the Creative Commons Attribution 4.0 License.
the Creative Commons Attribution 4.0 License.
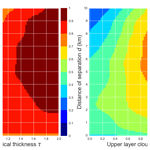
Evaluation of the MODIS Collection 6 multilayer cloud detection algorithm through comparisons with CloudSat Cloud Profiling Radar and CALIPSO CALIOP products
Benjamin Marchant
Steven Platnick
Kerry Meyer
Galina Wind
Since multilayer cloud scenes are common in the atmosphere and can be an important source of uncertainty in passive satellite sensor cloud retrievals, the MODIS MOD06 and MYD06 standard cloud optical property products include a multilayer cloud detection algorithm to assist with data quality assessment. This paper presents an evaluation of the Aqua MODIS MYD06 Collection 6 multilayer cloud detection algorithm through comparisons with active Cloud Profiling Radar (CPR) and Cloud-Aerosol Lidar with Orthogonal Polarization (CALIOP) products that have the ability to provide cloud vertical distributions and directly classify multilayer cloud scenes and layer properties. To compare active sensor products with an imager such as MODIS, it is first necessary to define multilayer clouds in the context of their radiative impact on cloud retrievals. Three main parameters have thus been considered in this evaluation: (1) the maximum separation distance between two cloud layers, (2) the thermodynamic phase of those layers and (3) the upper-layer cloud optical thickness. The impact of including the Pavolonis–Heidinger multilayer cloud detection algorithm, introduced in Collection 6, to assist with multilayer cloud detection has also been assessed. For the year 2008, the MYD06 C6 multilayer cloud detection algorithm identifies roughly 20 % of all cloudy pixels as multilayer (decreasing to about 13 % if the Pavolonis–Heidinger algorithm output is not used). Evaluation against the merged CPR and CALIOP 2B-CLDCLASS-lidar product shows that the MODIS multilayer detection results are quite sensitive to how multilayer clouds are defined in the radar and lidar product and that the algorithm performs better when the optical thickness of the upper cloud layer is greater than about 1.2 with a minimum layer separation distance of 1 km. Finally, we find that filtering the MYD06 cloud optical properties retrievals using the multilayer cloud flag improves aggregated statistics, particularly for ice cloud effective radius.
- Article
(2310 KB) - Full-text XML
- BibTeX
- EndNote
Detection of multilayer clouds using passive sensors such as the Moderate Resolution Imaging Spectroradiometer (MODIS) is a challenging but important remote sensing need. The existence of multiple cloud layers can strongly impact retrievals of cloud optical, microphysical, and cloud top properties under single-layer plane-parallel cloud assumptions. For example, the MODIS Collection 6/6.1 (C6/C6.1) cloud optical property retrievals (MOD06 and MYD06 for Terra and Aqua, respectively), which assume a homogeneous plane-parallel cloud model as in previous collections (Platnick et al., 2017), have been shown to have significant microphysical cloud retrieval errors or outright failures for pixels that are identified as multilayer. As such, a multilayer cloud detection algorithm (Wind et al., 2010) was first developed for Collection 5 as a quality assurance metric to identify multilayer cloudy scenes. The MYD06 multilayer cloud flag has subsequently been used synergistically with optical centroid cloud pressure derived from Ozone Monitoring Instrument (OMI) UV observations to further identify multilayer and vertically extended clouds (Joiner et al., 2010). Beyond MODIS, other passive multilayer cloud detection techniques use the O2 absorption bands, such as those from the Polarization and Directionality of the Earth's Reflectance (POLDER) instrument (Desmons et al., 2017), in addition to spectral signature differences between monolayer and multilayer cloud scenes determined from forward radiative transfer models (Pavolonis and Heidinger, 2004; Heidinger and Pavolonis, 2005; Nasiri and Baum, 2004; Jin and Rossow, 1997). Several studies have also been dedicated to the inference of cloud optical properties for multilayer cloud scenes, e.g., Watts et al. (2011), Sourdeval et al. (2014), and Chang and Li (2005). Those studies use a two-layer cloud model approximation coupled with, e.g., optimal estimation, to derive the cloud optical properties associated with the two cloud layers and thus inherently require robust multilayer cloud detection.
Evaluating the performance of multilayer cloud detection algorithms requires appropriate truth data sets and an understanding of the intent of the algorithm itself. For instance, the MOD06 and MYD06 multilayer cloud detection algorithm was initially evaluated using forward radiative transfer simulations (Wind et al., 2010), though these cannot fully capture the complexity of the real atmosphere. Active sensors, on the other hand, such as the CloudSat Cloud Profiling Radar (CPR) and the Cloud-Aerosol Lidar with Orthogonal Polarization (CALIOP) onboard the Cloud-Aerosol Lidar and Infrared Pathfinder Satellite Observation (CALIPSO) satellite, both in the afternoon “A-train” constellation, provide key details on cloud vertical structure. Merged CPR/CALIOP products that exploit the different yet complementary sensitivities of radar and lidar observations have demonstrated utility for evaluating passive multilayer cloud detection algorithms. In fact, the MOD06 and MYD06 multilayer cloud flag previously has been evaluated by Wang et al. (2016) using the 2B-CLDCLASS-LIDAR product for the years 2007–2010 and by Desmons et al. (2017), who in parallel evaluated the PARASOL-POLDER multilayer cloud detection algorithm using the 2B-GEOPROF-lidar and CALIOP 5 km cloud layer products for the years 2006–2010. These investigations, however, broadly defined multilayer clouds in the radar and lidar data sets and thus implicitly did not consider the intent of the MOD06 and MYD06 multilayer cloud detection algorithm, which is to identify scenes where a second cloud layer adversely impacts the optical property retrievals of the radiatively dominant cloud layer (the primary example being a thin ice cloud overlying an optically thicker liquid water cloud), rather than as a strict multilayer detection algorithm. For example, Desmons et al. (2017) defined multilayer cloud as when CPR and CALIOP detected two spatially distinct cloud layers, regardless of the separation distance between the cloud layers and cloud thermodynamic phase, while Wang et al. (2016) specified only that detected cloud layers must be separated vertically by at least 480 m to be considered multilayer.
