the Creative Commons Attribution 4.0 License.
the Creative Commons Attribution 4.0 License.
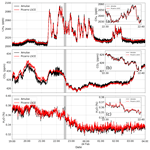
The development of the Atmospheric Measurements by Ultra-Light Spectrometer (AMULSE) greenhouse gas profiling system and application for satellite retrieval validation
Lilian Joly
Olivier Coopmann
Vincent Guidard
Thomas Decarpenterie
Nicolas Dumelié
Julien Cousin
Jérémie Burgalat
Nicolas Chauvin
Grégory Albora
Rabih Maamary
Zineb Miftah El Khair
Diane Tzanos
Joël Barrié
Éric Moulin
Patrick Aressy
Anne Belleudy
We report in this paper the development of an embedded ultralight spectrometer (<3 kg) based on tuneable diode laser absorption spectroscopy (with a sampling rate of 24 Hz) in the mid-infrared spectral region. This instrument is dedicated to in situ measurements of the vertical profile concentrations of three main greenhouse gases – carbon dioxide (CO2), methane (CH4) and water vapour (H2O) – via standard weather and tethered balloons. The plug and play instrument is compact, robust, cost-effective, and autonomous. The instrument also has low power consumption and is non-intrusive.
It was first calibrated during an in situ experiment on an ICOS (Integrated Carbon Observation System) site for several days, then used in two experiments with several balloon flights of up to 30 km altitude in the Reims region of France in 2017–2018 in collaboration with Météo-France CNRM (Centre National de Recherches Météorologiques).
This paper shows the valuable interest of the data measured by the AMULSE (Atmospheric Measurements by Ultra-Light Spectrometer) instrument during the APOGEE (Atmospheric Profiles of Greenhouse Gases) measurement experiment, specifically for the vertical profiles of CO2 and CH4, measurements of which remain very sparse. We have carried out several experiments showing that the measured profiles have several applications: the validation of simulations of infrared satellite observations, evaluating the quality of chemical profiles from chemistry transport models (CTMs) and evaluating the quality of retrieved chemical profiles from the assimilation of infrared satellite observations. The results show that the simulations of infrared satellite observations from IASI (Infrared Atmospheric Sounding Interferometer) and CrIS (Cross-track Infrared Sounder) instruments performed in operational mode for numerical weather prediction (NWP) by the radiative transfer model (RTM) RTTOV (Radiative Transfer for the TIROS Operational Vertical Sounder) are of good quality. We also show that the MOCAGE (Modèle de Chimie Atmosphérique de Grande Echelle) and CAMS (Copernicus Atmospheric Monitoring Service) CTMs modelled ozone profiles fairly accurately and that the CAMS CTM represents the methane in the troposphere well compared to MOCAGE. Finally, the measured in situ ozone profiles allowed us to show the good quality of the retrieved ozone profiles by assimilating ozone-sensitive infrared spectral radiances from the IASI and CrIS.
- Article
(5192 KB) - Full-text XML
- BibTeX
- EndNote
The climate of the Earth is currently undergoing rapid change. During the last few decades, evidence has accumulated that this climate change is directly related to human activities (IPCC, 2013; IPCC, 2019; Blunden and Arndt, 2019). The abrupt acceleration of technological progress and the explosion of industrial and agricultural activities, as well as the multiplication of the means of transport, have created a profound change in our environment, gradually modifying the chemical composition on the atmosphere at a global level. In particular, the minority chemical compounds of the atmosphere have a fundamental impact on the regulation of the radiative balance of the planet. Indeed, certain gases have the property of absorbing a part of the terrestrial infrared radiation. These gases called greenhouse gases (GHGs) are naturally present in the atmosphere (water vapour (H2O), carbon dioxide (CO2), methane (CH4), nitrogen oxide (NOx), etc.). The energy that they collect is then returned in all directions, at the same time not only towards space but also towards the surface and the various layers of the atmosphere. It is the natural greenhouse effect which makes it possible to have average surface temperatures of approximately 15 ∘C instead of the −18 ∘C that would be the case with terrestrial radiation if the atmosphere were transparent. Any modification of the atmospheric concentration of a GHG induces a modification of the climatic equilibrium. Thus, the atmosphere is certainly the medium most rapidly affected by the disturbances of the equilibrium of the environment, whether natural, as during major climate cycles, or linked to human activities. The main greenhouse gases related to human activities are CO2, CH4 and N2O.
Satellite observations have brought information of thermodynamic structure and atmospheric composition using hyperspectral infrared sounders such as the IASI (Infrared Atmospheric Sounding Interferometer), CrIS (Cross-track Infrared Sounder) or AIRS (Atmospheric Infrared Sounder). It is important to assess the quality of the atmospheric information provided by these instruments. Thus, several works have been carried out in order to validate the atmospheric profile retrievals (temperature, humidity, trace gases, etc.) from infrared satellite observations. Examples include those for IASI (Hilton et al., 2012), CrIS (Nalli et al., 2017a, b) or AIRS (Susskind et al., 2003; Chahine et al., 2005) or the work of Liang et al. (2017) that evaluated XCO2 satellite retrievals obtained from the Greenhouse Gases Observing Satellite (GOSAT) and Orbiting Carbon Observatory-2 (OCO-2) instruments by comparison with total carbon dioxide column observations measured by the international Total Carbon Column Observing Network (TCCON).
These observations are crucial to the study of, understanding of and follow-up work on atmospheric compounds to monitor greenhouse gases. Météo-France operational numerical weather prediction (NWP) systems use RTTOV (Radiative Transfer for the TIROS Operational Vertical Sounder) as a radiative transfer model (RTM) during data assimilation, as in many other NWP centres. In order to assimilate the satellite sounder observations, the actual observations have to be compared to the simulation from the model state with a RTM. Simulating infrared satellite observations from a RTM requires a priori knowledge of the state of the atmosphere and its chemical composition. The RTTOV uses chemical reference profiles that are constant in time and in space. This approximation may lead to possible errors in the simulations. The high quality of simulations is essential since the information extracted from these models is then used in data assimilation systems for weather forecasting, which is why there is a need to assess the quality of chemical profiles with in situ measurements.
In addition to the NWP models, chemistry transport models (CTMs) are available. Indeed, air pollution is a public health issue especially in big cities and is responsible for 790 000 deaths per year just in Europe (Lelieveld et al., 2019). One of the main pollutants in the troposphere is ozone which increases the death rate during pollution episodes. At large scales, the effect of global change continues to be important due to the increase in GHGs whose main contributors are carbon dioxide and methane. A CTM such as MOCAGE (Modèle de Chimie Atmosphérique de Grande Echelle) at Météo-France and C-IFS (Atmospheric Chemistry in the Integrated Forecasting System) of CAMS (Copernicus Atmospheric Monitoring Service) at the ECMWF (European Centre for Medium-Range Weather Forecasts) allows for the production of high-quality forecast of chemistry fields in the stratosphere and upper troposphere but forecast quality is weaker in the UTLS (upper troposphere–lower stratosphere). However, satellite sounding in the atmospheric boundary layer is more difficult, especially for infrared sensors because of cloud and aerosols that interfere with the signal; land surface emissivity and temperature uncertainties also are part of the problem. The data assimilation of satellite observations sensitive to atmospheric composition allows for an accurate chemical description of the atmosphere, especially in the UTLS, to be obtained. Presently, C-IFS assimilates Level 2 products from several satellite instruments. To assess the quality of the CTM forecasts of chemical fields, we need to have accurate measurements of these compounds in the atmospheric column. Despite many chemistry measurements at ground stations, information on chemistry is not widely available at high altitudes. To overcome this lack of data, many projects have been started such as the APOGEE (Atmospheric Profiles of Greenhouse Gases) experiment. The APOGEE experiment is derived from a collaboration between the GSMA (Groupe de Spectrométrie Moléculaire et Atmosphérique) at Reims University in France, LSCE (Laboratoire des Sciences du Climat et de l'Environnement) in France and CNRM (Centre National de Recherches Météorologiques) at Météo-France. The objective of the APOGEE experiment is to realize measurements of pressure, temperature, humidity and the main atmospheric chemical vertical profiles (CO2, CH4, O3 and H2O) at heights of up to 30 km including the atmospheric boundary layer. Ozone profile is measured using Vaisala in situ soundings with electrochemical cells. CO2, CH4 and H2O are measured using the AMULSE (Atmospheric Measurement Ultralight Spectrometer) instrument developed by the GSMA.
In Sect. 2, we will identify some ways to measure the chemical composition of the atmosphere. Then in Sect. 3, we will describe the different characteristics of the AMULSE instrument as well as make comparisons with another instrument. Finally, we will use the data from the APOGEE measurement experiment on one study case to evaluate the sensitivity of infrared observation simulations to CO2, CH4 and O3 information and we will use the in situ profiles to evaluate a priori profiles from the CTMs and retrieval profiles by 1D assimilation experiments.
2.1 Molecules of interest
CO2 and CH4 are long-lived gases. Atmospheric residence time is a few decades for CH4, whose reactivity makes it an important player in atmospheric chemistry (Voulgarakis et al., 2013), and a few hundred to thousands of years for CO2 which is inert in the atmosphere from a chemical point of view (Archer and Brovkin, 2008; Eby et al., 2009). The increase in these gases in the atmosphere is conditioned by anthropogenic emissions of them which add up to an active natural cycle. The CO2 cycle is the carbon cycle as this gas dominates the atmospheric composition of carbon compounds in terms of mass (nearly 215 times higher than that of methane). However, the effectiveness of CH4 in absorbing infrared radiation is much greater than that of carbon dioxide. Since the beginning of the industrial era in 1750 till October 2016, the mixing ratio of atmospheric CO2 has increased from around 280 to 401 ppm, an increase of more than 40 %. The increases in the CO2 and CH4 levels along with the uncertainty in H2O levels at high altitudes upset the radiative balance of the planet. Therefore, having information and data about the vertical distribution of these three GHGs is very useful for improving our knowledge of the future of our climate. Hence, we should improve the knowledge and estimation of the regional anthropogenic GHG natural sinks and emission sources for better quantification by
-
enhancing the atmospheric chemistry–transport models that are used to link the sources and the sinks to the atmospheric concentrations and
-
increasing atmospheric observations and measurements.
