the Creative Commons Attribution 4.0 License.
the Creative Commons Attribution 4.0 License.
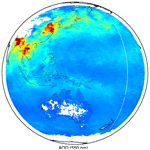
Applying the Dark Target aerosol algorithm with Advanced Himawari Imager observations during the KORUS-AQ field campaign
Robert C. Levy
Shana Mattoo
Lorraine A. Remer
Robert E. Holz
Andrew K. Heidinger
For nearly 2 decades we have been quantitatively observing the Earth's aerosol system from space at one or two times of the day by applying the Dark Target family of algorithms to polar-orbiting satellite sensors, particularly MODIS and VIIRS. With the launch of the Advanced Himawari Imager (AHI) and the Advanced Baseline Imagers (ABIs) into geosynchronous orbits, we have the new ability to expand temporal coverage of the traditional aerosol optical depth (AOD) to resolve the diurnal signature of aerosol loading during daylight hours. The Korean–United States Air Quality (KORUS-AQ) campaign taking place in and around the Korean peninsula during May–June 2016 initiated a special processing of full-disk AHI observations that allowed us to make a preliminary adoption of Dark Target aerosol algorithms to the wavelengths and resolutions of AHI. Here, we describe the adaptation and show retrieval results from AHI for this 2-month period. The AHI-retrieved AOD is collocated in time and space with existing AErosol RObotic NETwork stations across Asia and with collocated Terra and Aqua MODIS retrievals. The new AHI AOD product matches AERONET, and the standard MODIS product does as well, and the agreement between AHI and MODIS retrieved AOD is excellent, as can be expected by maintaining consistency in algorithm architecture and most algorithm assumptions. Furthermore, we show that the new product approximates the AERONET-observed diurnal signature. Examining the diurnal patterns of the new AHI AOD product we find specific areas over land where the diurnal signal is spatially cohesive. For example, in Bangladesh the AOD increases by 0.50 from morning to evening, and in northeast China the AOD decreases by 0.25. However, over open ocean the observed diurnal cycle is driven by two artifacts, one associated with solar zenith angles greater than 70∘ that may be caused by a radiative transfer model that does not properly represent the spherical Earth and the other artifact associated with the fringes of the 40∘ glint angle mask. This opportunity during KORUS-AQ provides encouragement to move towards an operational Dark Target algorithm for AHI. Future work will need to re-examine masking including snow mask, re-evaluate assumed aerosol models for geosynchronous geometry, address the artifacts over the ocean, and investigate size parameter retrieval from the over-ocean algorithm.
- Article
(7993 KB) - Full-text XML
-
Supplement
(4226 KB) - BibTeX
- EndNote
Atmospheric aerosols, small liquid or solid particles suspended in the atmosphere, play a key role in Earth's energy balance, cloud physics, geochemical cycles, and air quality/public health (Boucher et al., 2013; Rosenfeld et al., 2014a, b; Seinfeld et al., 2016; Jickells et al., 2005; Yu et al., 2015; Lim et al., 2012). These particles originate from both human activity and natural processes, and they can cover vast regions of the globe. Observations from satellite sensors provide the best means for monitoring and quantifying the extent and transport of large-scale aerosol events (Kaufman et al., 2005; Yu et al., 2012), and they provide some characterization of aerosol particle properties (Remer et al., 2005; Torres et al., 2013; Kalashnikova and Kahn, 2006; Kahn and Gaitley, 2015). Especially since the launch of NASA's Earth Observing System (EOS) and similar satellites by international agencies, the community has benefitted from nearly 2 decades of quantitative measures of the global aerosol system. While both passive and active sensors have contributed to our understanding of the global aerosol system, here we focus on only passive sensors. These, such as the Moderate Resolution Imaging Spectroradiometer (MODIS) (Levy et al., 2013; Hsu et al., 2013; Lyapustin et al., 2011), the Multi-angle Imaging SpectroRadiometer (MISR) (Diner et al., 1998; Martonchik, 1998; Kahn et al., 2010), the Ozone Monitoring Instrument (OMI) (Torres et al., 2013), and POLarization and Directionality of the Earth's Reflectances (POLDER) (Tanré et al., 2011), have provided instantaneous measures of aerosol loading, particle size, particle absorption, and aerosol type across the globe. The community has used these data to calculate decadal statistics of aerosol climatology, seasonal and monthly statistics, quantitative measures of intercontinental aerosol transport, and fertilization of ecosystems (Remer et al., 2008; Yu et al., 2012, 2013, 2015). These satellite aerosol products have been used to estimate aerosol radiative effects and climate forcing, associations between aerosols and cloud micro- and macrophysics, precipitation, air quality, and public health, and they have provided critical constraints on global climate modeling (Zhang et al., 2005; Koren et al., 2005; Lin et al., 2006; Wang and Christopher, 2003; Quaas et al., 2009; Patadia et al., 2008; to give just one early example of each application).
The sensors mentioned above all have been launched on polar orbiting satellites in low earth orbit (LEO). Such satellites are sun synchronous, passing over each location on Earth at approximately the same local solar time each day. A LEO sensor imaging a broad swath of Earth will image every spot on Earth and thus achieve full global coverage in 1 or 2 d. However, each spot on Earth is only viewed once per day in daylight and once per day at night, always at approximately the same local solar time. In contrast a geosynchronous (GEO) satellite orbits at a high altitude above Earth, matching the same period as the Earth's rotation. A sensor on a GEO satellite can scan the full or partial portion of Earth facing the satellite. Neither the sensor nor the Earth appear to move in these images, although the terminator between day and night on the Earth appears to move from east to west across the image over time. A GEO imager always views the same Earth locations across approximately one-third of the Earth and cannot by itself provide full global coverage. However, a sensor on a GEO satellite can provide information on the aerosol in any viewed location as a function of time of the day, enabling monitoring of the diurnal cycle.
For about a decade there has been a publicly available operational aerosol product derived from a GEO sensor. This is the GOES Aerosol Smoke Product (GASP) (Prados et al., 2007), where GOES stands for Geostationary Operational Environmental Satellite. GASP provides aerosol optical depth for the daylight section of the continental United States at 4 km spatial resolution every 30 min in near-real time, and the data are archived. The sensor has only five channels, one spectrally broad channel (0.52–0.71 µm) in the visible range and four in the near to thermal infrared range. The aerosol retrieval algorithm makes use of the infrared channels for cloud masking, but it must acquire all of its aerosol information from a single visible channel. The lack of a channel in the shortwave infrared range (e.g., 2.1 or 2.2 µm) prohibits application of an EOS-era Dark Target retrieval (Kaufman et al., 1997; Levy et al., 2007) and the lack of any channel in the blue range eliminates the possibility of a Deep Blue retrieval (Hsu et al., 2004, 2013). Thus the GASP retrieval is handicapped by the relative primitiveness of the GOES-13 sensor. Even so, aerosol optical depth (AOD) retrievals from GASP collocated and compared with AOD measurements from the AErosol RObotic NETwork (AERONET; Holben et al., 1998) at 10 sites in the northeastern US and Canada showed reasonable agreement. Regression of GASP and AERONET AOD derived correlation of 0.79, rms difference of 0.13, and absolute bias of less than 30 % for larger AOD (e.g., AOD >0.1). Validation in the southeast and western US was less good. The GASP validation statistics are reasonable, but not as good as those produced by MODIS AOD retrievals at the same AERONET locations. The main point of GASP, though, is not its absolute accuracy, but that it provides quantitative information on the diurnal cycle of aerosol across the continental United States and southern Canada.
We are now entering a new era in GEO observations. With the launch of the Advanced Himawari Imager (AHI) (Yu and Wu, 2016) and the Advanced Baseline Imager (ABI) on GOES-16 and GOES-17 (Kalluri et al., 2018; Kondragunta et al., 2019), we have sensors in GEO orbit with spectral capability similar to MODIS. AHI has 16 bands, including three in the visible range and another in the shortwave infrared (SWIR) range. ABI also has 16 bands, but distributed differently across the visible to the SWIR ranges. This spectral capability combined with nominal spatial resolution of 0.5 to 2 km creates opportunity for aerosol retrievals that can advance beyond what GASP could produce. Aerosol algorithms developed and implemented by the agencies responsible for the operations of the GEO satellites are or will be produced operationally and made public. These include the Japanese Meteorological Agency (JMA) for AHI on Himawari (Uesawa, 2016) and the National Oceanographic and Atmospheric Agency (NOAA) for ABI on the GOES-R series. In addition to these official operational products, other algorithms have been developed that make use of the new generation of GEO observations for aerosol retrievals, especially for AHI data (Sekiyama et al., 2015; Yumimoto et al., 2016; Lim et al., 2016, 2018a, b; Zhang et al., 2018; Yoshida et al., 2018; Yang et al., 2018; Shi et al., 2018; Yan et al., 2018; Choi et al., 2019). Some of these alternative aerosol products are research algorithms for specific purposes, while others could be of general interest and could be made public.
Because the capabilities of the new GEO sensors replicate the important spectral and spatial capabilities of the MODIS sensors, the MODIS Dark Target (DT) algorithms over land and ocean (Remer et al., 2005; Levy et al., 2010, 2015, 2018; Gupta et al., 2016) can be applied to AHI or ABI observations with only minor adjustments. The expectation is that the resulting aerosol product will match the original MODIS product in terms of accuracy and availability (number of retrievals). More than providing just another alternative aerosol product to the community, application of the traditional DT algorithm to GEO sensors offers continuity with a nearly 20-year well-studied, validated, and accepted aerosol product. The continuity of a DT AHI or ABI algorithm means that there could be an accurate MODIS-like aerosol product that resolves the daytime diurnal cycle, providing a well-understood quantitative measure of aerosol loading at fine temporal resolution at the large regional scale.
In this study we present the results of the first attempt at porting the MODIS DT aerosol algorithm to the AHI sensor on the Himawari-8 geosynchronous satellite. The study makes use of a special limited data set of AHI spectral reflectances, prepared for research purposes during the KORUS-AQ field campaign during May–June 2016. The purpose of this work is to test whether there is any skill in applying the DT to AHI and whether the goal of a continuous time series of retrieved AOD from MODIS to AHI has any probability of success. Furthermore, the study will identify issues that arise from the new geometry and demonstrate the ability of the new sensor to resolve aerosol signals using the DT algorithm that previous sensors could not.
The AHI inputs and the algorithm will be described in Sect. 2, with emphasis made on how the AHI algorithm differs compared to the MODIS implementation. Section 3 will present results and compare these with standard MODIS retrievals and collocated ground-based observations. Section 4 will explore the AHI aerosol product's diurnal cycle at AERONET stations for validation and then question how well the diurnal mean AOD inferred from once-a-day LEO observations compares with a truer diurnal mean compared from observations made at finer temporal resolution. Finally, results will be summarized and discussed in Sect. 5.
