the Creative Commons Attribution 4.0 License.
the Creative Commons Attribution 4.0 License.
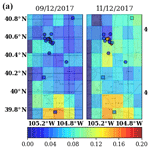
A low-cost monitor for measurement of fine particulate matter and aerosol optical depth – Part 2: Citizen-science pilot campaign in northern Colorado
Jeffrey R. Pierce
Eric Wendt
Marilee Long
Shantanu Jathar
John Mehaffy
Jessica Tryner
Casey Quinn
Lizette van Zyl
Christian L'Orange
Daniel Miller-Lionberg
John Volckens
A pilot field campaign was conducted in the fall and winter of 2017 in northern Colorado to test the deployment of the Aerosol Mass and Optical Depth (AMOD) instrument as part of the Citizen-Enabled Aerosol Measurements for Satellites (CEAMS) network. Citizen scientists were recruited to set up the device to take filter and optical measurements of aerosols in their backyards. The goal of the network is to provide more surface particulate matter and aerosol optical depth (AOD) measurements to increase the spatial and temporal resolution of ratios of fine particulate matter (PM2.5) to AOD and to improve satellite-based estimates of air quality. Participants collected 65 filters and 160 multi-wavelength AOD measurements, from which 109 successful PM2.5 : AOD ratios were calculated. We show that PM2.5, AOD, and their ratio (PM2.5 : AOD) often vary substantially over relatively short spatial scales; this spatial variation is not typically resolved by satellite- and model-based PM2.5 exposure estimates. The success of the pilot campaign suggests that citizen-science networks are a viable means for providing new insight into surface air quality. We also discuss lessons learned and AMOD design modifications, which will be used in future wider deployments of the CEAMS network.
- Article
(2348 KB) - Full-text XML
- Companion paper 1
- Companion paper 3
-
Supplement
(458 KB) - BibTeX
- EndNote
Exposure to particulate matter with diameters smaller than 2.5 µm (PM2.5) is a leading contributor to the global burden of disease (GBD; Cohen et al., 2017; Forouzanfar et al., 2015; Lim et al., 2012). In the US, 3 %–5 % of the annual deaths are attributable to PM2.5, while in many Asian countries (e.g., China and India), this value is on the order of 10 % (Cohen et al., 2017; https://vizhub.healthdata.org/gbd-compare/, last access: 6 November 2019). The GBD values are determined using population-level exposure estimates coupled with concentration-response functions from epidemiological studies. However, determining population-level PM2.5 exposure can be challenging, as there are a limited number of in situ instruments that monitor ground-level PM2.5 concentrations worldwide. Thus, other methods must be employed to estimate exposure.
The current GBD methods employ a combination of model and “satellite-based” estimates of ground-level PM2.5 concentrations (Brauer et al., 2012, 2015). While these PM2.5 concentration estimates are considered the best available, there are multiple limitations to these methods and inputs. Models can be limited by their emission inventories, meteorological input, and chemical mechanisms. Furthermore, models, particularly global models as used in the GBD, can have resolutions that are too coarse (tens to hundreds of kilometers) to resolve small-scale variability in PM2.5 concentrations, while satellite products generally have finer resolutions (1–10 km). Satellite observations can provide long-term records with near-global coverage of aerosols. However, satellite products relevant to air quality, such as aerosol optical depth (AOD), may have limited temporal and spatial resolution. Additionally, satellites view the entire atmospheric column; thus, fine particle concentrations at the Earth's surface (i.e., where exposures occur) are difficult to distinguish from particles aloft. A vast amount of research has been conducted relating satellite observations to surface-level air quality either through empirical relationships between satellite observations and surface measurements (e.g., Engel-Cox et al., 2004; Weber et al., 2010; Zhang et al., 2009) or through combining satellite observations with model output (e.g., van Donkelaar et al., 2006, 2013, 2014, 2015a, b; Liu et al., 2005, 2007). In particular, the original “satellite-based” PM2.5 estimates used satellite AOD and a model ratio of PM2.5 to AOD (often referred to as “η”) to determine surface PM2.5. The “satellite-based” estimate has since been updated to include more satellite and surface observations, regional bias corrections, and geographical data (e.g., van Donkelaar et al., 2015b). The PM2.5 concentration estimates developed from these endeavors have been used in a variety of health studies in addition to the GBD (e.g., Crouse et al., 2012; Evans et al., 2013; Fu et al., 2015; Hystad et al., 2012; Villeneuve et al., 2015).
However, each step of the process to estimate PM2.5 from satellite-based AOD and model data requires validation and/or bias correction. Satellite AOD products are often validated against ground-based observations, such as those from the Aerosol Robotic Network (AERONET; e.g., Levy et al., 2010; Remer et al., 2005). Model output is validated against several available datasets both in terms of satellite and surface measurements (e.g., Ford and Heald, 2012). Unfortunately, there are a limited number of co-located, ground-based PM2.5 and AOD measurements available for validation of η (the PM2.5 : AOD ratio). The Surface Particulate Matter Network (SPARTAN; https://www.spartan-network.org/, last access: 13 August 2019) was developed for this purpose (Snider et al., 2015), but there are currently only 17 sites in operation (3 others ran for a limited time period), limiting the ability to determine the spatial variability in η at sub-regional scales. The difficulty in designing a robust validation network of co-located AOD and PM2.5 measurements is that the standard instruments used to measure these quantities are expensive and costly to maintain both in terms of the actual instruments and staff. Thus, there is a need for more reliable, low-cost monitors that measure both PM2.5 and AOD.
This need is widely recognized; thus there has been an increase in the number of and interest in low-cost sensors that can be deployed by citizens to collect air quality data (e.g., Kumar et al., 2015; Snyder et al., 2013). Gupta et al. (2018) used data from sensors deployed by citizens and air quality agencies as part of the PurpleAir network (https://www2.purpleair.com/, last access: 6 November 2019) to examine the impact of wildfires on air quality in California. Snik et al. (2014) had participants in the Netherlands measure AOD using a mobile-phone application and an optical add-on to mobile-phone cameras. However, many of the lower-cost sensors used in these studies have moderate to high uncertainties (e.g., Gupta et al., 2018; Jerrett et al., 2017; Jiao et al., 2016; Zikova et al., 2017).
The Aerosol Mass and Optical Depth (AMOD) sampler was designed to provide high-quality surface-based PM2.5 and AOD measurements for the CEAMS (Citizen-Enabled Aerosol Measurements for Satellites) network. The AMOD measures (1) time-averaged PM2.5 concentrations (using a filter sample that can be analyzed for mass and composition), (2) AOD at four different wavelengths (using optically filtered photodiodes), and (3) continuous PM2.5 concentrations (using an optically based sensor). While more expensive than some commercially available low-cost sensors, the AMOD is a fraction of the cost (∼ USD 1000 for the instrument and ∼ USD 30 per filter for materials and analysis; Wendt et al., 2019) of traditional surface-based instruments used for regulatory monitoring of air quality and can be deployed by trained citizens to measure AOD, PM2.5, and η.
Our companion paper (Wendt et al., 2019) describes the design and validation of the AMOD. In this paper, we describe the results of a pilot study where citizen scientists were trained to deploy the AMOD at their place of residence. In addition to describing the pilot network, our goal is to demonstrate that (1) the CEAMS network measurements are representative of the regional air quality by comparing to standard surface and satellite measurements and (2) the CEAMS network has the potential to provide additional measurements that can better resolve the spatial and temporal variability in air quality than what is currently available from standard measurements. Results from the pilot network suggest that PM2.5, AOD, and η often vary on spatial scales that are finer than what is currently resolved by satellite- and model-based PM2.5 exposure estimates.
2.1 AMOD device and measurement data
A full description and validation of the AMOD sampler used for CEAMS is given in our companion paper, Wendt et al. (2019). In brief, the AMOD sampler has three main components for measuring air quality: (1) a filter-based PM2.5 measurement, (2) an AOD instrument, and (3) a real-time or continuous PM2.5 sensor (Plantower PMS5003). The AMOD also measures meteorological variables (temperature, pressure, and relative humidity) and GPS location. The sampler is powered by an internal lithium-ion battery pack that is charged before the start of each sampling event, and participants were provided with an optional solar-panel attachment to extend battery life. During our pilot study, each sampling event lasted for 48 h and 15 min (the additional 15 min period accommodates the extended AOD sampling at the 48 h mark). A diagram of measurements taken during a sampling event is given in Fig. S1 in the Supplement. The Plantower PMS5003 was not included in the first generation of instruments; thus, Plantower PMS5003 results are only available for measurements later in the pilot campaign period.
The design of the filter-based PM2.5 measurement in the AMOD is similar to that of the ultrasonic personal aerosol sampler (UPAS) described by Volckens et al. (2017). Ambient air is drawn into the AMOD at 2 L min−1 (using a mass flow sensor and calculating instantaneous changes in air density) by an internal piezoelectric micropump, and PM2.5 mass is collected on a 37 mm polytetrafluoroethylene (PTFE) filter after passing through a size-selective cyclone inlet. Previous laboratory and field comparisons between PM2.5 mass concentrations measured using the UPAS and an EPA Federal Equivalent Method (FEM) instrument found good agreement (R2 values from 0.87 to 0.96) across a broad range of concentrations (5 to 1000 µg m−3; Kelleher et al., 2018; Volckens et al., 2017). Additional validation of the filter-based PM2.5 measurement in the AMOD sampler against an in situ FEM instrument was done concurrently with our pilot study (R2=0.86), as described in our companion paper (Wendt et al., 2019). The average mass added to the filters that were used for sampling was 42±19 µg. The average difference in pre- and postweight mass for blank filters was 5±4 µg. Filter mass had to be greater than the corresponding blank mass and greater than 10 µg to be included in our analysis. Average PM2.5 concentrations were calculated using the added mass and the amount of sampled air.
