the Creative Commons Attribution 3.0 License.
the Creative Commons Attribution 3.0 License.
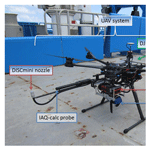
Characterization of the particle emission from a ship operating at sea using an unmanned aerial vehicle
Tommaso F. Villa
Reece A. Brown
E. Rohan Jayaratne
L. Felipe Gonzalez
Lidia Morawska
Zoran D. Ristovski
This research demonstrates the use of an unmanned aerial vehicle (UAV) to characterize the gaseous (CO2) and particle (10–500 nm) emissions of a ship at sea. The field study was part of the research voyage “The Great Barrier Reef as a significant source of climatically relevant aerosol particles” on board the RV Investigator around the Australian Great Barrier Reef. Measurements of the RV Investigator exhaust plume were carried out while the ship was operating at sea, at a steady engine load of 30 %.
The UAV system was flown autonomously using several different programmed paths. These incorporated different altitudes and distances behind the ship in order to investigate the optimal position to capture the ship plume. Five flights were performed, providing a total of 27 horizontal transects perpendicular to the ship exhaust plume. Results show that the most appropriate altitude and distance to effectively capture the plume was 25 m a.s.l. and 20 m downwind.
Particle number emission factors (EFPNs) were calculated in terms of number of particles emitted (no.) per weight of fuel consumed (kgfuel). Fuel consumption was calculated using the simultaneous measurements of plume CO2 concentration.
The calculated EFPN was no. kg which is in line with those reported in the literature for ship emissions ranging from 0.2 to 6.2×1016 no. kg.
This UAV system successfully assessed ship emissions to derive EFPN under real world conditions. This is significant as it provides a novel, relatively inexpensive and accessible way to assess ship EFPN at sea.
- Article
(1466 KB) - Full-text XML
-
Supplement
(316 KB) - BibTeX
- EndNote
Shipping is the most significant contributor to international freight, with almost 80 % of the worldwide merchandise trade by volume transported by ships in 2015 (UNCTAD, 2015). Emissions from this transportation mode are a significant contributor to air pollution, both locally and globally. Ships are a major pollutant source in areas surrounding harbours (Viana et al., 2014), with over 70 % of emissions reaching 400 km inland (Fuglestvedt et al., 2009). In 2012 exhaust from diesel engines, the predominant source of ship power, was classified as a Group 1 carcinogen by the International Agency for Research on Cancer (IARC). In 2007, pollution from ship exhaust was found to be responsible for approximately 60 000 cardiopulmonary and lung cancer deaths worldwide annually (Corbett et al., 2007). Such emissions are also a strong climate forcing agent, contributing to global warming through the absorbance of solar and terrestrial radiation (Hallquist et al., 2013; Lack et al., 2011; Winnes et al., 2016).
Despite these findings, emissions from shipping have consistently been subject to less regulation than those of land-based transport, with ship emissions in international waters remaining one of the least regulated parts of the global transportation system (Corbett and Koehler, 2003; Corbett and Farrell, 2002; Eyring et al., 2005; Streets et al., 1997; Cooper, 2001, 2005). Currently, no specific restrictions for ship-emitted particulate matter (PM) exist, with the only regulated pollutants being NOx and SO2. The International Maritime Organization (IMO) recently revised the regulation of these gaseous pollutants through the Annex VI of the International Convention for the Prevention of Pollution from Ships – the Marine Pollution Convention (MARPOL). The IMO expected that these regulations would lead to an indirect decrease in particle number (PN) concentration due to the reduction of NOx emissions and the use of fuel with lower sulfur content (Chen et al., 2005). However, it has been found that the use of some low sulfur fuels leads to increased PN concentrations at lower engine loads (Anderson et al., 2015), which stresses the importance for regulation specifically addressing PM.
The majority of emitted PM is in the ultrafine size range, <0.1 µm, which has been demonstrated to have a particularly significant impact on health and the environment (WHO, 2013). However, due to the lack of regulation, ultrafine particles, in terms of PN concentration, emitted from ships have remained unassessed in real world conditions. Quantifying PN concentration is critical to improving our understanding of shipping's impact on health and climate (Corbett and Farrell, 2002; Corbett et al., 2007; Isakson et al., 2001; Williams et al., 2009; Reda et al., 2015; Mueller et al., 2015; Anderson et al., 2015; Ristovski et al., 2012; Blasco et al., 2014). To achieve this, wide-scale evaluation of ship emission factors (EFs) is necessary. EFs are commonly expressed as the amount of pollutant (x) emitted per unit mass of fuel consumed g(x). (kgfuel)−1. Different methods have been used to investigate ship EFs, including laboratory test-bench studies, on-board measurements and measurement of ship emission plumes.
Test-bench studies (Anderson et al., 2015; Mueller et al., 2015; Reda et al., 2015; Petzold et al., 2008, 2010; Kasper et al., 2007) have been used to characterize emissions from different engines at various loads in laboratory conditions. However, engine performance and emissions have been shown to be different in real world operations when compared to laboratory studies. This calls for measurements of ship emissions in situ to collect reliable data for EF calculations (Agrawal et al., 2008; Murphy et al., 2009; Blasco et al., 2014). To date, only a few studies have been undertaken on board ships to calculate real emission factors (Juwono et al., 2013; Hallquist et al., 2013). This is attributed to the prohibitive costs and time commitments of setting up and maintaining on-board measurement equipment on commercial ships. Airborne ship plume measurements (Westerlund et al., 2015; Pirjola et al., 2014; Cappa et al., 2014; Beecken et al., 2014; Balzani Lööv et al., 2014; Berg et al., 2012; Lack et al., 2008, 2009; Sinha et al., 2003) offer an alternative method of in situ measurements without requiring on-board monitoring stations. In the past the deployment cost of these systems and the risks associated with manned aircraft have limited their feasibility. However, this has recently changed, with rapid advances being made in commercially available unmanned aerial vehicle (UAV) technology.
