the Creative Commons Attribution 4.0 License.
the Creative Commons Attribution 4.0 License.
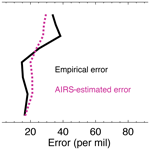
Comparison of optimal estimation HDO∕H2O retrievals from AIRS with ORACLES measurements
Robert L. Herman
John Worden
David Noone
Dean Henze
Kevin Bowman
Karen Cady-Pereira
Vivienne H. Payne
Susan S. Kulawik
Dejian Fu
In this paper we evaluate new retrievals of the deuterium content of water vapor from the Aqua Atmospheric InfraRed Sounder (AIRS), with aircraft measurements of HDO and H2O from the ObseRvations of Aerosols above Clouds and their intEractionS (ORACLES) field mission. Single-footprint AIRS radiances are processed with an optimal estimation algorithm that provides vertical profiles of the HDO∕H2O ratio, characterized uncertainties and instrument operators (i.e., averaging kernel matrix). These retrievals are compared to vertical profiles of the HDO∕H2O ratio from the Oregon State University Water Isotope Spectrometer for Precipitation and Entrainment Research (WISPER) on the ORACLES NASA P-3B Orion aircraft. Measurements were taken over the southeastern Atlantic Ocean from 31 August to 25 September 2016. HDO∕H2O is commonly reported in δD notation, which is the fractional deviation of the HDO∕H2O ratio from the standard reference ratio. For collocated measurements, the satellite instrument operator (averaging kernels and a priori constraint) is applied to the aircraft profile measurements. We find that AIRS δD bias relative to the aircraft is well within the estimated measurement uncertainty. In the lower troposphere, 1000 to 800 hPa, AIRS δD bias is −6.6 ‰ and the root-mean-square (rms) deviation is 20.9 ‰, consistent with the calculated uncertainty of 19.1 ‰. In the mid-troposphere, 800 to 500 hPa, AIRS δD bias is −6.8 ‰ and rms 44.9 ‰, comparable to the calculated uncertainty of 25.8 ‰.
- Article
(5637 KB) - Full-text XML
-
Supplement
(2291 KB) - BibTeX
- EndNote
The deuterium content of tropospheric water vapor is sensitive to the different types of atmospheric moisture sources, such as evaporation from the ocean or land, and the processing that occurs during transport, such as mixing or condensation (e.g., Craig, 1961; Dansgaard, 1964; Galewsky et al., 2016). Condensation and precipitation preferentially remove the heavier HDO isotopologue from the gas phase relative to the parent isotopologue H2O, whereas evaporation of precipitation at lower altitudes in the atmosphere can enrich HDO relative to H2O vapor. These unique, isotopic properties allow the HDO∕H2O ratio to be a tracer for the origin, condensation and evaporation history of an air parcel, and thus be useful for evaluating changes to the water cycle (e.g., Worden et al., 2007; Noone, 2012; Galewsky et al., 2016).
Early remote sensing of atmospheric HDO was made by the ATMOS (Atmospheric Trace Molecule Spectroscopy) mission on the Space Shuttle (Rinsland et al., 1991; Irion et al., 1996; Moyer et al., 1996; Kuang et al., 2003), retrieving in the upper troposphere–lower stratosphere. Global stratospheric HDO measurements have been provided by satellite instruments, including Envisat/MIPAS (Michelson Interferometer for Passive Atmospheric Sounding) (Steinwagner et al., 2007, 2010; Lossow et al., 2011), Odin/SMR (Sub-Millimetre Radiometer) (Murtagh et al., 2002; Urban et al., 2007) and SCISAT-1 (Scientific Satellite)/ACE-FTS (Atmospheric Chemistry Experiment Fourier transform spectrometer) (Bernath et al., 2005; Nassar et al., 2007; Lossow et al., 2011; Randel et al., 2012). Atmospheric columns densities of HDO and H2O have been retrieved from Sentinel-5 Precursor/TROPOMI (Tropospheric Monitoring Instrument) (Schneider et al., 2020).
In the last decade, satellite retrievals of tropospheric water vapor isotopic composition (HDO and H2O) have been developed, including Envisat/SCIAMACHY (Scanning Imaging Absorption Spectrometer for Atmospheric Chartography) (Frankenberg et al., 2009), IASI (Infrared Atmospheric Sounding Interferometer) aboard the MetOp satellites (Herbin et al., 2009; Schneider and Hase, 2011; Lacour et al., 2012) and TES (the Tropospheric Emission Spectrometer) on the Aura spacecraft (Worden et al., 2006, 2007). More recently, Worden et al. (2019) developed HDO retrievals from the Aqua Atmospheric InfraRed Sounder (AIRS) single-footprint Level 1B radiance data. These AIRS retrievals are the subject of the present comparison with aircraft data.
Satellite HDO measurements have been utilized to study tropical carbon–water feedbacks (Wright et al., 2017), moist processes in deep convection (e.g., Worden et al., 2007) and the global partitioning of transpiration to evapotranspiration (Good et al., 2015). A decadal record of HDO has promise for characterizing global shifts in moisture sources and atmospheric water balance in response to warming, climactic variability, and land use. For example, Bailey et al. (2017) show that a record of free tropospheric HDO∕H2O would provide an observational constraint on changes in the tropical water balance (evaporation minus precipitation) in response to shifts in ocean temperature. Wright et al. (2017) also show that free tropospheric deuterium measurements provide a fundamental new constraint in carbon–water dynamics in the Amazon. They use the TES isotope measurements to show that dry-season evapotranspiration is critical for initiating southern Amazon rainfall, which in turn is critical towards sustaining the Amazon rainforest (R. Fu et al., 2013). For these reasons a record of the deuterium content of water vapor from the long (17 years and continuing) record from AIRS holds significant potential to evaluate changes in the global water cycle.
This paper presents detailed comparisons between new AIRS measurements of the deuterium content of water vapor (or HDO∕H2O ratio) and accurate in situ HDO∕H2O measurements from an aircraft sensor during the NASA ObseRvations of Aerosols above Clouds and their intEractionS (ORACLES) field mission. In this paper, we denote the volume mixing ratios qD for HDO and qH for H2O. By standard convention, we report the isotopic abundance as δD (per mil or ‰) , where , based on the HDO∕H2O standard ratio for Vienna Standard Mean Ocean Water (SMOW).
