the Creative Commons Attribution 4.0 License.
the Creative Commons Attribution 4.0 License.
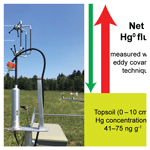
Eddy covariance flux measurements of gaseous elemental mercury over a grassland
Stefan Osterwalder
Werner Eugster
Iris Feigenwinter
Direct measurements of the net ecosystem exchange (NEE) of gaseous elemental mercury (Hg0) are important to improve our understanding of global Hg cycling and, ultimately, human and wildlife Hg exposure. The lack of long-term, ecosystem-scale measurements causes large uncertainties in Hg0 flux estimates. It currently remains unclear whether terrestrial ecosystems are net sinks or sources of atmospheric Hg0. Here, we show a detailed validation of direct Hg0 flux measurements based on the eddy covariance technique (Eddy Mercury) using a Lumex RA-915 AM mercury monitor. The flux detection limit derived from a zero-flux experiment in the laboratory was 0.22 ng m−2 h−1 (maximum) with a 50 % cutoff at 0.074 ng m−2 h−1. We present eddy covariance NEE measurements of Hg0 over a low-Hg soil (41–75 ng Hg g−1 in the topsoil, referring to a depth of 0–10 cm), conducted in summer 2018 at a managed grassland at the Swiss FluxNet site in Chamau, Switzerland (CH-Cha). The statistical estimate of the Hg0 flux detection limit under outdoor conditions at the site was 5.9 ng m−2 h−1 (50 % cutoff). We measured a net summertime emission over a period of 34 d with a median Hg0 flux of 2.5 ng m−2 h−1 (with a −0.6 to 7.4 ng m−2 h−1 range between the 25th and 75th percentiles). We observed a distinct diel cycle with higher median daytime fluxes (8.4 ng m−2 h−1) than nighttime fluxes (1.0 ng m−2 h−1). Drought stress during the measurement campaign in summer 2018 induced partial stomata closure of vegetation. Partial stomata closure led to a midday depression in CO2 uptake, which did not recover during the afternoon. The median CO2 flux was only 24 % of the median CO2 flux measured during the same period in the previous year (2017). We suggest that partial stomata closure also dampened Hg0 uptake by vegetation, resulting in a NEE of Hg0 that was dominated by soil emission. Finally, we provide suggestions to further improve the precision and handling of the “Eddy Mercury” system in order to assure its suitability for long-term NEE measurements of Hg0 over natural background surfaces with low soil Hg concentrations (< 100 ng g−1). With these improvements, Eddy Mercury has the potential to be integrated into global networks of micrometeorological tower sites (FluxNet) and to provide the long-term observations on terrestrial atmosphere Hg0 exchange necessary to validate regional and global mercury models.
Mercury (Hg) is a top priority environmental pollutant that is transported through the atmosphere in its gaseous elemental form (Hg0; > 95 % of total atmospheric Hg). Anthropogenic Hg emissions into the atmosphere exceed natural emissions by approximately a factor of 5 (Outridge et al., 2018). Atmospheric Hg has a lifetime of 8–13 months (Saiz-Lopez et al., 2018); this allows for long-range transport before deposition back onto the Earth's surface, which also occurs at remote locations far from pollution sources. Once deposited, Hg can be transformed into methylmercury that can bioaccumulate in the freshwater and marine food webs, thereby posing a threat to human and ecosystem health (Watras et al., 1998; Fitzgerald et al., 2007; Mason et al., 2012; Braune et al., 2015).
Atmospheric Hg deposition to terrestrial surfaces occurs predominantly as Hg0 dry deposition through stomatal uptake by vegetation or as wet or dry deposition after oxidation in the atmosphere to more soluble reactive mercury, Hg(II) (Lindberg et al., 2007; Driscoll et al., 2013; Jiskra et al., 2018). Wet deposition of Hg(II) via rain and snowfall is relatively well quantified by Hg deposition networks such as the National Atmospheric Deposition Program (NADP), the European Monitoring and Evaluation Programme (EMEP) and the Asia-Pacific Mercury Monitoring Network (APMMN). Dry deposition of Hg(II) is difficult to measure, and its contribution to total Hg deposition remains uncertain (Gustin et al., 2013; Jaffe et al., 2014; Miller et al., 2018; Lyman et al., 2020). Mercury stable isotope fingerprints have identified Hg0 as the dominant deposition pathway to terrestrial surfaces. Dry deposition of Hg0 through vegetation uptake contributes 65 %–90 % of the total Hg deposited to soils (Demers et al., 2007; Jiskra et al., 2015; Enrico et al., 2016; Zhang et al., 2016; Zheng et al., 2016; Obrist et al., 2017). However, Hg0 dry deposition remains poorly constrained due to the lack of long-term monitoring networks (Obrist et al., 2018). Reduction of Hg(II) in terrestrial surface pools and the subsequent emission of Hg0 back to the atmosphere prolongs the cycling of anthropogenic Hg emissions in the environment and can thereby delay the effects of curbing primary anthropogenic emissions on human Hg exposure (Zhu et al., 2016; Wang et al., 2016; Obrist et al., 2018). Net ecosystem exchange (NEE) of Hg0, which refers to the balance between Hg0 dry deposition and emission from foliage and soils, represents a major factor in how fast the environment will recover from anthropogenic Hg pollution. On the global scale, estimates of the terrestrial NEE of Hg0 remain uncertain. In the most recent global mercury assessment, soil emission estimates were lowered to 1000 Mg a−1 (UNEP, 2019) relative to 2200 Mg a−1 in the 2013 assessment (UNEP, 2013); however, the associated uncertainties remain large. A recent review of 132 direct flux measurement studies revealed a NEE Hg0 flux between −513 and 1653 Mg a−1 (the range of 37.5th and 62.5th percentiles, which refers to the central 25 % of the distribution) (Agnan et al., 2016). The database predominantly contains Hg0 flux measurements performed with dynamic flux chambers (85 % of all studies) that are ideal for short-term, mechanistic studies but are less suitable for quantitative flux estimations, especially over vegetated surfaces (Gustin et al., 1999; Eckley et al., 2016; Osterwalder et al., 2018). Year-round NEE measurements of Hg0 at the landscape scale are compelling to reduce measurement uncertainties. However, only four year-round whole-ecosystem Hg0 flux studies have been published, and all of these works used micrometeorological techniques, including the modified Bowen ratio and aerodynamic gradient methods (Fritsche et al., 2008a; Castro and Moore, 2016; Obrist et al., 2017), and the relaxed eddy accumulation (REA) technique (Osterwalder et al., 2017). These approaches use instruments that do not fulfill the fast Hg sensor response criterion that is required for eddy covariance (EC) flux measurements. Hence, these are not direct flux measurements and are consequently dependent on a number of assumptions. The main difficulty in using the modified Bowen ratio and aerodynamic gradient method is resolving a significant concentration gradient during turbulent conditions. During calm conditions, in contrast, it is challenging to determine a significant eddy diffusivity. Further drawbacks are (1) the potentially different sink/source characteristics of the footprint due to the two measurement heights, (2) temporally intermittent sampling between the two sampling inlets, and (3) the fact that transport characteristics are based on reference scalars like heat, water or CO2 (Businger et al., 1986; Stannard et al., 1997; Edwards et al., 2005; Sommar et al., 2013a). The REA technique (Businger and Oncley, 1990) circumvents most of these difficulties. However, uncertainties in the Hg0 flux calculations are introduced by the determination of the proportionality coefficient (β-value) and system-dependent shortcomings, such as a biased offset between the updraft and downdraft sampling lines or difficulties in controlling the air flow from the air inlets to the analyzer. Thus, it remains challenging to accurately measure very small concentration differences with REA (typically < 0.1 ng m−3) between updrafts and downdrafts over natural surfaces with low substrate Hg concentrations (Cobos et al., 2002; Bash and Miller, 2008; Sommar et al., 2013b; Osterwalder et al., 2016, Kamp et al., 2018).
The EC technique has been under development since the late 1940s to measure the surface–atmosphere exchange of heat, mass and momentum in the surface boundary layer, which refers to the lowest 20–50 m of the atmosphere (Montgomery, 1948; Obukhov, 1951; Swinbank, 1951). In order to estimate a vertical turbulent flux, the covariance of two concurrently measured variables is calculated, (1) the scalar quantity of interest (in our case Hg0) and (2) the turbulent fluctuations of the vertical wind velocity, which are both measured at high temporal resolution. Since the 1990s, a new generation of digital three-axis ultrasonic anemometers, infrared gas analyzers and comprehensive software packages have facilitated land–atmosphere exchange measurements of CO2 and H2O (McMillen, 1988). Today, the EC technique is considered the standard method of determining evapotranspiration and the NEE of energy and trace gases such as CO2, CH4, N2O, O2, O3 and volatile organic compounds using high-resolution (10–20 Hz), sometimes portable and generally very reliable equipment (Aubinet et al., 2012).
The first application of the EC technique to measure NEE of Hg0 reported an emission flux of 849 ng m−2 h−1 over contaminated soils (85 mg Hg kg−1 dry soil) during a pilot campaign in Nevada, USA (Pierce et al., 2015). The EC system was based on a fast response (25 Hz), field-deployable pulsed cavity ring-down spectrometer (CRDS; Faïn et al., 2010; Pierce et al., 2013). However, the minimum detection limit of 32 ng m−2 h−1 did not allow for Hg0 flux measurements over soils exhibiting background Hg concentrations (typically < 100 ng Hg g−1; Ericksen et al., 2006) (Pierce et al., 2015).
Here, we present EC measurements of the NEE of Hg0 over a grassland with typical background soil Hg concentrations. Our novel EC system makes use of a Lumex RA-915 AM mercury monitor (Lumex Ltd., St. Petersburg, Russia) atomic absorption spectrometer with Zeeman background correction, which allows for the measurement of Hg0 in ambient air at a relatively high sampling frequency of 1 Hz (Sholupov and Ganeyev, 1995; Sholupov et al., 1995). Ambient air Hg0 measurement comparison studies between the more frequently used Tekran® 2537 analyzer (Tekran Inc., Toronto, Canada) and the RA-915 AM were performed by the European Committee for Standardization's (CEN) Technical Committee 264 “Air Quality” EN 15852 and showed good agreement between the two instruments (Brown et al., 2010). Among other applications, the mercury monitor's precursor, the Lumex RA-915+ mercury analyzer was successfully deployed in the Global Mercury Observation System (GMOS) project at two sites in Russia and Suriname (Sprovieri et al., 2016).
The objective of this study was to test the performance of the RA-915 AM as a fast response analyzer as well as its suitability for EC flux measurements with the goal of reliably measuring the NEE of Hg0 over terrestrial ecosystems. Hereinafter, the new EC system is referred to as “Eddy Mercury”. We provide a description of the Eddy Mercury system and present the data analysis procedure to calculate the NEE of Hg0 in detail. We discuss the patterns in the NEE of Hg0 measured over a grassland during a 34 d pilot campaign and provide suggestions to improve the reliability and precision of the Eddy Mercury system for future long-term applications.