2.2 GHG atmospheric observations
The goal of atmospheric observations of GHGs is to follow the evolution of these gases. According to the IPCC (Intergovernmental Panel on Climate Change), this is necessary to be able to determine both the long-term trend related to global emissions, a seasonal cycle linked to vegetation activity or the availability of OH, and a synoptic variability linked to the transport of air masses over periods of time from a few hours to a few days. Typically, in 1 year, the air of the Northern Hemisphere is mixed with that of the Southern Hemisphere and vice versa. At mid-latitudes in the Northern Hemisphere, emissions are transported around the Earth in a few days by a zonal circulation of air masses that is much more efficient than mixing at latitudes. By nature, in situ measurements, therefore, require continuous and diversified observation means on a global scale: ground measurements (Integrated Carbon Observation System (ICOS), World Meteorological Organization (WMO), etc.), airborne measurements (Cammas et al., 2008; Filges et al., 2015; Nédélec et al., 2015; Petzold et al., 2015), satellite observations (Crevoisier et al., 2013; Thompson et al., 2012; Wecht et al., 2014) and vertical measurements using balloons (Ghysels et al., 2016). Tethered balloons measurements can be used at up to 800 m altitude, and they are cheap and allowed in France. They do not require a lot of preparation and logistics, and they offer controlled travelling speed as well as fixed-point measurements along with good payload options. On the other hand, a weather balloon offers high-altitude measurements (up to 30 km) but fixed-point measurements cannot be realized. In our previous papers (Joly et al., 2016; Khair et al., 2017) we discussed the advantages and disadvantages of the different ground and airborne measurement techniques as well as the need for observations on the vertical plane along the atmospheric column in order to complete spatial measurements. To complement the current observational system, stratospheric balloons are unique scientific research tools for accessing the stratosphere, an area inaccessible to airborne measurement methods. The information provided by the satellites is an integrated average over all or part of the atmospheric column with the exception of a few measurements taken at the limb which provide information with a low vertical resolution. The balloons’ ability to access the profiles makes them an ideal tool to explore the distribution of many atmospheric parameters between the surface and 40 km of altitude in a strategic zone where the masses of air mix a large number of particles and chemical compounds emitted from the Earth. We report in this paper the development of a lightweight instrument called AMULSE (for Atmospheric Measurements by Ultra-Light Spectrometer) that fulfils the requirements for weather balloon flights to increase atmospheric GHG measurements. This instrument was first tested at ground level during an intercomparison with the Picarro instrument of the LSCE laboratory in Paris, member of the ICOS network. It was then deployed for in situ measurements on tethered balloons and on a weather balloon up to the stratosphere at about a 30 km altitude for CO2 and CH4 while we simultaneously measured the H2O up to a 10 km altitude in order to calculate the mixing ratios in the dry air column. High-precision measurements and a vertical resolution of 5–7 m for in situ concentration profiles is achieved by using the diode laser spectroscopic technique (Durry and Megie, 1999a; Joly et al., 2007, 2016) combined with a weather balloon and tethered balloons. The developed optical sensor is then based on mid-infrared absorption spectroscopy which provides a compact, cost-effective, fully autonomous, low-power-consuming and non-intrusive probe to measure the targeted gases in the atmosphere using an open-path multipass cell.
In recent years, the AMULSE instrument has evolved in order to offer a single-gas CO2 version in 2014, a single-gas CH4 version in 2015, a dual-gases version in 2016 (CO2−CH4) and a tri-gas version () in 2017, still weighing less than 3 kg. We are also working to improve the accuracy and robustness of the instrument.
3.1 Principle
The high selectivity and sensitivity in the gases detection realized using the diode laser absorption spectroscopy is considered as the most advantageous technique for measuring atmospheric gas concentrations (Durry and Megie, 1999b). It is based on semiconductor diode lasers because they offer a continuous-mode emission, are tuneable and have a relatively low noise amplitude. The diodes used in this work emit in the near-infrared spectral region (NIR) where most atmospheric pollutant molecules feature suitable absorption lines. Direct absorption spectroscopy is the simplest application of this technique, and it is well adapted to in situ measurements. It requires that the tuneable laser beam with an intensity of I0 passes through the gas sample on a distance L and is then measured using a detector. When the frequency of the emitted light is close to a molecular transition ν0 of the gaseous sample, the light is then absorbed and the transmitted intensity I(ν) decreases. The concentration of the absorbing species in the gas mixture is then calculated according to Beer's law.
3.2 Technical description
The architecture of AMULSE is similar to that used in our previous papers (Joly et al., 2016; El Khair et al., 2017). This new version is equipped with two lasers (purchased from nanoplus GmbH, Gerbrunn, Germany). The first one is a GaSb-based distributed feedback (DFB) semiconductor diode emitting at 2.004 µm. A simple change on its scanning ramp allows us to target the CO2 (4992.51 cm−1) and H2O (4992.94 cm−1) molecules simultaneously in the same scanning window. The second laser is an interband cascade laser (ICL) DFB diode emitting at 3.24 µm detailed by El Khair et al. (2017) used to target the CH4 molecules (R(6) transition at 3085.86 cm−1). The lasers' driving current is ramped at 24 Hz (42 ms), ensuring a good spatial resolution (1–20 m) depending on the ascent and descent flight speed. After collimation, the laser beams then pass into a homemade open-path Herriott multipass cell achieving an optical path length of 16 m on the channel and 18 m on the CH4 channel. The mirrors are heated in order to avoid the condensation on their surfaces using 2 W heaters.
At the output of the cell, both laser beams are focalized onto the two photodetectors (purchased from Teledyne Judson Technologies, Montgomeryville, PA, USA). The central processing unit (National Instruments real-time module) records all data (spectra, pressure, temperature and humidity (PTU) and GPS from the on-board meteorological in situ sounding). The spectrometer weighs less than 3 kg in a flight-ready condition, and power supplies ensure about 6 h of operation. When operated under weather balloons, the spectrometer is equipped with a satellite communication system based on an iridium element. The iridium module sends various data such as meteorological parameters and GPS localization as well as monitoring parameters of the instrument during the flights. Under a tethered balloon, a Wi-Fi module replaces the iridium, allowing us to send to a ground station the recorded spectra in order to achieve nearly real-time data processing and hence determine the position and the concentration of the plume.
3.3 AMULSE compared to Picarro (2015–2018)
Each time AMULSE evolved, we made different comparisons with measurements from an ICOS site in order to characterize the impact of instrumental modifications. The table shows the improvement in sensitivity between 2015 and 2018 (Table 1). This improvement comes from the optimization of the optical cell, electronics and spectroscopy.
Table 1Evolution between 2015 (with two single-gas versions for CO2 and CH4) and 2018 of AMULSE sensitivities with respect to years and measurable molecular species. It highlights an increasing improvement in the performance of the instrument. It shows the standard deviation over a 1 s integration period.
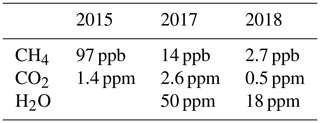
In order to better understand the values in this table, we will describe the last comparison measurement experiment that was achieved in February 2018. It took place at the LSCE on their ICOS site where a Picarro instrument (model G1301; Picarro, Inc.; Santa Clara, CA, USA) is installed. All Picarro concentrations are calibrated (every 6 h) with a WMO (World Meteorological Organization) standard gas. The analyser pulled air continuously at a few centimetres from the AMULSE for just over 3 continuous days. Figure 1 shows the intercomparison between the two instruments with the Picarro data plotted in green and the AMULSE data in blue. Insets (a), (b) and (c) in Fig. 1 correspond to a zoom-in focus on 10 min. The results show a good correlation between the two instruments for the three measured species. The average absolute difference between the two instruments is equal to 0.01 ppm with a standard deviation of 0.5 ppm (0.2 %) for the CO2 channel, 0.1 ppb with a standard deviation of 2.7 ppb (0.2 %) for the CH4 channel and 2 ppm with a standard deviation of 18 ppm (0.05 %) for the H2O channel.
Once the validation of the instrument was realized with the help of the Picarro instrument, several atmospheric measurement experiments were carried out.
4.1 Tethered balloon application (2017)
Tethered balloons offer great opportunities to characterize the temporal atmospheric evolution of the three measured species up to an 800 m altitude. In such applications we can control the motion speed of the balloon, have a bigger payload (which depends on the type of the balloon used) and we can even acquire data from a fixed stationary point. A tethered balloon is a carrier that allows measurements to be made at heights between fixed-tower and under-aircraft measurements. The costs of a tethered balloon are much lower than the installations mentioned above.
An experiment was conducted to monitor the evolution of the atmospheric boundary layer early in the morning at sunrise. We carried out 21 ascents and descents (up to 50 m above sea level). The time of an ascent is about 6 min, which results in a vertical resolution of between 20 and 50 cm.
These results were first interpolated over all the heights so that we visualized the spatio-temporal evolution of the boundary layer that morning (Fig. 2). The evolution of concentrations and temperature is consistent with what has previously been observed by Reid and Steyn (1997). AMULSE simultaneously records the concentration of CO2 (a), CH4 (b), H2O (c) and temperature (d).
