the Creative Commons Attribution 4.0 License.
the Creative Commons Attribution 4.0 License.
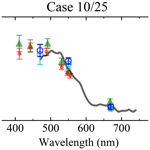
Inversion of multiangular polarimetric measurements from the ACEPOL campaign: an application of improving aerosol property and hyperspectral ocean color retrievals
Peng-Wang Zhai
Bryan A. Franz
Kirk Knobelspiesse
Amir Ibrahim
Brian Cairns
Susanne E. Craig
Guangliang Fu
Otto Hasekamp
Yongxiang Hu
P. Jeremy Werdell
NASA's Plankton, Aerosol, Cloud, ocean Ecosystem (PACE) mission, scheduled for launch in the time frame of late 2022 to early 2023, will carry the Ocean Color Instrument (OCI), a hyperspectral scanning radiometer, and two multiangle polarimeters (MAPs), the UMBC Hyper-Angular Rainbow Polarimeter 2 (HARP2) and the SRON Spectro-Polarimeter for Planetary EXploration one (SPEXone). One purpose of the PACE MAPs is to better characterize aerosol properties, which can then be used to improve atmospheric correction for the retrieval of ocean color in coastal waters. Though this is theoretically promising, the use of MAP data in the atmospheric correction of colocated hyperspectral ocean color measurements have not yet been well demonstrated. In this work, we performed aerosol retrievals using the MAP measurements from the Research Scanning Polarimeter (RSP) and demonstrate its application to the atmospheric correction of hyperspectral radiometric measurements from SPEX airborne. Both measurements were collected on the same aircraft from the Aerosol Characterization from Polarimeter and Lidar (ACEPOL) field campaign in 2017. Two cases over ocean with small aerosol loading (aerosol optical depth ∼0.04) are identified including colocated RSP and SPEX airborne measurements and Aerosol Robotic Network (AERONET) ground-based observations. The aerosol retrievals are performed and compared with two options: one uses reflectance measurement only and the other uses both reflectance and polarization. It is demonstrated that polarization information helps reduce the uncertainties of aerosol microphysical and optical properties. The retrieved aerosol properties are then used to compute the contribution of atmosphere and ocean surface for atmospheric correction over the discrete bands from RSP measurements and the hyperspectral SPEX airborne measurements. The water-leaving signals determined this way are compared with both AERONET and Moderate Resolution Imaging Spectroradiometer (MODIS) ocean color products for performance analysis. The results and lessons learned from this work will provide a basis to fully exploit the information from the unique combination of sensors on PACE for aerosol characterization and ocean ecosystem research.
- Article
(2464 KB) - Companion paper
- BibTeX
- EndNote
Ocean color remote sensing is a powerful tool for quantifying and monitoring global ocean ecosystems (Dierssen and Randolph, 2013) and provides valuable information for the estimation of phytoplankton biomass (Craig et al., 2012), primary productivity (Carr et al., 2006), and dissolved (Siegel et al., 2014) and particulate carbon pools (Fichot and Benner, 2011). Estimation of the ocean color signal from the total at-sensor spaceborne or airborne measurement is known as atmospheric correction, which removes the radiometric contributions of the atmosphere and ocean surface (Wang, 2010; Mobley et al., 2016). Quantifying the effect of atmospheric aerosols is a primary challenge in the atmospheric correction (Frouin et al., 2019), due to their diversity of size, composition, and morphology, as well as associated variability in absorption and scattering properties (Remer et al., 2019a). In addition, aerosol deposition into ocean waters contributes to the availability of nutrients that modulate phytoplankton growth and ultimately influence the trophic state of ocean ecosystems (Mahowald et al., 2005; Westberry et al., 2019). Furthermore, the ocean itself and the biological activity it supports may also be a source of aerosol (O'Dowd et al., 2002; McCoy et al., 2015; Croft et al., 2019). Better characterization of aerosol microphysical and optical properties is expected to improve the retrieved ocean color signal and, therefore, the derived geophysical products that describe ocean ecosystems (PACE, 2018; Werdell et al., 2019).
Multiangle polarimeters (MAPs), radiometers that measure spectral polarization states at multiple view angles, have been demonstrated to improve the retrieval performance of aerosol microphysical properties (Mishchenko and Travis, 1997; Chowdhary et al., 2001; Hasekamp and Landgraf, 2007; Knobelspiesse et al., 2012), including for applications over ocean waters (Jamet et al., 2019). A limited number of satellite missions carrying polarimetric payloads have been launched (Dubovik et al., 2019), including the Polarization and Directionality of the Earth's Reflectances (POLDER) instrument that was hosted on Polarization and Anisotropy of Reflectances for Atmospheric Sciences coupled with Observations from a Lidar (PARASOL; 2004–2013) and on the short-lived ADEOS and ADEOS-II missions (Tanré et al., 2011). Several more satellite missions with MAP instruments are planned to be launched in the time frame of 2022–2023, such as the European Space Agency's (ESA) Multi-viewing Multi-channel Multi-polarisation Imager (3MI) on Meteorological Operational Satellite – Second Generation (MetOp-SG) (Fougnie et al., 2018), the National Aeronautics and Space Administration (NASA) Multi-Angle Imager for Aerosols (MAIA) (Diner et al., 2018), and the NASA Plankton, Aerosol, Cloud, ocean Ecosystem (PACE) mission (Werdell et al., 2019).
The PACE observatory will include two MAPs, the UMBC Hyper-Angular Rainbow Polarimeter 2 (HARP2) (Martins et al., 2018) and the SRON Spectro-Polarimeter for Planetary EXploration one (SPEXone) (Hasekamp et al., 2019), as well as its primary instrument, a hyperspectral scanning radiometer referred to as the Ocean Color Instrument (OCI). The OCI will provide continuous spectral measurement from the ultraviolet (340 nm) to near infrared (890 nm) with 5 nm resolution, plus a set of discrete shortwave infrared (SWIR) bands centered on 940, 1038, 1250, 1378, 1615, 2130, and 2260 nm. OCI will tilt fore/aft, switching at the subsolar point, to minimize viewing sunglint. HARP2 is a wide field-of-view imager that measures polarized radiances at 440, 550, 670, and 865 nm, where the 670 nm band will be at 60 viewing angles and the other bands at 10 viewing angles. SPEXone is a narrow swath imager that performs multiangle measurements at 5 viewing angles of 0∘, , and on the ground, in a continuous spectral range spanning 385–770 nm with a resolution of 2–3 nm for intensity and 10–40 nm for polarization (Rietjens et al., 2019).
Through the combination of OCI and the two MAPs, the PACE mission provides a novel opportunity to bridge polarimetric and hyperspectral observations and advance the retrieval of both aerosol and ocean properties (Remer et al., 2019a, b; Chowdhary et al., 2019). Near-infrared (NIR) or SWIR bands are often used to derive aerosol properties over ocean waters, and that approach has been implemented for the Hyperspectral Imager for the Coastal Ocean (HICO) (Ibrahim et al., 2018). The multiband atmospheric correction (MBAC) approach which utilizes channels in the NIR to SWIR has been proposed for PACE OCI (Ibrahim et al., 2019). With the PACE instruments, a more accurate retrieval of the aerosol properties can potentially be achieved using the MAP measurements, and the improved aerosol knowledge can then be applied to advance the accuracy of atmospheric correction for OCI observations. This advancement would be especially valuable over coastal waters, where both aerosol and water optical properties are often complex. To date, there are only a few studies on performing atmospheric correction for hyperspectral radiometer measurements using aerosol properties retrieved from the colocated MAP measurements. This is primarily due to the limited availability of colocated MAP and hyperspectral radiometer measurements over ocean. One such dataset is available from the North Atlantic Aerosols and Marine Ecosystems Study (NAAMES) field campaign in 2015, where both the GEO-CAPE Airborne Simulator (GCAS) (a hyperspectral radiometer) and RSP were deployed. These datasets have been used to study the hyperspectral ocean color retrievals (Chowdhary et al., 2018).
In the fall of 2017, the Aerosol Characterization from Polarimeter and Lidar (ACEPOL) field campaign, a collaboration between NASA and Netherlands Institute for Space Research (SRON), was conducted with six passive and active instruments on the NASA ER2 high-altitude aircraft (Knobelspiesse et al., 2020). These included four MAPs: AirHARP (the airborne version of HARP2 and HARP CubeSat, McBride et al., 2020), AirMSPI (the Airborne Multiangle SpectroPolarimetric Imager) (Diner et al., 2013), SPEX airborne (the airborne version of SPEXone) (Smit et al., 2019) and the RSP (Research Scanning Polarimeter) (Cairns et al., 1999), and two lidars: HSRL-2 (the High Spectral Resolution Lidar 2) (Burton et al., 2015) and CPL (the Cloud Physics Lidar) (McGill et al., 2002). SPEX airborne collects hyperspectral radiometry and thus can be used as a proxy for OCI in developing hyperspectral ocean color algorithms. The colocated MAPs and hyperspectral SPEX airborne measurements are similar to the PACE payload and thus provide a proxy dataset to evaluate the aerosol retrieval results from MAPs and the use of these retrieved aerosol properties for hyperspectral atmospheric correction. The spectral range from the SPEX airborne measurements used in this study is from 470 to 750 nm. This does not cover the ultraviolet (UV) bands, which are nevertheless important and require further research for the PACE mission (Frouin et al., 2019; Chowdhary et al., 2019). In this study, we build further on our previous work (Gao et al., 2018, 2019) and use the MAP measurements from RSP to conduct aerosol retrievals with its well-documented measurement uncertainty analysis (Knobelspiesse et al., 2019), and we apply the results to the atmospheric correction of the SPEX airborne measurements. We identified two cases over the ocean from ACEPOL where SPEX airborne measurements and Aerosol Robotic Network (AERONET) ground-based observations are colocated with RSP.
In order to retrieve aerosol information from polarimetric measurements over the ocean, a number of advanced aerosol retrieval algorithms have been developed for both airborne and spaceborne MAPs, such as POLDER/PARASOL (Hasekamp et al., 2011; Dubovik et al., 2011, 2014), AirMSPI (Xu et al., 2016, 2019), SPEX airborne (Fu and Hasekamp, 2018; Fu et al., 2020; Fan et al., 2019), RSP (Chowdhary et al., 2005; Wu et al., 2015; Stamnes et al., 2018; Gao et al., 2018, 2019), and the Directional Polarimetric Camera (DPC) on GaoFen-5 (Wang et al., 2014; Li et al., 2018). In this study, we use the Multi-Angular Polarimetric Ocean coLor (MAPOL) retrieval algorithm, which is a joint aerosol and water-leaving radiance retrieval algorithm designed with the bio-optical models applicable to both open and coastal waters (Gao et al., 2018, 2019). MAP measurements from RSP include the visible, NIR, and SWIR (reflectance only) bands, which are used for joint aerosol and water-leaving signal retrievals. The impacts of including polarization information in the retrieval of the aerosol properties are studied by comparing the results with inputs of reflectance only and that of both reflectance and polarization in the MAPOL algorithm. We will also discuss the retrieval algorithm stability in terms of the sensitivity of the retrieval parameters to their initial guesses and compare with the uncertainty estimation based on error propagation (Knobelspiesse et al., 2012). The atmospheric correction using the aerosol properties from MAP retrievals is complementary to the approaches using the reflectance at NIR and/or SWIR bands to derive aerosol properties for the atmospheric correction on hyperspectral radiometers (Ibrahim et al., 2018, 2019) and is especially advantageous in scenarios where the aerosol properties in the visible or UV bands cannot be accurately extrapolated from measurements in the NIR–SWIR spectral range (Chowdhary et al., 2019).
The paper is organized into six sections: Sect. 2 describes the data used in the retrieval and validation of aerosol microphysical properties and water-leaving signals, Sect. 3 reviews the MAPOL retrieval algorithm and recent updates for application to hyperspectral atmospheric correction, Sects. 4 and 5 present the retrieval results and discussion, and Sect. 6 summarizes the conclusions.