2.1 AIRS instrument description
The Atmospheric InfraRed Sounder (AIRS) on the NASA Aqua satellite is a nadir-viewing, scanning thermal infrared grating spectrometer that covers the 3.7 to 15.4 µm spectral range with 2378 spectral channels (Pagano et al., 2003, Aumann et al., 2003). Launched on 4 May 2002, Aqua is in a sun-synchronous orbit at 705 km, with an approximately 13:30 UTC Equator crossing time, as part of the A-Train satellite constellation. AIRS continues to make daily measurements of most of the globe with its wide cross-scanning swath of coverage. For HDO retrievals, the single-footprint AIRS Level 1B (L1B) radiances are utilized. These footprint observations have a horizontal resolution of approximately 13.5 km at nadir. Absolute radiometric accuracy between 220 and 320 K at all observation angles is better than 0.2 K (Pagano et al., 2003, 2008). The algorithm applied to AIRS radiances to yield HDO is described below in Sect. 3.1.
2.2 WISPER system for aircraft measurements
Aircraft measurements were made on the NASA P-3B Orion aircraft during the NASA ORACLES field mission. ORACLES is a 5-year Earth Venture Suborbital (EVS-2) investigation with three Intensive Observation Periods (IOPs) designed to study key processes that determine the climate impacts of African biomass burning aerosols in 2016, 2017 and 2018. The ORACLES experiment provided multiyear airborne observations from the NASA P-3B Orion and ER-2 aircraft over the complete vertical column of the key parameters that drive aerosol–cloud interactions in the southeastern Atlantic Ocean region. The focus of the ORACLES field measurements was a biomass burning plume that advected west from the African continent to the Atlantic Ocean at 2 to 5 km altitude above sea level (a.s.l.). Here we use data from the ORACLES 2016 IOP (ORACLES Science Team, 2017), and report on aircraft versus satellite comparisons from eight flights (Fig. 1 and Table 1).
Table 1Summary of matches of aircraft WISPER δD measurements during NASA ORACLES* with collocated AIRS observations. The loose latitude–longitude constraint (second column) is that all AIRS geolocations within the same rectangle of maximum to minimum latitude and maximum to minimum longitude are selected for an aircraft vertical profile (∼100 to 300 km in length). The tighter latitude–longitude constraint (third column) is to match only AIRS geolocations within 0.3∘ (30 km) of the aircraft flight track.
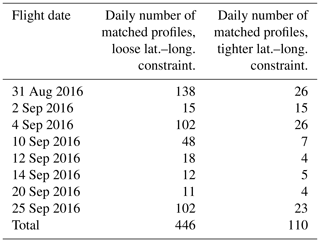
* NASA ORACLES is the “ObseRvations of Aerosols above Clouds and their intEractionS” Earth Venture Suborbital Mission.
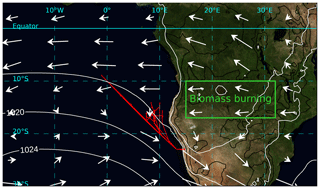
Figure 1Selected flight tracks (red lines) of the NASA P-3B Orion aircraft during the ORACLES 2016 IOP used in this study, with corresponding flight dates listed in Table 1. The map is the NASA new blue marble, a true-color Earth image from Stockli et al. (2011), ©NASA. Superimposed on the map are the September 2016 monthly mean 700 hPa winds (white vectors) and surface pressure (white isobars), along with the approximate biomass burning region (green rectangle).
Water vapor isotopic abundances (HDO∕H2O and ) were measured in situ on the aircraft with the Oregon State Water WISPER system (Water Isotope Spectrometer for Precipitation and Entrainment Research, Henze et al., 2020; Henze and Noone, 2017), which uses a modified commercial Picarro L2120-i δD/δ18O Ultra-High-Precision Isotopic Water Analyzer. The measurement technique is cavity ring-down (CRD) spectroscopy (O'Keefe and Deacon, 1988; Berden et al., 2000; Gupta et al., 2009). The majority of measurements analyzed in this paper are located within the biomass burning plume, characterized by elevated H2O (approximately 6000 ppmv) and elevated δD (−100 ‰ to −70 ‰). At these abundances of HDO∕H2O, the 1 Hz precision (1σ) of the measurements of δD is ±3 ‰ and the accuracy is ±6.5 ‰.
3.1 Retrieval algorithm
The single-footprint AIRS HDO profile data used in this work were produced using the retrieval algorithm, named the MUlti-SpEctra, MUlti-SpEcies, MUlti-Sensors (MUSES) algorithm (D. Fu et al., 2013, 2016, 2018, 2019; Worden et al., 2019). The MUSES algorithm can use radiances from multiple instruments, including AIRS and other instruments (CrIS, TES, OMI, OMPS, TROPOMI, and MLS), to quantify geophysical observables that affect the corresponding radiance. The AIRS single-footprint HDO profile retrievals have been described by Worden et al. (2019), and have heritage from the TES algorithm (Worden et al., 2004, 2006, 2007, 2011, 2012, 2013; Bowman et al., 2006, 2002). The Optimal Spectral Sampling (OSS) fast radiative transfer model (Moncet et al., 2008, 2015) for single-footprint AIRS measurements has been integrated into the MUSES algorithm, in support of the operational data production towards the multi-decadal record of global HDO profiles. The Supplement discusses the sensitivity of the retrievals to the choice of the forward model. The retrieval uses the optimal estimation (OE) method to quantify atmospheric HDO and H2O (Worden et al., 2006, 2012, 2019). For both AIRS and TES retrievals, height discrimination of the HDO∕H2O ratio in the troposphere is provided by spectral resolution of pressure-broadened and the temperature-broadened absorption features of their corresponding lines (Beer et al., 2002). The algorithms and spectral microwindows are described by Worden et al. (2019). Chemical species CH4, CO, HDO, and H2O are jointly retrieved along with atmospheric temperature, surface temperature, land emissivity and clouds (Worden et al., 2012). The retrieval optimizes the ratio of HDO to H2O, as opposed to either HDO or H2O alone (Worden et al., 2019, 2012, 2006). AIRS radiances at wavelengths from 8 to 12 µm are used here, excluding the 9.6 µm ozone band. The parent molecule H2O is retrieved at both 8 and 12 µm, but HDO is retrieved primarily from strong absorption lines in the 8 µm region (particularly in the wavenumber range 1210 to 1270 cm−1). Cloud optical depth and cloud top pressure are jointly retrieved with the chemical species, using the approach described in Kulawik et al. (2006). The cloud-clearing approach (Susskind et al., 2006), utilized in AIRS operational products up to and including AIRS version 6, where retrievals are reported on the 45 km Advanced Microwave Sounding Unit (AMSU) footprint, is not utilized here. As described by Worden et al. (2019), retrievals are performed on single AIRS 13.5 km footprints in order to preserve the Level 1B radiance noise characteristics (Irion et al., 2018; DeSouza-Machado et al., 2018).
For H2O, the a priori constraint vectors come from NASA's Goddard Earth Observing System (GEOS) data assimilation system version 5.12.4 processing stream (Rienecker et al., 2008). These are produced by the Global Modeling and Assimilation Office (GMAO) at the NASA Goddard Space Flight Center (GSFC). The GMAO GEOS-5.12.4 water mixing ratios are linearly interpolated to the latitudes, longitudes and log(pressure) levels of the satellite retrievals to generate the a priori profiles.