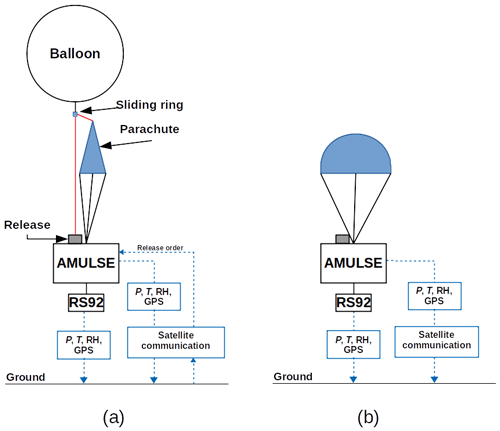
Figure 3A schematic diagram of the flight chain during (a) ascent and (b) descent. Transmission and reception of the information during the flight is performed using both satellite and radio communications. A Vaisala RS92 in situ sounding system is connected, which measures and transmits instantaneous P, T, relative humidity and GPS data to a ground mobile station to inject those data in the trajectory model and hence ensure the tracking and recovery of the instrument. Note that the trigger release system can be controlled by either a homemade smartphone application or a web application using an iridium satellite communication system. These applications can also track the AMULSE position as the AMULSE instrument transmits its GPS position, pressure and temperature data every 5 min ensuring a redundant retrieval system.
This illustrates the interest of this instrument on board a tethered balloon, as it allows the study of CO2, CH4 and H2O in the boundary layer.
4.2 APOGEE experiment (2017–2018)
4.2.1 Objectives
One of the goals of AMULSE is to carry out weather balloon measurements in co-location with the IASI satellite in orbit around the Earth, developed jointly by CNES (Centre national d'études spatiales) and EUMETSAT (European Organisation for the Exploitation of Meteorological Satellites). The measurements made by the AMULSE instrument will provide comparative data that can be used to validate CNRM–Météo France meteorological models. In addition, the combination of all these measures offers an opportunity to analyse physicochemical processes that have not yet been studied in the stratosphere and the interface between the troposphere and the stratosphere, commonly known as the UTLS (upper troposphere–lower stratosphere). To achieve these objectives, we carried out measurements from the site of our laboratory using meteorological balloons for the measurements of CO2, CH4, H2O, O3, P, T and relative humidity in co-location with Metop-A, Metop-B and Suomi NPP.
To measure all these parameters, the instruments used were as follows:
-
Vaisala RS92-SGP in situ soundings (pressure, temperature, relative humidity and GPS location measurement every second with a real-time transmission);
-
electrochemical concentration cell (ECC) ozonesondes from Science Pump Corporation, models 5A and 6A, with Vaisala RS-92 interfacing (ozone concentration measurement every second with a real-time transmission);
-
the AMULSE instrument presented above.
4.2.2 Description of the flight chain
The flight chain is composed of a carrier balloon, a parachute and the payload (AMULSE and in situ sounding; Fig. 3a). The balloon is inflated using a tare to ensure good repeatability of ascent speed. A wire is connected to both the parachute and the AMULSE release system; the carrier balloon is hooked up to the wire using a sliding ring. The balloon is released when the wire is cut, and it can be triggered either at a specific atmospheric pressure (fixed before the launch) or via a satellite communication system based on an iridium modem embedded onto the AMULSE electronic board or at a specific timeout fixed in advance. When the carrier balloon is released, the wire slides out of the ring and the instrumentation descends with the parachute at a speed lower than 5 m s−1 (Fig. 3b). A meteorological in situ sounding with a GPS probe fixed on the instrumentation and connected to a ground station allows the determination of the landing site which helps recovery of the instrumentation. Flight simulation software is used to estimate the trajectories and the landing point of the probe. This estimation is based on the wind forecasts from both Météo-France operational numerical weather prediction models: the mesoscale model AROME (Applications de la Recherche à l’Opérationnel à Méso-Echelle; Seity et al., 2011; Brousseau et al., 2016) and the global model ARPEGE (Action de Recherche Petite Echelle Grande Echelle; Courtier and Geleyn, 1988; Déqué et al., 1994). Hypotheses are made about both the ascending and the descending speed of the system and the release of the carrier balloon in order to run a first simulation. A measured vertical profile of horizontal winds by the recent sounding can also be used. The trajectories of the balloon are updated in real time by the rawinsonde RS92-measured wind. As the trajectories are adjusted in real time and the AMULSE release system can be remotely triggered, the landing area can be optimized in order to maximize the safety of landings.
4.2.3 Performed flights
The measurement experiment was held in France in the Champagne-Ardenne region. The launching site was located at the Campus Moulin de la Housse, in Reims (49∘14′29.608′′ N, 4∘4′4.709′′ E). The flights took place over the period from November 2017 to April 2018 (Table 2) and were carried out either by day or at night. Several launches could be carried out at the same time (Fig. 4).
Figure 5 shows examples of vertical concentration profiles of CO2, CH4 and H2O obtained from the flights, and we added temperature for the identification of the tropopause. The concentration of CO2 on the ground can vary greatly, depending on the time of measurement, because the boundary layer is enriched with CO2 when flying close to nighttime (plant respiration; see Schmidt et al., 2014). Concerning CH4, we see that there is a slight difference but the decrease always occurs at the tropopause level. Its decrease is due to its oxidation with the OH radical. For water measurements, we are currently using the iMet-1 in situ sounding. The measurements are consistent below a 10 km altitude, but above this altitude we consider that the measurements are biased. For this reason, one of the goals for the year 2020 is to make water vapour measurements by laser diode spectrometry more accurate in the stratosphere. It should also be taken into account that we generally see an increase in water vapour concentration above 10 km; this is due to the degassing of water from the instrument and the balloon during the ascent. For this reason, water measurements in the stratosphere are only possible during descents.
The data produced during the APOGEE measurement experiment have different applications. Initially, they were used as satellite validation data for the IASI and CrIS infrared sounders. They were then used to evaluate the quality of vertical ozone and methane profiles extracted from the MOCAGE and CAMS chemistry transport models. Finally, they were used as verification data to compare the ozone profiles returned in one-dimensional variational assimilation experiments (1D-Var) with the a priori ozone profiles from MOCAGE and CAMS.
5.1 Sensitivity of infrared satellite sensors to CO2, CH4 and O3 information
5.1.1 IASI and CrIS sensors
IASI is flying on board three European polar-orbiting satellites, Metop-A, Metop-B and Metop-C launched in 2006, 2012 and 2018, respectively. The IASI spectrum covers the range between 645 and 2760 cm−1, with a spectral sampling of 0.25 cm−1 and spectral resolution of 0.5 cm−1 after apodization. CrIS is part of the payload of the US polar-orbiting satellites Suomi NPP and NOAA-20 launched in 2011 and 2017, respectively. Only the so-called CrIS normal spectral resolution data are used in this study, leaving the CrIS spectrum divided into three parts: between 650 and 1095 cm−1 with a spectral resolution of 0.625 cm−1, between 1210 and 1750−1 (1.25 cm−1 spectral resolution), and between 2155 and 2550 cm−1 (2.5 cm−1 spectral resolution).
5.1.2 Radiative transfer model
During the assimilation process within the NWP analysis step, a compromise is found between a priori knowledge of the atmospheric state (a short-range forecast, in general) and various observations, including radiosoundings, aircraft measurements, ground stations, and space-borne passive sounders both in the microwave and in the infrared. When assimilating satellite radiances, e.g. infrared radiances, a radiative transfer model is needed in several ways. Firstly, the observations have to be compared to the prior atmospheric state: simulations of radiances are made using the temperature and humidity profiles and surface parameters from that state. The differences between observations and simulations are called innovations or first-guess departures. Then, the information contained in the first-guess departures is used to modify the atmospheric state so that the analysed atmospheric state is a compromise between all sources of information. To achieve this inverse problem, the RTM has to either have tangent linear and adjoint codes or compute the Jacobians (the Jacobians of IASI channels are described in Sect. 5.1.3). At Météo-France, the RTM used in the operational assimilation software is the fast radiative transfer model RTTOV (Saunders et al., 2018) developed and maintained by the NWP SAF (Satellite Application Facility) of EUMETSAT (European Organisation for the Exploitation of Meteorological Satellites).
For infrared sensor simulation, RTTOV requires not only temperature and humidity profiles but also a knowledge of the atmospheric composition. As few NWP models have in-line atmospheric composition yet or are coupled to a CTM, RTTOV also provides the users with average chemical profiles that are invariant in time and space, hereafter referenced to as REF profiles. These REF profiles are plotted in Fig. 7 for O3 (a), CO2 (b) and CH4 (c). In this study, we use RTTOV version 12, with coefficients for IASI and CrIS on 101 fixed-pressure levels.
5.1.3 Theoretical sensitivity of IASI and CrIS spectra to GHG
Infrared satellite observations measured by IASI and CrIS instruments are sensitive to not only atmospheric temperature and humidity and skin temperature but also atmospheric chemistry. To identify the channels sensitive to the different parameters, RTTOV offers the possibility of calculating the Jacobians which represent the sensitivity of the brightness temperature to the variation in a thermodynamic or chemical parameter. Thus we have represented in Fig. 6 the Jacobians in temperature (a), humidity (b), skin temperature (c), ozone (d), carbon dioxide (e) and methane (f) for the 8461 IASI channels using the reference chemical profiles for our case study according to the 101 RTM levels. It should be noted that temperature Jacobians are sensitive over a large part of the spectrum but at different altitudes. Indeed, channels from 650 to 770 cm−1 describe a temperature sensitivity from the top of the stratosphere to the surface. There is also high sensitivity near the surface to window channels from 790 to 980 and 1080 to 1150 cm−1. There is a sensitivity over the entire atmosphere for channels from 1000 to 1070 cm−1. Then, temperature Jacobians are sensitive in the troposphere from 1210 to 1650 cm−1. Humidity Jacobians are surface sensitive for channels from 650 to 770 and 1080 to 1150 cm−1 and in the troposphere from 650 to 1150 cm−1. Jacobians of skin temperature have essentially high values for window channels from 790 to 980 and 1080 to 1150 cm−1. Ozone Jacobians are slightly sensitive from 650 to 770 cm−1 and have a sensitivity over the entire atmosphere from 1000 to 1070 cm−1. We note that carbon dioxide Jacobians have high values over the entire atmospheric column for channels from 650 to 770 cm−1. Finally, methane Jacobians have high sensitivities for channels from 1210 to 1650 cm−1.