2.1 AHI sensor characteristics
The AHI was first launched on the Himawari-8 satellite in 2014 and became operational in July 2015. It is in geosynchronous orbit over the Equator at 140.7∘ E. The second AHI was launched on the Himawari-9 in November 2016 and remains in standby mode. The instrument has the capability to image a mesoscale region every 30 s while providing full-disk coverage every 10 min. In this study, the full-disk data have been used. The data to be presented here were obtained from the University of Wisconsin atmospheric Science Investigator lead Processing System (SIPS), which processed the NOAA's operational cloud operating system – extended (CLAVR-x), which provides radiance values at all 16 channels at a consistent 2 km resolution as a diagnostic/byproduct of the cloud retrieval. SIPS made the AHI data available specifically to support the KORUS-AQ campaign and for research purposes, and thus only 2 months of data were available. For this analysis we processed the DT algorithm at 1 h temporal resolution from 00:00 to 08:00 UTC, nine full-disk images per day. Figure 1 shows an example of the AHI full-disk image. AHI wavelengths used in the DT aerosol retrieval along with their spatial resolution are shown in Table 1, and compared with their counterparts from the MODIS and Visible Infrared Imaging Radiometer Suite (VIIRS) instruments. From Table 1 we see that AHI nearly matches MODIS and VIIRS, wavelength by wavelength in the bands needed by the DT algorithm, except for missing the 1.24 µm band that is used in the aerosol retrieval over ocean and also in masking snow–ice over land and sediments in the ocean. It is also missing the 1.38 µm channel that the DT aerosol algorithm has relied on for identifying and masking thin cirrus. For the bands that overlap MODIS, although close in spectral resolution, they do not exactly match. For this reason the algorithm lookup tables (LUTs), gas absorption corrections, etc. cannot be applied directly from the current MODIS algorithm and must be calculated specifically for AHI.
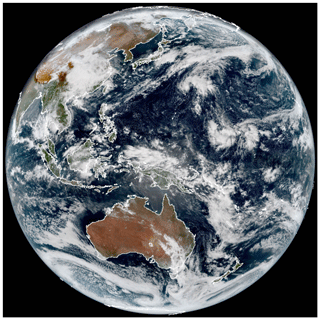
Figure 1Full-disk true color image from AHI using the 0.64, 0.51, and 0.47 µm channels. The image was taken on 20 October 2018 at 02:10 UTC. The image is created using following tool: https://rammb-slider.cira.colostate.edu/?sat=himawari, and it has been obtained from the Himawari-8 slider hosted by Colorado State University, which can be accessed at https://rammb-slider.cira.colostate.edu/?sat=himawari.
AHI's native spatial resolution is coarser than MODIS's, but comparable to VIIRS. Note, however, that the spatial resolution noted in Table 1 refers to the subsatellite point. The spatial resolution of Earth scenes at the edges of MODIS and VIIRS swaths or at the edge of the AHI disk will have spread from their subsatellite value. MODIS pixels spread by 4 times their nadir value, and VIIRS pixels spread by 2 times their nadir value. AHI pixels remain 1–3 times their size at the subsatellite point for all but the extreme edge of the full-disk image. Also note that the actual KORUS-AQ data used in this study have reduced spatial resolution in all channels (2 km).
The fact that the wavelengths and spatial resolution of AHI differ from the heritage DT aerosol algorithm means that while the structure, heritage, and experience of the MODIS DT algorithm can be adapted for AHI to maintain as much continuity as possible, the resulting AHI algorithm and product will not be an identical twin.
2.2 Dark Target AHI aerosol algorithm and research product
The DT aerosol algorithms are a family of algorithms, based on the original two algorithms, that retrieved aerosol over ocean and over land from the MODIS instruments aboard NASA's Terra and Aqua satellites. Levy et al. (2010, 2015, 2018) and the online Algorithm Theoretical Basis Document (https://darktarget.gsfc.nasa.gov, 15 January 2019) describe these algorithms in depth. Here we only provide an overview in order to highlight the differences between the original algorithms and the DT algorithm applied to AHI inputs. DT algorithms should not be confused with other operational NASA aerosol algorithms applied to MODIS inputs (e.g., Deep Blue: Hsu et al., 2013; and MAIAC: Lyapustin et al., 2011). Both DT ocean and DT land procedures use lookup tables (LUTs). LUTs are created by using radiative transfer (RT) code to simulate spectral top-of-atmosphere (TOA) reflectance for expected conditions of aerosols over a theoretical rough ocean surface or black land surface. These LUTs assume intrinsic physical and optical properties (size, shape, refractive index) as well as total column loading of atmospheric aerosols.
The original DT retrieval relies on seven reflective solar bands for aerosol retrieval and one for cirrus detection and masking (Table 1). Additional bands are used for tasks like cloud masking, snow identification, etc. The algorithm adapted for AHI makes use of six bands for the aerosol retrieval that are similar but not exactly the same as the original MODIS ones. The differences require new corrections for trace gas absorption in the channels and the calculations of new LUTs. It is thus impossible to exactly duplicate the DT algorithm as it is ported from sensor to sensor. However, the basic physical assumptions, RT codes, algorithm architecture, and intrinsic physical and optical properties used to calculate the LUTs are the same in the AHI DT algorithm as they are in the current MODIS and VIIRS DT algorithms.
Table 1MODIS, VIIRS, and AHI wavelengths in micrometers used directly in the DT algorithm (bold) and subsatellite point spatial resolution in kilometers. The table presents native resolution of sensors, but this study uses a special run of AHI where all spectral channels were reduced to a resolution of 2 km.
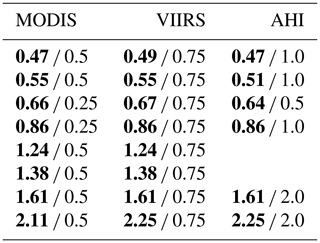
The greatest consequences to missing the 1.24 µm band is in sediment masking for ocean and snow–ice masking for land. New techniques that compensate for the missing information were applied to the AHI data. For sediment masking, we follow Li et al. (2003) as is standard for the DT algorithm, but substitute the 1.61 channel for the standard 1.24 µm channel. Physically this substitution should work, as both channels are expected to be black in sea water, which provides the background from which sediments are flagged, but the substitution has not yet been well-vetted. We have not yet devised a substitute for the overland snow–ice mask. The data analyzed and shown here are from May and June 2016, months when no snow is expected in the domain. Devising, testing, and implementing an AHI snow mask will be needed before the DT AHI algorithm can be applied year-round. In terms of the direct aerosol retrieval, the lack of the 1.24 µm information only affects the over-ocean algorithm slightly, as the information from the 0.86 µm and two longer wavelengths compensate for its absence (Tanré et al., 1996, 1997).
The loss of the 1.38 µm channel may have more pronounced consequences as it proved to be the first line of defense against thin cirrus contamination in the aerosol product (Gao and Kaufman, 1995). In this initial adaptation of the algorithm to AHI we have not implemented any alternative test for thin cirrus, and therefore cirrus contamination is expected in the results shown here. For clouds other than thin cirrus, we apply an internal cloud mask to the input radiances, similar to the traditional MODIS aerosol cloud mask. This mask is based on spatial variability of groupings of 3×3 input radiance pixels (Martins et al., 2002), and the same thresholds were used. However, while the MODIS aerosol cloud mask also incorporates specific tests from the standard MODIS cloud mask (MOD–MYD35: Frey et al., 2008) that are based on thermal infrared channels, those products are not available for AHI. No direct substitution is employed to compensate. The absence of these specific external cloud mask tests will mostly affect high, cold cloud identification. Because alternative methods have not been developed for masking clouds, and the alternative method for identifying sediments has not been vetted to the same extent as the original MODIS DT masking techniques, the possibility of contamination from these features affecting the aerosol retrievals is higher than expectations based on the MODIS heritage.
The traditional MODIS DT algorithm aggregates 20×20 pixels at 0.5 km resolution to form a “retrieval box”. These 400 pixels are screened for clouds, glint, sediments, improper land surfaces, and other elements. Then the remaining pixels that have escaped the masking are sorted from high to low reflectance, and the darkest and brightest “good” pixels are arbitrarily eliminated. Darkest is defined as the darkest 20 % over land and 25 % over ocean. Brightest is defined as the brightest 50 % over land and 25 % over ocean. At that point, the spectral reflectance from those pixels that remain after the two-tiered elimination process are averaged to represent the mean spectral reflectance in the nominal 10 km × 10 km retrieval box. The algorithm proceeds with the inversion using that representative spectral reflectance and produces one set of aerosol properties representative of the retrieval box.
The AHI retrieval algorithm adapts this MODIS process for its coarser spatial resolution by aggregating 10×10 pixels at 2.0 km resolution to create retrieval boxes that have nominal resolution of 20 km × 20 km (at the subsatellite point). The same two-tier elimination process using modified cloud, sediment, glint, etc. masking and removal of the darkest and brightest pixels is applied. Both MODIS and AHI remove the same percentage of dark and bright pixels. Because AHI starts the process with 100 pixels but MODIS starts with 400 pixels, there are fewer pixels to remove with AHI and a smaller number of pixels remaining to be used to represent the spectral reflectance in the box with AHI. After the representative reflectances have been calculated there are corrections for gas absorption (H2O, O3, CO2). The result is a single set of spectral reflectances in the six bands that is input to the retrieval algorithm. Additional inputs include ancillary data such as ozone profiles, wind speed, and water vapor columns from NOAA's Global Data Assimilation System (GDAS) reanalysis data and a global land–sea mask generated by CLAVR-x at 2 km resolution.
Whether ocean or land, the DT retrieval searches the pre-computed LUTs to find the best match to the spectral observations. The overland algorithm makes use of measured reflectance at 0.47, 0.66, and 2.1 µm and assumptions about the surface reflectance to determine the aerosol loading and establish the relative weights between two aerosol models, both defined by geographical location and season. Over ocean, the algorithm uses six wavelengths (0.55, 0.66, 0.86, 1.24, 1.61, and 2.13 µm) to determine the aerosol loading and define an aerosol model from one fine mode and one coarse mode and the relative weight between these modes. There are no restrictions on the distribution of modes by location and season in the ocean algorithm. Once the aerosol model is defined by the weighting between models or modes, the spectral extinction of the aerosol is defined. The retrieved aerosol loading can be translated to AOD at any wavelength because of the known spectral extinction, and all wavelengths are reported in the output. The primary wavelength we will use here is AOD at 0.55 µm. Two measures of aerosol particle size are given for the over-ocean retrieval, fine-mode weighting and Ångström exponent (AE). AHI-retrieved aerosol size parameters will not be examined in this paper. Although the ocean and land retrievals have similarities, the details are different because land surface optical properties are different than ocean. The ocean algorithm calculates a “rough” surface (whitecaps, foam, glitter), which is a function of wind speed, while the land algorithm assumes quasi-static ratios between blue (0.47 µm), red (0.64 µm), and shortwave infrared (e.g., 2.25 µm) wavelengths. Land surface ratios for the retrievals shown in this study are identical to those used by the standard MODIS Collection 6.1 algorithm. Different wavelengths and different viewing geometry may introduce unexpected uncertainties. Of particular concern is the assumption that LEO land surface ratios will hold for the new GEO view geometry. Previously land surface ratios were found to have only a weak dependence on the viewing geometry encountered by a LEO observation (Levy et al., 2007), but the range of geometries encountered by a GEO instrument are different and require further analysis. Still, the original assumption of predictable surface reflectance ratios is based on the physical linkage between chlorophyll and liquid water light absorption that should continue to transcend bidirectional reflectance distribution function (BRDF) and other angular effects. In addition to the aerosol properties, DT provides many diagnostics including quality assurance and confidence (QAC).
The new AHI DT algorithm was applied to input AHI full-disk radiances, the daylight portion of the disk only. View angles were confined to less than 72∘ and solar zenith angles were restricted to less than 80∘. The period of analysis spans 2 months (May–June 2016). Given nine images per day, the database for analysis thus includes more than 549 disk images of AOD derived from AHI inputs using the new AHI DT algorithm.
