the Creative Commons Attribution 4.0 License.
the Creative Commons Attribution 4.0 License.
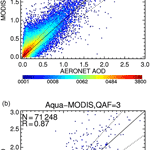
Validation of MODIS 3 km land aerosol optical depth from NASA's EOS Terra and Aqua missions
Lorraine A. Remer
Robert C. Levy
Shana Mattoo
In addition to the standard resolution product (10 km), the MODerate resolution Imaging Spectroradiometer (MODIS) Collection 6 (C006) data release included a higher resolution (3 km). Other than accommodations for the two different resolutions, the 10 and 3 km Dark Target (DT) algorithms are basically the same. In this study, we perform global validation of the higher-resolution aerosol optical depth (AOD) over global land by comparing against AErosol RObotic NETwork (AERONET) measurements. The MODIS–AERONET collocated data sets consist of 161 410 high-confidence AOD pairs from 2000 to 2015 for Terra MODIS and 2003 to 2015 for Aqua MODIS. We find that 62.5 and 68.4 % of AODs retrieved from Terra MODIS and Aqua MODIS, respectively, fall within previously published expected error bounds of ±(0.05 + 0.2 × AOD), with a high correlation (R= 0.87). The scatter is not random, but exhibits a mean positive bias of ∼ 0.06 for Terra and ∼ 0.03 for Aqua. These biases for the 3 km product are approximately 0.03 larger than the biases found in similar validations of the 10 km product. The validation results for the 3 km product did not have a relationship to aerosol loading (i.e., true AOD), but did exhibit dependence on quality flags, region, viewing geometry, and aerosol spatial variability. Time series of global MODIS–AERONET differences show that validation is not static, but has changed over the course of both sensors' lifetimes, with Terra MODIS showing more change over time. The likely cause of the change of validation over time is sensor degradation, but changes in the distribution of AERONET stations and differences in the global aerosol system itself could be contributing to the temporal variability of validation.
- Article
(9865 KB) - Full-text XML
- BibTeX
- EndNote
The MODerate resolution Imaging Spectroradiometer (MODIS) sensors, on board the Earth Observing System (EOS) Terra and Aqua satellites, have been providing observations of Earth and the atmosphere for almost two decades (Salomonson et al., 1989). These data have been used to create a long-term set of atmospheric aerosol properties including aerosol optical depth (AOD – a measure of aerosol loading in the total atmospheric column) (Kaufman et al., 1997; Levy et al., 2013). In particular, the Dark Target (DT) algorithms applied to MODIS observations provide aerosol retrievals over both ocean and dark vegetated land surfaces (Kaufman et al., 1997; Remer et al., 2005; Levy et al., 2007a, b, 2013). The DT products were designed with climate applications in mind and have been used to address a wide variety of geophysical science questions including the role of aerosols in climate-relevant processes (Kaufman et al., 2002; Christopher and Zhang, 2002; Yu et al., 2006), cloud and precipitation modifications (Koren et al., 2009, 2012; Yuan et al., 2011; Oreopoulos et al., 2016), and long-range transport of aerosols (Kaufman et al., 2005; Yu et al., 2012). Users have even applied the DT aerosol product to address needs for monitoring, evaluating, and forecasting air quality (al Saadi et al., 2005; Gupta et al., 2009; Van Donkelaar et al., 2015).
The MODIS DT algorithm produces an aerosol product, over land and ocean, at a nominal 10 × 10 km2 spatial resolution (referred to as “10 km” herein). This spatial resolution permits much selectivity in choosing which MODIS-measured reflectance pixels at 0.5 × 0.5 km2 resolution to include in the retrieval, and generally produces smooth and accurate fields of AOD and other aerosol parameters (Remer et al., 2012). By allowing the algorithm to discard up to 90 % of the available pixels and still produce a high-quality aerosol product, the algorithm avoids marginal situations unfavorable for an aerosol retrieval such as cloud fringes, fragments, and shadows, as well as land surfaces that do not agree with algorithm assumptions (Remer et al., 2012). The 10 km product has undergone lengthy evaluation and validation, updated after each major algorithm modification (Ichoku et al., 2002; Chu et al., 2002; Remer et al., 2002, 2005; Russell et al., 2007; Levy et al., 2005, 2010). Some of this evaluation was global in nature, while some was local to a particular field experiment, but all concerned the 10 km MODIS DT aerosol product.
For climate studies, the initial intention of the algorithm, 10 km spatial resolution was sufficient to characterize global and regional aerosol loading. However, as the community expanded the use of MODIS AOD to a wide variety of purposes, need arose for a finer-resolution product, and a nominal 3 × 3 km2 resolution (referred to as “3 km” herein) product was introduced as part of MODIS Collection 6 (Levy et al., 2013; Remer et al., 2013). The product is termed MYD04_3K for 3 km resolution aerosol parameters derived from the Aqua MODIS sensor and MOD04_3K for those derived from Terra MODIS. These products are produced operationally, over land and ocean, and the entire data records of Terra and Aqua have been reprocessed, creating a data record of almost two decades.
Before becoming operational, Remer et al. (2013) tested the algorithm by comparing 6 months of global 3 km retrievals from Aqua MODIS against available ground truth, while other independent studies (Munchak et al., 2013; Nichol and Bilal, 2016; He et al., 2017 and others) have done subsequent evaluation of the product regionally and locally. These limited comparisons suggested that the new AOD product would be sufficiently accurate to provide useful information and new perspective to the aerosol community. However, the studies also suggested that the finer-resolution product might introduce additional noise and/or bias that the original coarser-resolution product successfully avoided. Now that the multi-decadal 3 km product is operational and available publicly, it is time to perform a comprehensive evaluation of this finer-resolution MODIS DT aerosol product. We present here the results of an analysis of a comparison of the global long-term MODIS 3 km product with collocated AErosol RObotic NETwork (AERONET) (Holben et al., 1998) observations.
The MODIS DT algorithm and products are described in detail in Levy et al. (2013) and also in the MODIS DT online Algorithm Theoretical Basis Document (https://darktarget.gsfc.nasa.gov/atbd/overview, last access: 1 January 2018). In summary, to retrieve aerosol parameters over land, the algorithm makes use of the reflectances measured in three of MODIS' 36 spectral channels, 0.47, 0.65, and 2.1 µm (Levy et al., 2007a). These are provided in nominal spatial resolution of 0.5 × 0.5 km2 (at nadir), while other channels (some at 0.5 × 0.5 km2, some at 1 × 1 km2 resolution) are used for identifying appropriate surfaces for retrieval and for masking clouds, snow, and ice. While the 10 km standard product begins with an aggregation of 20 × 20 = 400 native-resolution pixels, the 3 km aerosol retrieval box starts with an aggregation of 6 × 6 = 36 such pixels. Native pixels are removed in order to retain only the ones most appropriate for a dark-target, over-land retrieval. Native pixels tagged as too bright for the DT algorithm, or identified as containing cloud, surface water, snow, or ice, are removed from the aggregation. The remaining native pixels are sorted from darkest to brightest, and the darkest 20 % and brightest 50 % of all remaining native pixels are removed as well. For the 3 km retrieval this means that at most 12 native pixels will remain and likely fewer. For minimum statistical robustness, the 3 km algorithm requires at least 5 native pixels (out of the initial 36). If there are insufficient native pixels (e.g < 5), output parameters are assigned fill values and no retrieval is attempted. Based on the aggregation and filtering, it is likely that there will be native pixels used by the 3 km retrieval that would have been discarded by the product with coarser (10 km) standard resolution (Remer et al., 2013). The remaining native pixels are averaged, leading to a single set of spectral reflectance values that drives the aerosol retrieval. Except for downstream decisions based on number of native pixels used, the 10 and 3 km retrievals proceed identically.
