the Creative Commons Attribution 4.0 License.
the Creative Commons Attribution 4.0 License.
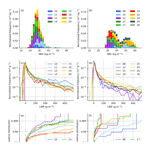
Investigating the liquid water path over the tropical Atlantic with synergistic airborne measurements
Felix Ament
Manuel Gutleben
Heike Konow
Mario Mech
Martin Wirth
Susanne Crewell
Liquid water path (LWP) is an important quantity to characterize clouds. Passive microwave satellite sensors provide the most direct estimate on a global scale but suffer from high uncertainties due to large footprints and the superposition of cloud and precipitation signals. Here, we use high spatial resolution airborne microwave radiometer (MWR) measurements together with cloud radar and lidar observations to better understand the LWP of warm clouds over the tropical North Atlantic. The nadir measurements were taken by the German High Altitude and LOng range research aircraft (HALO) in December 2013 (dry season) and August 2016 (wet season) during two Next-generation Advanced Remote sensing for VALidation (NARVAL) campaigns.
Microwave retrievals of integrated water vapor (IWV), LWP, and rainwater path (RWP) are developed using artificial neural network techniques. A retrieval database is created using unique cloud-resolving simulations with 1.25 km grid spacing. The IWV and LWP retrievals share the same eight MWR frequency channels in the range from 22 to 31 GHz and at 90 GHz as their sole input. The RWP retrieval combines active and passive microwave observations and is able to detect drizzle and light precipitation. The comparison of retrieved IWV with coincident dropsondes and water vapor lidar measurements shows root-mean-square deviations below 1.4 kg m−2 over the range from 20 to 60 kg m−2. This comparison raises the confidence in LWP retrievals which can only be assessed theoretically. The theoretical analysis shows that the LWP error is constant with 20 g m−2 for LWP below 100 g m−2. While the absolute LWP error increases with increasing LWP, the relative one decreases from 20 % at 100 g m−2 to 10 % at 500 g m−2. The identification of clear-sky scenes by ancillary measurements, here backscatter lidar, is crucial for thin clouds (LWP < 12 g m−2) as the microwave retrieved LWP uncertainty is higher than 100 %.
The analysis of both campaigns reveals that clouds were more frequent (47 % vs. 30 % of the time) in the dry than in the wet season. Their average LWP (63 vs. 40 g m−2) and RWP (6.7 vs. 2.7 g m−2) were higher as well. Microwave scattering of ice, however, was observed less frequently in the dry season (0.5 % vs. 1.6 % of the time). We hypothesize that a higher degree of cloud organization on larger scales in the wet season reduces the overall cloud cover and observed LWP. As to be expected, the observed IWV clearly shows that the dry season is on average less humid than the wet season (28 vs. 41 kg m−2). The results reveal that the observed frequency distributions of IWV are substantially affected by the choice of the flight pattern. This should be kept in mind when using the airborne observations to carefully mediate between long-term ground-based and spaceborne measurements to draw statistically sound conclusions.
- Article
(5251 KB) - Full-text XML
- BibTeX
- EndNote
Clouds and precipitation are a fundamental part of the Earth's climate system and significantly contribute to the water and energy cycle. However, the great variability of clouds, the complex interaction of small-scale processes involved, and their coupling to atmospheric circulation make them a major source of uncertainty in numerical climate and weather models (e.g., Bony et al., 2015; Boucher et al., 2013). Sherwood et al. (2014) attribute especially shallow marine clouds to contribute largely to the intermodel spread of climate models. Such clouds are particularly difficult to assess from spaceborne sensors due to their small size, with about 70 % appearing in sizes of less than 2 km over the tropical North Atlantic (Schnitt et al., 2017). The accurate observation of thin liquid clouds is an ongoing and important challenge as they cover more than a quarter of the globe and are an important contribution to Earth's energy balance (Turner et al., 2007).
Liquid water content (LWC) is the key parameter to describe clouds in atmospheric models. Due to the even higher difficulty in observing LWC profiles (Crewell et al., 2009), we focus on the liquid water path (LWP). It describes the total mass of all liquid water droplets in an atmospheric column above a unit surface area. However, care has to be taken as to whether LWP only denotes the contribution by cloud droplets, later on called CLWP, or whether it also includes the contribution by liquid precipitation, i.e., drizzle and raindrops (rainwater path, RWP). Thus, we define LWP as the sum of CLWP and RWP. Furthermore, the observed LWP per se is an average over the sensors' field of view, which is affected by cloud and rain inhomogeneity and the clear-sky contribution. Therefore, the spatial resolution is key information to interpret LWP statistics.
Few global (C)LWP datasets exist, and differences in global mean (C)LWP of a factor of 2 are reported by Lohmann and Neubauer (2018). These findings reflect the different sensing principles, i.e., microwave radiometry and visible–near-infrared techniques. Satellite microwave imagers such as the Special Sensor Microwave Imager (SSM/I) provide CLWP estimates for several decades but are limited to the ice-free oceans where the background signal is low. (C)LWP is mainly derived from the thermal emission signal in window regions with low water vapor contribution. Microwave receivers also sense rainwater within the satellite footprint which can be as large as several tens of kilometers. Recently, the Multisensor Advanced Climatology of Liquid Water Path (MAC-LWP; Elsaesser et al., 2017) covering the period 1988 to 2016 has been generated. Elsaesser et al. (2017) additionally estimate the contribution of RWP to the total LWP by a simple parametrization and recommend only using those values with a ratio RWP : LWP of less than 0.2. The average MAC RWP : LWP ratio in our area of interest is 0.23 and 0.30 in December 2013 and August 2016, respectively. Therefore, a more detailed assessment of the rain cloud partitioning is important to better interpret satellite measurements in our study area. Greenwald et al. (2018) evaluate MAC-LWP using measurements by the Moderate Imaging Spectroradiometer (MODIS), the CloudSat Profiling Radar (CPR; Stephens et al., 2002), and the Cloud-Aerosol Lidar with Orthogonal Polarization (CALIOP; Winker et al., 2007). They found in some cases a net LWP bias of more than 50 % of the mean CLWP due to the combined effects of the in-cloud and adjacent precipitation biases as well as the cloud–rain partition.
Visible–near-infrared techniques such as those applied to MODIS exploit the spectral response of reflected sunlight to derive CLWP from optical depth and effective radius retrievals and are therefore limited to daytime. As the signal mainly relates to the upper part of the cloud, assumptions of the cloud vertical structure introduce uncertainties (Zhou et al., 2016). The horizontal MODIS resolution of about 1 km is much better than that of microwave satellites. Therefore, MODIS data have also been used to assess the clear-sky bias of microwave retrievals (Greenwald et al., 2018), to combine them with microwaves for a better assessment of low clouds (Masunaga et al., 2002), and to detect the ratio of rainwater and cloud water in low-latitude shallow marine clouds via combination with CPR (Lebsock et al., 2011). In summary, quantifying the accuracy of CLWP and RWP observations is a major challenge as no absolute reference exists. While shipborne microwave observations have potential for satellite CLWP evaluation (Painemal et al., 2016), they fail during precipitation events, due to a wet radome.
In this study, we use the Next-generation Advanced Remote sensing for VALidation studies (NARVAL; Stevens et al., 2019) expeditions for investigating LWP and its uncertainty over the tropical North Atlantic. The NARVAL missions aim at improving the understanding of clouds, their role in the distribution of water in the atmosphere, and their interaction with the environment (Bony et al., 2015). Within NARVAL, the German High Altitude and LOng range research aircraft (HALO; Krautstrunk and Giez, 2012) was configured as an airborne cloud observatory combining active and passive microwave instruments with water vapor lidar, solar reflectance measurements, and dropsondes. Measurements taken during two campaigns in December 2013 (dry season) and in August 2016 (wet seasons) allow the study of clouds with similar but more sensitive and higher spatially resolving instrumentation than that available on satellites.
Schnitt et al. (2017) demonstrate the ability of the HALO NARVAL 2013 instrumentation to characterize shallow clouds over the tropical North Atlantic in terms of size, integrated water vapor (IWV), CLWP, and surface reaching precipitation using classical regression algorithms. Their study uses the 1 km resolution HAMP data to show the sub-footprint variability of spaceborne CLWP estimation of about 30 km resolution. Further they illustrate how MODIS products at 1 km resolution likely underestimate CLWP of thick clouds due to MODIS' sensitivity towards the upper part of the cloud. In this study, we refine the (C)LWP retrieval by making use of high-resolution simulations that start to resolve cloud-scale circulations and were performed over the full tropical North Atlantic with the ICON (ICOsahedral Non-hydrostatic) weather model to support the analysis (Klocke et al., 2017). We further assess the total LWP retrieval accuracy over a wide range of cases, extend the retrieval towards a separation of rain and clouds, and reanalyze the dry season measurements in relation to the wet season campaign.
First, we aim to provide an accurate LWP dataset including uncertainty estimates to support the NARVAL overall goals. For this purpose, we develop retrieval algorithms using multi-channel microwave radiometer measurements as input for LWP and – based on the similar principle – IWV. The novel cloud-resolving ICON simulations serve as a training dataset (Sect. 2). In contrast to LWP, IWV can be evaluated using simultaneous measurements by dropsondes and water vapor lidar. The evaluation is presented in Sect. 3. The assessment of LWP (Sect. 4) reveals the importance of using ancillary measurements, e.g., lidar measurements, for low LWP values and cloud radar measurements for lightly precipitating cases. For the latter an RWP retrieval is developed and assessed (Sect. 5). Finally, the campaign data are analyzed to investigate differences between dry and wet seasons (Sect. 6).