In this paper, the main purpose is to present an evaluation of the Aqua MODIS (MYD06) C6 multilayer cloud detection algorithm through comparisons with CPR and CALIOP merged products. In addition, we also will evaluate how multilayer clouds affect MYD06 cloud thermodynamic-phase results. In the first section we provide a short overview of the MOD06 and MYD06 multilayer cloud detection algorithm. The second section provides details about the data sets and the methodology used for the evaluation. The third section presents evaluation results as a function of three main parameters used to define a multilayer cloud scene in the CPR/CALIOP merged products: (1) the separation distance d between the two radiatively dominant cloud layers, (2) the thermodynamic phase of those layers and (3) the layer optical thicknesses, in particular of the upper cloud layer. Finally, in the last section, we show the impact of multilayer clouds on cloud effective radius (CER) retrievals.
Originally introduced in Collection 5 (C5), the MOD06 and MYD06 multilayer cloud detection algorithm was developed as a quality assurance (QA) flag to identify scenes where the single-layer cloud forward model assumption is likely violated. Its primary targets are those scenes where an optically thinner cloud overlies an optically thicker liquid cloud, either where the phases of the two layers differ (ice over liquid) or the vertical separation is sufficiently large such that retrievals of the optical properties of the radiatively dominant underlying cloud are adversely impacted. The algorithm operates on a pixel-level basis (1 km resolution at nadir), with cumulative results reported in the Cloud_Multi_Layer_Flag Science Data Set (SDS) in the MOD06 and MYD06 Level 2 files and individual test results reported as bit values in the Quality_Assurance_1 km SDS. Full details on the C5 algorithm can be found in Wind et al. (2010). Updates for C6/C6.1 are summarized in Platnick et al. (2017) and in the C6/C6.1 User's Guide (Platnick et al., 2017).
The algorithm is based primarily on four tests that are collectively used to classify a cloudy pixel as monolayer or multilayer.
-
A cloud thermodynamic-phase difference test, where divergent results between the IR phase algorithm (Baum et al., 2012) and the shortwave/IR optical properties phase algorithm (Marchant et al., 2016) yield a positive multilayer cloud result.
-
An above-cloud precipitable water (PW) difference test (ΔPW), using the relative difference between above-cloud PW derived from the CO2-slicing cloud top pressure result and that derived from the 0.94 µm channel with respect to the total PW (TPW) derived from ancillary atmospheric profiles. A relative difference larger than 8 % yields a positive multilayer cloud result.
-
A second above-cloud PW difference test (ΔPW900 mb), similar to the ΔPW test above but assuming the cloud is located at 900 mb when deriving above-cloud PW from the 0.94 µm channel. Again, a relative difference of 8 % yields a positive multilayer cloud result.
-
A test based on the algorithm of Pavolonis and Heidinger (2004) (hereafter referred to as PH04 for brevity), introduced in C6, that uses reflectance at 0.65, 1.6, and 1.38, 11 and 12 µm brightness temperatures; and brightness temperature differences.
A test based on the divergence of cloud optical thickness (COT) retrievals from the standard VNSWIR (visible, near infrared or shortwave infrared) 2.1 µm channel pair and the 1.6–2.1 µm channel pair was also introduced in C6, but updates to the optical properties retrieval solution logic rendered this test ineffective (see Platnick et al., 2017), and we do not consider it here. Note that the MOD06 and MYD06 multilayer cloud algorithm is only applied to pixels having COT larger than 4. Moreover, during algorithm development, the above tests, when positive, were assigned predefined confidence values, the summation of which is reported in the Cloud_Multi_Layer_Flag SDS and is intended to provide a pseudo-confidence level: a value of 0 indicates no cloud was detected, 1 indicates a monolayer cloud and values 2–10 indicate the cumulative weight of the positive multilayer tests. Thus, this analysis used MODIS MYD06 SDS with a value greater or equal to 2 to define multilayer clouds and the MYD06 1 km quality assurance to turn off the Pavolonis–Heidinger test.