Hexacopter UAVs have seen a wide-scale increase in industry and research applications due to their ease of use and comparatively low cost (Brady et al., 2016; Malaver Rojas et al., 2015; Gonzalez et al., 2011). Used in conjunction with air monitoring equipment, these systems provide, for the first time, the ability to perform relatively simplistic and cost-effective airborne measurements of ship emissions. However, to date no studies have reported the use of a UAV system capable of collecting data to calculate the EF of PN concentration for ships at sea.
This research utilized a customized hexacopter UAV carrying instruments for PN concentration and CO2 measurements to derive EFPN. The UAV system was deployed from the RV Investigator while at sea. Autonomous measurements of the RV Investigator's exhaust plume were taken over several flights at various altitudes and distances from the ship. Data collected were used to optimize the sampling flight path and successfully quantify the RV Investigator's EF for PN concentration.
Measurements were conducted as part of the research voyage “The Great Barrier Reef as a significant source of climatically relevant aerosol particles” aboard the RV Investigator over a 2-day period of the 13 and 14 October 2016 (day 1 and 2). Measurements of PN and CO2 concentration emitted by the RV Investigator were taken using a PN and CO2 monitor mounted on a customized DJI EVO S800 hexacopter UAV (DJI, 2014).
2.1 The RV Investigator and the voyage
The RV Investigator is an ocean research vessel configured to enable a wide range of atmospheric, biological, geoscience and oceanographic research. The vessel is 94 m long, has a gross weight of 6082 tons and has a fuel capacity of 700 tons of ultra-low sulfur diesel fuel. It is powered by three 9-cylinder 3000 kW MaK diesel engines, each coupled to a 690 V AC generator. Ship propulsion is achieved using two 2600 kW L3 AC reversible propulsion motors powered by these generators. The RV Investigator can host up to 30 crew members and 35 researchers for a maximum voyage period of 60 days, with a maximum cruising speed of 12 knots.
A suite of instrumentation for atmospheric research is available on the RV Investigator. This includes a radar system capable of collecting weather information within a 150 km radius of the vessel, and instruments measuring sunlight parameters; aerosol composition, particle concentration and size distributions; cloud condensation nuclei; gas concentrations; and various other components of the atmosphere. These instruments are housed inside two dedicated on-board laboratories for aerosol and for atmospheric chemistry research. An atmospheric aerosol sample is continuously drawn into the laboratories for analysis through a specialized inlet fitted to the foremast of the ship. Of particular interest to this study is that the ship contains a PICARRO (PICARRO Inc., Santa Clara, California, USA) G2401 analyser that continuously measures CO2, CO, H2O and CH4. It has an operation range between 0 and 1000 ppm and a parts-per-billion (ppb) sensitivity for CO2.
The 2-day UAV measurement study was possible as part of the RV Investigator voyage “The Great Barrier Reef as a significant source of climatically relevant aerosol particles”, which started in Brisbane on the 28 September 2016. The ship was used as both a floating platform to allow launch and recovery of the UAV system and as the source of an exhaust plume measured by the UAV system for EF calculation. During a stationary period several days long on the Great Barrier Reef off the coast of Australia, it was possible to measure the ship plume under stable real world conditions over two consecutive days. One of the three ship engines was maintained at a steady engine load of 25 %–30 % of the maximum engine power during all measurements.
2.2 UAV system
Measurements of PN and CO2 concentrations in the ship plume were performed using two commercial sensors mounted on board a hexacopter UAV. The UAV used (Fig. 1) is a composite material S800 EVO manufactured by DJI (DJI, 2014). The UAV is 800 mm wide and 320 mm high, with an unloaded weight of 3.7 kg. Minimum and maximum take-off weights are 6.7 and 8 kg, respectively. The UAV contains a 16 000 mAh LiPo 6 cell battery, which provides a hover time of approximately 20 min when operating at minimum take-off weight. The telemetry range of the UAV is 2 km, which was adequate to cover the desired sampling area (see Fig. 2).
The payload consisted of a PN concentration and a CO2 monitor mounted on board underneath the UAV. Careful placement of the payload was required to prevent flight issues caused by an altered centre of gravity. Also included was a carbon fibre rod, which extended outward horizontally from the UAV. The sampling lines for the monitors were attached to the end of this rod to ensure that measurements were not affected by the downwash of the UAV rotors. The total weight of the payload was 1.2 kg, which allowed the UAV system to fly for 12–15 min before landing at the home point (A) (see Fig. 2).
The S800 was used in conjunction with the DJI Wookong autopilot. The software provides an intuitive and easy-to-use interface in which autonomous flight paths can be planned, saved and uploaded into the UAV. In addition to this, the ground station allows for continuous, real-time monitoring of the status of the UAV during operation, which includes its longitude, latitude, altitude, waypoint tolerance and airspeed.
The DJI S800 was chosen for this study because it is designed to operate under the 20 kg all-up weight class of UAV. This reduces operational costs and avoids subjection to the tighter regulations of larger platforms. Small UAVs cannot be operated above any person or closer than 30 m to populated areas, houses and people. Furthermore, current Civil Aviation Safety Australia (CASA) regulations restrict the use of small UAVs (2 and 20 kg) to visual line-of-sight daylight operation, with a maximum altitude of approximately 120 m and within a radius of 3 nmi of an airport. UAVs in this category are not permitted for research unless the research institution has been granted a permit exception. These exceptions can be granted if the institution in question has or collaborates with an UAV operation team who must have an experienced UAV pilot who is also a radio controller specialist, a license for commercial UAV operation and appropriate liability insurance (CASA, 2014). Queensland University of Technology (QUT) has an unmanned operator certificate and four pilots who have UAV controller licenses.
2.2.1 Instrumentation
Instrumentation for PN concentration
This study measured PN concentration using the Miniature Diffusion Size Classifier (DISCmini), developed by the University of Applied Sciences, Windisch, Switzerland (Fierz et al., 2008). The DISCmini is a portable monitor used to measure the concentration of particles in the 10–500 nm diameter size range, with a time resolution of up to 1 s (1 Hz). It can measure PN concentrations between 103 and 106 N cm−3. Measurement accuracy is dependent upon the particle shape, size distribution and number concentration. The advantages of using the DISCmini are its relatively small dimensions (180 mm × 90 mm × 40 mm), low weight (640 g, 780 g with the sampling probe; Fig. 1) and long battery life of up to 8 h. These characteristics allow it to be easily integrated on the UAV.