5.1.4 Preprocessing of APOGEE measurements
We selected the sounding from 17 April 2018 at 10:00 UTC for which ozone, carbon dioxide and methane are measured. The profiles are plotted in Fig. 7. The ozone profile is available up to 6 hPa, the carbon dioxide up to 25 hPa and the methane up to 65 hPa. There are few differences between the reference and in situ profiles for ozone and methane, except around the tropopause., unlike for carbon dioxide, where the difference can be as much as 10 ppmv in the lower troposphere.
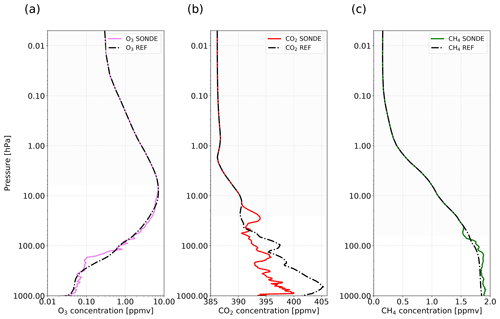
Figure 7Representation of reference profiles (dash-dotted black lines) of O3 (a), CO2 (b) and CH4 (c) and profiles measured by in situ sounding and convoluted with reference profiles of O3 in violet (a), CO2 in red (b) and CH4 in green (c).
In order to assess the impact of using the in situ chemical information instead of the reference profiles, we have interpolated the in situ profiles on the 101 fixed-pressure levels by making layer averages cantered on these levels. Moreover, the measured profiles do not reach the highest fixed-pressure level. Thus a polynomial function was used to link the in situ profiles to the reference profiles from the levels where the data are missing.
Then, modelled atmospheric profiles of temperature, humidity, surface temperature, surface humidity, surface pressure, and zonal and meridian wind come from the global model ARPEGE forecasts (every 3 h), which have been extracted for the same period and location as for the in situ sounding. The coefficient file used during the simulation process in RTTOV being on 101 fixed-pressure levels, the thermodynamic profiles of ARPEGE were interpolated on these same levels.
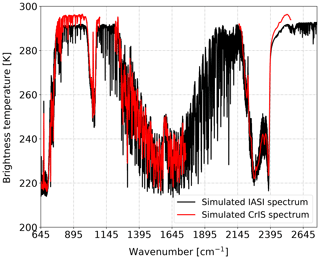
Figure 8Brightness temperature spectrum of simulated IASI (in black) and CrIS (in blue) observations w.r.t. wavenumbers using O3, CO2 and CH4 reference profiles for in situ sounding from 17 April 2018 at 10:00 UTC.
In this study we simulated satellite observations from two types of infrared instruments: IASI and CrIS. In order to evaluate the quality of our simulations, we sought to spatially and temporally co-locate the IASI (Metop-B) and CrIS (Suomi NPP) pixels as close as possible to the in situ soundings. To avoid problems related to clouds, we carried out the 17 April 2018 release at 10:00 UTC in clear-sky conditions. Thus, Fig. 8 represents the brightness temperature spectrum of the IASI observations simulations in black and CrIS in red with respect to the wavenumber using as RTTOV input the reference profiles of O3, CO2 and CH4 for the in situ sounding from 17 April 2018 at 10:00 UTC. There is a good agreement between the simulated IASI and CrIS spectrum except for the window channels. The difference seen between the simulated IASI and CrIS window channels is due to the temporal and spatial differences between the selected pixels, which implies a different skin temperature used in RTTOV. However, the skin temperature has an obvious impact on the simulation of infrared observations sensitive to the surface, hence these differences.
5.1.5 Impacts on simulations
We evaluated the impact on the simulations of IASI and CrIS observations of using chemical information from in situ measurements as input to RTTOV in replacement of the constant profiles. Figure 9 shows the difference between simulated brightness temperature (BTS) with O3 in purple (CO2 in red and CH4 in green) in situ measurement profiles (XM) and simulated brightness temperature with O3 (CO2 and CH4) reference profiles (XR) for in situ sounding from 17 April 2018 at 10:00 UTC for all channels contained in the IASI (a) and CrIS (b) spectrum, where EXP means experiment (for example, EXPO3 means experiment for ozone case, etc.), such that
The differences in BT affect ozone-sensitive channels located from 710 to 760, 980 to 1150 and 2060 to 2135 cm−1 (for IASI). Then, the differences in BT affect a larger part of the spectrum especially the CO2-sensitive channels with spectral intervals from 645 to 820, 930 to 980, 1030 to 1085, 1900 to 1940 (for IASI), 2010 to 2120 (for IASI) and 2200 to 2440 cm−1. Finally, the differences in BT affect CH4-sensitive channels located from 1200 to 1380 cm−1 and less so from 2650 to 2760 cm−1.
The maximum difference values of BT for CO2-sensitive channels are around 0.2 K and for CH4-sensitive channels around −0.4 K for both instruments. The maximum difference values of BT for ozone-sensitive channels are around 0.9 K for IASI and 0.75 K for CrIS. The differences in ΔBT values between the two instruments can be explained by the difference in spectral resolution.
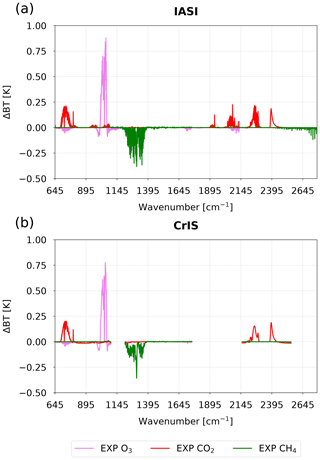
Figure 9Difference between simulated brightness temperature (BT) with O3 in purple (CO2 in red and CH4 in green) in situ profiles from SONDE and AMULSE and simulated brightness temperature with O3 (CO2 and CH4) reference profiles for in situ sounding from 17 April 2018 at 10:00 UTC for all channels contained in the IASI (a) and CrIS (b) spectrum.
Even if the reference profile is close to the in situ profile, as for example for ozone, this does not necessarily imply small differences between the simulations.
However, the spatial and temporal variability in atmospheric composition is very important, especially for ozone. In addition, differences in BT are too large in CO2 to have a direct impact on the quality of temperature forecasts, since we use channels sensitive to this species to retrieve temperature profiles.
Finally, in order to assess the quality of our simulations of infrared satellite observations for IASI and CrIS instruments using the reference profiles provided by RTTOV, we calculated the difference between real and simulated observations (O–B) using chemical information from reference profiles (black line) and from in situ profiles (red line) with respect to IASI-monitored (Fig. 10a) and CrIS-monitored (Fig. 10b) channels for in situ sounding from 17 April 2018 at 10:00 UTC. IASI and CrIS have 314 and 330 monitored channels, respectively.
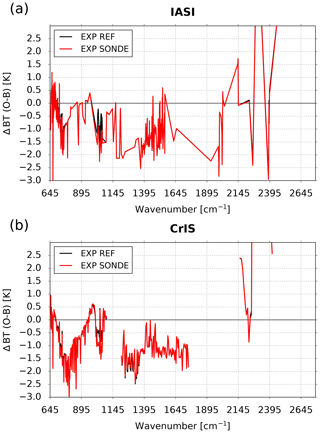
Figure 10Difference between real and simulated observations (O–B) using chemical information from reference profiles (black line) and from in situ profiles (red line) with respect to IASI-monitored (a) and CrIS-monitored (b) channels for in situ sounding from 17 April 2018 at 10:00 UTC.
For this case study the differences (O–B) using reference chemical (REF) profiles and simulations using in situ chemical (SONDE) profiles are small over all IASI (a) and CrIS (b) spectra. However, there are significant differences for ozone-sensitive channels with O–B values closer to zero when using the reference ozone. This could be explained by a smaller accuracy of ozone measurement with ozonesondes than with the AMULSE instrument. Another possibility is the existence of other biases (e.g. depending on the scan position) which are compensated for by bias in the opposite sign in the case of REF profiles. The same experiments were performed on other in situ sounding data with generally the same results (not shown here). For the case of 7 April 2018, it can be seen in Fig. 9 that our static reference profiles of carbon dioxide and methane lead to realistic simulations, in line with in situ profiles. However, it is more difficult to accurately simulate ozone-sensitive channels. Indeed, ozone differs from CO2 and CH4 because it has a very high spatial and temporal variability. Hopefully in the future, chemical measurements of this type will be carried out on a more regular basis across the globe allowing for more robust assessments.
5.2 Comparison between a priori, retrieval and in situ chemical profiles
In situ chemical profiles can directly be used as verification data for modelled profiles or retrievals from satellite sensors. In this section, two possible usages are illustrated with APOGEE data.
5.2.1 Forecasts of atmospheric composition
One possible use of these in situ profiles of CO2, CH4 and O3 is as comparison data to the a priori chemical profiles from chemistry transport models (CTMs). As part of our study, we compared our measured data against the profiles extracted from the CTMs MOCAGE and C-IFS. These two models differ in that the global version of the MOCAGE model does not assimilate any observation in operation, while C-IFS assimilates Level 2 products from several instruments such as MLS, OMI, SBUV/2, GOME-2A and GOME-2B, IASI, MOPITT, OMPS, and PMAp (Basart et al., 2018). C-IFS provides analyses and 5 d forecasts of atmospheric composition at regional and global scales in near real time. MOCAGE is an off-line global three-dimensional chemistry transport model (Guth et al., 2016). It provides the time evolution of the chemical air composition from the surface to the stratosphere. MOCAGE is used for operational daily forecasts and also for research studies. Thus, we extracted the profiles a priori from CH4 and O3 from the CTM MOCAGE and C-IFS for our case study of the in situ sounding from 17 April 2018 at 10:00 UTC. These profiles could therefore be compared to the in situ and reference profiles available in RTTOV. We have shown in Fig. 11 the ozone (a) and methane (b) in situ profiles in black lines compared to O3 and CH4 a priori profiles from CAMS in red lines and MOCAGE in blue lines and compared to O3 and CH4 reference profiles in dotted lines.