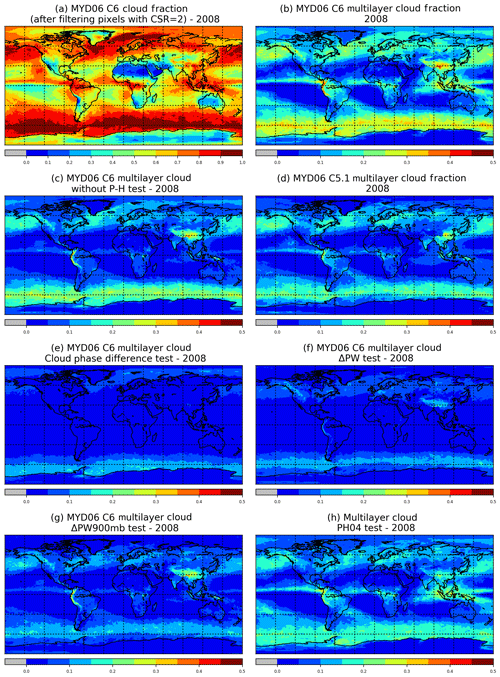
Figure 1A collection of aggregated (all pixel) Aqua MODIS Level 2 cloud products over the year 2008: (a) cloud fraction, (b) C6.1 multilayer cloud fraction, (c) C6.1 multilayer cloud fraction excluding the Pavolonis and Heidinger (PH04) test, and (d) C5.1 multilayer cloud fraction. Fractions determined from each individual C6.1 multilayer cloud detection test: (e) cloud-phase difference test, (f) ΔPW test (g) ΔPW900 mb test and (h) PH04 test. Note that panel (b) is a weighted combination of panels (e) to (h).
Figure 1 shows aggregated Aqua MODIS MYD06 Level 2 cloud products over the year 2008 (all data from C6.1 unless otherwise noted): (Fig. 1a) total cloud fraction from the MYD35 cloud mask product after removing pixels identified as heavy aerosol or sun glint by the MYD06 clear sky restoral (CSR) algorithm, (Fig. 1b) multilayer cloud fraction, (Fig. 1c) multilayer cloud fraction without the PH04 test, and (Fig. 1d) C5.1 multilayer cloud fraction. The multilayer cloud fractions determined by each individual C6/C6.1 multilayer cloud detection test are shown in the remaining panels: (Fig. 1e) cloud-phase difference test, (Fig. 1f) ΔPW test, (Fig. 1g) ΔPW900 mb test and (Fig. 1h) PH04 test. Note that the multilayer fraction shown in Fig. 1c uses a similar definition for multilayer clouds, i.e., excluding the PH04 test, as do the MOD08/MYD08 C6/C6.1 Level 3 (L3) aggregated products; this test was excluded during C6 L3 development after preliminary analysis indicated that it was overly aggressive in some circumstances. For the year 2008, we find that about 20 % of cloudy pixels are flagged as multilayer clouds, a number that decreases to 13 % if the PH04 test is excluded (similar to MOD06 and MYD06 C5 results, Fig. 1d). Considering the multilayer cloud fraction in Fig. 1b where all tests contribute to the results, we find that about 21 % of all positive multilayer cloud results have a positive cloud-phase difference test, 28% have a positive ΔPW test, 44 % have a positive ΔPW900 mb test and 74 % have a positive PH04 test.
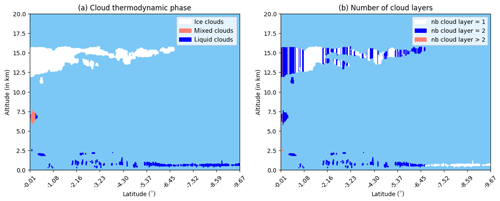
Figure 2An example 2B-CLDCLASS-lidar curtain (2008183012329_11573_CS_2B-CLDCLASS-LIDAR_GRANULE_P_R04_E02.hdf): (a) cloud thermodynamic phase for each detected cloud layer (ice, liquid or mixed) and (b) the number of cloud layers identified after merging cloud layers with a vertical separation distance less than 3 km.
We evaluate the MODIS C6 multilayer cloud detection algorithm using co-located A-Train CloudSat CPR and CALIPSO CALIOP data during the year 2008. Due to its location in the A-Train, only Aqua MODIS MYD06 data are used; note that the multilayer algorithm applied to Terra MODIS is identical to the one applied to Aqua MODIS. Rather than consider CPR data separately, we use the 2B-CLDCLASS-lidar CPR-CALIOP merged product in addition to the CALIOP Version 4 5 km cloud layer products. The 2B-CLDCLASS-lidar product combines CPR and CALIOP observations to provide cloud top and base heights jointly with cloud thermodynamic phase (ice, liquid or mixed) for each cloud layer (more details can be found in Wang et al., 2012). Note that in 2B-CLDCLASS-lidar, mixed phase is defined as when the lidar identifies a liquid layer cloud but the layer top temperature is colder than −7 ∘C and the corresponding CloudSat CPR Ze is large, implying the layer is dominated by ice particles. Figure 2 shows an example 2B-CLDCLASS-lidar curtain for a 1 July 2008 data segment starting at 1 h 23 min. This product provides up to 10 vertical cloud layers at 1 km horizontal resolution along-track. Since the upper cloud layer optical thickness is critical in understanding the impact of multilayer cloud scenes on MYD06 cloud optical property retrievals, cloud optical thickness from the CALIOP 5 km layer product is merged with the CLDCLASS-lidar product. This is accomplished by resampling the CALIOP product at 1 km and searching for matching cloud layers between the CALIOP 5 km and 2B-CLDCLASS-lidar 1 km cloud layer products. Co-located files of MODIS and 2B-CLDCLASS-lidar have also been created that contain the pixel indices of 2B-CLDCLASS-lidar and the nearest MODIS pixel in terms of spatial distance in the geographic coordinate system.