During the ACEPOL field campaign, there are four flight tracks with clear skies over the AERONET USC_SEAPRISM site, located at (33.564∘ N, 118.118∘ W) and mounted on an oil platform roughly 18km away from the coast (Knobelspiesse et al., 2020). This site is part of AERONET OC, which uses special instruments that observe the water-leaving radiance in addition to the atmospheric state (Zibordi et al., 2009). Of those four, we examined two cases in detail, as summarized in Table 1, with both RSP and SPEX airborne measurements colocated with the AERONET measurements at the USC_SEAPRISM site. The two measurements are at the time of 23 October 21:33 and 25 October 2017 21:07. Hereafter we will refer to the two cases as case 10/23 and case 10/25. The locations and viewing geometries for both RSP and SPEX are specified in Fig. 1. Case 10/23 is close to the principal plane with a relative azimuth angle of 8.7∘, while case 10/25 is almost perpendicular to the principal plane with a relative azimuth angle of 94.6∘. The two cases have similar solar zenith angles of 53.3 and 50.9∘.
RSP is the airborne version of the Aerosol Polarimetry Sensor for the NASA Glory mission and has been flown in multiple field campaigns since 1999 (Cairns et al., 1999). It is a multiangle scanner measuring 152 viewing angles within 60∘ fore and aft of nadir in the along-track direction in nine channels from visible to SWIR (center wavelengths 410, 470, 550, 670, 865, 960, 1590, 1880, and 2250 nm). SPEX airborne is a hyperspectral imager with the spectral range of 400–800 nm. Its spectral resolution is 10–20 nm for degree of linear polarization (DoLP) and 2–3 nm for intensity. SPEX airborne has nine viewing angles (different from the five viewing angles of SPEXone) within the angular range of . The SPEX measurements with wavelengths larger than 750 nm are excluded from our analysis due to a grating order overlap issue in the data (Smit et al., 2019; Fu et al., 2020). The RSP and SPEX airborne data files used in this study are listed in the Data availability section.
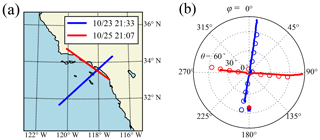
Figure 1(a) The flight tracks across the AERONET USC_SEAPRISM site. The legend shows the time at which the aircraft flew over the AERONET site. (b) The corresponding polar plot for the RSP (solid line) and SPEX (open circles) viewing directions as summarized in Table 1, where θ is the zenith angle, and φ is the relative azimuth angle between the instrument viewing azimuth angle and the sunlight azimuth angle. The asterisk symbols indicate the antisolar point.
To validate the aerosol retrieval results, we will compare our retrieved aerosol properties with the aerosol products from HSRL-2 and AERONET. The HSRL instruments provide accurate assessment of aerosol optical depth (AOD) at 355 and 532 nm (Hair et al., 2008). In this study, the HSRL-2 AOD product at 532 nm from the ACEPOL campaign with an assumed lidar ratio is used. The AERONET USC_SEAPRISM site is equipped with a CIMEL-based system called the Sea-Viewing Wide Field-of-View Sensor (SeaWiFS) Photometer Revision for Incident Surface Measurements (SeaPRISM) at eight wavelengths of 412, 443, 490, 532, 550, 667, 870, and 1020 nm with a bandwidth of 10 nm (Zibordi et al., 2009). The AERONET instrument provides direct Sun and diffuse sky-radiance measurements to infer aerosol properties (Holben et al., 1998). The measurements of direct Sun radiation are used to derive the spectral AOD (Holben et al., 1998, 2001; Eck et al., 1999; Smirnov et al., 2000). The AERONET version 3 level 2.0 data are used in this study, where the estimated AOD uncertainty is from 0.01 to 0.02 with the maximum uncertainty in the UV channels (Giles et al., 2019). The diffuse sky radiance measured at a wide range of scattering directions is used to infer the aerosol size, complex refractive index, and nonspherical particle ratio (Dubovik and King, 2000; Dubovik et al., 2006). The AERONET measurement capability can be extended to include photopolarimetric measurement with the next-generation Sun photometer, and its improvement on aerosol property retrievals has been demonstrated (Xu and Wang, 2015; Xu et al., 2015; Fedarenka et al., 2016), although such retrievals were not available for this study.
The retrieved ocean color results are compared to AERONET ocean color (AERONET OC) and the Moderate Resolution Imaging Spectroradiometer ocean color (MODIS OC) products. The MODIS OC product is processed with the atmospheric correction algorithm originating from Gordon and Wang (Gordon and Wang, 1994; Mobley et al., 2016) with the aerosol model from Ahmad et al. (2010) and is publicly available (NASA Ocean Color Web, 2020). MODIS OC provides a spatial coverage with 1km resolution at nadir. Since the aerosol properties and water-leaving signals are measured or derived at different times, geometries, and spatial resolutions, natural variation is a factor contributing to the difference when comparing the retrieved results. In order to evaluate the spatial variations when comparing with the retrieved water-leaving reflectance, we averaged the MODIS (on board Aqua) water-leaving reflectance within a 2 km region around the USC_SEAPRISM site and compute its standard deviation as its maximum uncertainty. If smaller than 5 %, the uncertainty is adopted as 5 %, which is the accuracy goal for blue band and clear water (Hu et al., 2013). The AERONET measurements are available in almost every hour and there are a total of eight measurements each day. The AERONET product provides good temporal coverage of the aerosol and ocean reflectance. We averaged the 1 d AERONET products and compared its mean with the retrieval results, where the standard deviation (6 % to 10 % for both cases) is used to represent the uncertainties. The reported uncertainty for AERONET OC Rrs is approximately 5 % between 410 and 550 nm (Zibordi et al., 2009). Note that the actual inversion uncertainties for the aerosol properties, such as the refractive index and single scattering albedo (SSA), may be larger than their daily averaged result for small AOD cases as reported by the AERONET version 3 uncertainty analysis (, ). In general, AERONET retrievals of aerosol microphysical properties become less certain as AOD decreases.
The AODs for the two cases in our discussion are 0.03–0.04 around 550 nm, as reported by HSRL-2 and AERONET observations. It is challenging to retrieve aerosol microphysical properties when the aerosol loading is small. Meanwhile the water-leaving signals are often represented by the remote sensing reflectance (Rrs; sr−1) as a ratio of the upwelling radiance and downwelling irradiance both just above the ocean surface (Mobley et al., 2016). In this study Rrs is also small with a value around 0.002–0.003 sr−1 from 400 to 550 nm reported by the AERONET ocean color product, which accounts for 5 % to 15 % of the total signal measured at the aircraft level. The percentage contribution of the water-leaving signals to the observations depends on the polarization state and the water conditions (Chowdhary et al., 2012; Zhai et al., 2017). Although the aerosol loading is small, its contribution is of the same order of magnitude as the water-leaving signal between the 400 and 550 nm range and hence remains important for atmospheric correction. Therefore, both the retrieval of aerosol microphysical properties and the water-leaving signals require high accuracy of the measurements from RSP and SPEX airborne.
Smit et al. provided a thorough comparison of the reflectance and polarization measurements between SPEX airborne and RSP from ACEPOL over ocean, cloud, and land scenes (Smit et al., 2019). For the ocean scenes, eight flight tracks were selected, and both the random and the systematic difference between the two sensors were analyzed. Over the four RSP bands of 410, 470, 550, and 670 nm, the random noise contributions to differences of reflectance are 2 %, 2 %, 2 %, and 4 %. RSP reflectance is slightly larger than SPEX reflectance at 410 and 470 nm as indicated by their systematic differences of around 4 % and 3 % respectively, which are larger than the random differences; the systematic differences at the other two bands are relatively small with values of 0 % and 1 %. For DoLP, the random differences are 0.007, 0.005, 0.003, and 0.008 for the four bands from 410 to 670 nm, and systematic differences are either similar or smaller. This suggests that DoLP differences are dominated by random errors, while reflectances show systematic difference larger than the random noise at 410 and 470 nm. The systematic difference in reflectance also poses challenges in atmospheric correction for SPEX airborne in the wavelengths between 410 and 470 nm. Polarization information shows a higher level of agreement between the two sensors and therefore should be more strongly weighted in the retrieval algorithm.
The MAPOL algorithm is designed to jointly retrieve the aerosol and water-leaving signals from MAP data, which have been validated with the synthetic simulated data (Gao et al., 2018) as well as the RSP measurements from field campaigns (Gao et al., 2019). The retrieval algorithm minimizes the difference between the MAP measurements and the forward model simulations computed from a vector radiative transfer forward model (Zhai et al., 2009, 2010). Two ocean bio-optical models are implemented in MAPOL: one with chlorophyll-a concentration as the single retrieval parameter applicable to open-ocean optical properties and the other with seven parameters applicable to complex coastal waters (Gao et al., 2019). In this study we perform retrievals near the USC_SEAPRISM site where waters are mostly clear so that the bio-optical model parameterized by chlorophyll-a concentration is used.
In the MAPOL algorithm used in this study, the aerosol size distribution is composed by five submodes, each with a lognormal distribution with fixed mean radius and variance (Dubovik et al., 2006; Xu et al., 2016, 2017). The first three submodes (median radii of 0.1, 0.1732, and 0.3 µm) represent the fine-mode aerosols, and the last two submodes (median radii of 1.0 and 2.9 µm) represent the coarse-mode aerosols. For a general study, Fu and Hasekamp discussed the representation of aerosol size distribution through various numbers of submodes and found that a similar five-mode approach can provide good retrievals for most aerosol parameters (Fu and Hasekamp, 2018). The aerosol refractive index spectra for both fine and coarse modes are approximated as , where m0 and α1 are fitting parameters, and p1(λ) is the first order of the principal components, computed from the dataset derived from Shettle and Fenn (1979), including spectral refractive indices of water, dust-like, biomass burning, industrial, soot, sulfate, water-soluble, and sea salt aerosols (d'Almeida et al., 1991; Wu et al., 2015). There are two sets of m0 and α1 for the real and imaginary refractive index spectra, respectively, for both fine and coarse modes. This means that there are a total of eight parameters for the refractive indices. In summary, the retrieval parameters include 5 volume densities (one for each submode), 8 parameters for the refractive indices of fine and coarse modes, 1 parameter for wind speed, and the chlorophyll-a concentration, with a total of 15 parameters. After the aerosol properties are retrieved from the discrete bands of the RSP data, the same parameters m0 and α1 are used in the aforementioned refractive index spectral representation to calculate the aerosol refractive indices at the SPEX airborne wavelengths, which are then used in the radiative transfer model to compute the contribution of aerosols at the corresponding wavelengths.
The Stokes parameters, Lt, Qt, and Ut, from RSP measurements are used to define the total measured reflectance and total DoLP , where F0 is the extraterrestrial solar irradiance, μ0 is the cosine of the solar zenith angle, and r is the Sun–Earth distance in astronomical units. Circular polarization (Stokes parameter V), not measured by any of the polarimeters in ACEPOL, is often ignored for atmospheric studies (Kawata, 1978). The cost function is used to quantify the difference between the measurement and the forward model simulation and is defined as
where and denote the total reflectance and DoLP simulated from the forward model, the state vector x contains retrieval parameters for aerosols and ocean, subscript i stands for the indices of the measurements at different viewing angles and wavelengths, and N is the total number of the measurements used in the retrieval. All RSP bands are used in our retrievals except for the two water vapor absorption bands at 960 and 1880 nm. The total uncertainties of the reflectance and DoLP used in the algorithm are denoted as σt and σP, which includes three components: the instrument measurement uncertainties as summarized in Knobelspiesse et al. (2019) (e.g. absolute radiometric and DoLP uncertainty averaged to 3 % and 0.002 for the RSP instrument used in ACEPOL), the variance from averaging nearby RSP pixels (the average of 5 consecutive pixels are used in this study, which corresponds to a surface pixel size of approximately 1 km), and the forward model uncertainties estimated as 0.015 and 0.002 for the radiometric and polarimetric uncertainties, respectively (Gao et al., 2019). All these uncertainties are added in the quadrature of σt and σP in Eq. (1) to represent the total uncertainties. The weight of the measurements in the cost function depends on the inverse square of σt and σP. As will be discussed shortly, there is higher weight on the DoLP than reflectance in the cost function. Because DoLP has less dependency on the noise correlation between angles due to its definition as a ratio of two observations (Knobelspiesse et al., 2012), the noise correlation has been ignored in this study.