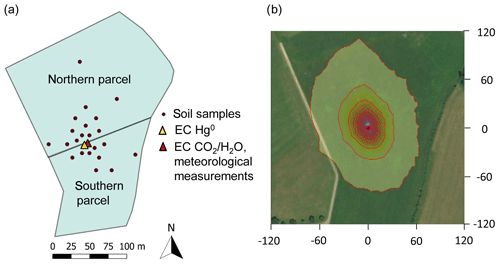
Figure 1(a) Schematic of the experimental setup at the Chamau (CH-Cha) research site showing the exact locations of topsoil samples for total Hg analysis (n=22) and eddy covariance (EC) flux measurements of Hg0, CO2 and H2O conducted between 20 July and 6 September 2018. Panel (b) shows the footprint contour lines of 10 % to 90 % in 10 % steps representing the flux source area during our measurement period. Numbers indicate the distance in meters from the EC station (black cross). The footprint was calculated by applying the footprint model presented in Kljun et al. (2015). Figure (b) is a direct output from the following online tool: http://footprint.kljun.net/ (last access: 6 February 2020).
2.1 Site description and instrumentation
The Eddy Mercury system was tested between 20 July and 6 September 2018 at the Swiss FluxNet site Chamau (CH-Cha), which is located in central Switzerland, about 30 km southwest of Zurich (47∘12′36.8′′ N, 8∘24′37.6′′ E; 393 m a.s.l.). In this study, NEE of Hg0 and CO2 was measured concurrently with two independent EC systems over the intensively managed grassland used for forage production. Details on grassland species composition, harvest, and fertilization practices are described in Zeeman et al. (2010), Merbold et al. (2014) and Fuchs et al. (2018). The tower for long-term EC greenhouse gas measurements was located between two adjacent grassland parcels (Fig. 1a). The northern parcel, measured when up-valley winds prevailed, was oversown with clover in March 2015 and April 2016 to investigate the N2O emission reduction potential compared with the conventionally fertilized grassland of the southern parcel, measured primarily when down-valley winds prevailed (Fig. 1b). The soil type is a Gleysol–Cambisol, with a bulk density of about 1 g cm−3, 30.6 % sand, 47.7 % silt and 21.7 % clay in the top 10 cm (Roth, 2006). A topsoil pH of 5.3 was determined by adding 25 mL of 0.01 M CaCl2 solution to 10 g of dry soil (Labor Ins AG, Kerzers, Switzerland) in 2014. The 24-year (1994–2017) average annual temperature measured at the nearby SwissMetNet surface weather station in Cham (CHZ, 444.5 m a.s.l.) was 10.1 ∘C, and the average annual precipitation was 997 mm.
The Eddy Mercury system was mounted approximately 3 m west of a fully equipped long-term EC tower measuring greenhouse gas exchange (CO2 and H2O) and meteorological variables at a height of 2 m (Fig. 1). The CO2 flux system consisted of a three-dimensional (3-D) ultrasonic anemometer (Solent R3-50, Gill Instruments, Lymington, UK) and an open-path infrared gas analyzer for CO2 and H2O concentrations running at a 20 Hz resolution (IRGA, LI-7500, LI-COR Biosciences, Lincoln, NE, USA). From the 20 Hz IRGA measurements, 30 min flux averages were calculated using the LI-COR EddyPro® software. The 30 min CO2 flux has been recorded continuously since 2005 (Eugster and Zeeman, 2006; Zeeman et al., 2010). The measured meteorological variables included temperature and relative humidity (Hygroclip S3 sensor, Rotronic AG, Switzerland), net all-wave radiation (CNR 1, Kipp & Zonen B.V., Delft, Netherlands), incoming and reflected photosynthetic active radiation (PAR lite, Kipp & Zonen B.V., Delft, Netherlands), and precipitation (height of 0.5 m; tipping bucket rain gauge from LAMBRECHT meteo GmbH, Göttingen, Germany). In addition, soil temperature was recorded at depths of 0.05, 0.1, 0.15, 0.25 and 0.4 m (T107, Campbell Scientific Inc., Logan, UT, USA).
2.2 Soil sampling and total mercury analysis
Topsoil samples (0–10 cm) were taken in a circular arrangement around the EC tower (Fig. 1a) using a core drill. The soil samples were transported to the laboratory in sealed plastic bags and stored in a fridge at 4 ∘C. The samples were filled into aluminum shells, weighed and dried at 40 ∘C until their weight remained constant. The samples were pestled and sieved through a 2 mm mesh to separate the fine earth and the skeleton. The fine earth was ground to powder using a laboratory scale ball mill. To get rid of all potential humidity, the ground samples were stored in small paper bags in a desiccator and dried again at 40 ∘C. The 22 topsoil samples were analyzed for total Hg using a DMA-80 direct mercury analyzer (MLS Mikrowellen GmbH, Leutkirch im Allgäu, Germany). Certified Hg standard solution (NIST-3133) was gravimetrically diluted to concentrations of 10 to 1000 ng g−1 and used for the calibration of the instrument. Repeated measurements of standard reference material (ERM-CC141 loam soil) 90.3±7.8 ng g−1 (mean ± standard deviation, n=3) agreed with the certified value (83±17 ng g−1).
2.3 Description of the Eddy Mercury system
The core of the Eddy Mercury system with respect to the measurement of the NEE of Hg0 is the RA-915 AM mercury monitor. The RA-915 AM uses atomic absorption spectrometry (AAS) with Zeeman background correction to continuously measure Hg0 in ambient air (Sholupov et al., 2004). The multipath sample cell of the RA-915 AM has an optical path length of 9.6 m and a cell volume of 0.7 L. Baseline corrections (zero drift) were performed automatically by the instrument using Hg-free air at user-defined intervals. Span corrections are carried out using an inbuilt calibration cell that contains Hg0 vapor. The measurement range lies between 0 and 2000 ng m−3, and the instrument detection limit is 0.5 ng m−3 according to the analytical specifications of the manufacturer. The air flow rate was increased to 14.3 L min−1 by bypassing the instrument pump in order to reduce the residence time in the measurement cell (normal flow of 7 L min−1). For this, a stronger external pump was connected (model MAA-V109-MD, GAST Manufacturing, MI, USA). The instrument was placed in a weatherproof, air-conditioned box (Elcase, Marthalen, Switzerland) to protect the sensitive RA-915 AM from rain and to reduce temperature fluctuations. A USB to RS232 serial data interface was used to establish a one-way communication link from the RA-915 AM to the data acquisition computer. The air inlet was mounted 24 cm below the center of the head of the 3-D ultrasonic anemometer (Gill R2A, Solent, UK) used for wind vector measurements that was installed 2 m above the ground. A micro-quartz fiber filter (Grade MK 360, 47 mm diameter, Ahlstrom–Munksjö, Sweden) was installed in a 47 mm perfluoroalkyl polymer (PFA) single-stage filter assembly (Savillex, Eden Prairie, USA) at the air inlet. The air inlet was connected to the RA-915 AM by a 2.8 m intake hose with a 11 mm inner diameter (i.d.) attached to a 0.35 m, 4 mm i.d. sample intake hose. Both hose segments were unheated, insulated PFA tubing. The median lag time of the turbulent airflow (Reynolds number of > 5000) from the tube inlet to the analyzer was in the order of 1.15 s.
2.4 Eddy covariance flux measurements
The RA-915 AM analyzer was configured to measure Hg0 concentrations at 1 Hz. The Hg0 concentrations and the 3-D wind vectors were measured from 20 July to 6 September 2018 using four different settings of the RA-915 AM analyzer with respect to the length of the measurement interval between two autocalibration cycles (zero and span): (1) 24 h intervals from 20 to 26 July 2018; (2) 4 h intervals from 1 to 26 August 2018; (3) 1 h intervals from 27 to 31 August 2018; (4) 4 min intervals from 31 August to 6 September 2018. The ultrasonic anemometer had an internal sampling frequency of 1000 Hz that was averaged (eight records of each acoustic sensor pair for each direction) to 20.83 Hz. The 1 Hz RA-915 AM data were merged with the ultrasonic anemometer's data stream by oversampling, as described in Eugster and Plüss (2010). Data were collected on a Linux-based Raspberry Pi computer equipped with a real-time clock chip and internet access. Because data transfer via the USB port from the embedded Windows 7 system of the RA-915AM was highly unreliable, only the system time stamps were synchronized with the Linux data acquisition system every second via a Windows PowerShell script. In cases when this communication also failed, an approximate time synchronization was done by polling the RA-915 AM time stamp via the Samba file-sharing protocol. Thus, in addition to the synchronization method described by Eugster and Plüss (2010), the merging of Hg0 measurements with wind vector data had to be carried out off-line in a separate data workup step. Fluxes were calculated over 60 min intervals to account for the low sampling frequency of Hg0 signals. Thus, using the previously mentioned interval modes (1) and (2), 3600 Hg0 measurements were used for each 1 h flux average.
2.5 Eddy covariance Hg0 flux calculations
Calculation of the NEE of Hg0 required some modifications of the standard procedure that has been established for CO2 fluxes (e.g., Aubinet et al., 2012). The modifications were carried out according to the five steps described in detail below (Sect. 2.5.1–2.5.5).
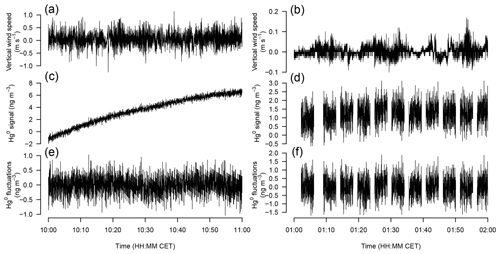
Figure 2Examples of raw data time series over a 1 h data segment: (a, c, e) during period 1 with 24 h calibrations only (21 July 2018, 10:00–11:00), and (b, d, f) during period 4 with 4 min instrument calibration intervals (6 September 2018, 01:00–02:00). Vertical wind speed (a, b) was not detrended. Hg0 concentrations are shown before detrending (c, d) and after detrending (e, f). While the 4 min calibration intervals clearly reduce the longer-term drift (d) compared with daily calibrations (c), the gaps during calibrations had to be filled using linear interpolation before calculating fluxes.
2.5.1 Preparation of raw Hg0 measurements
The RA-915 AM raw data files provide the following information at a 1 Hz resolution: date and time of measurement, photomultiplier current (arb. unit), air flow rate (L min−1), temperature of analyzed air (∘C), temperature of RA-915 AM (∘C), sample cell pressure (kPa), Hg0 raw concentration (ng m−3, including all online corrections), status code and status description. The status code (a numerical value) and status description (a text variable) are redundant and provide the necessary information to distinguish ambient air concentration measurements from zero and span calibration measurements. The Hg0 flux was calculated based on the Hg0 raw concentration. To account for drift and baseline drift, which both are unavoidable when longer measurement periods are used between calibration events, we proceeded as follows. After a calibration event, the Hg0 raw concentration was considered to be the best empirical estimate of the true Hg0 concentration. By the end of a measurement period (the beginning of the next calibration cycle), a linear drift correction was applied to bring the Hg0 raw concentration before the next calibration event to the level of the next calibration result (offset correction). As visual inspection of the data clearly indicated that there was more drift than a simple linear trend in the data (see examples in Fig. 2), a high-pass filter approach was used to minimize drift and optimize the determination of Hg0 fluctuations for EC flux measurements (Sect. 2.5.4).
2.5.2 Preparation of the ultrasonic anemometer data
The ultrasonic anemometer data contained the three wind speed components of the wind vector (all in m s−1), the speed of sound (m s−1) and the information sent from the RA-915 AM to the data acquisition system via the serial data link. The speed of sound (c) was converted to a virtual sonic temperature () in Kelvin (Kaimal and Gaynor, 1991). The vertical wind speed (w) was despiked using an iterative 7σ filter that discards w outside the range of the 6 h mean ±7 standard deviations.
