the stratospheric SCDs (Van Geffen et al., 2022b). For tropospheric AMF, the uncertainty caused by aerosol-related errors is estimated to be 10 % to 20 %, and the errors in a priori NO2 profile shapes is estimated to cause an AMF uncertainty of 10 % on average based on the sensitivity test. The contribution from cloud parameters and surface reflectance to the NO2 AMF uncertainty is estimated to be on the same level as that of a priori profile shapes. For pixels partly covered by low clouds over both clean and polluted regions, the AMF uncertainty contributed from the aerosol overcorrection issue is within 10 %. By adding these errors in quadrature, the overall NO2 AMF uncertainty is 25 % to 30 %.
Table 4Estimated uncertainty budget of monthly mean POMINO NO2 vertical columns.
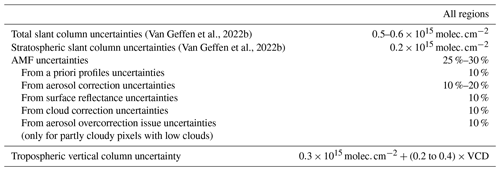
Note that the uncertainty in the total slant columns is mostly absorbed by the stratosphere–troposphere separation step and may not propagate into the tropospheric slant columns (Van Geffen et al., 2015).
By wrapping up the estimated relative contributions to the vertical column uncertainty, the total uncertainty of POMINO HCHO VCDs is estimated to be 85 % over regions with low columns, and 35 % over regions with high columns. For the POMINO NO2 retrieval, the overall uncertainty budget can be approximated as . This tentative estimation of the POMINO retrieval uncertainties is in agreement with the error analysis by De Smedt (2022) and Van Geffen et al. (2022b) and is supported by the validation results against the independent ground-based measurements (Sect. 6.1). Quantification of the errors at an individual pixel level have been achieved in previous studies (Boersma et al., 2004; Chong et al., 2024; Van Geffen et al., 2022b). As an alternative option to the Gaussian error propagation method, artificial-intelligence-based methods are an appealing approach to be tried in future work.
In this section, we present the validation results of POMINO and RPRO retrievals against independent ground-based measurements from the global MAX-DOAS network and PGN. Separate comparisons of tropospheric HCHO and NO2 columns are given in Sect. 6.1, the effect of vertical smoothing is discussed in Sect. 6.2, and the satellite-based and ground-based FNRs are evaluated in Sect. 6.3.
6.1 Validation of tropospheric HCHO and NO2 columns
Figure 12a and b present the scatterplots of daily satellite HCHO columns against ground-based measurements in April, July and October 2021 as well as in January 2022. Each data point represents a day and site. There is a lower slope and higher positive offset for POMINO compared with those of the RPRO product (slope: 0.56 versus 0.61; offset: 1.17 versus 0.24). This is in line with the discussion in Sect. 4.5 stating that POMINO employs higher HCHO columns from GEOS-CF for background correction, which is the major component of HCHO columns over areas with low HCHO level. Furthermore, at 13 polluted ground-based sites where HCHO columns are higher than , POMINO HCHO columns show a smaller bias at 8 sites (Fig. S14 in the Supplement). Overall, POMINO exhibits a smaller negative NMB (−30.8 %) than RPRO (−35.0 %). Statistics of separate validation results against MAX-DOAS and PGN measurements are given in Table S3 in the Supplement.
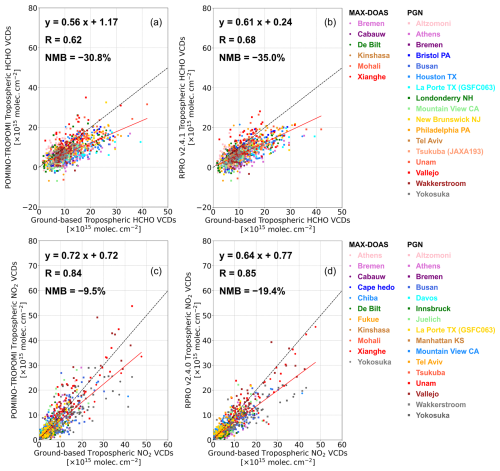
Figure 12Scatterplots of tropospheric HCHO (a, b) and NO2 (c, d) columns between satellite products (POMINO and RPRO) and ground-based measurements in April, July and October 2021 as well as in January 2022. The slope, offset and correlation from a linear regression using the robust Theil–Sen estimator and normalized mean bias (NMB) are given in each panel and plotted as the red line. The black dashed line is the 1:1 line. Each MAX-DOAS (marked by circles) and PGN site (marked by squares) is color-coded and listed on the right side.
For NO2, a better agreement with ground-based measurements is found for POMINO tropospheric columns than for RPRO (slope: 0.72 versus 0.64; offset: 0.72 versus 0.77; NMB: −9.5 % versus −19.4 %). At remote MAX-DOAS sites where tropospheric NO2 columns are around or less (Fig. S14), satellite tropospheric NO2 columns are higher by 0.3–. This is in line with the previous validation studies (Kanaya et al., 2014; Pinardi et al., 2020; Verhoelst et al., 2021; Zhang et al., 2023) and is probably because a majority of NO2 molecules over remote regions are in the free troposphere, which is above the detection height of ground-based MAX-DOAS instruments but can be well observed by spaceborne instruments. At the six most-polluted sites with mean tropospheric NO2 columns higher than , POMINO features a much-reduced bias of −14.5 % compared with the RPRO product (−22.0 %). This is because of the explicit correction for the aerosol shielding effect over highly polluted sites and lower surface reflectance, which reduces the NO2 scattering weights near the surface and hence increases the retrieved NO2 columns.
6.2 Effect of vertical smoothing for validation
To test the impact of different vertical sensitivities from the ground and space, MAX-DOAS FRM4DOAS v01.01 harmonized HCHO and NO2 datasets were used. The data provide 20-layer-resolved (from surface to ∼600 hPa) MAX-DOAS averaging kernels and vertical profiles (posterior and prior to the retrievals). Following the vertical smoothing technique (Rodgers and Connor, 2003), described in detail by Vigouroux et al. (2020), we first substituted the a priori profile shapes used in MAX-DOAS retrievals with either GEOS-CF or TM5-MP profile shapes to get corrected MAX-DOAS-retrieved profiles:
with denoting the corrected MAX-DOAS-retrieved profile, xMD the original MAX-DOAS profile, AMD the MAX-DOAS averaging kernel matrix, I the unit matrix, xMD,a the MAX-DOAS a priori profile and xSat,a the satellite a priori profile (i.e., from GEOS-CF or TM5-MP) regridded to the MAX-DOAS retrieval resolution from the surface to 600 hPa. To account for the trace gas content in the free troposphere, especially for HCHO, we further extend the corrected MAX-DOAS profile to the tropopause with the satellite profile above 600 hPa that is scaled to ensure vertical continuity of the overall tropospheric profile. After that, we perform the smoothing process using either POMINO or RPRO averaging kernels:
with the smoothed MAX-DOAS column, aSat the satellite averaging kernel vector and the corrected MAX-DOAS-retrieved profile from Eq. (5). We compare the smoothed MAX-DOAS data with satellite retrievals, and the statistics are summarized in Table 5.