This section presents the data and methods used in this study. That includes an introduction to the two NARVAL campaigns and the relevant measurements that were conducted during both campaigns. Furthermore, the generation of the retrieval database and the subsequent retrieval development are presented.
2.1 Campaign overview
During the NARVAL expeditions HALO was operated out of Grantley Adams International Airport in Barbados to observe trade wind cumuli and their environment over the tropical North Atlantic (Fig. 1). Different flight patterns were chosen to perform satellite underflights, survey the area, probe the environment of a tropical cyclone, and to determine the large-scale vertical motion by launching several dropsondes within circles of approx. 170 km diameter (Bony and Stevens, 2019). In total eight research flights were performed during NARVAL1-South in December 2013 and 10 flights during NARVAL2 in August 2016. NARVAL1 also included research flights in the northern sector of the Atlantic which are not considered here. For simplicity we refer to the southern part as NARVAL1 in the following.
Flights were scheduled during local daytime. Flight altitudes varied between 6.4 and 15.0 km, with an average speed above ground of 237 and 207 m s−1 during NARVAL1 and NARVAL2, respectively. All further analyses refer to the area from 37 to 60∘ W and 7 to 20∘ N. A detailed description of the different research flights can be found in Klepp et al. (2014) for NARVAL1 and Stevens et al. (2019) for NARVAL2.
2.2 Measurements
The microwave radiometer (MWR) being part of the HALO Microwave Package (HAMP; Mech et al., 2014) provides the key measurements for this study. HAMP was installed in a belly pod below the HALO fuselage in nadir-looking configuration. While the 26-channel MWR includes channels from 22 to 195 GHz, we only use the seven K-band channels (22.24–31.40 GHz) and the 90 GHz channel to retrieve LWP or IWV in the present work. At these frequencies ice particles do not influence the microwave signal substantially, with the exception of precipitation sized particles.
As we focus on warm clouds only, cases of ice precipitation are filtered using the differential response of two frequencies along the 60 and 118 GHz oxygen lines. The channels at 53.75 and 118.75±1.4 GHz have similar temperature weighting functions, but the higher frequency is more affected by ice scattering. The difference between a moving median of differential brightness temperature (BT) to the instantaneous differential BT is used to define the “ice flag”. This procedure flags 1.2 % of the measurements of both campaigns.
Both liquid water and water vapor emit microwave radiation across the full microwave spectrum albeit with different spectral sensitivity (Fig. 2). BTs around the 22.235 GHz water vapor rotational line increase with increasing water vapor. The effect is strongest at the line center and decreases along the pressure-broadened wing of the absorption line. However, due to water vapor continuum absorption, BTs at window frequencies near 30 and 90 GHz are still affected. In contrast, the influence of liquid water is more dominant in the higher-frequency window channels than in absorption channels due to increasing emission with frequency. This can be best seen under low-humidity conditions by the increasing BT with increasing frequency. The near-surface wind speed slightly alters the BTs through modification of surface reflectivity and emissivity, as also shown in Fig. 2. This influence will act as a random source of error on the LWP and IWV retrievals as no independent information to correct for wind influence is available.
Figure 2 illustrates the difficulty of retrieving LWP and IWV as in certain channels (e.g., 90 GHz) it is indistinguishable whether BT changes result from changes in IWV or LWP. Therefore, a combination of at least two channels is needed for retrieving IWV or LWP. Note that measurement errors in any of the channels affect both IWV and LWP retrievals (Crewell and Löhnert, 2003). This means that a good retrieval of either IWV or LWP indicates a good retrieval capability of the other. Thus, an accurate IWV retrieval is a prerequisite of a good LWP retrieval. Note that in most LWP retrievals (e.g., Wentz and Meissner, 2000) the liquid is assumed to consist of cloud droplets only, and therefore bulk approaches to calculate the liquid water absorption coefficients are used. However, for raindrops the Rayleigh approximation is not valid anymore, and Mie effects need to be considered, though the discrimination of the cloud and rain signal using MWRs is difficult.
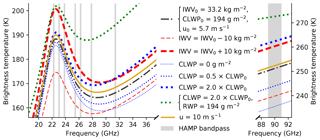
Figure 2Sensitivity of brightness temperatures in the K band and around 90 GHz to integrated water vapor (IWV), cloud liquid (CLWP), and rain water path (RWP), and 10 m surface wind (u). Dashed, dotted, and solid lines show variations in IWV and LWP (CLWP or RWP) and u, respectively. Bandpasses of the HALO Microwave Package (HAMP) channels are indicated by gray bars. Calculations are based on a thermodynamic dropsonde profile and a synthetic cloud in nadir geometry above the ocean.
The HAMP MWR measures BT with 1 s integration time and a noise level of less than 0.5 K in the considered channels. Despite ground calibration using hot/cold targets on the air field, BT offsets were identified by comparison with forward simulated dropsondes. Flight-dependent corrections were developed (Konow et al., 2018a), and corrected BTs are available in the Climate and Environmental Retrieval and Archive (CERA) (Konow et al., 2018b, c).
HAMP also includes a 35.5 GHz cloud radar with a sensitivity of −30 dBZ at 13 km distance in the NARVAL setup. Profiles of the radar reflectivity factor (Z) and the linear depolarization ratio are recorded with 30 m vertical and 1 s temporal resolution. To supplement HAMP, Vaisala RD94 dropsondes were launched from HALO to provide the thermodynamic conditions of the environment. In total 76 and 215 sondes were released during NARVAL1 and NARVAL2, respectively.
To distinguish between clear-sky and cloudy conditions as well as possible, Schnitt et al. (2017) derive a cloud mask for NARVAL1 based on the nadir spectral solar radiance measurements by HALO-SR (HALO Solar Radiation; Fricke et al., 2014). Unfortunately, sun glint in August deteriorated the cloud mask retrieval during NARVAL2. Therefore, Gödde (2018) developed a cloud mask product using the imaging spectrometer specMACS (spectrometer of the Munich Aerosol and Cloud Scanner; Ewald et al., 2016) which overcomes the sun glint problem. However, specMACS was not installed during NARVAL1. In order to have similar cloud mask performance during both campaigns the aerosol backscatter profile measured by the WAter vapor Lidar Experiment in Space (WALES) airborne demonstrator (Wirth et al., 2009) is used instead to provide an along-track cloud mask with 1 s resolution.
WALES also provides profiles of water vapor molecular number density based on the differential absorption lidar (DIAL) principle. These profiles are converted to volume mixing ratio profiles using temperature and pressure data from ECMWF analyses. A resolution of about 200 m vertical and 12 s temporal was chosen as a compromise between accuracy and resolution. The water vapor data are given on the vertical grid of the raw backscatter data which is 15 m but smoothed with an averaging kernel of 200 m width (full width at half maximum, FWHM). Water vapor profiles are provided down to about 250 m in cases with no or optically thin clouds, which can be penetrated by the lidar beam. Water vapor information is available below thin clouds, but the cloud itself is masked out in the profile.
The requirement of simultaneous measurements by all sensors reduces the dataset. While all research flights during NARVAL1 can be used, no data is available for some NARVAL2 flight days due to hardware issues as summarized in Table 1. The spatial sampling differs, even with the same temporal sampling due to footprints differences. The HAMP MWR has the largest beamwidth in its lowest-frequency channel of 5.0 (FWHM). The corresponding surface footprint at 10 km altitude is about 870 m across and 1090 m along track. The HAMP radar beamwidth is 0.6, whereas WALES has a field of view of 1.6 mrad. The respective footprints are about 105 m×335 m and 16 m×216 m. We reduce the along-track sampling differences by averaging temporally, but the cross-track sampling issues remain. This means a cloud covering a lateral part of the MWR footprint might be missed by the lidar or even the radar. Cross-track imagers such as specMACS could be used to assess these issues. However, specMACS was only installed on HALO for NARVAL2, and the detailed analysis of HAMP beam filling is beyond the scope of this study. The problem of different footprints and sensitivities of different NARVAL instruments for cloud masking is illustrated by Stevens et al. (2019).
2.3 Retrieval database
Recently, high-spatial-resolution simulations with the storm-resolving ICON model were able to show how resolved convection and its associated circulation interact with and form the larger-scale circulation within the Atlantic intertropical convergence zone (Klocke et al., 2017). These simulations serve as training and testing data for the retrieval algorithms. The simulations were performed on a triangular grid, with a horizontal spacing of about 1.25 km and 75 vertical levels. The simulations cover the area of 4∘ S to 18∘ N and 64 to 42∘ W. The data were spatially subsampled to reduce the computational effort while still covering the variability of atmospheric profiles. To eliminate atmospheric columns with a high degree of correlation, columns are selected on a 0.5×0.5 longitude–latitude grid, so that each time step includes 849 cases over the ocean as indicated in Fig. 1. Data from 24 d with hourly outputs each, spanning the period of each campaign, are alternately separated into test and training data. In general, the training and test data exclude cases with LWP greater than 1000 g m−2, and cases with ice. This means 86 % of all profiles over the ocean are used. This limitation is done as our focus is on liquid clouds and their transition to rain. Note that classical satellite algorithms (e.g., Wentz and Meissner, 2000) are trained with an upper LWP limitation of 300 g m−2.