Instrumentation for CO2 concentration measurements
A TSI (TSI, Shoreview, Minnesota, United States) IAQ-calc 7545 model was chosen to measure CO2 concentrations. Its sensor is based on a dual-wavelength NDIR (non-dispersive infrared) with a sensitivity range between 0 and 5000 ppm and an accuracy of ±3.0 % of reading or ±50 ppm (whichever is greater). The measurement resolution is 1 ppm with a maximum time resolution of 1 s. Similar to the DISCmini, the advantages of using the IAQ-calc are its small dimensions (178 mm × 84 mm × 44 mm); low weight (270 g, with batteries, significantly lower than the DISCmini); and a battery life of 10 h.
The readings of the IAQ-clac for CO2 were compared with those measured by the on-board PICARRO G2401 analyser.
Both the DISCmini and the IAQ-calc were tested and calibrated in the on-board laboratory using ambient aerosol measurements at sea prior to the commencement of the measurements (Fig. S2 in the Supplement). All data were logged with a 1 s time interval.
2.3 Meteorological data
Meteorological data (including air temperature, relative humidity, atmospheric pressure and wind speed and direction) were recorded by the RV Investigator's on-board instrumentation during the entire voyage with a 60 s time interval, 24 h a day.
2.4 Study design
During the two measurement days of this study, the vessel was heading into the wind whilst idling the UAV missions at sea. This positioning caused the exhaust plume to extend downwind, directly behind the ship. The UAV system was launched off the back deck, autonomously sampling at varying altitudes and distances into the downwind plume. Flight speed of the UAV was 1.5 m s−1, the minimum for the S800.
Day 1 was used to optimize the study design, focusing on finding the flight path most suitable to capture the ship plume. Figure 2 shows the programmed flight path, which consisted of a continuous flight beginning at a distance (D) and from an altitude (H) above the surface. Point A, located on the back deck of the RV Investigator, represents the home point. In UAV terminology this refers to the position where the UAV system takes off and lands. The UAV system was programmed to move horizontally by a distance (2 d), perpendicular to the ship, then climb vertically for 10 m (h) before flying in the opposite horizontal direction for the same distance (2 d). The UAV was then programmed to climb another 10 m (h) before repeating this pattern until the UAV reached an altitude of 65 m above the ocean. During day 1, the UAV system followed three different flight paths, each one with both a different distance D behind the ship (20, 50 and 100 m) and a different horizontal distance 2 d (50, 100 and 150 m).
The optimized flight path for day 2 started 20 m behind the ship and 25 m above the surface, with no altitude variation. The UAV path was limited to a continuous horizontal flight of 50 m (2 d) at a steady speed of 2 m s−1. This path and flying speed allowed up to four horizontal transects to capture the ship plume.
2.5 Experimental procedure
The UAV can fly either manually or autonomously. As a safety precaution, every take-off and landing was performed using the manual flight mode. Once in the air, the UAV was switched to autonomous flight mode, allowing the platform to follow the preprogrammed flight path discussed in the previous section. The flight path consisted of waypoints, which are three-dimensional GPS points that dictate the position of the UAV along the fight path. The waypoints and flight plans for each flight were programmed using the aforementioned DJI Wookong ground station software. The DISCmini and the IAQ-calc were fitted on the underside of the UAV at the beginning of each measuring day. Five flights were performed across the two measurement days, providing a total of 27 horizontal transects perpendicular to the ship's exhaust plume.
2.6 Emission factors
The calculation of an emission factor for particle number concentration (EFPN) from the collected ship plume measurements was performed using Eq. (1). This method has previously been used for ship (Westerlund et al., 2015), road vehicle (Hak et al., 2009) and aircraft (Mazaheri et al., 2009) emissions. The measured values of PN concentration were related to the amount of fuel consumed by the engine in question through the use of the simultaneous measurements of CO2 concentration taken by the UAV. This was achieved by using a published value for a ship emission factor of CO2 (EFgas) of 3.2 kg CO2 (kgfuel)−1 (Hallquist et al., 2013; Hobbs et al., 2000).
The ΔPN and Δgas in Eq. (1) represent the maximum particle concentration change above background in the measured particle number and CO2 concentrations, respectively. The DISCmini measurements were corrected against a reference condensation particle counter (CPC). For each transect data series of PN concentration and CO2, the averaged background concentrations were subtracted from the peak data corresponding to measurements inside the plume. The corrected peak data series were then fit with a Gaussian curve using the inbuilt Matlab curve fitting application. The least absolute residuals condition was used as this most closely fits the curve to the highest magnitude data points in the series. The maximum peak heights of the fitted Gaussian curves were used as ΔPNC and ΔCO2 in the calculation of emission factors for each transect.
3.1 Meteorological and Investigator data
Wind conditions were very stable during both day 1 and 2, following one main pattern for the entire flight time. The wind speed ranged from 3 to 13 m s−1. The wind direction was predominantly from the NE during day 1 and ESE during day 2. The wind rose graphs in Fig. 3a and b illustrate the wind data recorded with the on-board weather instrumentation during all horizontal transects flown during day 1 and 2 respectively. The prevalent wind direction was ESE, which corresponded to the heading of the RV Investigator (indicated by the rose triangle). The wind direction changed occasionally to E during the flight, causing the UAV to fail to capture the RV Investigator plume during some transects. As a result, two of the eight horizontal transects collected on day 2 were excluded from the analysis.
3.2 UAV system horizontal transects inside and outside the plume
The UAV system acquired data for a total of 27 horizontal transects for day 1 and 2. Data were collected at altitudes between 25 and 65 m above the water surface. During day 1 the plume was captured once when the UAV was at 25 m altitude and 20 m downwind of the ship, and again at both 25 and 35 m altitude 100 m downwind of the ship. These observations led to the optimized flight used on day 2, which started downwind at 25 m above the surface and 20 m behind the ship. On day 2 the UAV system successfully captured the plume during six of the eight transects performed. Across the 2 days, this led to a total of nine transects that captured the plume and which have been considered for discussion, shown in Table 1.