For the five MAX-DOAS sites available (Table 2), we find that after smoothing the linear regression slope gets improved for both HCHO products. The negative bias of POMINO is reduced by about 10 % but that of the RPRO product is increased by about 4 %. This is because POMINO HCHO averaging kernels are smaller than those of RPRO between the surface to about 800 hPa, resulting in lower smoothed MAX-DOAS HCHO columns compared to those using RPRO HCHO averaging kernels. Smaller POMINO HCHO averaging kernels at low altitudes are due to an enhanced shielding effect from explicit aerosol corrections and lower KNMI TROPOMI MLER than the OMI-based climatological monthly MLER used in RPRO HCHO.
For NO2, among the six sites (Table 2), after applying the vertical smoothing technique, the negative NMB increases in magnitude from −7.3 % to −15.7 % for POMINO and decreases in magnitude from −24.6 % to −8.5 % for RPRO, even though a better day-to-day correlation is found for both products. Again, such changes are caused by the different averaging kernels used in the two satellite products.
Due to the scarcity of the MAX-DOAS sites for analysis here (Tables 2 and 5) and the under-representativeness in their spatial distribution (Table 2), a general conclusion cannot be made on the overall impact of vertical smoothing now. Nevertheless, the comparison results indicate the importance of considering the different vertical sensitivities between spaceborne and ground-based MAX-DOAS instruments and the different a priori profile shapes used to derive the vertical columns during the validation practice (De Smedt et al., 2021; Dimitropoulou et al., 2022; Yombo Phaka et al., 2023).
6.3 Comparisons of FNR
The FNR is an important space-based indicator of the ozone chemistry regimes and its sensitivity to precursor emissions. Figure 13 shows the scatterplots of daily FNR derived from POMINO and RPRO products against ground-based measurements, i.e., MAX-DOAS and PGN, in April, July and October 2021 as well as in January 2022. A better agreement is found between POMINO and ground-based FNR with improved linear regression statistics (slope: 0.73 versus 0.69; offset: 0.15 versus 0.22) and reduced NMB (−14.8 % versus −21.1 %) compared to those of the RPRO products. Moreover, the regression results are better in the comparisons for FNR than those in the individual comparisons for either HCHO or NO2 tropospheric VCDs (Sect. 6.1). This demonstrates the potential of using POMINO HCHO and NO2 retrievals to improve the studies on the ozone sensitivity analysis for NOx as well as VOC emission controls.
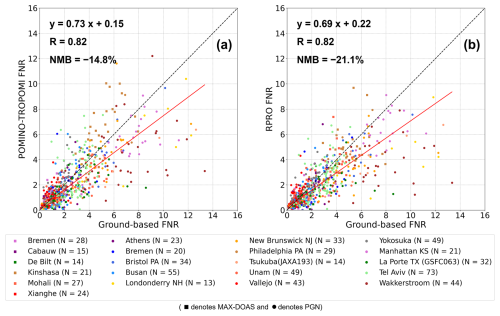
Figure 13Scatterplots of daily tropospheric column ratio of formaldehyde to nitrogen dioxide (FNR) derived from satellite products (a for POMINO and b for RPRO) and ground-based measurements in April, July and October 2021 as well as in January 2022. The slope, offset and correlation from a linear regression using the robust Theil–Sen estimator and normalized mean bias (NMB) are given in each panel and plotted as the red line.
Note that most ground-based sites used here are in North America, Europe, South Korea and Japan but very few or even no sites are in other countries or continents (Fig. S2). Thus, further validation with ground-based measurements in combination with model simulations is needed over other regions, especially those where ozone chemistry regimes change rapidly.
We developed an updated version of the POMINO algorithm providing HCHO and NO2 AMF calculations, which offers global tropospheric HCHO and NO2 VCD retrievals based on TROPOMI with improved consistency compared to current products. Compared to the independently developed RPRO HCHO and NO2 operational algorithms using different ancillary parameters, the POMINO algorithm includes (1) the surface reflectance anisotropy by using KNMI TROPOMI v2.0 DLER at 340 nm for HCHO and MODIS BRDF coefficients around 470 nm for NO2, (2) an explicit aerosol correction for both species based on GEOS-CF aerosol information and MODIS AOD at corresponding wavelengths, (3) high-resolution (0.25°×0.25°) a priori HCHO and NO2 profile shapes from the GEOS-CF dataset, and (4) a consistent cloud correction based on cloud-top pressures taken from the FRESCO-S cloud product and cloud fractions recalculated at 440 nm using the same ancillary parameters as those used in the NO2 AMF calculation.
High qualitative agreement for tropospheric HCHO and NO2 columns is found between the POMINO and RPRO products in April, July and October 2021 as well as in January 2022. However, RPRO HCHO columns are lower by 15 % on average than the POMINO HCHO columns over the polluted areas around the world, and the negative differences in RPRO tropospheric NO2 columns can reach −20 % over specific areas.
To clarify the reasons for the differences between POMINO and RPRO columns and to quantify the structural uncertainty from ancillary parameters in the AMF calculation, we performed a series of sensitivity tests on the cloud correction, aerosol correction, surface reflectance and a priori profile shapes. We find that based on POMINO-recalculated cloud fraction at 440 nm and FRESCO-S cloud-top pressures, differences between clear-sky AMFs and total AMFs vary from −25 % to more than 50 % for both HCHO and NO2, depending on the cloud fraction and the relative height between clouds and trace gases. When using cloud-top pressure data from OCRA/ROCINN-CRB instead of FRESCO-S, a large decrease in tropospheric HCHO columns is found () over the Amazon rainforest and southeast China, and the negative differences over polluted regions are about 20 % on average.
The influence of the implicit aerosol corrections used in operational products is within 10 % of the HCHO retrieval, while higher NO2 columns of 20 % to 40 % over the polluted areas in January 2022 are found with implicit aerosol corrections. Comparisons of retrieved NO2 columns using clear-sky AMFs and total AMFs with implicit aerosol corrections prove that the positive difference for NO2 is dominated by the enhanced shielding effect of clouds over NO2 layers. The directionality of the surface reflectance has a very small impact on the HCHO retrieval in the UV band, but the structural uncertainty of surface reflectance for NO2 over polluted areas can reach 30 %. The HCHO structural uncertainty from a priori profile shapes is 20 % to 30 % over the background areas and 10 % to 20 % over the polluted areas. In contrast, the NO2 differences due to different a priori profile shapes reach 30 % or more over the polluted areas. The additional test on the joint effects of these parameters shows notable nonlinear influences from aerosol correction, surface reflectance, cloud correction and a priori profile shapes in the RT calculation.
Direct comparisons of tropospheric HCHO and NO2 columns between satellite retrievals and ground-based measurements from the global MAX-DOAS network and PGN show that both POMINO HCHO and NO2 retrievals feature a reduced bias in comparison to RPRO products (HCHO: −30.8 % versus −35.0 %; NO2: −9.5 % versus −19.4 %), especially at the polluted sites. The effect of the vertical smoothing is significant and strongly depends on the satellite averaging kernels. A better agreement of daily FNR with smaller bias is also found between POMINO products and PGN measurements in comparison to results obtained with RPRO products (NMB: −14.8 % versus −21.1 %).
Overall, we demonstrate the promising performance of the TROPOMI-based POMINO algorithm for global HCHO and NO2 retrievals. However, there are still several limitations in our study. First, the aerosol overcorrection issue for partly cloudy pixels exists in the current POMINO algorithm, which has been discussed in detail in Sect. 4.1.1. The uncertainty due to this issue is estimated to be within 15 % for HCHO and 10 % for NO2. Given that TROPOMI-based O2–O2 cloud data have become available, we plan to improve the current POMINO algorithm by performing O2–O2 cloud retrievals for both cloud fraction and cloud-top pressure with explicit aerosol corrections in the future, as has been done in the POMINO-OMI and POMINO-GEMS products (Lin et al., 2015; Liu et al., 2019; Zhang et al., 2023).