2.5.3 Merging of ultrasonic anemometer data with the Hg0 time series
After the preparation of the two datasets, they were merged by accounting for the time difference between the RA-915 AM and the Linux data acquisition using the information that could be transferred via the serial link from the RA-915 AM to the Linux system (accurate to within 1 s). If no such information was received from the RA-915 AM, the time difference between the two systems was determined using a network time drift fallback option specifically added to the Linux system to overcome the problems with serial output from the RA-915 AM: during the field experiment we polled the most recent data record acquired by the RA-915 AM every 5 min using the Samba file-sharing protocol, and we associated that time stamp with the one of the EC system. This (somewhat less accurate) information was then adjusted during periods where both approaches overlapped to determine the time difference required to shift the Hg0 raw data relative to the ultrasonic anemometer data before merging the two datasets. To ascertain that Hg0 data were lagging the sonic data, we added a ≈1.5 s safety margin in the interpretation of the available time synchronization information received either via serial link or Samba file-sharing.
2.5.4 Determination of the time lag between vertical wind speed and Hg0 fluctuations
The merged dataset was then divided into 1 h segments for Hg0 flux calculations. Within each 1 h segment, the time lag between the two time series was fine-tuned using a cross-correlation procedure to find the best positive or negative correlation within a reasonable time window (0–4 s) around the physically expected time difference (the 1.15 s physical delay plus the 1.5 s safety margin used in Sect. 2.5.3). Because considerable nonturbulent drift of the Hg0 signal was still present after correcting for online calibration (Sect. 2.5.1), we detrended each 1 h segment using a third-order polynomial fit (Eq. 5) before computing the cross-covariance between the detrended Hg0 signal and w (Sect. 3.2.1). To account for the different sampling rates of w (20.83 Hz) and Hg0 (1 Hz), we used simple linear interpolation between individual Hg0 measurements and to bridge across calibration gaps. After a first automatic run, each best estimate for time lag was visually inspected and updated by a narrower search window for each 1 h segment that narrowed the search procedure down to the most realistic cross-correlation peak (positive or negative). Note that calibration gaps are relevant data gaps with setting 4 (from Sect. 2.4) but less problematic with settings 1–3. In all cases, the lack of variance in Hg0 data during the gaps reduces the computed Hg0 flux. Thus, our flux estimates are conservative estimates with respect to flux magnitudes.
2.5.5 Computation of Hg0 EC fluxes
After all data preparations according to Sect. 2.5.1 to 2.5.4, the Hg0 flux () was calculated as the covariance
where χ was the calibrated, detrended and linearly gap-filled Hg0 concentration in nanograms per cubic meter (ng m−3), and w was the vertical wind speed. For improved readability was converted from nanograms per square meter per second to nanograms per square meter per hour (ng m−2 s−1 to ng m−2 h−1) before reporting. In the notation used here, primes denote short-term deviations from the mean (after detrending according to Sect. 2.5.4) over an averaging period (1 h), and overbars denote the mean of a variable. Hg0 flux computations were carried out using R version 3.5.2 (R Core Team, 2018).
2.6 Determination of the Hg0 flux detection limit
To determine whether a calculated Hg0 flux is significantly different from a zero flux, we used two approaches: (1) an indoor zero-flux experiment, and (2) a statistical estimate of the flux detection limit following the concept by Eugster and Merbold (2015) that is an improvement of the concept presented by Eugster et al. (2007). The indoor zero-flux experiment was set up in the laboratory for 2 d before all of the equipment was installed in the field. The low-turbulence conditions in combination with the absence of local Hg0 sources in the laboratory allowed us to ascertain the fluxes that result from the procedure described above when there is no real Hg0 flux. Such zero-flux experiments tend to underestimate the flux detection limit under real-world outdoor conditions, while the second approach quantifies the statistical uncertainty of a calculated flux. The flux (covariance) is the product of the correlation coefficient (rw,χ) between w and χ and the square root of the variances of the two variables (e.g., Eugster and Merbold, 2015):
The significance of rw,χ can be estimated using a Student's t test (see Eugster and Merbold, 2015, for details). Thus, for each 1 h period, we computed the value of rw,χ that is significant at p=0.05, and we multiplied this value by measured σw and σχ to obtain a more realistic estimate of the flux detection limit. It should be noted that this concept was brought forward by Wienhold et al. (1996) using a visual empirical approach, and Eugster and Merbold (2015) then further developed the visual approach into a more objective time series statistical approach to perform the quantification of the flux detection limit. The threshold of significance of rw,χ can be estimated as follows:
where tp is the Student's t value for the significance level p (e.g., 0.05), and n is the autocorrelation-corrected number of independent samples in the time series,
where N is the number of samples in a time series, and ρ1 is the lag 1 autocorrelation coefficient of the scalar product time series w⋅χ.
2.7 Eddy covariance CO2 flux calculations and quality control flags
The 30 min CO2 flux was quantified in the conventional way established in ecosystem studies (see Aubinet et al., 2012) using the EddyPro® (LI-COR Inc., Lincoln, NE, USA) software (see Fuchs et al., 2018, for specific information related to the Chamau field site). For each 30 min CO2 flux interval, a flux quality control (QC) flag was determined following Mauder and Foken (2004): 0 (best data quality for detailed investigations), 1 (good data for longer-term studies) and 2 (poor quality). As there are no established quality control procedures for Hg0 fluxes yet, we used the QC information from the CO2 flux measurement to retain or reject concurrent Hg0 flux measurements. Thus, we only present Hg0 flux measurements with CO2 flux quality flags < 2. During CO2 flux processing using the EddyPro® software, coordinate rotation for tilt correction, angle of attack correction for wind components, Webb–Pearman–Leuning terms for compensation of density fluctuations (Webb et al., 1980), and analytical corrections for high-pass (Eugster and Senn, 1995; Moncrieff et al., 2004) and low-pass filtering effects (Horst, 1997) were applied. Furthermore, a self-heating correction for the open-path gas analyzer was conducted (Burba et al., 2008), and CO2 fluxes greater than 50 and less than −50 µmol m−2 s−1 were discarded.
3.1 Environmental conditions
In 2018, the annual mean air temperatures in Switzerland reached 6.9 ∘C, which was the highest value recorded since the onset of meteorological measurements in 1864 (MeteoSchweiz, 2019). This nationwide average temperature was 1.5 ∘C warmer than the average of the normal period from 1981 to 2010. Total precipitation measured from April to November 2018 was only 69 % of the long-term average (1981–2010). Thus, the period from April to November 2018 was the third driest period ever recorded in Switzerland (MeteoSchweiz, 2019). From the beginning of the growing season until the end of our measurement campaign (April to September 2018), air temperatures at the Cham (CHZ) SwissMetNet surface weather station were elevated by 2.2 ∘C compared with the long-term average from 1994 to 2017 (15.8 ∘C) during the same period. Total precipitation from April to September 2018 was only 72 % (467 mm) of the long-term average (648 mm) calculated for the period between 1994 and 2017. These specific conditions reduced CO2 uptake compared with the same period in 2017 (Sect. 3.3, Fig. 7) and led to lower grassland productivity and yields of only 6.8 t DM ha−1 a−1 (DM refers to dry matter) in 2018 compared with an average yield of 12.7 t DM ha−1 a−1 quantified from 2015 to 2017 (start of the clover experiment). Over the course of the 34 d campaign (from 20 July 2018 at 02:00 to 24 July 2018 at 08:00 and from 9 August 2018 at 12:00 to 6 September 2018 at 17:00; all times in central European time, CET, or UTC+1), sunny conditions prevailed with a mean solar irradiation (Rg) of 352 W m−2 during daytime (Rg ≥5 W m−2) and a mean irradiation of 606 W m−2 at 13:00. The hourly mean air and soil surface temperature ranged from 13.6 ∘C (06:00) to 24.1 ∘C (15:00) and from 18.1 ∘C (08:00) to 21.5 ∘C (18:00), respectively. The median daytime (Rg ≥5 W m−2) and nighttime (Rg < 5 W m−2) wind speed was 0.97 m s−1 (range of 0.05–5.77 m s−1) and 0.37 m s−1 (range of 0.06–2.49 m s−1), respectively. The prevailing wind direction during the day was north–northwest (47 %), whereas it was east–southeast (55 %) at night.
3.2 Performance of the Eddy Mercury system
3.2.1 High-frequency signal analysis
Two examples of the raw data used to compute fluxes (Eq. 1) are shown in Fig. 2, one from period 1 with 24 h calibration intervals (Fig. 2a, c, e) and one with frequent calibrations every 4 min (Fig. 2b, d, f). Frequent calibrations strongly reduced the instrument drift (Fig. 2d) compared with the long calibration intervals (Fig. 2c), although at the expense of some loss of variance and flux (as will be discussed below). In principle, block-averaging raw data within a sampling interval is the best approach to compute EC fluxes (Aubinet et al., 2012). In the case of substantial instrument drift, as is seen with the RA-915 AM (Fig. 2c), it is necessary to remove the drift using an adequate procedure. Because of the curvature of the drift of the analyzer, a simple linear detrending did not lead to satisfactory results in this case; hence, we used a third-order polynomial regression fit:
with t elapsed time within the averaging interval of 1 h. The turbulent Hg0 fluctuations after this additional detrending led to the time series shown in Fig. 2e and f. Lengthy discussions on the possible shortcomings of such a detrending can be found in Lee et al. (2005) and Aubinet et al. (2012) and are therefore not repeated here. Using the example data shown in Fig. 2e, we produced an artificial dataset with gaps that correspond to the 4 min recalibration scheme used during the period shown in Fig. 2f. This led to a loss in Hg0 flux in the order of 12 %. Although nonzero, this should be considered a robust finding given the general understanding that EC flux measurements are accurate to within 10 %–20 %, even with higher quality instrumentation (Aubinet et al., 2012). To obtain higher quality EC fluxes than we present here, the long-term stability of the instrument needs to be improved (Sect. 3.4); however, improving the gap-filling strategy is not expected to contribute significant new insights into Hg0 flux calculations. Drift of the current version of the Eddy Mercury system is substantial (Fig. 3a), which is an effect that is common in experimental sensor setups, although it is no longer prevalent in present-day CO2 sensors. The removal of any drift also reduces the variance of a signal and, subsequently, the flux covariance of interest. Thus, knowledge about the stability of an instrument over which no drift correction is required becomes important. The Allan variance plot (Fig. 3b; Allan, 1966; Werle et al., 1993) indicates that the optimum averaging time is approximately 54 s. For comparison, a CH4 analyzer tested by one of the authors (Eugster and Plüss, 2010) shows an optimum average time that is roughly 3 times as long (approximately 180 s) before the instrument drift starts to dominate the Allan variance. Figure 3b shows that the Allan variance caused by drift at integration times beyond 550 s exceeds the variance associated with turbulence at the 1 s integration time (see blue arrow in Fig. 3b). In a more ideal instrument, the long-term drift is smaller than the short-term variance of interest for EC measurements (see, e.g., Eugster and Plüss, 2010). Despite these findings, Fig. 3 clearly shows the potential and quality of the instrument for Hg0 flux measurements.
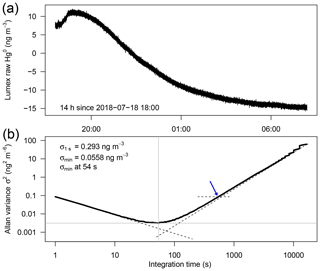
Figure 3Allan variance plot using 14 h of continuous measurements in the laboratory (zero-flux experiment), starting on 18 July 2018 at 18:00. Panel (a) shows the raw time series, and panel (b) shows the Allan variance as a function of integration time.