For all HDO retrievals, the initial profile of the isotopic ratio is set equal to a simulated tropical profile (Worden et al., 2006). In the AIRS HDO product files, a priori HDO is defined as the product of the local a priori H2O profile (GMAO GEOS-5.12.4) and one tropical a priori profile of the HDO∕H2O isotopic ratio (Worden et al., 2006). The initial guess profiles for H2O are set equal to the a priori.
3.2 Method of comparison
The AIRS HDO∕H2O retrievals are matched up in space and time with the aircraft in situ HDO∕H2O measurements. A critical aspect of validating satellite retrievals is obtaining data that span the altitudes where the satellite has sensitivity to HDO∕H2O. AIRS data are sensitive to the HDO∕H2O ratio in the atmosphere from the surface up to approximately 10 000 m altitude. The aircraft samples HDO and H2O from the surface up to 6000 m altitude, spanning most of the altitudes where the AIRS data are sensitive and therefore allows us to validate AIRS HDO∕H2O with in situ measurements.
For direct comparison of AIRS HDO∕H2O with in situ HDO∕H2O, the AIRS instrument operator (averaging kernel and a priori constraint) is applied to the in situ data (see Eq. 1 below), as described by Rodgers (2000). This has the effect of smoothing the in situ data to the same resolution as the satellite retrievals. The averaging kernel matrix A is the sensitivity of the AIRS estimate to the true concentration in the atmosphere (Rodgers, 2000). The in situ profile with applied averaging kernel xinsituw∕AK is calculated jointly for HDO and H2O using the AIRS operator:
Joint HDO∕H2O retrievals are performed on the logarithm of the volume mixing ratios, xD=ln (qD) and xH=ln (qH) (Worden et al., 2012, 2006). The data structure for AIRS HDO files is similar to TES HDO, with details provided by Herman et al. (2014).
For comparison with AIRS, the in situ HDO and H2O profiles are extended to cover the full range of AIRS levels. In the boundary layer, from the surface up to the lowest-altitude aircraft data, we assume constant values of HDO and H2O set equal to the first aircraft measurement. In the range of aircraft data (up to 6000 m flight ceiling), the aircraft in situ HDO and H2O data are interpolated to the levels of the AIRS forward model, smoothing fine-scale features. In the layers above the aircraft maximum altitude, the profile is extrapolated using a scaled a priori profile. In this paper, all comparisons have been completed by applying Eq. (1).
Validating the accuracy of AIRS HDO and H2O retrievals is important for studies of the hydrologic cycle, exchange processes in the troposphere and climate change. Comparisons of AIRS and TES over 5 years (2006–2010) indicate that the retrieval characteristics of the AIRS HDO∕H2O measurements have similar vertical resolution and uncertainty in the middle troposphere but slightly less sensitivity in the lower troposphere (Worden et al., 2019). Worden et al. (2019) reported that the calculated uncertainty of AIRS HDO∕H2O is ∼30 ‰ for a tropospheric average between 750 and 350 hPa, with a mean bias between TES and AIRS (TES minus AIRS) for the HDO∕H2O ratio of ‰ and a latitudinal variation of ∼7.6 ‰.
4.1 Comparison of AIRS with aircraft measurements
ORACLES 31/08/2016 to 25/9/2016 data comparison
In this section, we describe comparisons between AIRS and ORACLES aircraft HDO measurements. First, time segments of each aircraft flight are identified where the aircraft profiled from the boundary layer up to approximately 6000 m altitude. To minimize the impact of atmospheric spatial and temporal variability, same-day AIRS measurements are selected for the same latitude–longitude rectangle as each aircraft profile (Fig. 2). These matched pairs are compared by the method described in Sect. 3.2. The loose constraint (Table 1, column 2; Fig. 2, open circles; and Fig. 4a) is that, for an aircraft vertical profile (∼100 to 300 km in length), all AIRS geolocations within the same rectangle of maximum to minimum latitude and maximum to minimum longitude are selected. The only exceptions were the aircraft flights of 2 and 14 September 2016, which had different flight patterns and used smaller shapes to constrain AIRS geolocations. The tighter constraint (Table 1, column 3; Fig. 2, closed circles; and Fig. 4b) is to match only AIRS geolocations within 0.3∘ (30 km) of the aircraft flight track. The standard data retrieval quality flags for the retrieval are used in this analysis, which are based on the Aura TES data retrieval quality flags (Herman and Kulawik, 2018). For closer spatial coincidence, we also selected AIRS–aircraft measurement pairs within 0.3∘ (Fig. 2). Following Worden et al. (2007) and Brown et al. (2008), we filter data for a reasonable threshold of standard nadir data product degrees of freedom for signal (DOFS) > 1.1 but include all values of average cloud effective optical depth. The data product DOFS is the trace of the averaging kernel, and is a measure of the number of independent parameters for the retrieved HDO∕H2O profile. Average cloud effective optical depth is the retrieved cloud mean optical depth at wavenumbers from 975 to 1200 cm−1 from the final retrieval step (i.e., the same for all species) (Kulawik et al., 2006). Figure 3a shows a representative 31 August 2016 comparison between aircraft water vapor δD from WISPER and the coincident AIRS retrieval. Figure 3b shows the corresponding averaging kernels.
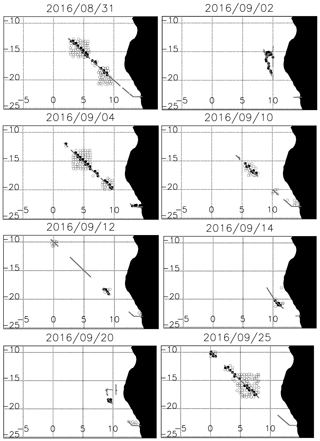
Figure 2ORACLES aircraft profiles (thin grey line segments) over the southeastern Atlantic Ocean to the west of Africa are matched to AIRS fields of view (FOVs) with a loose spatial match (open circles) and tighter spatial match within 30 km (closed circles) for eight flights in 2016.
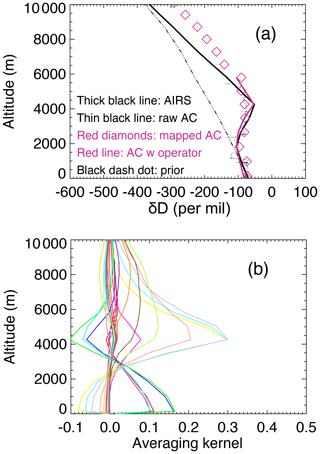
Figure 3(a) Sample comparison of the δD profiles by aircraft and satellite over the southeastern Atlantic Ocean during ORACLES on 31 August 2016: shown are AIRS δD (thick black line), the prior δD (dashed–dotted black line), nearest WISPER δD (thin black line), WISPER δD interpolated or extrapolated to satellite levels (red diamonds), and the WISPER δD with the AIRS operator (averaging kernel) applied (thick red line). (b) Averaging kernel corresponding to same AIRS profile on 31 August 2016, color-coded by pressure level. Averaging kernels with the largest positive sensitivity below 2000 m are from the lowest altitudes.