The retrieval uses a look-up table (LUT) procedure in which the LUT is constructed a priori of simulated top-of-atmosphere reflectances. LUT calculations use assumptions of aerosol optical properties based on AERONET inversions (Dubovik and King, 2000) and radiative transfer. The surface reflectance is constrained by assuming an empirically based relationship between reflectance at 2.1 µm and the reflectances at 0.65 and 0.47 µm (Levy et al., 2007a, b, 2013). The algorithm finds the AOD that minimizes the differences between the MODIS-observed mean spectral reflectances and the simulated reflectance values of the LUT. The primary output of the retrieval is the AOD at 0.55 µm. Using a series of tests, the algorithm assigns a quality assurance flag (QAF) of either 3, “good quality”, or 0, “bad quality”, to the retrieval. These values can be interpreted as “confidence” in the aerosol retrieval (whether the retrieval proceeded nominally and whether there are enough native-resolution pixels). For quantitative use of the 10 km product, the MODIS DT team has recommended limiting use to retrievals designated with QAF = 3. In the 10 km product there must be ≥ 51 native pixels surviving the selection process out of a possible 120 to reach this QAF level. The similar ratio for the 3 km product is ≥ 5 surviving pixels out of a possible 12. Fewer pixels would provide insufficient statistical information for confidence in an aerosol retrieval. Therefore, for the 3 km retrieval, a situation with fewer than 5 native pixels automatically receives the designation of “poor quality” (QAF = 0). For this resolution product there are no intermediate quality levels between 3 and 0 over land retrievals (Remer et al., 2013).
3.1 MODIS 3 km AOD
The primary data set of this study is the Collection 6 MODIS DT retrieved AOD at 3 km spatial resolution, derived from Terra reflectances (MOD04_3K), or Aqua reflectances (MYD04_3K), as described in Sect. 2. These are publicly available and can be downloaded from https://ladsweb.modaps.eosdis.nasa.gov/ (last access: 1 January 2018). Of the products in the data sets, we analyze only the AOD at 0.55 µm.
Applying identical algorithms to two different sensors does not guarantee identical results (Levy et al., 2015). The two MODIS DT data sets, one from Terra MODIS and one from Aqua MODIS, must be addressed separately as individual and independent products, even though they have been created from identical algorithms with no specific tuning of parameters for each sensor. While Terra MODIS and Aqua MODIS began as near-identical sensors, they have evolved over their lifetimes to develop their own instrumental characteristics. For example, some detectors in Aqua's detector array at some wavelengths have died, resulting in fewer available reflectance pixels at those wavelengths. Terra's detector array has not lost any detectors. At the same time, we have seen drift in some of Terra's wavelengths, resulting in measurable artificial trends in the Terra MODIS aerosol products (Levy et al., 2013; Sayer et al., 2015; Lyapustin et al., 2014). The most flagrant of those Terra MODIS trends have been mitigated by aggressive radiometric calibration (Toller et al., 2013), which has been applied in creating the C006 DT products. Note that some projects (e.g., Lyapustin et al., 2014; Sayer et al., 2015) have since introduced additional calibration drift mitigation. However, the DT retrieval has not applied these strategies. In this work, we will analyze the C006 aerosol products from the two MODIS sensors, independently, to provide users with clear information on the strengths and limitations of each one.
3.2 AERONET AOD
AERONET is NASA's global ground network of CIMEL sun–sky radiometers that make measurement of directly transmitted solar light and scattered sky light at several wavelengths during daylight hours (Holben et al., 1998). In this work, only the direct sun measurements will be used. The AERONET group processes these spectral measurements to derive AOD at the wavelengths corresponding to the direct sun measurements. The AERONET spectral AOD product is a community standard for satellite-derived AOD validation, given that AERONET's AOD uncertainty of 0.01–0.02 (Eck et al., 1999) is sufficiently more accurate and precise than can be expected by any satellite retrieval. The typical temporal frequency of direct sun measurements is every 15 min. The network consists of hundreds of stations, located globally, across all continents and in a wide variety of aerosol, meteorological, and surface type conditions. Only stations that sufficiently represent land areas will be used here, which means we are not comparing with observations taken on small islands, ocean platforms, or mobile ships.
The configuration of the spectral bands varies, but typically is centered at 0.34, 0.38, 0.44, 0.50, 0.67, 0.87, and 1.02 µm. Here we use a quadratic log–log fit (Eck et al., 1999) to interpolate AERONET AOD to 0.55 µm to match the primary MODIS AOD product. AOD data from AERONET are reported for three different quality levels: unscreened (Level 1.0), cloud screened (Level 1.5), and cloud screened and quality assured (Level 2.0). We will only use Version 2.0 Level 2.0 AERONET AODs in this study.
The validation procedure requires calculating the spatiotemporal statistics of a collocated MODIS-retrieved and AERONET-measured AOD pair (Ichoku et al., 2002; Petrenko et al., 2012; Munchak et al., 2013; Remer et al., 2012). We have created a collocated data set (CDS) of AOD at 0.55 µm from both Terra MODIS and Aqua MODIS, matched with AERONET, for nearly the entire mission (2003–2015 for Aqua and 2000–2015 for Terra). From here on, we use the term “pixels” to refer to the MODIS retrieval product (e.g., 3 or 10 km resolution); if referring to the native MODIS pixel resolution (e.g., 0.5 km) we will denote it as “native pixel”.
In previous validation studies of the standard 10 km product the spatial statistics were based on groupings of either 5 × 5 MODIS product pixels (∼ 50 × 50 km2 box) centered on the AERONET station (Ichoku et al., 2002; Levy et al., 2010) or all the MODIS product pixels within a 27.5 km radius around the AERONET station (Petrenko et al., 2012). These spatial statistics would be matched with the temporal statistics of ±30 min of AERONET observations centered at satellite overpass time. These large spatial collocation boxes will not properly test the accuracy of finer-resolution satellite products to represent small-scale aerosol gradients. Therefore, Remer et al. (2013) and Munchak et al. (2013) moved to a 7.5 km radius and ±30 min satellite overpass. The 7.5 km radius encompasses roughly 25 AOD pixels at nadir, which is analogous to the number of product pixels used with the coarser-resolution product. In this study spatial statistics are calculated from all MODIS product pixels falling within a box of 0.15∘ × 0.15∘ (latitude × longitude) centered over an AERONET location. Except for polar regions, this is similar to a 15 × 15 km2 box or 7.5 km radius at nadir. Temporal statistics are calculated from all AERONET observations of AOD within ±30 min of satellite overpass.
As recommended by the MODIS DT science team (Levy et al., 2010), unless otherwise specified, only AOD pixels with quality assurance flag “very good” (QAF = 3) are included in averaging over the AERONET sites. To be consistent with previous validation exercises (Levy et al., 2010), we have retained the CDSs only when there were at least 5 MODIS product pixels (out of a possible 25) and 2 AERONET measurements (out of a possible 2–4). The CDS consists of 574 AERONET stations with 90 162 collocated pairs for Terra MODIS and 71 248 collocated pairs for Aqua MODIS. Figure 1 shows the locations of these stations and the color-coding represents the number of collocated AERONET–MODIS AOD pairs over the station.