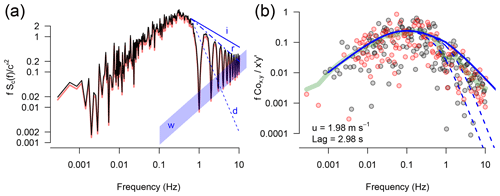
Figure 4Panel (a) shows an example spectrum of Hg0 fluctuation measurements, and panel (b) displays a cospectrum of the 1 h averaged Hg0 flux on 31 August 2018 from 14:00 to 15:00. (a) The power spectrum before (red line) and after (black line) detrending is shown, and the theoretical slopes in the inertial subrange are shown for ideal conditions (i, solid line, slope), for a rectangular oversampling at frequencies greater than 1 Hz (r, broken line, f−1 slope) and for a first-order damped spectrum (d, dashed line, slope). The approximated white noise level is shown using a color band (w, f+1 slope). (b) The flux cospectrum shows the absolute values of the cospectral densities, with black symbols denoting positive contributions to and red symbols denoting negative contributions. The light green bold line is a local polynomial regression fit to the data points, and the blue line denotes an idealized cospectrum. The two dashed blue lines show damped cospectra with respective damping constants of 0.1 and 0.3 s.
This interpretation is also supported by spectral and cospectral analyses (Fig. 4). Figure 4a shows an example spectrum of Hg0 measurements obtained over a 1 h interval. The difference between the red and black lines in Fig. 4a visualizes the effect of polynomial detrending on the power spectrum of Hg0, which is relatively small and of no real concern. As the RA-915 AM only delivers 1 Hz raw data, we had to oversample this digital Hg0 signal to match the 20.83 Hz resolution of the ultrasonic anemometer. Spectral densities at high frequencies > 0.5 Hz (the Nyquist frequency of the RA-915 AM is half of the sampling frequency) reflect the effect of oversampling. In the case of the RA-915 AM, oversampling leads to local minima in spectral densities at 1 Hz and all its harmonic multiples (2, 3, 4, … Hz), which is the result of linear interpolation between measurements. Between these local minima, the spectral density obeys the f−1 power law (line “r” in Fig. 4a), which is very close to the inertial subrange slope (line “i” in Fig. 4a). A damped signal (first-order damping; see Eugster and Senn, 1995) would follow a power law (line “d” in Fig. 4a); thus, it is obvious that our setup had an adequate flow rate through the RA-915 AM that did not lead to substantial damping of the turbulent Hg0 fluctuations. With the oversampling used here, the white noise level (blue band “w” in Fig. 4a) is artificially reduced below the level that we would obtain without oversampling.
After an adequate time lag correction to synchronize the detrended Hg0 signal with vertical wind speed fluctuations w′ was applied, the cospectra of fluxes that were significantly different from a random pattern closely agreed with the theoretical idealized cospectrum for neutral atmospheric stability derived from Kaimal et al. (1972) (see Eugster and Senn, 1995), shown using the solid blue line in Fig. 4b. Some minor signs of damping are seen at higher frequencies where the green spline deviates from the solid blue line (Fig. 4b). The comparison of cospectral densities with theoretical damped cospectra (dashed blue lines in Fig. 4b) clearly confirm the finding from the spectral analysis that the flow rate was high enough in the RA-915 AM sample cell to prevent significant damping effects that tend to be a problem with closed-path EC flux measurements.
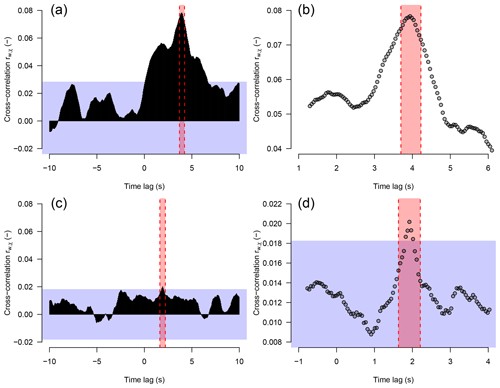
Figure 5Cross-correlation analysis to determine the time lag between the vertical wind speed (w) and Hg0 time series (χ). (a, b) An example with a clearly positive Hg0 flux (21 July 2018, 10:00–11:00), and (c, d) an example with a marginally positive flux (6 September 2018, 01:00–02:00). Panels (a) and (c) show the cross-correlation within a time lag window of ±10 s, and panels (b) and (d) zoom in to the search window used in this study (vertical red band). The blue horizontal band shows the range of zero fluxes (cross-correlation with p≥0.05).
When a clear Hg0 flux was statistically different from a zero flux, the cross-correlation peak was well defined (Fig. 5a, b). On some occasions with low fluxes relative to the flux detection limit (Sect. 3.2.2), the automatic detection of the cross-correlation peak was not successful. The peak often does not extend very strongly beyond the (expected) noise level, as shown in Fig. 5c. However, when zooming in (Fig. 5d), the peak becomes rather clear, although it is only marginally above the range of insignificant correlations shown by the blue background in Fig. 5. To minimize erroneous peak detections and, thus, incorrect flux estimates, we fine-tuned the search window (red band in Fig. 5) for each 1 h data segment by visually inspecting and selecting the search window within which the local maximum of the absolute correlation coefficient between w and χ was found.
3.2.2 Flux detection limit
The flux detection limit was calculated for each 1 h flux period (Sect. 2.6). The significance threshold for rw,χ was calculated for an error probability p=0.05, and the product of this threshold rw,χ and measured σw and σχ was determined as the flux detection limit for that specific 1 h period. Figure 6 shows the probability density function of the flux detection limits from all 1 h data segments. For comparison, the results from the 14 h zero-flux experiments in the laboratory are added as a blue boxplot in Fig. 6. This comparison clearly shows that a zero-flux experiment in the laboratory highly overestimated the quality of Hg0 flux measurements with a median (maximum) flux detection limit of 0.074 (0.22) ng m−2 h−1. The more realistic flux detection limits based on statistically significant (p < 0.05) correlations are rather in the order of 5.9 (50 % cutoff) to 24 ng m−2 h−1 (99 % cutoff) with a 95 % cutoff at 13.7 ng m−2 h−1. During the 34 d measurement campaign, 49.7 % of the Hg0 fluxes (363 out of 731 h) were significantly different from zero. Using the same approach but in a qualitative way, Pierce et al. (2015) estimated the flux detection limit of their system to be around 32 ng m−2 h−1.
3.2.3 Comparison of detection limits for Eddy Mercury, gradient-based and REA systems
The Eddy Mercury system circumvents major sources of uncertainty compared with gradient-based and REA systems, which are related to assumptions on similarity or equivalence of the eddy diffusivities of the scalar transfer coefficients (sensible heat flux, latent heat flux and trace gases). Generally, land–atmosphere Hg0 flux measurements using micrometeorological methods are scarce, and information on detection limits is even rarer. For gradient-based systems, a minimum resolvable Hg0 concentrations gradient (MRG) is determined by mounting the sampling lines at the same height for several days (same-air test) and computing the concentration differences between the lines that are used for flux calculations. The MRG threshold is usually defined as the average plus 1 standard deviation of the concentration difference obtained by the same-air test. Fluxes are considered significant when the Hg0 concentration difference is above the MRG. Exemplarily, Edwards et al. (2005) derived a flux gradient system-specific MRG of 0.01 ng m−3 and a flux detection limit of 1.5 ng m−2 h−1. To calculate the flux detection limit of the gradient sampling system, site characteristics and atmospheric conditions have to be considered (see Eq. 8 in Edwards et al., 2005). Fritsche et al. (2008a) derived a MRG of 0.02 ng m−3 for their setup. The minimum determinable gradient-based Hg0 flux was between 0.5 and 4.6 ng m−2 h−1 (Fritsche et al., 2008b). Converse et al. (2010) and Zhu et al. (2015b) reported a similar MRG for their gradient-based micrometeorological systems of 0.07 and 0.06 ng m−3, respectively. During Hg0 flux studies over agricultural land in China, 57 % and 62 % of the aerodynamic and modified Bowen ratio measurements were significant (Zhu et al., 2015b). For Hg0 flux REA systems, Zhu et al. (2015b) reported that the absolute precision in the updraft and downdraft Hg0 concentration difference was concentration-dependent at 0.069±0.022 [Hg0] (ng m−3), whereas Osterwalder et al. (2017) determined a detection limit of 0.05 and 0.04 ng m−3. Over wheat canopy, 55 % of the fluxes were significant (Zhu et al., 2015a), whereas 52 % of the fluxes were significant over a boreal peatland (Osterwalder et al., 2017). The share of significant Hg0 fluxes for the gradient-based, REA and Eddy Mercury methods is in a similar range of approximately 50 %. However, the same-air tests applied to determine the detection limit of gradient-based and REA fluxes is more appropriate to compare with our approach to determine the zero flux in the laboratory. With a median zero flux of 0.074 ng m−2 h−1, the share of significant fluxes measured with Eddy Mercury would increase to 99.7 %, which is not realistic for measurements outside the laboratory environment. Generally, the reported mean fluxes derived from the gradient-based, REA and Eddy Mercury methods should include data below the detection limit because otherwise the magnitudes of the average exchange rates would be overestimated (see Fritsche et al., 2008a; Osterwalder et al., 2016).
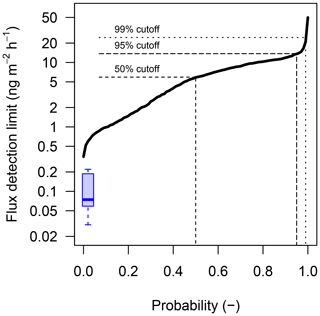
Figure 6Flux detection limit empirical probability distribution of the magnitude of flux measurements under outdoor conditions (black line). The boxplot insert shows the range of the magnitude of measured fluxes during the zero-flux experiment in the laboratory without Hg0 sources under very low-turbulence conditions. The black line shows the theoretical detection limit based on the statistical significance (p < 0.05) of the correlation coefficient between the vertical wind speed and Hg0 fluctuations.
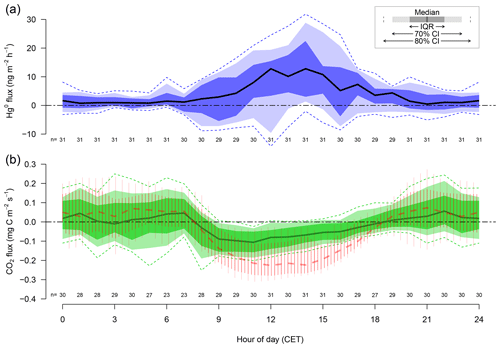
Figure 7Hourly aggregated diel cycle of (a) Hg0 fluxes and (b) simultaneously recorded CO2 fluxes (bold green line) and fluxes measured in 2017 (red bold dashed line) for the same period. Each hour of the day represents the quantiles obtained from a 3 h window centered on the respective hour of all technically valid observations. The bold lines represent median flux values. The interquartile range (IQR) is the range of the middle 50 % of the data. The 70 % and 80 % confidence intervals (CI) and the number of measurements per hour (n) are given. The median CO2 flux in 2017 is displayed (red bold dashed line). The IQR (red vertical lines) and the 70 % CI (lighter vertical lines) are indicated.