Second, it should be noted that the indirect aerosol effect on HCHO and NO2 retrievals through clouds is strongly sensitive to the cloud-top pressures and the trace gas profile shapes. Using OMI O2–O2-based cloud parameters or FRESCO-S cloud-top pressures stored in the operational NO2 L2 product before version 1.4.0, previous studies have shown lower NO2 columns over the polluted North China Plain when retrieved with implicit aerosol corrections (Lin et al., 2015; Liu et al., 2020). This is because the cloud-top pressures in those studies are higher, which result in larger AMF values when implicit (instead of explicit) aerosol corrections are used. Besides, certain biases still exist in the current FRESCO-S cloud-top pressures, such as the overestimation over the ITCZ. The effect of a priori profile shapes is also significant for both HCHO and NO2 retrievals, and it deserves more attention in future analysis. Comprehensive evaluations of cloud retrievals and model performance with independent measurements are needed in future studies.
Nevertheless, the POMINO algorithm that aims at improving the consistency in multi-gas retrievals shows great potential and can be easily adapted to other satellite instruments, e.g., GEMS, TEMPO, as well as Sentinel-4 and Sentinel-5 missions. The global tropospheric HCHO and NO2 VCD retrievals presented in our study are also of value for subsequent applications such as ozone chemistry analysis and emission controls.
The data presented in the study are available from the corresponding authors upon request. The S5P TROPOMI RPRO HCHO v2.4.1 L2 product and RPRO NO2 v2.4.0 L2 product are available at the Copernicus Data Space Ecosystem (https://doi.org/10.5270/S5P-9bnp8q8, ESA, 2025a). The ground-based MAX-DOAS measurements can be provided upon request to the corresponding authors. The PGN/Pandora direct-sun measurements are available at the ESA Validation Data Centre (EVDC; https://evdc.esa.int, ESA, 2025b) and the Pandonia Global Network (https://data.ovh.pandonia-global-network.org/, PGN, 2025).
The supplement related to this article is available online at https://doi.org/10.5194/amt-18-1561-2025-supplement.
YZ, JL, NT and MVR conceived this research. YZ, HY, IDS, JL, NT and MVR designed the algorithm. YZ, HY, IDS, JL, MVR, GP, AM and SC designed the validation process together. YZ performed all calculations. RS provided LIDORT model. RN, FR, SW, LC, JVG, ML, WS and LF provided data and technical support for satellite retrievals. GP and SC provided methodological support for validation. AMC is the network principal investigator (PI) for PGN instruments. GP, SC, AMC and MT provided the discussion for PGN uncertainty estimation. MVR, GP, AM, MMF, AR, AP, VK, VS, TW, YC, HT, YK and HI provided ground-based MAX-DOAS measurements. YZ wrote the paper with inputs from JL, NT, IDS and MVR. All co-authors revised and commented on the paper.
At least one of the (co-)authors is a member of the editorial board of Atmospheric Measurement Techniques. The peer-review process was guided by an independent editor, and the authors also have no other competing interests to declare.
Publisher's note: Copernicus Publications remains neutral with regard to jurisdictional claims made in the text, published maps, institutional affiliations, or any other geographical representation in this paper. While Copernicus Publications makes every effort to include appropriate place names, the final responsibility lies with the authors. Regarding the maps used in this paper, please note that Figs. 1 and 4–9 contain disputed territories.
This work contains modified S5P TROPOMI L1b data post-processed by BIRA-IASB. We thank all the colleagues for supporting the ground-based MAX-DOAS measurements used in this work. The PGN is a bilateral project supported with funding from NASA and the ESA. We thank all the PIs, support staff, and funders for establishing and maintaining all the PGN sites used in this investigation.
This work has been supported by the National Natural Science Foundation of China (grant nos. 42075175 and 42430603), the National Key Research and Development Program of China (grant no. 2023YFC3705802), the China Scholarship Council (CSC) (grant no. 202306010348) and the Royal Belgian Institute for Space Aeronomy (BIRA-IASB) (project code: 3TEAMUVVIS).
This paper was edited by Meng Gao and reviewed by three anonymous referees.
Beelen, R., Stafoggia, M., Raaschou-Nielsen, O., Andersen, Z. J., Xun, W. W., Katsouyanni, K., Dimakopoulou, K., Brunekreef, B., Weinmayr, G., Hoffmann, B., Wolf, K., Samoli, E., Houthuijs, D., Nieuwenhuijsen, M., Oudin, A., Forsberg, B., Olsson, D., Salomaa, V., Lanki, T., Yli-Tuomi, T., Oftedal, B., Aamodt, G., Nafstad, P., De Faire, U., Pedersen, N. L., Östenson, C.-G., Fratiglioni, L., Penell, J., Korek, M., Pyko, A., Eriksen, K. T., Tjønneland, A., Becker, T., Eeftens, M., Bots, M., Meliefste, K., Wang, M., Bueno-de-Mesquita, B., Sugiri, D., Krämer, U., Heinrich, J., de Hoogh, K., Key, T., Peters, A., Cyrys, J., Concin, H., Nagel, G., Ineichen, A., Schaffner, E., Probst-Hensch, N., Dratva, J., Ducret-Stich, R., Vilier, A., Clavel-Chapelon, F., Stempfelet, M., Grioni, S., Krogh, V., Tsai, M.-Y., Marcon, A., Ricceri, F., Sacerdote, C., Galassi, C., Migliore, E., Ranzi, A., Cesaroni, G., Badaloni, C., Forastiere, F., Tamayo, I., Amiano, P., Dorronsoro, M., Katsoulis, M., Trichopoulou, A., Vineis, P., and Hoek, G.: Long-term Exposure to Air Pollution and Cardiovascular Mortality: An Analysis of 22 European Cohorts, Epidemiology, 25, 368, https://doi.org/10.1097/EDE.0000000000000076, 2014.
Boersma, K. F., Eskes, H. J., and Brinksma, E. J.: Error analysis for tropospheric NO2 retrieval from space, J. Geophys. Res.-Atmos., 109, D04311, https://doi.org/10.1029/2003JD003962, 2004.
Boersma, K. F., Eskes, H. J., Dirksen, R. J., van der A, R. J., Veefkind, J. P., Stammes, P., Huijnen, V., Kleipool, Q. L., Sneep, M., Claas, J., Leitão, J., Richter, A., Zhou, Y., and Brunner, D.: An improved tropospheric NO2 column retrieval algorithm for the Ozone Monitoring Instrument, Atmos. Meas. Tech., 4, 1905–1928, https://doi.org/10.5194/amt-4-1905-2011, 2011.
Bovensmann, H., Burrows, J. P., Buchwitz, M., Frerick, J., Noël, S., Rozanov, V. V., Chance, K. V., and Goede, A. P. H.: SCIAMACHY: Mission Objectives and Measurement Modes, J. Atmos. Sci., 56, 127–150, https://doi.org/10.1175/1520-0469(1999)056<0127:SMOAMM>2.0.CO;2, 1999.
