the Creative Commons Attribution 4.0 License.
the Creative Commons Attribution 4.0 License.
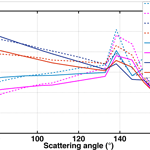
Cloud heterogeneity on cloud and aerosol above cloud properties retrieved from simulated total and polarized reflectances
Céline Cornet
Laurent C.-Labonnote
Fabien Waquet
Frédéric Szczap
Lucia Deaconu
Frédéric Parol
Claudine Vanbauce
François Thieuleux
Jérôme Riédi
Simulations of total and polarized cloud reflectance angular signatures such as the ones measured by the multi-angular and polarized radiometer POLDER3/PARASOL are used to evaluate cloud heterogeneity effects on cloud parameter retrievals. Effects on optical thickness, albedo, effective radius and variance of the cloud droplet size distribution and aerosol parameters above cloud are analyzed. Three different clouds that have the same mean optical thicknesses were generated: the first with a flat top, the second with a bumpy top and the last with a fractional cloud cover. At small scale (50 m), for oblique solar incidence, the illumination effects lead to higher total but also polarized reflectances. The polarized reflectances even reach values that cannot be predicted by the 1-D homogeneous cloud assumption. At the POLDER scale (7 km × 7 km), the angular signature is modified by a combination of the plane–parallel bias and the shadowing and illumination effects. In order to quantify effects of cloud heterogeneity on operational products, we ran the POLDER operational algorithms on the simulated reflectances to retrieve the cloud optical thickness and albedo. Results show that the cloud optical thickness is greatly affected: biases can reach up to −70, −50 or +40 % for backward, nadir and forward viewing directions, respectively. Concerning the albedo of the cloudy scenes, the errors are smaller, between −4.7 % for solar incidence angle of 20∘ and up to about +8 % for solar incidence angle of 60∘. We also tested the heterogeneity effects on new algorithms that allow retrieving cloud droplet size distribution and cloud top pressures and also aerosol above clouds. Contrary to the bi-spectral method, the retrieved cloud droplet size parameters are not significantly affected by the cloud heterogeneity, which proves to be a great advantage of using polarized measurements. However, the cloud top pressure obtained from molecular scattering in the forward direction can be biased up to about 60 hPa (around 550 m). Concerning the aerosol optical thickness (AOT) above cloud, the results are different depending on the available angular information. Above the fractional cloud, when only side scattering angles between 100 and 130∘ are available, the AOT is underestimated because of the plane–parallel bias. However, for solar zenith angle of 60∘ it is overestimated because the polarized reflectances are increased in forward directions.
- Article
(1501 KB) - Full-text XML
- BibTeX
- EndNote
Cloud properties such as effective radius, optical thickness and albedo are key parameters for studies concerning cloud radiative effects and hydrological cycle of Earth climatic system. In the context of climate change, these properties may be modified and result in a feedback, the sign of which remains largely uncertain. In parallel, anthropogenic activities modify the aerosol loading in the atmosphere and consequently play an important role on cloud through the indirect radiative effects of aerosols (Twomey, 1977). In addition, absorbing aerosol above clouds can generate a positive direct radiative forcing (i.e., warming), that is currently not well quantified, and modify the properties of the underlying cloud layer (Chand et al., 2008; Costantino and Bréon, 2013; Wilcox, 2010).
Currently, several satellite radiometers use solar and infrared reflectances to infer cloud and aerosols above cloud parameters. Generally, cloud optical thickness (COT) and albedo are obtained from visible channels. Depending on instrument capabilities, the effective radius can be retrieved jointly with the optical thickness from a combination of visible and near-infrared measurements (Nakajima and King, 1990) as it is done in the operational algorithm of the Moderate Resolution Imaging Radiometer (MODIS Platnick et al., 2003). These parameters can also be retrieved separately from multi-viewing total and polarized measurements (Buriez et al., 1997; Bréon and Goloub, 1998) as implemented for the optical thickness or under implementation for the effective radius with the POLarization and Directionality of the Earth's Reflectances radiometer (POLDER, Deschamps et al., 1994).
Concerning aerosols, spaceborne active instruments, such as the lidar CALIOP are dedicated tools to detect multi-layer situations and to retrieve Aerosol Above Cloud (AAC) properties (Hu et al., 2007; Chand et al., 2008; Young and Vaughan, 2009) and were used for climate studies (Zhang et al., 2016a). Passive measurements, that provide larger global coverage, can also be used and an operational algorithm was developed to retrieve AAC scenes from the polarization measurements provided by the POLDER instrument onboard PARASOL (Waquet et al., 2009, 2013a). It was used to provide global analysis of the aerosol above clouds properties (Waquet et al., 2013b). Further, (Peers et al., 2015) combined total and polarized radiance measurements to retrieve the aerosol absorption above clouds. A color ratio technique was also developed to retrieve the AAC optical thickness and the corrected cloud optical thickness from total radiance measurements. This method was adapted for the Ozone Monitoring Instrument (OMI) ultraviolet measurements and MODIS multi-spectral measurements (Torres et al., 2011; Meyer et al., 2015)
For computation time and simplicity reasons, all of these operational algorithms assume that clouds are flat, homogeneous and horizontally infinite, which is quite far from the reality. Numerous studies presented in the review of Davis and Marshak (2005) and Davis and Marshak (2010) showed that this assumption can lead to large errors on the retrieved cloud parameters. For example, the cloud optical thickness can be affected by the so-called plane–parallel bias induced by the sub-pixel heterogeneity and the non-linear relationship between reflectances and optical thickness. This bias usually leads to an effective optical thickness lower than the mean optical thickness (Cahalan, 1994; Szczap et al., 2000a). The sub-pixel optical thickness heterogeneity can also cause a positive bias on the mean effective radius retrieved following the bi-spectral technique (Szczap et al., 2000b; Zhang et al., 2012), whereas the sub-pixel microphysical heterogeneity, not studied in this paper, leads, on the contrary, to an underestimation of the effective radius (Marshak et al., 2006). The bias on effective radius can thus be positive or negative depending on sub-pixel heterogeneity of the cloud optical thickness and effective radius (Zhang et al., 2016b).
In addition to the sub-pixel heterogeneity, Loeb and Davies (1996) detected an increase of the retrieved optical thickness from AVHRR (Advanced Very High Resolution Radiometer) correlated with the solar zenith angle. Indeed, for oblique solar illumination, more energy is transmitted through the clouds along the cloud side (or bump). It leads to an increase in upward reflectances. Consequently, the cloud optical thickness retrieved under the homogeneous cloud assumption appears higher for tilted Sun than for overhead Sun. This effect is combined with angular effects, known as 3-D effects, which depend on the sensor viewing direction. Again, in the backward scattering direction, parts of the cloud sides illuminated by the Sun lead to a larger retrieved optical thickness value. Inversely, in viewing directions close to the forward scattering directions, some parts of the cloud are in the shadow resulting in smaller optical thickness or larger effective radius. This angular signature was observed on the retrieved cloud optical thickness by several radiometers such as AVHRR (Loeb and Coakley, 1998), MODIS (Varnai and Marshak, 2002) and POLDER (Buriez et al., 2001; Zeng et al., 2012).
Concerning aerosol above cloud (AAC), intercomparisons of passive and active retrievals were performed for case studies (Jethva et al., 2013) and for global and multi-year data (Deaconu et al., 2017). All the methods developed for passive instruments are based on 1-D calculations and, so, generally restricted to homogeneous cloudy pixels for which the 3-D effects are minimized. In case of aerosol retrieval in partial cloudy scenes, shadowing or enhancement of the clear areas by neighboring clouds can modify the retrieved aerosol properties. Errors on the retrieved aerosol properties are in general dependent of the cloud distribution, optical thickness and spatial resolution (Stap et al., 2016a, b).
Therefore, depending on the cloud heterogeneity, solar zenith angle and viewing geometry, cloud parameters (i.e., optical thickness and effective radius) and AAC parameters can be either under or overestimated. Several studies based on simulations of total reflectances were made at the scale of 1 km corresponding to a moderate resolution radiometer such as MODIS or the GLobal Imager (GLI/ADEOS2) to assess errors for liquid water clouds on optical thickness (Iwabuchi and Hayasaka, 2002; Zinner and Mayer, 2006) or on effective radius (Zhang et al., 2012). Kato et al. (2006) analyzed in addition the error on the albedo of the cloudy scenes, which is an important parameter for cloud radiative budget studies. At 1 km pixel size, they found significant errors ranging between −0.3 and 14 % (−5 and 30 %) from nadir (oblique) viewing depending on the cloud heterogeneity. Some recent studies were also made for ice clouds and found non negligible errors on retrieved COT from infrared (IR) measurements (Fauchez et al., 2015) or from visible and near-infrared measurements (Zhou et al., 2017). Concerning aerosol above cloud retrieval, to our knowledge, no study has been conducted to assess errors due to cloud heterogeneity.
In this paper, we investigate the impact of cloud heterogeneities of retrieved parameters on observations from the POLarization and Directionality of the Earth's Reflectances radiometer, POLDER, which was on board the platforms ADEOS1 in 1999, ADEOS2 in 2002 and PARASOL between 2005 and 2013. POLDER/PARASOL allows to measure multi-angular total reflectances from 443 to 1020 nm and multi-angular polarized reflectances for three channels (490, 670 and 865 nm). A review of the POLDER capabilities for cloud measurements and retrieval are presented in Parol et al. (2004). Comparisons with MODIS cloud products were analyzed for cloud fraction in Zeng et al. (2011), for cloud phase in Zeng et al. (2013) and cloud optical thickness in Zeng et al. (2012). In the latter, the plane-parallel bias and 3-D cloud effects were observed in the COT values retrieved from multi-angle measurements under oblique solar illumination: lower COT were retrieved in the forward viewing direction and larger COT in the backward viewing direction (Figs. 8 and 9 in Zeng et al., 2012). Reflectance simulations from known cloud properties help in quantitatively understanding the errors or biases on the retrieved cloud properties. In addition, assessment of POLDER algorithms will be helpful in a near future as the Multi-viewing, Multi-Channel, Multi-Polarization Imaging mission (3MI), a POLDER type follow-on instrument is planned to be part of the future generation of EUMETSAT polar satellites, EPS-SG (Marbach et al., 2015).