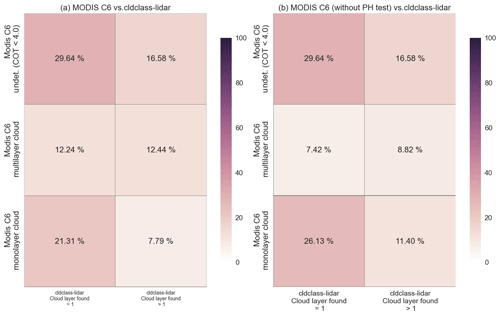
Figure 3Contingency tables of the MYD06 C6.1 multilayer cloud detection algorithm compared against multilayer clouds defined by the 2B-CLDCLASS-lidar products: MYD06 with (a) and without (b) the Pavolonis–Heidinger (PH04) test. The 2B-CLDCLASS-lidar multilayer clouds are defined regardless of the separation distance between the cloud layers, the cloud thermodynamic phase or the COT.
The global performance of the MYD06 multilayer cloud detection algorithm is shown in Fig. 3. Here, contingency tables comparing MYD06 multilayer classification results to those from the 2B-CLDCLASS-lidar products are shown when the PH04 test is (Fig. 3a) included and (Fig. 3b) excluded. Note that, for the 2B-CLDCLASS-lidar products, we use, in a first step, a naïve definition of multilayer clouds, namely all profiles where the merged product indicates more than one cloud layer regardless of layer phase, optical thickness or separation distance. Several conclusions can be inferred from these tables. First, for the cloudy pixel population for which the MYD06 multilayer detection algorithm is not applied (COT < 4, top rows), the 2B-CLDCLASS-lidar product indicates a quite high percentage of multilayer clouds, i.e., 16.58 % of the total cloudy population. As we will show in the next section, this imposed multilayer detection limit in MYD06 can impact CER retrieval statistics. For the cloudy pixel population for which the MYD06 multilayer detection algorithm is applied (COT > 4, middle and bottom rows), the MYD06 results including the PH04 test agree with the 2B-CLDCLASS-lidar monolayer and multilayer classifications 33.75 % of the time (21.31 % for monolayer and 12.44 % for multilayer) and disagree 20.03 % of the time (12.24 % false multilayer detection rate and 7.79 % false monolayer detection rate). When the PH04 test is not included, the agreement and disagreement percentages remain roughly the same, 34.95 % and 18.82 %, respectively, though the apportionment between true or false monolayer and multilayer detection changes.
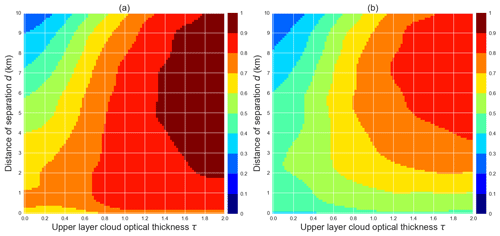
Figure 4Probabilities that MYD06 detects a multilayer cloud, with (a) and without (b) the Pavolonis–Heidinger (PH04) test, given the separation distance between two cloud layers and the cloud optical thickness of the upper layer derived from 2B-CLDCLASS-lidar and CALIOP 5 km cloud products, respectively.
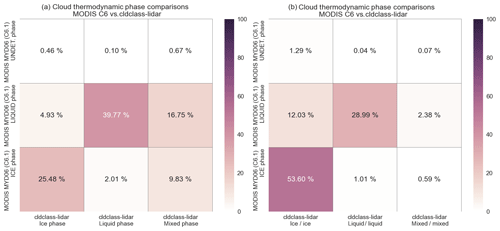
Figure 5MYD06 C6.1 cloud thermodynamic phase compared to 2B-CLDCLASS-lidar cloud phase: (a) monolayer clouds (about 63 % of the data set) and (b) multilayer clouds having the same phase (about 10 % of the co-located data set). Here, monolayer and multilayer clouds are defined by 2B-CLDCLASS-lidar.
While it is evident in Fig. 3 that MYD06 misses a relatively large percentage of multilayer clouds that the radar and lidar merged product detects (7.79 % or 11.40 % when the PH04 test is included or excluded, respectively), the active sensors are much more capable of detecting multilayer cloud scenes than MODIS. More importantly, as we will see in the next section, in many cases these missed multilayer scenes do not adversely impact the optical property retrieval statistics and are thus beyond the intent of the algorithm. It is therefore important to evaluate the algorithm's performance as a function of two parameters directly related to its intended targets, namely the optical thickness of the upper-layer cloud and the vertical separation distance of the cloud layers.
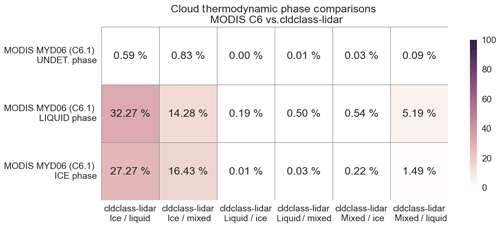
Figure 6MYD06 C6.1 cloud optical properties thermodynamic phase compared to 2B-CLDCLASS-lidar cloud phase for multilayer clouds that have a different cloud phase in the vertical profile. “Ice/liquid” refers to an upper ice layer overlying a liquid cloud layer and similarly for other x labels (about 20 % of the co-located data set).