2.3 MODIS aerosol products
The AOD retrieved from AHI using the DT retrieval will be compared with the more established and well-characterized DT AOD product from MODIS on board the Terra and Aqua satellites. Specifically we will be accessing Collection 6.1 Level 2 MOD04 and MYD04 data products, where MOD refers to products derived from Terra MODIS inputs and MYD refers to those derived from Aqua MODIS inputs. Level 2 refers to derived geophysical parameters from the Level 1b geolocated and calibrated measured radiance inputs. Level 2 data are provided in 5 min cut sections of the orbital image called granules. These images are not gridded, but instead follow directly from the instrument scan as it follows its orbital path. There are many individual aerosol and diagnostic parameters within each MOD and MYD04 file. This study makes use of only one parameter, Optical_Depth_Land_And_Ocean. This parameter combines the retrieved AOD at 0.55 µm from the independent algorithms applied separately over land and ocean, and it uses only those retrievals identified with the highest quality (QAC = 3 over land and QAC > 0 over ocean). MODIS granules were selected that fall within the daylight portion of the AHI radiances, corresponding to the same days of the AHI images analyzed. Further temporal (±0.5 h) and spatial (0.25×0.25∘) collocations have been performed for specific analysis.
2.4 AERONET aerosol products
AERONET is a global ground network of CIMEL sun–sky radiometers and data processing and analysis software commonly used to evaluate satellite-derived aerosol products (Holben et al., 1998). In this work, only the direct sun measurements will be used. AERONET processes these spectral measurements to derive AOD at the wavelengths corresponding to the direct sun measurements. The AERONET spectral AOD product is a community standard for satellite-derived AOD validation, given that AERONET's AOD uncertainty of 0.01–0.02 (Eck et al., 1999) is sufficiently more accurate and precise than can be expected by any satellite retrieval. The configuration of the spectral bands varies but typically is centered at 0.34, 0.38, 0.44, 0.50, 0.67, 0.87, and 1.02 µm. Here we use a quadratic log-log fit (Eck et al., 1999) to interpolate AERONET AOD to 0.55 µm to match the AHI AOD product. The typical temporal frequency of direct sun measurements is every 15 min. The network consists of hundreds of stations, located globally, across all continents and in a wide variety of aerosol, meteorological, and surface type conditions. Here, we only include stations within the AHI view disk. AOD data from AERONET are reported for three different quality levels: unscreened (level 1.0), cloud screened (level 1.5), and cloud screened and quality assured (level 2.0). We will only use Version 3 Level 2.0 AERONET AODs in this study.
2.5 Comparison with AERONET and MODIS DT
The new AHI DT algorithm was applied to AHI-measured radiances over the full disk (except for extreme viewing and solar angles), daily, through the nine measurement times (hourly: 00:00 to 08:00 UTC). We will test this new product by first validating it against collocated AERONET measurements and then comparing it with the well-vetted MODIS DT product.
2.6 Validation against collocated AERONET AOD
The validation procedure requires calculation of the spatiotemporal statistics of a collocated AHI-retrieved and AERONET-measured AOD pair (Ichoku et al., 2002; Petrenko et al., 2012; Munchak et al., 2013; Remer et al., 2013; Gupta et al., 2018). Thus, the temporal mean AOD of all AERONET AOD measurements within ± 30 min of an AHI scan will be compared with the spatial mean of all Level 2 AHI-retrieved AOD values within a 0.25∘ × 0.25∘ box centered at the AERONET station. This method of matching spatiotemporal statistics, in one form or another, has become a standard within the aerosol remote sensing community (Levy et al., 2010; Petrenko et al., 2012; Remer et al., 2013; Huang et al., 2016; Gupta et al., 2018). As new satellite aerosol product types have been introduced, the specifics of the spatiotemporal matchups have been re-evaluated. For example for the DT MODIS 3 km product different temporal and spatial averaging windows were investigated, with smaller windows chosen to better test the ability of the finer-resolution product to capture spatial gradients at less than 10 km scales (Remer et al., 2013). As the DT geosynchronous products mature, we will conduct a similar investigation into better ways to validate the ability of the new products to represent the immediate diurnal cycle of the AOD at an AERONET station. For now, our purpose is to see if the product from the ported algorithm can match AERONET at a very basic level, and we will use the standard matchup procedure at traditional scales. The validation exercise with AERONET only considers AHI AODs pairs with the highest-quality AHI retrievals.
From the collection of these ordered pairs of collocated AHI and AERONET AODs a set of correlation and regression statistics will be calculated, assuming that the AERONET AODs are the independent variables and the AHI AODs are the dependent variables. These include the number of AOD pairs (N), the correlation coefficient (R), the slope (m), the intercept (I) of the linear regression through the points, and the overall mean bias and root-mean-square error (RMSE) of the AHI AODs. Also, we apply the expected error (EE), based on previous validation of MODIS DT AODs against collocated AERONET (Levy et al., 2013). We show the percentage of AHI AODs that fall within the EE bounds. EE gives us a sense of whether a new product is meeting the standards of the original product, which in itself has become a standard within the aerosol remote sensing community. Another metric that could be used would be the Global Climate Observing System (GCOS) criteria for AOD, which is 0.03 or 10 %. This is a more stringent requirement than what we have been able to achieve with the DT algorithm applied to MODIS for 20 years or to VIIRS. Thus, the GCOS requirement is not shown on the validation plots, as it is certainly out of reach for this first test of DT applied to a GEO sensor.
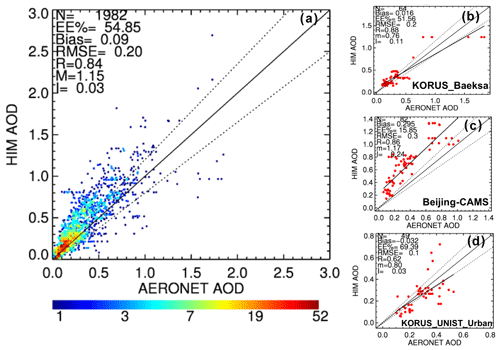
Figure 2Density scatterplots of retrieved AOD at 550 nm derived from AHI radiances using the new DT AHI algorithm versus AOD at 550 nm spectrally interpolated from measured AODs from AERONET instruments collocated in time and space. (a) All accumulated collocations in the AHI full-disk domain over the 2-month study period May–June 2016. Panels (b), (c), and (d) are the same for individual stations KORUS-Baeksa and KORUS-UNIST_Ulsan in Korea and Beijing CAMS in China. Shown in each panel are the number of collocations (N), percent within expected error as determined from MODIS DT analysis (EE %), mean bias (Bias), root-mean-square error (RMSE), correlation coefficient (R), slope (m), and intercept (I) of a linear regression equation through the points.
Figure 2 shows the results of this validation for the overland retrieval, with Fig. 2a showing the scatterplot of the accumulation of all collocations for the duration of the time period investigated and also specific panels showing the same but for individual AERONET sites. The specific stations were chosen to represent three different validation situations: when DT is biased high, biased low, and unbiased against AERONET. Figure 3 shows the validation statistics calculated for each AERONET location within the AHI domain. Altogether there were 1982 collocations during the period of the study, with a dynamic range spanning AERONET-measured AOD from less than 0.05 to nearly 2. The AHI AODs match AERONET observations with a correlation coefficient of 0.84, a mean bias of 0.09, and a RMSE of 0.20. Approximately 55 % of the retrievals fall within the EE that was based on MODIS validation. Figure 3 shows that the distribution of validation statistics varies from station to station but that correlations tend to be overall high across mainland Asia, while biases, RMSE, and percent within MODIS DT expected error vary more widely, even within tightly packed local networks. The variability in AHI AOD performance against AERONET over the domains is due to various reasons, including variations in surface reflectance characterization (i.e., different type of land use type), variability in assumed aerosol models within the algorithm, and availability of high-quality valid AOD retrievals over individual stations. Often AOD is biased high when surface reflectance ratios do not conform to assumptions. Such was the case for many years with urban surfaces, until Collection 6.1 made an alteration (Gupta et al., 2016). Even with that alteration, DT retrievals over Beijing continue to be high (Fig. 4). Low biases will occur when the assumed aerosol model underrepresents the amount of light absorption of the particles. The land aerosol model used in this region in this season is the moderately absorbing aerosol model in May and the non-absorbing model in June. If the aerosols are actually absorbing in June or more heavily absorbing in May in a particular locality, such as at KORUS_UNIST_Ulsan, then the retrieved AOD will be biased low. The DT algorithm is designed for global-scale representation of the aerosol system, which for GEO means full-disk retrievals. The goal is to provide the most accurate retrieval at each individual location, but the reality is that on the global scale we cannot fine-tune land surface and aerosol model assumptions for each individual location, and some locations will have products that are biased high and some biased low. The difficulty in matching AERONET at individual stations is one of the limitations of the current DT algorithm.
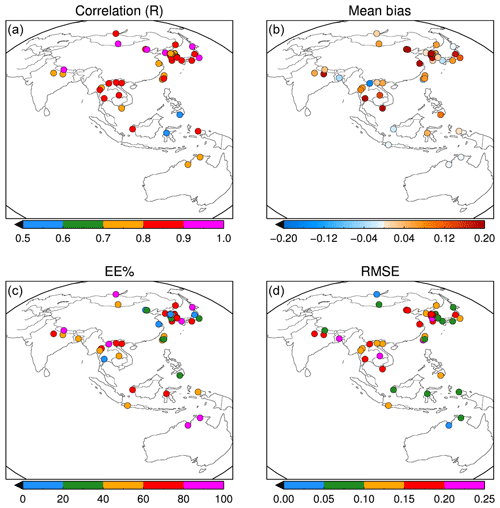
Figure 3Spatial distribution of the collocation statistics between retrieved AOD at 550 nm derived from AHI radiances using the new DT AHI algorithm versus AOD at 550 nm spectrally interpolated from measured AODs from AERONET instruments collocated in time and space. (a) Correlation (R); (b) mean bias; (c) percentage within expected error (EE %); (d) RMSE. Each point represents an AERONET station location.
As a comparison, Fig. 4 shows a similar set of plots but for MODIS DT retrievals against AERONET. These collocations were made at the same stations as in Fig. 2 and over the same time period. Both Terra and Aqua are included. First, we see about half as many points as were seen in the AHI collocations because Terra and Aqua MODIS each pass over the area only once per day during daylight hours, while AHI scans these sites up to nine times per day. Second, we notice that MODIS AODs match collocated AERONET AODs about the same as AHI AODs with R=0.91, bias = 0.10, RMSE = 0.19, and with 55 % within EE. Only the correlation between MODIS and AERONET is substantially better than between AHI and AERONET.
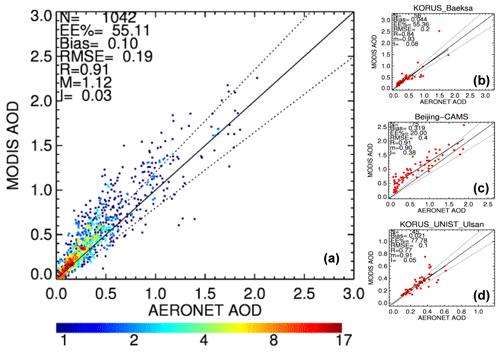
Figure 4Same as Fig. 2 except for density scatterplots of retrieved AOD at 550 nm derived from Terra and Aqua MODIS using the operational DT Collection 6.1 algorithm. These data represent same stations and time period as shown in Fig. 2.
We see from this limited validation that the AHI-retrieved AOD is sufficiently accurate to represent the aerosol in this region, during this time period, approaching the same validation statistics as the durable MODIS product. We note here that approaching the same validation statistics as the MODIS product will still fall short of the more stringent GCOS criteria. Additional analysis of AHI AOD biases as a function of surface reflectance, aerosol typing, season, and sensor and satellite geometry required data covering a longer time period. We plan to perform a more robust analysis in our ongoing and future research before making the product operational. We will next compare the full overlap of AHI-retrieved AOD with MODIS retrievals, regardless of AERONET.