Total and polarized reflectances were simulated at a small scale (50 m) from synthetic 3-D cloud fields and averaged at the POLDER pixel size (7 km × 7 km) to simulate POLDER measurements. The different clouds used in our study and presented in Sect. 2 are generated using an enhanced version of the 3DCLOUD model (Szczap et al., 2014; Alkasem et al., 2017) and the reflectances are computed using the 3DMCPOL model (Cornet et al., 2010). The POLDER cloud operational algorithm described in (Buriez et al., 1997) is then used to retrieve the COT and the albedo of the cloudy scene. Results are presented in Sect. 3.
Contrary to MODIS, POLDER does not make measurements in the near infrared to get information on cloud particle size. The first two moments of the cloud droplet distribution are obtained from polarized angular measurements (Bréon and Goloub, 1998; Breon and Doutriaux-Boucher, 2005) as well as the cloud top pressure (Goloub et al., 1994). Polarized reflectance measurements are also used for cloud droplet retrievals by the Research Scanning Polarimeter (Alexandrov et al., 2012). Cloud heterogeneity effects on polarized measurements of liquid clouds have been studied for a single flat cloud in (Cornet et al., 2013) and almost no effects were found. Here, we go further and present in Sect. 4.1 the differences between 3-D and 1-D polarized angular reflectances for different clouds and geometries. Consequences for 3-D cloud radiative effects on the effective radius, effective variance and cloud top pressure retrieval are presented in Sect. 4.2. The impacts of the 3-D effects on the POLDER above cloud AOT operational retrievals in case of fractional cloud were evaluated and presented in Sect. 4.3. Conclusions are summarized in Sect. 5.
The clouds used in this study have been generated with the 3DCLOUD model (Szczap et al., 2014; Alkasem et al., 2017). 3DCLOUD is a fast and flexible algorithm designed for generating realistic 3-D extinction or 3-D optical thickness for stratocumulus, cumulus and cirrus cloud fields. 3DCLOUD cloud fields share some pertinent statistical properties observed in real clouds such as a gamma distributed optical thickness and the Fourier spectral slope β close to between the smallest scale of the simulation to the outer scale Lout where the spectrum becomes flat. In addition, the user can specify the mean optical thickness COT, the heterogeneity parameter ρ (standard deviation of COT normalized by the mean of COT) and the cloud coverage C. In the first step, 3DCLOUD solves drastically simplified basic atmospheric equations and integrates user's prescribed large-scale meteorological profiles (humidity, pressure, temperature and wind speed), in order to simulate 3-D cloud structures of liquid water content (LWC). In the second step, the amplitude of the wavelet coefficient of the extinctions are manipulated with a 3-D wavelet transform of the whole 3-D cloudy volume to constrain the mean COT, ρ, β and Lout (Alkassem et al., 2017).
Here, we generated three cloud fields composed of 140 × 140 pixels with an initial horizontal resolution of 50 m resulting to a 7 km × 7 km field, which corresponds to a POLDER pixel size. The choice of 50 m for the pixel scale was made considering the mean free path of the photon, (corresponding to the inverse of the extinction coefficient so to about 70 m) but also considering computation time and virtual memory availability.
The three generated clouds have the same mean optical thickness, close to 10, at 865 nm. We created two stratocumulus clouds and one cumulus cloud. The latter is the result of instabilities of the boundary layer and led to fractional cloud cover and a larger heterogeneity parameter (Kawai and Teixeira, 2011). The flat and bumpy clouds, representative of overcast stratocumulus clouds, have the same heterogeneity parameter across the 140 × 140 pixels, ρ= 0.6, which is a typical value for stratocumulus cloud. The cumulus cloud has a fractional cloud cover equal to 0.76 and a heterogeneity parameter equal to 1.12 setting clear sky pixels to null values (0.95 if computed only with the cloudy pixels). These values are typical values obtained from Landsat data (Barker et al., 1996) for stratocumulus and cumulus clouds.
Figure 1 shows the vertical profiles of potential temperature and of vapor mixing ratio prescribed in this study to generate the three cloud fields. Globally, the vertical profiles of potential temperature and vapor mixing ratio give the cloud position. The mean cloud top height is mainly determined by the height where the potential temperature increases and the vapor mixing ratio decreases. Cloud top height fluctuations (shapes of top bumps) are mainly the result of the intensity of the vertical gradient of the potential temperature and vapor mixing ratio.
Figure 2 shows the horizontal cloud optical thickness fields and a vertical profile through each cloud. In this study, we focus on the effects of the optical thickness heterogeneity, which is supposed in real clouds to be more important than the microphysical heterogeneity (Magaritz-Ronen et al., 2016). Consequently, the cloud droplet size distribution is assumed to be uniform everywhere in the cloud and follows a log-normal distribution with an effective radius of 11 µm and an effective variance of 0.02.
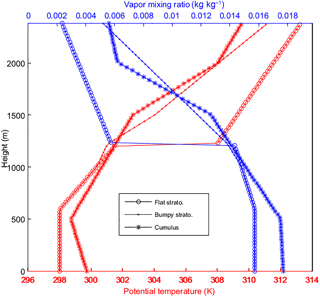
Figure 1Vertical profiles of potential temperature and vapor mixing ratio prescribed in this study to generate the flat stratocumulus (circle), the bumpy stratocumulus (point) and the cumulus (star) cloud fields.
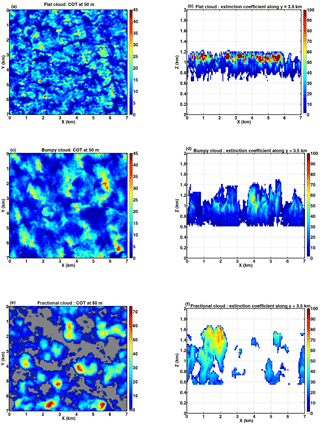
Figure 2Cloud optical thickness (COT) of the three clouds used for the study (a) the flat cloud, (c) the bumpy cloud and (e) the fractional cloud. Extinction coefficient (km−1) along the x−z axis for y= 3.5 km for (b) the flat cloud, (d) the bumpy cloud (f) and the fractional cloud.
From these 3-D cloud fields, we simulated the total and polarized bidirectional reflectances function for the viewing zenith angle θ and the viewing azimuthal angle ϕ. For convenience, we call them total reflectance R and polarized reflectance Rp in the following equation:
where and V(θ,φ) are the four Stokes parameters in W m−2 sr−1, F0 the solar flux in W m−2 and θ0 the solar zenith angle.
Reflectances for three solar incidence angles 20, 40 and 60∘ are computed with the 3-D radiative transfer model, 3DMCPOL. It is a forward Monte-Carlo model able to compute radiative reflected or transmitted Stokes vector as well as upwelling and downwelling fluxes in three-dimensional atmospheres. Initially developed for solar radiation (Cornet et al., 2010), it was next extended to thermal radiation (Fauchez et al., 2014). To save time and for an accurate computation of reflectances, the local estimate method (Marshak and Davis, 2005) is used. Periodic boundary conditions at the horizontal domain limits are used. For highly peaked phase function, the Potter truncation is implemented. Molecular scattering is computed according to the pressure profile. A heterogeneous surface can also be specified with Lambertian reflection, ocean or snow bidirectional function. The model participated and was improved during the International Polarized Radiative Transfer (IPRT) model intercomparisons on homogeneous cloud cases (Emde et al., 2015) and on 3-D cloud cases (Emde et al., 2018).
Simulations are run with a total of 107 and 109 photons for the homogeneous and heterogeneous clouds, respectively. The Monte-Carlo uncertainties are estimated with the computation of the standard deviation of 10 and 50 independent realizations of 106 and 20.106 photons for the homogeneous and heterogeneous cloud, respectively. For the homogeneous case, the relative standard deviation is below 0.12 % for the total reflectances and below 1.2 % for the polarized reflectances. For the heterogeneous clouds, at 50 m resolution, the mean relative standard deviation is below 1.3 % for the total reflectances. For polarized reflectances at 50 m, the mean relative standard deviation varies according to the angular geometry and is between 2 and 107 % for very small reflectance values with a mean value of 23 %. At 7 km resolution, as the reflectances are averaged, relative standard deviation values are much lower below 0.01 and 0.8 % for total and polarized reflectances, respectively.
At this stage, molecular scattering is integrated but no aerosols. To remain consistent with assumptions made within the POLDER operational algorithm, an oceanic surface with a wind speed of 7 m s−1 is included for total reflectances while a black surface is included for polarized reflectances. Indeed, for retrieval using polarized reflectances, the multi-angular ability of POLDER provides the advantage of not using the directions close to the sun-glint, where the polarized reflectances can be high. As POLDER measures up to 16 directions, we simulate reflectances for 16 POLDER typical zenith observation angles in the solar plane. Total reflectances of the three clouds are presented in Fig. 3 (first column) with a 50 m spatial resolution for a solar incidence angle of 60∘ in the cloudbow direction (42∘ from the backward direction). Polarized reflectance fields are discussed in Sect. 4.1.