Table 1Specifications of the transects considered for the data analysis.
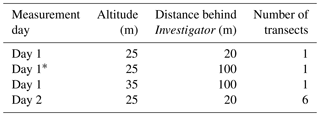
* indicates the transect of Day 1 of which PN concentration and CO2 profiles are presented in Fig. 4.
Figure 4 shows the PN concentration and CO2 profiles, collected during two (a, b) transects on day 2 and (c) during one transect of day 1 (spec. in Table 1, Day1*).
The PN concentration profiles for the (a) and (b) transects in Fig. 4 show that the concentration varied by 5 orders of magnitude between the outside and inside of the plume, while the CO2 profiles show an increase of up to 140 ppm above the background.
The profiles in (c) show that the PN concentration was 4 orders of magnitude greater inside the plume 100 m behind the ship and that the CO2 concentration was up to 70 ppm higher inside the plume.
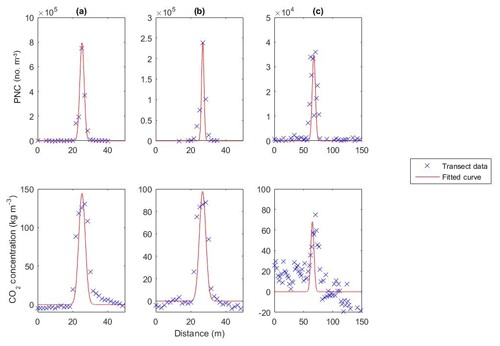
Figure 4Panels (a) and (b) show the measured PN and CO2 concentration profiles and fitted Gaussian curves for two different transects 20 m behind the ship, 25 m above the surface during day 2. Panel (c) shows the PN and CO2 concentration profiles and fitted Gaussian curves collected during flight 3 of day 1 100 m behind the ship, 25 m above the surface.
Figure 4a and b both show transects at 25 m altitude and 20 m behind the ship. Both the PN concentration and CO2 measurements show clear, single peaks as the UAV crosses the plume. As a consequence, these transects show a good fit with the corresponding Gaussian distribution curves, with R2 values of above 0.9 for both PNC and CO2. In contrast, Fig. 4c shows substantially less defined, wider peaks with lower pollutant concentrations. This is attributed to a difference in flight paths, with Fig. 4c representing data from a transect 100 m behind the ship. The additional time between emission and sampling has allowed the plume to broaden, become less homogenous and take on a skewed cross section. This results in a significantly lower R2 value for the fitted Gaussian curves, with a value of 0.4998 for the CO2 data in this transect. Therefore, whilst the 100 m transect does provide more data points inside the plume, the randomized variations inside the plume lead to less accurate calculations of emission factors.
Of further note in Fig. 4, the maximum PN concentration measured in (a) (7.5×105 no. cm−3) is approximately 3 times greater than that in (b) (2.4×105 no. cm−3), and the CO2 concentrations in (a) are 43 ppm greater than (b). The transect flight plan and ship engine load remained constant throughout these measurements. The variations between (a) and (b) are attributed to several factors which reduce the effectiveness of the UAV transect for capturing the plume. Slight changes in ambient conditions such as temperature, wind direction and intensity will alter the path of the plume as it moves away from the ship. The UAVs' automated flight path cannot account for these variations. Therefore, the degree to which the UAV enters the plume, and thus the concentrations it measures, will be different on each transect. Both CO2 and PN concentration measurements will be similarly affected by this variance. However, differences in instrument response rates in conjunction with these variances will be one of the major contributors to variations in calculated emission factors.
3.3 PN emission factors
Table 2 shows the distance and altitude of each transect, the R2 values of the fitted Gaussian curves for PNC and CO2 data, the calculated values of ΔPNC and ΔCO2 and the calculated EFPN.
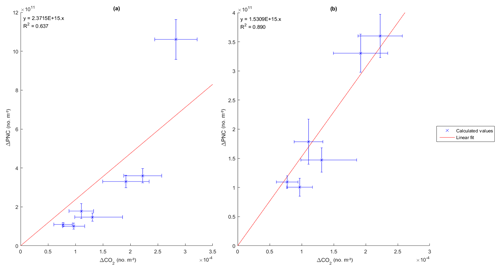
Figure 5(a) ΔPNC against ΔCO2 with a 95 % confidence interval for the six transects considered for the data analysis. (b) ΔPNC against ΔCO2 with a 95 % confidence interval with the removal of the outlier transect from the first flight of day 2.
Table 2Transect flight days and details, R2 values for the Gaussian curve fits to both PNC and CO2 data, ΔPNC and ΔCO2 concentration emission/rate of the RV Investigator and calculated emission factors for PN.
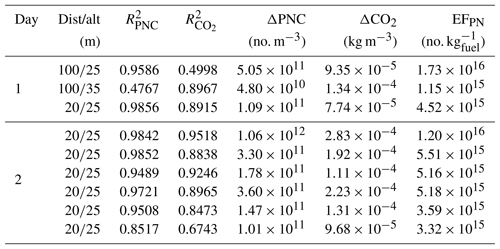
The calculated EFPN values for the RV Investigator ranged from 1.15×1015 to 1.73×1016 no. kg. The two 100 m transects provided the worst Gaussian fits as well as the highest and lowest calculated emission factors. This indicates that it is important to filter out transects with data which do not fit the expected Gaussian distribution suitably as they can generate significant error. To this end, the 100 m transects were excluded from further analysis. ΔPNC and ΔCO2 values for remaining transects were plotted against each other as shown in Fig. 5.
Figure 5a and b show the plots of the remaining transects ΔPNC against ΔCO2 with and without the values of the first flight of day 2. This transect represents a clear outlier in the linear trend, with the R2 value of the linear fit increasing from 0.637 to 0.890 with its exclusion. Furthermore, whilst the linear fit falls within the confidence interval of only one point in (a), it falls within all data points' confidence intervals in (b). This occurs despite both R2 values for the fitted Gaussians of this transect being very high (, ). This highlights a limitation with this methodology which can be best observed in the difference between Fig. 4a and b. The combination of UAV velocity, sampling rate and response time of the DISCmini results in the PNC transect data having only one data point defining the peak height of the transect. Relying on a single sample point leads to the potential for random instrumentation effects heavily biasing results in a way which does not strongly impact the R2 values of Gaussian fits used to identify successful transects. Therefore, it is unclear whether this is a variation in the ship emissions or an instrumentation error.