Burrows, J. P., Weber, M., Buchwitz, M., Rozanov, V., Ladstätter-Weißenmayer, A., Richter, A., DeBeek, R., Hoogen, R., Bramstedt, K., Eichmann, K.-U., Eisinger, M., and Perner, D.: The Global Ozone Monitoring Experiment (GOME): Mission Concept and First Scientific Results, J. Atmos. Sci., 56, 151–175, https://doi.org/10.1175/1520-0469(1999)056<0151:TGOMEG>2.0.CO;2, 1999.
Callies, J., Corpaccioli, E., Eisinger, M., Hahne, A., and Lefebvre, A.: GOME-2 – Metop's second-generation sensor for operational ozone monitoring, ESA Bull., 102, 28–36, 2000.
Chen, L., Lin, J., Martin, R., Du, M., Weng, H., Kong, H., Ni, R., Meng, J., Zhang, Y., Zhang, L., and van Donkelaar, A.: Inequality in historical transboundary anthropogenic PM2.5 health impacts, Sci. Bull., 67, 437–444, https://doi.org/10.1016/j.scib.2021.11.007, 2022.
Chong, H., González Abad, G., Nowlan, C. R., Chan Miller, C., Saiz-Lopez, A., Fernandez, R. P., Kwon, H.-A., Ayazpour, Z., Wang, H., Souri, A. H., Liu, X., Chance, K., O'Sullivan, E., Kim, J., Koo, J.-H., Simpson, W. R., Hendrick, F., Querel, R., Jaross, G., Seftor, C., and Suleiman, R. M.: Global retrieval of stratospheric and tropospheric BrO columns from the Ozone Mapping and Profiler Suite Nadir Mapper (OMPS-NM) on board the Suomi-NPP satellite, Atmos. Meas. Tech., 17, 2873–2916, https://doi.org/10.5194/amt-17-2873-2024, 2024.
Compernolle, S., Argyrouli, A., Lutz, R., Sneep, M., Lambert, J.-C., Fjæraa, A. M., Hubert, D., Keppens, A., Loyola, D., O'Connor, E., Romahn, F., Stammes, P., Verhoelst, T., and Wang, P.: Validation of the Sentinel-5 Precursor TROPOMI cloud data with Cloudnet, Aura OMI O2–O2, MODIS, and Suomi-NPP VIIRS, Atmos. Meas. Tech., 14, 2451–2476, https://doi.org/10.5194/amt-14-2451-2021, 2021.
Cooper, M. J., Martin, R. V., Hammer, M. S., Levelt, P. F., Veefkind, P., Lamsal, L. N., Krotkov, N. A., Brook, J. R., and McLinden, C. A.: Global fine-scale changes in ambient NO2 during COVID-19 lockdowns, Nature, 601, 380–387, https://doi.org/10.1038/s41586-021-04229-0, 2022.
Crutzen, P. J.: The influence of nitrogen oxides on the atmospheric ozone content, Q. J. Roy. Meteor. Soc., 96, 320–325, https://doi.org/10.1002/qj.49709640815, 1970.
De Smedt, I.: TROPOMI ATBD of the HCHO data product, Issue 2.4.1, Royal Belgian Institute for Space Aeronomy (BIRA-IASB), Brussels, Belgium, Document number: S5P-BIRA-L2-ATBD-400F, 2022.
De Smedt, I., Stavrakou, T., Müller, J.-F., van der A, R. J., and Van Roozendael, M.: Trend detection in satellite observations of formaldehyde tropospheric columns, Geophys. Res. Lett., 37, L18808, https://doi.org/10.1029/2010GL044245, 2010.
De Smedt, I., Theys, N., Yu, H., Danckaert, T., Lerot, C., Compernolle, S., Van Roozendael, M., Richter, A., Hilboll, A., Peters, E., Pedergnana, M., Loyola, D., Beirle, S., Wagner, T., Eskes, H., van Geffen, J., Boersma, K. F., and Veefkind, P.: Algorithm theoretical baseline for formaldehyde retrievals from S5P TROPOMI and from the QA4ECV project, Atmos. Meas. Tech., 11, 2395–2426, https://doi.org/10.5194/amt-11-2395-2018, 2018.
De Smedt, I., Pinardi, G., Vigouroux, C., Compernolle, S., Bais, A., Benavent, N., Boersma, F., Chan, K.-L., Donner, S., Eichmann, K.-U., Hedelt, P., Hendrick, F., Irie, H., Kumar, V., Lambert, J.-C., Langerock, B., Lerot, C., Liu, C., Loyola, D., Piters, A., Richter, A., Rivera Cárdenas, C., Romahn, F., Ryan, R. G., Sinha, V., Theys, N., Vlietinck, J., Wagner, T., Wang, T., Yu, H., and Van Roozendael, M.: Comparative assessment of TROPOMI and OMI formaldehyde observations and validation against MAX-DOAS network column measurements, Atmos. Chem. Phys., 21, 12561–12593, https://doi.org/10.5194/acp-21-12561-2021, 2021.
Dimitropoulou, E., Hendrick, F., Friedrich, M. M., Tack, F., Pinardi, G., Merlaud, A., Fayt, C., Hermans, C., Fierens, F., and Van Roozendael, M.: Horizontal distribution of tropospheric NO2 and aerosols derived by dual-scan multi-wavelength multi-axis differential optical absorption spectroscopy (MAX-DOAS) measurements in Uccle, Belgium, Atmos. Meas. Tech., 15, 4503–4529, https://doi.org/10.5194/amt-15-4503-2022, 2022.
Dittman, M. G., Ramberg, E., Chrisp, M., Rodriguez, J. V., Sparks, A. L., Zaun, N. H., Hendershot, P., Dixon, T., Philbrick, R. H., and Wasinger, D.: Nadir ultraviolet imaging spectrometer for the NPOESS Ozone Mapping and Profiler Suite (OMPS), Proc. SPIE, 4814, 111–119, https://doi.org/10.1117/12.453748, 2002.
ESA: S5P Products, Products Overview, Copernicus Data Space Ecosystem, ESA [data set], https://doi.org/10.5270/S5P-9bnp8q8, 2025a.
ESA: ESA Validation Data Center, ESA, https://evdc.esa.int, last access: 31 March 2025b.
Herman, J., Cede, A., Spinei, E., Mount, G., Tzortziou, M., and Abuhassan, N.: NO2 column amounts from ground-based Pandora and MFDOAS spectrometers using the direct-sun DOAS technique: Intercomparisons and application to OMI validation, J. Geophys. Res.-Atmos., 114, D13307, https://doi.org/10.1029/2009JD011848, 2009.
Herman, J., Abuhassan, N., Kim, J., Kim, J., Dubey, M., Raponi, M., and Tzortziou, M.: Underestimation of column NO2 amounts from the OMI satellite compared to diurnally varying ground-based retrievals from multiple PANDORA spectrometer instruments, Atmos. Meas. Tech., 12, 5593–5612, https://doi.org/10.5194/amt-12-5593-2019, 2019.
Irie, H., Takashima, H., Kanaya, Y., Boersma, K. F., Gast, L., Wittrock, F., Brunner, D., Zhou, Y., and Van Roozendael, M.: Eight-component retrievals from ground-based MAX-DOAS observations, Atmos. Meas. Tech., 4, 1027–1044, https://doi.org/10.5194/amt-4-1027-2011, 2011.
Irie, H., Boersma, K. F., Kanaya, Y., Takashima, H., Pan, X., and Wang, Z. F.: Quantitative bias estimates for tropospheric NO2 columns retrieved from SCIAMACHY, OMI, and GOME-2 using a common standard for East Asia, Atmos. Meas. Tech., 5, 2403–2411, https://doi.org/10.5194/amt-5-2403-2012, 2012.