In Fig. 11a we notice that the reference ozone profile is in relatively good agreement with the in situ profile except in the upper troposphere between 300 and 150 hPa. The ozone values of the reference profile are slightly higher than those of the in situ profile in the lower troposphere between 1000 and 350 hPa and in the lower stratosphere between 60 and 20 hPa. Then, we observe that the a priori profile of ozone from MOCAGE is very close to the profile measured over the entire lower stratosphere. The MOCAGE ozone profile follows the profile measured in the upper troposphere between 500 and 80 hPa, with lower values. The MOCAGE ozone values are overestimated compared to the profile measured in the lower troposphere between 1000 and 500 hPa. Finally, the ozone profile from CAMS is in very good agreement with the profile measured in the lower troposphere between 1000 and 450 hPa and in the lower stratosphere between 45 and 15 hPa. However, the CAMS ozone profile overestimates the values compared to the profile measured over a large part of the atmosphere between 500 and 50 hPa. Overall, the different ozone profiles are more or less close to the measured profile. The CTMs are able to simulate the shape of ozone profiles relatively well with good values for MOCAGE in the stratosphere and for CAMS in the lower troposphere. The reference profile seems to be a good compromise on this particular case.
Methane simulation is a much more difficult task in the field of CTMs. Indeed, there are major scientific questions about the increase in atmospheric methane concentration and its hypothetical sources (Archibald et al., 2020; Houweling et al., 2017; Naik et al., 2013). That is why assimilation into CTMs for this species is very useful. We notice again in Fig. 11b that the reference methane profile is rather good with the profile measured between 1000 and 70 hPa. Indeed, above 70 hPa, there is a faster decrease in the measured concentration than in the values of the reference profile. This decrease is simulated by the profile from MOCAGE but with a large underestimation over the entire profile of about 0.5 ppmv. This difference can be explained by a missing source in the model and/or too many OH molecules that are the main methane sink. Assimilation can solve this problem, so there is a very good agreement between the CAMS methane profile and the measured profile in the lower troposphere between 1000 and 300 hPa. Then the values of the CAMS profile are underestimated compared to the measured profile between 300 and 45 hPa.
Such in situ chemical profiles can be very useful in assessing the quality of simulations of atmospheric composition from CTMs, especially if they can be made on a regular basis within a network. For our case study, we note the general pattern and concentration of ozone are relatively well simulated by MOCAGE and CAMS, while methane is largely underestimated in MOCAGE and very well represented in the troposphere by CAMS.
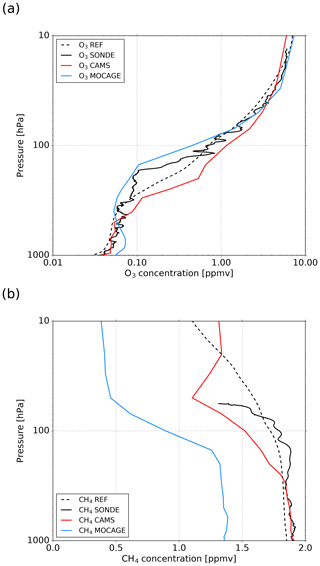
Figure 11Ozone (a) and methane (b) in situ profiles in black lines compared to O3 and CH4 a priori profiles from CAMS in red lines and MOCAGE in blue lines and compared to O3 and CH4 reference profiles in dotted lines for in situ sounding from 17 April 2018 at 10:00 UTC.
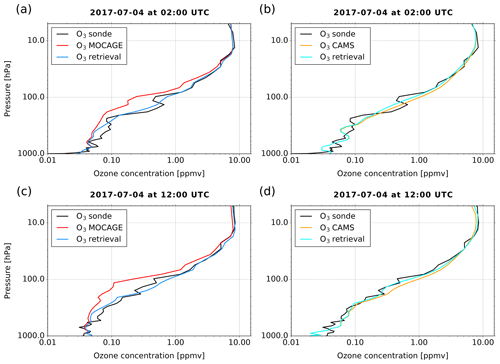
Figure 12Ozone in situ profile in black compared to O3 a priori profile from MOCAGE in red and O3 retrieval profile from CrIS in blue for in situ sounding from 4 July 2017 at 02:00 UTC (a) and 4 July 2017 at 12:00 UTC (c). Ozone in situ profile in black compared to O3 a priori profile from CAMS in orange and O3 retrieval profile from CrIS in cyan for in situ sounding from 4 July 2017 at 02:00 UTC (b) and 4 July 2017 at 12:00 UTC (d).
5.2.2 Ozone retrieval from 1D assimilation
Profiles of various atmospheric compounds can be retrieved from satellite data. Among them, hyperspectral infrared sounders like AIRS, CrIS and IASI have the ability to be used both in NWP models and for atmospheric composition purposes, which could be used in combining meteorological and chemistry transport models to achieve a so-called coupled assimilation. A precursor method is to add some chemical variable to the control variable of NWP models. This is the case, for example, for ozone in the IFS model of ECMWF, which assimilates 16 IASI ozone-sensitive channels in operational near real time, providing ozone analysis (Han and McNally, 2010). Such an approach can also be used at the pixel level to retrieve profiles or with cloud-cleared spectra as performed by the AIRS and NUCAPS systems (Susskind et al., 2003; Smith and Barnet, 2019). Indeed, Coopmann et al. (2018) show that the assimilation of 15 ozone-sensitive IASI channels makes it possible to simultaneously improve temperature, humidity and ozone retrievals. In both cases, it is also important to assess the quality of ozone analyses using independent comparative data. For ozone, this can be carried out in almost any region around the world since there are several stations that perform in situ soundings measuring ozone (Nalli et al., 2017b). However, if methane or carbon dioxide analyses are to be retrieved, in situ vertical profiles of these compounds are much sparser. This is why the data measured by the AMULSE instrument can be valuable when these compounds are reproduced by meteorological models. As part of the APOGEE measurement experiment, we carried out several assimilation experiments in a simplified one-dimensional framework (1D-Var) where we retrieved ozone. The NWP SAF (Satellite Application Facility) 1D-Var algorithm (Smith, 2016) is based on the optimal estimation method (OEM; Rodgers, 2009). This method makes it possible in a simplified way to get closer to what is carried out operationally in large NWP centres using variational assimilation methods. There are other methods of retrieval such as the piecewise linear regression used at EUMETSAT (EUMETSAT, 2014) or the NOAA Unique CrIS/ATMS Processing System (NUCAPS) for the infrared physical retrieval based on a regularized iterated least-squares minimization (Gambacorta et al., 2012; Nalli et al., 2013).
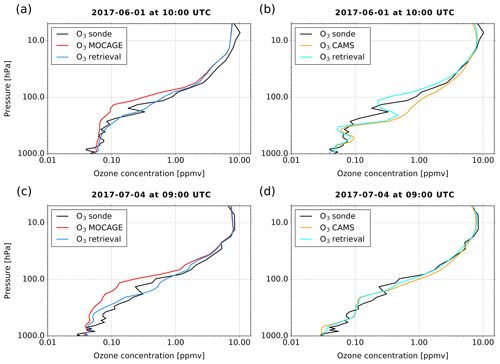
Figure 13As in Fig. 12, except for IASI for in situ sounding from 1 June at 10:00 UTC (b) and 4 July 2017 at 09:00 UTC (d).
The measured in situ ozone profiles could be used as comparative data to evaluate the quality of our retrievals. In this study we conducted these experiments using observations from IASI and CrIS, focusing on the simultaneous retrieval of temperature, humidity and ozone, taking the ozone background and a priori profile from MOCAGE or CAMS. Similar work on the validation of ozone restitution from IASI is presented in Dufour et al. (2012) and from CrIS in Nalli et al. (2017b). Soundings of 1 June at 10:00 UTC, 4 July at 02:00 UTC, 4 July at 09:00 UTC and 4 July 2017 at 12:00 UTC are selected for that case study. The methodology and techniques used in this study are the same as those used in Coopmann et al. (2018). To retrieve ozone using observations from IASI, we assimilated 123 channels used in operations at Météo-France plus 15 ozone-sensitive channels, using a full observation error covariance matrix diagnosed from the method described by Desroziers et al. (2005). Based on CrIS observations, we assimilated 68 channels used in operations at Météo-France and 14 ozone-sensitive channels, using a diagonal observation error covariance matrix whose values are derived from the operational settings at Météo-France. IASI and CrIS observations were collocated with in situ soundings. The ozone background errors available in the background error covariance matrix were calculated using ozonesondes based on the method described in the work of Coopmann et al. (2018). Finally, the thermodynamic a priori profiles and surface parameters were extracted from the global model ARPEGE and were extracted at the same coordinates and time as the in situ soundings, as were the ozone a priori profiles from MOCAGE and CAMS.