3.3 Net ecosystem exchange of Hg0 over grassland
The median (interquartile range, IQR) Hg0 flux measured at the Chamau (CH-Cha) research site using the Eddy Mercury system was 2.5 (−0.6 to 7.4) ng m−2 h−1. The Hg0 flux revealed a distinct diel pattern with median (IQR) daytime and nighttime fluxes of 8.4 (1.9 to 15) and 1.0 (−0.9 to 3.3) ng m−2 h−1, respectively. The minimum hourly median Hg0 flux (0.5 ng m−2 h−1) was detected at 21:00 (Fig. 7a). Emission of Hg0 reached a maximum between 11:00 and 14:00 (hourly median of 10.8 ng m−2 h−1). The diel Hg0 variation corresponded to solar radiation with the highest mean level of irradiance at 13:00 (606 W m−2). The flux of CO2 changed from net emission during the night to net uptake by vegetation with sunrise (Fig. 7b). At noon, CO2 fluxes were 26 % lower than the most negative flux occurring between 10:00 and 11:00 (−0.1 mg C m−2 s−1). The absence of a midday maximum CO2 uptake indicates a midday depression due to plant stress from exceptionally hot and dry conditions. The partial closure of their stomata during the warmest period of the day minimizes water loss through transpiration with the consequence of lower CO2 uptake. Overall, the median CO2 flux during our measurement campaign in 2018 was only 24 % compared with the same period in 2017 which exhibited average climatic conditions (red dashed line in Fig. 7b). The median CO2 uptake in 2018 was 0.031 mg C m−2 s−1 compared with 0.127 mg C m−2 s−1 measured in 2017. We suggest that the increased stomatal resistance of vegetation during the campaign in response to high drought stress not only led to the abovementioned minimized uptake of CO2 but also damped stomatal gas exchange in general, including the uptake of Hg0. Subsequently, soil emission was the dominating factor driving the NEE of Hg0 during summer in 2018.
The Hg0 flux measured at the CH-Cha site is comparable to Hg0 fluxes reported for other grassland sites worldwide (Zhu et al., 2016). A median Hg0 flux of 0.4 ng m−2 h−1 and a flux range between −18.7 and 41.5 ng m−2 h−1 (site-based average fluxes) was reported for nine studies (Poissant and Casimir, 1998; Schroeder et al., 2005; Ericksen et al., 2006; Obrist et al., 2006; Fu et al., 2008a, b; Fritsche et al. 2008a, b; Converse et al., 2010). Several studies reported net Hg0 emission during summer. Converse et al. (2010) reported net average Hg0 emission of 2.5 ng m−2 h−1 from a high-elevation wetland meadow in Virginia, USA. Zhang et al. (2001) measured a Hg0 flux of 7.6±1.7 ng m−2 h−1 from an open background site in Michigan, USA. The average Hg0 flux from a grassland in Québec, CA, was 2.95±2.15 ng m−2 h−1, and a correlation of the diel flux pattern with solar radiation was reported (Poissant and Casimir, 1998). Average net Hg0 emission of 1.1 ng m−2 h−1 was recorded from a pasture in Ontario, CA (Schroeder et al, 2005). The mean Hg0 flux from four grassland sites in the USA ranged from 0.3 to 2.5 ng m−2 h−1 between May 2003 and 2004 (Ericksen et al., 2006). Fu et al. (2008a) reported average Hg0 fluxes ranging from −1.7 to 13.4 ng m−2 h−1 from three grasslands in China in August 2006. The mechanism driving Hg0 emission from grasslands is not fully understood. Photoreduction has been reported to enhance Hg0 emission from soils and the foliage surface and from Hg within foliar tissue (Gustin et al., 2002; Moore and Carpi, 2005; Choi and Holsen, 2009; Yuan et al., 2019). Soil warming has been suggested to promote Hg0 emission (Poissant et al., 1999; Zhang et al., 2001; Gustin et al., 2002; Almeida et al., 2009), which is likely due to the increased decomposition of organic material (Fritsche et al., 2008c) and facilitated mass transfer of Hg0 through the topsoil to the atmosphere (Lin and Pehkonen, 1999). Zhang et al. (2001) reported a strong positive correlation of Hg0 fluxes with solar radiation and soil temperature. A solar shielding experiment resulted in a 65 % decrease in soil Hg0 emission, suggesting that photoreduction is a major factor but also that soil temperature cannot be neglected.
Few grassland studies have shown net Hg0 dry deposition. Fritsche et al. (2008a) reported an average Hg0 flux of −1.7 (modified Bowen ratio) and −4.3 ng m−2 h−1 (aerodynamic gradient) during the vegetation period over a sub-alpine grassland at Frübül in central Switzerland, 15 km southwest of our study site. More summertime Hg0 fluxes from three central European grasslands were measured on a campaign basis, and average grassland–atmosphere Hg0 fluxes ranged from −4.3 to 0.3 ng m−2 h−1. The highest variability of the fluxes was recorded for the Neustift site in Austria with a range from −76 to 37 ng m−2 h−1 (Fritsche et al., 2008b). A second full year Hg0 flux study was performed at an upland meadow in Maryland, USA (Castro and Moore, 2016). The hourly mean summertime Hg0 flux was −1.2 ng m−2 h−1 and ranged between −224 and 354 ng m−2 h−1.
We found that the southern source area of our grassland site has a 28 % higher Hg substrate concentration (mean ng Hg g−1) than the northern source area (mean ng Hg g−1) (Wilcoxon two sample t test, p < 0.05; Fig. 8a). The Eddy Mercury system was able to resolve a marginally significant greater daytime Hg0 flux (+44 %, p=0.0515; Fig. 8c) and insignificantly greater nighttime Hg0 flux (+68 %, p=0.296; Fig. 8b) from the southern source area that is enriched in Hg compared with the northern source area. The proportionality of Hg0 emission to soil Hg concentration has been shown across Hg-enriched soils (Eckley et al., 2015; Zhu et al., 2018; Osterwalder et al., 2019), but no significant correlation has been observed for low-Hg level background soils (Agnan et al., 2016). There are two possible explanations for the lack of a significant relationship between Hg0 flux and soil Hg concentration: (i) the analytical uncertainty of Hg0 flux measurements, or (ii) a masking of Hg0 emission by stomatal uptake of Hg0 at vegetated surfaces that is independent of the soil Hg concentration.
3.4 Suggestions to improve the Eddy Mercury system
Here we propose a number of adjustments that are expected to improve the Eddy Mercury system's performance in particular by (1) facilitating data transfer and processing, (2) increasing the measurement frequency and sample air flow through the RA-915 AM and (3) achieving more stable temperature conditions in the field. The length of data gaps that are mainly caused by system calibrations should be reduced to the point where discussions about gap-filling methods and detrending procedures can be considered obsolete.
Improve data transfer. The determination of the time lag between the wind speed measurement and the Hg0 concentration measurement introduced a considerable source of uncertainty, and cross-correlation peaks had to be visually verified (Sect. 2.5.4.). In the future, we aim for a real-time transfer of raw data to the serial port instead of data transfer via the USB port on the embedded Windows 7 system of the RA-915 AM. This will allow better synchronization between the Hg0 measurements and the ultrasonic anemometer (Sect. 2.4) and will significantly facilitate post-acquisition data treatment.
Increase measurement frequency. The pilot campaign was performed with a measurement frequency of 1 Hz. In the future, we wish to increase the measurement frequency up to between 10 and 20 Hz. Such an increase in measurement frequency is possible via software adaptations of the RA-915AM, will make the oversampling of the Hg0 signal performed here (Sect. 3.2) redundant and will result in better counting statistics.
Increase sample flow rate. During this pilot study we connected a more powerful pump to the RA-915 AM and managed to increase the flow rate from standard operation of 7–10 to 14.3 L min−1, resulting in a residence time in the measurement cell that was 2 times lower than the original residence time. The lower residence time in the cell reduced the dampening of the signal (Sect. 3.2). However, this high flow led to a reduction in the cell pressure (approximately 700 mbar) that affected the detection limit for the Hg0 concentration measurements. In the future, we propose to further reduce the residence time of the air in the measurement cell by increasing the sample air flow by another 30 % to 20 L min−1 using an external pump. To minimize the pressure drop, we propose to reduce the constrictions present in the RA-915 AM by increasing the internal diameter of the valves and the inlet tubing.
Improve the long-term stability of the instrument. The stability of RA-915 AM Hg0 concentration measurements is temperature dependent (Sect. 3.2). We encountered strong diurnal temperature fluctuations of the instrument during the pilot campaign. We took several measures during the campaign to increase the temperature stability (e.g., placing the pump outside the temperature-controlled analyzer box, insulation of the analyzer box and shading it from direct sunlight). To improve the temperature stability in the future, we suggest placing the RA-915AM in an instrument box that has a better insulation and more powerful temperature control or, ideally, placing it in a climate-controlled instrumental hut. For long-term deployments of the Eddy Mercury, the sampling hose can be extended to bridge the distance between the air inlet, which is located close to the sonic anemometer and the instrumental hut where the system is placed. In this case, however, it would be important to guarantee a turbulent flow in the tube (Reynolds number of > 3000–3500; Lenschow and Raupach, 1991; Leuning and King, 1992), an adequate refresh rate in the sampling cell and to ensure that the pressure drop in the sampling cell was within the requirements of the instruments (> 600 mbar; Vladimir Ryzhov from Lumex Ltd, personal communication, 2018).
This study demonstrates an application of the EC method for Hg0 flux measurements over a grassland site with low soil Hg concentrations (< 100 ng g−1). The maximum flux detection limit derived from a zero-flux experiment in the laboratory was 0.22 ng m−2 h−1. The statistical estimate of the flux detection limit under real-world conditions was 5.9 (50 % cutoff) to 13.7 ng m−2 h−1 (95 % cutoff). The Eddy Mercury system overcomes the major uncertainties of other micrometeorological methods that have previously been used for Hg0 flux measurements associated with intermittent sampling at two different levels (aerodynamic gradient methods) and the stringent sampling and analytical requirements (relaxed eddy accumulation). The Eddy Mercury system will considerably facilitate ecosystem-scale Hg0 flux measurement because it features a fully automated operation, cutting down operation costs for technical maintenance by experienced staff, argon supply and consumables. Eddy Mercury has the potential to be established as a standard micrometeorological method for long-term Hg0 flux measurements over grasslands and other terrestrial ecosystems. Such standardization of measurements is strongly required to obtain comparable data and properly evaluate controlling factors on the NEE of Hg0 on larger spatial and temporal scales (Obrist et al., 2018). Ultimately, the Eddy Mercury system could complement air pollution and greenhouse gas measurements within the global network of micrometeorological tower sites (FluxNet) (Baldocchi et al., 2001). The Eddy Mercury system also comes at an opportune time to include NEE measurements of Hg0 in the joint WHO and UN Environment project to “develop a plan for global monitoring of human exposure to and environmental concentration of mercury”.
The research data that support the findings of this study are openly available at https://doi.org/10.3929/ethz-b-000393131 (Osterwalder et al., 2020).
All authors contributed to designing the study, testing the RA-915 AM in the laboratory and performing fieldwork. WE analyzed the data. Soil samples were taken by IF and analyzed for total mercury by MJ. IF analyzed the CO2 flux and meteorological data. SO and MJ coordinated the study. SO, WE and MJ wrote the paper with contributions from IF.
The authors declare that they have no conflict of interest.