2.7 AHI versus MODIS DT
To collocate AHI and MODIS AOD, the Level 2 MODIS and AHI AOD data were mapped to a common 0.25∘ latitude by 0.25∘ longitude grid for each AHI full-disk scan. To fill the grid, we include all MODIS retrievals within ±30 min of the AHI scan. All the AOD retrievals falling within the above spatial and temporal windows were averaged and statistics are retained for further analysis. It takes MODIS approximately 35–45 min to cut a poleward-to-poleward swath across an AHI image and about six to seven swaths to transverse east–west across the disk. Thus, in the common grid, at any particular time, while most of the grid has the possibility to include an AHI retrieval when cloud and glint free, only a relatively small portion of the grid will be filled with MODIS retrievals to create the possibility of a collocation.
Figure 5 presents the scatter plots from matching the products of the Terra–Aqua and AHI sensors on the common grid in each subset. Terra and Aqua collocations are kept separate, as are overland and over-ocean retrievals. The DT AHI-retrieved AOD and the DT MODIS-retrieved AOD exhibit excellent correlation and similarity, as is expected from applying nearly the same retrieval algorithm to the radiance measurements of both sensors. Over ocean there are over 600 000 matchups for Terra and over 1 million for Aqua. The geosynchronous AHI retrievals match the polar-orbiting MODIS retrievals with essentially zero bias and RMSE of 0.05 or less. Correlation between the two data sets is 0.93 or greater. Over land, there are over 100 000 matchups for each satellite with no bias for Terra and 0.02 for Aqua and a RMSE of 0.09 or less. Correlations exceed 0.95 over dynamic ranges from 0.0 to approximately 2.0. The plots in Fig. 5 show how well the new AHI-retrieved AOD matches its MODIS counterpart when both AHI and MODIS offer retrievals for a particular time and location. These plots do not address situations in which a retrieval occurs for one satellite, but not the other, and therefore do not address typical retrieval issues such as cloud masking and choosing appropriate situations for the DT algorithm to make a retrieval. There can also be differences in AODs from two sensors due to differences in their viewing geometries. This is something beyond the scope of this paper and will be addressed in subsequent research.
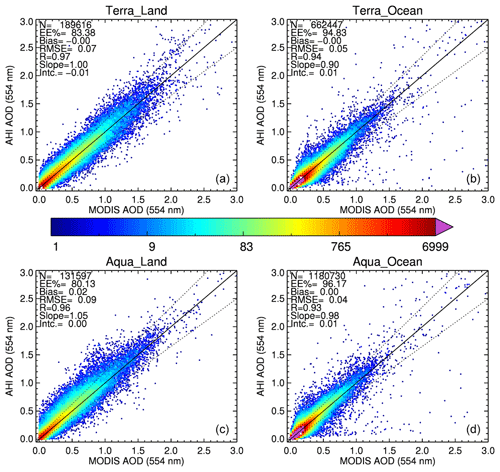
Figure 5Density scatterplots of retrieved AOD at 554 nm derived from AHI radiances using the new DT AHI algorithm versus retrieved AOD at 554 nm from the operational MODIS Collection 6.1 algorithm, collocated in time and space. (a, b) Terra MODIS. (c, d) Aqua MODIS. Panels (a, c) show results from the overland retrieval. Panels (b, d) show results from the over-ocean retrieval. The same collocation statistics are displayed as in Fig. 2.
Figure 6 shows the 2-month mean AOD over the AHI disk from AHI and Aqua MODIS, calculated from the data mapped to the common grid during our study period. The mean AODs plotted here are collocated and represent the AHI-derived AOD at approximately the same time as the MODIS overpass.
We see that the DT algorithm applied to both sensors results in very similar distributions of mean AOD across the AHI full-disk image (Fig. 6). This is despite the different sensor characteristics and very different viewing geometries. There is elevated aerosol across south and southeast Asia and a separate pocket of elevated aerosol in northeast China. Low AOD occurs across most of the tropical and southern oceans. Australia is very clean and both sensors show a bit of moderately elevated AODs over the Indonesian island of Java. The magnitude of mean AOD in these images ranges from near 0.0 to almost 1.0.
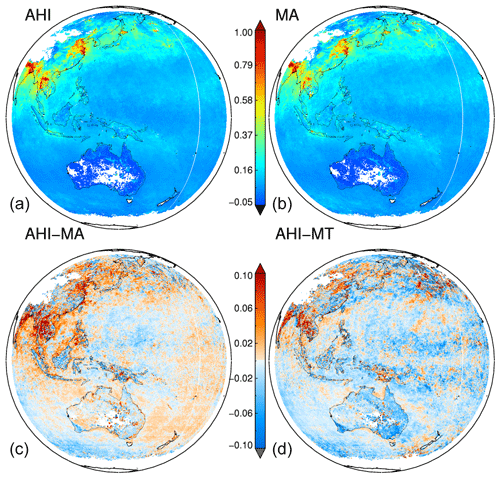
Figure 6(a, b) Mean AOD at 550 nm over the 2-month study period of May–June 2016. (a) Mean AOD derived from retrievals using the new DT AHI algorithm applied to AHI. (d) Mean AOD derived from the standard Aqua MODIS DT product. (c, d) Difference maps of mean AOD at 550. (c) Difference between the two maps in (a, b). (b) Similar difference map but between AOD from AHI and AOD from Terra MODIS (MT), instead of Aqua MODIS (MA).
Figure 6c and d show the absolute differences in AOD when subtracting the MODIS panel (b) from the AHI panel (a) and a similar difference map showing the differences between the AHI panel (a) values and a similar MODIS plot but from the Terra satellite. The difference maps are AHI minus MODIS so that positive values, in red, indicate that AHI is higher than MODIS, while the negative values, in blue, indicate that AHI is lower than MODIS. The range of differences spans +0.10 to about −0.08.
These plots indicate that over the elevated AOD regions, AHI retrievals are higher than MODIS retrievals by as much as 0.10. This higher AHI AOD is more prevalent and widespread with MODIS Aqua than with MODIS Terra. AHI tends to be about 0.02 to 0.03 higher than MODIS Aqua over much of the ocean regions surrounding the Asian and maritime continents, while AHI tends to be closer to MODIS Terra in these regions and sometimes even negative. Over Australia, AHI is less than MODIS Terra by as much as −0.08. Because AOD values over Australia are very low to begin with, this negative with respect to MODIS Terra indicates that AHI retrievals over Australia are often absolutely negative more consistently than the MODIS retrievals and suggest that some adjustment to the surface parameterization in the AHI DT retrieval will be required.
The inconsistencies between the two difference maps, one showing AHI with respect to MODIS Aqua and the other with respect to MODIS Terra, highlight the difficulty in producing consistent representations of the AOD field, even when applying the same algorithm to different sensors that should be exact duplicates of each other as in the case of MODIS Terra and MODIS Aqua (Levy et al., 2018). Given this inconsistency between the two MODIS instruments, the differences between AHI results and both MODIS instruments fall within expected and manageable ranges. The DT algorithm as applied to AHI produces a representation of the spatial distribution of AOD with the same level of fidelity as the original DT MODIS algorithm. This is the first attempt to apply the DT algorithm to AHI, and we expect that future refinements to algorithm assumptions that account for specific instrument characteristics and calibration will bring AHI AOD results even closer to MODIS and AERONET values of AOD.
3.1 Comparing AHI-derived diurnal signatures with AERONET
In the previous section we show how well the new DT AHI algorithm matches the AOD measurements from AERONET and the retrievals from MODIS. However, the point of applying an aerosol retrieval algorithm to a geosynchronous satellite sensor such as AHI is not to match the individual station data of AERONET nor the once-per-day retrievals from MODIS. The point of porting the DT aerosol algorithm to AHI is to represent the diurnal cycle of AOD over the broad regional area covered by the AHI full disk. In this section we explore the diurnal cycle of AOD derived from AHI and evaluate how much of the aerosol system MODIS has been missing because of its limited temporal sampling.
The diurnal cycle of AHI-derived AOD is compared with collocated diurnal patterns of AOD exhibited by AERONET stations within the AHI full-disk image. The diurnal cycle at each AERONET station was calculated by finding the mean AERONET AOD at seven specific times of the day corresponding to the time of an AHI scan. These times are 01:00, 02:00, 03:00, 04:00, 05:00, 06:00, and 07:00 UTC, corresponding to the hours of 10:00 to 16:00 in local Korean time. All AERONET AOD measurements ±30 min of the nominal time were included in the average to represent the mean AOD at the nominal time. In parallel, the mean AODs at these specific times were calculated from all high-quality AHI-derived level 2 AOD located within a 0.25∘ × 0.25∘ box centered around the AERONET station for all AHI scans taken at the nominal time. Thus, we created two representations of the diurnal cycle of AOD at each AERONET station, one from AERONET data and one from AHI-derived data, all from the collocation data set. This means that both AERONET and AHI must report at the same specific time for the instruments' AOD to be included in the calculated hourly average. This is the purest means to compare the actual retrieval, but will not reveal differences in sampling factors such as cloud masking because AHI will benefit from AERONET's cloud identification.
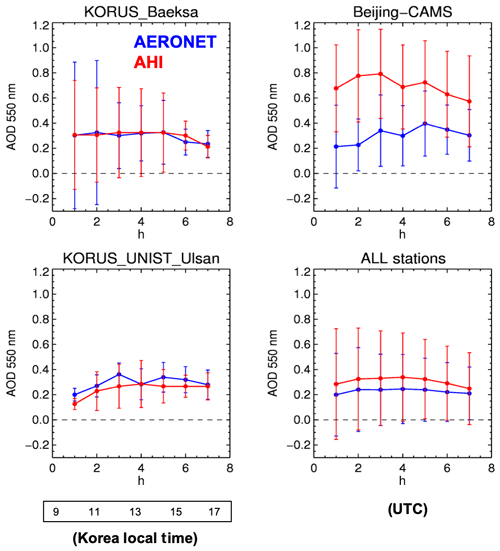
Figure 7Median AOD at 550 nm in each of seven time-of-day bins corresponding to 10:00 to 16:00 Korean standard time. Shown are three individual AERONET stations and also (lower right) the results of binning all of the AERONET stations across the full-disk image as shown in Fig. 3. Red indicates AOD derived from AHI using the new DT algorithm. Blue indicates AOD measured by AERONET. The statistics were calculated from the collocation database such that each bin contains the same number of observations from AHI and AERONET taken at the same time, although the number of observations in each diurnal bin will differ. Vertical error bars represent 1 standard deviation among different days for the same hour.
Figure 7 shows the calculated median AOD diurnal cycles from AERONET and AHI retrievals for three individual stations in Korea and China and also the median of all stations located in the AHI full-disk image and reporting during our period of study. Error bars represent the standard deviation of the sample in each hourly bin. At the three stations shown individually in Fig. 7, we see that the same biases seen in Fig. 2 also appear here, particularly with Beijing CAMS showing a strong positive bias. There is wide scatter in the AOD for each hourly bin, as portrayed by the relatively large error bars. The diurnal pattern of AOD, as measured by AERONET at KORUS Baeksa shows a sudden decrease after 05:00 UTC (14:00 Korean standard time), dropping from a steady 0.3 to 0.2 in 2 h. The AHI AOD retrievals match this pattern almost exactly. The other Korean station, KORUS_UNIST_Ulsan, shows an opposite daily pattern, with AOD increasing from a morning low of 0.2 at 01:00 UTC (10:00 Korean standard time) to 0.3–0.4 at midday and then a drop-off towards evening. The AHI AOD at this station is biased low throughout most of the day, but it does reflect the same diurnal signature of increasing AOD over the morning. At the third station, Beijing CAMS, the AHI AOD diurnal pattern does not match AERONET as well, but there is a strong positive bias there with very large scatter in each hour. With error bars spanning 0.5 AOD, it is difficult to discern diurnal changes with amplitudes of 0.2 AOD or less in either AERONET or AHI.