4.2 AIRS bias correction
TES HDO∕H2O ratios are biased compared to model and in situ measurements (Worden et al., 2006, 2007, 2011). We assess whether AIRS HDO has a bias relative to in situ measurements. As described above, AIRS and TES show a small bias for the HDO∕H2O ratio of ‰ (Worden et al., 2019) after a bias correction is applied, so it is reasonable to see how well in situ and AIRS data agree if the TES bias correction is applied to the AIRS HDO. Herman et al. (2014) estimated the TES bias δbias by minimizing the difference between bias-corrected TES and in situ δD with TES operator applied:
We apply the TES δbias to the AIRS data to evaluate against ORACLES aircraft data. There are 446 matched profiles of AIRS and ORACLES within the same latitude–longitude boxes and 110 closely matched profiles within 0.3∘ or approximately 30 km (Fig. 2). Comparisons with an averaging kernel applied are shown in Fig. 4 and Table 2. Over the range of aircraft data, 0 to 6 km altitude, AIRS δD has a mean bias of −6.7 ‰ relative to the aircraft profiles, well within the estimated measurement uncertainty of both AIRS and the WISPER calibration. This is consistent with TES δD (Worden et al., 2019; Herman et al., 2014). AIRS lower-troposphere δD bias is −6.6 ‰ and rms 20.9 ‰ (surface to 800 hPa). In the mid-troposphere, 800 to 500 hPa, AIRS δD bias is −6.8 ‰ and rms 44.9 ‰.
Table 2Summary of satellite–aircraft comparisons for 110 matched pairs in 2016 (Fig. 2, closed circles). Bias and rms (standard deviation) of AIRS δD relative to ORACLE aircraft, with averaging kernel applied (“BiasAK”, “rmsAK”), and for AIRS relative to mapped ORACLES aircraft, with no averaging kernel (“Bias”, “rms”). The reported rms here is the standard deviation, not including the bias.
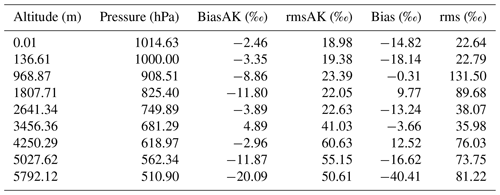
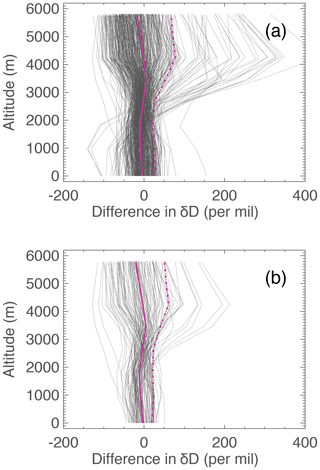
Figure 4(a) AIRS minus ORACLES aircraft δD for the 446 matches within the loose spatial matching constraint (Fig. 2, open circles). Lines are individual profiles (black lines), mean (solid red line) and rms (dashed–dotted red line). (b) AIRS minus ORACLES aircraft δD for the 110 matches within 0.3∘ (Fig. 2, closed circles).
In this section we characterize the a posteriori error budget for AIRS HDO∕H2O and assess this error by comparison with the ORACLES aircraft measurements. Error analysis in OE has been described in detail in the literature (Worden et al., 2004, 2006; Bowman et al., 2006; Rodgers, 2000). The error in the estimate of HDO∕H2O is defined as the true state x minus the linear estimate retrieved by AIRS (e.g., Worden et al., 2006, Eq. 15):
Similar to Herman et al. (2014), we define the estimated error of the AIRS isotopic ratio HDO∕H2O, (Eq. 4) as the observation error covariance (Worden et al., 2006):
where is the gain matrix of the HDO∕H2O retrieval, Sn is the measurement error covariance, and is the error covariance due to systematic errors and interference errors. Interference errors are due to CH4, N2O, surface emissivity, effects of temperature and clouds. The estimated error is given by the square roots of the diagonal elements of S, the best estimate of the AIRS observation error covariance for the HDO∕H2O retrieval.
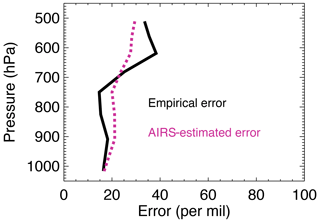
Figure 5Plot of AIRS error analysis for coincident AIRS and ORACLES δD on 31 August 2016, which shows that the empirical error is comparable to the AIRS-estimated error. The empirical error is obtained from the statistics of the satellite–aircraft comparison, while the estimated error is obtained from optimal estimation retrieval theory. Plotted here are the AIRS δD-estimated error, also known as AIRS observation error (dashed red line), and the AIRS δD empirical error (black line).
The estimated error (Eq. 4) is compared to the empirical error calculated from the AIRS–aircraft comparisons. It is seen that the error varies from ∼20 ‰ to ∼40 ‰ (Fig. 5). The empirical error (AIRS versus aircraft rms) is similar in magnitude to the estimated error but exceeds the estimated error at 500 to 600 hPa in the free troposphere. These differences between the OE-estimated error and the empirical error are likely due to uncertainties in atmospheric variability in space and time and in the collocation between satellite retrieval and aircraft measurements. The instrument operator (Eq. 1) accounts for error due to the mismatch in “vertical” sensitivity between the satellite retrieval and aircraft in situ vertical profiling. In the cases where AIRS is compared to in situ measurements without the instrument operator, there is an additional smoothing error (Table 2). The instrument operator does not account for error due to “horizontal” mismatch. The close coincidences are all within 30 km (0.3∘), but given the time differences, AIRS 15 km nadir footprint and limited in situ measurement, the satellite and aircraft are not necessarily measuring the same air mass. There is a collocation error on the order of ∼10 ‰ due to horizontal collocation and representativeness uncertainty.