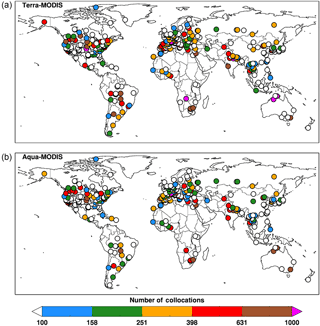
Figure 1Locations of AERONET stations used in the validation study. The color scale shows the number of coincident MODIS–AERONET data points over each station for the entire period. Panel (a) is for Terra MODIS and panel (b) for Aqua MODIS. Most stations operated for only a subset of the 13- to 15-year record.
Thus, a data set (i.e., CDS) of collocated MODIS–AERONET pairs of AOD at 0.55 µm is created that can be organized and subsampled in any number of configurations. In any subsample, or for the entire data set, these ordered pairs can be plotted, one against the other, to create a scatterplot, and collocation statistics are calculated. We will use the following statistical parameters to quantify how well the MODIS retrievals match their collocated AERONET counterparts (Hyer et al, 2011):
-
correlation coefficient (R),
-
slope of the linear regression line, and
-
root mean square error (RMSE).
The percentage of collocations falling within expected error (EE) is calculated as
The error ratio (ER) is calculated as
The coefficients in the EE equation were determined from evaluation of the 3 km product over the 6 months of Aqua data analyzed by Remer et al. (2013). Those limited results suggested that expected error bounds should be broadened to the values seen in Eq. (2) from those derived for the 10 km product (EE = ±[0.05 + 0.15 × AERONET AOD]).
The number of collocations (N) is another parameter used to evaluate the 3 km retrieval in the CDS.
Table 1Global statistics of comparison between MODIS 3 km AOD at 0.55 µm retrievals and collocated AERONET observations for both Terra and Aqua, corresponding to three quality assurance categories (QAF = 0 for poor quality, QAF = 0, 3 for all quality, and QAF = 3 for high quality). The data used for the 10 km validation do not represent the same time period as 3 km. Terra MODIS 10 km data period is March 2000 to June 2013 whereas Aqua MODIS 10 km data period is January 2003–June 2013.
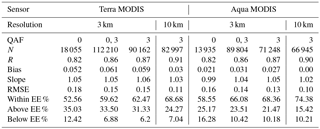
5.1 Global statistics
We first compare MODIS 3 km AOD retrievals against collocated AERONET values (Fig. 2), for both the recommended “high-quality” retrievals (QAF = 3) and for all the retrievals, regardless of quality, keeping Terra and Aqua results separate. Note that the 3 km product only tags data as either high quality or low quality. Table 1 presents the statistical parameters corresponding to this analysis while considering various combinations of QAFs.
Globally, there is strong correlation between MODIS 3 km AOD and collocated AERONET equivalents. However, there is scatter and a positive bias to the retrievals, more so for Terra than Aqua even though the correlation is similar between the satellites. The retrieval quality identified by the algorithm corresponds well to the product accuracy as determined by collocation with AERONET observations. Algorithm-identified high-quality retrievals (QAF = 3) have stronger correlation, smaller RMSE and more retrievals falling within expected error than do the low-quality (QAF = 0) retrievals (Table 1). However, the high-quality data set contains about 20 % fewer retrievals than does the total data set with retrievals of all quality levels included. Figure 3 shows that the differences between Terra and Aqua in how they match AERONET values are much more apparent than the differences between QAF levels of the same satellite sensors. We note that only the high-quality (QAF = 3) Aqua 3 km retrievals meet expectations in terms of falling within the standard expected error bars (Remer et al., 2012; and Eq. 2).
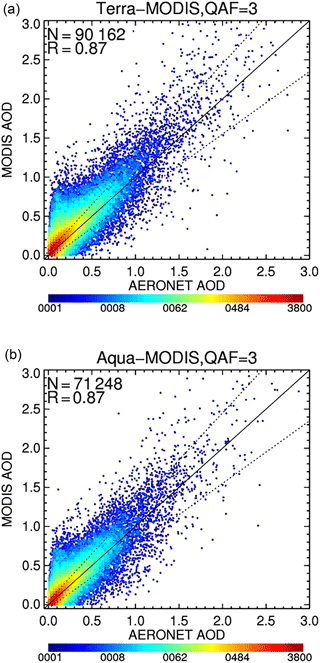
Figure 2Two-dimensional density scatterplot of MODIS 3 km AOD versus AERONET-observed AOD at 0.55 µm for the global collocation data set. Panel (a) is for Terra MODIS for only the retrievals identified as “high quality” (QAF = 3), and panel (b) is for Aqua MODIS for QAF = 3. The solid line denotes the 1:1 line, and the dashed lines denote the envelope of the expected error (EE), defined by Eq. (2).
Table 1 also shows the corresponding validation statistics for the 10 km product for QAF = 3, distinguishing between Terra and Aqua. The 10 km product, as expected, more closely matches AERONET values, having higher correlation, lower bias, and RMSE and producing more retrievals that fall within expected error bounds than does the 3 km product. We note that even in the 10 km validation statistics, mean bias for Terra is 0.03 higher than for Aqua, which is the same difference between sensors as found for the 3 km product. The results in Table 1 confirm the conclusion of Remer et al. (2013) that the 3 km product is less accurate than the standard 10 km product. The remainder of the paper will be devoted to exclusively analyzing the differences between the 3 km product and AERONET, without further reference to the standard 10 km product.
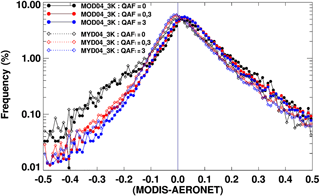
Figure 3Global distribution of mean bias in MODIS 3 km AOD retrievals with respect to collocated AERONET observations. The circular dots with solid lines are for Terra values and diamond dots with dotted lines are for Aqua values. The colors vary for the three quality levels (QAF = 0, poor quality; QAF = 3, high quality; and QAF = 0 and 3, all quality).
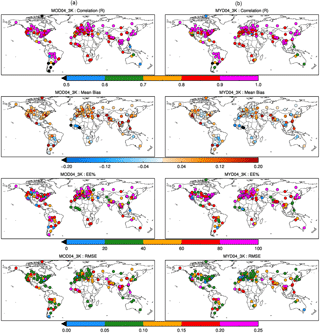
Figure 4Statistics calculated from the collocation database at each AERONET station, individually, for Terra on the left and Aqua on the right. Shown are values for correlation coefficient (R), mean bias, percentage within expected error (EE %), and RMSE. Only stations with at least 100 collocations are plotted, which may differ between the two satellites, and only collocations with MODIS retrievals of QAF = 3 are included.
5.2 Regional statistics
The accuracy of the 3 km AOD retrievals will be regionally and locally specific, depending on how well retrieval assumptions of surface and aerosol optical properties match actual conditions. Local cloud conditions also may introduce uncertainty into the retrieval. Furthermore, the spatial–temporal variability of the area may create biases in the collocation methodology that depends on assumptions of aerosol homogeneity. Here we investigate how well the MODIS 3 km product matches AERONET over individual AERONET stations.