The diurnal analysis shown in Fig. 7 suffers from relatively small data samples. The number of collocations for KORUS_Baeksa, KORUS_UNIST_Ulsan, and Beijing CAMS are 56, 45, and 75, respectively, distributed over 7-hourly bins. If clouds were not a factor, each hourly bin median might be constructed from only 6 to 11 samples. However, clouds are indeed a factor, with their own diurnal patterns. The actual number of AHI–AERONET collocations at any particular hour might be as few as three, and morning and afternoon bins reported in Fig. 7 might be constructed from entirely different days. Therefore, the diurnal patterns in Fig. 7 may be artificial composites and not representative of the actual changes in AOD over the course of a single day. However, the point of this comparison is not to speculate on the cause of the diurnal signatures but to establish that the AHI-derived AOD has the ability to describe the same mean diurnal pattern in the aerosol as AERONET for individual locations.
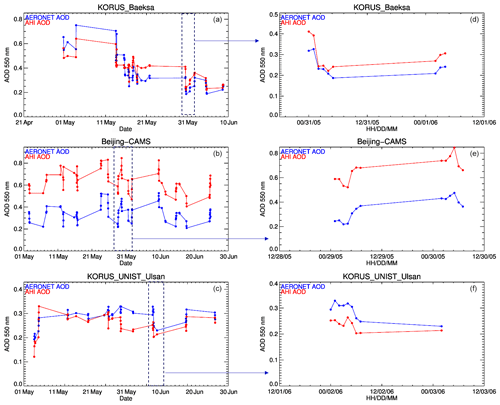
Figure 8The time series of spatiotemporal mean AODs from AERONET (blue) and AHI (red) for each hour of observation during the KORUS-AQ field campaign for the same three stations as shown in Fig. 7 (a–c). (d–f) Zoom of selected days as shown in the box with dotted lines in the left panels for each station.
Figure 8 further demonstrates the capability of AHI-retrieved AODs to represent realistic diurnal cycles over these three stations on individual days rather than in an average sense as shown in Fig. 7. This analysis shows that AHI-retrieved AODs follows AERONET AODs hour by hour and day by day with apparent positive and negative biases over different stations as discussed in the earlier section. Additional KORUS-AQ time series of AHI and AERONET AOD for 46 other stations are shown in the Supplement. While there are some stations where AHI AOD does not follow the AERONET temporal variability as well as those shown in Fig. 8, most do.
The ensemble statistics of the diurnal signature for all AERONET stations and collocated AHI retrievals in the AHI full-disk image show the high bias of the AHI retrievals, as per Fig. 2, but also that the ensemble mean diurnal signature of AHI AOD is mostly flat, as is the diurnal signature from AERONET. Both AHI and AERONET AOD exhibit a slight increase in AOD from morning to midday. Then, AHI decreases towards the end of the day, while AERONET stays flat. The scatter in each hourly bin is large, as shown by error bars that span 0.6 in AOD, and thus diurnal patterns with amplitudes of 0.1, exhibited by both AHI and AERONET, fall well below a significant signal-to-noise threshold. Still the basic agreement of AHI with AERONET in the overall ensemble diurnal statistics and in the individual time series comparisons is encouraging.
3.2 Full-disk AHI-derived AOD diurnal cycle
Previously, Fig. 6 showed the mean full-disk AHI AOD calculated for the approximate times of the MODIS overpasses. Now we calculate the overall mean AHI-derived AOD calculated over the entire daylight diurnal cycle and not just at MODIS overpass time, for the duration of our study period at each of the 0.25∘ latitude by 0.25∘ longitude grid squares. Figure 9 shows this overall period mean map, with all diurnal information lost. The period mean map at MODIS overpass time (Fig. 6) looks qualitatively very similar to the overall period mean map (Fig. 9), suggesting that MODIS sampling provides a good representation of the overall AOD distribution.
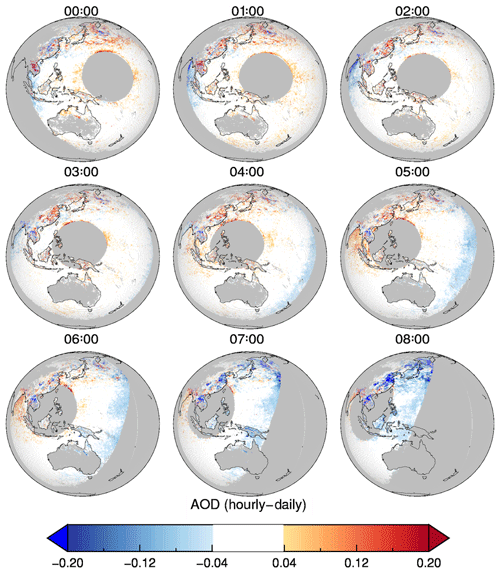
Figure 10Difference in hourly mean AOD at 550 nm as derived from the new DT AHI algorithm from the daily mean AOD, as plotted in Fig. 8. Red indicates the specific hour has higher AOD than the daily mean, and blue indicates the opposite.
Then we calculate the mean AOD for each AHI full-disk scan corresponding to a particular UTC hour, in each grid over the period of our study. Figure 10 shows the plots of the absolute difference (mean hourly AOD minus mean daily AOD) at each of these diurnal hours.
Figure 10 captures the diurnal signature of the aerosol over a broad region of Earth. Red colors indicate that at a particular hour of the day, the AOD is higher than the daily mean. Blue colors indicate that the hourly AOD is lower than the daily mean. The large gray circle that traverses the image from hour to hour is the glint mask preventing the over-ocean algorithm from retrieving an AOD value. The glint mask is set for glint angles , which unfortunately eliminates large portions of a geosynchronous image from being suitable for a DT aerosol retrieval. The glint mask proceeds across the image hour by hour so that the glint mask becomes indiscernible in the daily mean. That is why there is no apparent glint mask in the overall daily average of Fig. 9, nor in Fig. 6 constructed from AHI AOD collocated with MODIS. Continents and islands within the glint mask will call on the overland DT algorithm that does not mask for glint, and therefore, will return an AOD value.
The most striking feature in Fig. 10 is the blue shading at the edges of the over-ocean retrieval domains that begin the day to the west in the Indian Ocean and then switch to the east in the Pacific in the afternoon. This band of “lower than daily average” AOD is associated with solar zenith angle, not view angle, as it hugs the day–night terminator in the images, even when that terminator crosses the center of the full-disk image. By 07:00 and 08:00 UTC, the terminator artifact encompasses a broad geographical swath of ocean, which would introduce an incorrect interpretation of local diurnal AOD signal with amplitudes of 0.15, when daily mean values are only 0.10. Such strong diurnal swings in AOD over the remote ocean on global scales are unrealistic.
The problem may be introduced by the radiative transfer code used to create the lookup tables for the over-ocean retrieval (Ahmad and Fraser, 1982) that does not fully account for Earth's curvature. Although this code has served the DT retrieval well through the MODIS and VIIRS eras, those polar-orbiting satellites only encounter extreme solar zenith angles at the beginning and end of their orbits near the poles, where DT aerosol retrievals are rare due to other factors such as extreme cloudiness or snow and ice. The inability to properly model Earth's sphericity is likely to be of greater concern for geosynchronous satellites that encounter extreme solar zenith angles across all latitudes and in prime retrieval areas. See Fig. 11. Currently the AHI DT algorithm retrieves all geometries with a solar zenith angle <80∘. Figures 10 and 11 suggest that the terminator artifact could be mitigated by applying a more stringent threshold of 70∘. However, development and application of a spherical radiative transfer code is the more satisfying long-term solution.
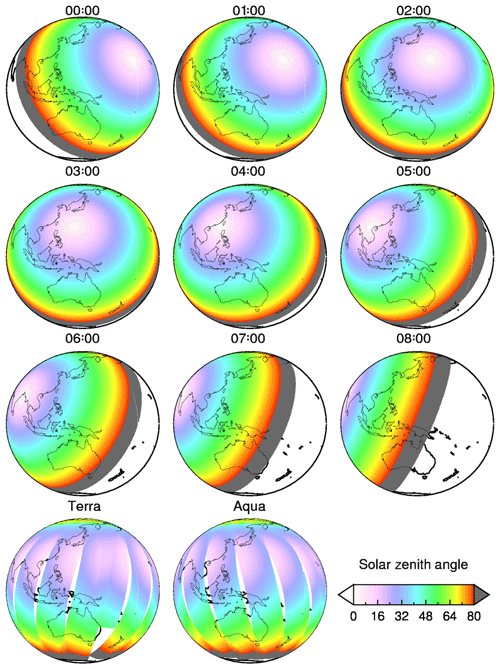
Figure 11Mean solar zenith angle associated with each of the diurnal hours from the AHI geometry and also for MODIS on Terra and Aqua for 29 May 2016.
There also appears to be another AOD retrieval artifact over the ocean associated with the glint angle. Here AODs seem artificially high. Incorrect estimation of wind speed from ancillary data or modeling of the rough ocean surface will introduce near-glint mask inaccuracies in the AOD retrieval. With MODIS, such areas were relatively small and the overall effect on global or regional AOD minimal. In the geosynchronous view, because the glint mask is such a dominant feature, the near-glint artifacts appear much more pronounced.
The good news seen in Fig. 10 is that the retrieval over land does not appear to have encountered any systematic artifacts. Blue and red shading is distributed across the Asian, Indonesian, and Australian landmasses. Without validation we cannot say for sure, but typically local factors determine aerosol diurnal trends, and thus the spotty blue and red shading could indicate that the retrieved AOD represents the consequences of these local diurnal forcing mechanisms. We have already seen in Figs. 7 and 8 that the AHI retrievals resolved the differing local diurnal patterns at three overland AERONET stations within relative close proximity. In terms of the overland retrieval, Fig. 10 demonstrates that the DT algorithm applied to AHI will identify land regions where the diurnal signal is more spatially cohesive. For example the east coast of India and Bangladesh experience an increase in AOD in the late afternoon, while the overall trend in northeast China is to decrease AOD in the afternoon, although there are local contradictions to these regional patterns.
3.3 AHI-derived AOD diurnal cycle over 5∘ squares
The factors that drive a diurnal AOD signature tend to be local in character. These include sources and sinks linked to time of day (rush-hour traffic, agricultural burning, afternoon convection/precipitation) or diurnally influenced mesoscale circulations and transport (sea breeze or mountain slope regimes). Thus, individual stations as shown in Fig. 7 exhibit stronger diurnal signatures than an ensemble average consisting of stations distributed across the region does (bottom right panel of Fig. 7). The full-disk plots of Fig. 10 suggest that there are regions of moderate extent that do experience a cohesive diurnal AOD pattern. To further investigate the ability of the DT AHI to provide insight into diurnal patterns of AOD during daylight hours, we calculate the average AOD in specific 5∘ latitude by 5∘ longitude boxes as a function of the hour of the day.