The slope and standard error of the linear fit for Fig. 4a was input unto Eq. (1) to calculate an overall emission factor of no. kg. As presented in Table 3, this value is comparable with those reported in the literature for cruise and cargo ship plumes, which range from 0.2 to 6.2×1016 no. kg (Alföldy et al., 2013; Beecken et al., 2014; Jonsson et al., 2011; Juwono et al., 2013; Lack et al., 2009, 2011; Pirjola et al., 2014; Sinha et al., 2003; Westerlund et al., 2015).
Table 3Comparison of the emission factor for the RV Investigator found in this study with other relevant values found in the literature.
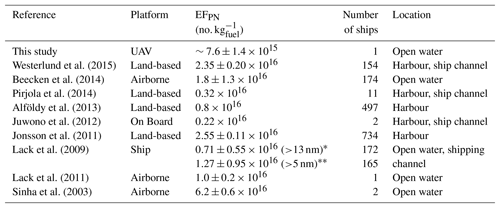
* PNEF for particles above 13 nm. PNEF for particles above 5 nm.
The calculated EFPN for the Investigator was lower compared to that reported by Beecken et al. (2014) for passenger ships while accelerating ( no. kg). However, the RV Investigator measurements were undertaken whilst its engine was under 30 % load. Accelerating ships will typically be under higher engine loads and hence have a correspondingly higher EFPN (Westerlund et al., 2015), which explains part of this discrepancy. Furthermore, the RV Investigator has high efficiency engines and utilizes ultra-low sulfur diesel fuel. Studies have shown that similar diesel engines burning fuel of this type have lower EFPNs than the same engine with higher sulfur content diesel (Chu-Van et al., 2017). Similar quality fuels used in the ground transport industry have yielded similar values of EFPN, ranging from 4.8×1014 (25 % engine load) to 7.2 (100 % engine load) ×1015 no. kg (Jayaratne et al., 2009).
3.4 Instrumentation limitations
Lightweight UAVs have the potential to achieve aerial measurements at significantly less upfront and operational costs than fixed-wing and manned aerial vehicles. Lightweight UAVs can be deployed faster with limited or no required launch and landing area compared to their manned and fixed wing counterparts. Yet, their primary disadvantage, particularly in this application, is a severely limited payload weight. To overcome this limitation, this project used the lightweight and portable DISCmini and IAQ-calc sensors. However, these instruments have lower sensitivities and greater uncertainties when compared to a high accuracy CPC and CO2 monitor for measurements, which can influence results.
The DISCmini has a manufacturer-listed measurement cut-off size of 10 nm. A previous study listed in Table 3 (Lack et al., 2009) shows that the cut-off size of instruments used to measure PNC is directly linked to the value of EFPN, with the measured EFPN doubling when the cut-off size is changed from 13 to 5 nm due to the large number of particles in this size range. This may have been another contributing factor to the EFPN measured in this study being in the lower end of measured values in the literature.
The two 100 m transects were not accounted for in the final calculation of EFPN due to their poor Gaussian curve fits. Whilst this has been attributed to the skewing of the plume at this distance, the limitations of the instrumentation could also have contributed. The lower concentrations of CO2 at this distance result in the difference above background inside the plume being the same order of magnitude as the manufacturer-specified error margin. Hence, the variability in the plume either side of the central peak as shown in Fig. 4c could be due in part to instrumentation error.
Calibrations of sensors in this study were performed through comparison with reference instruments for ambient measurements at sea. Ideally, calibration should be performed with in-plume measurements to have the same environmental conditions and range as the real measurements. However, it was not possible to access the plume with reference instrumentation on board the ship. Whilst this study provides a successful proof of concept with consistent results over 2 days and several flights, a validation study is needed. This should include independent measurements of EFPN using other established methodologies to ascertain more precise correction factors and uncertainties.
The UAV system used in this study successfully measured PN and CO2 concentrations from the exhaust plume of the RV Investigator whilst operating at sea. Several different flight paths were tested and an optimal transect flying perpendicular to the plume at a distance of 20 m from the ship was adopted. The EFPN calculated for the RV Investigator was no. kg at a constant 30 % engine load. This EFPN was in agreement with values reported in the literature, indicating that this UAV-based system has potential for EFPN quantification pending further evaluation.
In comparison with other methods, UAV systems have the potential to provide cost-effective and accessible solutions for the rapid measurement and quantification of ship EFPNs. Its ability for deployment both in harbours and at sea, coupled with the possibility of altering its flight path to account for variances in wind conditions, gives this UAV system a high degree of flexibility. Furthermore, the UAV can sample considerably closer to the plume emission source than other methodologies, providing higher concentration measurements for the calculation of EFPN.
Whilst further validation is necessary, results presented here indicate that this UAV system has the potential to be used as a low cost tool for the quantification of ultrafine particle emission factors from commercial shipping. This is critical to improving our understanding of shipping's impact on climate and health.
4.1 Recommendations
The potential of this UAV system extends far beyond what is described here. This study is intended as both a proof of concept and to provide useful information both for the future of this project and any other UAV sampling systems being developed. The most significant improvement to the method described would be the use of a UAV with a lower minimum airspeed. This would allow for more data points per transect and would minimize the impact of potential outliers in instrumentation data. Other related improvements to this include the use of different sensors with higher response rates and additional flight path investigations to find an optimal transect distance which provides the broadest plume cross section without the plume becoming distorted and accuracy being impacted.
Further optimization of the transect approach is necessary in order to conduct UAV measurements of shipping emissions on a larger scale. After location of the plume the system could be set to make several repeat passes across the plume in rapid succession to increase the sample size. Another alternative would involve the UAV hovering inside the plume over a period of time collecting a continuous series of measurements from the centre of the plume. These methods would both require real-time sensor feedback to the UAV pilot and potentially adaptive autonomous controls to achieve a suitable result. Further challenges include operation under less favourable weather conditions, measurements in which the UAV is not launched from the ship itself and measurements taken for ships moving at full speed. This methodology could also be expanded to measure other important ship emission factors, including NOx and volatile organic compounds.