Synthetic HAMP measurements, i.e., BTs and radar reflectivity profiles in nadir view, are simulated for each model column based on its thermodynamic profile and hydrometeors (cloud liquid water, rain, cloud ice, snow, and graupel). The Passive and Active Microwave TRAnsfer code (PAMTRA; Maahn et al., 2015; Cadeddu et al., 2017) is used. It is configured with 27 output levels to mimic different flight altitudes (6–15 km). The ICON model was set up with a one-moment microphysics scheme (Baldauf et al., 2011). In PAMTRA, cloud and rain particles and their size distributions are described according to the microphysical scheme of ICON, and the single scattering properties for each particle are approximated by the Lorentz–Mie theory. Cloud and rain particles are simulated with a 20 µm diameter monodisperse and exponential distribution of water spheres, respectively. The exponential distribution has its intersect N0 classically fixed to 0.08 cm−4 (Marshall and Palmer, 1948). Absorption coefficients of atmospheric gases (i.e., oxygen, water vapor, nitrogen) are calculated according to Rosenkranz (1998), with corrections of the water vapor continuum absorption according to Turner et al. (2009) and the line width modification of the 22.235 GHz water vapor line as proposed by Liljegren et al. (2005). The emissivity and reflectivity of the sea ocean surface are calculated using the FAST microwave Emissivity Model version 5 (FASTEM5; Liu et al., 2011), which is a modification of the Fresnel coefficients including corrections for ocean surface roughness and foam building as a function of wind speed.
To test the realism of the retrieval database, histograms of BTs were compared with their observed counterparts. Joint histograms of an absorption (22 GHz) and a window channel (31 or 90 GHz) show that the relations between channel pairs are depicted in the model and observations in the same way (Fig. 3). In clear-sky conditions absorption and window channels are highly correlated, with both increasing with increasing moisture, albeit the increase is less in the window channels. Clear-sky cases with low BT31 and BT90 are visible as a line of high occurrence and reveal the linear relation between absorption and window channel BTs as a function of IWV. The simulations and measurements show the same relations but differ slightly in terms of the BT combination distribution within this line as the underlying IWV sampling is slightly different. If liquid water clouds occur, the window channel BTs increase compared to clear-sky cases (solid lines in Fig. 3). The window channel at 90 GHz has a higher sensitivity towards LWP compared to BT31 as it can be seen by the increased LWP line spread. Rainy cases show higher emissions in all channels (dotted lines in Fig. 3). For thick clouds and rain the most-liquid-sensitive channel (90 GHz) experiences saturation effects, with BT90 approaching cloud temperatures. The joint histograms reveal the major signals by liquid and water vapor which are exploited within retrieval algorithms. However, multiple influence factors like the exact vertical structure lead to the variability illustrated in Fig. 3. Minor deviations between observations and simulations are visible in the frequency of combinations of BT31 and BT90 with high BT22. Those combinations are associated with heavy precipitation and were observed less frequently than present in the model as flight patterns avoided the heaviest precipitation.
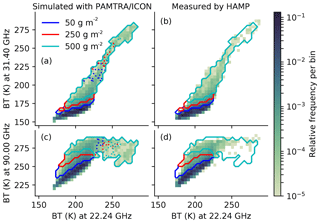
Figure 3Relation between brightness temperatures (BT) in an absorption channel (22.24 GHz) and two window channels, i.e., 31.40 GHz (a, b) and 90.00 GHz (c, d). Two-dimensional histograms of occurrences in simulations (a, c) and HAMP measurements (b, d). Solid contours highlight BT combinations in simulations that mostly occur with LWP higher than 50, 250, and 500 g m−2. Dotted lines highlight combinations of which RWP mostly exceeds the respective threshold. LWP contours in (b) and (d) are taken from (a) and (c) for guidance. HAMP data from all NARVAL2 flights and ICON–PAMTRA data of the corresponding dates are used. Profiles and measurements with ice are excluded.
2.4 Retrieval
The atmosphere emits radiation depending on the atmospheric state as illustrated in Fig. 2. In general, the retrieval of the atmospheric state from MWR measurements is under-determined as multiple atmospheric states can lead to the same set of BTs. Statistical relations have to be established to link the measurement to the most common atmospheric state that can provide those measurements. To account for nonlinearity in this inverse modeling problem, we use an artificial neural network (NN) model similar to Cadeddu et al. (2009) to relate BTs to IWV and LWP. The NN is configured with eight input neurons and 15 hidden neurons in one hidden layer.
For testing and training the retrievals, Gaussian noise of 0.5 K is added to the simulated BTs to account for uncertainties of the HAMP MWR, the radiative transfer, and absorption model. The database is separated by alternating days into test and training data. Retrieval parameters are derived for each PAMTRA output level to account for the altitude dependence of the microwave signal. The output levels are chosen such that a HALO flight level never deviates more than 90 m from the next output level. The parameters at output levels closest to HALO's altitude are interpolated to HALO's altitude in the retrieval application. Retrieval parameters are derived separately for both campaigns. For testing, each retrieval is applied to the test data of the campaign it is trained for.
In the retrievals, IWV and LWP, and later CLWP and RWP, are the integrals of the water vapor and liquid water over the whole column as seen from space. Despite the fact that HALO flies lower, we chose the total integrals as they prevent artificial flight-level-dependent biases in statistics and allow a comparison with satellite and model data. According to ICON model data, typically less than 0.1 kg m−2 water vapor is above a flight altitude of 10 km. About 1 kg m−2 of IWV is not seen by the MWR at the lowest NARVAL2 flight altitude of 6.4 km but is included in the retrieval. The LWP retrieval is trained with the integral of all liquid water, that is given by the model either as cloud water or rainwater. The sum of both is used due to the difficulty of MWRs to distinguish clouds and rain (Fig. 2).
Neural network LWP retrievals are compared with linear regression (REG) models as used by Schnitt et al. (2017). The regression relates measured brightness temperatures BTi to LWP including the quadratic terms of BTi:
where ai, bi, and c are regression coefficients. Such REGs are less susceptible than NNs to extrapolation towards unforeseen input data, i.e., data values or combinations that are not covered by the training data. However, NNs are better in representing nonlinear effects that are apparent in microwave radiative transfer and thus can better adjust to the extremes of the LWP target space. The application of the retrievals to test data reveals overall uncertainties between 0.5 and 0.6 kg m−2 for IWV for both approaches, i.e., NN and REG, and 22 and 26 g m−2 for LWP using the NN and REG, respectively. For LWP the uncertainty strongly depends on atmospheric conditions as will be investigated in Sect. 4.
When retrieval algorithms are applied to HALO measurements, slight biases of LWP from 0 with slow changes over time are observed during clear-sky scenes. To reduce these biases and to improve the retrieval of low LWP values, we follow the synergistic approach by van Meijgaard and Crewell (2005). Herein, we use the WALES cloud mask for clear-sky identification. HAMP measurements are considered clear sky if no cloud is detected by WALES within ±2 s flight time. The distance-weighted average clear-sky LWP within ±30 min is then subtracted from each a priori retrieved LWP value.
In thick clouds we occasionally observed that while the REG retrieval gave LWP >1000 g m−2, the NN LWP time series showed a sudden decline. This is likely caused by the clipping of the NN retrieval at 1000 g m−2, which is expected as the retrieval database is limited to LWP < 1000 g m−2, and thus BTs associated with higher amounts of liquid are unknown to the retrieval. To avoid this behavior, we use a second NN retrieval trained with an extended database up to 4000 g m−2 to flag scenes that are potentially above 1000 g m−2. Overall, 0.76 % of the measurements were masked in this way. Note that these measurements often coincide with ice scattering depressions in channels at higher frequencies.
To retrieve the contribution of raindrops (RWP) to the total LWP, the vertically integrated radar reflectivity is used in addition to the MWR channels in another NN retrieval. The aim is separating the LWP into CLWP and RWP, i.e., splitting the contributions from small cloud droplets and larger raindrops by estimating the fraction
This retrieval is based on the hydrometeor classes of rain and cloud liquid water in the ICON model. The RWP is calculated by multiplying f and the retrieved total LWP.
Three independent methods to derive IWV are available from HALO: the MWR retrieval, vertically integrated humidity from dropsondes, and vertically integrated humidity from WALES. Each of the three methods has its advantages and shortcomings. The microwave radiometry can not provide profile information but gives continuous IWV under nearly all-sky conditions. The dropsondes provide in situ measurements but no valid data up to about the first half kilometer below the aircraft because of the sensor's adjustment from the aircraft cabin conditions to the outside. Furthermore, wind drifts sondes out of the aircraft nadir with a typical horizontal drift during the decent of 4 km. The dropsonde relative humidity sensor has a repeatability of 2 % according to the manufacturer (Vaisala, 2017). This relates to an IWV accuracy of about 1.4 kg m−2. WALES provides water vapor profiles, but they are only available when no cloud extinguishes the laser beam. This limits the application of WALES for the IWV retrieval to clear-sky scenes.
To compute the numerical derivative in the DIAL equation, the first data point is at about 250 m above the sea surface and centered at the retrieval interval. Therefore, in the vertical integration, the missing near-surface information is filled with the median mixing ratio in the lowest five range bins. The median is chosen to reduce any surface artifacts which can occur, when the first raw data signal point used in the retrieval contains the surface reflex. We estimate that the error of this assumption is about 0.3 kg m−2 by analyzing dropsonde humidity profiles. The IWV estimation is discarded if information of more than 400 m above sea level is missing or there is a gap due to a thin cloud. Also, stability of the estimated WALES IWV is required, which means that the differences to the preceding and succeeding IWV estimations have to be smaller than 2 kg m−2.
An example of water vapor retrievals on 19 August 2016 is shown in Fig. 4. An elevated moisture layer between 3 and 4 km altitude is visible in the first half of the scene. Around 14:53 a plume of moist air reaching up into even higher levels causes an IWV gradient of nearly 10 kg m−2 (26–35 kg m−2) over a distance of about 110 km. This gradient is captured well by WALES and HAMP. The two dropsondes that were released between 14:45 and 14:55 reconstruct this gradient, but both have a dry offset. This offset might be due to drifting of the sonde towards the drier air mass. After a short outage of WALES at around 15:00, shallow clouds below 2 km prevent the determination of lidar IWV frequently. Most of the IWV measurements from dropsondes agree with the coincident remote sensing estimates within the sondes' uncertainty.