Figure 12 shows the diurnal AOD signatures of five of these 5∘ by 5∘ boxes. As suggested by Fig. 10, the AOD over northeastern China (Fig. 12, Box 1) exhibits its highest AOD during morning hours, 00:00 to 03:00 UTC, corresponding to local times of 08:00 to 11:00, and then experiences a slow decrease during the remainder of the day until sunset. Average mean AOD at 550 nm in this area ranges from morning values of 0.65 to late afternoon values of less than 0.40. Over Bangladesh (Fig. 12, Box 2) the glint mask does not interrupt ocean retrievals until the last diurnal hour of the day. Ocean and land retrievals exhibit very similar diurnal signatures in this area, slowly rising from morning lows of 0.3–0.4 to late afternoon highs of 0.8–0.9, at least over land. Another area containing both land and ocean retrievals is over northern Japan and the adjacent Pacific Ocean (Fig. 12, Box 3). This area is far enough north to not be hampered by the glint mask at this time of year. The over-ocean and overland diurnal patterns are similar with morning to midday values of 0.30–0.35 gradually decreasing through the afternoon to lows of 0.15 by sunset. This is a significant diurnal range of AOD over ocean.
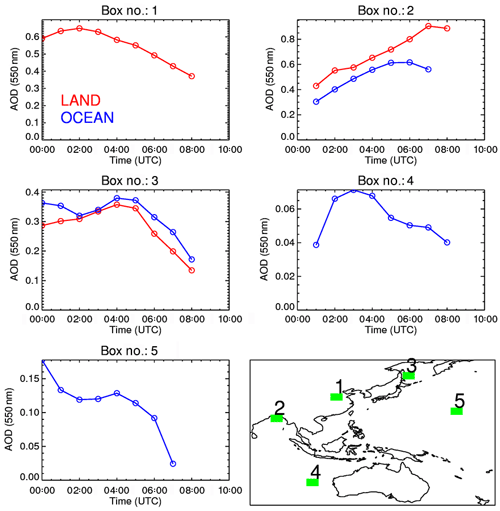
Figure 12Spatially averaged mean AOD at 550 nm from the derived DT AHI product for selected 5∘ by 5∘ latitude–longitude squares (boxes) in each hourly bin for the 2-month study period, producing AOD diurnal signatures for these selected areas. Red lines depict overland retrievals. Blue lines depict over-ocean retrievals. The x axes are in UTC hours, for the reference; the local time in Beijing is +8 h from UTC. The y axis scale varies from plot to plot. The green squares on the global map indicate location of the specific box.
Two areas over open ocean are shown in Fig. 12, one in the Indian Ocean west of Australia (Fig. 12, Box 4) and the other in the Pacific (Fig. 12, Box 5). Note that the scales on the y axes of these two plots are different. At the Indian Ocean area there appears to be a diurnal signal, but the amplitude of that signal is only about 0.02, well within the noise levels of both the retrieval itself and the sampling and statistics of calculating the diurnal pattern. Essentially there is no significant diurnal signal at this location and the mean AOD is about 0.05±0.01. In contrast the Pacific example exhibits a strong diurnal pattern, ranging almost an order of magnitude from 0.18 (∼0.2) to 0.02. It is in this area that the two ocean artifacts become apparent. During the early morning hours this area resides just north of the sun glint mask where insufficient modeling of the rough ocean surface creates an artifact in the retrieval, introducing a high bias. During late afternoon hours, the solar zenith angle increases to beyond 70∘ and the low bias artifact from the terminator affects the retrieval. It is only midday when this Pacific region escapes this artifact, and then we see little diurnal signature and a mean AOD of 0.11±0.015. Thus, the apparently strong diurnal signal here is in reality just the combination of two different artifacts in the retrieval.
The examples in Fig. 12 illustrate the variety of aerosol diurnal patterns over Asia with polluted regions like northeastern China and Bangladesh showing diurnal amplitudes of 0.25–0.50 in AOD, but with oppositely signed slopes. The need to understand and explain these different patterns across an area as large as Asia opens new research questions as to what the driving processes behind these AOD patterns are, how they will affect assimilation into global and regional models, and what the air quality and public health implications are. While the processes creating diurnal aerosol patterns are primarily local, the consequences of spatially cohesive patterns will have nonlocal consequences, and aerosol products from geosynchronous observations, such as the AHI DT product, are key to identifying and quantifying these spatially cohesive situations. The patterns seen in Fig. 12 may also suffer from the caveats imposed upon the individual station analysis of Fig. 7. The diurnal patterns may be artificial constructs of observations made at different times on different days and not represent the true change of aerosol loading over the span of daylight hours. However, because of the greater statistical sample offered by the larger spatial domain of the box there is greater confidence in the patterns of Fig. 12 than those of Fig. 7.
The examples in Fig. 12 also illustrate that artifacts still exist in the retrieval over the ocean, but that not all strong diurnal signatures over ocean are due to the artifacts, as shown in Fig. 12d where the ocean pattern mimics the artifact-free land pattern. Being aware of the possibility of artifacts and working towards mitigating those artifacts in the future will be essential to properly making use of any new geosynchronous product.
The traditional Dark Target (DT) aerosol retrieval algorithm was adapted for the Advanced Himawari Imager (AHI) and applied to AHI-measured spectral reflectances produced for a limited data set in support of KORUS-AQ for the 2-month period of May–June 2016. The adaptation makes use of the spectral similarity between AHI and its predecessor DT sensors (e.g., MODIS, VIIRS), but it omits certain important pixel selection procedures that require spectral bands unavailable from AHI. The lack of these specific masks may permit additional cirrus and cloud contamination in the results of this 2-month preliminary demonstration, although large-scale comparisons of collocated AHI and AERONET or AHI and MODIS retrievals do not reveal significant overall biases. However, AHI retrievals may be benefitting from AERONET or MODIS cloud masking in the collocations. Expanding the AHI retrieval into the winter months when snow and ice will be encountered will then certainly show contamination from such surfaces, as the current DT snow and ice mask requires the 1.24 µm channel that is missing from AHI. Before wintertime retrievals are made with AHI, a new cloud–ice mask for this sensor must be developed.
Collocations between AHI and AERONET demonstrate that AHI retrievals match AOD_550 nm at AERONET stations, and the MODIS DT aerosol product matches AERONET in terms of correlation, RMSE, overall bias, and percentage within expected error. Meeting previous MODIS DT validation criteria does not guarantee meeting the international standards set by GCOS, as those criteria are more stringent. Additionally, because AHI can make aerosol retrievals multiple times per day, there were approximately twice as many AHI–AERONET collocations as there were from MODIS–AERONET. Geostationary aerosol retrievals will significantly increase the sampling of retrieved AOD from current polar-orbiting sensors. Not only did the DT AHI product match AERONET, statistically, in scatterplots, it also represented the diurnal signal in AOD, as measured by AERONET at individual stations, and in the ensemble median statistics. The three stations shown are representative of varying retrieval biases and exhibit different diurnal signatures, even though they are in relatively close proximity. The AHI DT algorithm was able to distinguish these diurnal differences, although sample size was small and signal-to-noise ratio impeded inference of the diurnal signature.
Plotting the time series of the collocated data along the same axis shows that the AHI AOD matches the temporal variability of the AERONET AOD hour by hour, even when there is a strong bias in the magnitude. These time series plots are strong evidence that the DT retrieval algorithm applied to geosynchronous sensors such as AHI will be able to resolve short-duration events such as individual plumes when the algorithm moves to operational status.
Collocated AHI and MODIS retrievals demonstrate excellent agreement when applying the DT algorithm to the two different sensors. Both AHI and MODIS produce similar representations of the 2-month mean AOD across the AHI full-disk region. However, difference maps do show regional biases. Interestingly, AHI is overall biased low against MODIS Terra but biased high against MODIS Aqua and thus falls within the offsets already noted between the AODs of the two MODIS sensors (Levy et al., 2018). The one place that AHI differs in the same way from both MODIS Terra and MODIS Aqua is in its positive bias of 0.10 in the high-aerosol-loading regions of south, southeast, and northeast Asia. The fact that these biases are only seen in high aerosol loading suggests a problem with the traditional DT aerosol models, not the surface parameterization. We note that the overland aerosol models have never been tested for the unique geometry that AHI has brought to the table.
When the algorithm is applied to the full-disk image and hourly mean AOD plots are made, we notice immediately an artifact in the diurnal signature that affects only the over-ocean retrieval. This artifact occurs at the day–night terminator and is associated with extreme solar zenith angles, not view angles. Extreme solar zenith angles are much more prevalent in geosynchronous images than in polar-orbiting ones, and thus our previous experience with polar-orbiting sensors did not prepare us for this artifact. The most likely explanation for the solar zenith artifact is the inability of the original radiative transfer code to model spherical Earth. Earth's curvature when the sun is on the horizon will introduce uncertainties into the radiative transfer calculation and result in inaccurate aerosol retrievals. Until modifications can be made to the radiative transfer code, the solution to mitigating this artifact will be to limit retrievals to lower solar zenith angles over ocean (<70∘). This is unfortunate because already the retrieval loses a good section of the equatorial ocean because of the 40∘ glint mask when solar zenith angles are small. Because we also saw retrieval artifacts along the edge of the glint mask, it is unlikely that the 40∘ threshold can be relaxed. For now, the DT AHI retrieval over ocean should be limited to a small range of solar zenith angles that will avoid both the glint and the artifact at the terminator, and this will limit the diurnal range of AHI-retrieved AOD over ocean.
In a preliminary analysis meant to show the scientific potential of the AHI DT product, we found a balance between the local nature of diurnal signatures and the need of a substantial statistical sample by calculating the mean diurnal patterns of AOD in 5∘ latitude by 5∘ longitude boxes. The result of this analysis revealed a variety of diurnal patterns across Asia, as well as illustrating diurnal patterns of ocean areas affected and not affected by glint and solar zenith angle artifacts. A more mature AHI DT product will enable further exploration of these diurnal patterns and the consequences these patterns hold for climate processes, assimilation systems, and air quality.
To make progress towards a more mature algorithm beyond the preliminary version analyzed here, we will need to continue the analysis and investigate the following points.
-
What is the reason for the biases between AHI and both AERONET and MODIS?
-
Are these biases linked to solar zenith angle, view angle, or scattering angle?
-
Are these biases linked to surface parameterization, specifically change in surface ratios with viewing geometry?
-
Do we mitigate artifacts by employing a more realistic spherical radiative transfer code?
-
How do we mask for snow–ice without the 1.24 µm wavelength?
-
Can we characterize cloud and cirrus contamination in the retrievals and then mitigate those effects?
-
How does the retrieved AOD spectral dependence and size parameter from AHI compare to those from MODIS?
-
Can we surpass results obtained from the polar orbiting sensors by incorporating additional specific geosynchronous capabilities into the DT retrieval?
The short 2-month demonstration described and illustrated here is a preliminary assessment of the ability to bring the well-vetted DT aerosol retrieval to a geosynchronous satellite sensor. The results show that porting the algorithm is possible, that it can produce AOD that matches AERONET to the same degree as the MODIS product, and that it can distinguish local diurnal signatures at AERONET stations over land. The view from geosynchronous sensors will provide new insight into Earth's aerosol system, especially if that view is steeped in and compatible with the 20-year record of the DT polar-orbiting experience. This study puts us on the road to achieving this new perspective.
AERONET data used in this study are available from NASA’s AERONET data server and can be accessed from https://aeronet.gsfc.nasa.gov/data_push/V3/AOD/AOD_Level15_All_Points_V3.tar.gz (last access: 20 November 2019). Other data sets collected during the KORUS-AQ field campaign are also available from another NASA data center and can be accessed from https://www-air.larc.nasa.gov/cgi-bin/ArcView/korusaq?OTHER=1, last access: 28 November 2019. The Himawari-8 radiance and aerosol data sets used and processed in this study are not yet available in public domain due to their preliminary nature. Our team is working on making this algorithm operational, and in time all the data will be available in public domain through the NASA data center.
The supplement related to this article is available online at: https://doi.org/10.5194/amt-12-6557-2019-supplement.