For access to data and further information on analysis, contact the corresponding author.
The supplement related to this article is available online at: https://doi.org/10.5194/amt-12-691-2019-supplement.
TFV conducted the research following the plan developed with the other authors and drafted the paper. RAB contributed to the data analysis and writing and revision of the paper. ERJ helped with the research design and the data analysis and contributed to the writing of the paper. LFG contributed to the flight requirements and to the writing and revision of the paper. LM contributed to the revision of the paper. ZDR directed the research project and contributed to the revision of the entire paper.
The authors declare that they have no conflict of interest.
The authors would like to acknowledge the ARCAA Operations Team (Dirk
Lessner, Gavin Broadbent) who operated the unmanned aerial vehicle (S800).
This research was supported by the Australian Research Council Discovery
Grant DP150101649 and the Marine National Facility. The authors would like
to thank the Captain and the crew of the RV Investigator as well as the
on-board MNF support staff, as without their support and effort, this research
would not have been possible.
Edited by: Andreas Richter
Reviewed by: three anonymous referees
Agrawal, H., Malloy, Q. G. J., Welch, W. A., Wayne Miller, J., and Cocker III, D. R.: In-use gaseous and particulate matter emissions from a modern ocean going container vessel, Atmos. Environ., 42, 5504–5510, https://doi.org/10.1016/j.atmosenv.2008.02.053, 2008.
Alföldy, B., Lööv, J. B., Lagler, F., Mellqvist, J., Berg, N., Beecken, J., Weststrate, H., Duyzer, J., Bencs, L., Horemans, B., Cavalli, F., Putaud, J.-P., Janssens-Maenhout, G., Csordás, A. P., Van Grieken, R., Borowiak, A., and Hjorth, J.: Measurements of air pollution emission factors for marine transportation in SECA, Atmos. Meas. Tech., 6, 1777–1791, https://doi.org/10.5194/amt-6-1777-2013, 2013.
Anderson, M., Salo, K., Hallquist, Å. M., and Fridell, E.: Characterization of particles from a marine engine operating at low loads, Atmos. Environ., 101, 65–71, https://doi.org/10.1016/j.atmosenv.2014.11.009, 2015.
Balzani Lööv, J. M., Alfoldy, B., Gast, L. F. L., Hjorth, J., Lagler, F., Mellqvist, J., Beecken, J., Berg, N., Duyzer, J., Westrate, H., Swart, D. P. J., Berkhout, A. J. C., Jalkanen, J.-P., Prata, A. J., van der Hoff, G. R., and Borowiak, A.: Field test of available methods to measure remotely SOx and NOx emissions from ships, Atmos. Meas. Tech., 7, 2597–2613, https://doi.org/10.5194/amt-7-2597-2014, 2014.
Beecken, J., Mellqvist, J., Salo, K., Ekholm, J., and Jalkanen, J.-P.: Airborne emission measurements of SO2 , NOx and particles from individual ships using a sniffer technique, Atmos. Meas. Tech., 7, 1957–1968, https://doi.org/10.5194/amt-7-1957-2014, 2014.
Berg, N., Mellqvist, J., Jalkanen, J.-P., and Balzani, J.: Ship emissions of SO2 and NO2: DOAS measurements from airborne platforms, Atmos. Meas. Tech., 5, 1085–1098, https://doi.org/10.5194/amt-5-1085-2012, 2012.
Blasco, J., Duran-Grados, V., Hampel, M., and Moreno-Gutierrez, J.: Towards an integrated environmental risk assessment of emissions from ships' propulsion systems, Environ. Int., 66, 44–47, https://doi.org/10.1016/j.envint.2014.01.014, 2014.
Brady, J. M., Stokes, M. D., Bonnardel, J., and Bertram, T. H.: Characterization of a Quadrotor Unmanned Aircraft System for Aerosol-Particle-Concentration Measurements, Environ. Sci. Technol., 50, 1376–1383, https://doi.org/10.1021/acs.est.5b05320, 2016.
Cappa, C. D., Williams, E. J., Lack, D. A., Buffaloe, G. M., Coffman, D., Hayden, K. L., Herndon, S. C., Lerner, B. M., Li, S.-M., Massoli, P., McLaren, R., Nuaaman, I., Onasch, T. B., and Quinn, P. K.: A case study into the measurement of ship emissions from plume intercepts of the NOAA ship Miller Freeman, Atmos. Chem. Phys., 14, 1337–1352, https://doi.org/10.5194/acp-14-1337-2014, 2014.
Chen, G., Huey, L. G., Trainer, M., Nicks, D., Corbett, J., Ryerson, T., Parrish, D., Neuman, J. A., Nowak, J., Tanner, D., Holloway, J., Brock, C., Crawford, J., Olson, J. R., Sullivan, A., Weber, R., Schauffler, S., Donnelly, S., Atlas, E., Roberts, J., Flocke, F., Hübler, G., and Fehsenfeld, F.: An investigation of the chemistry of ship emission plumes during ITCT 2002, J. Geophys. Res.-Atmos., 110, D10S90, https://doi.org/10.1029/2004JD005236, 2005.
Chu-Van, T., Ristovski, Z., Pourkhesalian, A. M., Rainey, T., Garaniya, V., Abbassi, R., Jahangiri, S., Enshaei, H., Kam, U. S., Kimball, R., Yang, L., Zare, A., Bartlett, H., and Brown, R. J.: On-board measurements of particle and gaseous emissions from a large cargo vessel at different operating conditions, Environ. Pollut., 237, 832–841, https://doi.org/10.1016/j.envpol.2017.11.008, 2017.
Cooper, D. A.: Exhaust emissions from high speed passenger ferries, Atmos. Environ., 35, 4189–4200, https://doi.org/10.1016/S1352-2310(01)00192-3, 2001.
Cooper, D. A.: HCB, PCB, PCDD and PCDF emissions from ships, Atmos. Environ., 39, 4901–4912, https://doi.org/10.1016/j.atmosenv.2005.04.037, 2005.