HDO∕H2O estimates from AIRS single-footprint radiances been compared to coincident in situ airborne measurements on the P-3B Orion aircraft by the Oregon State Water WISPER system over the southeastern Atlantic Ocean. On 8 d between 31 August and 25 September 2016, there are collocated measurements between AIRS and the P-3B aircraft. We have shown that AIRS-only retrievals have sensitivity to HDO from the middle troposphere to the lower troposphere. We demonstrate that AIRS δD has a mean bias of −6.7 ‰ relative to aircraft, well within the estimated measurement uncertainty. In the lower troposphere, 1000 to 800 hPa, AIRS δD bias is −6.6 ‰ and the rms 20.9 ‰, consistent with the calculated uncertainty of 19.1 ‰. In the mid-troposphere, 800 to 500 hPa, AIRS δD bias is −6.8 ‰ and rms 44.9 ‰, comparable to the calculated uncertainty of 25.8 ‰. The errors are sufficiently small that the AIRS HDO∕H2O ratio retrievals are useful for scientific analysis. This long-term global data record has considerable potential utility.
The AIRS HDO data used in this analysis are publicly available from the following link: https://avdc.gsfc.nasa.gov/pub/data/satellite/Aura/TES/.AIRS/ (Worden et al., 2019, last access: 27 March 2020). The ORACLES aircraft data used in the data analysis can be freely downloaded from the following Digital Object Identifier: (https://doi.org/10.5067/Suborbital/ORACLES/P3/2016_V1 ORACLES Science Team, 2017, last access: 22 April 2017). Files in Interactive Data Language (IDL) format of the AIRS data shown and the forward model output are available from coauthor John Worden upon request: john.r.worden@jpl.nasa.gov.
The supplement related to this article is available online at: https://doi.org/10.5194/amt-13-1825-2020-supplement.
RLH carried out all steps of aircraft validation, from matching data and quality filtering to applying observation operator and statistics, while JW provided satellite-to-satellite validation. JW developed the retrieval strategies for both AIRS and TES HDO∕H2O retrievals. DF and SSK built the strategies of single AIRS footprint HDO∕H2O retrievals into the MUSES algorithm. KCP, RLH and VHP evaluated the sensitivities of retrievals to the choice of forward model. RLH, VHP, JW, SSK, DF, DN, DH and KB contributed to the text and interpretation of the results. JW and SSK helped in the estimation of HDO∕H2O measurement uncertainty, quality flagging and knowledge of the retrieval process. DN and DH provided ORACLES data, aircraft measurement uncertainty, and identified profiles in the aircraft data. All authors participated in writing the manuscript.
The authors declare that they have no conflict of interest.
This article is part of the special issue “New observations and related modelling studies of the aerosol–cloud–climate system in the Southeast Atlantic and southern Africa regions (ACP/AMT inter-journal SI)”. It is not associated with a conference.
Support for Robert L. Herman, John Worden, Kevin Bowman, Karen Cady-Pereira, Vivienne H. Payne, Susan S. Kulawik and Dejian Fu was provided by the NASA Aura Program. Part of the research described in this paper was carried out by the Jet Propulsion Laboratory, California Institute of Technology, under a contract with NASA.
This research was supported by the NASA Aura Tropospheric Emission Spectrometer (TES) Project. Participation by David Noone and Dean Henze was supported by the National Science Foundation Climate and Large-scale Dynamics and Atmospheric Chemistry programs (grant no. AGS 1564670).
This paper was edited by Joanna Joiner and reviewed by four anonymous referees.
Aumann, H. H., Chahine, M. T., Gautier, C., Goldberg, M. D., Kalnay, E., McMillin, L. M., Revercomb, H., Rosenkranz, P. W., Smith, W. L., Staelin, D. H., Strow, L. L., and Susskind, J.: AIRS/AMSU/HSB on the aqua mission: Design, science objectives, data products, and processing systems, IEEE T. Geosci. Remote, 41, 253–264, 2003.
Bailey, A., Blossey, P. N., Noone, D., Nusbaumer, J., and Wood, R.: Detecting shifts in tropical moisture imbalances with satellite-derived isotope ratios in water vapor, J. Geophys. Res.-Atmos., 122, 5763–5779, 2017.
Beer, R., Bowman, K. W., Brown, P. D., Clough, S. A., Eldering, A., Goldman, A., Jacob, D. J., Lampel, M., Logan, J. A., Luo, M., Murcray, F. J., Osterman, G. B., Rider, D. M., Rinsland, C. P., Rodgers, C. D., Sander, S. P., Shephard, M., Sund, S., Ustinov, E. A., Worden, H. M., Worden, J., and Syvertson, M. (Eds.): Tropospheric Emission Spectrometer (TES) Level 2 Algorithm Theoretical Basis Document, V. 1.16, Jet Propulsion Laboratory, Pasadena, CA, JPL D-16474, 27 June 2002, available at: http://eospso.gsfc.nasa.gov/atbd-category/53 (last access: 7 December 2010), 2002.
Berden, G., Peeters, R., and Meijer, G.: Cavity ring-down spectroscopy: Experimental schemes and applications, Int. Rev. Phys. Chem., 19, 565–607, https://doi.org/10.1080/014423500750040627, 2000.
Bernath, P. F., McElroy, C. T., Abrams, M. C., Boone, C. D., Butler, M., Camy-Peyret, C., Carleer, M., Clerbaux, C., Coheur, P.-F., Colin, R., DeCola, P., DeMazière, M., Drummond, J. R., Dufour, D., Evans, W. F. J., Fast, H., Fussen, D., Gilbert, K., Jennings, D. E., Llewellyn, E. J., Lowe, R. P., Mahieu, E., McConnell, J. C., McHugh, M., McLeod, S. D., Michaud, R., Midwinter, C., Nassar, R., Nichitiu, F., Nowlan, C., Rinsland, C. P., Rochon, Y. J., Rowlands, N., Semeniuk, K., Simon, P., Skelton, R., Sloan, J. J., Soucy, M.-A., Strong, K., Tremblay, P., Turnbull, D., Walker, K. A., Walkty, I., Wardle, D. A., Wehrle, V., Zander, R., and Zou, J.: Atmospheric Chemistry Experiment (ACE): Mission overview, Geophys. Res. Lett., 32, L15S01, https://doi.org/10.1029/2005GL022386, 2005.
Bowman, K. W., Worden, J., Steck, T., Worden, H. M., Clough, S., and Rodgers, C.: Capturing time and vertical variability of tropospheric ozone: A study using TES nadir retrievals, J. Geophys. Res.-Atmos., 107, 4723, https://doi.org/10.1029/2002JD002150, 2002.
Bowman, K. W., Rodgers, C. D., Kulawik, S. S., Worden, J., Sarkissian, E., Osterman, G., Steck, T., Luo, M., Eldering, A., Shephard, M. W., Worden, H., Lampel, M., Clough, S. A., Brown, P., Rinsland, C., Gunson, M., and Beer, R.: Tropospheric Emission Spectrometer: retrieval method and error analysis, IEEE T. Geosci. Remote, 44, 1297–1307, 2006.