In our pilot campaign, participants were given filters stored in barcoded sampling cassettes to minimize contamination during handling. In addition to filters for sampling, each participant was given an additional cassette that contained a filter blank for quality control. Filters were weighed to the nearest microgram (MX5 or XS3DU, Mettler Toledo) for total mass before and after sampling. Filters were also analyzed using the Magee Scientific SootScan instrument, which estimates the black carbon (BC) content of filter-bound aerosol via optical transmission (e.g., Ahmed et al., 2009; Kirchstetter and Novakov, 2007). Transmission data were converted to mass concentrations based on published mass-absorption values for combustion aerosol (Chylek et al., 1981). Elemental composition analysis was done using X-ray fluorescence (XRF) spectroscopy (Kellogg and Winberry, 1999); this analysis can give values for magnesium, aluminum, silicon, sulfur, potassium, calcium, titanium, chromium, iron, nickel, copper, zinc, gallium, arsenic, selenium, cadmium, indium, tin, tellurium, iodine, and lead. Only a subset of filters (those collected later in the campaign) were analyzed for composition. These filter-based PM2.5 mass and composition measurements were used to determine average concentrations for each sampling event.
The AOD instrument has four optically filtered photodiodes at 440, 520, 680, and 870 nm. The spectral bandpass of each photodiode is less than 15 nm. AOD measurements are direct Sun measurements that require participants to align the instrument (using a pinhole aperture and target) at the start of sampling. The AOD measurements were calibrated with AERONET measurements at one AERONET site and then validated at a different AERONET site (Wendt et al., 2019). The mean relative error between AMOD AOD and AERONET AOD was less than 0.0079 (∼10 %) across all wavelengths (Wendt et al., 2019). AOD measurements were taken every 24 h during the sampling period (so as to not require participants to realign every day), yielding three possible AOD values during the sampling event. The first AOD measurement is single and instantaneous; on subsequent days, the AMOD takes AOD measurements at 30 s intervals over a 30 min window centered around the 24 and 48 h marks. The 30 min measurement window facilitates compensation for daily Sun-position deviations and provides operators a chance to correct for mechanical misalignment (due to movement of the device or human error).
The four wavelengths were chosen so that AMOD AOD values could be compared directly with AOD values from AERONET and from satellite products. By having multiple wavelengths, we can also calculate the spectral dependence of the AOD, which can provide insight into the size and source of the aerosols (e.g., Eck et al., 1999). In particular, we calculated the Ångström exponent using the 440 and 870 nm wavelengths (as is commonly done). However, there can also be variability in the Ångström exponent based on the pair of wavelengths chosen. Thus, multiple sets are often used to calculate the curvature (or 2nd derivative) of the log of the AOD and the log of the wavelength (e.g., Eck et al., 1999; Kaskaoutis and Kambezidis, 2008). We do not present those results in this paper, but we plan to explore this further in future campaigns when we have a larger dataset. Additionally, we used the Ångström exponent for filtering our data.
For the pilot campaign, some minimal quality checks on the AOD values were conducted to screen out measurements that were impacted by clouds or misalignment of the instrument. These checks were implemented manually after sampling was completed. The requirements to be considered a successful AOD were as follows: (1) AOD had to be greater than zero, (2) AOD had to be less than 1 (which under heavy smoke or dust might be incorrect), (3) the Ångström exponent had to be greater than 0 (which under heavy dust might be incorrect), (4) measurements taken during the 30 min measurement window had to pass the triplet test (for three measurements taken in a 1 min period, the maximum and minimum AOD difference had to be less than 0.02 at all wavelengths, following Holben et al., 1998; Smirnov et al., 2000), and (5) the air mass factor had to be less than 5 (Smirnov et al., 2000). The data collected from the pilot campaign and ongoing testing will be used to improve and automate the AOD quality-control process in the future.
The continuous PM2.5 sensor (Plantower PMS5003) estimates the PM2.5 mass concentration based on the amount of 650 nm laser light scattered at a 90∘ angle. The resolution of the Plantower PMS5003 is 1 µg m−3, and it is stated by the manufacturers that it is effective for measuring concentrations in the range of 0–500 µg m−3, with an accuracy of ±10–15 µg m−3 (Yong, 2016). Our use of the PMS5003 estimates the wet PM2.5 mass in equilibrium with the ambient relative humidity. Because PM2.5 mass in equilibrium with 35 % relative humidity is used as a standard for the US EPA measurements (commonly used in health studies), we corrected continuous PM2.5 measurements using time-averaged PM2.5 concentration measured with the concurrent filter sample. Our correction of the PMS5003 PM2.5 using the filter PM2.5 also potentially provides correction for PMS5003 errors due to variability in the aerosol size and optical properties. This correction was done by multiplying the continuous PM2.5 measurements by the ratio of the average concentration determined from the filter mass to the average concentration reported by the Plantower PMS5003 sensor over the full filter sampling period; hence, variability in the water uptake, size distribution, and optical properties on timescales shorter than the 48 h filter sampling period may not be wholly resolved by this correction. For Plantower PMS5003 validation, we co-located an AMOD sampler with an FEM monitor at the Colorado State University main campus in Fort Collins, Colorado (Wendt et al., 2019). In general, for non-filter-corrected Plantower PMS5003, we found good agreement with a slightly low bias (∼1 µg m−3) at lower concentrations (<10 µg m−3) and a slightly high bias (∼2–3 µg m−3) at higher concentrations (10–20 µg m−3; Wendt et al., 2019). These results are similar to those reported by Kelly et al. (2017), Gupta et al. (2018), and Bulot et al. (2019) during laboratory and field evaluation of Plantower sensors. Laboratory testing (using set aerosol concentrations) by the South Coast Air Quality Management District (SCAQMD) suggests a precision of 95 %–100 % with regards to temperature, relative humidity, and mass loading comparisons, with slightly lower precision occurring when temperature, relative humidity, and mass loading were all low (SCAQMD, 2017b). When compared to a GRIMM FEM monitor in the lab, the sensors were well-correlated (R2 > 0.99) and had moderate to good accuracy (54 %–96 %) over the range of 0–250 µg m−3 (SCAQMD, 2017b). In field tests, the Plantower PMS5003 sensors were co-located with a GRIMM and a beta attenuation monitor (BAM), and concentrations were well-correlated for both (R2 > 0.93 and R2 > 0.86, respectively; SCAQMD, 2017a). However, these SCAQMD field tests were limited in duration, and there is some concern that the devices will degrade over time. There are few long-term validation studies, but Sayahi et al. (2019) did find lower correlation between hourly Plantower sensors compared to tapered element oscillating microbalance (TEOM; R2=0.87) and the 24 h average to the Federal Reference Method (FRM) monitors (R2=0.88) for Plantower sensors deployed for 320 d. They also noted that there was some seasonal variability in the agreement, and the bias was not consistent between instruments over time. Bulot et al. (2019) also deployed Plantower PMS5003 sensors for almost a year and found good correlations with a reference instrument (R2=0.72–0.77). They also show that the performance is only minimally impacted by temperature and relative humidity and that high mass loading leads to periods of poor performance. Continuous PM2.5 measurements and meteorological data measured by the AMOD were recorded every 30 s. For the results section, we average the filter-corrected continuous PM2.5 measurements to hourly increments.
The AMOD was mounted on a stationary camera tripod, and sampling and data collection were controlled by participants using the CEAMS app on a smartphone through a Bluetooth connection with the device, as described in Wendt et al. (2019). After each sampling event, participants used the smartphone application to download data from the instrument to their phone and then send the data to our server using file transfer protocol (FTP).
We also calculated PM2.5 : AOD ratios (η). Normally, η is calculated using the instantaneous AOD from the satellite (or a model AOD for the satellite overpass time) and the 24 h average PM2.5 concentration (e.g., van Donkelaar et al., 2013). Here, we calculate the η for each of the 3 d in the sampling period using the instantaneous AOD and the 48 h average PM2.5 filter concentration. In future measurements, we plan to analyze the sub-daily variability in η by using the PM2.5 concentrations from the real-time Plantower PMS5003. However, only a limited number of devices included the Plantower PMS5003 in the pilot network.
2.2 Citizen-science participants and pilot measurements
Participants were recruited through a partnership with the Community Collaborative Rain, Hail, and Snow (CoCoRaHS) network and through Colorado State University (graduate students and staff). CoCoRaHS is a citizen-science network focusing on precipitation measurement collections (Cifelli et al., 2005). Recruitment emails with an initial survey were sent to CoCoRaHS participants in Larimer and Weld counties in northern Colorado to gauge interest in air quality monitoring, general demographics, and participant motivation. A second survey was sent to participants who expressed interest in being contacted for sampling. The second survey provided information on the actual sampling process and the requirements for participation. This survey was used to determine which participants to invite to a training session, with selection based on availability for sampling, availability for training, and location.
Measurements for the pilot network were taken between October and December 2017. Each participant deployed the AMOD for at least one 48 h sampling event (Fig. S1). A map of sampling locations is shown in Fig. 1. Participants were allowed to initiate sampling on their own schedule; however, there were two 48 h periods (1–3 and 9–11 December 2017) when select participants were asked to sample concurrently to better determine the spatial and temporal variability in air quality across the Fort Collins metropolitan area during these distinct time periods.