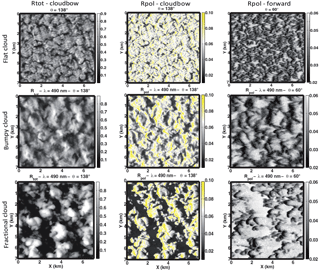
Figure 3Total and polarized reflectances for the flat cloud (first line), the bumpy cloud (second line) and the fractional cloud (third line). Total reflectances at 490 nm in the cloudbow scattering direction (first column), polarized reflectances at 490 nm in the cloudbow direction (second column) and polarized reflectances at 490 nm in the forward direction (third column). The Sun illuminates the scene from the left of the figures (SZA = 60∘). For polarized reflectances in the second column, yellow color corresponds to polarized reflectance values higher than the maximum value predicted with the homogeneous cloud assumption.
We averaged spatially the 50 m resolution reflectances fields at 7 km × 7 km to mimic the radiometer measurements and applied the POLDER operational algorithm on these synthetic measurements to obtain cloud optical thickness and albedo. In order to assess the retrieval errors due to the cloud homogeneous assumption without biases due to differences in reflectance computations, we also computed the 1-D reflectances of the three equivalent homogenous clouds, which are subsequently used for retrieval to act as references for the inhomogeneous cloud retrievals. The COT of the equivalent homogeneous clouds is the mean COT of the heterogeneous clouds, and their cloud top and base altitudes correspond to the maximum and minimum altitudes of the respective homogenous clouds.
Figure 4 summarizes the results obtained for the retrieved cloud optical thickness for the three solar zenith angles and the four cases, namely the homogeneous (1-D), the flat, the bumpy and the fractional cloud. The optical thicknesses are plotted as a function of sensor zenith angles with negative values corresponding to backward scattering directions and positive values to forward scattering directions. The homogeneous cloud values (1-D) are only plotted for control and we observe logically that the retrieved value is almost constant and close to 10, independently of the solar incidence angle, since the same assumption (1-D homogeneous cloud) is used in both the forward simulation and retrieval algorithm. Slight differences appear because of inclusion of aerosol optical thickness in the forward model used to build the look-up table (Buriez et al., 1997) but not in our simulations. The small angular difference in the backward direction at 20∘ can be attributed to interpolation in the LUT.
Looking at results concerning the heterogeneous clouds (3-D), we clearly note, in the angular range between about −30 and +30∘, the plane-parallel bias, which leads to retrieving optical thicknesses lower than the mean optical thickness. At nadir view, the relative error is between −10 and −20 % both for the flat cloud and bumpy cloud and is much larger for the fractional cloud, between −35 and −50 %. The flat and bumpy clouds were built with the same heterogeneity parameter (ρ= 0.6), whereas the fractional cloud has a larger heterogeneity parameter including the zeros (ρ= 1.12) due to its fractional nature. That confirms that heterogeneity parameters can be at first order used to characterize plane-parallel bias (Cahalan et al., 1994; Szczap et al., 2000a).
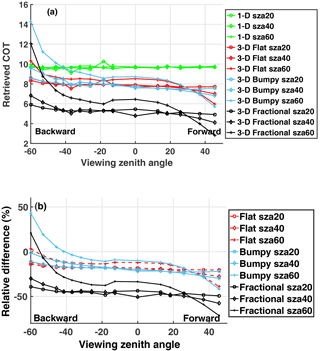
Figure 4(a) Cloud optical thickness (COT) retrieved with the POLDER operational algorithm as function of the viewing zenith angle for the four different simulated cloud cases (1-D, flat, bumpy and fractional clouds) and for different solar zenith angles (20, 40 and 60∘). (b) Relative differences [(COT3-D − COT1-D) ∕ COT1-D × 100] between the heterogeneous cloud (3-D) and the homogenous cloud (1-D) COT.
For solar zenith angle (SZA) equal to 20∘, the retrieved optical thickness is almost independent of the observation geometry whatever the cloud type, while for SZA = 60∘, significant differences between viewing angles are observed. We note indeed a strong decrease of the retrieved optical thickness value in the forward scattering direction leading to a relative bias on the retrieved optical thickness between −40 % for the flat and bumpy cloud and −70 % for the fractional cloud. On the contrary, we can notice an increase of the retrieved optical thickness value in the backscatter direction (relative bias ranging from +3 % for the flat cloud, +43 % for the bumpy cloud and +21 % for the fractional cloud). This angular behavior was already simulated by several authors at the resolution of 1 km (Loeb et al., 1998; Varnai, 2000; Iwabuchi and Hayasaka, 2002; Zinner and Mayer, 2006) and agrees with POLDER observations (Buriez et al., 2001; Zeng et al., 2012). In the backscatter directions, the cloud sides illuminated by the Sun make the cloud brighter, in contrast to the forward direction where cloud sides are in the shadow (Varnai and Davies, 1999). These effects are visible for the bumpy cloud but are much less pronounced for the flat cloud. The heterogeneity parameter thus seems well adapted to characterize quantitatively the plane-parallel bias (Szczap et al., 2000a) but not sufficient to characterize the amplitude of the 3-D effects. Indeed, the flat and bumpy clouds, which are characterized by the same heterogeneity parameter value show close plane-parallel bias (between −10 and 20 % for nadir view) but quite different amplitudes of the 3-D effects, especially in the backward direction for SZA = 60∘. We note also that this error in the backward direction is larger for the bumpy cloud (about +40 %) compared to the fractional cloud (about +20 %) because for the latter the plane-parallel bias is stronger (about −40 % at nadir view).
The following step in the POLDER operational algorithm consists in computing the albedo of the cloudy scene, corresponding to the upward flux normalized by the solar incident flux, from the retrieved cloud optical thickness using look-up tables (Buriez et al., 1997). The albedo is not derived from a single view, as computed in Kato et al. (2006), at 1 km × 1 km but from all view angles. The multi-angular capabilities of POLDER allow averaging over the different values using a directional weighting function. The aim of this weighting function is to limit the influence of directions for which the microphysical or 3-D effects can be important as for example in the cloudbow, glory and forward directions (Buriez et al., 2005).
The assessment of cloud heterogeneity effects on cloud albedo is realized by comparing the retrieved POLDER algorithm albedos with the ones directly computed with the 3DMCPOL radiative transfer model identified as the true one. Direct comparisons of retrieved albedo values from homogeneous or from heterogeneous clouds, as done for other parameters, are not suitable for cloud albedo. Indeed, the plane-parallel bias leads to reflectances off of a heterogeneous cloud lower than the reflectances off of an equivalent homogenous cloud with the same (mean) COT. The retrieved optical thickness is thus lower than the mean optical thickness of 10 (Fig. 4). Using it to recompute the albedo in the POLDER algorithm leads to a too low value comparing to the albedo of the equivalent homogeneous cloud. In contrast, using 1-D cloud radiative model in the inversion and in the forward computation as it is done in the operational algorithm is consistent and leads to a sound cloud albedo. The plane-parallel bias is indeed almost canceled.
Table 1For each cloud case, albedo of the cloudy scene obtained from simulation with 3DMCPOL (first line), retrieved with the POLDER operational algorithm (second line) and relative differences [(Retrieval − simulation) ∕ Simulation × 100] between the two values (third line, in bold) for the homogeneous cloud (for control) and for the flat, bumpy and fractional clouds for three solar zenith angles (20, 40 and 60∘). The mean optical thickness of each cloud is 10 and the effective radius is fixed to 11 µm.
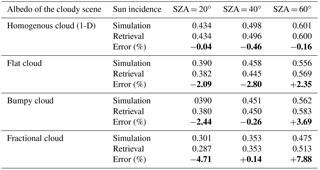
Values of the computed and retrieved albedos and their relative differences are indicated in Table 1. The first line (homogeneous cloud) shows very good consistency between the 3DMCPOL radiative transfer code and the retrieved values using the POLDER operational algorithm. Relative differences between computed and retrieved albedos remain smaller than 0.5 %.
For SZA = 20∘, the POLDER operational algorithm slightly underestimates the albedo for the flat and bumpy cloud with relative differences under −2.5 %. The relative error is slightly larger for the fractional cloud (−4.7 %). The relative differences are low compared to optical thickness errors because, as explained above, the same cloud model (i.e., the homogeneous cloud) is used to retrieve and to compute the albedo. The slight underestimation of the retrieved albedo comes from differences in the non-linear relationship between reflectances and albedo as a function of the optical thickness. It implies that effects of the plane-parallel bias are not the same for reflectances and albedos. Inversely, for SZA = 60∘, the albedo is overestimated by 2.35 % for the flat cloud case and 7.88 % for the fractional cloud case because illumination effects in the backscattering direction are not completely canceled by the weighting function.
At SZA = 40∘, negative differences due to the plane parallel biases are on contrary almost canceled by illumination effects for bumpy and fractional cloud leading to very small errors of −0.26 and +0.14 %, respectively.
4.1 Cloud heterogeneity effects on polarized reflectances
As explained before, we simulated using 3DMCPOL, the polarized reflectances for the three wavelengths used in the POLDER retrieval algorithms (e.g., 490, 670 and 865 nm). Total and polarized reflectances at 490 nm for 50 m resolution are presented in Fig. 3 (second and third columns) for SZA = 60∘. First of all, we can see that for flat cloud, the polarized reflectance field appears smoother than the total reflectance field. As polarized reflectances level off for optical thickness greater than about three, all cloudy pixels with higher optical thickness provide almost the same polarized reflectance. Therefore, cloud heterogeneity effects are visually less discernible on polarized reflectance fields compared to the total reflectance fields.
For the bumpy or fractional clouds, the polarized reflectance field appears much rougher. In the cloudbow viewing directions (second column), some parts of the cloud facing to Sun appear brighter and other parts in the shadow darker. At this small spatial scale (50 m), a large part of the total amount of pixels exhibits polarized reflectance higher than the maximum value predicted by the 1-D homogeneous cloud model (yellow pixels) and thus cannot be obtained with 1-D radiative transfer simulation: at 490 nm, their ratio reaches 41 % of the total number of pixels for the flat cloud, 52 % for the bumpy cloud and 38 % for the fractional cloud. This phenomenon of illumination and shadowing was already highlighted simply with a step cloud in Cornet et al. (2010).