Irie, H., Nakayama, T., Shimizu, A., Yamazaki, A., Nagai, T., Uchiyama, A., Zaizen, Y., Kagamitani, S., and Matsumi, Y.: Evaluation of MAX-DOAS aerosol retrievals by coincident observations using CRDS, lidar, and sky radiometer inTsukuba, Japan, Atmos. Meas. Tech., 8, 2775–2788, https://doi.org/10.5194/amt-8-2775-2015, 2015.
Jethva, H., Torres, O., and Ahn, C.: A 12-year long global record of optical depth of absorbing aerosols above the clouds derived from the OMI/OMACA algorithm, Atmos. Meas. Tech., 11, 5837–5864, https://doi.org/10.5194/amt-11-5837-2018, 2018.
Jiang, Z., Zhu, R., Miyazaki, K., McDonald, B. C., Klimont, Z., Zheng, B., Boersma, K. F., Zhang, Q., Worden, H., Worden, J. R., Henze, D. K., Jones, D. B. A., Denier van der Gon, H. A. C., and Eskes, H.: Decadal Variabilities in Tropospheric Nitrogen Oxides Over United States, Europe, and China, J. Geophys. Res.-Atmos., 127, e2021JD035872, https://doi.org/10.1029/2021JD035872, 2022.
Jin, X. and Holloway, T.: Spatial and temporal variability of ozone sensitivity over China observed from the Ozone Monitoring Instrument, J. Geophys. Res.-Atmos., 120, 7229–7246, https://doi.org/10.1002/2015JD023250, 2015.
Jin, X., Fiore, A. M., Murray, L. T., Valin, L. C., Lamsal, L. N., Duncan, B., Folkert Boersma, K., De Smedt, I., Abad, G. G., Chance, K., and Tonnesen, G. S.: Evaluating a Space-Based Indicator of Surface Ozone-NO-VOC Sensitivity Over Midlatitude Source Regions and Application to Decadal Trends, J. Geophys. Res.-Atmos., 122, 10439–10461, https://doi.org/10.1002/2017JD026720, 2017.
Jin, X., Fiore, A., Boersma, K. F., De Smedt, I., and Valin, L.: Inferring Changes in Summertime Surface Ozone–NOx–VOC Chemistry over U. S. Urban Areas from Two Decades of Satellite and Ground-Based Observations, Environ. Sci. Technol., 54, 6518–6529, https://doi.org/10.1021/acs.est.9b07785, 2020.
Jin, X., Fiore, A. M., and Cohen, R. C.: Space-Based Observations of Ozone Precursors within California Wildfire Plumes and the Impacts on Ozone-NOx-VOC Chemistry, Environ. Sci. Technol., 57, 14648–14660, https://doi.org/10.1021/acs.est.3c04411, 2023.
Kai-Sikhakhane, R. F., Scholes, M. C., Piketh, S. J., van Geffen, J., Garland, R. M., Havenga, H., and Scholes, R. J.: Assessing Nitrogen Dioxide in the Highveld Troposphere: Pandora Insights and TROPOMI Sentinel-5P Evaluation, Atmosphere-Basel, 15, 1187, https://doi.org/10.3390/atmos15101187, 2024.
Kanaya, Y., Irie, H., Takashima, H., Iwabuchi, H., Akimoto, H., Sudo, K., Gu, M., Chong, J., Kim, Y. J., Lee, H., Li, A., Si, F., Xu, J., Xie, P.-H., Liu, W.-Q., Dzhola, A., Postylyakov, O., Ivanov, V., Grechko, E., Terpugova, S., and Panchenko, M.: Long-term MAX-DOAS network observations of NO2 in Russia and Asia (MADRAS) during the period 2007–2012: instrumentation, elucidation of climatology, and comparisons with OMI satellite observations and global model simulations, Atmos. Chem. Phys., 14, 7909–7927, https://doi.org/10.5194/acp-14-7909-2014, 2014.
Keller, C. A., Knowland, K. E., Duncan, B. N., Liu, J., Anderson, D. C., Das, S., Lucchesi, R. A., Lundgren, E. W., Nicely, J. M., Nielsen, E., Ott, L. E., Saunders, E., Strode, S. A., Wales, P. A., Jacob, D. J., and Pawson, S.: Description of the NASA GEOS Composition Forecast Modeling System GEOS-CF v1.0, J. Adv. Model. Earth Sy., 13, e2020MS002413, https://doi.org/10.1029/2020MS002413, 2021.
Kim, J., Jeong, U., Ahn, M.-H., Kim, J. H., Park, R. J., Lee, H., Song, C. H., Choi, Y.-S., Lee, K.-H., Yoo, J.-M., Jeong, M.-J., Park, S. K., Lee, K.-M., Song, C.-K., Kim, S.-W., Kim, Y. J., Kim, S.-W., Kim, M., Go, S., Liu, X., Chance, K., Miller, C. C., Al-Saadi, J., Veihelmann, B., Bhartia, P. K., Torres, O., Abad, G. G., Haffner, D. P., Ko, D. H., Lee, S. H., Woo, J.-H., Chong, H., Park, S. S., Nicks, D., Choi, W. J., Moon, K.-J., Cho, A., Yoon, J., Kim, S., Hong, H., Lee, K., Lee, H., Lee, S., Choi, M., Veefkind, P., Levelt, P. F., Edwards, D. P., Kang, M., Eo, M., Bak, J., Baek, K., Kwon, H.-A., Yang, J., Park, J., Han, K. M., Kim, B.-R., Shin, H.-W., Choi, H., Lee, E., Chong, J., Cha, Y., Koo, J.-H., Irie, H., Hayashida, S., Kasai, Y., Kanaya, Y., Liu, C., Lin, J., Crawford, J. H., Carmichael, G. R., Newchurch, M. J., Lefer, B. L., Herman, J. R., Swap, R. J., Lau, A. K. H., Kurosu, T. P., Jaross, G., Ahlers, B., Dobber, M., McElroy, C. T., and Choi, Y.: New Era of Air Quality Monitoring from Space: Geostationary Environment Monitoring Spectrometer (GEMS), B. Am. Meteorol. Soc., 101, E1–E22, https://doi.org/10.1175/BAMS-D-18-0013.1, 2020.
Kleipool, Q. L., Dobber, M. R., de Haan, J. F., and Levelt, P. F.: Earth surface reflectance climatology from 3 years of OMI data, J. Geophys. Res.-Atmos., 113, D18308, https://doi.org/10.1029/2008JD010290, 2008.
Kong, H., Lin, J., Chen, L., Zhang, Y., Yan, Y., Liu, M., Ni, R., Liu, Z., and Weng, H.: Considerable Unaccounted Local Sources of NOx Emissions in China Revealed from Satellite, Environ. Sci. Technol., 56, 7131–7142, https://doi.org/10.1021/acs.est.1c07723, 2022.
Kumar, V., Beirle, S., Dörner, S., Mishra, A. K., Donner, S., Wang, Y., Sinha, V., and Wagner, T.: Long-term MAX-DOAS measurements of NO2, HCHO, and aerosols and evaluation of corresponding satellite data products over Mohali in the Indo-Gangetic Plain, Atmos. Chem. Phys., 20, 14183–14235, https://doi.org/10.5194/acp-20-14183-2020, 2020.