The relative uncertainties for reflectance and DoLP, denoted as σt∕ρt and σP∕Pt, are defined as the ratio of the total uncertainties over the measurement and summarized in Table 2. The magnitude of uncertainties often depends on the viewing angles as shown in the panels (c) and (d) of Figs. 2 and 3. Table 2 shows the minimum value among the viewing angles at each band, which corresponds to the largest weight in Eq. (1) for the corresponding band. The value of σt∕ρt is 3.4 % from 410 to 865 nm and around 4 % to 5 % for the SWIR bands. σP∕Pt is between 0.4 % and 1.8 % for the bands from 410 to 865 nm, and for the SWIR bands, σP∕Pt is between 6.1 % and 15.1 %. The percentage uncertainties of the polarizations in the two SWIR bands further increase when the DoLP value decreases. We have tested the effects of the DoLP at the two SWIR bands on the aerosol retrieval and found that including them does not improve the retrieval accuracies, so the SWIR DoLPs are not used in our retrievals. Moreover, the PACE MAPs do not include polarimetric SWIR measurements, but the PACE OCI includes several SWIR bands measured at a single viewing angle and may have higher accuracy; a synergy of PACE OCI SWIR with MAP measurements may further improve aerosol retrievals. In summary we use seven bands of ρt and five bands of Pt in our retrievals, and the corresponding cost function is denoted as 7ρt+5Pt. In order to understand the impacts of the polarization information, we also conducted the retrievals with only reflectance in the cost function, which is denoted as 7ρt.
Table 2The minimum relative uncertainties of reflectance (ρt) and DoLP (Pt) for the RSP bands. The SWIR DoLPs denoted by asterisks are not used in the retrievals.
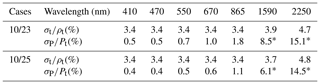
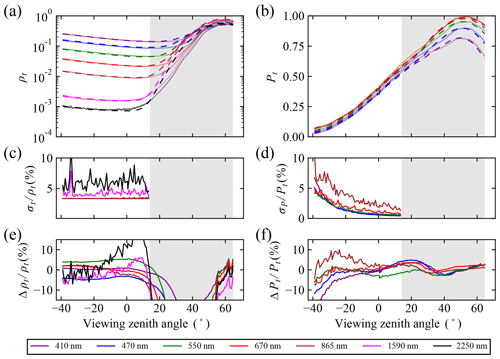
Figure 2(a, b) The comparison of the RSP measurement and model fitting of reflectance ρt and DoLP Pt for case 10/23; (c) and (d) are the total percentage uncertainties relative to the measurements for reflectance (100σt∕ρt) and DoLP (100σP∕Pt); (e) and (f) are the percentage residuals between the measurements and fittings relative to the measurements for reflectance (100Δρt∕ρt) and DoLP (100ΔPt∕Pt). The solid line in (a) and (b) is the measurement data and the dashed line is the simulation results from the retrieval. The shaded area indicates the angles not used in the retrieval (uncertainties are not calculated).
The use of oceanic sunglint from satellite measurements has been proposed and demonstrated to help aerosol absorption retrievals (Kaufman et al., 2002; Ottaviani et al., 2013). However, we tested the retrievals with the sunglint data included for case 10/23 (as shown in Fig. 2) and found that the sunglint reflection cannot be modeled well using the isotropic Cox–Munk model (Cox and Munk, 1954), which depends on the wind speed only. This may be due to the high spatial resolution of 200 m from RSP measurement or the insufficient representation with a scalar wind speed. Therefore, the sunglint region for only case 10/23 is removed within an angle of 40∘ around the specular reflection direction of the direct solar light as indicated in Fig. 3. There are no data removed by the glint mask for case 10/25 due to its cross-principal plane geometry. The remaining range of scattering angles used in the retrieval is shown in Table 1.
The retrieved aerosol properties will be compared and validated with the AERONET products in the next section and then used to conduct atmospheric correction for both RSP and SPEX airborne measurement. The resultant water-leaving signals as represented by the remote sensing reflectance can be computed using the water-leaving reflectance reaching the sensor as
where td is the downward transmittance of the solar irradiance to the surface, and tu is the upward transmittance of the water-leaving radiance to the sensor (Gao et al., 2019). The water-leaving reflectance represents the signals originating from scattering in the ocean that reached the sensor and can be derived from the atmospheric correction process as
where is the reflectance contribution of the atmosphere and ocean surface at the sensor (Mobley et al., 2016; Gao et al., 2019). In order to compare the water-leaving signals measured at different times derived from RSP, MODIS, and AERONET instruments, the directional dependence of the water-leaving reflectance is removed to obtain the signal at the nadir direction (Mobley et al., 2016).
After obtaining the aerosol properties from RSP retrievals, the atmospheric contributions including all the scattering and absorption process related to the aerosols, molecules, and ocean surface, and the td and tu transmittance are computed for the hyperspectral SPEX spectral bands with the vector radiative transfer model by Zhai et al. (2009, 2010, 2018) and subtracted from the hyperspectral SPEX airborne measurement. The gas absorption in the radiative transfer simulation, including contributions from ozone, oxygen, water vapor, nitrogen dioxide, methane, and carbon dioxide, is accounted for by using the US standard atmospheric constituent profiles (Anderson et al., 1986) but with a scaled amount of water vapor, ozone, and oxygen. The total ozone column density used to scale the ozone profile is obtained from the Modern-Era Retrospective analysis for Research and Applications, Version 2 (MERRA-2) from NASA's Global Modeling and Assimilation Office (Gelaro et al., 2017).
We then simulated the reflectance spectra under SPEX geometries with the retrieved aerosol properties and various amounts of oxygen and water vapor. The simulated spectra are compared with SPEX airborne measurement, and the best amounts of water vapor and oxygen are chosen to minimize the difference between the measurements and simulations. During this process the aerosol properties and ozone density are kept unchanged. The hyperspectral variations of gas absorption are incorporated in the radiative transfer simulation using a method similar to the double-k method (Zhai et al., 2018).
The MAPOL retrieval algorithm was applied to the RSP measurements for the cases 10/23 and 10/25. To evaluate the retrieval stability and uncertainties, 150 sets of random initial guesses for all the 15 retrieval parameters were used for the cost functions of both 7ρt+5Pt and 7ρt. Each parameter was varied within a boundary as specified in Gao et al. (2018, 2019), where the wind speed is less than 10 m s−1 and the chlorophyll-a concentration is less than 30 mg m−3, the aerosol refractive index varies effectively between 1.3 and 1.6 in its real part and between 0 and 0.03 in its imaginary part, and random mixing fractions of the five aerosol volume densities are constrained by a maximum total AOD of 0.3. The minimum cost function value for case 10/23 and 10/25 is 2.8 and 3.8 with the cost function of 7ρt+5Pt and reduced to 0.6 and 0.4 with the cost function of 7ρt (polarization information not considered, all else the same). The minimum cost function value corresponds to the best aerosol retrievals, and the remaining residuals relate to the measurements which cannot be completely represented by the forward models.
Using the best aerosol retrievals corresponding to for 7ρt+5Pt, the reflectance and DoLP are simulated and compared with the measurements as shown in Figs. 2 and 3, where the viewing zenith angles are the same as defined in Fig. 1b with the positive sign referring to the glint side ( or ) and the negative sign referring to the other hemisphere containing the antisolar point. The solid line indicates the measurement data and the dashed line indicates the simulation results using the retrieved aerosol and ocean properties for the reflectance (ρt) and DoLP (Pt) as plotted in panels (a) and (b). The total uncertainties as discussed in Sect. 2 are plotted in panels (c) and (d) for the reflectance and DoLP respectively. The percentage difference between measurements and fittings is plotted in panels (e) and (f). For case 10/23, the viewing directions are close to the principal plane. The sunglint is indicated by the shaded area in Fig. 2, which was excluded in the retrieval as discussed in Sect. 3. Using the retrieved aerosol properties, the reflectance and DoLP were also computed in the glint region. Although both the reflectance and DoLP measurements in the glint region were not considered in this study, the comparison of the measurement and simulated results indicates a better agreement of the DoLP than the reflectance, which can be explained by the fact that the DoLP from reflection on the ocean surface does not depend on the assumed distribution of surface slopes. For the remaining angles, the absolute residuals larger than 10 % in Fig. 2c and d are mostly associated with the small values of the measurement, such as the SWIR bands for ρt and the DoLP at viewing angles less than . On average, the residuals for both reflectance and DoLP are smaller than 6 %. For case 10/25, the viewing angles are almost perpendicular to the principal plane, the DoLP is always larger than 0.3, and the residuals for DoLP are even smaller than the previous case with a value less than 2 % on average. For the SWIR bands at the viewing angles between −10 and 20∘, Fig. 3e shows residuals larger than 10 % at 1590 and 2250 nm, which indicates the measurements cannot be modeled by the forward model. However, the difference is not observed in the DoLP comparisons in Fig. 3f. Due to the presence of invalid measurements in the 2250 nm band between −10 and 20∘, some measurement uncertainties in this portion are not computed as shown in Fig. 3c, and the corresponding measurements are not counted in the cost function.
The histogram and cumulative probability of the cost functions for the 150 converged cases with random initial values are compared in Fig. 4. For the cost function 7ρt more than 50 % of cases are converged within a cost function value of 2 (the residual is times the measurement uncertainty), while to have the same 50 % of cases converged for 7ρt+5Pt, the cost function value needs to be within 6. The wider spread of the cost function values is related to the higher sensitivity of DoLP in the cost function, which is consistent with Table 2 showing that the DoLP has much smaller uncertainties. In order to evaluate the uncertainties of the best retrieval results, we consider the retrieval cases to be converged within a cutoff cost function value of and compute the standard deviation of all the aerosol properties and water-leaving signals retrieved from these cases. This corresponds to the evaluation using all the retrieval cases converged within the cost function range of [, ]. A total of 50 % of the retrievals on average converged within this cost function range for 7ρt, while 30 % of all the retrievals converged within this requirement for 7ρt+5Pt. More discussion on the cutoff cost function value is in the next section. A comparison of the results with 75 and 150 cases demonstrated that the results are converged with enough samples to compute the standard deviation. The uncertainties due to the impact of the initial values are in addition to the uncertainties from the error propagation method, where the measurement uncertainties are propagated to the retrieval parameters through the Jacobian matrix (e.g. Knobelspiesse et al., 2012). A further discussion in the next section suggests that the uncertainties evaluated using these two methods may also relate to each other.
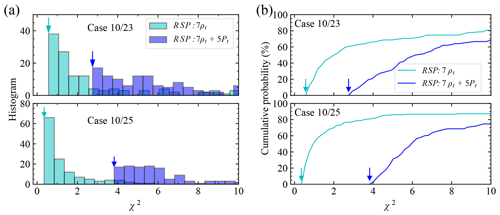
Figure 4(a) The histogram for case 10/23 and 10/25 with cost function 7ρt and 7ρt+5Pt and a bin size of 0.5; (b) the corresponding cumulative probability. The arrows indicate the minimum cost function values .