For the regional and local analyses, we will use only QAF = 3 retrievals and calculate the same collocation statistics for each station individually. Figure 4 plots the values for correlation coefficient, mean bias, percentage within expected error, and RMSE for each station that reported at least 100 collocations over the entire time series. In general, the MODIS 3 km retrievals show high correlations over much of the northern midlatitudes where there are AERONET stations in abundance. Correlation is weaker at some stations in California and the arid southwest of North America, in the Caribbean, Central America, insular SE Asia, Australia, and especially in southern South America. These are locations where the standard 10 km product also shows poor agreement with AERONET (https://darktarget.gsfc.nasa.gov/validation/maps, last access: 1 January 2018). In most of these regions, like the arid southwest of North America, the surface properties do not agree with the assumptions used in the global retrieval, thereby introducing error in the retrieval.
Not all stations with strong correlations exhibit small mean biases. For example, MODIS 3 km retrievals severely under predict AOD in the stations of West Africa, falling well below expected error there, even though those stations report high correlations with AERONET. Such a validation pattern is symptomatic of incorrect assumptions of aerosol properties. In West Africa, the interplay of heavy dust and heavy smoke, often occurring simultaneously in the atmospheric column at the same time, creates difficult situations to properly model in the aerosol retrieval. Likely the poor agreement between MODIS and AERONET there can be attributed to this difficulty. Stations in Australia show relatively small mean biases and high percentages meeting expectations, despite poor correlations. This apparent contradiction suggests that the poor correlations are the result of small dynamic range in the scatterplots that occur when AOD is consistently low.
In Fig. 4, we see the local nature of the validation statistics. Stations in close proximity to each other sometimes report very different statistics. For example, the stations clustered across northern India and those in an array across central South America (Brazil) range from strong positive to negative mean biases and RMSE from 0.05 to 0.20, even though these groupings of stations will fall within the same region as defined in Fig. 5. This is apparent in almost any region. Some of this variability may be due to differences in the temporal extent of the AERONET record at each individual station, so that even if stations are in close proximity in space they may actually be making measurements in entirely different years or seasons. Other differences may be related to topography, urban surfaces, or other factors. Still, the variability seen in Fig. 4 shows how local conditions, and possibly the individual characteristics of the time series, affect validation statistics.
Table 2Regional statistics of intercomparison between MODIS and AERONET. This is using joint data sets of Terra and Aqua for QAF = 3 only.
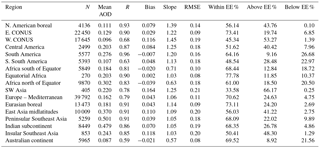
The final point to note in Fig. 4 is the difference between Terra and Aqua. For example, in the mean bias plots we see how the mean bias across the North American central plains decreases from approximately positive 0.04–0.05 for Terra to slightly negative for Aqua. For many of the stations, positive mean AOD biases are higher for Terra than for Aqua. This is in agreement with the global statistics presented in Table 1.
We next group individual stations into 17 regions, defined following Hyer et al. (2011). These are shown in Fig. 5 with Table 2 presenting the regional validation statistics for each of the defined regions. We know from previous analyses presented above that there are distinctive differences between Terra and Aqua mean biases; however, in calculating the regional statistics of Table 2, we combine Terra and Aqua collocations.
The majority of collocations are found in the northern midlatitude regions, with E. and W. CONUS (east and west continental United States) representing 25 % of the total collocations and Mediterranean Europe and boreal Eurasia representing another 34 % of the total. MODIS 3 km retrievals from E. CONUS, Mediterranean Europe, and boreal Eurasia show very good overall agreement with AERONET, exhibiting R ≥ 0.78, bias ≤ 0.05, and at least two-thirds of retrievals falling within expected error. W. CONUS retrievals agree with AERONET less well, exhibiting some of the highest positive biases of any region on the globe. These four regions drive the global validation statistics, which reflect both the good agreement of E. CONUS and Europe and the high bias of W. CONUS. Validation statistics are especially poor in southwest Asia, where there are very few stations and collocations.
5.3 Error dependencies
We next explore the relationship between MODIS–AERONET 3 km AOD differences and various parameters for the global collocation data set. At each collocation, the AERONET AOD is subtracted from the MODIS AOD, so that a positive difference indicates a positive MODIS bias. The data are then sorted according a particular parameter in the database. Collocations are grouped into 87 bins for Terra and 67 bins for Aqua, each containing 1000 collocations. Thus, there are equal numbers of collocations in each bin, but the bins are not equally spaced along the x axis. The mean, median, and standard deviations (SD) of the MODIS–AERONET differences are calculated for each bin.
Figure 6 shows the results of this analysis as a function of AOD, both the true AOD, as measured by AERONET, and the MODIS-retrieved AOD. The differences between MODIS and AERONET AOD depend very little on the true AOD. There is some suggestion of a positive–negative–positive shift of differences at the very lowest AOD (< 0.1), but overall the differences are flat. Terra exhibits an overall positive mean bias against AERONET of about 0.06, with the bias in Aqua much less noticeable. We plot these differences against the MODIS-retrieved AOD to create a metric of retrieval accuracy that can be used to evaluate individual MODIS AOD retrievals in the absence of AERONET. Here we see a distinctive pattern between MODIS AOD bias and MODIS AOD. The higher the retrieved AOD is, the greater the positive difference between MODIS and AERONET. Significant biases of > 0.10 are seen for MODIS AOD values > 0.40. For retrieved AOD < 0.10, the mean differences between MODIS and AERONET are negative. This indicates that a high value of retrieved AOD has greater probability of being too high than too low, and a low value of retrieved AOD has a greater probability of being too low than too high. These results are expected, as high AOD retrievals are more sensitive to true aerosol properties whereas true surface properties become more important in low AOD retrieval.
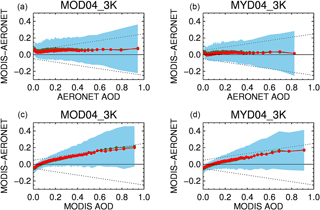
Figure 6AOD differences between the MODIS 3 km product and AERONET for the global collocation data set, QAF = 3, as a function of AERONET AOD (a, b) MODIS AOD (c, d). The left column shows Terra values and the right column shows Aqua. The global data set was sorted according to AOD, binned into bins with equal number of collocations, and then mean, median, and standard deviation of each bin were calculated. Red dots and lines show the mean. Black dots and lines show the median. The blue cloud indicates 1 standard deviation of each bin. The horizontal black line denotes zero difference, and the dashed lines indicate EE envelopes. Positive values indicate that MODIS AOD is higher than AERONET.
Panel a of Fig. 7 shows how MODIS–AERONET AOD differences vary as a function of AOD variability. SD of the retrievals in the 5 × 5 collocation box is a measure of the homogeneity of the aerosol across the box. The collocation methodology assumes that MODIS spatial statistics will match AERONET temporal statistics, which holds best if the aerosol field is homogeneous. As variability across the box increases (i.e., SD of the AOD), we expect differences between MODIS AOD and AERONET AOD to grow. We see from Fig. 7a that differences are increasingly positive as variability increases. This is because the SD is not normalized, and the differences increase simply because the AOD is increasing as it does in Fig. 6.