To better understand the multilayer cloud scenes, we focus on multilayer cloud scenes with only two cloud layers (which represent about 77 % of the multilayer cloud population in our co-located data set). Figure 4 shows the probability that MYD06 correctly identifies a multilayer cloud, using the 2B-CLDCLASS-lidar data as truth, given the separation distance d (the distance between the cloud base of the upper cloud and the cloud top of the bottom cloud) and the upper-layer COT τ defined by the CALIOP 5 km cloud layer products. Results are shown when (Fig. 4a) including and (Fig. 4b) excluding the PH04 test. Note that all 2B-CLDCLASS-lidar multilayer cloud scenes are included in the baseline here regardless of layer thermodynamic phase. One can see from Fig. 4a that the PH04 test is very sensitive to multilayer clouds, even if d and τ are quite small, at the expense of a larger false positive rate (see Fig. 3a). On the other hand, if the PH04 test is not used (Fig. 4b), one can see that the probability of correctly detecting a multilayer cloud scene increases with both d and τ. Regardless of the inclusion of the PH04 test, however, the results shown here indicate that it is probable that MYD06 will detect a multilayer cloud if the separation distance d is greater than 1 km and the upper-layer COT is greater than about 1.2.
In addition to cloud layer detection, the 2B-CLDCLASS-lidar products also provide a cloud thermodynamic phase classification, i.e., liquid, ice or mixed phase, for each detected cloud layer that can be used to evaluate the performance of the MYD06 cloud optical properties phase algorithm in multilayer scenes. Note that the C6 and C6.1 MOD06 and MYD06 phase algorithm was tuned and validated against the CALIOP 1 and 5 km cloud layer products using 2 months of co-located data, though only for scenes where CALIOP observed only a single phase in the profile (Marchant et al., 2016). Figure 5a shows a similar single-phase validation using the 2B-CLDCLASS-lidar products for monolayer clouds only with a single cloud phase in 2008. While agreement for liquid and ice phase results is 65.22 %, 26.62 % of 2B-CLDCLASS-lidar monolayer clouds are identified as mixed phase, of which MYD06 identifies 9.83 % and 16.75 % as ice and liquid phase, respectively.
Extending this monolayer analysis to multilayer cloud scenes, two types of multilayer cases can be distinguished in the 2B-CLDCLASS-lidar product, namely profiles where the multiple cloud layers share the same thermodynamic phase and those where they do not. Figure 5b shows the comparison between the MYD06 cloud optical properties phase and the 2B-CLDCLASS-lidar product for two cloud layers sharing the same cloud phase (roughly 10 % of the co-located data set). When 2B-CLDCLASS-lidar identifies two ice layers or two liquid layers in the profile, the MYD06 phase agrees 82.59 % of the time. However, in 12.03 % of the multilayer cases, MYD06 misidentifies an ice cloud overlapping another ice cloud as liquid cloud phase.
Figure 6 shows phase comparison results for the cases where 2B-CLDCLASS-lidar identifies two cloud phases in the vertical profile (roughly 20 % of the co-located data set). The most frequent cloud scene is an ice cloud overlapping a liquid cloud (59.54 % of these cases, first column), for which MYD06 identifies fractions of 27.27 % ice and 32.27 % liquid clouds. For ice clouds overlapping mixed-phase clouds, the second most frequent scene (30.71 % of these cases, second column), MYD06 is more likely to identify ice phase (16.43 %) rather than liquid phase (14.28 %).
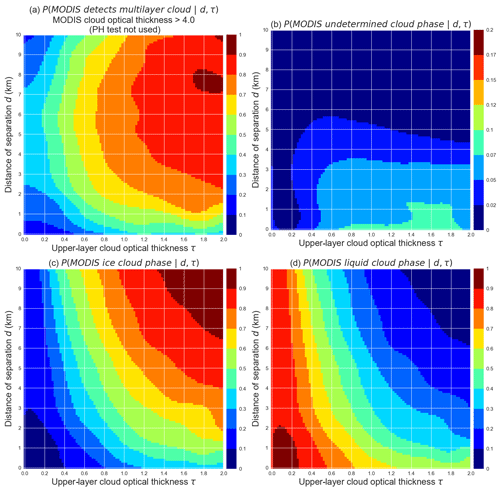
Figure 7(a) Probability that the MYD06 multilayer cloud detection algorithm detects an ice cloud overlapping a liquid cloud (with the PH test turned off) given the separation distance (d) between the two cloud layers and the upper-layer cloud optical thickness (τ) defined by the 2B-CLDCLASS-lidar products, and probabilities that the MYD06 cloud optical properties phase algorithm provides an undetermined (b), ice (c) or liquid (d) cloud phase given d and τ.
The ambiguity of the results in Fig. 6 underscores the difficulty of determining a single phase in a multilayer scene using MODIS when there is no unique answer about the true column phase. Moreover, because the MYD06 cloud optical properties phase is a radiatively derived designation, it must depend on, for example, the upper-layer COT and the sun- and satellite-viewing geometryḞocusing only on the case of ice clouds overlapping liquid clouds, Fig. 7 shows the probability that MYD06 (Fig. 7a) correctly identifies a multilayer cloud (PH04 test excluded), and the probabilities of (Fig. 7b) undetermined, (Fig. 7c) ice-phase, and (Fig. 7d) liquid-phase results, each as a function of layer separation distance d and upper-layer COT τ. The probability that MYD06 correctly identifies an ice cloud overlapping a liquid cloud as multilayer (Fig. 7a) is similar in pattern to the probabilities for all multilayer scenes regardless of the cloud-layer phase in Fig. 4b, though the magnitude of the probabilities here is larger. The MYD06 phase result probabilities (Fig. 7b–d) are largely what one would expect, in particular that the probability of an ice cloud result increases as the upper ice COT increases, while the probability of a liquid cloud result shows the opposite pattern; the probability of an undetermined phase result is largest when the two cloud layers are vertically close and the upper-layer COT is greater than 0.7.