Figure 12 represents the ozone in situ profile in black compared to the O3 a priori profile from MOCAGE in red and O3 retrieval profile from CrIS in blue for in situ sounding from 4 July at 02:00 UTC (a) and 4 July 2017 at 12:00 UTC (c) and the ozone in situ profile in black compared to the O3 a priori profile from CAMS in orange and O3 retrieval profile from CrIS in cyan for in situ sounding from 4 July at 02:00 UTC (b) and 4 July 2017 at 12:00 UTC (d). In Fig. 12a, we notice that overall the MOCAGE a priori profile underestimates the ozone concentration compared to the measured profile except in the lower troposphere and above 25 hPa. In this case, the ozone retrieval profile is very close to the measured profile. In Fig. 12b, the CAMS a priori profile is generally closer to the measured profile than the MOCAGE a priori profile. However, there is some overestimation of CAMS ozone concentration between 300–200 and 150–50 hPa. We note that with this a priori profile, the ozone retrieval profile is less modified than previously. In the same way as with the previous in situ sounding, we notice in Fig. 12c that the MOCAGE a priori profile underestimates the ozone concentration compared to the measured profile except in the lower troposphere. The ozone retrieval profile is again of very good quality. Finally, we note in Fig. 12d that the CAMS a priori profile underestimates the concentration of ozone in the lower troposphere compared to the measured profile and overestimates it between 500 and 20 hPa. In this experiment, the ozone retrieval profile is very close to the measured profile except in the lower troposphere.
Figure 13 represents the ozone in situ profile in black compared to the O3 a priori profile from MOCAGE in red and O3 retrieval profile from IASI in blue for in situ sounding from 1 June at 10:00 UTC (a) and 4 July 2017 at 09:00 UTC (c) and the ozone in situ profile in black compared to the O3 a priori profile from CAMS in orange and O3 retrieval profile from IASI in cyan for in situ sounding from 1 June at 10:00 UTC (b) and 4 July 2017 at 09:00 UTC (d). Figure 13a shows that the a priori profile from MOCAGE underestimates the ozone concentration compared to the measured profile except in the lower troposphere. This experiment makes it possible to retrieve a profile very close to the measured profile except above 50 hPa where the latter remains close to the a priori profile. In Fig. 13b, the a priori profile from CAMS is generally close to the measured profile except at the UTLS where there is an overestimation of the ozone concentration. It can be seen that, with this a priori profile, the retrieval profile approaches the measured profile and shows the same structure as the measured profile in the UTLS. Then Fig. 13c shows that the a priori profile from MOCAGE underestimates the ozone concentration compared to the profile measured in this case except in the lower troposphere and above 25 hPa. The retrieval profile is also of good quality. Finally, we note in Fig. 13d that the a priori profile from CAMS underestimates ozone concentration in the lower troposphere compared to the measured profile and overestimates it at 150–40 hPa. This last experiment allows us to retrieve an ozone profile very close to the measured profile except between 300 and 150 hPa.
This article shows the possibilities of the AMULSE instrument for the fast and accurate measurement of CO2, CH4 and H2O in the atmosphere. The APOGEE measurement experiment was an opportunity to highlight the various benefits of the data from the AMULSE instrument for different research projects: satellite validation, model comparison, verification data, etc. The measurements will continue with monthly radiosoundings on the Reims site to get more consistent data sets.
The prime aim of this study was to assess the sensitivity of infrared satellite observations to chemical information. The objective was to use carbon dioxide, methane and ozone profiles measured as part of the APOGEE measurement experiment to validate the quality of IASI and CrIS observation simulations. The in situ profiles were measured for ozone using an electrochemical cell and the CO2 and CH4 profiles using the AMULSE instrument. These instruments were placed under meteorological balloons to produce vertical profiles of up to 30 km in altitude. Experiments for one case study showed us that infrared observations are extremely sensitive to changes in chemical concentrations. The RTM RTTOV model allowed us to perform several simulations of infrared observations under several configurations of chemical a priori profiles provided as input. This is how we were able to highlight differences in simulation between the use of the in situ and reference profiles. However, despite these differences, we have shown little impact on differences between the real and simulated observations using either the reference chemical profiles or the in situ profiles except for ozone. Indeed, the latter reacts differently in the atmosphere and is highly variable compared to CO2 and CH4. The data measured by the AMULSE instrument are valuable for evaluating the quality of our simulations, which is essential for NWP models. Indeed, as mentioned above, the a priori information of gases provided to RTTOV is invariant in time and space. In reality these gases such as CO2, CH4, O3 or CO show significant variability, both temporarily and spatially in the atmosphere. In addition, carbon-dioxide-sensitive channels are often used to extract information on atmospheric temperature. However, the approximation of using a fixed CO2 concentration for the simulation of infrared satellite observations can have a negative impact on the quality of temperature retrieval as shown by the work of Engelen et al. (2001) from the AIRS instrument. Finally, data assimilation systems use a bias correction method called VarBC (Auligné et al., 2007). However, this method can correct systematic biases that do not necessarily take into account the specificity of the variability in certain gases. Thus, it is preferable to correct biases at the source through an improvement of simulations in radiative transfer models, which will improve the analysis of temperature, humidity and wind in global model assimilations.
Secondly, the measured profiles in the APOGEE framework were also valuable for comparison with the chemical profiles derived from the CTMs. Thus, we extracted ozone and methane profiles from the MOCAGE and CAMS CTMs for a case study. This comparison with the measured profiles showed us that the CTMs represent atmospheric ozone rather well, especially in the stratosphere for MOCAGE and in the troposphere for CAMS. Having good chemical concentrations in the troposphere for CAMS is important as it also provides air quality forecasts. We also showed that methane is very well simulated by the CAMS CTM in the troposphere but underestimated by 0.5 ppmv by the MOCAGE CTM. The difference is mainly due to the fact that the CAMS C-IFS model assimilates Level 2 products compared to MOCAGE which does not assimilate anything. Once again, we can see the importance of these in situ profiles for the comparison and validation of chemical predictions from CTMs.
Finally, a method to obtain more accurate chemical profiles was used. In this case, ozone profiles were retrieved using one-dimensional assimilation (1D-Var) using observations from IASI and CrIS and a priori ozone profiles from MOCAGE and CAMS for four in situ soundings. The in situ profiles have allowed us to highlight very encouraging results since the method used in this study allows us to retrieve ozone profiles very close to the measured ozone profiles, particularly in the UTLS which remains an area difficult to model by CTMs. We have also shown that some retrieved ozone profiles simulate very well the complex structures that some measured ozone profiles generated by isentropic transport in the UTLS can have.
AMULSE regularly evolves in terms of performance, weight and number of detectable gas molecules. Different perspectives are possible, such as the measurement of other molecular species of atmospheric interest in tethered balloons or weather balloons for measurements in the stratosphere. Thus, the data measured by the AMULSE instrument are useful for many scientific studies. However, the small number of measurements performed remains an important limitation in making conclusions from comparisons with satellite observations or chemical fields from models. Therefore, it would be interesting to extend these chemical measurements on a regular basis over the whole globe in the same way as is done with the ozone measurement networks.
The radiative transfer model RTTOV (Saunders et al., 2018) and the unidimensional data assimilation system 1D-Var (Smith, 2016) are developed within the framework of the EUMETSAT Satellite Application Facility on Numerical Weather Prediction (NWP SAF). The partners in NWP SAF are the Met Office, ECMWF, DWD and Météo-France. The software is available from the NWP SAF website https://www.nwpsaf.eu/site/software (NWPSAF, 2020).
IASI data are available from EUMETSAT https://www.eumetsat.int (EUMETSAT, 2020); CrIS data are available from NOAA https://doi.org/10.7289/V59C6VGK (Han et al., 2012); and CAMS data are freely available from https://atmosphere.copernicus.eu/ (CAMS, 2020). All other data used for this study in Section 5 can be obtained by emailing olivier.coopmann@umr-cnrm.fr.
All authors participated in the field campaign. LJ, OC and VG are the principal authors of the article. LJ is the principal investigator (PI) of the AMULSE instrument and conceptualized the project with OC and VG. DT, JB, EM, PA, AB and OC managed the flights (trajectory forecast, flight preparation, regulation, ozonesonde preparation and data acquisition). OC and VG prepared the satellite colocations, sensitivity studies and retrievals. TD realized all the electronics for each version and evolution of AMULSE and participated in the measurement campaigns. ND carried out all the spectroscopic processing and data analysis during the comparison with the Picarro instrument and participated in the measurement campaigns. JC carried out the acquisition and monitoring programme as well as the optical cell work. JB with ND carried out a programme of trajectory follow-up and separation of the balloons with the data transmitted by the iridium. NC and GA designed and produced all the mechanical parts (optical cell, thermal insulation, supports, etc.) and took part in the measurement campaigns. RM and ZMEK worked on the calibration of the instrument.
The authors declare that they have no conflict of interest.
This research based on data from the APOGEE measurement experiment has been conducted within the framework of Olivier Coopmann's PhD thesis, which funded by CNES (Centre national d'études spatiales) and the Région Occitanie. We thank Michel Ramonet (from LSCE) and his team for allowing us to perform the measurements on their ICOS site.
This paper was edited by Troy Thornberry and reviewed by two anonymous referees.