Another test of the collocation methodology assumptions is to look for error dependencies on the number of MODIS retrievals within the 5 × 5 collocation box. Note that the methodology requires at least 5 retrievals to represent the box and may have as many as 25. We see from Fig. 7b that there are dependencies. Fewer numbers of retrievals are associated with positive differences, but having almost all of the 25 retrievals available is associated with negative differences. We understand how collocation statistics might be skewed by having fewer numbers of retrievals available to match AERONET, especially if the aerosols across the collocation box were not spatially homogeneous. Also fewer numbers of retrievals may be a result of marginal retrieval conditions caused by clouds and unfavorable surface conditions. It is less easy to understand the negative differences when the box is especially well represented with sufficient retrievals, and this require further investigation on individual situations.
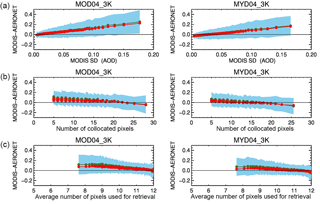
Figure 7Same as Fig. 6 except for standard deviation of MODIS AOD within the 5 × 5 collocation box (a), number of MODIS retrievals with the 5 × 5 collocation box (b), and number of MODIS reflectance native pixels used by the retrieval, averaged for all retrievals made in the 5 × 5 collocation box (c).
Panel c of Fig. 7 shows the MODIS–AERONET AOD differences as a function of the average number of native pixels (0.5 km) used by the MODIS 3 km retrieval in producing a value of AOD. The retrieval begins with 36 native pixels, and after masking, sorting, and discarding between 5 and 12 pixels remain. The number of pixels used by the retrieval is an indication of how much masking was required. If 12 pixels remain, then no masking was required, and the situation is cloud-free and taking place over favorable surfaces. If only 5 pixels remain, there are conditions that could raise concerns. In Fig. 7, we see that the fewer the pixels used by the retrieval (i.e., more masking is needed), the higher the positive bias is, especially for Terra. This suggests, in the Terra retrieval, that clouds or unfavorable surface conditions are contributing to the high bias seen in the global data set. Interestingly, MODIS–AERONET differences are negative when masking is at a minimum, similar as to when the collocation box contains almost all possible retrievals. It seems that cloud-free situations with appropriate surface features are associated with MODIS under predicting AERONET AOD. The same functional relationship is apparent in the Aqua data set also, but the biases, both high and low, are less pronounced.
Figure 8 shows the MODIS–AERONET AOD differences as a function of geometry. Panel a plots the differences against scattering angle, where we see positive bias increasing towards the extreme backscattering angles. The functional relationship is similar in both Terra and Aqua, but Terra's positive bias is more pronounced. Panel b plots the differences against sensor view (i.e., zenith) angle, where the Aqua differences show little dependence on view angle, but the Terra differences increase positive biases in near-nadir views. Geometrical dependencies in bias generally point to systematic inaccuracies in retrieval assumptions. These can be either in terms of surface angular functions or in aerosol optical properties. However, the difference between Terra and Aqua sensor zenith angle dependencies suggests an issue with instrument characterization, which could include geometrical functionality due to the need to calibrate across the scan mirror.
5.4 Temporal changes
Examining temporal changes of validation statistics across the entire time series of the collocation database further characterizes the accuracy of the 3 km AOD product. Figure 9 plots monthly mean error ratios (Eq. 3) and number of collocations for the time series of Terra (red) and Aqua (blue) separately. The ER compare the actual error (bias) to the EE. The −1 ≤ ER ≤ 1 means the actual errors are smaller than EE, whereas |EE| > 1 indicates a poor match. Even if the MODIS sensors and the algorithm were entirely consistent during the time series, AERONET stations go on- and offline. This causes global validation statistics to shift in local and regional emphasis and introduces temporal variation in the global results. Therefore, we have selected 26 AERONET stations (Table 3, Fig. 9) with long-term data records with consistent collocation over the entire time series for this analysis. The analysis over these selected stations allows us to examine the change in bias (and ER) over a longer time period without change in spatial and temporal distribution of AERONET stations. Only QAF = 3 retrievals are used. During the 15 years of the collocation data set many factors have changed. For example, satellite sensor characterization is an ongoing process that employs several different measures to monitor radiometric drift and then continuously adjusts calibration parameters to compensate for that drift. Thus, even though the algorithm remains consistent throughout the data record, the inputs to that algorithm may not be, despite the best efforts of the MODIS Characterization Team.
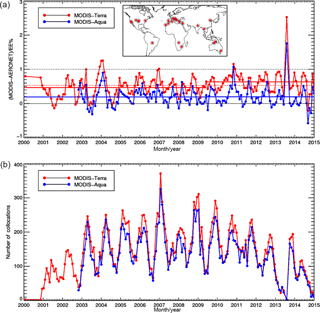
Figure 9Time series of monthly mean error ratios (Eq. 3) (a) and number of collocations (b) for the global collocation data set from 26 selected long-term AERONET stations. The Terra record is in red, and Aqua in blue. Note that Aqua's record begins 2 years after Terra, and the total number of collocations is temporally variant. Only MODIS 3 km retrievals with QAF = 3 are included. The horizontal red and blue lines are temporal means of ER. Dotted red and blue horizontal lines indicate long-term temporal mean ER for each satellite. Solid red lines are temporal mean ER calculated for Terra for two periods (2000–2010) and (2011–2015). The map in the inset shows locations of AERONET stations used in this analysis with more details provided in Table 3.
The time series of the monthly statistics shows strong seasonal variation of mean bias and number of collocations. Strong positive bias occurs in the April–August time period, followed by low or even negative bias in the October–February period. In addition to the seasonal variability, Fig. 9 also exposes long-term temporal trends. There is a steady increase of the number of collocations per month, as the AERONET network expands over time. The number of collocations nearly doubles from the early years, up and through the beginning of 2012. The last few years of the record show a decrease in collocations, in some part attributed to the lag in promoting AERONET records from Level 1.5 to Level 2.0. We only use AERONET Level 2.0 for collocations.
The temporal mean biases for the entire time series are 0.04 and 0.014 for Terra and Aqua, respectively, corresponding to temporal mean ER of 0.55 and 0.2, respectively. The mean biases also exhibit temporal trends with biases beginning to increase around 2008–2009. The bias for Terra increased from 0.038 to 0.048 whereas these numbers for Aqua are 0.014 and 0.016. The corresponding ER increase for Terra in 2008 is from 0.48 to 0.65. The increase in ER for Aqua is negligible.
The systematic higher biases exhibited by Terra as compared with Aqua agree with the global analysis presented above. This offset in bias between the two MODIS sensors appears systematic from the beginning of the Aqua record to the end of the time series, although the magnitude of that offset increases over time as Terra's biases grow. The systematic greater number of collocations in the Terra data set than in Aqua's may result from diurnal cloud patterns that create cloudier conditions in the afternoon during Aqua overpass than during Terra's morning one. More clouds in the afternoon (King et al., 2013) may reduce the number of possible collocations. However, instrumental differences affecting available retrievals are another possibility.
To validate the MODIS 3 km AOD products (MOD04_3K and MYD04_3K), which became publicly available in the MODIS Collection 6 release, we created a database of collocations of the product with AERONET observations. The collocation data set spanned the extent of the MODIS record from 2000 to 2015. Collocation criteria employed 0.15 × 0.15 degree latitude × longitude MODIS retrievals centered at the AERONET station and all AERONET observations ±30 min of satellite overpass. Thus, the collocation box is approximately 15 km per side for nadir views. Version 2, Level 2.0, cloud-screened and quality-assured AERONET observations are used, and AERONET AOD is interpolated to 0.55 µm to match MODIS values. Overall there are over 90 162 high-quality collocations of Terra retrievals and over 71 248 high-quality collocations for Aqua.