4.1 Aerosol microphysical properties
We compared the retrieved aerosol size distribution, fine-mode refractive index, fine-mode SSA, and total AOD with the averaged AERONET product on the same day in Fig. 5. The aerosol size distribution is represented by the volume density as a function of radius in the logarithmic scale. Both the shaded area and the error bar indicate 1σ uncertainty. The retrieval values and uncertainties are summarized in Table 3, which includes the wind speed, refractive index, SSA, and AOD for both fine and coarse modes, as well as remote sensing reflectance. The overall SSA for the two-mode mixture is computed as the ratio of the number-density-weighted averages for the scattering and extinction cross sections (Bohren and Huffman, 1998).
For case 10/23, the retrieved wind speed for both cost functions is 3.4–3.5 m s−1, with similar uncertainties. For 10/25, a wind speed of 5.4 m s−1 is retrieved under 7ρt+5Pt, which is 1.5 m s−1 faster than the results from 7ρt and with the uncertainty reduced by a factor of 2. Due to the exclusion of the glint in the retrieval for case 10/23 and the cross-principal plane geometry for case 10/25, the wind speed is not ideal for retrieval. However, the retrieval uncertainties are within 0.5 m s−1 for both cases with 7ρt+5Pt, which suggests that the measurements used in the retrievals are still influenced by wind speed.
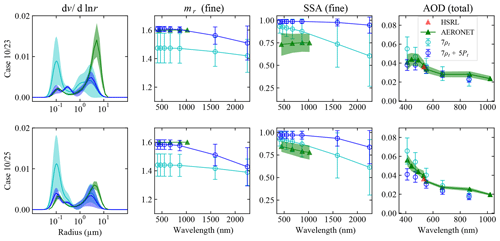
Figure 5The aerosol size distribution (dv∕dln r), fine-mode refractive index (mr), fine-mode SSA, and total AOD of the two cases on 10/23 and 10/25 retrieved with the cost functions of 7ρt+5Pt and 7ρt. The vertical widths for the aerosol size distribution and the error bars for other aerosol properties of the retrieved results indicate 1σ retrieval uncertainties. The results from the AERONET product are plotted in green, and the vertical width indicates its daily variation. The HSRL AOD at 532 nm is indicated by the red triangle.
Table 3The averaged retrieval values and uncertainties (in parentheses) from the cases converged within the range of cost function [, ] for the fine- and coarse-mode effective radius (reff), effective variance (veff), refractive index (mr), SSA, as well as total AOD and SSA, and remote sensing reflectance (Rrs). AOD, SSA, and mr are all at 550 nm, and Rrs is at both 470 and 550 nm.
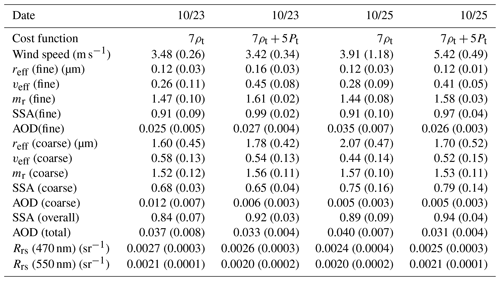
Figure 5 shows that the aerosol size distribution retrieved with the polarized information 7ρt+5Pt is closer to the AERONET results than that of the reflectance-only retrieval (7ρt): the maximum fine-mode volume density reduced by 4 and 2.5 times for case 10/23 and 10/25, and the uncertainties reduced by 5 times for both cases. The fine-mode effective radii of 7ρt and 7ρt+5Pt are similar for each, but the effective variances become larger when DoLP observations are included with an increase of around 0.1 to 0.2, as shown in Table 3. An increase of the fine-mode effective variances can also be observed in Fig. 5 with a wider fine-mode distribution. To compensate for the much smaller fine-mode density from the retrievals with 7ρt+5Pt, the retrieved fine-mode refractive indices increase from 1.47 to 1.61 and from 1.44 to 1.58, for cases 10/23 and 10/25 respectively. To compare with the AERONET results which assume that both fine and coarse modes have the same refractive index, we define the volume-averaged refractive index as (fine)+ (coarse), where fv is the fine-mode volume fraction (Hasekamp et al., 2011; Gao et al., 2018). For cases 10/23 and 10/25 with 7ρt, mv is 1.49 and 1.48 respectively. While with 7ρt+5Pt mv becomes 1.58 and 1.56 for these 2 d, which agrees better with the AERONET refractive index of 1.6.
Meanwhile, a larger fine-mode SSA is also retrieved with the polarization information (from 0.91 to 0.99 for case 10/23 and from 0.91 to 0.97 for case 10/25), which suggests the aerosols have almost no absorption. The coarse-mode SSAs are 0.7–0.8 for both days and both cost function options. The AERONET SSAs at 550 nm are around 0.8 (with a daily variation of 0.1), which is smaller than the retrieved overall SSA at 550nm with a value of 0.92 for case 10/23 and 0.94 for case 10/25 with 7ρt+5Pt. For AOD comparisons, the HSRL AODs are 0.036 at 532 nm for both case 10/23 and 10/25; the AERONET AODs at the same wavelength are 0.038 and 0.040 respectively and become 0.034 at 550 nm. These AOD results agree well with the retrieved AODs at 550 nm (0.033 for case 10/23 and 0.031 for case 10/25). The AERONET AOD spectra are in good agreement with the RSP AOD spectra in terms of both shape and magnitude for case 10/23 but are slightly larger than that of case 10/25 with a difference of 0.011 at 410 nm.
When including polarization in the retrievals, the fine-mode retrieval uncertainties become 0.02–0.03 for refractive index, 0.02–0.04 for SSA, and 0.004 for AOD, with most values reduced by more than one-half. The uncertainties for most coarse-mode properties remain with similar magnitudes. However, the small aerosol signals in our cases can be easily influenced by other environmental factors not modeled by the forward model; more case studies are required to evaluate the overall agreement and bias.
4.2 Water-leaving reflectance
The aerosol properties retrieved with the best-fit results using 7ρt+5Pt were applied to the atmospheric correction of the hyperspectral measurements from SPEX airborne for both case 10/23 and 10/25 using the methods discussed in Sect. 3. The retrieval uncertainties of the water-leaving reflectance for the RSP Rrs were computed similarly to that for the aerosol properties. The results are compared with the MODIS OC products and the SeaPRISM measurements from AERONET OC in Fig. 6. The AERONET and MODIS OC agree well with each other for case 10/25; for case 10/23 MODIS Rrs is lower than AERONET Rrs by a value of 0.001 sr−1 at 470 nm. The uncertainties from MODIS OC and AERONET OC data as defined in Sect. 2 are smaller than the RSP retrieval results, which indicate the small temporal and spatial variations of the water-leaving reflectance, and therefore their results can be used to compare with the RSP retrievals at a slightly different time and location (Table 1).
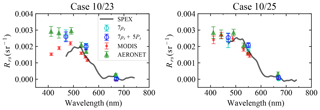
Figure 6The remote sensing reflectance, Rrs, from MODIS OC, AERONET OC, and the atmospheric corrections for RSP and SPEX airborne for case 10/23 and 10/25. The error bars for the RSP-retrieved results with cost functions of 7ρt and 7ρt+5Pt indicate 1σ retrieval uncertainties. SPEX airborne atmospheric correction uses the same RSP-retrieved aerosol models and therefore shares the same retrieval uncertainties (not indicated in plot). The error bar for the AERONET OC Rrs indicates its daily variation.
The RSP 410 nm band is excluded from comparison due to the observation of a 4 % decrease in its radiometric throughput, while other RSP bands remain stable within ∼1 % in the radiometric calibration and ∼0.1 % in the polarimetric calibration (Knobelspiesse et al., 2020). The SPEX Rrs at wavelengths shorter than 470 nm is not compared because of the observed absolute systematic difference with the RSP 410 and 470 nm bands as discussed in Sect. 2. The retrieved RSP Rrs using the aerosol properties from 7ρt+5Pt and 7ρt shows similar values and uncertainties for both case 10/23 and 10/25. The following discussions refer to RSP Rrs results with cost function 7ρt+5Pt. RSP Rrs shows good agreements with AERONET Rrs for both cases. As shown in Table 3, the RSP Rrs values at 470 and 550 nm are 0.0026 and 0.0020 respectively for case 10/23 and 0.0025 and 0.0021 respectively for case 10/25. For AERONET Rrs, the values at 442, 490, and 550 nm are 0.0027, 0.0028, and 0.0017 sr−1 for case 10/23 and 0.0028, 0.0029, and 0.0017 sr−1 for case 10/25. Using the interpolated value of AERONET Rrs at RSP bands, the differences between RSP and AERONET Rrs are within 0.0003 sr−1.
When comparing Rrs from SPEX airborne, RSP, MODIS OC, and AERONET OC, case 10/25 shows good agreements. SPEX Rrs values at 470 and 550 nm are 0.0022 and 0.0017 sr−1 with a difference between AERONET and RSP Rrs of less than 0.0004 sr−1. For case 10/23, the SPEX Rrs spectrum is consistent with the AERONET and RSP results for the wavelengths longer than 500 nm, while Rrs from SPEX is smaller than both AERONET and RSP at shorter wavelengths. At 470 nm, the SPEX Rrs is 0.0014 sr−1, and the MODIS Rrs is between that of RSP and SPEX Rrs. The maximum difference between SPEX Rrs and the AERONET Rrs is around 0.001 sr−1 at 470 nm, which is larger than the retrieval uncertainty of 0.0003 sr−1 as shown in Table 3. Note that the PACE requirement on the water-leaving reflectance between 400 and 600 nm is 0.002 or 5 % (Werdell et al., 2019), which corresponds to 0.0006 sr−1 in Rrs. The Rrs retrieval uncertainties and difference between RSP and AERONET Rrs are within this requirement, but the difference between SPEX and AERONET Rrs for case 10/23 is larger than the requirement. The larger difference of RSP, SPEX, and MODIS Rrs at wavelengths smaller than 500 nm may be related to the measurement uncertainties where the reflectances are larger at shorter wavelengths. Another possible reason for the discrepancy between the MODIS Rrs and others is the different aerosol models used for atmospheric correction; for MODIS, it is determined from the two NIR bands of 748 and 869 nm, while others are based on polarimeter retrievals.
As shown in Fig. 6, for both case 10/23 and 10/25, there are two small dips between 600 and 700 nm in the remote sensing reflectance which are related to the oxygen absorption bands that peaked at 688 nm (B band) and 629 nm (γ band). It is challenging to correct for the impacts from the strong gas absorption due to interaction between multiple scattering and gas absorption. Further improvement requires knowledge of aerosol height and the exact instrument line shape function.
Accurate determination of water-leaving signals is key to derive ocean water optical properties and ocean biogeochemical conditions. In this work we have shown an example of how information-rich observations of the atmosphere (from a MAP) can be used to perform an atmospheric correction on highly spectrally resolved measurements of the ocean. As discussed in Sect. 2, reflectance measured by RSP is larger than SPEX airborne measurement by a systematic difference of 4 % and 3 % at 410 and 470 nm respectively. The impact on the aerosol retrievals is likely mitigated by relying more on the polarization information with much smaller uncertainties than reflectance and better agreement between RSP and SPEX airborne measurements, but the computation of the water-leaving signals cannot avoid the bias in reflectance. The atmospheric correction process requires the subtraction of the total measured reflectance by the simulated contributions from atmosphere and ocean surface. Therefore, the uncertainties and bias in the measurements can directly impact the water-leaving signal retrievals.