2.3 Datasets used for comparisons
To contextualize our citizen-science measurement data, we compared our results from the CEAMS pilot study to routine air quality datasets collected in northern Colorado. The AMOD PM2.5 measurements were compared to measurements taken by a US EPA Air Quality System (EPA-AQS; https://www.epa.gov/aqs, last access: 11 September 2019; monitoring site 08-069-0009) FEM light-scattering PM2.5 monitor (EDM 180, GRIMM, Ainring, Germany) located on the Colorado State University campus in Fort Collins, Colorado. The AMOD AOD measurements were compared to ground-based and satellite-based AOD. Note that here we are comparing our measurements to nearby monitors; the validation of the AMOD using co-located measurements is detailed in Part 1 (Wendt et al., 2019).
For ground-based AOD, we compared the AMOD results to AOD from AERONET sites, a network of Sun photometers which has been in operation for more than 25 years (Holben et al., 1998). Specific sites used for comparison are listed in Supplement Table S1; each site had to have at least one valid measurement during the duration of the pilot network campaign (data available here: https://aeronet.gsfc.nasa.gov/, last access: 30 September 2019). The Cimel Electronique Sun and sky radiometers in the network measure direct Sun, aureole, and sky radiance at multiple wavelengths in the range of 300–1020 nm (with 440, 670, 870, 940, and 1020 nm being standard). The AOD derivation from these stationary, ground-based instruments requires minimal assumptions, and the instruments are well calibrated; thus, the data have been found to be highly accurate. AERONET AOD is considered to be ground truth for the validation of satellite and other remotely sensed products. Holben et al. (1998) estimate that the total uncertainty in (cloud-free) AOD retrieved from a newly calibrated instrument is less than ±0.01 for wavelengths greater than 440 nm (< ±0.02 for shorter wavelengths).
The most widely used satellite AOD products come from the Moderate Resolution Imaging Spectroradiometer (MODIS) instruments aboard the Terra and Aqua satellite platforms, which measure scattered radiances at 36 wavelengths (King et al., 1999). Different algorithms have been developed for AOD retrievals over ocean and land to account for the contribution of surface reflectance (e.g., Kaufman et al., 1997; Tanré et al., 1997). The standard AOD products are at 550 nm (Collection 6.1, Level 2 AOD data available here for Aqua: https://ladsweb.modaps.eosdis.nasa.gov/archive/allData/61/MYD04_L2/, last access: 9 October 2019; data are available here for Terra: https://ladsweb.modaps.eosdis.nasa.gov/archive/allData/61/MOD04_L2/, last access: 9 October 2019). Numerous studies have evaluated MODIS AOD through comparisons with ground-based AERONET sites. Expected uncertainties in the MODIS-derived AOD over land are ±( AOD) (Levy et al., 2010). When comparing MODIS AOD to the CEAMS AOD, we use MODIS AOD values within a 15 km radius and a 2 h window centered around the CEAMS measurement. Because AERONET measurements are more frequent, we average the AERONET AOD within a 2 h window around the time of the MODIS AOD value closest (less than 15 km) to the AERONET site on a given day.
3.1 PM2.5 filter measurements
During the pilot campaign, 65 filters were collected by participants. Not all filter results are shown because some of the filters had low or unrealistic weights due to issues with a device inlet, a device falling over, and a microbalance used for weighing the filters; our analysis includes results from 51 filters. A distribution of the 48 h average PM2.5 mass concentrations measured using these filter samples is shown in Fig. 2. To note, in the figure, we are comparing the CEAMS 48 h average PM2.5 concentrations to 24 h averages from the EPA-AQS site in Fort Collins. This is not an exact comparison, as the CEAMS measurements have overlapping days and different sampling periods (different start and end times). However, our goal is not to validate our measurements (for validation, see Part 1 by Wendt et al., 2019) but to demonstrate that our measurements are able to capture a similar view of air quality to a traditional reference monitor. Concentrations were relatively low, and only a few filters measured concentrations above 12 µg m−3. This distribution is as expected in wintertime in Colorado, as shown by the comparison to the distribution of daily average concentrations in Fort Collins for the same time period. Higher PM2.5 concentrations in the wintertime in northern Colorado are often associated with lower boundary-layer heights and stagnant air masses. Additionally, higher concentrations measured by CEAMS participants were generally located near city centers (discussed later; Figs. 3 and 4).
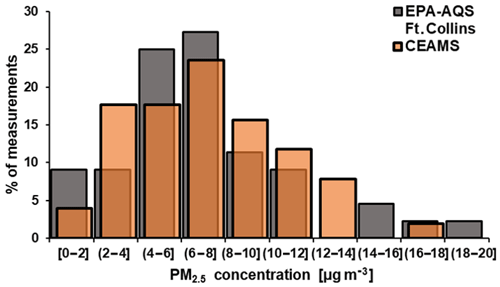
Figure 2Distribution of 48 h average filter mass concentrations collected by CEAMS participants during the pilot campaign in northern Colorado in fall and winter 2017, and 24 h average PM2.5 concentrations measured at the EPA-AQS site in Fort Collins, Colorado, for 28 October–15 December 2017.
An example of 48 h average filter concentrations measured by CEAMS participants and AQS monitor 24 h average PM2.5 concentrations measured on 13 November 2017 is given in Fig. 3. During this sample period, the highest concentrations were recorded by the AQS monitors in Greeley and Longmont, while the lowest concentrations were measured by CEAMS participants along the foothills northwest of Loveland (Fig. 3).
In Fig. 4, we show the 48 h average PM2.5 mass concentrations, the BC concentrations, and the percentages of PM2.5 that are BC for two different sampling periods in Fort Collins in December 2017. There are notable differences between the results from the two sampling periods. The first sampling period (1–3 December 2017) generally saw higher concentrations in total PM2.5. Black carbon concentrations on the western edge of the city were higher during the first period compared to the second (potassium mass – which can be a marker for soil dust or biomass burning – e.g., Kreidenweis et al., 2001 – was also slightly higher Fig. S2). Meteorological differences between the two sampling periods could explain some of these differences. While temperatures were similar, conditions were slightly warmer, sunnier, drier, and windier during the second sampling period. Additionally, winds during the first sampling period were more variable, while winds during the second sampling period mainly came from the south and southeast, where there are more sources (Fig. S3). For both sampling periods, the highest BC concentrations were measured in high-traffic areas (e.g., the city center) and are likely associated with vehicular combustion sources; however, BC concentrations in the city center were slightly lower during the first sampling period compared to the second sampling period. It is important to note that the two sampling periods do not cover the same weekday period: the first sampling period was from Friday through Sunday, and the second was from Saturday through Monday. Thus, differences in weekday and weekend traffic patterns could also impact the concentrations. This is corroborated by comparing the distribution of daily average concentrations by weekday for monitoring sites in northern Colorado. At these sites, concentrations are generally highest on Fridays and lower on weekends (Fig. S4).
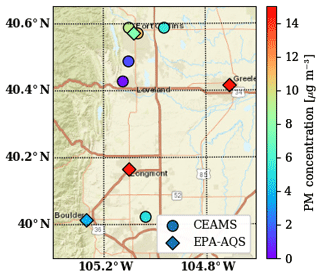
Figure 3Average PM2.5 concentration measured by CEAMS participants (48 h average) and at AQS monitoring sites (24 h average) in northern Colorado for 13 November 2017. Map generated using World Street Maps (credit: Esri, HERE, Garmin, USGS, Intermap, INCREMENT P, NRCan, Esri Japan, METI, Esri China – Hong Kong, NOSTRA, ©OpenStreetMap contributors, and the GIS user community). ©OpenStreetMap contributors 2019. Distributed under a Creative Commons BY-SA License.
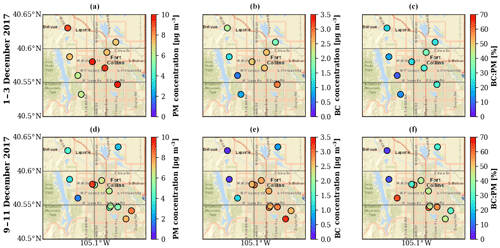
Figure 4Map of the 48 h average PM2.5 concentrations, black carbon concentrations, and the percentage of PM2.5 that is black carbon measured in Fort Collins for 1–3 December (a, b, c) and 9–11 December 2017 (d, e, f). Maps generated using World Street Maps (credit: Esri, HERE, Garmin, USGS, Intermap, INCREMENT P, NRCan, Esri Japan, METI, Esri China – Hong Kong, NOSTRA, ©OpenStreetMap contributors, and the GIS user community). ©OpenStreetMap contributors 2019. Distributed under a Creative Commons BY-SA License.
We had several citizen scientists participate in both December sampling periods; thus, we have measurements collected for both sampling periods at some sites. One site in central Fort Collins saw similar concentrations between the two sampling periods, while the northwestern site (in Laporte, Colorado) measured PM2.5 and BC concentrations that were twice as high during the first sampling period compared to the second. These differences could be due to the aforementioned differences in meteorology; however, the participant in Laporte, Colorado, also noted a nearby idling vehicle overnight with high exhaust emissions during the first sampling period (this will be further noted in Sect. 3.3).