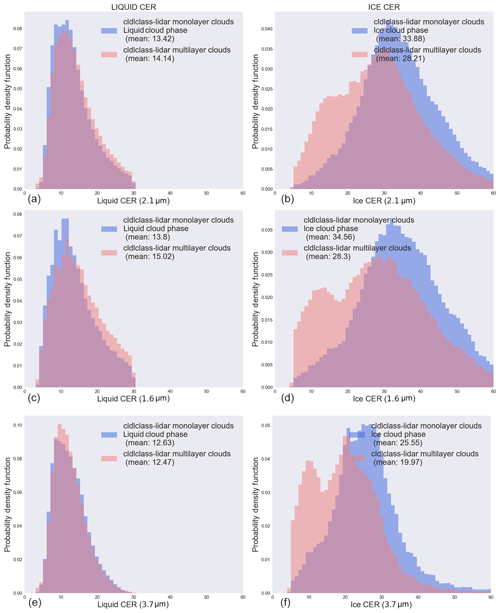
Figure 8MYD06 1.6, 2.1, and 3.7 µm liquid (a, c, e) and ice (b, d, e) CER retrieval distributions for monolayer (light blue) and multilayer (light red) cloud populations as determined by the 2B-CLDCLASS-lidar products regardless of the cloud layer separation distance or the upper-layer cloud optical thickness.
Given the intent of the MOD06 and MYD06 multilayer cloud detection algorithm, namely to identify scenes that do not conform to the single-layer cloud forward model assumption, we assess the utility of the multilayer algorithm's results as a QA tool for the cloud optical property retrievals. In particular, we focus on CER retrievals, where multilayer scenes are expected to have retrieval artifacts or uninterpretable results due to the mixing of particle scattering properties from multiple cloud layers having different phases and/or microphysics. To facilitate the analysis, we again use the co-located MYD06 and 2B-CLDCLASS-lidar 2008 data set, and consider two cloudy pixel populations: (1) a reference population containing only monolayer clouds as determined by the 2B-CLDCLASS-lidar product for which the cloud thermodynamic phase is in agreement with that of MYD06 and (2) a population of multilayer clouds, defined as those for which the 2B-CLDCLASS-lidar product identifies more than one cloud layer regardless of the cloud layer separation distance, the upper-layer COT or the cloud thermodynamic phase.
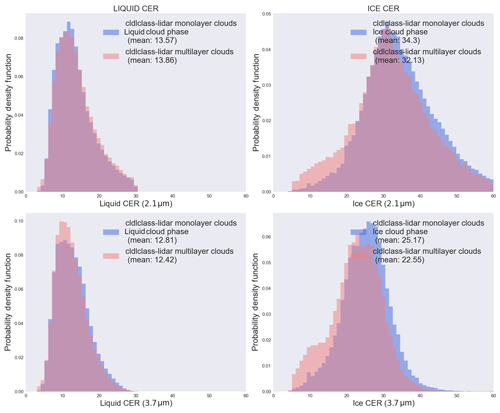
Figure 10The same as Fig. 9 but excluding the Pavolonis–Heidinger detection algorithm in the MYD06 multilayer cloud detection algorithm.
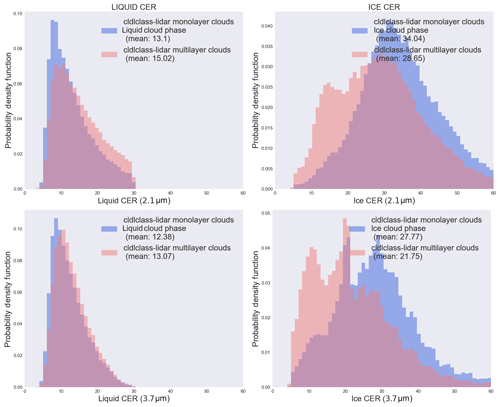
Figure 11Differences in MYD06 CER distributions for monolayer (in blue) and multilayer (in red) clouds for the population that have MYD06 cloud optical thickness lower than 4.
Figure 8 presents the results for liquid (Fig. 8a, c, e) and ice (Fig. 8b, d, f) clouds for the three primary CER retrievals reported in the MYD06 cloud optical products, namely those associated with three particle absorptive bands at 2.1, 1.6 and 3.7 µm. One can see the differences between the monolayer cloud (blue) and multilayer cloud (red) populations. The liquid CER distributions have relatively small differences, with the multilayer cloud populations tending towards larger CER, while ice CER populations exhibit the largest differences. In particular, the ice CER distributions for the multilayer cloud population have a secondary mode at an effective radius around 10–15 µm. This secondary mode can be explained by a large fraction of cases in the co-located data set having ice that overlaps liquid clouds (see Fig. 6, left column). Since liquid droplets are less absorptive than ice crystals in these spectral channels for a given size, identifying these scenes as ice phase can yield smaller ice CER retrievals. Indeed, if we remove those cloudy pixels classified by MYD06 as multilayer from the multilayer population, as shown in Fig. 9 for cases where MYD06 COT exceeds 4, one can see that the secondary peaks in the ice effective radius distributions for multilayer clouds (red) have disappeared. Therefore, though the MYD06 multilayer cloud detection is not able to detect all multilayer clouds, it can be used to filter CER retrievals that are radiatively impacted by multilayer cloud scenes. Even if the PH04 algorithm is ignored in the MYD06 multilayer cloud detection algorithm (Fig. 10), the multilayer detection results remain useful for removing most of the differences between the two populations, though some portion of the small ice cloud effective radii remain.