In the forward direction (Θ= 60∘) at 490 nm (third column in Fig. 3), the “shadow areas” are not dark anymore contrary to the total reflectance images (first column in Fig. 3) and appear even brighter than cloudy part. For short wavelength and forward scattering angles, molecular signal is stronger than the cloud signal and thus enhances the polarized signal in the shadow parts.
In Fig. 5, we plot the average polarized reflectances as would be measured by POLDER at 7 km × 7 km resolution as a function of the scattering angle Θ for a solar zenith angle SZA = 60∘, and for the three wavelengths. As we can see in Fig. 5a, the main differences between homogeneous and heterogeneous clouds appear in the cloudbow direction (Θ= 140∘) and in the forward direction (Θ < 80∘). In the cloudbow direction, the 3-D polarized reflectances are lower than the 1-D ones for the three clouds. Similar to the total reflectances, this is mainly due to the plane-parallel bias. In these directions, the relative differences (Fig. 5b) are about −9, −12 and −35 % for the flat, bumpy and fractional cloud, respectively. We note that the relative difference is slightly lower for 490 nm because of the smoothing effects by molecular scattering above the cloud.
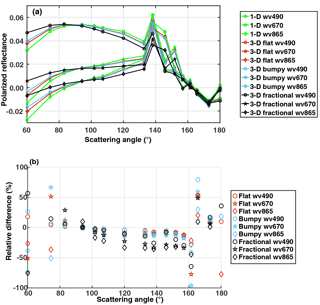
Figure 5(a) Polarized reflectance as a function of the scattering angle for three wavelengths (490, 670 and 865 nm) for the homogeneous cloud (1-D), the flat cloud, the bumpy cloud and the fractional cloud. (b) Relative difference between 3-D and 1-D polarized reflectances, [(Rp3-D − Rp1-D) ∕ Rp1-D × 100]. The solar zenith angle is 60∘.
In the forward scattering directions, the consequences of the 3-D effects in terms of absolute polarized reflectances appear differently depending on the wavelength. At 490 nm, the 3-D effects enhance the absolute polarization, while at 865 nm they reduce it. At 490 nm, atmospheric molecular scattering is very strong. The 3-D polarized reflectances appear greater than the 1-D ones because, as seen in Fig. 3, the polarization in the shadow parts of the cloud is enhanced by this molecular scattering. At 865 nm, the shadow parts appear dark with small positive values that reduce the negative polarization of the cloud and consequently the absolute polarization. The relative difference (Fig. 5b) is consequently positive for 490 nm (about +55 % for the fractional cloud) and negative for 865 nm (about −75 % for the fractional cloud). At 670 nm, the polarized reflectance in the shadow part is only slightly enhanced by the molecular scattering but more compared to 865 nm. Polarized reflectances thus become positive for the fractional cloud but not for the flat and bumpy clouds. Note that in the backward direction, the polarized reflectances are very weak thus no heterogeneity or 3-D effects can be detected.
Figure 5 illustrates results obtained for simulations for SZA = 60∘ with a scattering angular range between 60 and 180∘. For SZA = 20 and SZA = 40∘, the plots are similar with a reduced scattering angular range comprised between 100 and 180∘ for SZA = 20∘, and between 80 and 180∘ for SZA = 40∘. Consequently, for SZA = 20 and SZA = 40∘ the attenuation due to the plane-parallel bias is the main effect that impacts the polarized reflectances.
4.2 Consequences for droplet size distribution and cloud top pressure retrievals
The polarized signal is used as input of a POLDER retrieval algorithm developed to retrieve effective radius, effective variance and cloud top pressure. It uses the polarized information as presented in Bréon and Goloub (1998). The position of the cloudbow as well as the position of the supernumerary bows gives information on the effective radius. The amplitude of the supernumerary bows gives information on the effective variance of the cloud droplet size distribution. For cloud top pressure, the algorithm uses the information given by the molecular scattering that depends, in the forward scattering directions, on the atmospheric air mass factor (Goloub et al., 1994). The algorithm, under implementation in the POLDER operational algorithm, is based on an optimal estimation method (Rodgers, 2000) and provides errors associated to each of the retrieved parameters. It is also possible to add in the forward model variance-covariance matrix an error due to the non-retrieved parameter. Following previous computations made in Waquet et al. (2013a) for the misrepresentation of the cloud heterogeneity effects, the error added in the variance-covariance matrix on the reflectances is 7.5 % in the directions close to the cloudbow and 5 % elsewhere.
Table 2Retrieved cloud droplet effective radius (Reff), effective variance (Veff) and cloud top altitude (CTOP) from polarized reflectances with an optimal estimation algorithm. First column is the input, second column the retrieval for the homogeneous cloud (1-D), third column for the flat cloud, fourth column for the bumpy cloud and fifth column for the fractional cloud. The last line is the final cost function with NC meaning no convergence. The solar zenith angle is 60∘. Note that the cloud top altitude is different according to the heterogeneous cloud leading to three different lines.
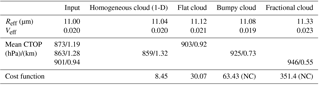
The retrieved values obtained with this algorithm based on the homogeneous cloud assumption, are presented in Table 2. We again use the homogeneous cloud (1-D) to check the consistency of our simulations. For all clouds, even if differences in polarized reflectances are large in amplitude, the retrieval algorithm captures the general angular features the three wavelengths, which results of small errors on the retrieved effective radius and effective variance. The algorithm is able to retrieve an effective radius of 11 µm and an effective variance of 0.02 with relative error compared to the input under 2.6 and 2.1 %, respectively (see Table 2). Indeed, as the cloud heterogeneity effects do not modify the cloudbow position and the number of supernumerary bows, the retrieval of the droplet size distribution parameters is not really affected by 3-D effects. This is a fundamental advantage of the polarized measurements compared to the bi-spectral method (Zhang et al., 2012), usually used when visible and shortwave infrared wavelengths are available. However, we note that the cost function, which is the root-mean-square-difference between the model and measurements weighted by the respective variance–covariance matrix is larger for 3-D clouds than for the homogeneous cloud. It means that the forward model (homogeneous model) used for the retrieval does not allow perfectly matching the heterogeneous cloud reflectances used as input. For the bumpy and fractional cloud, the algorithm does not even converge meaning that the forward model is not able to represent the signal within the allocated uncertainties. The main impact of cloud heterogeneities appears for cloud top pressure retrieval. In Table 2, we report the mean cloud top height for each heterogeneous cloud and the retrieved value. The 1-D homogeneous values used for control were set to the intermediate mean cloud top altitude. We note slight differences about −4 hPa (+37 m) between input and 1-D retrieval, which reveals slight differences between the radiative transfer codes used for the simulation and for the retrieval. However, differences between 3-D and 1-D are much larger, especially for the bumpy and fractional cloud with values of +62 hPa (−550 m) and +45 hPa (−390 m). As already explained, the polarized reflectance in the shortwave wavelengths (490 nm) is very high because of molecular scattering. The retrieval of the cloud top pressure is based on the amount of molecular scattering occurring above the cloud when looking in forward scattering (for scattering angle ranging between 60 and 120∘). Consequently, as shadowing effects modify the polarized reflectances in the forward scattering directions, the cloud top pressure retrieval is impacted, especially for the fractional and bumpy cloud.
4.3 Impacts for aerosol above cloud retrieval
Polarized reflectances of POLDER are also used to retrieve aerosol optical thickness (AOT) of an aerosol layer above cloud (Waquet et al., 2009, 2013a). Waquet et al. (2013a) describes two algorithms for aerosol above clouds (AAC) retrieval using POLDER polarization measurements: (i) the research algorithm is an optimal estimation method that retrieves a large number of aerosol and cloud parameters and (ii) the operational algorithm is based on LUTs calculations and allows to retrieve the AOT at 865 nm and the Ångström exponent of aerosol above clouds. The “operational algorithm” is the one considered in the present study. The LUT calculations are performed with the successive order of scattering code that assumes a plane-parallel atmosphere (Lenoble et al., 2007). It uses assumptions of particle microphysics: six fine mode spherical aerosol models (effective radius varying between 0.09 and 0.24 µm) are considered and a constant complex refractive index of 1.47+0.01i is assumed. The errors due to the assumption made for the complex refractive index were estimated at around 20 % on average for the AOT (Peers et al., 2015) and maximal relative error may reach 25 % in case of extreme aerosol events (AOT > 0.6 at 550 nm). One additional non-spherical mineral dust model is also considered in the LUTs.