Boxe, C. S., Worden, J. R., Bowman, K. W., Kulawik, S. S., Neu, J. L., Ford, W. C., Osterman, G. B., Herman, R. L., Eldering, A., Tarasick, D. W., Thompson, A. M., Doughty, D. C., Hoffmann, M. R., and Oltmans, S. J.: Validation of northern latitude Tropospheric Emission Spectrometer stare ozone profiles with ARC-IONS sondes during ARCTAS: sensitivity, bias and error analysis, Atmos. Chem. Phys., 10, 9901–9914, https://doi.org/10.5194/acp-10-9901-2010, 2010.
Brown, D., Worden, J., and Noone, D.: Comparison of atmospheric hydrology over convective continental regions using water vapor isotope measurements from space, J. Geophys. Res.-Atmos., 113, D15124, https://doi.org/10.1029/2007JD009676, 2008.
Craig, H.: Isotopic Variations in Meteoric Waters, Science, 133, 1702–1703, https://doi.org/10.1126/science.133.3465.1702, 1961.
Dansgaard, W.: Stable isotopes in precipitation, Tellus, 16, 436–468, 1964.
DeSouza-Machado, S., Strow, L. L., Tangborn, A., Huang, X., Chen, X., Liu, X., Wu, W., and Yang, Q.: Single-footprint retrievals for AIRS using a fast TwoSlab cloud-representation model and the SARTA all-sky infrared radiative transfer algorithm, Atmos. Meas. Tech., 11, 529–550, https://doi.org/10.5194/amt-11-529-2018, 2018.
Frankenberg, C., Yoshimura, K., Warneke, T., Aben, I., Butz, A., Deutscher, N., Griffith, D., Hase, F., Notholt, J., Schneider, M., Schrijver, H., and Röckmann, T.: Dynamic Processes Governing Lower-Tropospheric HDO∕H2O Ratios as Observed from Space and Ground, Science, 325, 1374, https://doi.org/10.1126/science.1173791, 2009.
Fu, D., Worden, J. R., Liu, X., Kulawik, S. S., Bowman, K. W., and Natraj, V.: Characterization of ozone profiles derived from Aura TES and OMI radiances, Atmos. Chem. Phys., 13, 3445–3462, https://doi.org/10.5194/acp-13-3445-2013, 2013.
Fu, D., Bowman, K. W., Worden, H. M., Natraj, V., Worden, J. R., Yu, S., Veefkind, P., Aben, I., Landgraf, J., Strow, L., and Han, Y.: High-resolution tropospheric carbon monoxide profiles retrieved from CrIS and TROPOMI, Atmos. Meas. Tech., 9, 2567–2579, https://doi.org/10.5194/amt-9-2567-2016, 2016.
Fu, D., Kulawik, S. S., Miyazaki, K., Bowman, K. W., Worden, J. R., Eldering, A., Livesey, N. J., Teixeira, J., Irion, F. W., Herman, R. L., Osterman, G. B., Liu, X., Levelt, P. F., Thompson, A. M., and Luo, M.: Retrievals of tropospheric ozone profiles from the synergism of AIRS and OMI: methodology and validation, Atmos. Meas. Tech., 11, 5587–5605, https://doi.org/10.5194/amt-11-5587-2018, 2018.
Fu, D., Millet, D. B., Wells, K. C., Payne, V. H., Yu, S., Guenther, A., and Eldering, A.: Direct retrieval of isoprene from satellite-based infrared measurements, Nat. Commun., 10, 3811, https://doi.org/10.1038/s41467-019-11835-0, 2019.
Fu, R., Yin, L., Wenhong, L., Arias, P. A., Dickinson, R. E., Huang, L., Chakraborty, S., Fernandes, K., Liebmann, B., Fisher, R., and Myneni, R. B.,: Increased dry-season length over Amazonia and its implication for future climate projection, P. Natl. Acad. Sci. USA, 110, 18110–18115, https://doi.org/10.1073/pnas.1302584110, 2013.
Galewsky, J., Steen-Larsen, H.-C., Field, R. D., Worden, J., Risi, C., and Schneider, M.: Stable isotopes in atmospheric water vapor and applications to the hydrologic cycle, Rev. Geophys., 54, 809–865, 2016.
Good, S. P., Noone, D., and Bowen, G.: Hydrologic connectivity constrains partitioning of global terrestrial water fluxes, Science, 349, 175–177, 2015.
Gupta, P., Noone, D., Galewsky, J., Sweeny, C., and Vaughn, B. H.: Demonstration of high precision continuous measurements of water vapor isotopologues in laboratory and remote field deployments using WS-CRDS technology, Rapid Commun. Mass Sp., 23, 2534–2542, https://doi.org/10.1002/rcm.4100, 2009.
Henze, D. and Noone, D.: The Dependence of Entrainment and Drizzle in Marine Stratiform Clouds on Biomass Burning Aerosols Derived from Stable Isotope and Thermodynamic Profiles, AGU Fall Meeting, New Orleans, Louisiana, United States, Abstract A11C-0048, 11 December 2017.
Henze, D., Toohey, D., and Noone, D.: The Water Isotope Spectrometer for Precipitation and Entrainment Research (WISPER), in preparation, 2020.
Herbin, H., Hurtmans, D., Turquety, S., Wespes, C., Barret, B., Hadji-Lazaro, J., Clerbaux, C., and Coheur, P.-F.: Global distributions of water vapour isotopologues retrieved from IMG/ADEOS data, Atmos. Chem. Phys., 7, 3957–3968, https://doi.org/10.5194/acp-7-3957-2007, 2007.
Herbin, H., Hurtmans, D., Clerbaux, C., Clarisse, L., and Coheur, P.-F.: and HDO measurements with IASI/MetOp, Atmos. Chem. Phys., 9, 9433–9447, https://doi.org/10.5194/acp-9-9433-2009, 2009.
Herman, R. L. and Kulawik, S. S. (Eds.): Tropospheric Emission Spectrometer TES Level 2 (L2) Data User's Guide, D-38042, version 7.0, Jet Propulsion Laboratory, California Institute of Technology, Pasadena, CA, available at: https://eosweb.larc.nasa.gov/project/tes/guide/TESDataUsersGuideV7_0_Sep_27_2018_FV-2.pdf, last access: 18 October 2018.
Herman, R. L. and Osterman, G. B. (Eds.): Tropospheric Emission Spectrometer Data Validation Report (Version F08_11 data), D-33192, Version 7.0, Jet Propulsion Laboratory, California Institute of Technology, Pasadena, CA, available at: https://eosweb.larc.nasa.gov/sites/default/files/project/tes/readme/TES_Validation_Report_v7_Final.pdf, last access: 15 October 2018.