We wish to acknowledge Nina Buchmann and Paul Linwood for scientific and on-site technical support, respectively. During fieldwork, we appreciated the technical and logistical assistance from Rudolf Osterwalder. We thank Ingvar Wängberg of the Swedish Environmental Research Institute (IVL) in Gothenburg for providing preliminary 1 Hz data of Hg0 in ambient air and encouraging the authors to carry out the present study. We thank the Federal Office of Meteorology and Climatology MeteoSwiss for providing data from the Cham (CHZ) weather station. We gratefully acknowledge the Lumex Instruments staff members, namely Vladimir Ryzhov, Sergey Sholupov and Georg Debus, for their enthusiasm and invaluable technical support on the RA-915 AM instrument and fruitful discussions during the field campaign and data analysis.
This research was funded by the Institute of Agricultural Sciences, ETH Zurich; the Department of Environmental Geosciences, University of Basel; and the Freiwillige Akademische Gesellschaft (FAG), Basel. Stefan Osterwalder received funding from the Swiss National Science Foundation (SNSF) Postdoc.Mobility grant (grant no. P400P2_180796) and the Research Fund for Junior Researchers of the University of Basel. Iris Feigenwinter was funded by the European Union Horizon 2020 Research and Innovation Programme (grant no. 774124). Martin Jiskra received funding from the SNSF Ambizione grant (grant no. PZ00P2_174101).
This paper was edited by Robyn Schofield and reviewed by two anonymous referees.
Agnan, Y., Le Dantec, T., Moore, C. W., Edwards, G. C., and Obrist, D.: New constraints on terrestrial surface–atmosphere fluxes of gaseous elemental mercury using a global database, Environ. Sci. Technol., 50, 507–524, https://doi.org/10.1021/acs.est.5b04013, 2016.
Allan, D. W.: Statistics of atomic frequency standards, P. IEEE, 54, 221–231, https://doi.org/10.1109/PROC.1966.4634, 1966.
Almeida, M. D., Marins, R. V., Paraquetti, H. H. M., Bastos, W. R., and Lacerda, L. D.: Mercury degassing from forested and open field soils in Rondônia, Western Amazon, Brazil, Chemosphere, 77, 60–66, https://doi.org/10.1016/j.chemosphere.2009.05.018, 2009.
Aubinet, M., Vesala, T., and Papale, D.: Eddy Covariance: A Practical Guide to Measurement and Data Analysis, Springer, Dordrecht, Heidelberg, London, New York, 438 pp., 2012.
Baldocchi, D., Falge, E., Gu, L. H., Olson, R., Hollinger, D., Running, S., Anthoni, P., Bernhofer, C., Davis, K., Evans, R., Fuentes, J., Goldstein, A., Katul, G., Law, B., Lee, X. H., Malhi, Y., Meyers, T., Munger, W., Oechel, W., U, K., Pilegaard, K., Schmid, H. P., Valentini, R., Verma, S., Vesala, T., Wilson, K., and Wofsy, S.: FLUXNET: A new tool to study the temporal and spatial variability of ecosystem-scale carbon dioxide, water vapor, and energy flux densities, B. Am. Meteorol. Soc., 82, 2415–2434, https://doi.org/10.1175/1520-0477(2001)082<2415:FANTTS>2.3.CO;2, 2001.
Bash, J. O. and Miller, D. R.: A relaxed eddy accumulation system for measuring surface fluxes of total gaseous mercury, J. Atmos. Ocean. Tech., 25, 244–257, https://doi.org/10.1175/2007JTECHA908.1, 2008.
Braune, B., Chetelat, J., Amyot, M., Brown, T., Clayden, M., Evans, M., Fisk, A., Gaden, A., Girard, C., Hare, A., Kirk, J., Lehnherr, I., Letcher, R., Loseto, L., Macdonald, R., Mann, E., McMeans, B., Muir, D., O'Driscoll, N., Poulain, A., Reimer, K., and Stern, G.: Mercury in the marine environment of the Canadian Arctic: Review of recent findings, Sci. Total Environ., 509, 67–90, https://doi.org/10.1016/j.scitotenv.2014.05.133, 2015.
Brown, R., Pirrone, N., van Hoek, C., Horvat, M., Kotnik, J., Wängberg, I., Corns, W., Bieber, E., and Sprovieri, F.: Standardization of a European measurement method for the determination of total gaseous mercury: results of the field trial campaign and determination of a measurement uncertainty and working range, Accredit. Qual. Assur., 15, 359–366, https://doi.org/10.1007/s00769-010-0636-2, 2010.
Burba, G. G., McDermitt, D. K., Grelle, A., Anderson, D. J., and Xu, L.: Addressing the influence of instrument surface heat exchange on the measurements of CO2 flux from open-path gas analyzers, Glob. Change Biol., 14, 1854–1876, https://doi.org/10.1111/j.1365-2486.2008.01606.x, 2008.
Businger, J. and Oncley, S.: Flux Measurement with Conditional Sampling, J. Atmos. Ocean. Tech., 7, 349–352, https://doi.org/10.1175/1520-0426(1990)007<0349:FMWCS>2.0.CO;2, 1990.
Businger, J. A.: Evaluation of the Accuracy with Which Dry Deposition Can Be Measured with Current Micrometeorological Techniques, J. Clim. Appl. Meteorol., 25, 1100–1124, https://doi.org/10.1175/1520-0450(1986)025<1100:EOTAWW>2.0.CO;2, 1986.
Castro, M. S. and Moore, C. W.: Importance of Gaseous Elemental Mercury Fluxes in Western Maryland, Atmosphere, 7, 110, https://doi.org/10.3390/atmos7090110, 2016.
Choi, H. D. and Holsen, T. M.: Gaseous mercury emissions from unsterilized and sterilized soils: The effect of temperature and UV radiation, Environ. Pollut., 157, 1673–1678, https://doi.org/10.1016/j.envpol.2008.12.014, 2009.
Cobos, D. R., Baker, J. M., and Nater, E. A.: Conditional sampling for measuring mercury vapor fluxes, Atmos. Environ., 36, 4309–4321, https://doi.org/10.1016/S1352-2310(02)00400-4, 2002.
Converse, A. D., Riscassi, A. L., and Scanlon, T. M.: Seasonal variability in gaseous mercury fluxes measured in a high-elevation meadow, Atmos. Environ., 44, 2176–2185, https://doi.org/10.1016/j.atmosenv.2010.03.024, 2010.
Demers, J. D., Driscoll, C. T., Fahey, T. J., and Yavitt, J. B.: Mercury cycling in litter and soil in different forest types in the Adirondack region, New York, USA, Ecol. Appl., 17, 1341–1351, 2007.
Driscoll, C. T., Mason, R. P., Chan, H. M., Jacob, D. J., and Pirrone, N.: Mercury as a global pollutant: sources, pathways, and effects, Environ. Sci. Technol., 47, 4967–4983, https://doi.org/10.1021/es305071v, 2013.
Eckley, C. S., Blanchard, P., McLennan, D., Mintz, R., and Sekela, M.: Soil–Air Mercury Flux near a Large Industrial Emission Source before and after Closure (Flin Flon, Manitoba, Canada), Environ. Sci. Technol., 49, 9750–9757, https://doi.org/10.1021/acs.est.5b01995, 2015.
Eckley, C. S., Tate, M. T., Lin, C.-J., Gustin, M., Dent, S., Eagles-Smith, C., Lutz, M. A., Wickland, K. P., Wang, B., Gray, J. E., Edwards, G. C., Krabbenhoft, D. P., and Smith, D. B.: Surface-air mercury fluxes across Western North America: A synthesis of spatial trends and controlling variables, Sci. Total Environ., 568, 651–665, https://doi.org/10.1016/j.scitotenv.2016.02.121, 2016.
Edwards, G. C., Rasmussen, P. E., Schroeder, W. H., Wallace, D. M., Halfpenny-Mitchell, L., Dias, G. M., Kemp, R. J., and Ausma, S.: Development and evaluation of a sampling system to determine gaseous mercury fluxes using an aerodynamic micrometeorological gradient method, J. Geophys. Res.-Atmos., 110, D10306, https://doi.org/10.1029/2004JD005187, 2005.
Enrico, M., Roux, G. L., Marusczak, N., Heimbürger, L.-E., Claustres, A., Fu, X., Sun, R., and Sonke, J. E.: Atmospheric Mercury Transfer to Peat Bogs Dominated by Gaseous Elemental Mercury Dry Deposition, Environ. Sci. Technol., 50, 2405–2412, https://doi.org/10.1021/acs.est.5b06058, 2016.
Ericksen, J. A., Gustin, M. S., Xin, M., Weisberg, P. J., and Fernandez, G. C. J.: Air–soil exchange of mercury from background soils in the United States, Sci. Total Environ., 366, 851–863, https://doi.org/10.1016/j.scitotenv.2005.08.019, 2006.
Eugster, W. and Merbold, L.: Eddy covariance for quantifying trace gas fluxes from soils, SOIL, 1, 187–205, https://doi.org/10.5194/soil-1-187-2015, 2015.
Eugster, W. and Plüss, P.: A fault-tolerant eddy covariance system for measuring CH4 fluxes, Agric. For. Meteorol., 150, 841–851, https://doi.org/10.1016/j.agrformet.2009.12.008, 2010.
Eugster, W. and Senn, W.: A cospectral correction model for measurement of turbulent NO2 flux, Bound.-Lay. Meteorol., 74, 321–340, https://doi.org/10.1007/BF00712375, 1995.
Eugster, W. and Zeeman, M. J.: Micrometeorological techniques to measure ecosystem-scale greenhouse gas fluxes for model validation and improvement, Int. Congr. Ser., 1293, 66–75, https://doi.org/10.1016/j.ics.2006.05.001, 2006.
Eugster, W., Zeyer, K., Zeeman, M., Michna, P., Zingg, A., Buchmann, N., and Emmenegger, L.: Methodical study of nitrous oxide eddy covariance measurements using quantum cascade laser spectrometery over a Swiss forest, Biogeosciences, 4, 927–939, https://doi.org/10.5194/bg-4-927-2007, 2007.
Faïn, X., Moosmüller, H., and Obrist, D.: Toward real-time measurement of atmospheric mercury concentrations using cavity ring-down spectroscopy, Atmos. Chem. Phys., 10, 2879–2892, https://doi.org/10.5194/acp-10-2879-2010, 2010.
Fitzgerald, W. F., Lamborg, C. H., and Hammerschmidt, C. R.: Marine biogeochemical cycling of mercury, Chem. Rev., 107, 641–662, https://doi.org/10.1021/cr050353m, 2007.
Fritsche, J., Obrist, D., Zeeman, M. J., Conen, F., Eugster, W., and Alewell, C.: Elemental mercury fluxes over a sub-alpine grassland determined with two micrometeorological methods, Atmos. Environ., 42, 2922–2933, https://doi.org/10.1016/j.atmosenv.2007.12.055, 2008a.
Fritsche, J., Wohlfahrt, G., Ammann, C., Zeeman, M., Hammerle, A., Obrist, D., and Alewell, C.: Summertime elemental mercury exchange of temperate grasslands on an ecosystem-scale, Atmos. Chem. Phys., 8, 7709–7722, https://doi.org/10.5194/acp-8-7709-2008, 2008b.
Fritsche, J., Obrist, D., and Alewell, C.: Evidence of microbial control of Hg0 emissions from uncontaminated terrestrial soils, J. Plant Nutr. Soil Sci., 171, 200–209, https://doi.org/10.1002/jpln.200625211, 2008c.