The operational algorithm uses a specific strategy to retrieve aerosol properties above clouds that depends on the aerosol type and also on the available viewing geometries (see Fig. 4 in Waquet et al., 2013a). In case of fine mode particles, the retrieval is restricted to the use of observations acquired for scattering angles smaller than 130∘, where polarization measurements are highly sensitive to scattering by fine mode particles (such as biomass burning aerosol) and only weakly sensitive to cloud microphysics. This is illustrated in Fig. 6 with the dashed lines representing polarized reflectances for a homogeneous cloud with an aerosol layer above (dark blue and red curves) and without aerosol above (clear blue and pink curves). The increase of the polarized reflectances for scattering angles less than 130∘ is clearly visible when an aerosol layer is present above a cloud. Non-spherical particles in the coarse mode, such as mineral dust particles, cannot be handled with this method as they do not polarize light much. When dust particles are transported above clouds, they reduce the magnitude of the primary cloud bow. The operational algorithm then includes the primary bow in order to retrieve the above cloud dust AOT. In this case, as the magnitude of the primary cloud bow primarily depends on the cloud droplet effective radius, it must be estimated or included in the retrieval process. Collocated cloud properties from MODIS at high resolution (1 km × 1 km) are used to characterize and to select the cloudy scenes within a POLDER pixel (6 km × 7 km at nadir) and the MODIS cloud products can then be used in the operational algorithm to estimate the droplets effective radius. As the magnitude of the primary cloud bow is only weakly impacted by the choice of the droplet effective variance, this parameter is assumed to be constant and equal to 0.06. Several filters are eventually applied to obtain a quality-assessed product. For instance, the retrievals are restricted to cloudy pixels associated with cloud optical thicknesses larger than 3.0, since the polarized radiation reflected by the cloud layer is then saturated and does not depend anymore on the cloud optical thickness. Criteria are also used to reject inhomogeneous and fractional cloudy pixels and to avoid cirrus cloud contamination. We refer to Sect. 3.4 in (Waquet et al., 2013a) for a detailed description of the operational algorithm.
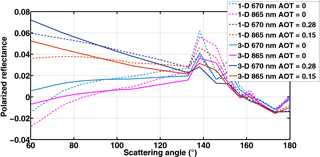
Figure 6Polarized reflectances as a function of the scattering angle. Dashed lines are for homogeneous cloud with and without a biomass burning aerosol layer above; solid lines are for the fractional cloud with and without a biomass burning aerosol layer above. The solar zenith angle is 60∘.
In the POLDER operational algorithm, the underneath cloud is assumed to be homogeneous. Empirical criterions are used to reject heterogeneous and fractional cloudy pixels but a misclassification of the cloudy scenes is still possible. Moreover, it is also important to evaluate the AOT retrieval errors due to 3-D effects in case of fractional cloud covers. These scenes, for which aerosols and clouds are potentially mixed, remain untreated and are of primarily importance for climate studies. In the following, we investigate the possibility of using the operational algorithm to handle these scenes and we evaluate the biases observed in the polarized reflectances and in the AOT retrieval errors due to 3-D effects. In order to check the AOT value retrieved for such cases, we use the 3-D polarized reflectances generated for the fractional cloud case, with and without aerosol, and we used these 3-D simulations as inputs for the operational algorithm. Note that for the synthetic retrievals discussed here, we assumed that the operational algorithm knows the effective radius and effective variance of the cloud droplets.
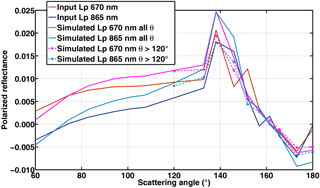
Figure 73-D Polarized reflectances used as input for the aerosol above cloud algorithm (Waquet et al., 2013a) and polarized reflectances simulated with the algorithm after the convergence of the retrieval. Reflectances at all angles were used (solid line) and reflectances with only scattering angles above 120∘ (dashed line).
The 3-D polarized reflectances used as input of the algorithm and the ones simulated after the adjustment of the aerosol model and optical thickness are plotted in Fig. 7 (solid lines). When a large scattering angular range is available (between 60 and 180∘), the algorithm works in an efficient way. The lateral polarized reflectances in scattering angular range between 80 and 120∘ exhibit low or negative values. Consequently, no aerosol (AOT = 0) were retrieved. However, we note that the primary cloudbow is not well reproduced by the 1-D simulation provided by the operational algorithm. In the POLDER measurements, the range of sampled scattering angles varies with the geographical position. In some cases, the scattering angle range sampled by the instrument can be quite narrow. We tested the algorithm without observations acquired for scattering angles smaller than 120∘ (dashed lines in Fig. 7). The cloudbow signal is then better matched but the inversion method retrieves erroneous AOT values of 0.31 at 670 nm and 0.28 at 865 nm instead of zero for both.
Table 3Retrieved aerosol properties for a biomass aerosol layer above the fractional cloud with the operational algorithm described in Waquet et al. (2013a). Aerosol optical thickness at 670 nm (AOT670), at 865 nm (AOT865) and Ångström coefficient for three solar zenith angles (SZA). Relative differences [(Fractional − Homogeneous) ∕ Homogeneous × 100] are indicated in bold. Last two lines, RMSE computed between the input and the recalculated polarized reflectances for the homogenous and fractional cloud.
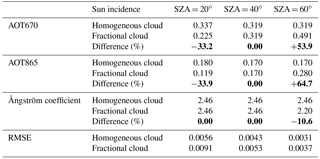
A second test is made with simulated reflectances including a biomass-burning aerosol layer lofted above the fractional cloud. For the simulation, the AOT of the aerosol layer is fixed to 0.28 and 0.15, the single scattering albedo to 0.93 and 0.91 at 670 and 865 nm, respectively. In order to avoid retrieval errors related to the choice of aerosol model, we used one of the biomass burning aerosol model included in the operational algorithm. The particles effective radius is 0.15 µm and the single scattering albedo is equal to 0.91 at 865 nm. The simulated 3-D angular polarized reflectances as a function of the scattering angles are presented in Fig. 6 (solid blue and red lines). Compared to the 1-D reflectances with aerosols above cloud (dashed blue and red lines), the cloud heterogeneity effects amplify the increase of the forward signal and the decrease of the cloudbow signal. As with molecular scattering (Sect. 4.1), aerosol scattering contributes to enhance the polarized reflectances in the shadow and cloud-free parts leading to higher averaged polarized reflectances in the forward direction. In the cloudbow direction (near 140∘), and to a lesser extent, in the side scattering (between 100 and 130∘ in scattering angle), the polarized reflectances are additionally attenuated because of the plane-parallel biases. Note that for other solar zenith angles (not shown here), the plots are similar with a more restricted scattering angular range (between 100 and 180∘ for SZA = 20∘ and between 80 and 180∘ for SZA = 40∘). Consequently, only the attenuation due to the plane-parallel bias impacts the polarized reflectances.
The results obtained with the operational algorithm are presented in Table 3. We repeat that the same input AOT is used in the 1-D and 3-D simulations (AOT of 0.15 at 865 nm). The Ångström exponent is related to the ratio of two optical thicknesses at two wavelengths and corresponds in the retrieval to the best-selected model. As expected, the AOTs retrieved by the algorithm for homogenous clouds are close to the input one, whatever the SZA value. The retrieved AOTs only slightly overestimate the input (0.15) and are respectively equal to 0.180, 0.170 and 0.170 for SZA of 20, 40 and 60∘. This overestimation is likely due to the approximations used in the retrieval algorithm (e.g. interpolation in the LUTs). Comparing with the retrieved values from homogeneous cloud, significant departures are observed for fractional clouds especially for SZA = 60∘. The AOTs retrieved at 865 nm are then equal to 0.119, 0.170 and 0.280 for SZA of 20, 40 and 60∘, respectively. For a given solar zenith angle, the viewing geometries and the angular resolution are identical for the homogeneous and fractional clouds. The differences observed in AOT between the 1-D and 3-D calculations are then necessarily due to cloud heterogeneity effects. For SZA = 40∘, the best model that minimized the cost function is the same for the homogeneous and fractional clouds. Differences for the retrieved AOT are negligible, but we note that the RMSE between the input and recalculated reflectances is slightly larger for the fractional cloud than for the homogeneous one. For SZA = 20∘, the operational algorithm also successfully retrieves the input aerosol model for the homogeneous and fractional cloud. However, the AOT retrieved by the operational algorithm, under the homogenous assumption, is underestimated with an error of about −33 %. For SZA = 20∘, the range of scattering angles effectively used for the retrieval is between 100 and 130∘. Polarized reflectances for SZA = 20∘ are not shown but they are similar to the ones shown in Fig. 7 between 100 and 180∘. Between 100 and 130∘, as shown in Fig. 7, 3-D polarized reflectances are lower than the 1-D ones because of the plane-parallel biases, which explains why the AOT retrieved by the algorithm is underestimated. However, as the differences are mainly due the plane-parallel bias, which is similar for the two wavelengths, the cloud heterogeneity effects do not affect the selection of the best aerosol model. For SZA = 60∘, the range of scattering angles used is between 60 and 130∘. Between 60 and 90∘, there is an increase of the forward scattering signal due to 3-D effects, which is interpreted by the operational algorithm as an increase in the AOT. We note also that 3-D effects bias the aerosol model for this case as a smaller value of Ångström exponent (corresponding to a larger effective radius) is retrieved for the fractional cloud. The retrieved AOT is thus higher (AOT of 0.28 comparing to 0.17) with a relative error up to 65 %.
Note that the operational algorithm is not applied for pixels too heterogeneous. Those are filtered using the standard deviation of the COT retrieved at 1 km by MODIS that should not exceed five. For the fractional cloud of this study, we checked the standard deviation value computed from the input cloud optical thickness (different from the retrieved one) and found seven. It is slightly above the homogeneity limit fixed in the aerosol above cloud algorithm developed for POLDER (Waquet et al., 2013a). The results presented here for aerosol above cloud retrieval can thus be seen as an upper limit for the operational algorithm.
This study used simulations to understand and quantify the effects of cloud heterogeneities on POLDER total and polarized reflectances. We investigated the consequences of heterogeneous cloud radiative effects on the retrieved values of cloud optical thickness, droplet effective radius, effective variance, cloud pressure and optical properties (optical thickness and Ångström exponent) of above cloud aerosol, provided by operational and research algorithms of the POLarization and Directionality of Earth's Reflectances (POLDER) instrument. 3-D cloud fields were generated with the 3DCLOUD model (Szczap et al., 2014) and the 1-D and 3-D radiative transfer simulations were done with the Monte Carlo 3DMCPOL model (Cornet et al., 2010). Three types of heterogeneous water cloud were studied: a flat, a bumpy and a fractional cloud.