PG performed the analyses, prepared the figures, and wrote sections of the paper. LAR advised the analysis, helped to organize the structure of the paper, wrote sections of the paper, and provided thorough edits. RCL is the PI of the MODIS DT project and led the research effort and provided suggestions and edits to the paper. SM is the lead programmer for the MODIS DT project; she imported and maintained the AHI algorithm products and ran the experiments during the KORUS-AQ campaign. REH and AKH provided the Himawari-8 CLARV-x data for retrieval and analysis.
The authors declare that they have no conflict of interest.
This work was supported by the NASA ROSES program NNH17ZDA001N: Making Earth System Data Records for Use in Research Environments and NASA's EOS program managed by Hal Maring. We thank the Space Science and Engineering Center (SSEC), University of Wisconsin-Madison for providing Himawari-8 data. We thank MCST for their efforts to maintain and improve the radiometric quality of MODIS data and LAADS/MODAPS for the continued processing of the MODIS products. The AERONET team (GSFC and site PIs) is thanked for the creation and continued stewardship of the sun photometer data record, which is available from http://aeronet.gsfc.nasa.gov (last access: 20 November 2019).
This research has been supported by the NASA (grant no. NNH17ZDA001N).
This paper was edited by Marloes Gutenstein-Penning de Vries and reviewed by Shobha Kondragunta and one anonymous referee.
Ahmad, Z. and Fraser, R.: An iterative radiative transfer code for ocean-atmosphere systems, J. Atmos. Sci., 32, 656–665, https://doi.org/10.1175/1520-0469(1982)039, 1982.
Boucher, O., Randall, D., Artaxo, P., Bretherton, C., Feingold, G., Forster, P., Kerminen, V.-M., Kondo, Y., Liao, H., Lohmann, U., Rasch, P., Satheesh, S. K., Sherwood, S., Stevens, B., and Zhang, X. Y.: Clouds and aerosols, in: Climate Change 2013: The Physical Science Basis, Contribution of Working Group I to the Fifth Assessment Report of the Intergovernmental Panel on Climate Change, edited by: Stocker, T. F., Qin, D., Plattner, G.-K., Tignor, M., Allen, S. K., Boschung, J., Nauels, A., Xia, Y., Bex, V., and Midgley, P. M., Cambridge University Press, Cambridge, United Kingdom and New York, NY, USA, 571–657, 2013.
Choi, M., Lim, H., Kim, J., Lee, S., Eck, T. F., Holben, B. N., Garay, M. J., Hyer, E. J., Saide, P. E., and Liu, H.: Validation, comparison, and integration of GOCI, AHI, MODIS, MISR, and VIIRS aerosol optical depth over East Asia during the 2016 KORUS-AQ campaign, Atmos. Meas. Tech., 12, 4619–4641, https://doi.org/10.5194/amt-12-4619-2019, 2019.
Diner, D. J., Beckert, J. C., Reilly, T. H., Bruegge, C. J., Conel, J. E., Kahn, R. A., Martonchik, J. V., Ackerman, T. P., Davies, R., Gerstl, S. A. W., Gordon, H. R., Muller, J.-P., Myneni, R. B., Sellers, P. J., Pinty, B., and Verstraete, M. M.: Multi-angle Imaging SpectroRadiometer (MISR) instrument description and experiment overview, IEEE T. Geosci. Remote, 36, 1072–1087, https://doi.org/10.1109/36.700992, 1998.
Diner, D. J., Abdou, W. A., Ackerman, T. P., Crean, K., Gordon, H. R., Kahn, R. A., Martonchik, J. V., McMuldroch, S., Paradise, S. R., Pinty, B., Verstraete, M. M., Wang, M., and West, R. A.: Multi-Angle Imaging SpectroRadiometer Level 2 Aerosol Retrieval Algorithm Theoretical Basis, Revision G, JPL D-11400, Jet Propulsion Laboratory, California Institute of Technology, Pasadena, USA, 2008.
Eck, T. F., Holben, B. N., Reid, J. S., Dubovik, O., Smirnov, A., O'Neill, N. T., Slutsker, I., and Kinne, S., Wavelength dependence of the optical depth of biomass burning, urban, and desert dust aerosols, J. Geophys. Res.-Atmos., 104, 31333–31349, https://doi.org/10.1029/1999JD900923, 1999.
Frey, R. A., Ackerman, S. A., Liu, Y., Strabala, K. I., Zhang, H., Key, J. R., and Wang, X.: Cloud detection with MODIS. Part I: Improvements in the MODIS cloud mask for collection 5, J. Atmos. Ocean. Tech., 25, 1057–1072, 2008.
Gupta, P., Levy, R. C., Mattoo, S., Remer, L. A., and Munchak, L. A.: A surface reflectance scheme for retrieving aerosol optical depth over urban surfaces in MODIS Dark Target retrieval algorithm, Atmos. Meas. Tech., 9, 3293–3308, https://doi.org/10.5194/amt-9-3293-2016, 2016.
Gupta, P., Remer, L. A., Levy, R. C., and Mattoo, S.: Validation of MODIS 3 km land aerosol optical depth from NASA's EOS Terra and Aqua missions, Atmos. Meas. Tech., 11, 3145–3159, https://doi.org/10.5194/amt-11-3145-2018, 2018.
Hsu, N. C., Tsay, S. C., King, M. D., and Herman, J. R.: Aerosol properties over bright-reflecting source regions, IEEE T. Geosci. Remote, 42, 557–569, https://doi.org/10.1109/TGRS.2004.824067, 2004.
Hsu, N. C., Jeong, M.-J., Bettenhausen, C., Sayer, A. M., Hansell, R., Seftor, C. S., Huang, J., and Tsay, S.-C.: Enhanced Deep Blue aerosol retrieval algorithm: The second generation, J. Geophys. Res.-Atmos., 118, 9296–9315, https://doi.org/10.1002/jgrd.50712, 2013.
Ichoku, C., Chu, D. A., Mattoo, S., Kaufman, Y. J., Remer, L. A., Tanre, D., Slutsker, I., and Holben, B. N.: A spatio-temporal approach for global validation and analysis of MODIS aerosol products, Geophys. Res. Lett., 29, 1616, https://doi.org/10.1029/2001GL013205, 2002.
Jickells, T., An, Z., Andersen, K. K., Baker, A., Bergametti, G., Brooks, N., Cao, J., Boyd, P., Duce, R., and Hunter, K.: Global iron connections between desert dust, ocean biogeochemistry, and climate, Science, 308, 67–71, https://doi.org/10.1126/science.1105959, 2005.
Kahn, R. A., Gaitley, B. J., Garay, M. J., Diner, D. J., Eck, T., Smirnov, A., and Holben, B. N.: Multiangle Imaging SpectroRadiometer global aerosol product assessment by comparison with the Aerosol Robotic Network, J. Geophys. Res., 115, D23209, https://doi.org/10.1029/2010JD014601, 2010.
Kahn, R. A. and Gaitley, B. J.: An analysis of global aerosol type as retrieved by MISR, J. Geophys. Res.-Atmos., 120, 4248–4281, https://doi.org/10.1002/2015JD02332, 2015.
Kalashnikova, O. V. and Kahn, R.: Ability of multiangle remote sensing observations to identify and distinguish mineral dust types: Part 2, Sensitivity over dark water, J. Geophys. Res.-Atmos., 111, D11207, https://doi.org/10.1029/2005JD00675, 2006.
Kalluri, S., Alcala, C., Carr, J., Griffith, P., Lebair, W., Lindsey, D., Race, R., Wu, X., and Zierk, S.: From photons to pixels: Processing data from the Advance Baseline Imager, Remote Sens., 10, 177, https://doi.org/10.3390/rs10020177, 2018.
Kaufman, Y. J., Koren, I., Remer, L. A., Tanré, D., Ginoux, P., and Fan, S.: Dust transport and deposition observed from the Terra-MODIS spacecraft over the Atlantic Ocean, J. Geophys. Res., 110, D10S12, https://doi.org/10.1029/2003JD004436, 2005.
Kondragunta, S., Laszlo, I., Zhang, H., Ciren, P., and Huff, A.: Air Quality Applications of Aerosol Products from the GOES-R ABI, A book chapter https://doi.org/10.1016/B978-0-12-814327-8.00017-2, 2019.
Koren, I., Kaufman, Y. J., Rosenfeld, D., Remer, L. A., and Rudich, Y.: Aerosol invigoration and restructuring of Atlantic convective clouds, Geophys. Res. Lett., 32, LI4828, https://doi.org/10.1029/2005GL023187, 2005.
Levy, R. C., Remer, L. A., and Dubovik, O.: Global aerosol optical properties and application to Moderate Resolution Imaging Spectroradiometer aerosol retrieval over land, J. Geophys. Res.-Atmos., 112, D13210, https://doi.org/10.1029/2006JD007815, 2007a.
Levy, R. C., Remer, L. A., Mattoo, S., Vermote, E. F., and Kaufman, Y. J.: Second-generation operational algorithm: Retrieval of aerosol properties over land from inversion of Moderate Resolution Imaging Spectroradiometer spectral reflectance, J. Geophys. Res.-Atmos., 112, D13211, https://doi.org/10.1029/2006JD007811, 2007b.
Levy, R. C., Remer, L. A., Kleidman, R. G., Mattoo, S., Ichoku, C., Kahn, R., and Eck, T. F.: Global evaluation of the Collection 5 MODIS dark-target aerosol products over land, Atmos. Chem. Phys., 10, 10399–10420, https://doi.org/10.5194/acp-10-10399-2010, 2010.
Levy, R. C., Mattoo, S., Munchak, L. A., Remer, L. A., Sayer, A. M., Patadia, F., and Hsu, N. C.: The Collection 6 MODIS aerosol products over land and ocean, Atmos. Meas. Tech., 6, 2989–3034, https://doi.org/10.5194/amt-6-2989-2013, 2013.
Levy, R. C., Munchak, L. A., Mattoo, S., Patadia, F., Remer, L. A., and Holz, R. E.: Towards a long-term global aerosol optical depth record: applying a consistent aerosol retrieval algorithm to MODIS and VIIRS-observed reflectance, Atmos. Meas. Tech., 8, 4083–4110, https://doi.org/10.5194/amt-8-4083-2015, 2015.
Levy, R. C., Mattoo, S., Sawyer, V., Shi, Y., Colarco, P. R., Lyapustin, A. I., Wang, Y., and Remer, L. A.: Exploring systematic offsets between aerosol products from the two MODIS sensors, Atmos. Meas. Tech., 11, 4073–4092, https://doi.org/10.5194/amt-11-4073-2018, 2018.
Li, R.-R., Kaufman, Y. J., Gao, B.-C., and Davis, C. O.: Remote sensing of suspended sediments and shallow coastal waters, IEEE Trans. Geosci. Remote, 41, 559–566, 2003.
Lim, H., Choi, M., Kim, M., Kim, J., and Chan, P. W.: Retrieval and validation of aerosol optical properties using Japanese next generation meteorological satellite, Himawari-8, Korean J. Radiol., 32, 681–691, 2016.
Lim, H., Choi, M., Kim, M., Kim, J., Go, S., and Lee, S.: Intercomparing the Aerosol Optical Depth Using the Geostationary Satellite Sensors (AHI, GOCI and MI) from Yonsei AErosol Retrieval (YAER) Algorithm, J. Korean Earth Sci. Soc., 39, 119–130, 2018.
Lim, H., Choi, M., Kim, J., Kasai, Y., and Chan, P. W.: AHI/Himawari-8 Yonsei Aerosol Retrieval (YAER): Algorithm, Validation and Merged Products, Remote Sens., 10, 699, https://doi.org/10.3390/rs10050699, 2018.