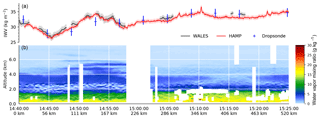
Figure 4Water vapor time series from NARVAL2 research flight 6 on 19 August 2016. (a) IWV time series by HAMP, WALES, and dropsondes with their uncertainties. (b) WALES water vapor profiles. White areas denote masked lidar data. The scene represents a circle around 14.8∘ N and 51.0∘ W over a distance of 520 km.
A more quantitative comparison is achieved by considering all measurements from both campaigns which cover a wide variety of water vapor conditions (Fig. 5). Overall, the sondes agree well with HAMP over the whole observed range from very low (20 kg m−2) to very high (60 kg m−2) values of IWV (Fig. 5a). The root-mean-square deviation (RMSD) is 1.39 kg m−2 (1.28 kg m−2), with a mean bias of 0.28 kg m−2 (0.47 kg m−2) during NARVAL1 (NARVAL2) as summarized in Table 2. The positive biases of HAMP are most likely caused by the retrieval, which is trained with the whole column IWV, whereas the sonde IWV is only integrated along its measurement path. Most sondes were released above 9 km which would miss an IWV of about 0.2 kg m−2 according to ICON data. Note that dropsondes released from below 6.5 km are discarded in the comparison to avoid an artificial bias. The random error between HAMP and sondes (1.2 kg m−2) is smaller than the estimated uncertainties of the dropsonde (1.4 kg m−2) and the MWR retrieval (0.6 kg m−2), which indicates the high quality of the measurements as uncertainties due to spatiotemporal mismatch are included in the RMSD as well. Note that uncertainties due to MWR calibration are largely compensated for as offsets between measured BT and those derived by radiative transfer calculations based on dropsondes have been corrected by Konow et al. (2018a).
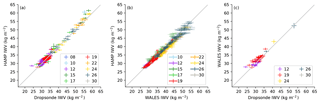
Figure 5IWV comparison of HAMP MWR and dropsondes (a), HAMP MWR and WALES (b), and WALES and dropsondes (c) during NARVAL2. The colors indicate the flight days in August 2016. Scores are given in Table 2.
WALES IWV can be used for continuous comparison to HAMP IWV along the flight track in clear-sky scenes. A comparison of all coincident measurements during NARVAL2 is depicted in Fig. 5b. The average bias between HAMP and WALES IWV is −0.59 kg m−2. The bias is cut in half when considering only the 40 simultaneous measurements during which a dropsonde was launched (Table 2). The random error is smaller in contrast to the HAMP–dropsonde comparison. This is likely due to the better spatial match between the two nadir measurements compared to a drifting sonde. However, higher RMSD between HAMP and WALES IWV can be found during NARVAL1, which is mostly related to a higher bias. The bias increases to 1.70 kg m−2 in the HAMP–WALES comparison when only considering measurements during which a sonde was released. A bias of similar magnitude is apparent between WALES and the dropsondes. Most likely the dry bias of WALES is due to the method of how the 12s water vapor profiles are derived. The profiles only contain raw profiles (within the 12 s) that are not blocked by a cloud. For small-scale boundary layer convection, this means preferred sampling of downdraft regions. In these downdraft regions dry air is entrained from the rather dry free troposphere into the convection layer during NARVAL1 (Stevens et al., 2017). This results in biased sampling of rather dry profiles. During NARVAL2 humidity was reaching higher altitudes, which resulted in less entrainment of dry air in cloud gaps.
Table 2Comparison of IWV retrieved from HAMP, WALES, and dropsondes. Pairwise observations of two instruments and the subsets of the observations for that all instruments were available. Bias, root-mean-square deviation (RMSD), and bias-corrected RMSD (standard deviation, SD) in kilograms per square meter (kg m−2). Observed by all implies only small or no clouds.
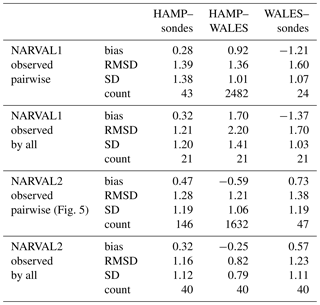
A small confounding effect from liquid water in cloudy scenes can be derived from the separation of the HAMP–dropsonde comparison into all (observed pairwise) and clear sky (observed by all, i.e., when WALES is also available) in Table 2. In the NARVAL1 dataset, the bias for cloudy-sky sondes (0.24 kg m−2) is somewhat smaller than that for clear sky (0.32 kg m−2). However, RMSD and SD in cloudy scenes are about 0.3 kg m−2 larger than in clear sky. NARVAL2 also shows a larger bias in cloudy sky of about 0.53 kg m−2 in comparison to clear sky (0.28 kg m−2). The cloudy-sky RMSD and SD of 1.32 and 1.21 kg m−2, respectively, are only slightly larger than their clear-sky counterparts. An increase of the random error for cloudy scenes is expected as also higher water vapor variations are expected in heterogeneous cloud fields.
With the exception of the HAMP–WALES comparison during NARVAL1, the RMSD between the different instrument pairs is found between 0.8 and 1.4 kg m−2 (Table 2). This corresponds to an error of 2 % to 7 % over the observed range of 20 to 60 kg m−2. For comparison, Mears et al. (2015) found random IWV deviations between different spaceborne MWR and ground-based GPS (Global Positioning System) instruments of 1.7 to 2.0 kg m−2 over a similar IWV range using 26 small island stations located mainly in the tropics.
The HAMP IWV retrieval has a theoretical uncertainty of about 0.6 kg m−2, which is derived by applying the IWV retrieval to simulated measurements from the test database (Sect. 2.4) and is constant over a wide IWV range (not shown). This is well in line with the RMSD derived in the pairwise comparisons, taking into account the estimated uncertainties of WALES and dropsondes as well as uncertainties due to the spatiotemporal mismatch. In summary, the pairwise comparisons in relation to the individual uncertainties indicate high HAMP IWV performance and the suitability of our retrieval approach.
There are no independent measurements of sufficient quality to assess the quality of the LWP product. However, the large retrieval database (173 339 ice-free cases in the test dataset) allows a theoretical in-depth analysis of the retrieval performance. This approach is supported by the good consistency between the BTs in the database and the HAMP measurements in terms of relation resemblance (Fig. 3) and performance of IWV retrieval (Sect. 3). We analyze the retrieval error as a function of the true LWP as well as of the retrieved LWP using the database.
First, we analyze the difference of retrievals developed with all ice-free cases of the training database (all sky) and with cloudy cases only, which reduces the dataset size to about one-quarter. A model profile is regarded as cloudy if LWP > 1 g m−2. REG and NN retrievals are trained with the all-sky and the cloudy-sky datasets separately. The errors of retrieved LWP from the test database are calculated for bins of the true LWP. Both REG and NN show similar behavior of the RMSD between the retrieved LWP and the model truth with increasing LWP (Fig. 6a). The RMSD is constant for LWP below about 30 g m−2 and increases with LWP, e.g., 50 at 500 g m−2. For LWP values > ∼800 g m−2 the number of test cases reduces strongly, leading to less robust results. For LWP < 30 g m−2, the errors are smaller for REG and NN retrieval types if the clear-sky cases are included in the training (compare Fig. 6a and b). Including clear sky in the training, the retrieval errors decrease slightly for a REG model and are almost cut to half for an NN. This shows the ability of an NN to nonlinearly relate a variety of BT combinations to zero LWP. However, retrievals that are especially trained for all-sky scenes have a considerably larger RMSD for LWP > 20 g m−2 than those trained with cloudy cases only as clear-sky cases make up 77 % of the data. Since we are targeting clouds and not clear sky, we chose a retrieval trained with data excluding the clear-sky model profiles. Instead of including clear sky directly in the retrieval, we make use of lidar measurements, which are better suited than MWR for cloud masking.
Regarding cloudy-sky retrievals, the RMSD for a given (true) LWP less than 40 g m−2 is smaller when using the NN retrieval instead of a REG model (Fig. 6a). This is related to a suppression of unphysical negative LWP values by the NN. Thus, in contrast to a REG which has a nearly Gaussian error characteristic, the NN tends to overestimate LWP. This results in a more negative mean LWP error (true minus retrieved) of clouds with less than 10 g m−2 but also in a smaller interquartile range of errors when using the NN instead of the REG. However, the retrieval error for true LWP < 10 g m−2 remains on the order of 10 to 18 g m−2, even when using the NN.
The bias errors visible in Fig. 6a can not be used to adjust the retrieved LWP as the true LWP value is not known in practice. For the application of the error analysis on measurements, it is important to analyze the LWP error as a function of the retrieved LWP. The RMSDs of the NN and REG retrievals are larger than 100 % for a retrieved LWP below 12 g m−2, which can be regarded as a detection limit (Fig. 7). Therefore, ancillary measurements with higher sensitivity are needed to detect these thin liquid water clouds. The RMSD is below 20 g m−2 for REG LWP < 50 g m−2 and NN LWP < 100 g m−2 and moderately increases with increasing LWP. Therefore, the relative RMSD decreases from 50 % for a retrieved LWP of about 40 g m−2 to 20 % for LWP > 100 g m−2 for both retrieval types. While the RMSD is rather similar for REG and NN, the NN succeeds in capturing the nonlinear retrieval, providing a nearly zero bias across the full LWP range, and is therefore preferred over REG.