Corbett, J. J., and Farrell, A.: Mitigating air pollution impacts of passenger ferries, Transport Res. D-Tr. E., 7, 197–211, https://doi.org/10.1016/S1361-9209(01)00019-0, 2002.
Corbett, J. J. and Koehler, H. W.: Updated emissions from ocean shipping, J. Geophys. Res. Atmos., 108, https://doi.org/10.1029/2003JD003751, 2003.
Corbett, J. J., Winebrake, J. J., Green, E. H., Kasibhatla, P., Eyring, V., and Lauer, A.: Mortality from Ship Emissions: A Global Assessment, Environ. Sci. Technol., 41, 8512–8518, https://doi.org/10.1021/es071686z, 2007.
DJI S800-evo: http://www.dji.com/product/spreading-wings-s800-evo (last access: 6 February 2017), 2014.
Eyring, V., Köhler, H. W., van Aardenne, J., and Lauer, A.: Emissions from international shipping: 1. The last 50 years, J. Geophys. Res.-Atmos., 110, D17305, https://doi.org/10.1029/2004JD005619, 2005.
Fierz, M., Burtscher, H., Steigmeier, P., and Kasper, M.: Field measurement of particle size and number concentration with the Diffusion Size Classifier (DiSC), SAE Technical Paper, https://doi.org/10.4271/2008-01-1179, 2008.
Fuglestvedt, J., Berntsen, T., Eyring, V., Isaksen, I., Lee, D. S., and Sausen, R.: Shipping Emissions: From Cooling to Warming of Climate – and Reducing Impacts on Health, Environ. Sci. Technol., 43, 9057–9062, https://doi.org/10.1021/es901944r, 2009.
Gonzalez, F., Castro, M. P. G., Narayan, P., Walker, R., and Zeller, L.: Development of an autonomous unmanned aerial system to collect time-stamped samples from the atmosphere and localize potential pathogen sources, J. Field Robot., 28, 961–976, https://doi.org/10.1002/rob.20417, 2011.
Hak, C. S., Hallquist, M., Ljungström, E., Svane, M., and Pettersson, J. B. C.: A new approach to in-situ determination of roadside particle emission factors of individual vehicles under conventional driving conditions, Atmos. Environ., 43, 2481–2488, https://doi.org/10.1016/j.atmosenv.2009.01.041, 2009.
Hallquist, Å. M., Fridell, E., Westerlund, J., and Hallquist, M.: Onboard Measurements of Nanoparticles from a SCR-Equipped Marine Diesel Engine, Environ. Sci. Technol., 47, 773–780, https://doi.org/10.1021/es302712a, 2013.
Hobbs, P. V., Garrett, T. J., Ferek, R. J., Strader, S. R., Hegg, D. A., Frick, G. M., Hoppel, W. A., Gasparovic, R. F., Russell, L. M., and Johnson, D. W.: Emissions from ships with respect to their effects on clouds, J. Atmos. Sci., 57, 2570–2590, 2000.
Isakson, J., Persson, T. A., and Selin Lindgren, E.: Identification and assessment of ship emissions and their effects in the harbour of Göteborg, Sweden, Atmos. Environ., 35, 3659–3666, https://doi.org/10.1016/S1352-2310(00)00528-8, 2001.
Jayaratne, E. R., Ristovski, Z. D., Meyer, N., and Morawska, L.: Particle and gaseous emissions from compressed natural gas and ultralow sulphur diesel-fuelled buses at four steady engine loads, Sci. Total Environ., 407, 2845–2852, https://doi.org/10.1016/j.scitotenv.2009.01.001, 2009.
Jonsson, Å. M., Westerlund, J., and Hallquist, M.: Size-resolved particle emission factors for individual ships, Geophys. Res. Lett., 38, https://doi.org/10.1029/2011GL047672, 2011.
Juwono, A. M., Johnson, G. R., Mazaheri, M., Morawska, L., Roux, F., and Kitchen, B.: Investigation of the airborne submicrometer particles emitted by dredging vessels using a plume capture method, Atmos. Environ., 73, 112–123, https://doi.org/10.1016/j.atmosenv.2013.03.024, 2013.
Kasper, A., Aufdenblatten, S., Forss, A., Mohr, M., and Burtscher, H.: Particulate Emissions from a Low-Speed Marine Diesel Engine, Aerosol Sci. Tech., 41, 24–32, https://doi.org/10.1080/02786820601055392, 2007.
Lack, D., Lerner, B., Granier, C., Baynard, T., Lovejoy, E., Massoli, P., Ravishankara, A. R., and Williams, E.: Light absorbing carbon emissions from commercial shipping, Geophys. Res. Lett., 35, L13815, https://doi.org/10.1029/2008GL033906, 2008.
Lack, D. A., Corbett, J. J., Onasch, T., Lerner, B., Massoli, P., Quinn, P. K., Bates, T. S., Covert, D. S., Coffman, D., Sierau, B., Herndon, S., Allan, J., Baynard, T., Lovejoy, E., Ravishankara, A. R., and Williams, E.: Particulate emissions from commercial shipping: Chemical, physical, and optical properties, J. Geophys. Res.-Atmos., 114, D00F04, https://doi.org/10.1029/2008JD011300, 2009.
Lack, D. A., Cappa, C. D., Langridge, J., Bahreini, R., Buffaloe, G., Brock, C., Cerully, K., Coffman, D., Hayden, K., Holloway, J., Lerner, B., Massoli, P., Li, S.-M., McLaren, R., Middlebrook, A. M., Moore, R., Nenes, A., Nuaaman, I., Onasch, T. B., Peischl, J., Perring, A., Quinn, P. K., Ryerson, T., Schwartz, J. P., Spackman, R., Wofsy, S. C., Worsnop, D., Xiang, B., and Williams, E.: Impact of fuel quality regulation and speed reductions on shipping emissions: implications for climate and air quality, Environ. Sci. Technol., 45, 9052, https://doi.org/10.1021/es2013424, 2011.