Archer, D. and Brovkin, V.: The millennial atmospheric lifetime of anthropogenic CO2, Climatic Change, 90, 283–297, https://doi.org/10.1007/s10584-008-9413-1, 2008. a
Archibald, A. T., O'Connor, F. M., Abraham, N. L., Archer-Nicholls, S., Chipperfield, M. P., Dalvi, M., Folberth, G. A., Dennison, F., Dhomse, S. S., Griffiths, P. T., Hardacre, C., Hewitt, A. J., Hill, R. S., Johnson, C. E., Keeble, J., Köhler, M. O., Morgenstern, O., Mulcahy, J. P., Ordóñez, C., Pope, R. J., Rumbold, S. T., Russo, M. R., Savage, N. H., Sellar, A., Stringer, M., Turnock, S. T., Wild, O., and Zeng, G.: Description and evaluation of the UKCA stratosphere–troposphere chemistry scheme (StratTrop vn 1.0) implemented in UKESM1, Geosci. Model Dev., 13, 1223–1266, https://doi.org/10.5194/gmd-13-1223-2020, 2020. a
Auligné, T., McNally, A., and Dee, D.: Adaptive bias correction for satellite data in a numerical weather prediction system, Q. J. Roy. Meteor. Soc., 133, 631–642, 2007. a
Basart, S., Benedictow, A., Bennouna, Y., Blechschmidt, A-M., Chabrillat, S., Christophe, Y., Clark, H., Cuevas, E., Eskes, H., Hansen, K. M., Im, U., Kapsomenakis, J., Langerock, B., Petersen, K., Schulz, M., Wagner, A., and Zerefos, C.: Addendum to: Upgrade verification note for the CAMS near-real time global atmospheric composition service Evaluation of the e-suite experiment gu42, Ref: CAMS84_2015SC3_D84.3.1.5_201802_esuite_v1, 2018. a
Blunden, J. and Arndt, D. S.: State of the Climate in 2018, B. Am. Meteorol. Soc., 100, Si–S306, https://doi.org/10.1175/2019bamsstateoftheclimate.1 2019.
Brousseau, P., Seity, Y., Ricard, D., and Léger, J.: Improvement of the forecast of convective activity from the AROME-France system, Q. J. Roy. Meteor. Soc., 142, 2231–2243, https://doi.org/10.1002/qj.2822, 2016. a
Cammas, J.-P., Athier, G., Boulanger, D., Chéroux, F., Cousin, J.-M., Girod, F., Karcher, F., Law, K., Nédélec, P., Peuch, V.-H., Smit, H., Stoll, M., Texier, D., Thouret, V., Volz-Thomas, A., and Zbinden, R.: Les programmes aéroportés Mozaic et Iagos (1994–2008), La Météorologie, 8, 62, https://doi.org/10.4267/2042/19172, 2008. a
CAMS: Copernicus Atmosphere Monitoring Service Database, available at: https://atmosphere.copernicus.eu/, last access: 26 May 2020.
Chahine, M., Barnet, C., Olsen, E., Chen, L., and Maddy, E.: On the determination of atmospheric minor gases by the method of vanishing partial derivatives with application to CO2, Geophys. Res. Lett., 32, https://doi.org/10.1029/2005GL024165, 2005. a
Coopmann, O., Guidard, V., Fourrié, N., and Plu, M.: Assimilation of IASI ozone-sensitive channels in preparation for an enhanced coupling between Numerical Weather Prediction and Chemistry Transport Models, J. Geophys. Res.-Atmos., 123, 12452–12473, https://doi.org/10.1029/2017JD027901, 2018. a, b, c
Courtier, P. and Geleyn, J.-F.: A global numerical weather prediction model with variable resolution: Application to the shallow-water equations, Q. J. Roy. Meteor. Soc., 114, 1321–1346, https://doi.org/10.1002/qj.49711448309, 1988. a
Crevoisier, C., Nobileau, D., Armante, R., Crépeau, L., Machida, T., Sawa, Y., Matsueda, H., Schuck, T., Thonat, T., Pernin, J., Scott, N. A., and Chédin, A.: The 2007–2011 evolution of tropical methane in the mid-troposphere as seen from space by MetOp-A/IASI, Atmos. Chem. Phys., 13, 4279–4289, https://doi.org/10.5194/acp-13-4279-2013, 2013. a
Déqué, M., Dreveton, C., Braun, A., and Cariolle, D.: The ARPEGE/IFS atmosphere model: a contribution to the French community climate modelling, Clim. Dynam., 10, 249–266, https://doi.org/10.1007/BF00208992, 1994. a
Desroziers, G., Berre, L., Chapnik, B., and Poli, P.: Diagnosis of observation, background and analysis-error statistics in observation space, Q. J. Roy. Meteor. Soc., 131, 3385–3396, 2005. a
Dufour, G., Eremenko, M., Griesfeller, A., Barret, B., LeFlochmoën, E., Clerbaux, C., Hadji-Lazaro, J., Coheur, P.-F., and Hurtmans, D.: Validation of three different scientific ozone products retrieved from IASI spectra using ozonesondes, Atmos. Meas. Tech., 5, 611–630, https://doi.org/10.5194/amt-5-611-2012, 2012. a
Durry, G. and Megie, G.: Atmospheric CH4 and H2O monitoring with near-infrared InGaAs laser diodes by the SDLA, a balloonborne spectrometer for tropospheric and stratospheric in situ measurements, Appl. Optics, 38, 7342, https://doi.org/10.1364/AO.38.007342, 1999a. a
Durry, G. and Megie, G.: Atmospheric CH4 and H2O monitoring with near-infrared InGaAs laser diodes by the SDLA, a balloonborne spectrometer for tropospheric and stratospheric in situ measurements, Appl. Optics, 38, 7342–7354, 1999b. a
Eby, M., Zickfeld, K., Montenegro, A., Archer, D., Meissner, K. J., Weaver, A. J., Eby, M., Zickfeld, K., Montenegro, A., Archer, D., Meissner, K. J., and Weaver, A. J.: Lifetime of Anthropogenic Climate Change: Millennial Time Scales of Potential CO2 and Surface Temperature Perturbations, J. Climate, 22, 2501–2511, https://doi.org/10.1175/2008JCLI2554.1, 2009. a
El Khair, Z. M., Joly, L., Cousin, J., Decarpenterie, T., Dumelié, N., Maamary, R., Chauvin, N., and Durry, G.: In situ measurements of methane in the troposphere and the stratosphere by the Ultra Light SpEctrometer Amulse, Appl. Phys. B, 123, 281, https://doi.org/10.1007/s00340-017-6850-4, 2017. a, b
EUMETSAT: IASI L2 PPF v6 Validation Report, Tech. rep., EUM/TSS/REP/14/776443, v4C. 13 October, 2014. a
EUMETSAT: IASI Observations Database, available at: https://www.eumetsat.int, last access: 26 May 2020.
Engelen, R. J., Stephens, G. L., and Denning, A. S.: The effect of CO2 variability on the retrieval of atmospheric temperatures, Geophys. Res. Lett., 28, 3259–3262, 2001. a
Filges, A., Gerbig, C., Chen, H., Franke, H., Klaus, C., and Jordan, A.: The IAGOS-core greenhouse gas package: a measurement system for continuous airborne observations of CO2, CH4, H2O and CO, Tellus B, 67, 27989, https://doi.org/10.3402/tellusb.v67.27989, 2015. a
Gambacorta, A., Barnet, C., Wolf, W., Goldberg, M., King, T., Nalli, N., Maddy, E., Xiong, X., and Divakarla, M.: The NOAA unique CrIS/ATMS processing system (NUCAPS): First light retrieval results, in: Proceedings of the ITWG meeting, ITWG, Toulouse, France, March, 2012. a
Ghysels, M., Riviere, E. D., Khaykin, S., Stoeffler, C., Amarouche, N., Pommereau, J.-P., Held, G., and Durry, G.: Intercomparison of in situ water vapor balloon-borne measurements from Pico-SDLA H2O and FLASH-B in the tropical UTLS, Atmos. Meas. Tech., 9, 1207–1219, https://doi.org/10.5194/amt-9-1207-2016, 2016. a
Guth, J., Josse, B., Marécal, V., Joly, M., and Hamer, P.: First implementation of secondary inorganic aerosols in the MOCAGE version R2.15.0 chemistry transport model, Geosci. Model Dev., 9, 137–160, https://doi.org/10.5194/gmd-9-137-2016, 2016. a
Han, W. and McNally, A.: The 4D-Var assimilation of ozone-sensitive infrared radiances measured by IASI, Q. J. Roy. Meteor. Soc., 136, 2025–2037, 2010. a
Han, Y., Qiu, S., and NOAA JPSS Program Office: NOAA JPSS Cross-track Infrared Sounder (CrIS) Science Sensor Data Record (SDR) from IDPS, NOAA National Centers for Environmental Information, https://doi.org/10.7289/V59C6VGK, 2012.
Hilton, F., Armante, R., August, T., Barnet, C., Bouchard, A., Camy-Peyret, C., Capelle, V., Clarisse, L., Clerbaux, C., Coheur, P.-F., Collard, A., Crevoisier, C., Dufour, G., Edwards, D., Faijan, F., Fourrié, N., Gambacorta, A., Goldberg, M., Guidard, V., Hurtmans, D., Illingworth, S., Jacquinet-Husson, N., Kerzenmacher, T., Klaes, D., Lavanant, L., Masiello, G., Matricardi, M., McNally, A., Newman, S., Pavelin, E., Payan, S., Péquignot, E., Peyridieu, S., Phulpin, T., Remedios, J., Schlüssel, P., Serio, C., Strow, L., Stubenrauch, C., Taylor, J., Tobin, D., Wolf, W., and Zhou, D.: Hyperspectral Earth observation from IASI: Five years of accomplishments, B. Am. Meteorol. Soc., 93, 347–370, 2012. a
Houweling, S., Bergamaschi, P., Chevallier, F., Heimann, M., Kaminski, T., Krol, M., Michalak, A. M., and Patra, P.: Global inverse modeling of CH4 sources and sinks: an overview of methods, Atmos. Chem. Phys., 17, 235–256, https://doi.org/10.5194/acp-17-235-2017, 2017. a
IPCC: Climate Change 2013: The Physical Science Basis. Contribution of Working Group I to the Fifth Assessment Report of the Intergovernmental Panel on Climate Change, edited by: Stocker, T. F., Qin, D., Plattner, G.-K., Tignor, M., Allen, S. K., Boschung, J., Nauels, A., Xia, Y., Bex, V., and Midgley, P. M., Cambridge University Press, Cambridge, United Kingdom and New York, NY, USA, 1535 pp., https://doi.org/10.1017/CBO9781107415324, 2013. a
IPCC: Climate Change and Land: an IPCC special report on climate change, desertification, land degradation, sustainable land management, food security, and greenhouse gas fluxes in terrestrial ecosystems, edited by: Shukla, P. R., Skea, J., Calvo Buendia, E., Masson-Delmotte, V., Pörtner, H.-O., Roberts, D. C., Zhai, P., Slade, R., Connors, S., van Diemen, R., Ferrat, M., Haughey, E., Luz, S., Neogi, S., Pathak, M., Petzold, J., Portugal Pereira, J., Vyas, P., Huntley, E., Kissick, K., Belkacemi, M., and Malley, J., in press, https://www.ipcc.ch/srccl/cite-report/, 2019.