The reflectances simulated at small spatial scale (50 m) and averaged at the POLDER spatial scale (7 km × 7 km) are used as realistic input of the different cloud operational and research algorithms. For high solar illumination (SZA = 20∘), the optical thickness retrieval yields, as it was already shown in numerous studies, to lower optical thickness than the averaged ones because of the plane-parallel bias. For POLDER, the retrieved optical thicknesses are underestimated by 10 or 35 % depending on the cloud type. For oblique solar incidence, the POLDER algorithm yields to higher optical thickness in the backscattering directions due to solar illumination effects and much lower optical thickness (up to −70 % for the fractional cloud) in the forward scattering directions due to shadowing effects. The errors on albedo are weaker with largest bias for albedo between −5 % for high solar illumination and +8 % for solar zenith angle of 60∘.
We next analyzed the cloud heterogeneity effects on polarized reflectances. We showed a reduction of the cloudbow and side reflectances due to the plane-parallel bias and the shadowing effects. In the forward scattering direction, the effects are spectrally dependent. For the shortest wavelength (490 nm), the molecular scattering in the shadow areas increases the averaged polarized signal and leads to an increase of the polarized reflectances. At 865 nm, the weak positive polarized reflectances of the shadow areas reduce the polarization of the clouds, which is negative for these scattering angles. However, even if the polarized angular signature is modified, the retrieved effective radius and effective variance are hardly affected because cloud heterogeneities do not modify the positions of the cloudbow and supernumerary bows. The Rayleigh cloud top pressure is, in contrast, biased for a solar zenith angle of 60∘ by about 60 hPa corresponding to a cloud 550 m lower in the atmosphere.
We also tested the aerosol above cloud algorithm (Waquet et al., 2013a). Even in the absence of aerosol, the algorithm retrieves non-negligible AOT values when only larger scattering angles (between 120 and 180∘) are available. With aerosols above a fractional cloud, the AOT can be underestimated for a high solar elevation (SZA = 20∘) because of the plane-parallel bias and on contrary overestimated for low solar elevation (SZA = 60∘) because of the shadowed effects that increase polarized reflectances. The Ångström exponent is affected by these shadowing effects for SZA = 60∘ but not by the plane-parallel bias since the plane-parallel biases for 490 and 865 nm is almost spectrally neutral and since the information used to select the aerosol model is related to the ratio of those two wavelengths.
These results mainly show that 3-D effects for fractional clouds are primarily significant at forward scattering geometries in case of low solar elevation (scattering angle < 80∘ and SZA = 60∘) and in the rainbow region (scattering angle of about 140∘ ± 5∘). The range of scattering angles sampled between 60 and 80∘ is not necessarily useful for an accurate retrieval of the above cloud AOT. So, reducing the range of scattering angles to scattering angle values larger than 80∘ will help to reduce the errors associated with the AOT retrievals. The algorithm largely overestimates the AOT when the primary bow is included in the retrieval process and when forward and side scattering viewing geometries are not available. This result suggests that polarized measurements acquired for this configuration should not be used for AAC properties retrievals, at least with a retrieval algorithm based on 1-D calculations.
Assessment of retrieval errors due to cloud heterogeneity is challenging for the next generation of retrieval algorithms. Indeed, in the future, it appears crucial to have not only values of retrieved parameters but also estimations of their uncertainties. Realistic simulations with known input parameters are very useful tools to assess accurately theses errors including their dependence on the available angular sampling. Such simulations can also be used to test the next generation of operational algorithms.
Further to those assessments of cloud heterogeneity uncertainties, more complex methods should also be developed to retrieve aerosol and cloud properties accounting for the cloud heterogeneities. Several theoretical or case studies have already been conducted. Some tends to mitigate cloud contamination for aerosol property retrieval (Davis et al., 2013; Stap et al., 2016b). Others aim to use 3-D radiative transfer model to retrieve 3-D cloud properties and hence account for some cloud heterogeneity effects. It then requires more complex inversion methods. Feasibility studies has been conducted using a neural network method (Cornet et al., 2004, 2005), 3-D tomography with a surrogate function (Levis et al., 2015, 2017) or adjoint method (Martin et al., 2014; Martin and Hasekamp, 2018). The latter two methods are very promising but have been developed in the framework of high resolution measurements (ten to hundred meters) involving no or small plane-parallel bias. They are consequently not directly applicable to POLDER/PARASOL measurements.
The Multi-viewing, Multi-Channel, Multi-Polarization Imaging mission (3MI) that will fly on METOP-A SG as part of EUMETSAT Polar System after 2021, will have a spatial resolution of 4 × 4 km. The plane-parallel bias is thus expected to be slightly lower than for the POLDER instrument. In addition, as 3MI will be on the same platform as the Visible Infrared Imager, a multispectral radiometer with a resolution of 500 m, the correction of the plane parallel biases may be possible while the multi-angular capability of 3MI would help to detect the illumination and shadowing effects.
The source code of the 3DCLOUD algorithm is available online at http://wwwobs.univ-bpclermont.fr/atmos/fr/restricted (last access: 21 June 2018) under restricted access. Please contact the authors for the password. The 3DMCPOL model and the input data used as input for the retrieval algorithms can be made available upon request to the corresponding author.
The authors declare that they have no conflict of interest.
This work has been supported by the French Programme National de
Télédétection Spatiale (PNTS, http://www.insu.cnrs.fr/pnts, last access: 21 June 2018),
grant no. PNTS-2014-02 and by the Centre National d'Etudes
Spatiales (CNES). The authors are very grateful for the interesting and pertinent comments made by the reviewers. They contributed to greatly improve the manuscript.
Edited by: Piet Stammes
Reviewed by: Zhibo Zhang, Feng Xu, Gerard van Harten,
and one anonymous referee
Alexandrov, M. D., Cairns, B., Emde, C., Ackerman, A. S., and van Diedenhoven, B.: Accuracy assessments of cloud droplet size retrievals from polarized reflectance measurements by the research scanning polarimeter, Remote Sens. Environ., 125, 92–111, https://doi.org/10.1016/j.rse.2012.07.012, 2012.
Alkasem, A., Szczap, F., Cornet, C., Shcherbakov, V., Gour, Y., Jourdan, O., Labonnote, L. C., and Mioche, G.: Effects of cirrus heterogeneity on lidar CALIOP/CALIPSO data, J. Quant. Spectrosc. Ra., 202, 38–49, https://doi.org/10.1016/j.jqsrt.2017.07.005, 2017.
Barker, H. W., Wiellicki, B. A., and Parker, L.: A Parameterization for Computing Grid-Averaged Solar Fluxes for Inhomogeneous Marine Boundary Layer Clouds. Part II: Validation Using Satellite Data, J. Atmos. Sci., 53, 2304–2316, https://doi.org/10.1175/1520-0469(1996)053<2304:APFCGA>2.0.CO;2, 1996.
Breon, F. M. and Doutriaux-Boucher, M.: A comparison of cloud droplet radii measured from space, IEEE T. Geosci. Remote, 43, 1796–1805, https://doi.org/10.1109/TGRS.2005.852838, 2005.
Bréon, F.-M. and Goloub, P.: Cloud droplet effective radius from spaceborne polarization measurements, Geophys. Res. Lett., 25, 1879–1882, https://doi.org/10.1029/98GL01221, 1998.
Buriez, J. C., Vanbauce, C., Parol, F., Goloub, P., Herman, M., Bonnel, B., Fouquart, Y., Couvert, P., and Seze, G.: Cloud detection and derivation of cloud properties from POLDER, Int. J. Remote Sens., 18, 2785–2813, https://doi.org/10.1080/014311697217332, 1997.
Buriez, J.-C., Doutriaux-Boucher, M., Parol, F., and Loeb, N. G.: Angular Variability of the Liquid Water Cloud Optical Thickness Retrieved from ADEOS–POLDER, J. Atmos. Sci., 58, 3007–3018, https://doi.org/10.1175/1520-0469(2001)058<3007:AVOTLW>2.0.CO;2, 2001.
Buriez, J.-C., Parol, F., Cornet, C., and Doutriaux-Boucher, M.: An improved derivation of the top-of-atmosphere albedo from POLDER/ADEOS-2: Narrowband albedos, J. Geophys. Res.-Atmos., 110, D05202, https://doi.org/10.1029/2004JD005243, 2005.
Cahalan, R. F.: Bounded cascade clouds: albedo and effective thickness, Nonlin. Processes Geophys., 1, 156–167, https://doi.org/10.5194/npg-1-156-1994, 1994.
Chand, D., Anderson, T. L., Wood, R., Charlson, R. J., Hu, Y., Liu, Z., and Vaughan, M.: Quantifying above-cloud aerosol using spaceborne lidar for improved understanding of cloudy-sky direct climate forcing, J. Geophys. Res.-Atmos., 113, D13206, https://doi.org/10.1029/2007JD009433, 2008.
Cornet, C., Isaka, H., Guillemet, B., and Szczap, F.: Neural network retrieval of cloud parameters of inhomogeneous clouds from multispectral and multiscale radiance data: Feasibility study, J. Geophys. Res.-Atmos., 109, D12203, https://doi.org/10.1029/2003JD004186, 2004.
Cornet, C., Buriez, J.-C., Riédi, J., Isaka, H., and Guillemet, B.: Case study of inhomogeneous cloud parameter retrieval from MODIS data, Geophys. Res. Lett., 32, L13807, https://doi.org/10.1029/2005GL022791, 2005.
Cornet, C., C.-Labonnote, L., and Szczap, F.: Three-dimensional polarized Monte Carlo atmospheric radiative transfer model (3DMCPOL): 3-D effects on polarized visible reflectances of a cirrus cloud, J. Quant. Spectrosc. Ra., 111, 174–186, https://doi.org/10.1016/j.jqsrt.2009.06.013, 2010.