If the MODIS COT is lower than 4, there are important uncertainties in the CER retrievals and the multilayer cloud detection algorithm is not applied since forward modeling indicated that there is not enough information to discriminate monolayer and multilayer clouds (Wind et al., 2010). However, Fig. 11 shows that some noticeable differences can still be found in the MODIS CER distributions for monolayer and multilayer clouds, as identified by the 2B-CLDCLASS-lidar products. It is then not possible to directly screen out the CER that is strongly biased by the presence of multilayer cloud scenes as we showed previously.
This paper presented an evaluation of the Aqua MODIS MYD06 C6 multilayer cloud detection algorithm via comparison with merged CloudSat CPR and CALIOP products. As expected, the results are quite sensitive to the definition of a multilayer cloud scene for active sensor products. Therefore, three main parameters have been used to defined a multilayer cloud scene: (1) the maximum separation distance d between the two cloud layers, (2) the thermodynamic phase of those layers, and (3) the upper-layer optical thicknesses. Overall, the global MODIS multilayer cloud detection algorithm skill performs well when the optical thickness of the upper layer is greater than about 1–2 and the separation distance d is greater than 1 km. In parallel, the impact of using a 1.38 µm channel in a multilayer algorithm (PH04, Pavolonis and Heidinger, 2004) was studied; PH04 was added as a separate test to the MODIS multilayer algorithm beginning with Collection 6. It was found that this algorithm flags too many cloudy scenes as multilayer, leading to an increase in false positive occurrences, i.e. cloudy pixels wrongly flagged as multilayer.
This study also allowed for an expanded evaluation of the MODIS cloud thermodynamic phase (Marchant et al., 2016) that was based on single-layer CALIOP observations to the more general case of multilayer cloud scenes. For monolayer clouds, the current analysis based on CPR and CALIOP gives results similar to Marchant et al. (which used a different time period) in terms of showing a phase agreement fraction of about 91 %. For two spatially separated cloud layers detected by the CPR and CALIOP sensors, scenes with the same cloud phase in the two layers were analyzed separately from scenes that have different layer phases. When the cloud phase is liquid in both cloud layers, there is good agreement between the MODIS and active sensor cloud phases. When an ice cloud layer overlies another ice layer, the MODIS phase is often retrieved as liquid; further investigation is needed for these cases. When the cloud phase is different in the two cloud layers, the preferred phase for MODIS should be based on the radiative contribution from each layer to the observed signal. For instance, the most frequent cases, according to 2B-CLDCLASS-lidar products, are ice overlying liquid clouds for which the fraction of ice or liquid cloud retrieved by MODIS are about the same, but this includes radiatively thin upper cloud layers. MYD06 is more and more likely to identify ice phase rather than liquid phase with the increase in the ice COT.
Even though the MODIS C6 multilayer cloud detection algorithm is not able to detect all multilayer cloud scenes compared to the merged CPR and CALIOP product (MYD06 results including the PH04 test agree with the 2B-CLDCLASS-lidar monolayer and multilayer classifications 33.73 % of the time and disagree 20.04 % of the time), the algorithm is reasonably skilled in its intended use, i.e., discriminating those pixels for which the CER may be biased by layers that have different microphysics (phase and/or effective particle size). MODIS ice-phase categorized clouds have effective radius retrievals that are most impacted by multilayer cloud scenes with a small radius bias. If the PH04 detection algorithm output is not used, the fraction of multilayer clouds flagged by MODIS is smaller but the MODIS multilayer cloud algorithm then has less skill to screen out CER impacted by the presence of multilayer clouds. Finally, it was found that when the column COT is less than 4, the cutoff used by the MODIS algorithm, CER retrievals can still be impacted by multilayer clouds identified with the active sensor products. Further work on extending the MODIS multilayer cloud detection algorithm to smaller column cloud optical thicknesses is warranted.
Thus, the main practical implications and conclusions found during this analysis are as follows.
-
MODIS MYD06 multilayer cloud detection (corresponding to MODIS MYD06 multilayer cloud SDS greater or equal to 2) should primarily be used as a cloud optical property retrieval quality indicator.
-
As a quality indicator, the MODIS MYD06 multilayer cloud SDS performs well when used to remove cloud effective radius retrievals impacted by multilayer clouds, particularly for ice clouds.
-
The Pavolonis–Heidinger multilayer cloud detection test (that can be found on MODIS MYD06 C6 QA 1 km flag) added in MODIS MYD06 C6 has the primary goal of detecting all multilayer clouds regardless of the impact of the cloud optical retrievals. This explains why this test substantially increased the fraction of MODIS C6 multilayer cloud compared to MODIS C5 and why this test is turned off in order to aggregate MODIS C5 multilayer cloud to L3.
The data used in this analysis are publicly available and can be found at the ICARE Data and Services Center. Random sample datasets created for this analysis can be downloaded from https://www.science-emergence.com/Jupyter/MODIS_myd06_collection_6_multilayer_clouds_analysis/ (last access: 18 June 2020). They can also be obtained directly from Benjamin Marchant (benjamin.marchant@nasa.gov).
BM developed the statistical tools and prepared the manuscript with the help of the co-authors.
The authors declare that they have no conflict of interest.
We thank the four anonymous reviewers, whose comments and suggestions helped improve and clarify this paper.
This paper was edited by Alexander Kokhanovsky and reviewed by four anonymous referees.