The validation statistics examined include mean bias, regression slope, correlation coefficient, and percentage falling within expected error bounds. In this validation exercise we hold the 3 km AOD product to expected error thresholds of ±(0.05 + 20 %) (Remer et al., 2013). We find that the global 3 km AOD product displays skill in matching AERONET observations with a correlation coefficient of 0.87, but there is a RMSE of 0.15 and 0.13 for Terra and Aqua, respectively. The scatter is not random, but exhibits a mean positive bias of 0.06 for Terra and 0.03 for Aqua. The Remer et al. (2013) error bounds capture two-thirds of the Aqua 3 km AOD retrieval, but less than 63 % of the Terra retrievals. There is significant degradation of validation accuracy if MODIS retrievals of poor data quality (QAF = 0) are included in the analysis. Thus, on a global basis we recommend using only QAF = 3 MODIS 3 km retrievals for quantitative analysis. If doing so, then the expected error for the Aqua product is ±(0.05 + 0.20 × AOD), on a global basis, but only ±(0.06 + 0.20 × AOD) for Terra, where AOD is the true AOD. However, a more accurate representation of Terra's expected error is to account for the positive bias with asymmetrical error bounds: −0.03–0.20 and +0.13 + 0.20 AOD. The expected error bounds contain two-thirds of all AOD retrievals. To assess the mean bias of the retrieval based on the retrieved AOD, we find that the mean bias can be modeled as 0.19 + 0.17 × ln(AOD_MODIS + 0.25) for Terra and 0.15 + 0.14 × ln(AOD_MODIS + 0.25) for Aqua. Note that mean bias itself is subject to uncertainty.
We find a wide range of accuracy in the 3 km product locally and regionally, with spatially contiguous stations sometimes exhibiting significantly different validation statistics. The distribution of validation sites is highly skewed towards the northern midlatitudes with over 50 % of all collocations in the database resulting from these areas. Within the northern midlatitudes, eastern North America, Europe, and boreal Eurasia show some of the best agreement with AERONET. However, western North America, also in the northern midlatitudes, exhibits some of the poorest agreement. Regions outside of the northern midlatitudes are less well represented in the database, but we find that north–central South America including the Amazon, equatorial–southern Africa, and Australia show good agreement with AERONET. Mexico, the Caribbean, southern South America, SW Asia, East Asia, and the maritime continent of Southeast Asia generally show poor agreement. No attempt was made to isolate urban regions from rural ones or to otherwise sort the data by surface type.
The difference between MODIS-retrieved 3 km AOD and AERONET-observed values are mostly independent of true AOD. This is unexpected as error bounds are defined as a function of the percentage of AOD ±(0.05 + 0.20 × AOD). However, the mean differences between MODIS 3 km AOD and AERONET are dependent on AOD variability. The more variable the AOD is, the higher the positive offset between MODIS and AERONET. Some of this is due to the conditions of the original MODIS retrieval, and some is due to the difficulties of a spatiotemporal match-up in the collocation methodology. We also find that the greater the need for masking clouds and unfavorable surfaces in the original retrieval, the greater the offset between MODIS and AERONET. Interesting and unexplained is the tendency for the differences between MODIS and AERONET to go negative when conditions appear to be homogeneous and cloud-free. We also find error dependencies on geometry, with greater error in the far backscattering region, and, for Terra only, greater error in near-nadir views. Some of these geometrical errors are introduced by uncertainties in the assumptions of surface characteristics and aerosol optical properties in the MODIS retrieval, but the difference between Terra and Aqua suggests differences in the sensors themselves.
We continue to see differences between the sensors in how validation statistics have evolved over time. By limiting our time series analysis to only 26 AERONET stations that span the entire time series, we eliminate changes in validation statistics due to changing AERONET station distribution. We find that both sensors exhibit time series with strong seasonal dependence. Both sensors have higher positive biases against AERONET in the northern spring and summer than in northern fall and winter, with Terra's positive bias always greater than Aqua's. However, during the early years of the time series, both sensors were reporting similar number of retrievals falling within expected error. This changed during 2007–2009, when Terra's accuracy began to fall off and its positive biases increased. Aqua's bias against AERONET also increased during this time frame, but not as rapidly as Terra's. While, these drifts in validation accuracy suggest changes in characterization accuracy of the MODIS sensors themselves, there are other factors. The number of collocations has fallen off towards the end of the time series. We attribute this to a lag for AERONET observations to be elevated to Level 2 status. Because of this lag, there may in fact be a change in the distribution of AERONET stations in the temporal collocation database, despite our best intentions. This may introduce a temporal trend in the validation statistics. Furthermore, the aerosol system itself has undergone significant changes since 2000, with the US and Europe drastically reducing their urban and industrial emissions and substituting other types of aerosol such as wildfire smoke in the western US, biogenic particles during the summer in the southeastern US (Hand et al., 2014; Toon et al., 2016), and transported Saharan dust around the Mediterranean (Karnieli et al., 2009). Likewise emissions and resulting AOD from other regions experience both long-term trends and interannual variability. The combination of variations in AERONET station distribution and the changing aerosol system over the time series examined may be contributing to the trends seen in the validation statistics. However, the differences between Terra and Aqua are difficult to explain without pointing to sensor characterization stability.
The standard 10 km product that meets expected error at 67 and 74 % levels for Terra and Aqua, respectively, on a global basis is measurably more accurate than the 3 km product examined here in detail. Similarly the global standard 10 km AOD product exhibits half of the mean bias with Terra and no bias at all for Aqua. These validation statistics for the 10 km standard product are preliminary. Once a more comprehensive evaluation of the 10 km Collection 6 product is completed, these validation statistics are likely to change. The 10 km product numbers are provided here only to lend perspective to our results with the finer-resolution product. Given this perspective, we confirm the Remer et al. (2013) recommendation that users whose interests are global should use the more robust and accurate 10 km product and leave the 3 km product for specific applications that require the finer-resolution representation of the AOD field.
This validation study only addressed the 3 km AOD product over land and did not evaluate the over-water product. The study took a global and regional view, not a local one. Users of the product on a local level are encouraged to consider particular biases that may occur due to local conditions. For example, we know that the MODIS Collection 6 DT AOD retrieval is systematically biased over urban surfaces (Gupta et al., 2016). This is true for both the 10 and 3 km DT products. This problem has been addressed and is substantially mitigated with the release of the Collection 6.1 version of the algorithm (Gupta et al., 2016). In the meantime, the results here show that overall the DT MODIS Collection 6 algorithm is producing an AOD product at 3 km resolution with sufficient accuracy and with well-characterized biases. The product can now be used quantitatively in a wide variety of science and practical applications.
The AERONET team (GSFC and site PIs) are thanked for the creation and continued stewardship of the sun photometer data record, which is available from http://aeronet.gsfc.nasa.gov (Holben, 2018).
The authors declare that they have no conflict of interest.
This work was supported by the NASA ROSES program NNH13ZDA001N-TERAQEA:
Terra and Aqua – Algorithms – Existing Data Products and NASA's EOS
program managed by Hal Maring. We thank MCST for their efforts to maintain
and improve the radiometric quality of MODIS data and LAADS/MODAPS for the
continued processing of the MODIS products.