Cornet, C., Szczap, F., C.-Labonnote, L., Fauchez, T., Parol, F., Thieuleux, F., Riedi, J., Dubuisson, P., and Ferlay, N.: Evaluation of cloud heterogeneity effects on total and polarized visible radiances as measured by POLDER/PARASOL and consequences for retrieved cloud properties, Proceedings of the International Radiation Symposium (IRC/IAMAS), AIP Publishing, 99–102, https://doi.org/10.1063/1.4804717, 2013.
Costantino, L. and Bréon, F.-M.: Aerosol indirect effect on warm clouds over South-East Atlantic, from co-located MODIS and CALIPSO observations, Atmos. Chem. Phys., 13, 69–88, https://doi.org/10.5194/acp-13-69-2013, 2013.
Davis, A. B. and Marshak, A.: Solar radiation transport in the cloudy atmosphere: a 3-D perspective on observations and climate impacts, Rep. Prog. Phys., 73, 026801, https://doi.org/10.1088/0034-4885/73/2/026801, 2010.
Davis, A. B., Garay, M. J., Xu, F., Qu, Z., and Emde, C.: 3-D radiative transfer effects in multi-angle/multispectral radio-polarimetric signals from a mixture of clouds and aerosols viewed by a non-imaging sensor, Presented at the Polarization Science and Remote Sensing VI, International Society for Optics and Photonics, p. 887309, https://doi.org/10.1117/12.2023733, 2013.
Deaconu, L. T., Waquet, F., Josset, D., Ferlay, N., Peers, F., Thieuleux, F., Ducos, F., Pascal, N., Tanré, D., Pelon, J., and Goloub, P.: Consistency of aerosols above clouds characterization from A-Train active and passive measurements, Atmos. Meas. Tech., 10, 3499–3523, https://doi.org/10.5194/amt-10-3499-2017, 2017.
Deschamps, P.-Y., Breon, F.-M., Leroy, M., Podaire, A., Bricaud, A., Buriez, J.-C., and Seze, G.: The POLDER mission: instrument characteristics and scientific objectives, IEEE T. Geosci. Remote, 32, 598–615, https://doi.org/10.1109/36.297978, 1994.
Emde, C., Barlakas, V., Cornet, C., Evans, F., Korkin, S., Ota, Y., Labonnote, L. C., Lyapustin, A., Macke, A., Mayer, B., and Wendisch, M.: IPRT polarized radiative transfer model intercomparison project – Phase A, J. Quant. Spectrosc. Ra., 164, 8–36, https://doi.org/10.1016/j.jqsrt.2015.05.007, 2015.
Emde, C., Barkalas, V., Cornet, C., Evans, F., Wang, Z., Labonnote, L.C., Macke, A., Mayer, B., and Wendisch, M.: IPRT polarized radiative transfer model intercomparison project – Three-dimensional test cases (phase B), J. Quant. Spectrosc. Ra., 209, 19–44, https://doi.org/10.1016/j.jqsrt.2018.01.024, 2018.
Fauchez, T., Cornet, C., Szczap, F., Dubuisson, P., and Rosambert, T.: Impact of cirrus clouds heterogeneities on top-of-atmosphere thermal infrared radiation, Atmos. Chem. Phys., 14, 5599–5615, https://doi.org/10.5194/acp-14-5599-2014, 2014.
Fauchez, T., Dubuisson, P., Cornet, C., Szczap, F., Garnier, A., Pelon, J., and Meyer, K.: Impacts of cloud heterogeneities on cirrus optical properties retrieved from space-based thermal infrared radiometry, Atmos. Meas. Tech., 8, 633–647, https://doi.org/10.5194/amt-8-633-2015, 2015.
Goloub, P., Deuze, J. L., Herman, M., and Fouquart, Y.: Analysis of the POLDER polarization measurements performed over cloud covers, IEEE T. Geosci. Remote, 32, 78–88, https://doi.org/10.1109/36.285191, 1994.
Hu, Y., Vaughan, M., Liu, Z., Powell, K., and Rodier, S.: Retrieving Optical Depths and Lidar Ratios for Transparent Layers Above Opaque Water Clouds From CALIPSO Lidar Measurements, IEEE Geosci. Remote S., 4, 523–526, https://doi.org/10.1109/LGRS.2007.901085, 2007.
Iwabuchi, H. and Hayasaka, T.: Effects of Cloud Horizontal Inhomogeneity on the Optical Thickness Retrieved from Moderate-Resolution Satellite Data, J. Atmos. Sci., 59, 2227–2242, https://doi.org/10.1175/1520-0469(2002)059<2227:EOCHIO>2.0.CO;2, 2002.
Jethva, H., Torres, O., Waquet, F., Chand, D., and Hu, Y.: How do A-train sensors intercompare in the retrieval of above-cloud aerosol optical depth? A case study-based assessment, Geophys. Res. Lett., 41, 186–192, https://doi.org/10.1002/2013GL058405, 2013.
Kato, S., Hinkelman, L. M., and Cheng, A.: Estimate of satellite-derived cloud optical thickness and effective radius errors and their effect on computed domain-averaged irradiances, J. Geophys. Res.-Atmos., 111, D17201, https://doi.org/10.1029/2005JD006668, 2006.
Kawai, H. and Teixeira, J.: Probability Density Functions of Liquid Water Path and Total Water Content of Marine Boundary Layer Clouds: Implications for Cloud Parameterization., J. Climate, 25, 2162–2177, https://doi.org/10.1175/JCLI-D-11-00117.1, 2011.
Lenoble, J., Herman, M., Deuzé, J.L., Lafrance, B., Santer, R., and Tanré, D.: A successive order of scattering code for solving the vector equation of transfer in the earth's atmosphere with aerosols, J. Quant. Spectrosc. Ra., 107, 479–507, https://doi.org/10.1016/j.jqsrt.2007.03.010, 2007.
Levis, A., Schechner, Y. Y., Aides, A., and Davis, A. B.: Airborne Three-Dimensional Cloud Tomography, in: 2015 IEEE International Conference on Computer Vision (ICCV), Presented at the 2015 IEEE International Conference on Computer Vision (ICCV), 3379–3387, https://doi.org/10.1109/ICCV.2015.386, 2015.
Levis, A., Schechner, Y. Y., and Davis, A. B.: Multiple-Scattering Microphysics Tomography, IEEE, 5797–5806, https://doi.org/10.1109/CVPR.2017.614, 2017.
Loeb, N. G. and Coakley, J. A.: Inference of Marine Stratus Cloud Optical Depths from Satellite Measurements: Does 1-D Theory Apply?, J. Climate, 11, 215–233, https://doi.org/10.1175/1520-0442(1998)011<0215:IOMSCO>2.0.CO;2, 1998.
Loeb, N. G. and Davies, R.: Observational evidence of plane parallel model biases: Apparent dependence of cloud optical depth on solar zenith angle, J. Geophys. Res.-Atmos., 101, 1621–1634, https://doi.org/10.1029/95JD03298, 1996.
Magaritz-Ronen L., Khain A., and Pinsky M.: About the horizontal variability of effective radius in stratocumulus clouds, J. Geophys. Res.-Atmos., 121, 9640–9660, https://doi.org/10.1002/2016JD024977, 2016.
Marbach, T., Riedi, J., Lacan, A., and Schlüssel, P.: The 3MI mission: multi-viewing-channel-polarisation imager of the EUMETSAT polar system: second generation (EPS-SG) dedicated to aerosol and cloud monitoring, Proc. SPIE 9613, Polarization Science and Remote Sensing VII, 961310, https://doi.org/10.1117/12.2186978, 2015.
Marshak, A. and Davis, A. (Eds.): 3-D Radiative Transfer in Cloudy Atmospheres, Physics of Earth and Space Environments, Springer-Verlag, Berlin/Heidelberg, 2005.
Marshak, A., Platnick, S., Várnai, T., Wen, G., and Cahalan, R. F.: Impact of three-dimensional radiative effects on satellite retrievals of cloud droplet sizes, J. Geophys. Res.-Atmos., 111, D09207, https://doi.org/10.1029/2005JD006686, 2006.
Martin, W. and Hasekamp, O. P.: A demonstration of adjoint methods for multi-dimensional remote sensing of the atmosphere and surface, J. Quant. Spectrosc. Ra., 204, 215–231, https://doi.org/10.1016/j.jqsrt.2017.09.031, 2018.
Martin, W., Cairns, B., and Bal, G.: Adjoint methods for adjusting three-dimensional atmosphere and surface properties to fit multi-angle/multi-pixel polarimetric measurements, J. Quant. Spectrosc. Ra., 144, 68–85, https://doi.org/10.1016/j.jqsrt.2014.03.030, 2014.
Meyer, K., Platnick, S., and Zhang, Z.: Simultaneously inferring above-cloud absorbing aerosol optical thickness and underlying liquid phase cloud optical and microphysical properties using MODIS, J. Geophys. Res.-Atmos., 2015, JD023128, https://doi.org/10.1002/2015JD023128, 2015.
Nakajima, T. and King, M. D.: Determination of the Optical Thickness and Effective Particle Radius of Clouds from Reflected Solar Radiation Measurements. Part I: Theory, J. Atmos. Sci., 47, 1878–1893, https://doi.org/10.1175/1520-0469(1990)047<1878:DOTOTA>2.0.CO;2, 1990.
Parol, F., Buriez, J. C., Vanbauce, C., Riedi, J., C.-Labonnote, L., Doutriaux-Boucher, M., Vesperini, M., Sèze, G., Couvert, P., Viollier, M., and Bréon, F. M.: Review of capabilities of multi-angle and polarization cloud measurements from POLDER. Adv. Space Res., Climate Change Processes in the Stratosphere, Earth-Atmosphere-Ocean Systems, and Oceanographic Processes from Satellite Data 33, 1080–1088. https://doi.org/10.1016/S0273-1177(03)00734-8, 2004.