Latsch, M., Richter, A., Eskes, H., Sneep, M., Wang, P., Veefkind, P., Lutz, R., Loyola, D., Argyrouli, A., Valks, P., Wagner, T., Sihler, H., van Roozendael, M., Theys, N., Yu, H., Siddans, R., and Burrows, J. P.: Intercomparison of Sentinel-5P TROPOMI cloud products for tropospheric trace gas retrievals, Atmos. Meas. Tech., 15, 6257–6283, https://doi.org/10.5194/amt-15-6257-2022, 2022.
Leitão, J., Richter, A., Vrekoussis, M., Kokhanovsky, A., Zhang, Q. J., Beekmann, M., and Burrows, J. P.: On the improvement of NO2 satellite retrievals – aerosol impact on the airmass factors, Atmos. Meas. Tech., 3, 475–493, https://doi.org/10.5194/amt-3-475-2010, 2010.
Levelt, P. F., van den Oord, G. H. J., Dobber, M. R., Malkki, A., Visser, H., Vries, J. de, Stammes, P., Lundell, J. O. V., and Saari, H.: The ozone monitoring instrument, IEEE T. Geosci. Remote, 44, 1093–1101, https://doi.org/10.1109/TGRS.2006.872333, 2006.
Li, J., Wang, Y., Zhang, R., Smeltzer, C., Weinheimer, A., Herman, J., Boersma, K. F., Celarier, E. A., Long, R. W., Szykman, J. J., Delgado, R., Thompson, A. M., Knepp, T. N., Lamsal, L. N., Janz, S. J., Kowalewski, M. G., Liu, X., and Nowlan, C. R.: Comprehensive evaluations of diurnal NO2 measurements during DISCOVER-AQ 2011: effects of resolution-dependent representation of NOx emissions, Atmos. Chem. Phys., 21, 11133–11160, https://doi.org/10.5194/acp-21-11133-2021, 2021.
Li, X., Wang, P., Wang, W., Zhang, H., Shi, S., Xue, T., Lin, J., Zhang, Y., Liu, M., Chen, R., Kan, H., and Meng, X.: Mortality burden due to ambient nitrogen dioxide pollution in China: Application of high-resolution models, Environ. Int., 176, 107967, https://doi.org/10.1016/j.envint.2023.107967, 2023.
Lin, J.-T.: Satellite constraint for emissions of nitrogen oxides from anthropogenic, lightning and soil sources over East China on a high-resolution grid, Atmos. Chem. Phys., 12, 2881–2898, https://doi.org/10.5194/acp-12-2881-2012, 2012.
Lin, J.-T., Martin, R. V., Boersma, K. F., Sneep, M., Stammes, P., Spurr, R., Wang, P., Van Roozendael, M., Clémer, K., and Irie, H.: Retrieving tropospheric nitrogen dioxide from the Ozone Monitoring Instrument: effects of aerosols, surface reflectance anisotropy, and vertical profile of nitrogen dioxide, Atmos. Chem. Phys., 14, 1441–1461, https://doi.org/10.5194/acp-14-1441-2014, 2014.
Lin, J.-T., Liu, M.-Y., Xin, J.-Y., Boersma, K. F., Spurr, R., Martin, R., and Zhang, Q.: Influence of aerosols and surface reflectance on satellite NO2 retrieval: seasonal and spatial characteristics and implications for NOx emission constraints, Atmos. Chem. Phys., 15, 11217–11241, https://doi.org/10.5194/acp-15-11217-2015, 2015.
Liu, M., Lin, J., Boersma, K. F., Pinardi, G., Wang, Y., Chimot, J., Wagner, T., Xie, P., Eskes, H., Van Roozendael, M., Hendrick, F., Wang, P., Wang, T., Yan, Y., Chen, L., and Ni, R.: Improved aerosol correction for OMI tropospheric NO2 retrieval over East Asia: constraint from CALIOP aerosol vertical profile, Atmos. Meas. Tech., 12, 1–21, https://doi.org/10.5194/amt-12-1-2019, 2019.
Liu, M., Lin, J., Kong, H., Boersma, K. F., Eskes, H., Kanaya, Y., He, Q., Tian, X., Qin, K., Xie, P., Spurr, R., Ni, R., Yan, Y., Weng, H., and Wang, J.: A new TROPOMI product for tropospheric NO2 columns over East Asia with explicit aerosol corrections, Atmos. Meas. Tech., 13, 4247–4259, https://doi.org/10.5194/amt-13-4247-2020, 2020.
Liu, O., Li, Z., Lin, Y., Fan, C., Zhang, Y., Li, K., Zhang, P., Wei, Y., Chen, T., Dong, J., and de Leeuw, G.: Evaluation of the first year of Pandora NO2 measurements over Beijing and application to satellite validation, Atmos. Meas. Tech., 17, 377–395, https://doi.org/10.5194/amt-17-377-2024, 2024a.
Liu, S., Valks, P., Pinardi, G., Xu, J., Chan, K. L., Argyrouli, A., Lutz, R., Beirle, S., Khorsandi, E., Baier, F., Huijnen, V., Bais, A., Donner, S., Dörner, S., Gratsea, M., Hendrick, F., Karagkiozidis, D., Lange, K., Piters, A. J. M., Remmers, J., Richter, A., Van Roozendael, M., Wagner, T., Wenig, M., and Loyola, D. G.: An improved TROPOMI tropospheric NO2 research product over Europe, Atmos. Meas. Tech., 14, 7297–7327, https://doi.org/10.5194/amt-14-7297-2021, 2021.
Liu, S., Valks, P., Curci, G., Chen, Y., Shu, L., Jin, J., Sun, S., Pu, D., Li, X., Li, J., Zuo, X., Fu, W., Li, Y., Zhang, P., Yang, X., Fu, T.-M., and Zhu, L.: Satellite NO2 Retrieval Complicated by Aerosol Composition over Global Urban Agglomerations: Seasonal Variations and Long-Term Trends (2001–2018), Environ. Sci. Technol., 58, 7891–7903, https://doi.org/10.1021/acs.est.3c02111, 2024b.
Lorente, A., Folkert Boersma, K., Yu, H., Dörner, S., Hilboll, A., Richter, A., Liu, M., Lamsal, L. N., Barkley, M., De Smedt, I., Van Roozendael, M., Wang, Y., Wagner, T., Beirle, S., Lin, J.-T., Krotkov, N., Stammes, P., Wang, P., Eskes, H. J., and Krol, M.: Structural uncertainty in air mass factor calculation for NO2 and HCHO satellite retrievals, Atmos. Meas. Tech., 10, 759–782, https://doi.org/10.5194/amt-10-759-2017, 2017.
Loyola, D. G., Gimeno García, S., Lutz, R., Argyrouli, A., Romahn, F., Spurr, R. J. D., Pedergnana, M., Doicu, A., Molina García, V., and Schüssler, O.: The operational cloud retrieval algorithms from TROPOMI on board Sentinel-5 Precursor, Atmos. Meas. Tech., 11, 409–427, https://doi.org/10.5194/amt-11-409-2018, 2018.
Martin, R. V., Chance, K., Jacob, D. J., Kurosu, T. P., Spurr, R. J. D., Bucsela, E., Gleason, J. F., Palmer, P. I., Bey, I., Fiore, A. M., Li, Q., Yantosca, R. M., and Koelemeijer, R. B. A.: An improved retrieval of tropospheric nitrogen dioxide from GOME, J. Geophys. Res.-Atmos., 107, ACH9-1–ACH9-21, https://doi.org/10.1029/2001JD001027, 2002.