The highest 48 h PM2.5 concentration (13 µg m−3) from the two sampling periods was measured by a participant in Milliken, Colorado, during the second sampling period (not shown in Fig. 4 due to this location being ∼25 miles away from other participants and outside the map area). The metal analysis of this filter found high concentrations of silicon and aluminum, potentially suggesting that the nearby (< 0.5 miles) concrete operation impacted air quality in the participant's residential neighborhood. Across all participants, non-carbonaceous elemental mass accounted for 10 %–40 % of the blank-corrected PM2.5 concentration. The elements with the highest concentrations were aluminum, iron, silicon, potassium, and calcium, which suggest soil or dust sources (Fig. S5). Potassium can also be a marker for biomass burning, particularly when the ratio of potassium to iron is higher (e.g., Calloway et al., 1989; Kreidenweis et al., 2001; Fig. S2).
3.2 AOD measurements
Over 160 AOD measurements were taken during the pilot campaign. These measurements were taken once per day during each 48 h sampling event. Not every sampling event yielded three useable AOD values due to the following reasons: some of the devices' batteries died before finishing the sampling event, some of the devices fell over due to wind, and some devices were misaligned. Out of the 160 AOD measurements, more AOD values were filtered out by our quality check procedure (to remove clouds and measurements taken with a high air mass factor, for example; details in the Methods section). However, there could still be some erroneous values due to a slight misalignment or the presence of thin clouds. After removing measurements that did not meet our criteria, we ended up with 115 AOD values for our analysis; as we used the same filtering criteria in Wendt et al. (2019), we would assume the same quality as that quantified in those validation experiments.
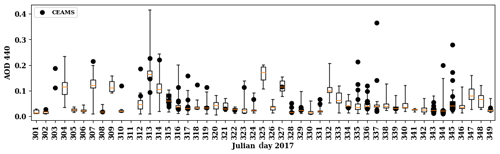
Figure 5Box-and-whisker plots showing the distribution of AERONET AOD at 440 nm (870 nm comparison Fig. S3) at all sites in northern Colorado (listed in Table S1; not all sites have values for every day) for each day from 28 October–15 December 2017. Box denotes 1st and 3rd quartile, whiskers denote maximum and minimum, and orange line shows median. CEAMS AOD values at 440 nm are overlaid as black dots.
In Fig. 5, we compare the CEAMS AOD measurements to AERONET AOD measurements taken in northern Colorado during the same period (AERONET sites are shown in Fig. 1) for the 440 nm wavelength (chosen because it is an exact wavelength match; 870 nm wavelength comparison shown in Fig. S6). In general, our AOD results fall within the range of AOD values measured at the AERONET sites on the same day (distribution shows all AOD measured throughout the day at all AERONET sites in northern Colorado), although there are several days when the CEAMS AOD values are higher. The discrepancies could be due to several factors. AERONET uses a more rigorous quality check than our measurements, and some cloud-contaminated AMOD measurements may have gone unfiltered (especially if there were thin clouds present). Additionally, most of the AERONET sites are located south of our sites (Fig. 1) and take measurements multiple times a day, so the CEAMS measurements and the AERONET measurements may not be representative of the same air masses. This is likely on at least a few of the days, as we also note a large spread among values from the different AERONET sites. Additionally, co-location of the AMOD with AERONET instruments showed very good agreement (Wendt et al., 2019). Thus, our results here show that (1) CEAMS measurements show similar magnitude and variability in AOD compared to AERONET sites and (2) there is spatial and temporal variability in AOD captured by the CEAMS measurements that cannot be represented by the spatial coverage of AERONET sites.
We also compared our CEAMS AOD (520 nm) and AERONET AOD (500 nm) to satellite-based AOD (MODIS 550 nm) in the region (Fig. 6; ignoring the difference between the wavelengths). For this, we used both the MODIS Aqua and Terra 10 km AOD products. We matched the location of each measurement to the nearest satellite grid center point (distance had to be less than 15 km) for the same day (we used a 2 h window around the observations). In general, MODIS seems to overpredict compared to AERONET and CEAMS AOD. Some of these differences might be due to the timing or location of the measurements (we used a 2 h window and 15 km radius centered around the CEAMS measurements), measurement bias from the CEAMS instrument (though CEAMS AOD was shown to be within 10 % of AERONET AOD in Wendt et al., 2019, and CEAMS and AERONET both similarly show lower AOD than MODIS) or MODIS, or the presence of clouds. However, we would also not necessarily expect to see good agreement between the satellite-based and ground-based observations. Agreement between MODIS and AERONET tends to be lower in the western US compared with the eastern US (e.g., Ford and Heald, 2016; Li et al., 2015; Sayer et al., 2013), which is partially due to issues with the surface reflectance (most of the AERONET sites used here are in the foothills of the Rocky Mountains) and/or a larger presence of coarse-mode aerosols. Furthermore, we would expect there to be variability in AOD over northern Colorado at a resolution not captured by a 10 km satellite product due to local sources.
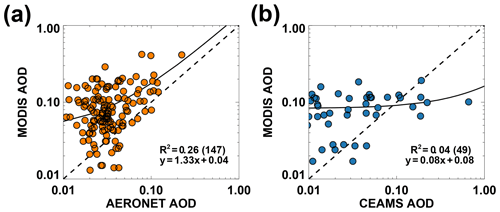
Figure 6Satellite AOD (MODIS at 550 nm) compared to ground-based AOD in northern Colorado measured at (a) AERONET sites (500 nm, specific sites used listed in Table S1; n=94) and (b) CEAMS participant sites (520 nm; n=49) for 28 October–15 December 2017. Dashed lines are 1-to-1 lines, and the solid lines are linear fits (equations provided in figure).
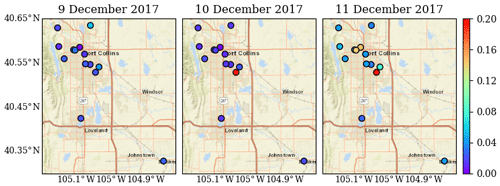
Figure 7Map of the 440 nm AOD measurements for 9–11 December 2017. Maps generated using World Street Maps (credit: Esri, HERE, Garmin, USGS, Intermap, INCREMENT P, NRCan, Esri Japan, METI, Esri China – Hong Kong, NOSTRA, ©OpenStreetMap contributors, and the GIS user community). ©OpenStreetMap contributors 2019. Distributed under a Creative Commons BY-SA License.
AOD values measured by CEAMS participants during the 9–11 December sampling days are shown in Fig. 7. AOD values were generally very low on these 3 d; however, most of the AOD measurements during the 9–11 December sampling days were taken in the morning (between 09:00 and 13:00 LT), when PM2.5 concentrations were generally at their lowest value (see Fig. 8). AOD values were higher on the third day, which was a Monday. The first 2 d were weekend days, so these results could indicate a difference in weekday versus weekend pollution patterns. The sites with the highest AOD values correspond to the sites with the highest PM2.5 concentrations (Fig. 4). However, the AOD values at these few sites are larger than those measured at the AERONET sites (Fig. 5). Additionally, these sites had low Ångström exponent values (calculated from the 870 and 440 nm wavelengths; Fig. 8). As the Ångström exponent is inversely proportional to the size of the particles, this could suggest either (1) larger particles like dust or (2) thin cloud contamination in the AOD measurement. Unfortunately, with only one AOD measurement per day per site, it is difficult to attribute the source of the high AOD values; future sampling will include multiple measurements per day per site, enabled by solar tracking functionality in the second-generation AMOD.
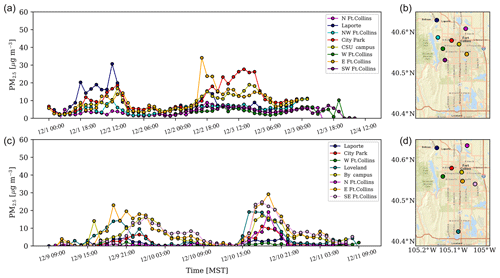
Figure 8Time series of filter-corrected hourly averaged PM2.5 concentrations from the Plantower PMS5003 sensor inside the AMOD for 1–3 December (a, b) and 9–11 December (c, d) sampling periods. Maps show sampling locations for each sampling period (uncorrected time series shown in Fig. S8). Maps generated using World Street Maps (credit: Esri, HERE, Garmin, USGS, Intermap, INCREMENT P, NRCan, Esri Japan, METI, Esri China – Hong Kong, NOSTRA, ©OpenStreetMap contributors, and the GIS user community). ©OpenStreetMap contributors 2019. Distributed under a Creative Commons BY-SA License.
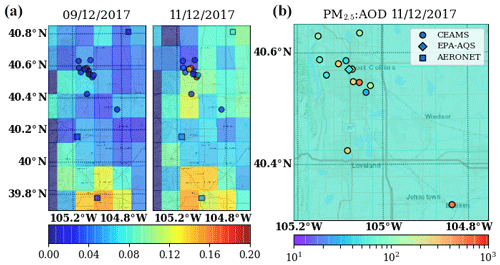
Figure 9MODIS AOD overlaid with CEAMS (circles) and AERONET (boxes) AOD for 9 and 11 December 2017 in northern Colorado (a) and PM2.5 : AOD ratio (described in text) from CEAMS for 11 December 2017 (b, note different spatial scale). In the right panel, the background color represents the value that would be assumed for the whole region if relying only on the single EPA-AQS monitoring site located at the CSU Facilities. Maps generated using World Street Maps (credit: Esri, HERE, Garmin, USGS, Intermap, INCREMENT P, NRCan, Esri Japan, METI, Esri China – Hong Kong, NOSTRA, ©OpenStreetMap contributors, and the GIS user community). ©OpenStreetMap contributors 2019. Distributed under a Creative Commons BY-SA License.