Herman, R. L., Cherry, J. E., Young, J., Welker, J. M., Noone, D., Kulawik, S. S., and Worden, J.: Aircraft validation of Aura Tropospheric Emission Spectrometer retrievals of HDO∕H2O, Atmos. Meas. Tech., 7, 3127–3138, https://doi.org/10.5194/amt-7-3127-2014, 2014.
Irion, F. W., Moyer, E. J., Gunson, M. R., Rinsland, C. P., Yung, Y. L., Michelsen, H. A., Salawitch, R. J., Chang, A. Y., Newchurch, M. J., Abbas, M. M., Abrams, M. C., and Zander, R.: Stratospheric observations of CH3D and HDO from ATMOS infrared solar spectra: Enrichments of deuterium in methane and implications for HD, Geophys. Res. Lett., 23, 2381–2384, 1996.
Irion, F. W., Kahn, B. H., Schreier, M. M., Fetzer, E. J., Fishbein, E., Fu, D., Kalmus, P., Wilson, R. C., Wong, S., and Yue, Q.: Single-footprint retrievals of temperature, water vapor and cloud properties from AIRS, Atmos. Meas. Tech., 11, 971–995, https://doi.org/10.5194/amt-11-971-2018, 2018.
Kuang, Z., Toon, G. C., Wennberg, P. O., and Yung, Y. L.: Measured HDO∕H2O ratios across the tropical tropopause, Geophys. Res. Lett., 30, 1372, https://doi.org/10.1029/2003GL017023, 2003.
Kulawik, S. S., Worden, J., Eldering, A., Bowman, K., Gunson, M., Osterman, G. B., Zhang, L., Clough, S., Shephard, M. W., and Beer, R.: Implementation of cloud retrievals for Tropospheric Emission Spectrometer (TES) atmospheric retrievals: part 1. Description and characterization of errors on trace gas retrievals, J. Geophys. Res.-Atmos., 111, D24204, https://doi.org/10.1029/2005JD006733, 2006.
Lacour, J.-L., Risi, C., Clarisse, L., Bony, S., Hurtmans, D., Clerbaux, C., and Coheur, P.-F.: Mid-tropospheric δ D observations from IASI/MetOp at high spatial and temporal resolution, Atmos. Chem. Phys., 12, 10817–10832, https://doi.org/10.5194/acp-12-10817-2012, 2012.
Lossow, S., Steinwagner, J., Urban, J., Dupuy, E., Boone, C. D., Kellmann, S., Linden, A., Kiefer, M., Grabowski, U., Glatthor, N., Höpfner, M., Röckmann, T., Murtagh, D. P., Walker, K. A., Bernath, P. F., von Clarmann, T., and Stiller, G. P.: Comparison of HDO measurements from Envisat/MIPAS with observations by Odin/SMR and SCISAT/ACE-FTS, Atmos. Meas. Tech., 4, 1855–1874, https://doi.org/10.5194/amt-4-1855-2011, 2011.
Moncet, J.-L., Uymin, G., Lipton, A. E., and Snell, H. E.: Infrared radiance modeling by optimal spectral sampling, J. Atmos. Sci., 65, 3917–3934, https://doi.org/10.1175/2008JAS2711.1, 2008.
Moncet, J.-L., Uymin, G., Liang, P., and Lipton, A. E.: Fast and accurate radiative transfer in the thermal regime by simultaneous optimal spectral sampling over all channels, J. Atmos. Sci., 72, 2622–2641, https://doi.org/10.1175/JAS-D-14-0190.1, 2015.
Moyer, E. J., Irion, F. W., Yung, Y. L., and Gunson, M. R.: ATMOS stratospheric deuterated water and implications for troposphere-stratosphere transport, Geophys. Res. Lett., 23, 2385–2388, 1996.
Murtagh, D., Frisk, U., Merino, F., Ridal, M., Jonsson, A., Stegman, J., Witt, G., Eriksson, P., Jiménez, C., Megie, G., de la Noë, J., Ricaud, P., Baron, P., Pardo, J. R., Hauchcorne, A., Llewellyn, E. J., Degenstein, D. A., Gattinger, R. L., Lloyd, N. D., Evans, W. F. J., McDade, I. C., Haley, C. S., Sioris, C., von Savigny, C., Solheim, B. H., McConnell, J. C., Strong, K., Richardson, E. H., Leppelmeier, G. W., Kyrölä, E., Auvinen, H., and Oikarinen, L.: An overview of the Odin atmospheric mission, Can. J. Phys., 80, 309–319, https://doi.org/10.1139/p01-157, 2002.
Nassar, R., Bernath, P. F., Boone, C. D., Gettelman, A., McLeod, S. D., and Rinsland, C. P.: Variability in HDO∕H2O abundance ratios in the tropical tropopause layer, J. Geophys. Res., 112, D21305, https://doi.org/10.1029/2007JD008417, 2007.
Noone, D.: Pairing Measurements of the Water Vapor Isotope Ratio with Humidity to Deduce Atmospheric Moistening and Dehydration in the Tropical Midtroposphere, J. Climate, 25, 4476–4494, 2012.
O'Keefe, A. and Deacon, D. A. G.: Cavity ringdown optical spectrometer for absorption measurements using pulsed laser sources, Rev. Sci. Instrum., 59, 2544, https://doi.org/10.1063/1.1139895, 1988.
ORACLES Science Team: Suite of Aerosol, Cloud, and Related Data Acquired Aboard P3 During ORACLES 2016, Version 1, NASA Ames Earth Science Project Office, https://doi.org/10.5067/Suborbital/ORACLES/P3/2016_V1, 2017.
Pagano, T. S., Aumann, H. H., Hagan, D. E., and Overoye, K.: Prelaunch and in-flight radiometric calibration of the Atmospheric Infrared Sounder (AIRS), IEEE T. Geosci. Remote, 41, 265–273, 2003.
Pagano, T. S., Aumann, H., Schindler, R., Elliott, D., Broberg, S., Overoye, K., and Weiler, M.: Absolute Radiometric Calibration Accuracy of the Atmospheric Infrared Sounder, Proc. SPIE, 7081-46, San Diego, California, 10 August 2008.
Randel, W. J., Moyer, E., Park, M., Jensen, E., Bernath, P., Walker, K., and Boone, C.: Global variations of HDO and HDO∕H2O ratios in the upper troposphere and lower stratosphere derived from ACE-FTS satellite measurements, J. Geophys. Res.-Atmos., 117, D06303, https://doi.org/10.1029/2011JD016632, 2012.
Rienecker, M. M., Suarez, M. J., Todling, R., Bacmeister, J., Takacs, L., Liu, H.-C., Gu, W., Sienkiewicz, M., Koster, R. D., Gelaro, R., Stajner, I., and Nielson, J. E.: The GEOS-5 Data Assimilation System: Documentation of Versions 5.0, 5.1.0 and 5.2.0, NASA TM 104606, 27, NASA Technical Report Series on Global Modeling and Data Assimilation, 2008.