Pandonia Global Network (PGN): Pandonia data archive, Pandonia Global Network (PGN) [data set], https://data.ovh.pandonia-global-network.org/, last access: 31 March 2025.
Pinardi, G., Van Roozendael, M., Hendrick, F., Theys, N., Abuhassan, N., Bais, A., Boersma, F., Cede, A., Chong, J., Donner, S., Drosoglou, T., Dzhola, A., Eskes, H., Frieß, U., Granville, J., Herman, J. R., Holla, R., Hovila, J., Irie, H., Kanaya, Y., Karagkiozidis, D., Kouremeti, N., Lambert, J.-C., Ma, J., Peters, E., Piters, A., Postylyakov, O., Richter, A., Remmers, J., Takashima, H., Tiefengraber, M., Valks, P., Vlemmix, T., Wagner, T., and Wittrock, F.: Validation of tropospheric NO2 column measurements of GOME-2A and OMI using MAX-DOAS and direct sun network observations, Atmos. Meas. Tech., 13, 6141–6174, https://doi.org/10.5194/amt-13-6141-2020, 2020.
Richter, A. and Burrows, J. P.: Tropospheric NO2 from GOME measurements, Adv. Space Res., 29, 1673–1683, https://doi.org/10.1016/S0273-1177(02)00100-X, 2002.
Richter, A., Burrows, J. P., Nüß, H., Granier, C., and Niemeier, U.: Increase in tropospheric nitrogen dioxide over China observed from space, Nature, 437, 129–132, https://doi.org/10.1038/nature04092, 2005.
Rodgers, C. D. and Connor, B. J.: Intercomparison of remote sounding instruments, J. Geophys. Res.-Atmos., 108, 4116, https://doi.org/10.1029/2002JD002299, 2003.
Roujean, J.-L., Leroy, M., and Deschamps, P.-Y.: A bidirectional reflectance model of the Earth's surface for the correction of remote sensing data, J. Geophys. Res.-Atmos., 97, 20455–20468, https://doi.org/10.1029/92JD01411, 1992.
Schaepman-Strub, G., Schaepman, M. E., Painter, T. H., Dangel, S., and Martonchik, J. V.: Reflectance quantities in optical remote sensing – definitions and case studies, Remote Sens. Environ., 103, 27–42, https://doi.org/10.1016/j.rse.2006.03.002, 2006.
Sen, P. K.: Estimates of the Regression Coefficient Based on Kendall's Tau, J. Am. Stat. Assoc., 63, 1379–1389, https://doi.org/10.1080/01621459.1968.10480934, 1968.
Shindell, D. T., Faluvegi, G., Koch, D. M., Schmidt, G. A., Unger, N., and Bauer, S. E.: Improved Attribution of Climate Forcing to Emissions, Science, 326, 716–718, https://doi.org/10.1126/science.1174760, 2009.
Stavrakou, T., Müller, J.-F., Bauwens, M., De Smedt, I., Van Roozendael, M., and Guenther, A.: Impact of Short-Term Climate Variability on Volatile Organic Compounds Emissions Assessed Using OMI Satellite Formaldehyde Observations, Geophys. Res. Lett., 45, 8681–8689, https://doi.org/10.1029/2018GL078676, 2018.
Su, W., Liu, C., Chan, K. L., Hu, Q., Liu, H., Ji, X., Zhu, Y., Liu, T., Zhang, C., Chen, Y., and Liu, J.: An improved TROPOMI tropospheric HCHO retrieval over China, Atmos. Meas. Tech., 13, 6271–6292, https://doi.org/10.5194/amt-13-6271-2020, 2020.
Tilstra, G.: TROPOMI ATBD of the directionally dependent surface Lambertian-equivalent reflectivity, 2024.
Tilstra, L. G., de Graaf, M., Trees, V. J. H., Litvinov, P., Dubovik, O., and Stammes, P.: A directional surface reflectance climatology determined from TROPOMI observations, Atmos. Meas. Tech., 17, 2235–2256, https://doi.org/10.5194/amt-17-2235-2024, 2024.
van Geffen, J. H. G. M., Boersma, K. F., Van Roozendael, M., Hendrick, F., Mahieu, E., De Smedt, I., Sneep, M., and Veefkind, J. P.: Improved spectral fitting of nitrogen dioxide from OMI in the 405–465 nm window, Atmos. Meas. Tech., 8, 1685–1699, https://doi.org/10.5194/amt-8-1685-2015, 2015.
van Geffen, J., Boersma, K. F., Eskes, H., Sneep, M., ter Linden, M., Zara, M., and Veefkind, J. P.: S5P TROPOMI NO2 slant column retrieval: method, stability, uncertainties and comparisons with OMI, Atmos. Meas. Tech., 13, 1315–1335, https://doi.org/10.5194/amt-13-1315-2020, 2020.
van Geffen, J., Eskes, H., Compernolle, S., Pinardi, G., Verhoelst, T., Lambert, J.-C., Sneep, M., ter Linden, M., Ludewig, A., Boersma, K. F., and Veefkind, J. P.: Sentinel-5P TROPOMI NO2 retrieval: impact of version v2.2 improvements and comparisons with OMI and ground-based data, Atmos. Meas. Tech., 15, 2037–2060, https://doi.org/10.5194/amt-15-2037-2022, 2022a.
Van Geffen, J. H. G. M., Eskes, H. J., Boersma, K. F., and Veefkind, J. P.: TROPOMI ATBD of the total and tropospheric NO2 data products, Issue 2.4.0, Royal Netherlands Meteorological Institute (KNMI), De Bilt, the Netherlands, Document number: S5P-KNMI-L2-0005-RP, 2022b.
Van Roozendael, M., Hendrick, F., Friedrich, M. M., Fayt, C., Bais, A., Beirle, S., Bösch, T., Navarro Comas, M., Friess, U., Karagkiozidis, D., Kreher, K., Merlaud, A., Pinardi, G., Piters, A., Prados-Roman, C., Puentedura, O., Reischmann, L., Richter, A., Tirpitz, J.-L., Wagner, T., Yela, M., and Ziegler, S.: Fiducial Reference Measurements for Air Quality Monitoring Using Ground-Based MAX-DOAS Instruments (FRM4DOAS), Remote Sens.-Basel, 16, 4523, https://doi.org/10.3390/rs16234523, 2024.
Vasilkov, A., Krotkov, N., Yang, E.-S., Lamsal, L., Joiner, J., Castellanos, P., Fasnacht, Z., and Spurr, R.: Explicit and consistent aerosol correction for visible wavelength satellite cloud and nitrogen dioxide retrievals based on optical properties from a global aerosol analysis, Atmos. Meas. Tech., 14, 2857–2871, https://doi.org/10.5194/amt-14-2857-2021, 2021.
Veefkind, J. P., Aben, I., McMullan, K., Förster, H., de Vries, J., Otter, G., Claas, J., Eskes, H. J., de Haan, J. F., Kleipool, Q., van Weele, M., Hasekamp, O., Hoogeveen, R., Landgraf, J., Snel, R., Tol, P., Ingmann, P., Voors, R., Kruizinga, B., Vink, R., Visser, H., and Levelt, P. F.: TROPOMI on the ESA Sentinel-5 Precursor: A GMES mission for global observations of the atmospheric composition for climate, air quality and ozone layer applications, Remote Sens. Environ., 120, 70–83, https://doi.org/10.1016/j.rse.2011.09.027, 2012.