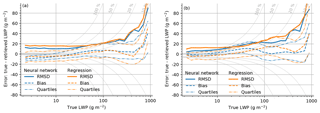
Figure 6Expected retrieval error as a function of true LWP for neural network and linear regression LWP retrievals. Retrievals (a) trained for 1 g m−2 < LWP < 1000 g m−2. Retrievals (b) trained including clear-sky cases (LWP < 1000 g m−2). Error measures (colored lines) for logarithmically distributed bins with 10 bins per LWP power of 10. Gray dashed lines denote the corresponding relative LWP error.
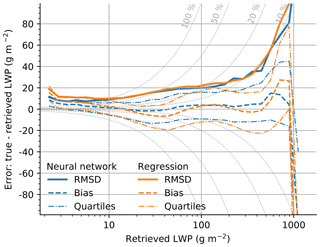
Figure 7As Fig. 6 but with errors shown as a function of retrieved LWP. Retrievals are trained and tested with 1 g m−2 < LWP < 1000 g m−2. The first bin contains all data with retrieved LWP < 2.5 g m−2 (including negative).
Analyzing the retrieved LWP distribution for clear-sky scenes is a widely used method to assess an LWP retrieval (e.g., Liu et al., 2001; Greenwald et al., 2018) because this characterization can be made from measurements using ancillary observations that define clear-sky scenes. We use WALES measurements for the indication of cloud and clear sky. The distributions of LWP from HAMP MWR are depicted in Fig. 8 for observed clear-sky scenes (blue lines) along the track for both campaigns. The distributions are compared to the theoretical ones of retrieved LWP from all clear-sky (true LWP < 1 g m−2) cases of the respective campaign in the ICON–PAMTRA database (orange lines in Fig. 8). The latter distributions are closely related to the retrieval uncertainty of the lowest LWP bin in Fig. 6a as this represents the retrieval uncertainty for true LWP < 2.5 g m−2. The distributions roughly resemble Gaussian behavior, with mean values of about 10 g m−2 and widths of about 9 g m−2. Some differences between NARVAL1 and NARVAL2 exist which are even stronger for the measured distributions. During NARVAL1, the measured distribution is skewed towards higher values. This might be caused by cloud patches that were only present in a lateral part of the MWR footprint such that the scene was falsely identified as clear sky by the lidar, which only slices though the center of the MWR footprint. As this effect is not visible for NARVAL2 measurements, it might be that clouds were generally smaller and more frequent during NARVAL1 (see Sect. 6).
For both campaigns the similar widths and standard deviations of the retrieved LWP indicate a good agreement between simulations and measurements for clear sky (Fig. 8). The apparent second mode at 20 g m−2 in the observed clear-sky LWP distribution during NARVAL2 is caused by different mean deviations during different flights, probably influenced by the calibration. Overall, the narrow Gaussian widths (11.4 and 8.3 g m−2 for NARVAL1 and NARVAL2) of the retrieved clear-sky LWP distributions demonstrate the good performance of HAMP compared to evaluation studies by Liu et al. (2001) (28 g m−2, airborne) and Greenwald et al. (2018) (∼30 g m−2, satellite). The better HAMP performance is likely due to its smaller footprint, additional frequency channels, and more recent technology. The sensor synergy of using the lidar cloud mask for clear-sky bias correction (Sect. 2.4) reduces the bias in clear-sky conditions to values barely above zero as small cloud patches can still be in the outer area of the MWR footprint, which is not transected by the lidar beam. The bias correction further narrows the clear-sky LWP distributions. Note that a good agreement (small bias) is expected as the lidar cloud mask is also used to define clear sky. The deviations of the observed clear-sky LWP distributions from delta distributions are due to the moving window in the bias correction.
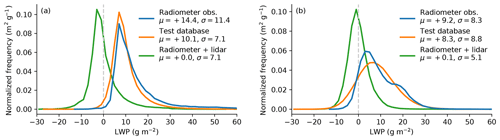
Figure 8LWP distributions retrieved during clear-sky scenes only. Shown are the LWP retrieved with the neural network based on radiometer observations (blue lines) during (a) NARVAL1 and (b) NARVAL2, the retrieved LWP from the test database (orange lines) for profiles with LWP < 1 g m−2, and the observed LWP after applying the lidar clear-sky correction (green lines). Mean (μ) and standard deviation (σ) are given for each distribution in grams per square meter (g m−2).
In summary, the ICON–PAMTRA database allows the expected uncertainty of the LWP retrieval to be estimated. This reveals a lower retrieval limit of about 12 g m−2 and an RMSD below 20 g m−2 for LWP below 100 g m−2 and below 20 % above 100 g m−2 for the NN retrieval. A narrow clear-sky distribution of HAMP measurements (SD ∼10 g m−2) is found that is in good agreement with the theoretical assessment, but a small bias on the order of 12 g m−2 remains, which is eliminated by the clear-sky correction. The synergy of MWR and lidar removes the bias and reduces the clear-sky LWP noise to 5 to 7 g m−2.
To investigate the formation of rain with HAMP measurements, this section extends the applicability of the LWP retrieval to drizzle and light precipitation by combining cloud radar with MWR. As described in Sect. 2.4, RWP is retrieved as the fraction by a NN using eight BTs and integrated radar reflectivity as input. Two physical effects are considered in the retrieval: hydrometeor scattering, which becomes more important with increasing droplet size and microwave frequency, and radar backscatter being sensitive to D6, where D is the droplet diameter. The first effect is considered by including the 90 GHz channel as proposed by Cadeddu et al. (2017). For the latter effect, the vertically integrated (linear) radar reflectivity is used as retrieval input in addition to the MWR channels also used in the LWP retrieval. This integrated reflectivity as a columnar quantity is more comparable to a BT and less noisy than the reflectivity of a single range gate and is thus used as retrieval input.
The Gilbert skill score (GSS) (Hogan et al., 2010), also known as equitable threat score (ETS), is used to rate how well retrieval “yes” events correspond to true yes events while accounting for hits due to chance. Yes events mark RWP above a given threshold. The GSS is defined as
using the common entries of the contingency table and the hits due to chance:
GSS ranges from to 1, with 1 being the perfect score.
The retrieval of RWP is evaluated for different RWP thresholds (Fig. 9). The GSS shows good performance, being higher than 0.75 for RWP thresholds from 10 g m−2 to about 50 g m−2 and higher than 0.5 for RWP up to 250 g m−2. Note that 762, 295, and 62 of the test cases have RWP greater than 10, 50, and 250 g m−2, respectively, and only few samples with higher RWP are available. The hit rate is higher than 80 % for thresholds between 10 and 250 g m−2, but the 250 g m−2 threshold also generates 37 % false alarms. Especially the high GSS for low RWP thresholds makes the f retrieval a useful tool combining cloud radar and MWR for detecting measurements that contain warm precipitation.
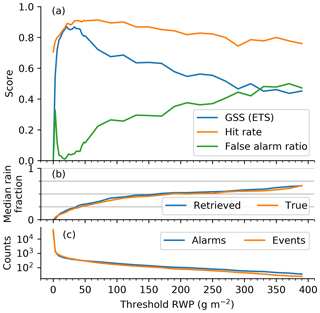
Figure 9Scores for detecting an atmospheric profile with RWP higher than the respective threshold. (a) Gilbert skill score (GSS), hit rate, and false alarm ratio. (b) Median fraction of rainwater as a function of RWP threshold. (c) Number of alarms and events for retrieved and true RWP above the threshold, respectively.
A case study of two showering shallow cumuli is shown in Fig. 10 to illustrate the capabilities of retrieving CLWP and RWP separately. The figure shows how HAMP is able to resolve spatial features of showering cells, which were observed with a cross section of several HAMP footprints. The precipitating core of both cells had maximum RWPs of probably more than 200 g m−2. The stronger relative gradients of RWP compared to CLWP indicate the narrowness of the precipitating core. Note how the higher horizontally resolved information by radar (MWR footprints 3.3∘ to 5.0∘ vs. radar footprint 0.6∘) contributes relatively stronger to the RWP retrieval than to the CLWP retrieval. The RWP retrieval consistently indicates no rain except for the time when the radar signal touches the surface or when there is a clearly visible fallstreak (17:42:30). The two showering clouds reveal maximum total LWP of more than 700 and 1000 g m−2. The second shower core likely contains more water than indicated, as the retrieval sets the clipping flag. This case study also demonstrates the higher sensitivity of the lidar and the (C)LWP retrieval, which shows cloud signals between 17:38:30 and 17:39:10 of clouds which are too thin to be detected by the radar.
The synergy of lidar, radar, and MWR is necessary to understand the difference of clouds in the dry and wet season as all instruments have their specific limitations. The lidar cloud mask indicates the more frequent occurrence of clear sky during the wet season (70.0 %) compared to the dry season (53.3 %, Table 3), even though the environment is characterized by less humid air in the dry season (Fig. 11a, b). The IWV distribution is clearly confined to moderate values with a mean of 28 kg m−2 in the dry season, which is mainly due to a rather dry middle troposphere seen in the lidar water vapor profiles. During the wet season, IWV values up to 60 kg m−2 were observed, distributed into two modes around 35 and 52 kg m−2. These two modes are clearly distinct from the single mode observed in the dry season and reveal the expected humid characteristic of the wet season. The most-humid air during NARVAL1 was sampled in a deep convective system on the southernmost leg of research flight 2 on 11 December 2013. This was the NARVAL1 flight during which HALO was closest to the intertropical convergence zone (ITCZ). The NARVAL2 IWV distribution seems to be driven by the vicinity of the flight track to deeper convective systems and the ITCZ as it can be analyzed from satellite images and thus also by the selection of flight patterns.