The reflectances ρt measured by RSP at 410 and 470 nm are 0.15 and 0.09, respectively. Based on the definition of Rrs, the 4 % and 3 % systematic difference in reflectance will transfer into a large Rrs biases around 0.002 and 0.0009 sr−1. Therefore the Rrs values from both RSP and SPEX airborne with wavelengths less than 470 nm are not compared. The random difference between RSP and SPEX measurements at 470 nm band is 2 % as discussed by Smit et al. (2019), which can transfer to 0.0006 sr−1 in Rrs. The differences of the Rrs from RSP and SPEX at 470 nm (0.0012 for case 10/23 and 0.0003 for case 10/25 with 7ρt+5Pt) may be due to the combined effects of the random and systematic differences in their measurements.
Accurate retrieval of Rrs requires a higher level of measurement accuracy, especially for the shorter wavelengths where the total reflectance is more dominated by the contribution of Rayleigh scattering. To remove the bias in measurement and improve atmospheric corrections, vicarious correction techniques using ground-based measurements have been developed for ocean color radiometry (Franz et al., 2007) and have also been applied to hyperspectral measurements (Ibrahim et al., 2018). Cross calibration between a MAP and hyperspectral radiometer will be critical for combining their results together for the purpose of ocean color remote sensing. Challenges in the measurement accuracy for ocean color observations will be addressed in the PACE mission, where the hyperspectral OCI measurement will achieve high calibration accuracies through prelaunch calibration campaigns, on-orbit gain adjustment through solar diffuser and lunar measurements, and on-orbit vicarious calibration (Werdell et al., 2019). On-orbit MAP cross calibration with OCI will be possible – for example, measurements at the viewing angle of SPEXone are expected to be cross calibrated with OCI, transferring the high radiometric accuracy from OCI to SPEXone (Werdell et al., 2019)
Aerosol microphysical properties are important to compute their optical properties and conduct atmospheric correction. As shown in Table 3, the retrieved Rrs for both cases 10/23 and 10/25 is not sensitive to the exact aerosol microphysical properties. Both 7ρt and 7ρt+5Pt produce similar Rrs and small relative uncertainties of 0.0003–0.0004 sr−1 at 470 nm. This is due to the small aerosol loading, the relatively simple aerosol microphysical properties with almost no absorption, and the flat spectral dependency of the refractive index in the visible bands. Meanwhile, we have shown polarization information can help to reduce retrieval uncertainties in the retrieval of aerosol optical depth, fine-mode refractive index, and SSA as shown in Table 3, but the retrieval accuracies are limited by the low AOD. Besides the theoretical retrieval accuracy analysis, validations with direct measurements are important to account for unknown uncertainties. The AOD results from polarimetric retrievals can be validated with ground-based measurements such as AERONET and lidar measurements such as HSRL; however, it is challenging to validate the complex aerosol refractive index, SSA, and size distribution for the entire atmospheric column due to the lack of direct measurements. Such a validation requires well-planned airborne field campaigns, concepts for which are under development (Plankton, Aerosol, Cloud, ocean Ecosystem (PACE) mission, 2020). The reduced retrieval uncertainties from polarimetric retrievals in the aerosol microphysical properties can potentially help to determine aerosol type and its composition when there is sufficient aerosol loading and therefore provide valuable information in the study of aerosol deposition to the ocean and its impact on the ocean ecosystem and, potentially, the role of the ocean in aerosol formation.
Furthermore, the retrieval uncertainties evaluated in this study are for the retrievals converged within a range of cost function value [, ]. This approach provides a statistical evaluation of the uncertainties relating to the cost function sensitivity and impact of initial values. When considering [, ], 50 % of data considered for 7ρt+5Pt and 80 % for 7ρt fall in this range as shown in Fig. 4b, which lead to the uncertainties of the fine-mode refractive index increasing to 0.04–0.05 for 7ρt+5Pt, which is still smaller than the uncertainties of 7ρt with a value around 0.08–0.1 (Fig. 7). The choice of cutoff cost function value used for retrievals in practice would depend on the accuracy requirement and the algorithm to determine the initial values. The study by Knobelspiesse et al. (2012) estimated retrieval uncertainties using the error propagation method for the Aerosol Polarimetry Sensor (similar to RSP characteristics used in this study, although they used SWIR polarization) with various optical depths and aerosol types. The solar zenith and azimuth angles used by Knobelspiesse et al. (2012) are both 45∘, which is similar to the solar zenith angles used in this study and between the two solar azimuth angles (8.7 and 94.6∘) as shown in Table 1. Their results showed the uncertainties for the fine-mode refractive index, SSA, and total AOD to be 0.015, 0.02, and 0.005 when AOD =0.039. The corresponding uncertainties evaluated in this study with a similar AOD are 0.02–0.03, 0.02–0.04, and 0.004 for fine-mode refractive index, SSA, and total AOD as shown in Table 3.
The uncertainty results computed from two different approaches are comparable to each other in magnitudes. The error propagation method directly relates the retrieval uncertainties to the measurement uncertainties by projecting them from measurement to state space using Jacobians calculated from the radiative transfer model. This method accurately represents retrieval uncertainty if (1) the measurement uncertainty is correct, (2) the forward model is an accurate and complete representation of physical reality, (3) the state space is locally linear about the retrieval, and (4) the retrieval algorithm is able to successfully converge to the smallest cost function value without artifacts. In practice, (2) is nearly always approximate, and (3) and (4) are not always the case, so the methodology used in Knobelspiesse et al. (2012) can be considered the best case retrieval uncertainty. It is, however, a convenient metric for retrieval uncertainty estimation since Jacobians are often calculated as part of the retrieval process and can be reused for this purpose. The retrieval uncertainty method used in this work (expressing the volume in state space containing cost function values calculated with many retrievals performed using randomly generated initial values) is similar in some respects, as it also relies on an accurate measurement uncertainty model (1) and forward model (2). However, it may be more accurate in some cases, since it does not make the assumption of local linearity (3) and can incorporate some potential convergence artifacts (4). Because this technique requires execution of many retrievals to have a sufficient distribution of the cost functions, it is computationally inefficient for operational use. It is, however, very relevant for a (data) limited study such as this, as it provides more realistic uncertainty estimate.
We have retrieved aerosol properties using multiangle polarimetric measurement from RSP in the ACEPOL field campaign over the AERONET USC_SEAPRISM site. The aerosol properties are then applied to the hyperspectral SPEX airborne measurement to compute water-leaving signals. This is a proof of concept for the application of MAP data on PACE to the atmospheric correction of the OCI spectrometer on the same mission. We demonstrated the improved accuracy when combining the reflectance and polarization in the retrievals compared to reflectance-only retrievals. After adding DoLP in the retrieval cost functions, the uncertainties for aerosol refractive index, SSA, and AOD are mostly reduced by a factor of 2 for the two cases considered in the study. The absolute values also agree better with the AERONET aerosol product except SSA probably due to the large uncertainty of SSA from both the MAP and AERONET inversions at low AOD. Moreover, the higher accuracy in DoLP measurements introduces larger weights of DoLP in the cost function relative to the reflectance measurement, and this therefore provides resilience to the uncertainties and bias in the reflectance measurements and produces a higher aerosol retrieval quality.
In order to apply the retrieved aerosol properties from the MAP measurements to hyperspectral atmospheric correction, the principal components of the aerosol refractive index spectra are interpolated into the bands specified for SPEX airborne. The retrieval uncertainties on RSP Rrs are within 0.0004 sr−1 (same to SPEX Rrs), while the comparison of the two cases with the AERONET Rrs shows a difference less than 0.0003 sr−1 for RSP Rrs and a maximum difference of 0.0004 sr−1 (case 10/25) and 0.001 sr−1 (case 10/23) for SPEX Rrs. The difference of SPEX Rrs for case 10/23 is larger than the retrieval uncertainties, which is likely due to the radiometric uncertainties from the sensors. In the context of the PACE mission, the aerosol properties can be retrieved from the two PACE MAPs – SPEXone and HARP2 – and applied to the hyperspectral measurement from the OCI which has a much higher radiometric accuracy (although SPEXone and HARP2 have different characteristics than RSP). Although the hyperspectral atmospheric correction for wavelength less than 470 nm cannot be demonstrated by the SPEX airborne data in this study, the PACE OCI will provide high-quality hyperspectral measurement from 340 to 890 nm and a few SWIR bands, and the demonstration of the atmospheric correction including UV spectral range will require future studies. With the MAPs and OCI on PACE, both aerosol microphysical properties and hyperspectral ocean color signals will be obtained simultaneously with a global coverage and the knowledge will help the study, monitoring and protection of the ocean ecosystem.
The data files for RSP, SPEX airborne, and HSRL-2 used in this study are listed below. The RSP data are available from the NASA GISS website https://data.giss.nasa.gov/pub/rsp (NASA RSP Data Archive, 2020). The SPEX airborne L1C data are provided by the SPEX team. The HSRL-2 files are available from the ACEPOL website (https://www-air.larc.nasa.gov/cgi-bin/ArcView/acepol, ACEPOL team, 2020).
-
Case 10/23
RSP: RSP2-ER2_L1B-RSPGEOL1B-GeolocatedRadiances_20171023T210750Z_V001-20171024T034314Z.h5.
SPEX: L1C-20171023_211047_150-213119_220_1000m_radiance.h5.
HSRL-2: ACEPOL-HSRL2_ER2_20171023_R1.h5. -
Case 10/25
RSP: RSP2-ER2_L1B-RSPGEOL1B-GeolocatedRadiances_20171025T204909Z_V001-20171026T030529Z.h5.
SPEX: L1C-20171025_204857_50-210929_170_1000m_radiance.h5.
HSRL-2: ACEPOL-HSRL2_ER2_20171025_R1.h5.
MG generated the scientific data used in this paper and wrote the original manuscript. P-WZ and BAF formulated the original concept for this study. MG and P-WZ developed the retrieval algorithm. P-WZ developed the hyperspectral radiative transfer code used in this study. KK and BC advised on the retrieval uncertainty evaluation and aerosol typing. AI, BAF, and YH advised on the atmospheric correction. SEC advised on the aerosol interaction with the ocean. PJW advised on the PACE instruments. OH and GF provided and advised on the SPEX airborne data. All authors participated the writing and editing of this paper.
The authors declare that they have no conflict of interest.
The authors would like to thank the ACEPOL team for conducting the field campaign and providing the data, as well as the Oregon State University and the University of Southern California teams for maintaining the AERONET USC_SEAPRISM site. Meng Gao would like to thank Ivona Cetinić, Chris Proctor, Snorre Stamnes, and Minwei Zhang for constructive discussions.
Funding for the ACEPOL field campaign came from NASA (ACE and CALIPSO missions) and SRON. Part of this work has been funded by the NWO/NSO project ACEPOL (project no. ALW-GO/16-09). The ACEPOL campaign has been supported by the Radiation Sciences Program. Meng Gao, Bryan A. Franz, Brian Cairns, Kirk Knobelspiesse, Amir Ibrahim, Susanne E. Craig, and P. Jeremy Werdell have been supported by the NASA PACE project. Peng-Wang Zhai has been supported by NASA (grant no. 80NSSC18K0345).
This paper was edited by Alexander Kokhanovsky and reviewed by three anonymous referees.