Edited by: Manfred Wendisch
Reviewed by: two anonymous referees
Al-Saadi, J., Szykman, J., Pierce, R. B., Kittaka, C., Neil, D., Chu, D. A., Remer, L. A., Gumley, L., Prins, E., Weinstock, L., MacDonald, C., Wayland, R., Dimmick, F., and Fishman, J.: Improving national air quality forecasts with satellite aerosol observations, B. Am. Meteorol. Soc., 86, 1249–1261, https://doi.org/10.1175/BAMS-86-9-1249, 2005.
Christopher, S. A. and Zhang, J.: Shortwave aerosol radiative forcing from MODIS and CERES observations over the oceans, Geophys. Res. Lett., 29, 1859, https://doi.org/10.1029/2002GL014803, 2002.
Chu, D. A., Kaufman, Y. J., Ichoku, C., Remer, L. A., Tanre, D., and Holben, B. N.: Validation of MODIS aerosol optical depth retrieval over land, Geophys. Res. Lett., 29, 1617, https://doi.org/10.1029/2001GL013205, 2002.
Dubovik, O. and King, M. D.: A flexible inversion algorithm for retrieval of aerosol optical properties from Sun and sky radiance measurements, J. Geophys. Res., 105, 20673–20696, 10.1029/2000JD900282, 2000.
Eck, T. F., Holben, B. N., Reid, J. S., Dubovik, O., Smirnov, A., O'Neill, N. T., Slutsker, I., and Kinne, S.: Wavelength dependence of the optical depth of biomass burning, urban, and desert dust aerosols, J. Geophys. Res., 104, 31333–31349, 1999.
Gupta, P. and Christopher, S. A.: Particulate matter air quality assessment using integrated surface, satellite, and meteorological products: Multiple regression approach, J. Geophys. Res., 114, D14205, https://doi.org/10.1029/2008JD011496, 2009.
Gupta, P., Levy, R. C., Mattoo, S., Remer, L. A., and Munchak, L. A.: A surface reflectance scheme for retrieving aerosol optical depth over urban surfaces in MODIS Dark Target retrieval algorithm, Atmos. Meas. Tech., 9, 3293–3308, https://doi.org/10.5194/amt-9-3293-2016, 2016.
Hand, J. L., Schichtel, B. A., Malm, W. C., Copeland, S., Molenar, J. V., Frank, N., and Pitchford, M.: Widespread reductions in haze across the United States from the early 1990s through 2011, Atmos. Environ., 94, 671–679, https://doi.org/10.1016/j.atmosenv.2014.05.062, 2014.
He, Q., Zhang, M., Huang, B., and Tong, X.: MODIS 3 km and 10 km aerosol optical depth for China: Evaluation and comparison, Atmos. Environ., 153, 150–162, https://doi.org/10.1016/j.atmosenv.2017.01.023, 2017.
Holben, B.: NASA Goddard Space Flight Center, available at: http://aeronet.gsfc.nasa.gov, last access: 1 January 2018.
Holben, B. N., Eck, T. F., Slutsker, I., Tanre, D., Buis, J. P., Setzer, A., Vermote, E., Reagan, J. A., Kaufman, Y. J., Nakajima, T., Lavenu, F., Jankowiak, I., and Smirnov, A.: AERONET – A federated instrument network and data archive for aerosol characterization, Remote Sens. Environ., 66, 1–16, 1998.
Hyer, E. J., Reid, J. S., and Zhang, J.: An over-land aerosol optical depth data set for data assimilation by filtering, correction, and aggregation of MODIS Collection 5 optical depth retrievals, Atmos. Meas. Tech., 4, 379–408, https://doi.org/10.5194/amt-4-379-2011, 2011.
Ichoku, C., Chu, D. A., Mattoo, S., Kaufman, Y. J., Remer, L. A., Tanré, D., Slutsker, I., and Holben, B. N.: A spatio-temporal Approach for Global Validation and Analysis of MODIS Aerosol Products, Geophys. Res. Lett., 29, 1616, https://doi.org/10.1029/2001GL013205, 2002.
Karnieli, A., Derimian, Y., Indoitu, R., Panov, N., Levy, R. C., Remer, L. A., Maenhaut, W., and Holben, B. N.: Temporal trend in anthropogenic sulfur aerosol transport from central and eastern Europe to Israel, J. Geophys. Res., 114, D00D19, https://doi.org/10.1029/2009JD011870, 2009.
Kaufman, Y. J., Tanre, D., Remer, L., Vermote, E., Chu, A., and Holben, B. N.: Operational remote sensing of tropospheric aerosol over land from EOS moderate resolution imaging spectroradiometer, J. Geophys. Res.-Atmos., 102, 17051–17067, 1997.
Kaufman, Y. J., Gobron, N., Pinty, B., Widlowski, J., and Verstraete, M. M.: Relationship between surface reflectance in the visible and mid-IR used in MODIS aerosol algorithm – theory, J. Geophys. Res., 29, 2116, https://doi.org/10.1029/2001GL014492, 2002.
Kaufman, Y. J., Koren, I., Remer, L. A., Tanre, D., Ginoux, P., and Fan, S.: Dust transport and deposition observed from the Terra- Moderate Resolution Imaging Spectroradiometer (MODIS) spacecraft over the Atlantic Ocean, J. Geophys. Res., 110, D10S12, https://doi.org/10.1029/2003JD004436, 2005.
King, M. D., Platnick, S. E., Menzel, W. P., Ackerman, S. A., and Hubanks, P. A.: Spatial and temporal distribution of clouds observed by MODIS onboard the Terra and Aqua satellites, IEEE T. Geosci. Remote, 51, 3826–3852, 2013.
Koren, I., Feingold, G., Jiang, H., and Altaratz, O.: Aerosol effects on the inter-cloud region of a small cumulus cloud field, Geophys. Res. Lett., 36, L14805, https://doi.org/10.1029/2009GL037424, 2009.
Koren, I., Altaratz, O., Remer, L. A., Feingold, G., Martins, J. V., and Heiblum, R. H.: Aerosol-induced intensification of rain from the tropics to the mid-latitudes, Nat. Geosci., 5, 118–122, https://doi.org/10.1038/ngeo1364, 2012.
Levy, R. C., Remer, L. A., Martins, J. V., Kaufman, Y. J., Plana-Fattori, A., Redemann, J., Russell, P. B., and Wenny, B.: Evaluation of the MODIS aerosol retrievals over ocean and land during CLAMS, J. Atmos. Sci., 62, 974–992, 2005.
Levy, R. C., Remer, L. A., and Dubovik, O.: Global aerosol optical properties and application to Moderate Resolution Imaging Spectroradiometer aerosol retrieval over land, J. Geophys. Res.-Atmos., 112, D13210, https://doi.org/10.1029/2006JD007815, 2007a.
Levy, R. C., Remer, L. A., Mattoo, S., Vermote, E. F., and Kaufman, Y. J.: Second-generation operational algorithm: retrieval of aerosol properties over land from inversion of Moderate Resolution Imaging Spectroradiometer spectral reflectance, J. Geophys. Res.-Atmos., 112, D13211, https://doi.org/10.1029/2006JD007811, 2007b.