Malaver Rojas, J. A., Gonzalez, L. F., Motta, N., Villa, T. F., Etse, V. K., and Puig, E.: Design and flight testing of an integrated solar powered UAV and WSN for greenhouse gas monitoring emissions in agricultural farms, International Conference on Intelligent Robots and Systems, Big Sky, Montana, USA, 2015 IEEE/RSJ, 1–6, 2015.
Mazaheri, M., Johnson, G. R., and Morawska, L.: Particle and Gaseous Emissions from Commercial Aircraft at Each Stage of the Landing and Takeoff Cycle, Environ. Sci. Technol., 43, 441–446, https://doi.org/10.1021/es8013985, 2009.
Mueller, L., Jakobi, G., Czech, H., Stengel, B., Orasche, J., Arteaga-Salas, J. M., Karg, E., Elsasser, M., Sippula, O., Streibel, T., Slowik, J. G., Prevot, A. S. H., Jokiniemi, J., Rabe, R., Harndorf, H., Michalke, B., Schnelle-Kreis, J., and Zimmermann, R.: Characteristics and temporal evolution of particulate emissions from a ship diesel engine, Appl. Energ., 155, 204–217, https://doi.org/10.1016/j.apenergy.2015.05.115, 2015.
Murphy, S., Agrawal, H., Sorooshian, A., Padró, L. T., Gates, H., Hersey, S., Welch, W. A., Jung, H., Miller, J. W., Cocker Iii, D. R., Nenes, A., Jonsson, H. H., Flagan, R. C., and Seinfeld, J. H.: Comprehensive simultaneous shipboard and airborne characterization of exhaust from a modern container ship at sea, Environ. Sci. Technol., 43, 4626–4640, https://doi.org/10.1021/es802413j, 2009.
NPRM 1309OS – Remotely Piloted Aircraft Systems: https://www.casa.gov.au/standard-page/nprm-1309os-remotely-piloted-aircraft-systems?WCMS\%3ASTANDARD\%3A\%3Apc=PC_102028 (last access: 12 November 2016), 2014.
Petzold, A., Hasselbach, J., Lauer, P., Baumann, R., Franke, K., Gurk, C., Schlager, H., and Weingartner, E.: Experimental studies on particle emissions from cruising ship, their characteristic properties, transformation and atmospheric lifetime in the marine boundary layer, Atmos. Chem. Phys., 8, 2387–2403, https://doi.org/10.5194/acp-8-2387-2008, 2008.
Petzold, A., Weingartner, E., Hasselbach, J., Lauer, P., Kurok, C., and Fleischer, F.: Physical properties, chemical composition, and cloud forming potential of particulate emissions from a marine diesel engine at various load conditions, Environ. Sci. Technol., 44, 3800–3805, https://doi.org/10.1021/es903681z, 2010.
Pirjola, L., Pajunoja, A., Walden, J., Jalkanen, J.-P., Rönkkö, T., Kousa, A., and Koskentalo, T.: Mobile measurements of ship emissions in two harbour areas in Finland, Atmos. Meas. Tech., 7, 149–161, https://doi.org/10.5194/amt-7-149-2014, 2014.
Reda, A. A., Schnelle-Kreis, J., Orasche, J., Abbaszade, G., Lintelmann, J., Arteaga-Salas, J. M., Stengel, B., Rabe, R., Harndorf, H., Sippula, O., Streibel, T., and Zimmermann, R.: Gas phase carbonyl compounds in ship emissions: Differences between diesel fuel and heavy fuel oil operation, Atmos. Environ., 112, 370–380, https://doi.org/10.1016/j.atmosenv.2015.03.057, 2015.
Ristovski, Z. D., Miljevic, B., Surawski, N. C., Morawska, L., Fong, K. M., Goh, F., and Yang, I. A.: Respiratory health effects of diesel particulate matter, Respirology, 17, 201–212, https://doi.org/10.1111/j.1440-1843.2011.02109.x, 2012.
Sinha, P., Hobbs, P. V., Yokelson, R. J., Christian, T. J., Kirchstetter, T. W., and Bruintjes, R.: Emissions of trace gases and particles from two ships in the southern Atlantic Ocean, Atmos. Environ., 37, 2139–2148, https://doi.org/10.1016/S1352-2310(03)00080-3, 2003.
Streets, D. G., Carmichael, G. R., and Arndt, R. L.: Sulfur dioxide emissions and sulfur deposition from international shipping in Asian waters, Atmos. Environ., 31, 1573–1582, https://doi.org/10.1016/S1352-2310(96)00204-X, 1997.
UNCTAD: Review of Maritime Transport 2015, United Nations Conference on Trade and Development UNCTAD, available at: https://unctad.org/en/PublicationsLibrary/rmt2015_en.pdf (last access: 12 November 2016), 2015.
Viana, M., Hammingh, P., Colette, A., Querol, X., Degraeuwe, B., Vlieger, I. d., and Van Aardenne, J.: Impact of maritime transport emissions on coastal air quality in Europe, Atmos. Environ., 90, 96–105, https://doi.org/10.1016/j.atmosenv.2014.03.046, 2014.
Westerlund, J., Hallquist, M., and Hallquist, Å. M.: Characterization of fleet emissions from ships through multi-individual determination of size-resolved particle emissions in a coastal area, Atmos. Environ., 112, 159–166, https://doi.org/10.1016/j.atmosenv.2015.04.018, 2015.
WHO Regional Office for Europe: Review of evidence on health aspects of air pollution – REVIHAAP Project: Technical Report, Copenhagen: WHO Regional Office for Europe, available at: https://www.ncbi.nlm.nih.gov/books/NBK361805/ (last access: 10 November 2016). 2013.
Williams, E. J., Lerner, B. M., Murphy, P. C., Herndon, S. C., and Zahniser, M. S.: Emissions of NOx, SO2, CO, and HCHO from commercial marine shipping during Texas Air Quality Study (TexAQS) 2006, J. Geophys. Res.-Atmos., 114, D21306, https://doi.org/10.1029/2009JD012094, 2009.
Winnes, H., Moldanová, J., Anderson, M., and Fridell, E.: On-board measurements of particle emissions from marine engines using fuels with different sulphur content, P. I. Mech. Eng. M-J. Eng., 230, 45–54, https://doi.org/10.1177/1475090214530877, 2016.