3.3 Real-time PM2.5 measurements
Plantower PMS5003 sensors were only included in some of the AMOD first-generation devices (they will be included in all future devices); thus, we have fewer results compared to the AOD and filter measurements, but the results do provide additional information. In particular, real-time PM2.5 measurements provide insight into the diurnal variability in air quality. For Fig. 8, we present hourly averages of the filter-corrected PM2.5 concentrations measured by the Plantower PMS5003 sensors during the 1–3 and 9–11 December sampling periods. For both sampling periods, measurements showed peak concentration overnight at several sites. Northern Colorado winter nights are often marked by cold temperatures, low boundary-layer heights, and weak winds, which can lead to the buildup of pollutants. Additionally, many homes still rely on wood burning for heating (potassium mass on the filters was only slightly higher during the first sampling period, which was slightly colder; Fig. S2). These increased emissions at night, coupled with the low boundary-layer heights, could be the cause of the overnight peak concentrations. This hypothesis is corroborated by the spatial distribution of PM2.5 concentrations, as the locations with the largest overnight peaks are located in regions with older homes (e.g., the Fort Collins City Park and CSU campus sites – Fig. 8 – in Old Town Fort Collins). The Laporte, Colorado, site also saw high overnight concentrations on 1–2 December; while there is also a lot of residential wood burning in Laporte (this site had higher potassium concentrations and higher potassium-to-iron ratios; Fig. S2), the participant also noted an idling vehicle with significant emissions at a neighbor's home in the middle of the night on 1–2 December.
3.4 PM2.5 : AOD ratio
A major goal of this network is to better estimate η, the PM2.5 : AOD ratio, in order to improve the methods for using satellite observations to determine surface air quality in regions and time periods without extensive surface monitoring. From our measurements, we calculated PM2.5 : AOD ratios for each of the 3 d in the sampling period using the instantaneous AOD and the 48 h average PM2.5 filter concentration. Only 109 ratios were calculated from the 65 filters and 160 AOD values due to (1) not including filters from the device with the inlet or microbalance issue and (2) not using AOD values that did not pass our quality checks listed in the Methods section.
An example of the calculated ratio is shown in Fig. 9, which also shows the AOD from MODIS (gridded average of Aqua and Terra) and from the CEAMS measurements (using the 520 nm wavelength) for 2 d. In this figure, the highest ratios are nearer to the Fort Collins city center, while sites farther out have generally lower ratios. High PM2.5 : AOD ratios can indicate more particles near the surface, which often happens near emission sources. A low PM2.5 : AOD ratio can indicate (1) particles being mixed through more of the atmospheric column (or lofted above the surface), (2) the presence of larger particles, or (3) thin cloud contamination in the AOD. Thus, the variability may be due to distance from the aerosol sources, variability in the aerosol size distribution (which is supported by the variability in Ångström exponents calculated from the 870 and 440 nm wavelengths measured at the different sites; Fig. S7), or differing diurnal variability in PM2.5 concentrations compared to the timing of the AOD measurement.
This difference in measurement duration is likely important. Our PM2.5 values are an integrated 48 h measurement, while the AOD is an instantaneous measurement. In studies that use the satellite AOD to calculate η, this is generally done using an instantaneous AOD value (for some daylight time that varies based on location and overpass) and the 24 h average PM2.5 concentration (e.g., van Donkelaar et al., 2013, 2016; Ford and Heald et al., 2016). This is a methodological decision, as the desire is to use an instantaneous satellite AOD value to determine a 24 h average PM2.5 concentration in a region with no surface-based measurement. Thus, while the PM2.5 : AOD ratio is often strongly related to the vertical distribution of aerosols in the atmospheric column (e.g., van Donkelaar et al., 2010; Ford and Heald, 2016), because of the time element, the determined η values are not necessarily indicative of the time-averaged vertical distribution of aerosols for a region. Therefore, because our measurements are not taken at a set time, we can expect some variability in the ratios just based on the timing of the AOD measurements. To explore this further, we also calculated “instantaneous” η values from our measurements. If we use the Plantower PMS5003 PM2.5 data (hourly average; not available for all samples) to calculate η rather than the filter, we find that using the 48 h average concentration rather than the hourly average concentration often overpredicts η due to higher PM2.5 concentrations at night compared to the concentrations at the time of the daytime AOD measurement (Fig. S9). In other seasons or locations with a different diurnal pattern, we might find the opposite. We plan to further explore this temporal variability in future studies by taking multiple measurements of AOD throughout the day (there will still be some bias, as we can only measure AOD during daylight hours).
In Fig. 9, we also compared the PM2.5 : AOD ratio from the CEAMS sites (again, calculated from the daily AOD and the 48 h average filter concentration) to what would be the assumed η if we just used the daily average PM2.5 from one long-term surface monitoring site (CSU Facilities) with the MODIS AOD at that location. Our CEAMS measurements show high variability in η (orders of magnitude) over a relatively short distance. While some of this variability may be due to measurement error (or the timing of the measurement as discussed previously), the number of measurements with a range of values gives confidence that there should be more variability than what can be determined from the single PM2.5 measurement site and satellite AOD. We also looked at the distribution of ratios collected from our CEAMS measurements compared to the distribution of ratios using MODIS AOD and the PM2.5 concentrations from the EPA-AQS site (Fig. S10). We have a greater fraction of higher η values. This could suggest that our AOD values are biased low, or our PM2.5 values are biased high, or it could suggest that our CEAMS locations are located nearer to sources than the EPA-AQS monitor. Studies have shown that MODIS AOD is often biased quite high in the western US (mainly due to issues representing the surface reflectance; e.g., Ford and Heald, 2016; Li et al., 2015; Sayer et al., 2013), which we also see in the comparison to AERONET in Fig. 6. Thus, the EPA-AQS / MODIS ratios are likely biased low. Additionally, the EPA-AQS monitor is on the roof of the facilities building on the Colorado State University campus (primarily a walking campus); thus, it is slightly removed from some sources due to the height and location (for example, road dust, residential wood burning, etc.) and may show slightly lower concentrations. These discrepancies again suggest that η calculated from a single PM2.5 measurement site and satellite AOD may not correctly capture the regional variability in η. Thus, more co-located AOD and PM2.5 measurements are necessary.
Validation of the AMOD device measurements showed good agreement with standard validation measurements (Wendt et al., 2019). However, not all of our CEAMS measurements taken by participants were successful. Several participants failed to align the device correctly because it required them to find a small sunspot on the device and then tighten the camera tripod to lock the AMOD into place. In addition, AOD measurements were negatively affected by cloud contamination, high winds that shifted the alignment of the device (or toppled it completely), and the fact that the device only took a single (valid) AOD measurement per day. Overall, we obtained a limited number of useful AOD measurements from the pilot campaign (115 out of a predicted 204). In the conclusions section, we discuss improvements to the AMOD device that are being implemented to address these issues prior to expanding the network for future sampling campaigns.
In regard to participation, we found that CoCoRaHS participants were highly motivated and responsive. Participants recruited from elsewhere were less likely to finish taking their measurements and required more prompting and reminders to set up their devices. Thus, as we anticipated, partnering with the already-established CoCoRaHS citizen-science network was beneficial in finding reliable and engaged participants. We plan to continue this partnership for future deployments. For training, we found that hands-on training significantly improved the success rate of participants as opposed to training videos and manuals. Participants who came to small-group training sessions were also more positive about their experience and required far less “troubleshooting” feedback from CEAMS staff during their deployments than participants who relied on manuals or videos.
We also found that participants wanted immediate access to the data they collected. In the pilot campaign, we collected all the data and then later provided plotted results to the participants. However, many participants were no longer actively engaged. For the wider deployment, we plan to provide near real-time access to data and put more effort into data visualization for participants.
The pilot campaign network in northern Colorado allowed for an opportunity to test the AMOD device and an initial sampling strategy for the CEAMS network. Even with the limited number of participants and samples taken during the pilot campaign, we found that citizen scientists were able to successfully use low-cost devices to provide useful information on the spatial and temporal variability in air pollution. The measurements taken by participants were representative of the air quality measured by standard air quality instruments during the same time period but provided information at a higher spatial resolution. The measurements also provide insight on the diurnal and spatial variability in surface pollution, emission sources, the vertical distribution of pollution, and aerosol sizes. Thus, a wider deployment of the AMOD device through the CEAMS network can be used to (1) more comprehensively investigate local air quality and (2) provide information that can be used in conjunction with satellite observations to better understand air quality worldwide.
Future deployments are planned for other regions of the western US, locations which were strategically chosen to target situations that are notoriously difficult for using satellite observations to infer surface air quality. We are using the feedback from our citizen scientists and the information gained from analysis of the collected data to improve the device and our sampling strategy. In these future campaigns, we plan to deploy more instruments for an extended period of time. An improved version of the AMOD device is also being developed to address many of the issues encountered during the pilot campaign. We are updating the wavelength selection for the AMOD to exactly match that of the AERONET instruments, which will allow for a direct comparison. Because the Plantower PMS5003 results proved interesting and useful, we are including them in all future devices. The new AMOD also includes active solar tracking and will automate the AOD measurement to reduce misalignment errors. Additionally, the device will be able to take multiple AOD measurements per day, which will increase the amount of data collected and reduce the chance of missing a measurement day due to cloud contamination. By automating the AOD measurement and including a larger battery, we will also be able to increase the sampling period from 2 d to 5 d. The set-up time required for participants will be reduced; thus we will get more measurements without increasing an individual's effort. Additionally, we will be able to use the multiple AOD measurements with the real-time PM2.5 data to understand the sub-daily variability in the PM2.5 : AOD ratio.