Lim, S. S., Vos, T., Flaxman, A. D., et al.: A comparative risk assessment of burden of disease and injury attributable to 67 risk factors and risk factor clusters in 21 regions, 1990–2010: a systematic analysis for the Global Burden of Disease Study 2010, Lancet, 380, 2224–2260, 2012.
Lin, J. C., Matsui, T., Pielke Sr., R. A., and Kummerow, C.: Effects of biomass-burning-derived aerosols on precipitation and clouds in the Amazon Basin: a satellite-based empirical study, J. Geophys. Res., 111, D19204, https://doi.org/10.1029/2005JD006884, 2006.
Lyapustin, A., Wang, Y., Laszlo, I., Kahn, R., Korkin, S., Remer, L., Levy, R., and Reid, J. S.: Multiangle implementation of atmospheric correction (MAIAC): 2. Aerosol algorithm, J. Geophys. Res., 116, D03211, https://doi.org/10.1029/2010JD014986, 2011.
Martins, J. V., Tanré, D., Remer, L., Kaufman, Y., Mattoo, S., and Levy, R.: MODIS Cloud screening for remote sensing of aerosols over oceans using spatial variability, Geophys. Res. Lett., 29, 32139, https://doi.org/10.1029/2001GL013252, 2002.
Martonchik, J. V., Diner, D. J., Kahn, R., Ackerman, T. P., Verstraete, M. M., Pinty, B., and Gordon, H. R.: Techniques for the retrieval of aerosol properties over land and ocean using multiangle imaging, IEEE T. Geosci. Remote, 36, 1212–1227, 1998.
Munchak, L. A., Levy, R. C., Mattoo, S., Remer, L. A., Holben, B. N., Schafer, J. S., Hostetler, C. A., and Ferrare, R. A.: MODIS 3 km aerosol product: applications over land in an urban/suburban region, Atmos. Meas. Tech., 6, 1747–1759, https://doi.org/10.5194/amt-6-1747-2013, 2013.
Patadia, F., Gupta, P., and Christopher, S. A.: First observational estimates of global clear sky shortwave aerosol direct radiative effect over land, Geophys. Res. Lett., 35, L04810, https://doi.org/10.1029/2007GL032314, 2008.
Petrenko, M., Ichoku, C., and Leptoukh, G.: Multi-sensor Aerosol Products Sampling System (MAPSS), Atmos. Meas. Tech., 5, 913–926, https://doi.org/10.5194/amt-5-913-2012, 2012.
Prados, A. I., Kondragunta, S., Ciren, P., and Knapp, K. R.: GOES Aerosol/Smoke Product (GASP) over North America: Comparisons to AERONET and MODIS observations, J. Geophys. Res., 112, D15201, https://doi.org/10.1029/2006JD007968, 2007.
Quaas, J., Ming, Y., Menon, S., Takemura, T., Wang, M., Penner, J. E., Gettelman, A., Lohmann, U., Bellouin, N., Boucher, O., Sayer, A. M., Thomas, G. E., McComiskey, A., Feingold, G., Hoose, C., Kristjánsson, J. E., Liu, X., Balkanski, Y., Donner, L. J., Ginoux, P. A., Stier, P., Grandey, B., Feichter, J., Sednev, I., Bauer, S. E., Koch, D., Grainger, R. G., Kirkevåg, A., Iversen, T., Seland, Ø., Easter, R., Ghan, S. J., Rasch, P. J., Morrison, H., Lamarque, J.-F., Iacono, M. J., Kinne, S., and Schulz, M.: Aerosol indirect effects – general circulation model intercomparison and evaluation with satellite data, Atmos. Chem. Phys., 9, 8697–8717, https://doi.org/10.5194/acp-9-8697-2009, 2009.
Remer, L. A., Kaufman, Y. J., Tanré, D., Mattoo, S., Chu, D. A., Martins, J. V., Li, R., Ichoku, C., Levy, R. C., Kleidman, R. G., Eck, T. F., Vermote, E., and Holben, B. N.: The MODIS Aerosol Algorithm, Products, and Validation, J. Atmos. Sci., 62, 947–973, 2005.
Remer, L. A., Kleidman, R. G., Levy, R. C., Kaufman, Y. J., Tanré, D., Mattoo, S., Martins, J. V., Ichoku, C., Koren, I., Yu, H., and Holben, B. N.: Global aerosol climatology from the MODIS satellite sensors, J. Geophys. Res.-Atmos., 113, D14S07, doi:10.1029/2007JD009661, 2008.
Remer, L. A., Mattoo, S., Levy, R. C., Heidinger, A., Pierce, R. B., and Chin, M.: Retrieving aerosol in a cloudy environment: aerosol product availability as a function of spatial resolution, Atmos. Meas. Tech., 5, 1823–1840, https://doi.org/10.5194/amt-5-1823-2012, 2012.
Remer, L. A., Mattoo, S., Levy, R. C., and Munchak, L. A.: MODIS 3 km aerosol product: algorithm and global perspective, Atmos. Meas. Tech., 6, 1829–1844, https://doi.org/10.5194/amt-6-1829-2013, 2013.
Rosenfeld, D., Sherwood, S., Wood, R., and Donner, L.: Climate effects of aerosol-cloud interactions, Science, 343, 379–380, https://doi.org/10.1126/science.1247490, 2014a.
Rosenfeld, D., Andreae, A., and Asmi, A.: Global observations of aerosol-cloud-precipitation-climate interactions, Rev. Geophys., 52, 750–808, https://doi.org/10.1002/2013RG000441, 2014b.
Seinfeld, J. H., Bretherton, C., Carslaw, K. S., Coe, H., DeMott, P. J., Dunlea, E. J., Feingold, G., Ghan, S., Guenther, A. B., Kahn, R., Kraucunas, I., Kreidenweis, S. M., Molina, M. J., Nenes, A., Penner, J. E., Prather, K. A., Ramanathan, V., Ramaswamy, V., Rasch, P. J., Ravishankara, A. R., Rosenfeld, D., Stephens, G., and Wood, R.: Improving our fundamental understanding of the role of aerosol-cloud interactions in the climate system, P. Natl. Acad. Sci. USA, 113, 5781–5790, 2016.
Sekiyama, T. T., Yumimoto, K., Tanaka, T. Y., Nagao, T., Kikuchi, M., and Murakami, H.: Data assimilation of Himawari-8 aerosol observations: Asian dust forecast in June 2015, Sola, 12, 86–90, 2016.
Shi, S., Cheng, T., Gu, X., Letu, H., Guo, H., Chen, H., and Wu, Y.: Synergistic Retrieval of Multi-temporal Aerosol Optical Depth over North China Plain Using Geostationary Satellite Data of Himawari-8, J. Geophys. Res.-Atmos., 123, 5525–5537, https://doi.org/10.1029/2017JD027963, 2018.
Tanré, D., Herman, M., and Kaufman, Y.: Information on the aerosol size distribution contained in the solar reflected spectral radiances, J. Geophys. Res., 101, 19043–19060, 1996.
Tanré, D., Kaufman, Y. J., Herman, M., and Mattoo, S.: Remote sensing of aerosol properties over oceans using the MODIS/EOS spectral radiances, J. Geophys. Res., 102, 16971–16988, 1997.
Tanré, D., Bréon, F. M., Deuzé, J. L., Dubovik, O., Ducos, F., François, P., Goloub, P., Herman, M., Lifermann, A., and Waquet, F.: Remote sensing of aerosols by using polarized, directional and spectral measurements within the A-Train: the PARASOL mission, Atmos. Meas. Tech., 4, 1383–1395, https://doi.org/10.5194/amt-4-1383-2011, 2011.
Torres, O., Tanskanen, A., Veihelman, B. , Ahn, C. , Braak, R., Bhartia, P. K., Veefkind, P., and Levelt, P.: Aerosols and surface UV products from OMI observations: An overview, J. Geophys. Res., 112, D24S47, https://doi.org/10.1029/2007JD008809, 2007.
Torres, O., Ahn, C., and Chen, Z.: Improvements to the OMI near-UV aerosol algorithm using A-train CALIOP and AIRS observations. Atmos. Meas. Tech., 6, 3257-3270, https://doi.org/10.5194/amt-6-3257-2013, 2013.
Uesawa, D.: Aerosol Optical Depth product derived from Himawari-8 data for Asian dust monitoring, Meteorological Satellite Center Technical Note, No. 61, 2016.
Wang, J. and Christopher, S. A.: Intercomparison between satellite-derived aerosol optical thickness and PM2.5 mass: Implications for air quality studies, Geophys, Res. Lett., 30, 2095, https://doi.org/10.1029/2003GL018174, 2003.
Yan, X., Li, Z., Luo, N., Shi, W., Zhao, W., Yang, X., and Jin, J.: A minimum albedo aerosol retrieval method for the new-generation geostationary meteorological satellite Himawari-8, Atmos. Res., 207, 14–27, 2018.
Yang, F., Wang, Y., Tao, J., Wang, Z., Fan, M., de Leeuw, G., and Chen, L.: Preliminary Investigation of a New AHI Aerosol Optical Depth (AOD) Retrieval Algorithm and Evaluation with Multiple Source AOD Measurements in China, Remote Sens., 10, 748, https://doi.org/10.3390/rs10050748, 2018.
Yoshida, M., Kikuchi, M., Nagao, T. M., Murakami, H., Nomaki, T., and Higurashi, A.: Common retrieval of aerosol properties for imaging satellite sensors, J. Meteorol. Soc. Jpn., 96, 193–209, https://doi.org/10.2151/jmsj.2018-039, 2018.
Yu, F. and Wu, X.: Radiometric Inter-Calibration between Himawari-8 AHI and S-NPP VIIRS for the Solar Reflective Bands, Remote Sens., 8, 165, https://doi.org/10.3390/rs8030165, 2016.
Yu, H., Remer, L. A., Chin, M., Bian, H., Tan, Q., Yuan, T., and Zhang, Y.: Aerosols from overseas rival domestic emissions over North America, Science, 337, 566–569, https://doi.org/10.1126/science.1217576, 2012.
Yu, H., Remer, L. A., Kahn, R. A., Chin, M., and Zhang, Y.: Satellite perspective of aerosol intercontinental transport: From qualitative tracking to quantitative characterization, Atmos. Res., 124, 73–100, https://doi.org/10.1016/j.atmosres.2012.12.013, 2013.
Yu, H., Chin, M., Yuan, T. L., Bian, H., Remer, L. A., Prospero, J. M., Omar, A., Winker, D., Yang, Y. K., Zhang, Y., Zhang, Z., and Zhao, C.: The fertilizing role of African dust in the Amazon rainforest: A first multiyear assessment based on data from Cloud-Aerosol Lidar and Infrared Pathfinder Satellite Observations, Geophys. Res. Lett., 42, 1984–1991, https://doi.org/10.1002/2015GL063040, 2015.
Yumimoto, K., Nagao, T. M., Kikuchi, M., Sekiyama, T. T., Murakami, H., Tanaka, T. Y., and Arai, K.: Aerosol data assimilation using data from Himawari-8, a next-generation geostationary meteorological satellite, Geophys. Res. Lett., 43, 5886–5894, 2016.
Zhang, J., Christopher, S. A., Remer, L. A., and YKaufman, Y. J.: Shortwave aerosol radiative forcing over cloud-free oceans from Terra: 2. Seasonal and global distributions, J. Geophys. Res., 110, D10S24, https://doi.org/10.1029/2004JD005009, 2005.
Zhang, W., Xu, H., and Zheng, F.: Aerosol Optical Depth Retrieval over East Asia Using Himawari-8/AHI Data, Remote Sens., 10, 137, https://doi.org/10.3390/rs10010137, 2018.