Joly, L., Parvitte, B., Zeninari, V., and Durry, G.: Development of a compact CO2 sensor open to the atmosphere and based on near-infrared laser technology at 2.68 µm, Appl. Phys. B, 86, 743–748, https://doi.org/10.1007/s00340-006-2568-4, 2007. a
Joly, L., Maamary, R., Decarpenterie, T., Cousin, J., Dumelié, N., Chauvin, N., Legain, D., Tzanos, D., Durry, G., Joly, L., Maamary, R., Decarpenterie, T., Cousin, J., Dumelié, N., Chauvin, N., Legain, D., Tzanos, D., and Durry, G.: Atmospheric Measurements by Ultra-Light SpEctrometer (AMULSE) Dedicated to Vertical Profile in Situ Measurements of Carbon Dioxide (CO2) Under Weather Balloons: Instrumental Development and Field Application, Sensors, 16, 1609, https://doi.org/10.3390/s16101609, 2016. a, b, c
Reid, K. H. and Steyn, D. G.: Diurnal variations of boundary layer carbon dioxide in a coastal city-observations and comparison with model, Atmos. Environ., 31, 3101–3114, 1997. a
Khair, Z. M. E., Joly, L., Cousin, J., Decarpenterie, T., Dumelié, N., Maamary, R., Chauvin, N., and Durry, G.: In situ measurements of methane in the troposphere and the stratosphere by the Ultra Light SpEctrometer Amulse, Appl. Phys. B, 123, 281, https://doi.org/10.1007/s00340-017-6850-4, 2017. a
Lelieveld, J., Klingmüller, K., Pozzer, A., Pöschl, U., Fnais, M., Daiber, A., and Münzel, T.: Cardiovascular disease burden from ambient air pollution in Europe reassessed using novel hazard ratio functions, Eur. Heart J., 40, 1590–1596, 2019. a
Liang, A., Gong, W., Han, G., and Xiang, C.: Comparison of satellite-observed XCO2 from GOSAT, OCO-2, and ground-based TCCON, Remote Sens., 9, 1033, https://doi.org/10.3390/rs9101033, 2017. a
Naik, V., Voulgarakis, A., Fiore, A. M., Horowitz, L. W., Lamarque, J.-F., Lin, M., Prather, M. J., Young, P. J., Bergmann, D., Cameron-Smith, P. J., Cionni, I., Collins, W. J., Dalsøren, S. B., Doherty, R., Eyring, V., Faluvegi, G., Folberth, G. A., Josse, B., Lee, Y. H., MacKenzie, I. A., Nagashima, T., van Noije, T. P. C., Plummer, D. A., Righi, M., Rumbold, S. T., Skeie, R., Shindell, D. T., Stevenson, D. S., Strode, S., Sudo, K., Szopa, S., and Zeng, G.: Preindustrial to present-day changes in tropospheric hydroxyl radical and methane lifetime from the Atmospheric Chemistry and Climate Model Intercomparison Project (ACCMIP), Atmos. Chem. Phys., 13, 5277–5298, https://doi.org/10.5194/acp-13-5277-2013, 2013. a
Nalli, N. R., Barnet, C. D., Reale, A., Tobin, D., Gambacorta, A., Maddy, E. S., Joseph, E., Sun, B., Borg, L., Mollner, A. K., Morris, V. R., Liu, X., Divakarla, M., Minnett, P. J., Knuteson, R. O., King, T. S., and Wolf, W. W.: Validation of satellite sounder environmental data records: Application to the Cross-track Infrared Microwave Sounder Suite, J. Geophys. Res.-Atmos., 118, 13–628, 2013. a
Nalli, N. R. Gambacorta, A., Liu, Q., Barnet, C. D., Tan, C., Iturbide-Sanchez, F., Reale, T., Sun, B., Wilson, M., Borg, L., and Morris, V. R.: Validation of atmospheric profile retrievals from the SNPP NOAA-unique combined atmospheric processing system. Part 1: Temperature and moisture, IEEE T. Geosci. Remote, 56, 180–190, 2017a. a
Nalli, N. R., Gambacorta, A., Liu, Q., Tan, C., Iturbide-Sanchez, F., Barnet, C. D., Joseph, E., Morris, V. R., Oyola, M., and Smith, J. W.: Validation of Atmospheric Profile Retrievals from the SNPP NOAA-Unique Combined Atmospheric Processing System. Part 2: Ozone, IEEE T. Geosci. Remote, 56, 598–607, 2017b. a, b, c
Nédélec, P., Blot, R., Boulanger, D., Athier, G., Cousin, J.-M., Gautron, B., Petzold, A., Volz-Thomas, A., and Thouret, V.: Instrumentation on commercial aircraft for monitoring the atmospheric composition on a global scale: the IAGOS system, technical overview of ozone and carbon monoxide measurements, Tellus B, 67, 27791, https://doi.org/10.3402/tellusb.v67.27791, 2015. a
NWPSAF: Current Software Packages, available at: https://www.nwpsaf.eu/site/software, last access: 26 May 2020.
Petzold, A., Thouret, V., Gerbig, C., Zahn, A., Brenninkmeijer, C. A. M., Gallagher, M., Hermann, M., Pontaud, M., Ziereis, H., Boulanger, D., Marshall, J., Nédélec, P., Smit, H. G. J., Friess, U., Flaud, J.-M., Wahner, A., Cammas, J.-P., and Volz-Thomas, A.: Global-scale atmosphere monitoring by in-service aircraft – current achievements and future prospects of the European Research Infrastructure IAGOS, Tellus B, 67, 28452, https://doi.org/10.3402/tellusb.v67.28452, 2015. a
Rodgers, C. D.: Inverse methods for atmospheric sounding: theory and practice, vol. 2, World Scientific, Oxford, UK, 2000. a
Saunders, R., Hocking, J., Turner, E., Rayer, P., Rundle, D., Brunel, P., Vidot, J., Roquet, P., Matricardi, M., Geer, A., Bormann, N., and Lupu, C.: An update on the RTTOV fast radiative transfer model (currently at version 12), Geosci. Model Dev., 11, 2717–2737, https://doi.org/10.5194/gmd-11-2717-2018, 2018. a
Schmidt, M., Lopez, M., Yver Kwok, C., Messager, C., Ramonet, M., Wastine, B., Vuillemin, C., Truong, F., Gal, B., Parmentier, E., Cloué, O., and Ciais, P.: High-precision quasi-continuous atmospheric greenhouse gas measurements at Trainou tower (Orléans forest, France), Atmos. Meas. Tech., 7, 2283–2296, https://doi.org/10.5194/amt-7-2283-2014, 2014. a
Seity, Y., Brousseau, P., Malardel, S., Hello, G., Bénard, P., Bouttier, F., Lac, C., and Masson, V.: The AROME-France Convective-Scale Operational Model, Mon. Weather Rev., 139, 976–991, https://doi.org/10.1175/2010MWR3425.1, 2011. a
Smith, F.: NWPSAF 1D-Var User Manual, Met Office, Exeter, UK, NWPSAF-MO-UD-032, 2016. a
Smith, N. and Barnet, C. D.: Uncertainty Characterization and Propagation in the Community Long-Term Infrared Microwave Combined Atmospheric Product System (CLIMCAPS), Remote Sens., 11, 1227, https://doi.org/10.3390/rs11101227, 2019. a
Susskind, J., Barnet, C. D., and Blaisdell, J. M.: Retrieval of atmospheric and surface parameters from AIRS/AMSU/HSB data in the presence of clouds, IEEE T. Geosci. Remote, 41, 390–409, 2003. a, b
Thompson, D. R., Chris Benner, D., Brown, L. R., Crisp, D., Malathy Devi, V., Jiang, Y., Natraj, V., Oyafuso, F., Sung, K., Wunch, D., Castaño, R., and Miller, C. E.: Atmospheric validation of high accuracy CO2 absorption coefficients for the OCO-2 mission, J. Quant. Spectrosc. Ra., 113, 2265–2276, https://doi.org/10.1016/j.jqsrt.2012.05.021, 2012. a
Voulgarakis, A., Naik, V., Lamarque, J.-F., Shindell, D. T., Young, P. J., Prather, M. J., Wild, O., Field, R. D., Bergmann, D., Cameron-Smith, P., Cionni, I., Collins, W. J., Dalsøren, S. B., Doherty, R. M., Eyring, V., Faluvegi, G., Folberth, G. A., Horowitz, L. W., Josse, B., MacKenzie, I. A., Nagashima, T., Plummer, D. A., Righi, M., Rumbold, S. T., Stevenson, D. S., Strode, S. A., Sudo, K., Szopa, S., and Zeng, G.: Analysis of present day and future OH and methane lifetime in the ACCMIP simulations, Atmos. Chem. Phys., 13, 2563–2587, https://doi.org/10.5194/acp-13-2563-2013, 2013. a
Wecht, K. J., Jacob, D. J., Frankenberg, C., Jiang, Z., and Blake, D. R.: Mapping of North American methane emissions with high spatial resolution by inversion of SCIAMACHY satellite data, J. Geophys. Res.-Atmos., 119, 7741–7756, https://doi.org/10.1002/2014JD021551, 2014. a