Ahmad, Z., Franz, B. A., McClain, C. R., Kwiatkowska, E. J., Werdell, J., Shettle, E. P., and Holben, B. N.: New aerosol models for the retrieval of aerosol optical thickness and normalized water-leaving radiances from the SeaWiFS and MODIS sensors over coastal regions and open oceans, Appl. Optics, 49, 5545–5560, 2010. a
Anderson, G., Clough, S., Kneizys, F., Chetwynd, J., and Shettle, E.: AFGL Atmospheric Constituent Profiles (0.120 km), Air Force Geophysics Lab., Hanscom AFB, MA, USA, p. 46, 1986. a
Bohren, C. F. and Huffman, D. R.: Absorption and Scattering of Light by Small Particles, WILEY‐VCH Verlag GmbH & Co. KGaA, 1998. a
Burton, S. P., Hair, J. W., Kahnert, M., Ferrare, R. A., Hostetler, C. A., Cook, A. L., Harper, D. B., Berkoff, T. A., Seaman, S. T., Collins, J. E., Fenn, M. A., and Rogers, R. R.: Observations of the spectral dependence of linear particle depolarization ratio of aerosols using NASA Langley airborne High Spectral Resolution Lidar, Atmos. Chem. Phys., 15, 13453–13473, https://doi.org/10.5194/acp-15-13453-2015, 2015. a
Cairns, B., Russell, E. E., and Travis, L. D.: Research Scanning Polarimeter: calibration and ground-based measurements, Proc. SPIE, 3754, 186–196, https://doi.org/10.1117/12.366329, 1999. a, b
Carr, M.-E., Friedrichs, M. A., Schmeltz, M., Aita, M. N., Antoine, D., Arrigo, K. R., Asanuma, I., Aumont, O., Barber, R., Behrenfeld, M., Bidigare, R., Buitenhuis, E. T., Campbell, J., Ciotti, A., Dierssen, H., Dowell, M., Dunne, J., Esaias, W., Gentili, B., Gregg, W., Groom, S., Hoepffner, N., Ishizaka, J., Kameda, T., Quéré, C. L., Lohrenz, S., Marra, J., Mélin, F., Moore, K., Morel, A., Reddy, T. E., Ryan, J., Scardi, M., Smyth, T., Turpie, K., Tilstone, G., Waters, K., and Yamanaka, Y.: A comparison of global estimates of marine primary production from ocean color, Deep-Sea Res. Pt. II, 53, 741–770, https://doi.org/10.1016/j.dsr2.2006.01.028, 2006. a
Chowdhary, J., Cairns, B., Mishchenko, M., and Travis, L.: Retrieval of aerosol properties over the ocean using multispectral and multiangle Photopolarimetric measurements from the Research Scanning Polarimeter, Geophys. Res. Lett., 28, 243–246, https://doi.org/10.1029/2000GL011783, 2001. a
Chowdhary, J., Cairns, B., Mishchenko, M. I., Hobbs, P. V., Cota, G. F., Redemann, J., Rutledge, K., Holben, B. N., and Russell, E.: Retrieval of Aerosol Scattering and Absorption Properties from Photopolarimetric Observations over the Ocean during the CLAMS Experiment, J. Atmos. Sci., 62, 1093–1117, https://doi.org/10.1175/JAS3389.1, 2005. a
Chowdhary, J., Cairns, B., Waquet, F., Knobelspiesse, K., Ottaviani, M., Redemann, J., Travis, L., and Mishchenko, M.: Sensitivity of multiangle, multispectral polarimetric remote sensing over open oceans to water-leaving radiance: Analyses of RSP data acquired during the MILAGRO campaign, Remote Sens. Environ., 118, 284–308, 2012. a
Chowdhary, J., Stamnes, S., Zhang, M., Scarino, A., Wasilewski, A., and Cairns, B.: Combining multispectral VIS-SWIR polarimetry and UV-NIR hyperspectral imagery to retrieve aerosol and ocean color properties from remote sensing: case studies for airborne RSP and GCAS observations, American Geophysical Union, Fall Meeting, abstract #OS11D-1435, 2018. a
Chowdhary, J., Zhai, P.-W., Boss, E., Dierssen, H., Frouin, R., Ibrahim, A., Lee, Z., Remer, L. A., Twardowski, M., Xu, F., Zhang, X., Ottaviani, M., Espinosa, W. R., and Ramon, D.: Modeling Atmosphere-Ocean Radiative Transfer: A PACE Mission Perspective, Front. Earth Sci., 7, 100, https://doi.org/10.3389/feart.2019.00100, 2019. a, b, c
Cox, C. and Munk, W.: Measurement of the Roughness of the Sea Surface from Photographs of the Sun's Glitter, J. Opt. Soc. Am., 44, 838–850, 1954. a
Craig, S. E., Jones, C. T., Li, W. K., Lazin, G., Horne, E., Caverhill, C., and Cullen, J. J.: Deriving optical metrics of coastal phytoplankton biomass from ocean colour, Remote Sens. Environ., 119, 72–83, https://doi.org/10.1016/j.rse.2011.12.007, 2012. a
Croft, B., Martin, R. V., Leaitch, W. R., Burkart, J., Chang, R. Y.-W., Collins, D. B., Hayes, P. L., Hodshire, A. L., Huang, L., Kodros, J. K., Moravek, A., Mungall, E. L., Murphy, J. G., Sharma, S., Tremblay, S., Wentworth, G. R., Willis, M. D., Abbatt, J. P. D., and Pierce, J. R.: Arctic marine secondary organic aerosol contributes significantly to summertime particle size distributions in the Canadian Arctic Archipelago, Atmos. Chem. Phys., 19, 2787–2812, https://doi.org/10.5194/acp-19-2787-2019, 2019. a
d'Almeida, G. A., Koepke, P., and Shettle, E. P.: Atmospheric aerosols: global climatology and radiative characteristics, Hampton, Va, USA, A. Deepak Pub., 1991 a
Aerosol Robotic Network (Aeronet) Technical Document: Description of Aerosol Inversion Uncertainty for Level 2 Products, available at: https://aeronet.gsfc.nasa.gov/new_web/optical_properties.html, last access: 7 July 2020. a
Dierssen, H. M. and Randolph, K.: Remote Sensing of Ocean Color, Springer New York, New York, NY, 439–472, https://doi.org/10.1007/978-1-4614-5684-1_18, 2013. a
Diner, D. J., Xu, F., Garay, M. J., Martonchik, J. V., Rheingans, B. E., Geier, S., Davis, A., Hancock, B. R., Jovanovic, V. M., Bull, M. A., Capraro, K., Chipman, R. A., and McClain, S. C.: The Airborne Multiangle SpectroPolarimetric Imager (AirMSPI): a new tool for aerosol and cloud remote sensing, Atmos. Meas. Tech., 6, 2007–2025, https://doi.org/10.5194/amt-6-2007-2013, 2013. a
Diner, D. J., Boland, S. W., Brauer, M., Bruegge, C., Burke, K. A., Chipman, R., Girolamo, L. D., Garay, M. J., Hasheminassab, S., Hyer, E., Jerrett, M., Jovanovic, V., Kalashnikova, O. V., Liu, Y., Lyapustin, A. I., Martin, R. V., Nastan, A., Ostro, B. D., Ritz, B., Schwartz, J., Wang, J., and Xu, F.: Advances in multiangle satellite remote sensing of speciated airborne particulate matter and association with adverse health effects: from MISR to MAIA, J. Appl. Remote Sens., 12, 1–22, https://doi.org/10.1117/1.JRS.12.042603, 2018. a
Dubovik, O. and King, M. D.: A flexible inversion algorithm for retrieval of aerosol optical properties from Sun and sky radiance measurements, J. Geophys. Res.-Atmos., 105, 20673–20696, 2000. a
Dubovik, O., Sinyuk, A., Lapyonok, T., Holben, B. N., Mishchenko, M., Yang, P., Eck, T. F., Volten, H., Muñoz, O., Veihelmann, B., van der Zande, W. J., Leon, J.-F., Sorokin, M., and Slutsker, I.: Application of spheroid models to account for aerosol particle nonsphericity in remote sensing of desert dust, J. Geophys. Res.-Atmos., 111, D11208, https://doi.org/10.1029/2005JD006619, 2006. a, b
Dubovik, O., Herman, M., Holdak, A., Lapyonok, T., Tanré, D., Deuzé, J. L., Ducos, F., Sinyuk, A., and Lopatin, A.: Statistically optimized inversion algorithm for enhanced retrieval of aerosol properties from spectral multi-angle polarimetric satellite observations, Atmos. Meas. Tech., 4, 975–1018, https://doi.org/10.5194/amt-4-975-2011, 2011. a
Dubovik, O., Lapyonok, T., Litvinov, P., Herman, M., Fuertes, D., Ducos, F., Lopatin, A., Chaikovsky, A., Torres, B., Derimian, Y., Huang, X., Aspetsberger, M., and Federspiel, C.: GRASP: a versatile algorithm for characterizing the atmosphere, SPIE Newsroom, https://doi.org/10.1117/2.1201408.005558, 2014. a
Dubovik, O., Li, Z., Mishchenko, M. I., Tanré, D., Karol, Y., Bojkov, B., Cairns, B., Diner, D. J., Espinosa, W. R., Goloub, P., Gu, X., Hasekamp, O., Hong, J., Hou, W., Knobelspiesse, K. D., Landgraf, J., Li, L., Litvinov, P., Liu, Y., Lopatin, A., Marbach, T., Maring, H., Martins, V., Meijer, Y., Milinevsky, G., Mukai, S., Parol, F., Qiao, Y., Remer, L., Rietjens, J., Sano, I., Stammes, P., Stamnes, S., Sun, X., Tabary, P., Travis, L. D., Waquet, F., Xu, F., Yan, C., and Yin, D.: Polarimetric remote sensing of atmospheric aerosols: Instruments, methodologies, results, and perspectives, J. Quant. Spectrosc. Ra., 224, 474–511, https://doi.org/10.1016/j.jqsrt.2018.11.024, 2019. a
Eck, T. F., Holben, B. N., Reid, J. S., Dubovik, O., Smirnov, A., O'Neill, N. T., Slutsker, I., and Kinne, S.: Wavelength dependence of the optical depth of biomass burning, urban, and desert dust aerosols, J. Geophys. Res.-Atmos., 104, 31333–31349, https://doi.org/10.1029/1999JD900923, 1999. a
Fan, C., Fu, G., Di Noia, A., Smit, M., H.H. Rietjens, J., A. Ferrare, R., Burton, S., Li, Z., and P. Hasekamp, O.: Use of A Neural Network-Based Ocean Body Radiative Transfer Model for Aerosol Retrievals from Multi-Angle Polarimetric Measurements, Remote Sens., 11, 2877, https://doi.org/10.3390/rs11232877, 2019. a
Fedarenka, A., Dubovik, O., Goloub, P., Li, Z., Lapyonok, T., Litvinov, P., Barel, L., Gonzalez, L., Podvin, T., and Crozel, D.: Utilization of AERONET polarimetric measurements for improving retrieval of aerosol microphysics: GSFC, Beijing and Dakar data analysis, J. Quant. Spectrosc. Ra., 179, 72–97, https://doi.org/10.1016/j.jqsrt.2016.03.021, 2016. a
Fichot, C. G. and Benner, R.: A novel method to estimate DOC concentrations from CDOM absorption coefficients in coastal waters, Geophys. Res. Lett., 38, L03610, https://doi.org/10.1029/2010GL046152, 2011. a
Fougnie, B., Marbach, T., Lacan, A., Lang, R., Schlüssel, P., Poli, G., Munro, R., and Couto, A. B.: The multi-viewing multi-channel multi-polarisation imager – Overview of the 3MI polarimetric mission for aerosol and cloud characterization, J. Quant. Spectrosc. Ra., 219, 23–32, https://doi.org/10.1016/j.jqsrt.2018.07.008, 2018. a
Franz, B. A., Bailey, S. W., Werdell, P. J., and McClain, C. R.: Sensor-independent approach to the vicarious calibration of satellite ocean color radiometry, Appl. Optics, 46, 5068–5082, https://doi.org/10.1364/AO.46.005068, 2007. a