Rinsland, C. P., Gunson, M. R., Foster, J. C., Toth, R. A., Farmer, C. B., and Zander, R.: Stratospheric Profiles of Heavy Water Vapor Isotopes and CH3D From Analysis of the ATMOS Spacelab 3 Infrared Solar Spectra, J. Geophys. Res., 96, 1057–1068, 1991.
Rodgers, C. D.: Inverse Methods for Atmospheric Sounding: Theory and Practice, World Science, London, 2000.
Schneider, A., Borsdorff, T., aan de Brugh, J., Aemisegger, F., Feist, D. G., Kivi, R., Hase, F., Schneider, M., and Landgraf, J.: First data set of H2O∕HDO columns from the Tropospheric Monitoring Instrument (TROPOMI), Atmos. Meas. Tech., 13, 85–100, https://doi.org/10.5194/amt-13-85-2020, 2020.
Schneider, M. and Hase, F.: Optimal estimation of tropospheric H2O and δD with IASI/METOP, Atmos. Chem. Phys., 11, 11207–11220, https://doi.org/10.5194/acp-11-11207-2011, 2011.
Schneider, M., Toon, G. C., Blavier, J.-F., Hase, F., and Leblanc, T.: H2O and δD profiles remotely-sensed from ground in different spectral infrared regions, Atmos. Meas. Tech., 3, 1599–1613, https://doi.org/10.5194/amt-3-1599-2010, 2010.
Steinwagner, J., Milz, M., von Clarmann, T., Glatthor, N., Grabowski, U., Höpfner, M., Stiller, G. P., and Röckmann, T.: HDO measurements with MIPAS, Atmos. Chem. Phys., 7, 2601–2615, https://doi.org/10.5194/acp-7-2601-2007, 2007.
Steinwagner, J., Fueglistaler, S., Stiller, G., von Clarmann, T., Kiefer, M., Borsboom, P. P., van Delden, A., and Rockmann, T.: Tropical dehydration processes constrained by the seasonality of stratospheric deuterated water, Nat. Geosci., 3, 262–266, 2010.
Stockli, R., Vermote, E., Saleous, N., Simmon, R., and Herring, D.: True color earth data set includes seasonal dynamics, EOS, 87, 55, https://doi.org/10.1029/2006EO050002, 2011.
Urban, J., Lautie, N., Murtagh, D., Eriksson, P., Kasai, Y., Lossow, S., Dupuy, E., de la Noe, J., Frisk, U., Olberg, M., Le Flochmoen, E., and Ricaud, P.: Global observations of middle atmospheric water vapour by the Odin satellite: An overview, Planet. Space Sci., 55, 1093–1102, https://doi.org/10.1016/j.pss.2006.11.021, 2007.
Worden, H. M., Logan, J. A., Worden, J. R., Beer, R., Bowman, K., Clough, S. A., Eldering, A., Fisher, B. M., Gunson, M. R., Herman, R. L., Kulawik, S. S., Lampel, M. C., Luo, M., Megretskaia, I. A., Osterman, G. B., and Shephard, M. W.: Comparisons of Tropospheric Emission Spectrometer (TES) ozone profiles to ozonesondes: Methods and initial results, J. Geophys. Res., 112, D03309, https://doi.org/10.1029/2006JD007258, 2007.
Worden, J., Kulawik, S., Shephard, M., Clough, S., Worden, H., Bowman, K., and Goldman, A.: Predicted errors of Tropospheric Emission Spectrometer nadir retrievals from spectral window selection, J. Geophys. Res., 109, D09308, https://doi.org/10.1029/2004JD004522, 2004.
Worden, J., Bowman, K., Noone, D., Beer, R., Clough, S., Eldering, A., Fisher, B., Goldman, A., Gunson, M., Herman, R., Kulawik, S. S., Lampel, M., Luo, M., Osterman, G., Rinsland, C., Rodgers, C., Sander, S., Shephard, M., and Worden, H.: Tropospheric Emission Spectrometer observations of the tropospheric HDO∕H2O ratio: Estimation approach and characterization, J. Geophys. Res., 111, D16309, https://doi.org/10.1029/2005JD006606, 2006.
Worden, J., Noone, D., Bowman, K., and TES science team and data contributors: Importance of rain evaporation and continental convection in the tropical water cycle, Nature, 445, 528–532, https://doi.org/10.1038/nature05508, 2007.
Worden, J., Noone, D., Galewsky, J., Bailey, A., Bowman, K., Brown, D., Hurley, J., Kulawik, S., Lee, J., and Strong, M.: Estimate of bias in Aura TES HDO∕H2O profiles from comparison of TES and in situ HDO∕H2O measurements at the Mauna Loa observatory, Atmos. Chem. Phys., 11, 4491–4503, https://doi.org/10.5194/acp-11-4491-2011, 2011.
Worden, J., Wecht, K., Frankenberg, C., Alvarado, M., Bowman, K., Kort, E., Kulawik, S., Lee, M., Payne, V., and Worden, H.: CH4 and CO distributions over tropical fires during October 2006 as observed by the Aura TES satellite instrument and modeled by GEOS-Chem, Atmos. Chem. Phys., 13, 3679–3692, https://doi.org/10.5194/acp-13-3679-2013, 2013.
Worden, J., Kulawik, S., Frankenberg, C., Payne, V., Bowman, K., Cady-Peirara, K., Wecht, K., Lee, J.-E., and Noone, D.: Profiles of CH4, HDO, H2O, and N2O with improved lower tropospheric vertical resolution from Aura TES radiances, Atmos. Meas. Tech., 5, 397–411, https://doi.org/10.5194/amt-5-397-2012, 2012.
Worden, J. R., Kulawik, S. S., Fu, D., Payne, V. H., Lipton, A. E., Polonsky, I., He, Y., Cady-Pereira, K., Moncet, J.-L., Herman, R. L., Irion, F. W., and Bowman, K. W.: Characterization and evaluation of AIRS-based estimates of the deuterium content of water vapor, Atmos. Meas. Tech., 12, 2331–2339, https://doi.org/10.5194/amt-12-2331-2019, 2019 (data available at: https://avdc.gsfc.nasa.gov/pub/data/satellite/Aura/TES/.AIRS/, last access: 27 March 2020).
Wright, J. S., Fu, R., Worden, J. R., Chakraborty, S., Clinton, N. E., Risi, C., Sun, U., and Yin, L., Rainforest-initiated wet season onset over the southern Amazon, P. Natl. Acad. Sci. USA, 114, 32, 8481–8486, https://doi.org/10.1073/pnas.1621516114, 2017.