Interestingly, clouds show a higher mean LWP of about 63 g m−2 in the dry season compared to a mean LWP of 40 g m−2 in the wet season. Likewise, thicker clouds (LWP > 50 g m−2) were more frequent in the dry season (Fig. 11c, d); i.e., 27.1 % of the time when a cloud was seen in the dry season, it contained LWP > 50 g m−2, whereas only 18.6 % of the time in the wet season, clouds exceeded this value. The dry-season clouds tend to produce light precipitation more frequently than the wet-season clouds as indicated by the more frequent exceedance of RWP thresholds (Table 3). The cumulative distributions of RWP occurrences of all cloudy measurements with LWP > 50 g m−2 are depicted in Fig. 11e and f for each flight in the two seasons, when radar measurements are available. The vast majority (NARVAL1: 91 %; NARVAL2: 96 %) of all these measurements show RWP < 10 g m−2. Higher amounts of light rain seem to be more frequent in the dry season dataset, although the small number of heavy RWP observations inhibits a statistical sound statement as RWP > 100 g m−2 was only observed for 162 and 49 s in the radar–radiometer datasets of the dry and wet season, respectively. These time spans exclude measurements flagged as clipping (LWP > 1000 g m−2) or frozen precipitation (ice scattering). While warm precipitation seems to occur less often, clouds associated with frozen precipitation were more often observed in the wet season (1.6 % of the time) than in the dry season (0.5 %). Therefore, the lower LWP of the wet season clouds might be due to a higher precipitation efficiency compared to the dry season.
The higher LWP in the dry season might partly be explained by the choice of flight patterns. However, an analysis of ground-based LWP measurements at the Barbados Cloud Observatory (Stevens et al., 2016) over the years 2013–2018 confirms the generally higher LWP values during December than August (not shown). Thus, also changes in the organization of clouds could cause the differences in cloud fraction and LWP. The fact that the medium LWP range from 100 to 400 g m−2 is less frequent in the wet season could be due to the higher degree of organization causing more clear-sky areas and more intense clusters with higher amounts of precipitation. In that sense the latter would be missed by our flight patterns as we avoided strongly convective scenes with the formation of large ice particles.
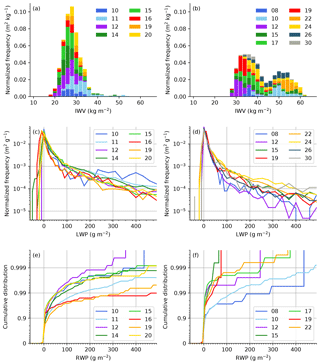
Figure 11Frequency distribution functions of IWV during the dry season (NARVAL1, a) and wet season (NARVAL2, b), LWP during NARVAL1 (c) and NARVAL2 (d), and cumulative distribution functions of RWP during NARVAL1 (e) and NARVAL2 (f). Colors denote the day of the month of the respective study. Colors in (a) and (b) denote the contribution of each flight to the total distribution. The bin edges are represented as minor ticks in (c) to (f). LWP distributions only include measurements for which the lidar cloud flag reports a cloud within ±2 s. RWP distribution is based on the non-clear-sky-corrected LWP dataset (see note a in Table 3), where LWP > 50 g m−2.
Table 3Comparing NARVAL1 and NARVAL2 cloud properties observed south of 20∘ N and with HALO altitude above 6 km. Percentages of flight time with available corresponding datasets during each study.
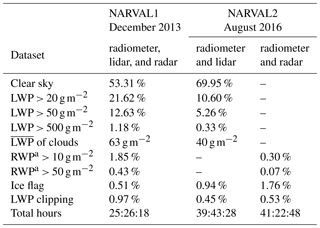
a Based on non-clear-sky-corrected LWP as radar and lidar cloud mask were only available during 5 of 10 flights during NARVAL2.
Clouds play a critical role in the development of the future climate, and especially marine low-level clouds have been identified as source of uncertainty. An important cloud macrophysical quantity is LWP. Global observations are limited by satellite resolution or accuracy, and ground-based observations over the oceans are few. To fill this observational gap, the NARVAL studies were initiated to assess North Atlantic trade wind clouds using the HALO research aircraft. We use a multichannel microwave radiometer, a cloud radar, a lidar, and a dropsonde system deployed to HALO to provide insights into clouds on the kilometer scale. For NARVAL1 (December 2013) and NARVAL2 (August 2016) a unique retrieval training and test database was developed based on ICON simulations with 1.25 km grid spacing. The database contains more than 350 000 physically consistent profiles that characterize the thermodynamic state of the atmosphere and the hydrometeor distributions during each of the two campaigns. Synthetic HAMP measurements in terms of BTs and radar reflectivity profiles in nadir view were simulated for each profile using PAMTRA. The synthetic BT measurements show bivariate relations that are consistent with those observed and therefore show reliably that the database can be used to develop retrievals and assess LWP quality.
To estimate IWV, LWP, and RWP from HAMP measurements, artificial neural networks are trained with the retrieval database. BTs of seven K-band channels and the 90 GHz channel are used for IWV and LWP; vertically integrated radar reflectivity is used in addition for RWP.
Similar to LWP, an IWV retrieval is based on the spectral BT characteristics between the same water vapor absorption and window channels. A good retrieval of either IWV or LWP is a prerequisite for the other. The IWV comparison to dropsonde measurements and the continuous along-track comparison to the water vapor lidar WALES show good agreement, with an RMSD smaller than 1.4 kg m−2 and no distinct error dependence of IWV itself. Overall, the IWV assessment shows the good practical performance of HAMP and the suitability of the ICON–PAMTRA database for developing microwave retrievals for NARVAL1 and NARVAL2.
LWP retrievals are theoretically assessed as functions of retrieved LWP and true LWP. A slight advantage of the neural network compared to a linear regression retrieval is evident, especially at the limits of the LWP range (1 to 1000 g m−2). Both approaches show relative errors greater than 100 % for a retrieved LWP < 12 g m−2, which can be regarded as detection limits. If more liquid water is contained in the column, the random error decreases to 20 % at LWP ≈ 100 g m−2 and 10 % at LWP ≈ 800 g m−2. Both retrievals show an offset error smaller than the random component for LWP < 10 g m−2, with different signs depending on whether it is analyzed as a function of true or retrieved LWP. Because of the ambiguity of the error sign, we conclude that this bias can not be accounted for with the MWR retrieval alone, and we developed a synergistic clear-sky offset correction using the WALES lidar cloud mask. The cloud mask reduces the noise of clear-sky LWP to 7.1 and 5.0 g m−2 for NARVAL1 and NARVAL2, respectively.
To allow the onset of precipitation to be investigated, a neural network retrieval is trained to estimate the fraction between RWP and LWP from a combination of integrated radar reflectivity factors and BTs. Using the test database, a Gilbert skill score above 0.75 is found for RWP thresholds between 10 and 50 g m−2, which shows good applicability for detection of rain or drizzle onset.
We used data from 36 flight hours in December 2013 (dry season, NARVAL1) and 64 flight hours in August 2016 (wet season, NARVAL2) to investigate differences between the seasons. The analysis shows that although clouds were more frequent and their LWP and RWP were higher during the flights in the dry season, more microwave scattering of ice was observed in the wet season, indicating strong precipitation events. The difference between and is clearly larger than the LWP retrieval uncertainty. As expected, the IWV histograms reveal the dry season as being drier and more uniform and the wet season as more humid. However, the IWV distributions also reveal sampling biases due to flight track choices, especially for the wet season. Therefore, the airborne measurements need to be combined with long-term ground-based and spaceborne measurements to draw statistically sound conclusions. The fine-scale airborne microwave observations such as the measurements obtained with HAMP can be used to investigate the sub-satellite-footprint inhomogeneity of LWP and rain for a better error characterization of satellite measurements. Sound conclusions on the diurnal cycle can not be drawn from the data presented here, as the spatial variability of the clouds on the observed mesoscale was higher than an expected effect of the diurnal cycle.
The synergy of active and passive microwave observations could further benefit from using an optimal estimation approach including the full radar profile and all MWR channels to improve the partition of rain and cloud droplets and frozen particles (e.g., Battaglia et al., 2016). With respect to trade wind cumuli, the products of the present study in combination with cloud boundary estimations from the radar and backscatter lidar will be used to evaluate the condensate loads of different shallow trade wind cumulus types in large eddy simulations. For example, radar and lidar both detect shallow convection or shallow outflow anvils as depicted in Fig. 10. But in addition, the lidar also allows boundary-layer-driven clouds, which have tops around 1 km and are below the radar sensitivity, to be detected.
An extension of the NARVAL observations is planned by the EUREC4A field study in early 2020 (elucidating the couplings between clouds, convection, and circulation; Bony et al., 2017), which among other objectives will investigate convective aggregation. The algorithms presented here will be applied, and together with additional measurements a better understanding of the governing processes that cause differences between the dry and wet season will be analyzed. For that, the campaign will provide additional observations of large-scale dynamics, horizontally resolved remote sensing, and in situ observations by additional aircraft in the cumulus layer. Also, more locally targeted flights, distributed over the daytime, are planned to study the diurnal cycle. Together with ship, drone, and buoy measurements, a unique dataset for a better understanding of precipitation onset will be generated.
The time series of IWV, LWP, and RWP are available in the CERA (Jacob et al., 2019a, b). The HAMP MWR, HAMP radar, and dropsonde data are published and described by Konow et al. (2018a, b, c). WALES data are made available through the DLR Institute for Atmospheric Physics in the HALO database (German Aerospace Center, 2016).