Levy, R. C., Remer, L. A., Kleidman, R. G., Mattoo, S., Ichoku, C., Kahn, R., and Eck, T. F.: Global evaluation of the Collection 5 MODIS dark-target aerosol products over land, Atmos. Chem. Phys., 10, 10399–10420, https://doi.org/10.5194/acp-10-10399-2010, 2010.
Levy, R. C., Mattoo, S., Munchak, L. A., Remer, L. A., Sayer, A. M., Patadia, F., and Hsu, N. C.: The Collection 6 MODIS aerosol products over land and ocean, Atmos. Meas. Tech., 6, 2989–3034, https://doi.org/10.5194/amt-6-2989-2013, 2013.
Levy, R. C., Munchak, L. A., Mattoo, S., Patadia, F., Remer, L. A., and Holz, R. E.: Towards a long-term global aerosol optical depth record: applying a consistent aerosol retrieval algorithm to MODIS and VIIRS-observed reflectance, Atmos. Meas. Tech., 8, 4083–4110, https://doi.org/10.5194/amt-8-4083-2015, 2015.
Lyapustin, A., Wang, Y., Xiong, X., Meister, G., Platnick, S., Levy, R., Franz, B., Korkin, S., Hilker, T., Tucker, J., Hall, F., Sellers, P., Wu, A., and Angal, A.: Scientific impact of MODIS C5 calibration degradation and C6+ improvements, Atmos. Meas. Tech., 7, 4353–4365, https://doi.org/10.5194/amt-7-4353-2014, 2014.
Munchak, L. A., Levy, R. C., Mattoo, S., Remer, L. A., Holben, B. N., Schafer, J. S., Hostetler, C. A., and Ferrare, R. A.: MODIS 3 km aerosol product: applications over land in an urban/suburban region, Atmos. Meas. Tech., 6, 1747–1759, https://doi.org/10.5194/amt-6-1747-2013, 2013.
Nichol, J. E. and Bilal, M.: Validation of MODIS 3 km Resolution Aerosol Optical Depth Retrievals Over Asia, Remote Sens., 8, 328, https://doi.org/10.3390/rs8040328, 2016.
Oreopoulos, L., Cho, N., Lee, D., and Kato, S.: Radiative effects of global MODIS cloud regimes, J. Geophys. Res.-Atmos., 121, 2299–2317, https://doi.org/10.1002/2015jd024502, 2016.
Petrenko, M., Ichoku, C., and Leptoukh, G.: Multi-sensor Aerosol Products Sampling System (MAPSS), Atmos. Meas. Tech., 5, 913–926, https://doi.org/10.5194/amt-5-913-2012, 2012.
Remer, L. A., Tanre, D., Kaufman, Y. J., Ichoku, C., Mattoo, S., Levy, R., Chu, D. A., Holben, B., Dubovik, O., Smirnov, A., Martins, J. V., Li, R. R., and Ahmad, Z.: Validation of MODIS aerosol retrieval over ocean, Geophys. Res. Lett., 29, 1618, https://doi.org/10.1029/2001GL013204, 2002.
Remer, L. A., Kaufman, Y. J., Tanré, D., Mattoo, S., Chu, D. A., Martins, J. V., Li, R. R., Ichoku, C., Levy, R. C., Kleidman, R. G., Eck, T. F., Vermote, E., and Holben, B. N.: The MODIS aerosol algorithm, products, and validation, J. Atmos. Sci., 62, 947–973, https://doi.org/10.1175/JAS3385.1, 2005.
Remer, L. A., Mattoo, S., Levy, R. C., Heidinger, A., Pierce, R. B., and Chin, M.: Retrieving aerosol in a cloudy environment: aerosol product availability as a function of spatial resolution, Atmos. Meas. Tech., 5, 1823–1840, https://doi.org/10.5194/amt-5-1823-2012, 2012.
Remer, L. A., Mattoo, S., Levy, R. C., and Munchak, L. A.: MODIS 3 km aerosol product: algorithm and global perspective, Atmos. Meas. Tech., 6, 1829–1844, https://doi.org/10.5194/amt-6-1829-2013, 2013.
Russell, P. B., Livingston, J. M., Redemann, J., Schmid, B., Ramirez, S. A., Eilers, J., Kahn, R., Chu, A., Remer, L., Quinn, P. K., Rood, M. J., and Wang, W.: Multi-gridcell validation of satellite aerosol property retrievals in INTEX/ITCT/ICARTT 2004, J. Geophys. Res., 112, D12S09, https://doi.org/10.1029/2006JD007606, 2007.
Salomonson, V. V., Barnes, W. L., Maymon, P. W., Montgomery, H. E., and Ostrow, H.: MODIS: advanced facility instrument for studies of the Earth as a system, IEEE T. Geosci. Remote, 27, 145–153, 1989.
Sayer, A.M., Hsu, N.C., Bettenhausen, C., Jeong, M., and Meister, G.: Effect of MODIS Terra radiometric calibration improvements on Collection 6 Deep Blue aerosol products: validation and Terra/Aqua consistency, J. Geophys. Res, 120, 12157–12174, https://doi.org/10.1002/2015JD023878, 2015.
Toller, G., Xiong, X., Sun, J., Wenny, B. N., Geng, X., Kuyper, J., Angal, A., Chen, H., Madhavan, S., and Wu, A.: Terra and Aqua moderate-resolution imaging spectroradiometer collection 6 level 1B algorithm, J. Appl. Remote Sens., 7, 3557, https://doi.org/10.1117/1.JRS.7.073557, 2013.
Toon, O. B., Maring, H., Dibb, J., Ferrare, R., Jacob, D. J., Jensen, E. J., Luo, Z. J., Mace, G. G., Pan, L. L., Pfister, L., Rosenlof,K. H., Redemann, J., Reid, J. S., Singh, H. B., Thompson, A. M., Yokelson, R., Minnis, P., Chen, G., Jucks, K. W., and Pszenny, A.: Planning, implementation and scientific goals of the Studies of Emissions and Atmospheric Composition, Clouds and Climate Coupling by Regional Surveys (SEAC4RS) field mission, J. Geophys. Res., 121, 4967–5009, https://doi.org/10.1002/2015JD024297, 2016.
van Donkelaar, A., Martin, R. V., Brauer, M., and Boys, B. L.: Use of Satellite Observations for Long-Term Exposure Assessment of Global Concentrations of Fine Particulate Matter, Environ. Health Persp., 123, 135–143, https://doi.org/10.1289/ehp.1408646, 2015.
Yu, H., Kaufman, Y. J., Chin, M., Feingold, G., Remer, L. A., Anderson, T. L., Balkanski, Y., Bellouin, N., Boucher, O., Christopher, S., DeCola, P., Kahn, R., Koch, D., Loeb, N., Reddy, M. S., Schulz, M., Takemura, T., and Zhou, M.: A review of measurement-based assessments of the aerosol direct radiative effect and forcing, Atmos. Chem. Phys., 6, 613–666, https://doi.org/10.5194/acp-6-613-2006, 2006.
Yu, H., Remer, L., Chin, M., Bian, H., Tan, Q., Yuan, T., and Zhang, Y.: Aerosols from Overseas Rival Domestic Emissions over North America, Science, 337, 566–569, 2012.
Yuan, T., Remer, L. A., Pickering, K. E., and Yu, H.: Observational evidence of aerosol enhancement of lightning activity and convective invigoration, Geophys. Res. Lett., 38, L04701, https://doi.org/10.1029/2010GL046052, 2011.