Verhoelst, T., Compernolle, S., Pinardi, G., Lambert, J.-C., Eskes, H. J., Eichmann, K.-U., Fjæraa, A. M., Granville, J., Niemeijer, S., Cede, A., Tiefengraber, M., Hendrick, F., Pazmiño, A., Bais, A., Bazureau, A., Boersma, K. F., Bognar, K., Dehn, A., Donner, S., Elokhov, A., Gebetsberger, M., Goutail, F., Grutter de la Mora, M., Gruzdev, A., Gratsea, M., Hansen, G. H., Irie, H., Jepsen, N., Kanaya, Y., Karagkiozidis, D., Kivi, R., Kreher, K., Levelt, P. F., Liu, C., Müller, M., Navarro Comas, M., Piters, A. J. M., Pommereau, J.-P., Portafaix, T., Prados-Roman, C., Puentedura, O., Querel, R., Remmers, J., Richter, A., Rimmer, J., Rivera Cárdenas, C., Saavedra de Miguel, L., Sinyakov, V. P., Stremme, W., Strong, K., Van Roozendael, M., Veefkind, J. P., Wagner, T., Wittrock, F., Yela González, M., and Zehner, C.: Ground-based validation of the Copernicus Sentinel-5P TROPOMI NO2 measurements with the NDACC ZSL-DOAS, MAX-DOAS and Pandonia global networks, Atmos. Meas. Tech., 14, 481–510, https://doi.org/10.5194/amt-14-481-2021, 2021.
Vigouroux, C., Langerock, B., Bauer Aquino, C. A., Blumenstock, T., Cheng, Z., De Mazière, M., De Smedt, I., Grutter, M., Hannigan, J. W., Jones, N., Kivi, R., Loyola, D., Lutsch, E., Mahieu, E., Makarova, M., Metzger, J.-M., Morino, I., Murata, I., Nagahama, T., Notholt, J., Ortega, I., Palm, M., Pinardi, G., Röhling, A., Smale, D., Stremme, W., Strong, K., Sussmann, R., Té, Y., van Roozendael, M., Wang, P., and Winkler, H.: TROPOMI–Sentinel-5 Precursor formaldehyde validation using an extensive network of ground-based Fourier-transform infrared stations, Atmos. Meas. Tech., 13, 3751–3767, https://doi.org/10.5194/amt-13-3751-2020, 2020.
Wei, J., Liu, S., Li, Z., Liu, C., Qin, K., Liu, X., Pinker, R. T., Dickerson, R. R., Lin, J., Boersma, K. F., Sun, L., Li, R., Xue, W., Cui, Y., Zhang, C., and Wang, J.: Ground-Level NO2 Surveillance from Space Across China for High Resolution Using Interpretable Spatiotemporally Weighted Artificial Intelligence, Environ. Sci. Technol., 56, 9988–9998, https://doi.org/10.1021/acs.est.2c03834, 2022.
Williams, J. E., Boersma, K. F., Le Sager, P., and Verstraeten, W. W.: The high-resolution version of TM5-MP for optimized satellite retrievals: description and validation, Geosci. Model Dev., 10, 721–750, https://doi.org/10.5194/gmd-10-721-2017, 2017.
Yombo Phaka, R., Merlaud, A., Pinardi, G., Friedrich, M. M., Van Roozendael, M., Müller, J.-F., Stavrakou, T., De Smedt, I., Hendrick, F., Dimitropoulou, E., Bopili Mbotia Lepiba, R., Phuku Phuati, E., Djibi, B. L., Jacobs, L., Fayt, C., Mbungu Tsumbu, J.-P., and Mahieu, E.: Ground-based Multi-AXis Differential Optical Absorption Spectroscopy (MAX-DOAS) observations of NO2 and H2CO at Kinshasa and comparisons with TROPOMI observations, Atmos. Meas. Tech., 16, 5029–5050, https://doi.org/10.5194/amt-16-5029-2023, 2023.
Zhang, C., Liu, C., Chan, K. L., Hu, Q., Liu, H., Li, B., Xing, C., Tan, W., Zhou, H., Si, F., and Liu, J.: First observation of tropospheric nitrogen dioxide from the Environmental Trace Gases Monitoring Instrument onboard the GaoFen-5 satellite, Light-Sci. Appl., 9, 66, https://doi.org/10.1038/s41377-020-0306-z, 2020.
Zhang, Y., Lin, J., Kim, J., Lee, H., Park, J., Hong, H., Van Roozendael, M., Hendrick, F., Wang, T., Wang, P., He, Q., Qin, K., Choi, Y., Kanaya, Y., Xu, J., Xie, P., Tian, X., Zhang, S., Wang, S., Cheng, S., Cheng, X., Ma, J., Wagner, T., Spurr, R., Chen, L., Kong, H., and Liu, M.: A research product for tropospheric NO2 columns from Geostationary Environment Monitoring Spectrometer based on Peking University OMI NO2 algorithm, Atmos. Meas. Tech., 16, 4643–4665, https://doi.org/10.5194/amt-16-4643-2023, 2023.
Zhou, Y., Brunner, D., Spurr, R. J. D., Boersma, K. F., Sneep, M., Popp, C., and Buchmann, B.: Accounting for surface reflectance anisotropy in satellite retrievals of tropospheric NO2, Atmos. Meas. Tech., 3, 1185–1203, https://doi.org/10.5194/amt-3-1185-2010, 2010.
Zoogman, P., Liu, X., Suleiman, R. M., Pennington, W. F., Flittner, D. E., Al-Saadi, J. A., Hilton, B. B., Nicks, D. K., Newchurch, M. J., Carr, J. L., Janz, S. J., Andraschko, M. R., Arola, A., Baker, B. D., Canova, B. P., Chan Miller, C., Cohen, R. C., Davis, J. E., Dussault, M. E., Edwards, D. P., Fishman, J., Ghulam, A., González Abad, G., Grutter, M., Herman, J. R., Houck, J., Jacob, D. J., Joiner, J., Kerridge, B. J., Kim, J., Krotkov, N. A., Lamsal, L., Li, C., Lindfors, A., Martin, R. V., McElroy, C. T., McLinden, C., Natraj, V., Neil, D. O., Nowlan, C. R., O'Sullivan, E. J., Palmer, P. I., Pierce, R. B., Pippin, M. R., Saiz-Lopez, A., Spurr, R. J. D., Szykman, J. J., Torres, O., Veefkind, J. P., Veihelmann, B., Wang, H., Wang, J., and Chance, K.: Tropospheric emissions: Monitoring of pollution (TEMPO), J. Quant. Spectrosc. Ra., 186, 17–39, https://doi.org/10.1016/j.jqsrt.2016.05.008, 2017.
- Abstract
- Introduction
- Method and data
- Comparison of HCHO and NO2 columns between POMINO and RPRO products
- Sensitivity tests on AMF input parameters
- Uncertainty estimates
- Validation against global MAX-DOAS network and PGN measurements
- Conclusions
- Data availability
- Author contributions
- Competing interests
- Disclaimer
- Acknowledgements
- Financial support
- Review statement
- References
- Supplement
- Abstract
- Introduction
- Method and data
- Comparison of HCHO and NO2 columns between POMINO and RPRO products
- Sensitivity tests on AMF input parameters
- Uncertainty estimates
- Validation against global MAX-DOAS network and PGN measurements
- Conclusions
- Data availability
- Author contributions
- Competing interests
- Disclaimer
- Acknowledgements
- Financial support
- Review statement
- References
- Supplement