All air quality data collected during the pilot network are available at the following URL: https://doi.org/10.25675/10217/198163 (Ford et al., 2019).
The supplement related to this article is available online at: https://doi.org/10.5194/amt-12-6385-2019-supplement.
BF, JRP, ML, SJ, and JV designed the study. ML, LVZ, and BF recruited scientists and created training materials. EW, DML, CQ, JT, JV, and CL designed and validated the AMOD device. CQ designed the mobile application. CL and JM led the filter analysis. BF, LVZ, EW, and JT trained participants. BF led the paper with JRP and JV; and all co-authors contributed to interpretation of results and paper editing.
The authors declare that they have no conflict of interest.
The authors wish to thank all the citizen scientists who participated in CEAMS. We also wish to thank the CoCoRaHS team, specifically Nolan Doesken, Noah Newman, and Peter Goble. We are thankful to the MODIS satellite teams and the AERONET teams and principal investigators for each site. This work was funded by NASA grant NNX17AF94A and 80NSSC18M0120.
This research has been supported by NASA (grant nos. NNX17AF94A and 80NSSC18M0120).
This paper was edited by Francis Pope and reviewed by two anonymous referees.
2015 TIGER/Line Shapefiles: (machinereadable data files)/prepared by the U.S. Census Bureau, available at: https://www.census.gov/geographies/mapping-files/time-series/geo/carto-boundary-file.2015.html (last access: 10 October 2019), 2015.
Ahmed, T., Dutkiewicz, V. A., Shareef, A., Tuncel, G., Tuncel, S., and Husain, L.: Measurement of black carbon (BC) by an optical method and a thermal-optical method: Intercomparison for four sites, Atmos. Environ., 43, 6305–6311, https://doi.org/10.1016/j.atmosenv.2009.09.031, 2009.
Amante, C. and Eakins, B. W.: ETOPO1 1 Arc-Minute Global Relief Model: Procedures, Data Sources and Analysis, NOAA Technical Memorandum NESDIS NGDC-24, National Geophysical Data Center, NOAA, https://doi.org/10.7289/V5C8276M, 2009.
Brauer, M., Amann, M., Burnett, R. T., Cohen, A., Dentener, F., Ezzati, M., Henderson, S. B., Krzyzanowski, M., Martin, R. V., Van Dingenen, R., van Donkelaar, A., and Thurston, G. D.: Exposure Assessment for Estimation of the Global Burden of Disease Attributable to Outdoor Air Pollution, Environ. Sci. Technol., 46, 652–660, https://doi.org/10.1021/es2025752, 2012.
Brauer, M., Freedman, G., Frostad, J., van Donkelaar, A., Martin, R. V., Dentener, F., Dingenen, R. van, Estep, K., Amini, H., Apte, J. S., Balakrishnan, K., Barregard, L., Broday, D., Feigin, V., Ghosh, S., Hopke, P. K., Knibbs, L. D., Kokubo, Y., Liu, Y., Ma, S., Morawska, L., Sangrador, J. L. T., Shaddick, G., Anderson, H. R., Vos, T., Forouzanfar, M. H., Burnett, R. T., and Cohen, A.: Ambient Air Pollution Exposure Estimation for the Global Burden of Disease 2013, Environ. Sci. Technol., 50, 79–88, https://doi.org/10.1021/acs.est.5b03709, 2015.
Bulot, F. M. J., Johnston, S. J., Basford, P. J., Easton, N. H. C., Apetroaie-Cristea, M., Foster, G. L., Morris, A. K. R., Cox, S. J., and Loxham, M.: Long-Term Field Comparison of Multiple Low-Cost Particulate Matter Sensors in an Outdoor Urban Environment, Sci. Rep., 9, 1–13, https://doi.org/10.1038/s41598-019-43716-3, 2019.
Calloway, C. P., Li, S., Buchanan, J. W., and Stevens, R. K.: A refinement of the potassium tracer method for residential wood smoke, Atmos. Environ., 23, 67–69, https://doi.org/10.1016/0004-6981(89)90098-X, 1989.
Chylek, P., Ramaswamy, V., Cheng, R., and Pinnick, R. G.: Optical properties and mass concentration of carbonaceous smokes, Appl. Opt., 20, 2980–2985, https://doi.org/10.1364/AO.20.002980, 1981.
Cifelli, R., Doesken, N., Kennedy, P., Carey, L. D., Rutledge, S. A., Gimmestad, C., and Depue, T.: The Community Collaborative Rain, Hail, and Snow Network: Informal Education for Scientists and Citizens, B. Am. Meteorol. Soc., 86, 1069–1078, https://doi.org/10.1175/BAMS-86-8-1069, 2005.
Cohen, A. J., Brauer, M., Burnett, R., Anderson, H. R., Frostad, J., Estep, K., Balakrishnan, K., Brunekreef, B., Dandona, L., Dandona, R., Feigin, V., Freedman, G., Hubbell, B., Jobling, A., Kan, H., Knibbs, L., Liu, Y., Martin, R., Morawska, L., Pope, C. A., Shin, H., Straif, K., Shaddick, G., Thomas, M., Dingenen, R. van, Donkelaar, A. van, Vos, T., Murray, C. J. L., and Forouzanfar, M. H.: Estimates and 25-year trends of the global burden of disease attributable to ambient air pollution: an analysis of data from the Global Burden of Diseases Study 2015, Lancet, 389, 1907–1918, https://doi.org/10.1016/S0140-6736(17)30505-6, 2017.
Crouse, D. L., Peters, P. A., van Donkelaar, A., Goldberg, M. S., Villeneuve, P. J., Brion, O., Khan, S., Atari, D. O., Jerrett, M., Pope, C. A., Brauer, M., Brook, J. R., Martin, R. V., Stieb, D., and Burnett, R. T.: Risk of Nonaccidental and Cardiovascular Mortality in Relation to Long-term Exposure to Low Concentrations of Fine Particulate Matter: A Canadian National-Level Cohort Study, Environ. Health Persp., 120, 708–714, https://doi.org/10.1289/ehp.1104049, 2012.
Eck, T. F., Holben, B. N., Reid, J. S., Dubovik, O., Smirnov, A., O'Neill, N. T., Slutsker, I., and Kinne, S.: Wavelength dependence of the optical depth of biomass burning, urban, and desert dust aerosols, J. Geophys. Res.-Atmos., 104, 31333–31349, https://doi.org/10.1029/1999JD900923, 1999.
Engel-Cox, J. A., Hoff, R. M., and Haymet, A. D. J.: Recommendations on the use of satellite remote-sensing data for urban air quality, J. Air Waste Manage., 54, 1360–1371, 2004.
Evans, J., van Donkelaar, A., Martin, R. V., Burnett, R., Rainham, D. G., Birkett, N. J., and Krewski, D.: Estimates of global mortality attributable to particulate air pollution using satellite imagery, Environ. Res., 120, 33–42, https://doi.org/10.1016/j.envres.2012.08.005, 2013.
Ford, B. and Heald, C. L.: An A-train and model perspective on the vertical distribution of aerosols and CO in the Northern Hemisphere, J. Geophys. Res., 117, D06211, https://doi.org/10.1029/2011JD016977, 2012.
Ford, B. and Heald, C. L.: Exploring the uncertainty associated with satellite-based estimates of premature mortality due to exposure to fine particulate matter, Atmos. Chem. Phys., 16, 3499–3523, https://doi.org/10.5194/acp-16-3499-2016, 2016.
Ford, B., Pierce, J. R., Wendt, E., Long, M., Jathar, S., Mehaffy, J., Tryner, J., Quinn, C., van Zyl, L., L'Orange, C., Miller-Lionberg, D., and Volckens, J.: Data collected during the pilot campaign of the Citizen-Enabled Aerosol Measurements for Satellites (CEAMS) network in Northern Colorado, https://doi.org/10.25675/10217/198163, 2019.