Fu, X. W., Feng, X. B., and Wang, S. F.: Exchange fluxes of Hg between surfaces and atmosphere in the eastern flank of Mount Gongga, Sichuan province, southwestern China, J. Geophys. Res.-Atmos., 113, D20306, https://doi.org/10.1029/2008JD009814, 2008a.
Fu, X. W., Feng, X. B., Wang, S. F., Qiu, G. L., and Li, P.: Mercury flux rate of two types of grasslands in Guiyang, Huanjing Kexue Yanjiu, 20, 33–37, 2008b (in Chinese with English Abstract).
Fuchs, K., Hörtnagl, L., Buchmann, N., Eugster, W., Snow, V., and Merbold, L.: Management matters: testing a mitigation strategy for nitrous oxide emissions using legumes on intensively managed grassland, Biogeosciences, 15, 5519–5543, https://doi.org/10.5194/bg-15-5519-2018, 2018.
Grigal, D. F.: Mercury sequestration in forests and peatlands: A review, J. Environ. Qual.,, 32, 393–405, 2003.
Gustin, M. S., Lindberg, S., Marsik, F., Casimir, A., Ebinghaus, R., Edwards, G., Hubble-Fitzgerald, C., Kemp, R., Kock, H., Leonard, T., London, J., Majewski, M., Montecinos, C., Owens, J., Pilote, M., Poissant, L., Rasmussen, P., Schaedlich, F., Schneeberger, D., Schroeder, W., Sommar, J., Turner, R., Vette, A., Wallschlaeger, D., Xiao, Z., and Zhang, H.: Nevada STORMS project: Measurement of mercury emissions from naturally enriched surfaces, J. Geophys. Res.-Atmos., 104, 21831–21844, https://doi.org/10.1029/1999JD900351, 1999.
Gustin, M. S., Biester, H., and Kim, C. S.: Investigation of the light-enhanced emission of mercury from naturally enriched substrates, Atmos. Environ., 36, 3241–3254, https://doi.org/10.1016/S1352-2310(02)00329-1, 2002.
Gustin, M. S., Huang, J., Miller, M. B., Peterson, C., Jaffe, D. A., Ambrose, J., Finley, B. D., Lyman, S. N., Call, K., Talbot, R., Feddersen, D., Mao, H., and Lindberg, S. E.: Do We Understand What the Mercury Speciation Instruments Are Actually Measuring? Results of RAMIX, Environ. Sci. Technol., 47, 7295–7306, https://doi.org/10.1021/es3039104, 2013.
Horst, T. W.: A simple formula for attenuation of eddy fluxes measured with first-order-response scalar sensors, Bound.-Lay. Meteorol., 82, 219–233, https://doi.org/10.1023/A:1000229130034, 1997.
Jaffe, D. A., Lyman, S., Amos, H. M., Gustin, M. S., Huang, J., Selin, N. E., Levin, L., Schure, A., Mason, R. P., Talbot, R., Rutter, A., Finley, B., Jaeglé, L., Shah, V., McClure, C., Ambrose, J., Gratz, L., Lindberg, S., Weiss-Penzias, P., Sheu, G.-R., Feddersen, D., Horvat, M., Dastoor, A., Hynes, A.J., Mao, H., Sonke, J. E., Slemr, F., Fisher, J. A., Ebinghaus, R., Zhang, Y., and Edwards, G. Progress on Understanding Atmospheric Mercury Hampered by Uncertain Measurements, Environ. Sci. Technol., 48, 7204–7206, https://doi.org/10.1021/es5026432, 2014.
Jiskra, M., Wiederhold, J. G., Skyllberg, U., Kronberg, R. M., Hajdas, I., and Kretzschmar, R.: Mercury deposition and re-emission pathways in boreal forest soils investigated with Hg isotope signatures, Environ. Sci. Technol., 49, 7188–7196, https://doi.org/10.1021/acs.est.5b00742, 2015.
Jiskra, M., Sonke, J. E., Obrist, D., Bieser, J., Ebinghaus, R., Myhre, C. L., Pfaffhuber, K. A., Wängberg, I., Kyllönen, K., Worthy, D., Martin, L. G., Labuschagne, C., Mkololo, T., Ramonet, M., Magand, O., and Dommergue, A.: A vegetation control on seasonal variations in global atmospheric mercury concentrations, Nat. Geosci., 11, 244–250, https://doi.org/10.1038/s41561-018-0078-8, 2018.
Kaimal, J. C. and Gaynor, J. E.: Another look at sonic thermometry, Bound.-Lay. Meteorol., 56, 401–410, https://doi.org/10.1007/BF00119215, 1991.
Kaimal, J. C., Wyngaard, J. C., Izumi, Y., and Coté, O. R.: Spectral characteristics of surface-layer turbulence, Q. J. Roy. Meteor. Soc., 98, 563–589, https://doi.org/10.1002/qj.49709841707, 1972.
Kamp, J., Skov, H., Jensen, B., and Sørensen, L. L.: Fluxes of gaseous elemental mercury (GEM) in the High Arctic during atmospheric mercury depletion events (AMDEs), Atmos. Chem. Phys., 18, 6923–6938, https://doi.org/10.5194/acp-18-6923-2018, 2018.
Kljun, N., Calanca, P., Rotach, M. W., and Schmid, H. P.: A simple two-dimensional parameterisation for Flux Footprint Prediction (FFP), Geosci. Model Dev., 8, 3695–3713, https://doi.org/10.5194/gmd-8-3695-2015, 2015.
Lee, X., Finnigan, J., and Paw U, K.T.: Coordinate Systems and Flux Bias Error, in: Handbook of Micrometeorology: A Guide for Surface Flux Measurement and Analysis, edited by: Lee, X., Massman, W., and Law, B., Atmospheric and Oceanographic Sciences Library, Springer Netherlands, Dordrecht, 33–66, https://doi.org/10.1007/1-4020-2265-4_3, 2005.
Lenschow, D. H. and Raupach, M. R.: The attenuation of fluctuations in scalar concentrations through sampling tubes, J. Geophys. Res., 96, 15259–15268, https://doi.org/10.1029/91JD01437, 1991.
Leuning, R. and King, K. M.: Comparison of eddy-covariance measurements of CO2 fluxes by open- and closed-path CO2 analysers, Bound.-Lay. Meteorol., 59, 297–311, https://doi.org/10.1007/BF00119818, 1992.
Lin, C. J. and Pehkonen, S. O.: The chemistry of atmospheric mercury: a review, Atmos. Environ., 33, 2067–2079, https://doi.org/10.1016/S1352-2310(98)00387-2, 1999.
Lindberg, S., Bullock, R., Ebinghaus, R., Engstrom, D., Feng, X., Fitzgerald, W., Pirrone, N., Prestbo, E., and Seigneur, C.: A synthesis of progress and uncertainties in attributing the sources of mercury in deposition, Ambio, 36, 19–32, https://doi.org/10.1579/0044-7447(2007)36[19:asopau]2.0.co;2, 2007.
Lyman, S. N., Cheng, I., Gratz, L. E., Weiss-Penzias, P., and Zhang, L.: An updated review of atmospheric mercury, Sci. Total Environ., 707, 135575, https://doi.org/10.1016/j.scitotenv.2019.135575, 2020.
Mason, R. P., Choi, A. L., Fitzgerald, W. F., Hammerschmidt, C. R., Lamborg, C. H., Soerensen, A. L., and Sunderland, E. M.: Mercury biogeochemical cycling in the ocean and policy implications, Environ. Res., 119, 101–117, https://doi.org/10.1016/j.envres.2012.03.013, 2012.
Mauder, M. and Foken, T.: Documentation and instruction manual of the eddy covariance software package TK2, Work Report, University of Bayreuth, Bayreuth, 2004.
McMillen, R. T.: An Eddy-Correlation Technique with Extended Applicability to Non-Simple Terrain, Bound.-Lay. Meteorol., 43, 231–245, https://doi.org/10.1007/BF00128405, 1988.
Merbold, L., Eugster, W., Stieger, J., Zahniser, M., Nelson, D., and Buchmann, N.: Greenhouse gas budget (CO2, CH4 and N2O) of intensively managed grassland following restoration, Glob. Change Biol., 20, 1913–1928, https://doi.org/10.1111/gcb.12518, 2014.
MeteoSchweiz: Klimareport 2018, Bundesamt für Meteorologie und Klimatologie MeteoSchweiz, Zürich, 94 pp., 2019.
Miller, M. B., Gustin, M. S., and Edwards, G. C.: Reactive mercury flux measurements using cation exchange membranes, Atmos. Meas. Tech. Discuss., https://doi.org/10.5194/amt-2018-360, 2018.
Moncrieff, J., Clement, R., Finnigan, J., and Meyers, T.: Averaging, detrending, and filtering of eddy covariance time series, in: Handbook of Micrometeorology: a guide for surface flux measurement and analysis, 29, edited by: Law, B. E., Lee, X., and Massmann, W. J., Kluwer Academic, Dordrecht, 7–31, 2004.
Montgomery, R. B.: Vertical eddy flux of heat in the atmosphere, J. Meteorol., 5, 265–274, https://doi.org/10.1175/1520-0469(1948)005<0265:VEFOHI>2.0.CO;2, 1948.
Moore, C. and Carpi, A.: Mechanisms of the emission of mercury from soil: Role of UV radiation, J. Geophys. Res.-Atmos., 110, D24302, https://doi.org/10.1029/2004JD005567, 2005.
Obrist, D., Conen, F., Vogt, R., Siegwolf, R., and Alewell, C.: Estimation of Hg0 exchange between ecosystems and the atmosphere using 222Rn and Hg0 concentration changes in the stable nocturnal boundary layer, Atmos. Environ., 40, 856–866, https://doi.org/10.1016/j.atmosenv.2005.10.012, 2006.
Obrist, D., Agnan, Y., Jiskra, M., Olson, C. L., Colegrove, D. P., Hueber, J., Moore, C. W., Sonke, J. E., and Helmig, D.: Tundra uptake of atmospheric elemental mercury drives Arctic mercury pollution, Nature, 547, 201–204, https://doi.org/10.1038/nature22997, 2017.
Obrist, D., Kirk, J. L., Zhang, L., Sunderland, E. M., Jiskra, M., and Selin, N. E.: A review of global environmental mercury processes in response to human and natural perturbations: Changes of emissions, climate, and land use, Ambio, 47, 116–140, https://doi.org/10.1007/s13280-017-1004-9, 2018.
Obukhov, A. M.: Characteristics of the Micro-structure of the Wind in the Surface Layer of the Atmosphere, Izv. AN SSSR, Ser. Geofiz. 3, 49–68, 1951.
Osterwalder, S., Fritsche, J., Alewell, C., Schmutz, M., Nilsson, M. B., Jocher, G., Sommar, J., Rinne, J., and Bishop, K.: A dual-inlet, single detector relaxed eddy accumulation system for long-term measurement of mercury flux, Atmos. Meas. Tech., 9, 509–524, https://doi.org/10.5194/amt-9-509-2016, 2016.
Osterwalder, S., Bishop, K., Alewell, C., Fritsche, J., Laudon, H., Åkerblom, S., and Nilsson, M. B.: Mercury evasion from a boreal peatland shortens the timeline for recovery from legacy pollution, Sci. Rep.-UK, 7, 16022, https://doi.org/10.1038/s41598-017-16141-7, 2017.