Peers, F., Waquet, F., Cornet, C., Dubuisson, P., Ducos, F., Goloub, P., Szczap, F., Tanré, D., and Thieuleux, F.: Absorption of aerosols above clouds from POLDER/PARASOL measurements and estimation of their direct radiative effect, Atmos. Chem. Phys., 15, 4179–4196, https://doi.org/10.5194/acp-15-4179-2015, 2015.
Platnick, S., King, M. D., Ackerman, S. A., Menzel, W. P., Baum, B. A., Riedi, J. C., and Frey, R. A.: The MODIS cloud products: algorithms and examples from Terra, IEEE T. Geosci. Remote, 41, 459–473, https://doi.org/10.1109/TGRS.2002.808301, 2003.
Rodgers, C. D.: Inverse methods for atmospheric sounding: theory and practice, World Scientific Publishing Co. Ltd, London, UK, p. 238, 2000.
Stap, F. A., Hasekamp, O. P., Emde, C., and Röckmann, T.: Influence of 3-D effects on 1-D aerosol retrievals in synthetic, partially clouded scenes, J. Quant. Spectrosc. Ra., 170, 54–68, https://doi.org/10.1016/j.jqsrt.2015.10.008, 2016a.
Stap, F. A., Hasekamp, O. P., Emde, C., and Röckmann, T.: Multiangle photopolarimetric aerosol retrievals in the vicinity of clouds: Synthetic study based on a large eddy simulation, J. Geophys. Res.-Atmos., 121, 12914–12935, https://doi.org/10.1002/2016JD024787, 2016b.
Szczap, F., Isaka, H., Saute, M., Guillemet, B., and Ioltukhovski, A.: Effective radiative properties of bounded cascade nonabsorbing clouds: Definition of the equivalent homogeneous cloud approximation, J. Geophys. Res.-Atmos., 105, 20617–20633, https://doi.org/10.1029/2000JD900146, 2000a.
Szczap, F., Isaka, H., Saute, M., Guillemet, B., and Ioltukhovski, A.: Effective radiative properties of bounded cascade absorbing clouds: Definition of an effective single-scattering albedo, J. Geophys. Res.-Atmos., 105, 20635–20648, https://doi.org/10.1029/2000JD900145, 2000b.
Szczap, F., Gour, Y., Fauchez, T., Cornet, C., Faure, T., Jourdan, O., Penide, G., and Dubuisson, P.: A flexible three-dimensional stratocumulus, cumulus and cirrus cloud generator (3DCLOUD) based on drastically simplified atmospheric equations and the Fourier transform framework, Geosci. Model Dev., 7, 1779–1801, https://doi.org/10.5194/gmd-7-1779-2014, 2014.
Torres, O., Jethva, H., and Bhartia, P. K.: Retrieval of Aerosol Optical Depth above Clouds from OMI Observations: Sensitivity Analysis and Case Studies, J. Atmos. Sci., 69, 1037–1053, https://doi.org/10.1175/JAS-D-11-0130.1, 2011.
Twomey, S.: The Influence of Pollution on the Shortwave Albedo of Clouds, J. Atmos. Sci., 34, 1149–1152, https://doi.org/10.1175/1520-0469(1977)034<1149:TIOPOT>2.0.CO;2, 1977.
Varnai, T.: Influence of Three-Dimensional Radiative Effects on the Spatial Distribution of Shortwave Cloud Reflection, J. Atmos. Sci., 57, 216–229, https://doi.org/10.1175/1520-0469(2000)057<0216:IOTDRE>2.0.CO;2, 2000.
Varnai, T. and Davies, R.: Effects of Cloud Heterogeneities on Shortwave Radiation: Comparison of Cloud-Top Variability and Internal Heterogeneity, J. Atmos. Sci., 56, 4206–4224, https://doi.org/10.1175/1520-0469(1999)056<4206:EOCHOS>2.0.CO;2, 1999.
Varnai, T. and Marshak, A.: Observations of Three-Dimensional Radiative Effects that Influence MODIS Cloud Optical Thickness Retrievals, J. Atmos. Sci., 59, 1607–1618, https://doi.org/10.1175/1520-0469(2002)059<1607:OOTDRE>2.0.CO;2, 2002.
Waquet, F., Riedi, J., Labonnote, L.C., Goloub, P., Cairns, B., Deuzé, J.-L., and Tanré, D.: Aerosol Remote Sensing over Clouds Using A-Train Observations, J. Atmos. Sci., 66, 2468–2480, https://doi.org/10.1175/2009JAS3026.1, 2009.
Waquet, F., Cornet, C., Deuzé, J.-L., Dubovik, O., Ducos, F., Goloub, P., Herman, M., Lapyonok, T., Labonnote, L. C., Riedi, J., Tanré, D., Thieuleux, F., and Vanbauce, C.: Retrieval of aerosol microphysical and optical properties above liquid clouds from POLDER/PARASOL polarization measurements, Atmos. Meas. Tech., 6, 991–1016, https://doi.org/10.5194/amt-6-991-2013, 2013a.
Waquet, F., Peers, F., Ducos, F., Goloub, P., Platnick, S., Riedi, J., Tanré, D., and Thieuleux, F.: Global analysis of aerosol properties above clouds, Geophys. Res. Lett., 40, 5809–5814, https://doi.org/10.1002/2013GL057482, 2013b.
Wilcox, E. M.: Stratocumulus cloud thickening beneath layers of absorbing smoke aerosol, Atmos. Chem. Phys., 10, 11769–11777, https://doi.org/10.5194/acp-10-11769-2010, 2010.
Young, S. A. and Vaughan, M. A.: The Retrieval of Profiles of Particulate Extinction from Cloud-Aerosol Lidar Infrared Pathfinder Satellite Observations (CALIPSO) Data: Algorithm Description, J. Atmos. Ocean., Tech., 26, 1105–1119, https://doi.org/10.1175/2008JTECHA1221.1, 2009.
Zeng, S., Parol, F., Riedi, J., Cornet, C., and Thieuleux, F.: Examination of POLDER/PARASOL and MODIS/Aqua Cloud Fractions and Properties Representativeness, J. Climate, 24, 4435–4450, https://doi.org/10.1175/2011JCLI3857.1, 2011.
Zeng, S., Cornet, C., Parol, F., Riedi, J., and Thieuleux, F.: A better understanding of cloud optical thickness derived from the passive sensors MODIS/AQUA and POLDER/PARASOL in the A-Train constellation, Atmos. Chem. Phys., 12, 11245–11259, https://doi.org/10.5194/acp-12-11245-2012, 2012.
Zeng, S., Riedi, J., Parol, F., Cornet, C., and Thieuleux, F.: An assessment of cloud top thermodynamic phase products obtained from A-Train passive and active sensors, Atmos. Meas. Tech. Discuss., 6, 8371–8411, https://doi.org/10.5194/amtd-6-8371-2013, 2013.
Zhang, Z., Ackerman, A. S., Feingold, G., Platnick, S., Pincus, R., and Xue, H.: Effects of cloud horizontal inhomogeneity and drizzle on remote sensing of cloud droplet effective radius: Case studies based on large-eddy simulations, J. Geophys. Res.-Atmos., 117, D19208, https://doi.org/10.1029/2012JD017655, 2012.
Zhang, Z., Meyer, K., Yu, H., Platnick, S., Colarco, P., Liu, Z., and Oreopoulos, L.: Shortwave direct radiative effects of above-cloud aerosols over global oceans derived from 8 years of CALIOP and MODIS observations, Atmos. Chem. Phys., 16, 2877–2900, https://doi.org/10.5194/acp-16-2877-2016, 2016a.
Zhang, Z., Werner, F., Cho, H.-M., Wind, G., Platnick, S., Ackerman, A. S., Di Girolamo, L., Marshak, A., and Meyer, K.: A framework based on 2-D Taylor expansion for quantifying the impacts of subpixel reflectance variance and covariance on cloud optical thickness and effective radius retrievals based on the bispectral method, J. Geophys. Res.-Atmos., 121, 2016JD024837, https://doi.org/10.1002/2016JD024837, 2016b.
Zhou, Y., Sun, X., Zhang, R., Zhang, C., Li, H., Zhou, J., and Li, S.: Influences of cloud heterogeneity on cirrus optical properties retrieved from the visible and near-infrared channels of MODIS/SEVIRI for flat and optically thick cirrus clouds, J. Quant. Spectrosc. Ra., 187, 232–246, https://doi.org/10.1016/j.jqsrt.2016.09.020, 2017.
Zinner, T. and Mayer, B.: Remote sensing of stratocumulus clouds: Uncertainties and biases due to inhomogeneity, J. Geophys. Res.-Atmos., 111, D14209, https://doi.org/10.1029/2005JD006955, 2006.
- Abstract
- Introduction
- Description of the synthetically generated clouds and radiative transfer simulations
- Impacts on total reflectances and consequences for optical thickness and albedo retrievals
- Differences between 3-D and 1-D polarized reflectances and consequences for microphysical distribution, cloud pressure and aerosol above cloud retrievals
- Conclusions
- Data availability
- Competing interests
- Acknowledgements
- References
- Abstract
- Introduction
- Description of the synthetically generated clouds and radiative transfer simulations
- Impacts on total reflectances and consequences for optical thickness and albedo retrievals
- Differences between 3-D and 1-D polarized reflectances and consequences for microphysical distribution, cloud pressure and aerosol above cloud retrievals
- Conclusions
- Data availability
- Competing interests
- Acknowledgements
- References