Forouzanfar, M. H., Alexander, L., Anderson, H. R., Bachman, V. F., Biryukov, S., Brauer, M., Burnett, R., Casey, D., Coates, M. M., Cohen, A., Delwiche, K., Estep, K., Frostad, J. J., KC, A., Kyu, H. H., Moradi-Lakeh, M., Ng, M., Slepak, E. L., Thomas, B. A., Wagner, J., Aasvang, G. M., Abbafati, C., Ozgoren, A. A., Abd-Allah, F., Abera, S. F., Aboyans, V., Abraham, B., Abraham, J. P., Abubakar, I., Abu-Rmeileh, N. M. E., Aburto, T. C., Achoki, T., Adelekan, A., Adofo, K., Adou, A. K., Adsuar, J. C., Afshin, A., Agardh, E. E., Al Khabouri, M. J., Al Lami, F. H., Alam, S. S., Alasfoor, D., Albittar, M. I., Alegretti, M. A., Aleman, A. V., Alemu, Z. A., Alfonso-Cristancho, R., Alhabib, S., Ali, R., Ali, M. K., Alla, F., Allebeck, P., Allen, P. J., Alsharif, U., Alvarez, E., Alvis-Guzman, N., Amankwaa, A. A., Amare, A. T., Ameh, E. A., Ameli, O., Amini, H., Ammar, W., Anderson, B. O., Antonio, C. A. T., Anwari, P., Cunningham, S. A., Arnlöv, J., Arsenijevic, V. S. A., Artaman, A., Asghar, R. J., Assadi, R., Atkins, L. S., Atkinson, C., Avila, M. A., Awuah, B., Badawi, A., Bahit, M. C., Bakfalouni, T., Balakrishnan, K., Balalla, S., Balu, R. K., Banerjee, A., Barber, R. M., Barker-Collo, S. L., Barquera, S., Barregard, L., Barrero, L. H., Barrientos-Gutierrez, T., Basto-Abreu, A. C., Basu, A., Basu, S., Basulaiman, M. O., Ruvalcaba, C. B., Beardsley, J., Bedi, N., Bekele, T., Bell, M. L., Benjet, C., Bennett, D. A., et al.: Global, regional, and national comparative risk assessment of 79 behavioural, environmental and occupational, and metabolic risks or clusters of risks in 188 countries, 1990–2013: a systematic analysis for the Global Burden of Disease Study 2013, Lancet, 386, 2287–2323, https://doi.org/10.1016/S0140-6736(15)00128-2, 2015.
Fu, J., Jiang, D., Lin, G., Liu, K., and Wang, Q.: An ecological analysis of PM2.5 concentrations and lung cancer mortality rates in China, BMJ Open, 5, e009452, https://doi.org/10.1136/bmjopen-2015-009452, 2015.
Gupta, P., Doraiswamy, P., Levy, R., Pikelnaya, O., Maibach, J., Feenstra, B., Polidori, A., Kiros, F., and Mills, K. C.: Impact of California Fires on Local and Regional Air Quality: The Role of a Low-Cost Sensor Network and Satellite Observations, GeoHealth, 2, 172–181, https://doi.org/10.1029/2018GH000136, 2018.
Holben, B. N., Eck, T. F., Slutsker, I., Tanré, D., Buis, J. P., Setzer, A., Vermote, E., Reagan, J. A., Kaufman, Y. J., Nakajima, T., Lavenu, F., Jankowiak, I., and Smirnov, A.: AERONET – A Federated Instrument Network and Data Archive for Aerosol Characterization, Remote Sens. Environ., 66, 1–16, https://doi.org/10.1016/S0034-4257(98)00031-5, 1998.
Hystad, P., Demers, P. A., Johnson, K. C., Brook, J., Donkelaar, A. van, Lamsal, L., Martin, R., and Brauer, M.: Spatiotemporal air pollution exposure assessment for a Canadian population-based lung cancer case-control study, Environ. Health, 11, 22, https://doi.org/10.1186/1476-069X-11-22, 2012.
Jerrett, M., Donaire-Gonzalez, D., Popoola, O., Jones, R., Cohen, R. C., Almanza, E., de Nazelle, A., Mead, I., Carrasco-Turigas, G., Cole-Hunter, T., Triguero-Mas, M., Seto, E., and Nieuwenhuijsen, M.: Validating novel air pollution sensors to improve exposure estimates for epidemiological analyses and citizen science, Environ. Res., 158, 286–294, https://doi.org/10.1016/j.envres.2017.04.023, 2017.
Jiao, W., Hagler, G., Williams, R., Sharpe, R., Brown, R., Garver, D., Judge, R., Caudill, M., Rickard, J., Davis, M., Weinstock, L., Zimmer-Dauphinee, S., and Buckley, K.: Community Air Sensor Network (CAIRSENSE) project: evaluation of low-cost sensor performance in a suburban environment in the southeastern United States, Atmos. Meas. Tech., 9, 5281–5292, https://doi.org/10.5194/amt-9-5281-2016, 2016.
Kaskaoutis, D. G. and Kambezidis, H. D.: Comparison of the Ångström parameters retrieval in different spectral ranges with the use of different techniques, Meteorol. Atmos. Phys., 99, 233–246, https://doi.org/10.1007/s00703-007-0279-y, 2008.
Kaufman, Y. J., Tanre, D., Remer, L. A., Vermote, E. F., Chu, A., and Holben, B. N.: Operational remote sensing of tropospheric aerosol over land from EOS moderate resolution imaging spectroradiometer, J. Geophys. Res.-Atmos., 102, 17051–17067, 1997.
Kelleher, S., Quinn, C., Miller-Lionberg, D., and Volckens, J.: A low-cost particulate matter (PM2.5) monitor for wildland fire smoke, Atmos. Meas. Tech., 11, 1087–1097, https://doi.org/10.5194/amt-11-1087-2018, 2018.
Kellogg, B. and Winberry, W. T. J.: Determination of metals in ambient particulate matter using x-ray fluorescence (XRF) spectroscopy, in Compendium of Methods for the Determination of 20 Inorganic Compounds in Ambient Air (EPA/625/R-96/010a), p. 36, Center for Environmental Research Information, Office of Research and Development, US Environmental Protection Agency, Cincinatti, OH, 1999.
Kelly, K. E., Whitaker, J., Petty, A., Widmera, C., Dybwadc, A., Sleeth, D., Martine, R., and Butterfield, A.: Ambient and laboratory evaluation of a low-cost particulate matter sensor, Environ. Pollut., 221, 491–500, https://doi.org/10.1016/j.envpol.2016.12.039, 2017.
King, M. D., Kaufman, Y. J., Tanré, D., and Nakajima, T.: Remote Sensing of Tropospheric Aerosols from Space: Past, Present, and Future, B. Am. Meteorol. Soc., 80, 2229–2260, https://doi.org/10.1175/1520-0477(1999)080<2229:RSOTAF>2.0.CO;2, 1999.
Kirchstetter, T. W. and Novakov, T.: Controlled generation of black carbon particles from a diffusion flame and applications in evaluating black carbon measurement methods, Atmos. Environ., 41, 1874–1888, https://doi.org/10.1016/j.atmosenv.2006.10.067, 2007.
Kreidenweis, S. M., Remer, L. A., Bruintjes, R., and Dubovik, O.: Smoke aerosol from biomass burning in Mexico: Hygroscopic smoke optical model, J. Geophys. Res.-Atmos, 106, 4831–4844, https://doi.org/10.1029/2000JD900488, 2001.
Kumar, P., Morawska, L., Martani, C., Biskos, G., Neophytou, M., Di Sabatino, S., Bell, M., Norford, L., and Britter, R.: The rise of low-cost sensing for managing air pollution in cities, Environ. Int., 75, 199–205, https://doi.org/10.1016/j.envint.2014.11.019, 2015.
Levy, R. C., Remer, L. A., Kleidman, R. G., Mattoo, S., Ichoku, C., Kahn, R., and Eck, T. F.: Global evaluation of the Collection 5 MODIS dark-target aerosol products over land, Atmos. Chem. Phys., 10, 10399–10420, https://doi.org/10.5194/acp-10-10399-2010, 2010.
Li, J., Carlson, B. E., and Lacis, A. A.: How well do satellite AOD observations represent the spatial and temporal variability of PM2.5 concentration for the United States?, Atmos. Environ., 102, 260–273, https://doi.org/10.1016/j.atmosenv.2014.12.010, 2015.
Lim, S. S., Vos, T., Flaxman, A. D., Danaei, G., Shibuya, K., Adair-Rohani, H., AlMazroa, M. A., Amann, M., Anderson, H. R., Andrews, K. G., Aryee, M., Atkinson, C., Bacchus, L. J., Bahalim, A. N., Balakrishnan, K., Balmes, J., Barker-Collo, S., Baxter, A., Bell, M. L., Blore, J. D., Blyth, F., Bonner, C., Borges, G., Bourne, R., Boussinesq, M., Brauer, M., Brooks, P., Bruce, N. G., Brunekreef, B., Bryan-Hancock, C., Bucello, C., Buchbinder, R., Bull, F., Burnett, R. T., Byers, T. E., Calabria, B., Carapetis, J., Carnahan, E., Chafe, Z., Charlson, F., Chen, H., Chen, J. S., Cheng, A. T.-A., Child, J. C., Cohen, A., Colson, K. E., Cowie, B. C., Darby, S., Darling, S., Davis, A., Degenhardt, L., Dentener, F., Des Jarlais, D. C., Devries, K., Dherani, M., Ding, E. L., Dorsey, E. R., Driscoll, T., Edmond, K., Ali, S. E., Engell, R. E., Erwin, P. J., Fahimi, S., Falder, G., Farzadfar, F., Ferrari, A., Finucane, M. M., Flaxman, S., Fowkes, F. G. R., Freedman, G., Freeman, M. K., Gakidou, E., Ghosh, S., Giovannucci, E., Gmel, G., Graham, K., Grainger, R., Grant, B., Gunnell, D., Gutierrez, H. R., Hall, W., Hoek, H. W., Hogan, A., Hosgood III, H. D., Hoy, D., Hu, H., Hubbell, B. J., Hutchings, S. J., Ibeanusi, S. E., Jacklyn, G. L., Jasrasaria, R., Jonas, J. B., Kan, H., Kanis, J. A., Kassebaum, N., Kawakami, N., Khang, Y.-H., Khatibzadeh, S., Khoo, J.-P., et al.: A comparative risk assessment of burden of disease and injury attributable to 67 risk factors and risk factor clusters in 21 regions, 1990–2010: a systematic analysis for the Global Burden of Disease Study 2010, Lancet, 380, 2224–2260, https://doi.org/10.1016/S0140-6736(12)61766-8, 2012.