Osterwalder, S., Sommar, J., Åkerblom, S., Jocher, G., Fritsche, J., Nilsson, M. B., Bishop, K., and Alewell, C.: Comparative study of elemental mercury flux measurement techniques over a Fennoscandian boreal peatland, Atmos. Environ., 172, 16–25, https://doi.org/10.1016/j.atmosenv.2017.10.025, 2018.
Osterwalder, S., Huang, J.-H., Shetaya, W. H., Agnan, Y., Frossard, A., Frey, B., Alewell, C., Kretzschmar, R., Biester, H., and Obrist, D.: Mercury emission from industrially contaminated soils in relation to chemical, microbial, and meteorological factors, Environ. Pollut., 250, 944–952, https://doi.org/10.1016/j.envpol.2019.03.093, 2019.
Osterwalder, S., Eugster, W., Feigenwinter, I., and Jiskra, M.: Dataset of eddy covariance flux measurements of gaseous elemental mercury over a grassland, ETH Bibliography, https://doi.org/10.3929/ethz-b-000393131, 2020.
Outridge, P. M., Mason, R. P., Wang, F., Guerrero, S., and Heimbürger-Boavida, L. E.: Updated Global and Oceanic Mercury Budgets for the United Nations Global Mercury Assessment 2018, Environ. Sci. Technol., 52, 11466–11477, https://doi.org/10.1021/acs.est.8b01246, 2018.
Pierce, A., Obrist, D., Moosmüller, H., Faïn, X., and Moore, C.: Cavity ring-down spectroscopy sensor development for high-time-resolution measurements of gaseous elemental mercury in ambient air, Atmos. Meas. Tech., 6, 1477–1489, https://doi.org/10.5194/amt-6-1477-2013, 2013.
Pierce, A. M., Moore, C.W., Wohlfahrt, G., Hörtnagl, L., Kljun, N., and Obrist, D.: Eddy covariance flux measurements of gaseous elemental mercury using cavity ring-down spectroscopy, Environ. Sci. Technol., 49, 1559–1568, https://doi.org/10.1021/es505080z, 2015.
Poissant, L. and Casimir, A.: Water-air and soil-air exchange rate of total gaseous mercury measured at background sites, Atmos. Environ., 32, 883–893, https://doi.org/10.1016/S1352-2310(97)00132-5, 1998.
Poissant, L., Pilote, M., and Casimir, A.: Mercury flux measurements in a naturally enriched area: Correlation with environmental conditions during the Nevada Study and tests of the release of mercury from soils (STORMS), J. Geophys. Res.-Atmos., 104, 21845–21857, https://doi.org/10.1029/1999JD900092, 1999.
R Core Team: R: A language and environment for statistical computing, R Foundation for Statistical Computing, Vienna, Austria, 2018.
Roth, K.: Bodenkartierung und GIS-basierte Kohlenstoffinventur von Graslandböden, Diploma Thesis, University of Zurich, 2006.
Saiz-Lopez, A., Sitkiewicz, S. P., Roca-Sanjuán, D., Oliva-Enrich, J. M., Dávalos, J. Z., Notario, R., Jiskra, M., Xu, Y., Wang, F., Thackray, C. P., Sunderland, E. M., Jacob, D. J., Travnikov, O., Cuevas, C. A., Acuña, A. U., Rivero, D., Plane, J. M. C., Kinnison, D. E., and Sonke, J. E.: Photoreduction of gaseous oxidized mercury changes global atmospheric mercury speciation, transport and deposition, Nat. Commun., 9, 4796, https://doi.org/10.1038/s41467-018-07075-3, 2018.
Schroeder, W. H., Beauchamp, S., Edwards, G., Poissant, L., Rasmussen, P., Tordon, R., Dias, G., Kemp, J., Van Heyst, B., and Banic, C. M.: Gaseous mercury emissions from natural sources in Canadian landscapes, J. Geophys. Res.-Atmos., 110, D18302, https://doi.org/10.1029/2004JD005699, 2005.
Sholupov, S. and Ganeyev, A.: Zeeman atomic absorption spectrometry using high frequency modulated light polarization, Spectrochim. Acta B, 50, 1227–1236, https://doi.org/10.1016/0584-8547(95)01316-7, 1995.
Sholupov, S., Pogarev, S., Ryzhov, V., Mashyanov, N., and Stroganov, A.: Zeeman atomic absorption spectrometer RA-915+ for direct determination of mercury in air and complex matrix samples, Fuel Process. Technol., 85, 473–485, https://doi.org/10.1016/j.fuproc.2003.11.003, 2004.
Sommar, J., Zhu, W., Lin, C.-J., and Feng, X.: Field Approaches to Measure Hg Exchange Between Natural Surfaces and the Atmosphere A Review, Crit. Rev. Environ. Sci. Technol. 43, 1657–1739, https://doi.org/10.1080/10643389.2012.671733, 2013a.
Sommar, J., Zhu, W., Shang, L., Feng, X., and Lin, C.-J.: A whole-air relaxed eddy accumulation measurement system for sampling vertical vapour exchange of elemental mercury, Tellus B, 65, 19940, https://doi.org/10.3402/tellusb.v65i0.19940, 2013b.
Sprovieri, F., Pirrone, N., Bencardino, M., D'Amore, F., Carbone, F., Cinnirella, S., Mannarino, V., Landis, M., Ebinghaus, R., Weigelt, A., Brunke, E.-G., Labuschagne, C., Martin, L., Munthe, J., Wängberg, I., Artaxo, P., Morais, F., Barbosa, H. D. M. J., Brito, J., Cairns, W., Barbante, C., Diéguez, M. D. C., Garcia, P. E., Dommergue, A., Angot, H., Magand, O., Skov, H., Horvat, M., Kotnik, J., Read, K. A., Neves, L. M., Gawlik, B. M., Sena, F., Mashyanov, N., Obolkin, V., Wip, D., Feng, X. B., Zhang, H., Fu, X., Ramachandran, R., Cossa, D., Knoery, J., Marusczak, N., Nerentorp, M., and Norstrom, C.: Atmospheric mercury concentrations observed at ground-based monitoring sites globally distributed in the framework of the GMOS network, Atmos. Chem. Phys., 16, 11915–11935, https://doi.org/10.5194/acp-16-11915-2016, 2016.
Stannard, D. I.: A theoretically based determination of bowen-ratio fetch requirements, Bound.-Lay. Meteorol., 83, 375–406, https://doi.org/10.1023/A:1000286829849, 1997.
Swinbank, W. C.: The measurement of vertical transfer of heat and water vapor by eddies in the lower atmosphere, J. Meteorol., 8, 135–145, https://doi.org/10.1175/1520-0469(1951)008<0135:TMOVTO>2.0.CO;2, 1951.
UN Environment: Global Mercury Assessment 2013: Sources, Emissions, Releases and Environmental Transport, UNEP Chemicals Branch, Geneva, Switzerland, 2013.
UN Environment: Global Mercury Assessment 2018, UN Environment Programme, Chemicals and Health Branch Geneva, Switzerland, 2019.
Wang, X., Lin, C.-J., Yuan, W., Sommar, J., Zhu, W., and Feng, X.: Emission-dominated gas exchange of elemental mercury vapor over natural surfaces in China, Atmos. Chem. Phys., 16, 11125–11143, https://doi.org/10.5194/acp-16-11125-2016, 2016.
Watras, C. J., Back, R. C., Halvorsen, S., Hudson, R. J. M., Morrison, K. A., and Wente, S. P.: Bioaccumulation of mercury in pelagic freshwater food webs, Sci. Total. Environ., 219, 183–208, https://doi.org/10.1016/S0048-9697(98)00228-9,1998.
Webb, E. K., Pearman, G. I., and Leuning, R.: Correction of flux measurements for density effects due to heat and water vapour transfer, Q. J. Roy. Meteor. Soc., 106, 85–100, https://doi.org/10.1002/qj.49710644707, 1980.
Werle, P., Mücke, R., and Slemr, F.: The limits of signal averaging in atmospheric trace-gas monitoring by tunable diode-laser absorption spectroscopy (TDLAS), Appl. Phys. B, 57, 131–139, https://doi.org/10.1007/BF00425997, 1993.
Wienhold, F. G., Fischer, H., and Harris, G. W.: Fast response tunable diode laser spectroscopy for trace gas flux measurements, Infrared Phys. Techn., 37, 67–74, https://doi.org/10.1016/1350-4495(95)00114-X, 1996.
Yuan, W., Sommar, J., Lin, C.-J., Wang, X., Li, K., Liu, Y., Zhang, H., Lu, Z., Wu, C., and Feng, X.: Stable Isotope Evidence Shows Re-emission of Elemental Mercury Vapor Occurring after Reductive Loss from Foliage, Environ. Sci. Technol., 53, 651–660, https://doi.org/10.1021/acs.est.8b04865, 2019.
Zeeman, M. J., Hiller, R., Gilgen, A. K., Michna, P., Plüss, P., Buchmann, N., and Eugster, W.: Management and climate impacts on net CO2 fluxes and carbon budgets of three grasslands along an elevational gradient in Switzerland, Agr. Forest Meteorol., 150, 519–530, https://doi.org/10.1016/j.agrformet.2010.01.011, 2010.
Zhang, H., Lindberg, S. E., Marsik, F. J., and Keeler, G. J.: Mercury Air/Surface Exchange Kinetics of Background Soils of the Tahquamenon River Watershed in the Michigan Upper Peninsula, Water Air Soil Poll., 126, 151–169, https://doi.org/10.1023/A:1005227802306, 2001.
Zhang, L., Wu, Z., Cheng, I., Wright, L. P., Olson, M. L., Gay, D. A., Risch, M. R., Brooks, S., Castro, M. S., Conley, G. D., Edgerton, E. S., Holsen, T. M., Luke, W., Tordon, R., and Weiss-Penzias, P.: The estimated six-year mercury dry deposition across North America, Environ. Sci. Technol., 50, 12864–12873, https://doi.org/10.1021/acs.est.6b04276, 2016.
Zheng, W., Obrist, D., Weis, D., and Bergquist, B. A.: Mercury isotope compositions across North American forests, Global Biogeochem. Cy., 30, 1475–1492, https://doi.org/10.1002/2015GB005323, 2016.
Zhu, W., Sommar, J., Lin, C.-J., and Feng, X.: Mercury vapor air–surface exchange measured by collocated micrometeorological and enclosure methods – Part I: Data comparability and method characteristics, Atmos. Chem. Phys., 15, 685–702, https://doi.org/10.5194/acp-15-685-2015, 2015a.
Zhu, W., Sommar, J., Lin, C.-J., and Feng, X.: Mercury vapor air–surface exchange measured by collocated micrometeorological and enclosure methods – Part II: Bias and uncertainty analysis, Atmos. Chem. Phys., 15, 5359–5376, https://doi.org/10.5194/acp-15-5359-2015, 2015b.
Zhu, W., Lin, C.-J., Wang, X., Sommar, J., Fu, X., and Feng, X.: Global observations and modeling of atmosphere–surface exchange of elemental mercury: a critical review, Atmos. Chem. Phys., 16, 4451–4480, https://doi.org/10.5194/acp-16-4451-2016, 2016.
Zhu, W., Li, Z., Li, P., Yu, B., Lin, C.-J., Sommar, J., and Feng, X.: Re-emission of legacy mercury from soil adjacent to closed point sources of Hg emission, Environ. Pollut., 242, 718–727, https://doi.org/10.1016/j.envpol.2018.07.002, 2018.