FA and SC were initiators of the DFG HAMP project. MG and MW derived the lidar cloud mask and water vapor profiles. HK provided quality-controlled HAMP and dropsonde data in a unified file format. MJ and SC conceptualized this study. MM designed the PAMTRA simulations. MJ developed the HAMP retrievals, conducted the analysis, and wrote the paper with support and input from all co-authors.
The authors declare that they have no conflict of interest.
We would like to thank Daniel Klocke for running the ICON simulations and the German Climate Computing Centre (DKRZ) for storing and supplying the data. Daniel Klocke is supported by the Hans Ertel Centre for Weather Research (HErZ) by the DWD. Sabrina Schnitt and Mareike Burba are thanked for comments improving the manuscript. We appreciate the dedication of Bjorn Stevens as the driving force behind the tropical NARVAL activities. The authors gratefully acknowledge the constructive comments by two anonymous referees who helped improve the paper.
The work has been supported by the German Research Foundation (Deutsche Forschungsgemeinschaft, DFG) within the DFG Priority Program (SPP 1294) Atmospheric and Earth System Research with the Research Aircraft HALO (High Altitude and LOng Range Research Aircraft) under grant CR111/10-11.
This paper was edited by Piet Stammes and reviewed by two anonymous referees.
Baldauf, M., Seifert, A., Förstner, J., Majewski, D., Raschendorfer, M., and Reinhardt, T.: Operational Convective-Scale Numerical Weather Prediction with the COSMO Model: Description and Sensitivities, Mon. Weather Rev., 139, 3887–3905, https://doi.org/10.1175/MWR-D-10-05013.1, 2011. a
Battaglia, A., Mroz, K., Lang, T., Tridon, F., Tanelli, S., Tian, L., and Heymsfield, G. M.: Using a multiwavelength suite of microwave instruments to investigate the microphysical structure of deep convective cores, J. Geophys. Res.-Atmos., 121, 9356–9381, https://doi.org/10.1002/2016JD025269, 2016. a
Bony, S. and Stevens, B.: Measuring area-averaged vertical motions with dropsondes, J. Atmos. Sci., 76, 767–783, https://doi.org/10.1175/JAS-D-18-0141.1, 2019. a
Bony, S., Stevens, B., Frierson, D., Jakob, C., Kageyama, M., Pincus, R., Shepherd, T., Sherwood, S., Siebesma, A., Sobel, A., Watanabe, M., and Webb, M.: Clouds, circulation and climate sensitivity, Nat. Geosci., 8, 261–268, https://doi.org/10.1038/ngeo2398, 2015. a, b
Bony, S., Stevens, B., Ament, F., Bigorre, S., Chazette, P., Crewell, S., Delanoë, J., Emanuel, K., Farrell, D., Flamant, C., Gross, S., Hirsch, L., Karstensen, J., Mayer, B., Nuijens, L., Ruppert, J. H., Sandu, I., Siebesma, P., Speich, S., Szczap, F., Totems, J., Vogel, R., Wendisch, M., and Wirth, M.: EUREC4A: A Field Campaign to Elucidate the Couplings Between Clouds, Convection and Circulation, Surv. Geophys., 38, 1529–1568, https://doi.org/10.1007/s10712-017-9428-0, 2017. a
Boucher, O., Randall, D., Artaxo, P., Bretherton, C., Feingold, G., Forster, P., Kerminen, V.-M., Kondo, Y., Liao, H., Lohmann, U., Rasch, P., Satheesh, S., Sherwood, S., Stevens, B., and Zhang, X.: Clouds and Aerosols, book section 7, 571–658, Cambridge University Press, Cambridge, United Kingdom and New York, NY, USA, https://doi.org/10.1017/CBO9781107415324.016, 2013. a
Cadeddu, M., Turner, D., and Liljegren, J.: A Neural Network for Real-Time Retrievals of PWV and LWP From Arctic Millimeter-Wave Ground-Based Observations, IEEE T. Geosci. Remote, 47, 1887–1900, https://doi.org/10.1109/tgrs.2009.2013205, 2009. a
Cadeddu, M. P., Marchand, R., Orlandi, E., Turner, D. D., and Mech, M.: Microwave Passive Ground-Based Retrievals of Cloud and Rain Liquid Water Path in Drizzling Clouds: Challenges and Possibilities, IEEE T. Geosci. Remote, 55, 6468–6481, https://doi.org/10.1109/tgrs.2017.2728699, 2017. a, b
Crewell, S. and Löhnert, U.: Accuracy of cloud liquid water path from ground-based microwave radiometry 2, Sensor accuracy and synergy, Radio Sci., 38, 71–73, 2003. a
Crewell, S., Ebell, K., Löhnert, U., and Turner, D. D.: Can liquid water profiles be retrieved from passive microwave zenith observations?, Geophys. Res. Lett., 36, L06803, https://doi.org/10.1029/2008GL036934, 2009. a
Elsaesser, G. S., O’Dell, C. W., Lebsock, M. D., Bennartz, R., Greenwald, T. J., and Wentz, F. J.: The Multisensor Advanced Climatology of Liquid Water Path (MAC-LWP), J. Climate, 30, 10193–10210, https://doi.org/10.1175/JCLI-D-16-0902.1, 2017. a, b
Ewald, F., Kölling, T., Baumgartner, A., Zinner, T., and Mayer, B.: Design and characterization of specMACS, a multipurpose hyperspectral cloud and sky imager, Atmos. Meas. Tech., 9, 2015–2042, https://doi.org/10.5194/amt-9-2015-2016, 2016. a
Fricke, C., Ehrlich, A., Jäkel, E., Bohn, B., Wirth, M., and Wendisch, M.: Influence of local surface albedo variability and ice crystal shape on passive remote sensing of thin cirrus, Atmos. Chem. Phys., 14, 1943–1958, https://doi.org/10.5194/acp-14-1943-2014, 2014. a
German Aerospace Center: HALO database, available at: https://halo-db.pa.op.dlr.de/ (last access: 14 June 2019), https://doi.org/10.17616/r39q0t, 2016. a
Gödde, F.: Detecting Clouds in the Presence of Sunglint: An Approach Using Spectral Water Vapor Absorption, Master's thesis, Ludwig-Maximilians-University Munich, available at: https://macsserver.physik.uni-muenchen.de/campaigns/documents/theses/master_thesis_felix_goedde_2018.pdf (last access: 14 June 2019), 2018. a
Greenwald, T. J., Bennartz, R., Lebsock, M., and Teixeira, J.: An Uncertainty Data Set for Passive Microwave Satellite Observations of Warm Cloud Liquid Water Path, J. Geophys. Res.-Atmos., 123, 3668–3687, https://doi.org/10.1002/2017JD027638, 2018. a, b, c, d
Hogan, R. J., Ferro, C. A. T., Jolliffe, I. T., and Stephenson, D. B.: Equitability Revisited: Why the “Equitable Threat Score” Is Not Equitable, Weather Forecast., 25, 710–726, https://doi.org/10.1175/2009WAF2222350.1, 2010. a
Jacob, M., Ament, F., Gutleben, M., Konow, H., Mech, M., Wirth, M., and Crewell, S.: Liquid water path and integrated water vapor over the tropical Atlantic during NARVAL-South, https://doi.org/10.26050/WDCC/HALO_measurements_5, 2019a. a
Jacob, M., Ament, F., Gutleben, M., Konow, H., Mech, M., Wirth, M., and Crewell, S.: Liquid water path and integrated water vapor over the tropical Atlantic during NARVAL2, https://doi.org/10.26050/WDCC/HALO_measurements_6, 2019b. a
Klepp, C., Ament, F., Bakan, S., Hirsch, L., and Stevens, B.: NARVAL Campaign Report, Reports on Earth System Science 164, Max-Planck-Institut für Meteorologie, available at: http://www.mpimet.mpg.de/fileadmin/publikationen/Reports/WEB_BzE_164_last.pdf (last access: 14 June 2019), 2014. a
Klocke, D., Brück, M., Hohenegger, C., and Stevens, B.: Rediscovery of the doldrums in storm-resolving simulations over the tropical Atlantic, Nat. Geosci., 10, 891–896, https://doi.org/10.1038/s41561-017-0005-4, 2017. a, b
Konow, H., Jacob, M., Ament, F., Crewell, S., Ewald, F., Hagen, M., Hirsch, L., Jansen, F., Mech, M., and Stevens, B.: A unified data set of airborne cloud remote sensing using the HALO Microwave Package (HAMP), Earth Syst. Sci. Data Discuss., https://doi.org/10.5194/essd-2018-116, in review, 2018a. a, b, c
Konow, H., Jacob, M., Ament, F., Crewell, S., Ewald, F., Hagen, M., Hirsch, L., Jansen, F., Mech, M., and Stevens, B.: HALO Microwave Package measurements during Next-generation Remote sensing for VALidation Studies – South (NARVAL-South), https://doi.org/10.1594/WDCC/HALO_measurements_2, 2018b. a, b
Konow, H., Jacob, M., Ament, F., Crewell, S., Ewald, F., Hagen, M., Hirsch, L., Jansen, F., Mech, M., and Stevens, B.: HALO Microwave Package measurements during Next-generation Remote sensing for VALidation Studies 2 (NARVAL2), https://doi.org/10.1594/WDCC/HALO_measurements_3, 2018c. a, b