Frouin, R. J., Franz, B. A., Ibrahim, A., Knobelspiesse, K., Ahmad, Z., Cairns, B., Chowdhary, J., Dierssen, H. M., Tan, J., Dubovik, O., Huang, X., Davis, A. B., Kalashnikova, O., Thompson, D. R., Remer, L. A., Boss, E., Coddington, O., Deschamps, P.-Y., Gao, B.-C., Gross, L., Hasekamp, O., Omar, A., Pelletier, B., Ramon, D., Steinmetz, F., and Zhai, P.-W.: Atmospheric Correction of Satellite Ocean-Color Imagery During the PACE Era, Front. Earth Sci., 7, 145, https://doi.org/10.3389/feart.2019.00145, 2019. a, b
Fu, G. and Hasekamp, O.: Retrieval of aerosol microphysical and optical properties over land using a multimode approach, Atmos. Meas. Tech., 11, 6627–6650, https://doi.org/10.5194/amt-11-6627-2018, 2018. a, b
Fu, G., Hasekamp, O., Rietjens, J., Smit, M., Di Noia, A., Cairns, B., Wasilewski, A., Diner, D., Seidel, F., Xu, F., Knobelspiesse, K., Gao, M., da Silva, A., Burton, S., Hostetler, C., Hair, J., and Ferrare, R.: Aerosol retrievals from different polarimeters during the ACEPOL campaign using a common retrieval algorithm, Atmos. Meas. Tech., 13, 553–573, https://doi.org/10.5194/amt-13-553-2020, 2020. a, b
Gao, M., Zhai, P.-W., Franz, B., Hu, Y., Knobelspiesse, K., Werdell, P. J., Ibrahim, A., Xu, F., and Cairns, B.: Retrieval of aerosol properties and water-leaving reflectance from multi-angular polarimetric measurements over coastal waters, Opt. Express, 26, 8968–8989, https://doi.org/10.1364/OE.26.008968, 2018. a, b, c, d, e, f
Gao, M., Zhai, P.-W., Franz, B. A., Hu, Y., Knobelspiesse, K., Werdell, P. J., Ibrahim, A., Cairns, B., and Chase, A.: Inversion of multiangular polarimetric measurements over open and coastal ocean waters: a joint retrieval algorithm for aerosol and water-leaving radiance properties, Atmos. Meas. Tech., 12, 3921–3941, https://doi.org/10.5194/amt-12-3921-2019, 2019. a, b, c, d, e, f, g, h, i
Gelaro, R., McCarty, W., Suárez, M. J., Todling, R., Molod, A., Takacs, L., Randles, C. A., Darmenov, A., Bosilovich, M. G., Reichle, R., Wargan, K., Coy, L., Cullather, R., Draper, C., Akella, S., Buchard, V., Conaty, A., da Silva, A. M., Gu, W., Kim, G.-K., Koster, R., Lucchesi, R., Merkova, D., Nielsen, J. E., Partyka, G., Pawson, S., Putman, W., Rienecker, M., Schubert, S. D., Sienkiewicz, M., and Zhao, B.: The Modern-Era Retrospective Analysis for Research and Applications, Version 2 (MERRA-2), J. Climate, 30, 5419–5454, https://doi.org/10.1175/JCLI-D-16-0758.1, 2017. a
Giles, D. M., Sinyuk, A., Sorokin, M. G., Schafer, J. S., Smirnov, A., Slutsker, I., Eck, T. F., Holben, B. N., Lewis, J. R., Campbell, J. R., Welton, E. J., Korkin, S. V., and Lyapustin, A. I.: Advancements in the Aerosol Robotic Network (AERONET) Version 3 database – automated near-real-time quality control algorithm with improved cloud screening for Sun photometer aerosol optical depth (AOD) measurements, Atmos. Meas. Tech., 12, 169–209, https://doi.org/10.5194/amt-12-169-2019, 2019. a
Gordon, H. R. and Wang, M.: Retrieval of water-leaving radiance and aerosol optical thickness over the oceans with SeaWiFS: a preliminary algorithm, Appl. Optics, 33, 443–452, 1994. a
Hair, J. W., Hostetler, C. A., Cook, A. L., Harper, D. B., Ferrare, R. A., Mack, T. L., Welch, W., Izquierdo, L. R., and Hovis, F. E.: Airborne High Spectral Resolution Lidar for profiling aerosol optical properties, Appl. Optics, 47, 6734–6752, https://doi.org/10.1364/AO.47.006734, 2008. a
Hasekamp, O. P. and Landgraf, J.: Retrieval of aerosol properties over land surfaces: capabilities of multiple-viewing-angle intensity and polarization measurements, Appl. Optics, 46, 3332–3344, https://doi.org/10.1364/AO.46.003332, 2007. a
Hasekamp, O. P., Litvinov, P., and Butz, A.: Aerosol properties over the ocean from PARASOL multiangle photopolarimetric measurements, J. Geophys. Res.-Oceans, 116, D14204, https://doi.org/10.1029/2010JD015469, 2011. a, b
Hasekamp, O. P., Fu, G., Rusli, S. P., Wu, L., Noia, A. D., aan de Brugh, J., Landgraf, J., Smit, J. M., Rietjens, J., and van Amerongen, A.: Aerosol measurements by SPEXone on the NASA PACE mission: expected retrieval capabilities, J. Quant. Spectrosc. Ra., 227, 170–184, https://doi.org/10.1016/j.jqsrt.2019.02.006, 2019. a
Holben, B., Eck, T., Slutsker, I., Tanré, D., Buis, J., Setzer, A., Vermote, E., Reagan, J., Kaufman, Y., Nakajima, T., Lavenu, F., Jankowiak, I., and Smirnov, A.: AERONET–A Federated Instrument Network and Data Archive for Aerosol Characterization, Remote Sens. Environ., 66, 1–16, https://doi.org/10.1016/S0034-4257(98)00031-5, 1998. a, b
Holben, B. N., Tanré, D., Smirnov, A., Eck, T. F., Slutsker, I., Abuhassan, N., Newcomb, W. W., Schafer, J. S., Chatenet, B., Lavenu, F., Kaufman, Y. J., Castle, J. V., Setzer, A., Markham, B., Clark, D., Frouin, R., Halthore, R., Karneli, A., O'Neill, N. T., Pietras, C., Pinker, R. T., Voss, K., and Zibordi, G.: An emerging ground-based aerosol climatology: Aerosol optical depth from AERONET, J. Geophys. Res.-Atmos., 106, 12067–12097, https://doi.org/10.1029/2001JD900014, 2001. a
Hu, C., Feng, L., and Lee, Z.: Uncertainties of SeaWiFS and MODIS remote sensing reflectance: Implications from clear water measurements, Remote Sens. Environ., 133, 168–182, https://doi.org/10.1016/j.rse.2013.02.012, 2013. a
Ibrahim, A., Franz, B., Ahmad, Z., Healy, R., Knobelspiesse, K., Gao, B.-C., Proctor, C., and Zhai, P.-W.: Atmospheric correction for hyperspectral ocean color retrieval with application to the Hyperspectral Imager for the Coastal Ocean (HICO), Remote Sens. Environ., 204, 60–75, https://doi.org/10.1016/j.rse.2017.10.041, 2018. a, b, c
Ibrahim, A., Franz, B. A., Ahmad, Z., and Bailey, S. W.: Multiband Atmospheric Correction Algorithm for Ocean Color Retrievals, Front. Earth Sci., 7, 116, https://doi.org/10.3389/feart.2019.00116, 2019. a, b
Jamet, C., Ibrahim, A., Ahmad, Z., Angelini, F., Babin, M., Behrenfeld, M. J., Boss, E., Cairns, B., Churnside, J., Chowdhary, J., Davis, A. B., Dionisi, D., Duforêt-Gaurier, L., Franz, B., Frouin, R., Gao, M., Gray, D., Hasekamp, O., He, X., Hostetler, C., Kalashnikova, O. V., Knobelspiesse, K., Lacour, L., Loisel, H., Martins, V., Rehm, E., Remer, L., Sanhaj, I., Stamnes, K., Stamnes, S., Victori, S., Werdell, J., and Zhai, P.-W.: Going Beyond Standard Ocean Color Observations: Lidar and Polarimetry, Front. Mar. Sci., 6, 251, https://doi.org/10.3389/fmars.2019.00251, 2019. a
Kaufman, Y. J., Martins, J. V., Remer, L. A., Schoeberl, M. R., and Yamasoe, M. A.: Satellite retrieval of aerosol absorption over the oceans using sunglint, Geophys. Res. Lett., 29, 34-1–34-4, https://doi.org/10.1029/2002GL015403, 2002. a
Kawata, Y.: Circular polarization of sunlight reflected by planetary atmospheres, Icarus, 33, 217–232, https://doi.org/10.1016/0019-1035(78)90035-0, 1978. a
Knobelspiesse, K., Cairns, B., Mishchenko, M., Chowdhary, J., Tsigaridis, K., van Diedenhoven, B., Martin, W., Ottaviani, M., and Alexandrov, M.: Analysis of fine-mode aerosol retrieval capabilities by different passive remote sensing instrument designs, Opt. Express, 20, 21457–21484, https://doi.org/10.1364/OE.20.021457, 2012. a, b, c, d, e, f, g
Knobelspiesse, K., Tan, Q., Bruegge, C., Cairns, B., Chowdhary, J., van Diedenhoven, B., Diner, D., Ferrare, R., van Harten, G., Jovanovic, V., Ottaviani, M., Redemann, J., Seidel, F., and Sinclair, K.: Intercomparison of airborne multi-angle polarimeter observations from the Polarimeter Definition Experiment, Appl. Optics, 58, 650–669, https://doi.org/10.1364/AO.58.000650, 2019. a, b
Knobelspiesse, K., Barbosa, H. M. J., Bradley, C., Bruegge, C., Cairns, B., Chen, G., Chowdhary, J., Cook, A., Di Noia, A., van Diedenhoven, B., Diner, D. J., Ferrare, R., Fu, G., Gao, M., Garay, M., Hair, J., Harper, D., van Harten, G., Hasekamp, O., Helmlinger, M., Hostetler, C., Kalashnikova, O., Kupchock, A., Longo De Freitas, K., Maring, H., Martins, J. V., McBride, B., McGill, M., Norlin, K., Puthukkudy, A., Rheingans, B., Rietjens, J., Seidel, F. C., da Silva, A., Smit, M., Stamnes, S., Tan, Q., Val, S., Wasilewski, A., Xu, F., Xu, X., and Yorks, J.: The Aerosol Characterization from Polarimeter and Lidar (ACEPOL) airborne field campaign, Earth Syst. Sci. Data Discuss., https://doi.org/10.5194/essd-2020-76, in review, 2020. a, b, c
Li, Z., Hou, W., Hong, J., Zheng, F., Luo, D., Wang, J., Gu, X., and Qiao, Y.: Directional Polarimetric Camera (DPC): Monitoring aerosol spectral optical properties over land from satellite observation, J. Quant. Spectrosc. Ra., 218, 21–37, https://doi.org/10.1016/j.jqsrt.2018.07.003, 2018. a
Mahowald, N. M., Baker, A. R., Bergametti, G., Brooks, N., Duce, R. A., Jickells, T. D., Kubilay, N., Prospero, J. M., and Tegen, I.: Atmospheric global dust cycle and iron inputs to the ocean, Global Biogeochem. Cy., 19, GB4025, https://doi.org/10.1029/2004GB002402, 2005. a
Martins, J. V., Fernandez-Borda, R., McBride, B., Remer, L., and Barbosa, H. M. J.: The Harp Hype Ran Gular Imaging Polarimeter and the Need for Small Satellite Payloads with High Science Payoff for Earth Science Remote Sensing, in: IGARSS 2018–2018 IEEE International Geoscience and Remote Sensing Symposium, 6304–6307, https://doi.org/10.1109/IGARSS.2018.8518823, 2018. a
McBride, B. A., Martins, J. V., Barbosa, H. M. J., Birmingham, W., and Remer, L. A.: Spatial distribution of cloud droplet size properties from Airborne Hyper-Angular Rainbow Polarimeter (AirHARP) measurements, Atmos. Meas. Tech., 13, 1777–1796, https://doi.org/10.5194/amt-13-1777-2020, 2020. a