Baum, B. A., Menzel, W. P., Frey, R. A., Tobin, D. C., Holz, R. E., Ackerman, S. A., Heidinger, A. K., and Yang, P.: MODIS Cloud-Top Property Refinements for Collection 6, J. Appl. Meteorol. Climatol., 51, 1145–1163, https://doi.org/10.1175/jamc-d-11-0203.1, 2012.
Chang, F.-L. and Li, Z.: A New Method for Detection of Cirrus Overlapping Water Clouds and Determination of Their Optical Properties, J. Atmos. Sci., 62, 3993–4009, https://doi.org/10.1175/jas3578.1, 2005.
Desmons, M., Ferlay, N., Parol, F., Riédi, J., and Thieuleux, F.: A Global Multilayer Cloud Identification with POLDER/PARASOL, J. Appl. Meteorol. Clim., 56, 1121–1139, https://doi.org/10.1175/jamc-d-16-0159.1, 2017.
Heidinger, A. K. and Pavolonis, M. J.: Global Daytime Distribution of Overlapping Cirrus Cloud from NOAA's Advanced Very High-Resolution Radiometer, J. Climate, 18, 4772–4784, https://doi.org/10.1175/jcli3535.1, 2005.
Jin, Y. and Rossow, W. B.: Detection of cirrus overlapping low-level clouds, J. Geophys. Res.-Atmos., 102, 1727–1737, https://doi.org/10.1029/96jd02996, 1997.
Joiner, J., Vasilkov, A. P., Bhartia, P. K., Wind, G., Platnick, S., and Menzel, W. P.: Detection of multi-layer and vertically-extended clouds using A-train sensors, Atmos. Meas. Tech., 3, 233–247, https://doi.org/10.5194/amt-3-233-2010, 2010.
Marchant, B., Platnick, S., Meyer, K., Arnold, G. T., and Riedi, J.: MODIS Collection 6 shortwave-derived cloud phase classification algorithm and comparisons with CALIOP, Atmos. Meas. Tech., 9, 1587–1599, https://doi.org/10.5194/amt-9-1587-2016, 2016.
Nasiri, S. L. and Baum, B. A.: Daytime Multilayered Cloud Detection Using Multispectral Imager Data, J. Atmos. Ocean. Tech., 21, 1145–1155, https://doi.org/10.1175/1520-0426(2004)021<1145:dmcdum>2.0.co;2, 2004.
Pavolonis, M. J. and Heidinger, A. K.: Daytime Cloud Overlap Detection from AVHRR and VIIRS, J. Appl. Meteorol., 43, 762–778, https://doi.org/10.1175/2099.1, 2004.
Platnick, S., Meyer, K. G., King, M. D., Wind, G., Amarasinghe, N., Marchant, B., Arnold, G. T., Zhang, Z., Hubanks, P. A., Holz, R. E., Yang, P., Ridgway, W. L., and Riedi, J.: Te MODIS cloud optical and microphysical products: Collection 6 updates and examples from Terra and Aqua, IEEE T. Geosci. Remote, 55, 502–525, 2017.
Sourdeval, O., C.-Labonnote, L., Baran, A. J., and Brogniez, G.: A methodology for simultaneous retrieval of ice and liquid water cloud properties. Part I: Information content and case study, Q. J. Roy. Meteor. Soc., 141, 870–882, https://doi.org/10.1002/qj.2405, 2014.
Wang, T., Fetzer, E. J., Wong, S., Kahn, B. H., and Yue, Q.: Validation of MODIS cloud mask and multilayer flag using CloudSat-CALIPSO cloud profiles and a cross-reference of their cloud classifications, J. Geophys. Res.-Atmos., 121, 11620–11635, https://doi.org/10.1002/2016jd025239, 2016.
Wang, Z., Vane, D., Stephens, G., and Reinke, D.: Level 2 Combined Radar and Lidar Cloud Scenario Classification Product Process Description and Interface Control Document, available at: http://www.cloudsat.cira.colostate.edu/sites/default/files/products/files/2B-CLDCLASS-LIDAR_PDICD.P_R04.20120522.pdf (last access: 3 January 2019), 2012.
Watts, P. D., Bennartz, R., and Fell, F.: Retrieval of two-layer cloud properties from multispectral observations using optimal estimation, J. Geophys. Res., 116, https://doi.org/10.1029/2011jd015883, 2011.
Wind, G., Platnick, S., King, M. D., Hubanks, P. A., Pavolonis, M. J., Heidinger, A. K., Yang, P., and Baum, B. A.: Multilayer Cloud Detection with the MODIS Near-Infrared Water Vapor Absorption Band, J. Appl. Meteorol. Clim., 4911, 2315–2333, https://doi.org/10.1175/2010jamc2364.1, 2010.
- Abstract
- Introduction
- The MOD06 and MYD06 multilayer cloud detection algorithm
- Data sets and methodology
- Evaluation of the MYD06 C6 multilayer cloud detection algorithm
- Assessing the MYD06 multilayer cloud flag as an optical property retrieval quality indicator
- Conclusions
- Data availability
- Author contributions
- Competing interests
- Acknowledgements
- Review statement
- References
- Abstract
- Introduction
- The MOD06 and MYD06 multilayer cloud detection algorithm
- Data sets and methodology
- Evaluation of the MYD06 C6 multilayer cloud detection algorithm
- Assessing the MYD06 multilayer cloud flag as an optical property retrieval quality indicator
- Conclusions
- Data availability
- Author contributions
- Competing interests
- Acknowledgements
- Review statement
- References