Krautstrunk, M. and Giez, A.: The Transition From FALCON to HALO Era Airborne Atmospheric Research, in: Atmospheric Physics, 609–624, Springer Berlin Heidelberg, https://doi.org/10.1007/978-3-642-30183-4_37, 2012. a
Lebsock, M. D., L’Ecuyer, T. S., and Stephens, G. L.: Detecting the ratio of rain and cloud water in low-latitude shallow marine clouds, J. Appl. Meteorol. Climatol., 50, 419–432, https://doi.org/10.1175/2010JAMC2494.1, 2011. a
Liljegren, J. C., Boukabara, S.-A., Cady-Pereira, K., and Clough, S. A.: The effect of the half-width of the 22-GHz water vapor line on retrievals of temperature and water vapor profiles with a 12-channel microwave radiometer, IEEE T. Geosci. Remote, 43, 1102–1108, 2005. a
Liu, G., Curry, J. A., Haggerty, J. A., and Fu, Y.: Retrieval and characterization of cloud liquid water path using airborne passive microwave data during INDOEX, J. Geophys. Res.-Atmos., 106, 28719–28730, https://doi.org/10.1029/2000JD900782, 2001. a, b
Liu, Q., Weng, F., and English, S. J.: An Improved Fast Microwave Water Emissivity Model, IEEE T. Geosci. Remote, 49, 1238–1250, https://doi.org/10.1109/TGRS.2010.2064779, 2011. a
Lohmann, U. and Neubauer, D.: The importance of mixed-phase and ice clouds for climate sensitivity in the global aerosol–climate model ECHAM6-HAM2, Atmos. Chem. Phys., 18, 8807–8828, https://doi.org/10.5194/acp-18-8807-2018, 2018. a
Maahn, M., Löhnert, U., Kollias, P., Jackson, R. C., and McFarquhar, G. M.: Developing and Evaluating Ice Cloud Parameterizations for Forward Modeling of Radar Moments Using In Situ Aircraft Observations, J. Atmos. Ocean. Tech., 32, 880–903, https://doi.org/10.1175/JTECH-D-14-00112.1, 2015. a
Marshall, J. S. and Palmer, W. M. K.: The Distribution of Raindrops with Size, J. Meteor., 5, 165–166, https://doi.org/10.1175/1520-0469(1948)005<0165:TDORWS>2.0.CO;2, 1948. a
Masunaga, H., Nakajima, T. Y., Nakajima, T., Kachi, M., and Suzuki, K.: Physical properties of maritime low clouds as retrieved by combined use of Tropical Rainfall Measuring Mission (TRMM) Microwave Imager and Visible/Infrared Scanner 2. Climatology of warm clouds and rain, J. Geophys. Res.-Atmos., 107, 4367, https://doi.org/10.1029/2001JD001269, 2002. a
Mears, C. A., Wang, J., Smith, D., and Wentz, F. J.: Intercomparison of total precipitable water measurements made by satellite-borne microwave radiometers and ground-based GPS instruments, J. Geophys. Res.-Atmos., 120, 2492–2504, https://doi.org/10.1002/2014JD022694, 2015. a
Mech, M., Orlandi, E., Crewell, S., Ament, F., Hirsch, L., Hagen, M., Peters, G., and Stevens, B.: HAMP – the microwave package on the High Altitude and LOng range research aircraft (HALO), Atmos. Meas. Tech., 7, 4539–4553, https://doi.org/10.5194/amt-7-4539-2014, 2014. a
Painemal, D., Greenwald, T., Cadeddu, M., and Minnis, P.: First extended validation of satellite microwave liquid water path with ship-based observations of marine low clouds, Geophys. Res. Lett., 43, 6563–6570, 2016. a
Rosenkranz, P. W.: Water vapor microwave continuum absorption: A comparison of measurements and models, Radio Sci., 33, 919–928, https://doi.org/10.1029/98RS01182, 1998. a
Schnitt, S., Orlandi, E., Mech, M., Ehrlich, A., and Crewell, S.: Characterization of Water Vapor and Clouds During the Next-Generation Aircraft Remote Sensing for Validation (NARVAL)-South Studies, IEEE J. Sel. Top. Appl., 10, 3114–3124, https://doi.org/10.1109/JSTARS.2017.2687943, 2017. a, b, c, d
Sherwood, S. C., Bony, S., and Dufresne, J.-L.: Spread in model climate sensitivity traced to atmospheric convective mixing, Nature, 505, 37–42, https://doi.org/10.1038/nature12829, 2014. a
Stephens, G. L., Vane, D. G., Boain, R. J., Mace, G. G., Sassen, K., Wang, Z., Illingworth, A. J., O'connor, E. J., Rossow, W. B., Durden, S. L., Miller, S. D., Austin, R. T., Benedetti, A., and Mitrescu, C. a.: THE CLOUDSAT MISSION AND THE A-TRAIN, B. Am. Meteorol. Soc., 83, 1771–1790, https://doi.org/10.1175/BAMS-83-12-1771, 2002. a
Stevens, B., Farrell, D., Hirsch, L., Jansen, F., Nuijens, L., Serikov, I., Brügmann, B., Forde, M., Linne, H., Lonitz, K., and Prospero, J. M.: The Barbados Cloud Observatory: Anchoring Investigations of Clouds and Circulation on the Edge of the ITCZ, B. Am. Meteorol. Soc., 97, 787–801, https://doi.org/10.1175/BAMS-D-14-00247.1, 2016. a
Stevens, B., Brogniez, H., Kiemle, C., Lacour, J.-L., Crevoisier, C., and Kiliani, J.: Structure and Dynamical Influence of Water Vapor in the Lower Tropical Troposphere, Surv. Geophys., 38, 1371–1397, https://doi.org/10.1007/s10712-017-9420-8, 2017. a
Stevens, B., Ament, F., Bony, S., Crewell, S., Ewald, F., Gross, S., Hansen, A., Hirsch, L., Jacob, M., Kölling, T., Konow, H., Mayer, B., Wendisch, M., Wirth, M., Wolf, K., Bakan, S., Bauer-Pfundstein, M., Brueck, M., Delanoë, J., Ehrlich, A., Farrell, D., Forde, M., Gödde, F., Grob, H., Hagen, M., Jäkel, E., Jansen, F., Klepp, C., Klingebiel, M., Mech, M., Peters, G., Rapp, M., Wing, A. A., and Zinner, T.: A high-altitude long-range aircraft configured as a cloud observatory – the NARVAL expeditions, B. Am. Meteorol. Soc., in press, https://doi.org/10.1175/BAMS-D-18-0198.1, 2019. a, b, c
Turner, D. D., Vogelmann, A. M., Austin, R. T., Barnard, J. C., Cady-Pereira, K., Chiu, J. C., Clough, S. A., Flynn, C., Khaiyer, M. M., Liljegren, J., Johnson, K., Lin, B., Long, C., Marshak, A., Matrosov, S. Y., McFarlane, S. A., Miller, M., Min, Q., Minimis, P., O'Hirok, W., Wang, Z., and Wiscombe, W.: Thin Liquid Water Clouds: Their Importance and Our Challenge, B. Am. Meteorol. Soc., 88, 177–190, https://doi.org/10.1175/BAMS-88-2-177, 2007. a
Turner, D. D., Cadeddu, M. P., Löhnert, U., Crewell, S., and Vogelmann, A. M.: Modifications to the water vapor continuum in the microwave suggested by ground-based 150-GHz observations, IEEE T. Geosci. Remote, 47, 3326–3337, https://doi.org/10.1109/TGRS.2009.2022262, 2009. a
Vaisala: Vaisala RD94 Dropsonde Datasheet, Technical data, Vaisala, available at: https://www.vaisala.com/sites/default/files/documents/RD94-Datasheet-B210936EN-B.pdf (last access: 14 June 2019), 2017. a
van Meijgaard, E. and Crewell, S.: Comparison of model predicted liquid water path with ground-based measurements during CLIWA-NET, Atmos. Res., 75, 201–226, https://doi.org/10.1016/j.atmosres.2004.12.006, 2005. a
Wentz, F. J. and Meissner, T.: Algorithm Theoretical Basis Document (ATBD), Version 2, AMSR Ocean Algorithm, Tech. rep., Remote Sensing Systems, http://images.remss.com/papers/rsstech/2000_121599A-1_Wentz_AMSR_Ocean_Algorithm_ATBD_Version2.pdf (last access: 14 June 2019), 2000. a, b
Winker, D. M., Hunt, W. H., and McGill, M. J.: Initial performance assessment of CALIOP, Geophys. Res. Lett., 34, L19803, https://doi.org/10.1029/2007GL030135, 2007. a
Wirth, M., Fix, A., Mahnke, P., Schwarzer, H., Schrandt, F., and Ehret, G.: The airborne multi-wavelength water vapor differential absorption lidar WALES: system design and performance, Appl. Phys. B, 96, 201–213, https://doi.org/10.1007/s00340-009-3365-7, 2009. a
Zhou, L., Liu, Q., Liu, D., Xie, L., Qi, L., and Liu, X.: Validation of MODIS liquid water path for oceanic nonraining warm clouds: Implications on the vertical profile of cloud water content, J. Geophys. Res.-Atmos., 121, 4855–4876, 2016. a
- Abstract
- Introduction
- Material and methods
- Assessment of integrated water vapor
- Assessment of liquid water path
- Assessment of rain
- Comparison of dry and wet season
- Summary and conclusions
- Data availability
- Author contributions
- Competing interests
- Acknowledgements
- Financial support
- Review statement
- References
- Abstract
- Introduction
- Material and methods
- Assessment of integrated water vapor
- Assessment of liquid water path
- Assessment of rain
- Comparison of dry and wet season
- Summary and conclusions
- Data availability
- Author contributions
- Competing interests
- Acknowledgements
- Financial support
- Review statement
- References