Liu, Y., Sarnat, J. A., Kilaru, V., Jacob, D. J., and Koutrakis, P.: Estimating ground-level PM2.5 in the eastern United States using satellite remote sensing, Environ. Sci. Technol., 39, 3269–3278, 2005.
Liu, Y., Franklin, M., Kahn, R., and Koutrakis, P.: Using aerosol optical thickness to predict ground-level PM2.5 concentrations in the St. Louis area: A comparison between MISR and MODIS, Remote Sens. Environ., 107, 33–44, https://doi.org/10.1016/j.rse.2006.05.022, 2007.
Remer, L. A., Kaufman, Y. J., Tanre, D., Mattoo, S., Chu, D. A., Martins, J. V., Li, R. R., Ichoku, C., Levy, R. C., Kleidman, R. G., Eck, T. F., Vermote, E., and Holben, B. N.: The MODIS aerosol algorithm, products, and validation, J. Atmos. Sci., 62, 947–973, 2005.
Sayahi, T., Butterfield, A., and Kelly, K. E.: Long-term field evaluation of the Plantower PMS low-cost particulate matter sensors, Environ. Pollut., 245, 932–940, https://doi.org/10.1016/j.envpol.2018.11.065, 2019.
Sayer, A. M., Hsu, N. C., Bettenhausen, C., and Jeong, M.-J.: Validation and uncertainty estimates for MODIS Collection 6 “Deep Blue” aerosol data, J. Geophys. Res.-Atmos., 118, 7864–7872, https://doi.org/10.1002/jgrd.50600, 2013.
Smirnov, A., Holben, B. N., Eck, T. F., Dubovik, O., and Slutsker, I.: Cloud-Screening and Quality Control Algorithms for the AERONET Database, Remote Sens. Environ., 73, 337–349, https://doi.org/10.1016/S0034-4257(00)00109-7, 2000.
Snider, G., Weagle, C. L., Martin, R. V., van Donkelaar, A., Conrad, K., Cunningham, D., Gordon, C., Zwicker, M., Akoshile, C., Artaxo, P., Anh, N. X., Brook, J., Dong, J., Garland, R. M., Greenwald, R., Griffith, D., He, K., Holben, B. N., Kahn, R., Koren, I., Lagrosas, N., Lestari, P., Ma, Z., Vanderlei Martins, J., Quel, E. J., Rudich, Y., Salam, A., Tripathi, S. N., Yu, C., Zhang, Q., Zhang, Y., Brauer, M., Cohen, A., Gibson, M. D., and Liu, Y.: SPARTAN: a global network to evaluate and enhance satellite-based estimates of ground-level particulate matter for global health applications, Atmos. Meas. Tech., 8, 505–521, https://doi.org/10.5194/amt-8-505-2015, 2015.
Snik, F., Rietjens, J. H. H., Apituley, A., Volten, H., Mijling, B., Di Noia, A., Heikamp, S., Heinsbroek, R. C., Hasekamp, O. P., Smit, J. M., Vonk, J., Stam, D. M., van Harten, G., de Boer, J., Keller, C. U., and 3187 iSPEX citizen scientists: Mapping atmospheric aerosols with a citizen science network of smartphone spectropolarimeters, Geophys. Res. Lett., 41, 2014GL061462, https://doi.org/10.1002/2014GL061462, 2014.
Snyder, E. G., Watkins, T. H., Solomon, P. A., Thoma, E. D., Williams, R. W., Hagler, G. S. W., Shelow, D., Hindin, D. A., Kilaru, V. J., and Preuss, P. W.: The Changing Paradigm of Air Pollution Monitoring, Environ. Sci. Technol., 47, 11369–11377, https://doi.org/10.1021/es4022602, 2013.
South Coast Air Quality Management District (SCAQMD): Field Evaluation Purple Air PM Sensor, available at: http://www.aqmd.gov/docs/default-source/aq-spec/field-evaluations/purpleair]---field-evaluation.pdf?sfvrsn=2 (last access: 6 Novemer 2019), 2017a.
South Coast Air Quality Management District (SCAQMD): Laboratory Evaluation Purple Air PA-II PM Sensor, available at: http://www.aqmd.gov/docs/default-source/aq-spec/field-evaluations/purple-air-pa-ii---field-evaluation.pdf?sfvrsn=4 (last access: 6 November 2019), 2017b.
Tanre, D., Kaufman, Y. J., Herman, M., and Mattoo, S.: Remote sensing of aerosol properties over oceans using the MODIS/EOS spectral radiances, J. Geophys. Res.-Atmos., 102, 16971–16988, 1997.
van Donkelaar, A., Martin, R. V., and Park, R. J.: Estimating ground-level PM2.5 using aerosol optical depth determined from satellite remote sensing, J. Geophys. Res.-Atmos., 111, D21, https://doi.org/10.1029/2005JD006996, 2006.
van Donkelaar, A., Martin, R. V., Brauer, M., Kahn, R., Levy, R., Verduzco, C., and Villeneuve, P. J.: Global Estimates of Ambient Fine Particulate Matter Concentrations from Satellite-Based Aerosol Optical Depth: Development and Application, Environ. Health Persp., 118, 847–855, https://doi.org/10.1289/ehp.0901623, 2010.
van Donkelaar, A., Martin, R. V., Spurr, R. J. D., Drury, E., Remer, L. A., Levy, R. C., and Wang, J.: Optimal estimation for global ground-level fine particulate matter concentrations, J. Geophys. Res.-Atmos., 118, 5621–5636, https://doi.org/10.1002/jgrd.50479, 2013.
van Donkelaar, A., Martin, R. V., Brauer, M., and Boys, B. L.: Use of Satellite Observations for Long-Term Exposure Assessment of Global Concentrations of Fine Particulate Matter, Environ. Health Persp., 123, 135–43, https://doi.org/10.1289/ehp.1408646, 2014.
van Donkelaar, A., Martin, R. V., Spurr, R. J. D., and Burnett, R. T.: High-Resolution Satellite-Derived PM2.5 from Optimal Estimation and Geographically Weighted Regression over North America, Environ. Sci. Technol., 49, 10482–10491, https://doi.org/10.1021/acs.est.5b02076, 2015a.
van Donkelaar, A., Martin, R. V., Brauer, M., and Boys, B.: Use of Satellite Observations for Long-Term Exposure Assessment of Global Concentrations of Fine Particulate Matter, Environ. Health Persp., 123, 135–143, https://doi.org/10.1289/ehp.1408646, 2015b.
van Donkelaar, A., R.V. Martin, M. Brauer, N. C. Hsu, R. A. Kahn, R. C Levy, A. Lyapustin, A. M. Sayer, and D. M Winker, Global Estimates of Fine Particulate Matter using a Combined Geophysical-Statistical Method with Information from Satellites, Models, and Monitors, Environ. Sci. Technol., 50, 3762–3772, https://doi.org/10.1021/acs.est.5b05833, 2016.
Villeneuve, P. J., Weichenthal, S. A., Crouse, D., Miller, A. B., To, T., Martin, R. V., van Donkelaar, A., Wall, C., and Burnett, R. T.: Long-term Exposure to Fine Particulate Matter Air Pollution and Mortality Among Canadian Women, Epidemiol. Camb. Mass, 26, 536–545, https://doi.org/10.1097/EDE.0000000000000294, 2015.
Volckens, J., Quinn, C., Leith, D., Mehaffy, J., Henry, C. S., and Miller-Lionberg, D.: Development and Evaluation of an Ultrasonic Personal Aerosol Sampler (UPAS), Indoor Air, https://doi.org/10.1111/ina.12318, 2017.
Weber, S. A., Engel-Cox, J. A., Hoff, R. M., Prados, A. I., and Zhang, H.: An improved method for estimating surface fine particle concentrations using seasonally adjusted satellite aerosol optical depth, J. Air Waste Manage., 60, 574–585, 2010.
Wendt, E. A., Quinn, C. W., Miller-Lionberg, D. D., Tryner, J., L'Orange, C., Ford, B., Yalin, A. P., Pierce, J. R., Jathar, S., and Volckens, J.: A low-cost monitor for simultaneous measurement of fine particulate matter and aerosol optical depth – Part 1: Specifications and testing, Atmos. Meas. Tech., 12, 5431–5441, https://doi.org/10.5194/amt-12-5431-2019, 2019.
Yong, Z.: Digital Universal Particle Concentration Sensor PMS5003 Series Data Manual, available at: http://www.aqmd.gov/docs/default-source/aq-spec/resources-page/plantower-pms5003-manual_v2-3.pdf (last access: 7 July 2019), 2016.
Zhang, H., Hoff, R. M., and Engel-Cox, J. A.: The relation between Moderate Resolution Imaging Spectroradiometer (MODIS) aerosol optical depth and PM2.5 over the United States: a geographical comparison by U.S. Environmental Protection Agency regions, J. Air Waste Manage., 59, 1358–1369, 2009.
Zikova, N., Masiol, M., Chalupa, D. C., Rich, D. Q., Ferro, A. R., and Hopke, P. K.: Estimating Hourly Concentrations of PM2.5 across a Metropolitan Area Using Low-Cost Particle Monitors, Sensors, 17, 1922, https://doi.org/10.3390/s17081922, 2017.
- Article
(2348 KB) - Full-text XML