McCoy, D. T., Burrows, S. M., Wood, R., Grosvenor, D. P., Elliott, S. M., Ma, P.-L., Rasch, P. J., and Hartmann, D. L.: Natural aerosols explain seasonal and spatial patterns of Southern Ocean cloud albedo, Sci. Adv., 1, e1500157, https://doi.org/10.1126/sciadv.1500157, 2015. a
McGill, M., Hlavka, D., Hart, W., Scott, V. S., Spinhirne, J., and Schmid, B.: Cloud Physics Lidar: instrument description and initial measurement results, Appl. Optics, 41, 3725–3734, https://doi.org/10.1364/AO.41.003725, 2002. a
Mishchenko, M. I. and Travis, L. D.: Satellite retrieval of aerosol properties over the ocean using polarization as well as intensity of reflected sunlight, J. Geophys. Res.-Atmos., 102, 16989–17013, https://doi.org/10.1029/96JD02425, 1997. a
Mobley, C. D., Werdell, J., Franz, B., Ahmad, Z., and Bailey, S.: Atmospheric Correction for Satellite Ocean Color Radiometry, National Aeronautics and Space Administration, Goddard Space Flight CenterGreenbelt, Maryland, 2016. a, b, c, d, e
Ocean Biology Processing Group (OBPG): NASA Ocean Color Web, available at: https://oceancolor.gsfc.nasa.gov/atbd/rrs/, last access: 7 July 2020. a
NASA Goddard Institute for Space Studies (GISS): NASA RSP Data Archive, available at: https://data.giss.nasa.gov/pub/rsp, last access: 7 July 2020. a
O'Dowd, C. D., Jimenez, J. L., Bahreini, R., Flagan, R. C., Seinfeld, J. H., Hämeri, K., Pirjola, L., Kulmala, M., Jennings, S. G., and Hoffmann, T.: Marine aerosol formation from biogenic iodine emissions, Nature, 417, 632–636, https://doi.org/10.1038/nature00775, 2002. a
Ottaviani, M., Knobelspiesse, K., Cairns, B., and Mishchenko, M.: Information content of aerosol retrievals in the sunglint region, Geophys. Res. Lett., 40, 631–634, https://doi.org/10.1002/grl.50148, 2013. a
PACE: Pre-Aerosol,Clouds, and ocean Ecosystem (PACE) Mission Science Definition Team Report, The National Aeronautics and Space Administration (NASA), available at: https://pace.oceansciences.org/docs/PACE_TM2018-219027_Vol_2.pdf (last access: 7 July 2020), 2018. a
PACE Science Data Product Validation Plan: PACE Science Data Product Validation Plan, available at: https://pace.oceansciences.org/docs/PACE_Validation_Plan_DRAFT_version_24March2020_posted.pdf, last access: 7 July 2020. a
Remer, L. A., Davis, A. B., Mattoo, S., Levy, R. C., Kalashnikova, O. V., Coddington, O., Chowdhary, J., Knobelspiesse, K., Xu, X., Ahmad, Z., Boss, E., Cairns, B., Dierssen, H. M., Diner, D. J., Franz, B., Frouin, R., Gao, B.-C., Ibrahim, A., Martins, J. V., Omar, A. H., Torres, O., Xu, F., and Zhai, P.-W.: Retrieving Aerosol Characteristics From the PACE Mission, Part 1: Ocean Color Instrument, Front. Earth Sci., 7, 152, https://doi.org/10.3389/feart.2019.00152, 2019a. a, b
Remer, L. A., Knobelspiesse, K., Zhai, P.-W., Xu, F., Kalashnikova, O. V., Chowdhary, J., Hasekamp, O., Dubovik, O., Wu, L., Ahmad, Z., Boss, E., Cairns, B., Coddington, O., Davis, A. B., Dierssen, H. M., Diner, D. J., Franz, B., Frouin, R., Gao, B.-C., Ibrahim, A., Levy, R. C., Martins, J. V., Omar, A. H., and Torres, O.: Retrieving Aerosol Characteristics From the PACE Mission, Part 2: Multi-Angle and Polarimetry, Front. Environ. Sci., 7, 94, https://doi.org/10.3389/fenvs.2019.00094, 2019b. a
Rietjens, J., Campo, J., Chanumolu, A., Smit, M., Nalla, R., Fernandez, C., Dingjan, J., van Amerongen, A., and Hasekamp, O.: Expected performance and error analysis for SPEXone, a multi-angle channeled spectropolarimeter for the NASA PACE mission, in: Polarization Science and Remote Sensing IX, edited by: Craven, J. M., Shaw, J. A., and Snik, F., 11132, 34–47, International Society for Optics and Photonics, SPIE, https://doi.org/10.1117/12.2530729, 2019. a
Shettle, E. P. and Fenn, R. W.: Models for the aerosols of the lower atmosphere and the effects of humidity variations on their optical properties, Environmental Research Papers, Air Force Geophysics Lab., Hanscom AFB, MA. Optical Physics Div, 1979. a
Siegel, D. A., Buesseler, K. O., Doney, S. C., Sailley, S. F., Behrenfeld, M. J., and Boyd, P. W.: Global assessment of ocean carbon export by combining satellite observations and food-web models, Global Biogeochem. Cy., 28, 181–196, https://doi.org/10.1002/2013GB004743, 2014. a
Smirnov, A., Holben, B., Eck, T., Dubovik, O., and Slutsker, I.: Cloud-Screening and Quality Control Algorithms for the AERONET Database, Remote Sens. Environ., 73, 337–349, https://doi.org/10.1016/S0034-4257(00)00109-7, 2000. a
Smit, J. M., Rietjens, J. H. H., van Harten, G., Noia, A. D., Laauwen, W., Rheingans, B. E., Diner, D. J., Cairns, B., Wasilewski, A., Knobelspiesse, K. D., Ferrare, R., and Hasekamp, O. P.: SPEX airborne spectropolarimeter calibration and performance, Appl. Optics, 58, 5695–5719, https://doi.org/10.1364/AO.58.005695, 2019. a, b, c, d
Stamnes, S., Hostetler, C., Ferrare, R., Burton, S., Liu, X., Hair, J., Hu, Y., Wasilewski, A., Martin, W., van Diedenhoven, B., Chowdhary, J., Cetinić, I., Berg, L. K., Stamnes, K., and Cairns, B.: Simultaneous polarimeter retrievals of microphysical aerosol and ocean color parameters from the “MAPP” algorithm with comparison to high-spectral-resolution lidar aerosol and ocean products, Appl. Optics, 57, 2394–2413, https://doi.org/10.1364/AO.57.002394, 2018. a
Tanré, D., Bréon, F. M., Deuzé, J. L., Dubovik, O., Ducos, F., François, P., Goloub, P., Herman, M., Lifermann, A., and Waquet, F.: Remote sensing of aerosols by using polarized, directional and spectral measurements within the A-Train: the PARASOL mission, Atmos. Meas. Tech., 4, 1383–1395, https://doi.org/10.5194/amt-4-1383-2011, 2011. a
Wang, J., Xu, X., Ding, S., Zeng, J., Spurr, R., Liu, X., Chance, K., and Mishchenko, M.: A numerical testbed for remote sensing of aerosols, and its demonstration for evaluating retrieval synergy from a geostationary satellite constellation of GEO-CAPE and GOES-R, J. Quant. Spectrosc. Ra., 146, 510–528, https://doi.org/10.1016/j.jqsrt.2014.03.020, 2014. a
Wang, M. (Ed.): Atmospheric Correction for Remotely-Sensed Ocean-Colour, International Ocean Colour Coordinating Group (IOCCG), IOCCG Report Number 10, 2010. a
Werdell, P. J., Behrenfeld, M. J., Bontempi, P. S., Boss, E., Cairns, B., Davis, G. T., Franz, B. A., Gliese, U. B., Gorman, E. T., Hasekamp, O., Knobelspiesse, K. D., Mannino, A., Martins, J. V., McClain, C. R., Meister, G., and Remer, L. A.: The Plankton, Aerosol, Cloud, Ocean Ecosystem Mission: Status, Science, Advances, B. Am. Meteorol. Soc., 100, 1775–1794, https://doi.org/10.1175/BAMS-D-18-0056.1, 2019. a, b, c, d, e
Westberry, T., Shi, Y., Yu, H., Behrenfeld, M., and Remer, L.: Satellite-Detected Ocean Ecosystem Response to Volcanic Eruptions in the Subarctic Northeast Pacific Ocean, Geophys. Res. Lett., 46, 11270–11280, https://doi.org/10.1029/2019GL083977, 2019. a
Wu, L., Hasekamp, O., van Diedenhoven, B., and Cairns, B.: Aerosol retrieval from multiangle, multispectral photopolarimetric measurements: importance of spectral range and angular resolution, Atmos. Meas. Tech., 8, 2625–2638, https://doi.org/10.5194/amt-8-2625-2015, 2015. a, b
Xu, F., Dubovik, O., Zhai, P.-W., Diner, D. J., Kalashnikova, O. V., Seidel, F. C., Litvinov, P., Bovchaliuk, A., Garay, M. J., van Harten, G., and Davis, A. B.: Joint retrieval of aerosol and water-leaving radiance from multispectral, multiangular and polarimetric measurements over ocean, Atmos. Meas. Tech., 9, 2877–2907, https://doi.org/10.5194/amt-9-2877-2016, 2016. a, b
Xu, F., van Harten, G., Diner, D. J., Kalashnikova, O. V., Seidel, F. C., Bruegge, C. J., and Dubovik, O.: Coupled retrieval of aerosol properties and land surface reflection using the Airborne Multiangle SpectroPolarimetric Imager, J. Geophys. Res.-Atmos., 122, 7004–7026, https://doi.org/10.1002/2017JD026776, 2017. a
Xu, F., Diner, D. J., Dubovik, O., and Schechner, Y.: A Correlated Multi-Pixel Inversion Approach for Aerosol Remote Sensing, Remote Sens., 11, https://doi.org/10.3390/rs11070746, 2019. a
Xu, X. and Wang, J.: Retrieval of aerosol microphysical properties from AERONET photopolarimetric measurements: 1. Information content analysis, J. Geophys. Res.-Atmos., 120, 7059–7078, https://doi.org/10.1002/2015JD023108, 2015. a
Xu, X., Wang, J., Zeng, J., Spurr, R., Liu, X., Dubovik, O., Li, L., Li, Z., Mishchenko, M. I., Siniuk, A., and Holben, B. N.: Retrieval of aerosol microphysical properties from AERONET photopolarimetric measurements: 2. A new research algorithm and case demonstration, J. Geophys. Res.-Atmos., 120, 7079–7098, https://doi.org/10.1002/2015JD023113, 2015. a
Zhai, P.-W., Hu, Y., Trepte, C. R., and Lucker, P. L.: A vector radiative transfer model for coupled atmosphere and ocean systems based on successive order of scattering method, Opt. Express, 17, 2057–2079, 2009. a, b
Zhai, P.-W., Hu, Y., Chowdhary, J., Trepte, C. R., Lucker, P. L., and Josset, D. B.: A vector radiative transfer model for coupled atmosphere and ocean systems with a rough interface, J. Quant. Spectrosc. Ra., 111, 1025–1040, 2010. a, b
Zhai, P.-W., Knobelspiesse, K., Ibrahim, A., Franz, B. A., Hu, Y., Gao, M., and Frouin, R.: Water-leaving contribution to polarized radiation field over ocean, Opt. Express, 25, A689–A708, https://doi.org/10.1364/OE.25.00A689, 2017. a
Zhai, P.-W., Boss, E., Franz, B., Werdell, P. J., and Hu, Y.: Radiative Transfer Modeling of Phytoplankton Fluorescence Quenching Processes, Remote Sens., 10, 1309, https://doi.org/10.3390/rs10081309, 2018. a, b
Zibordi, G., Mélin, F., Berthon, J.-F., Holben, B., Slutsker, I., Giles, D., D’Alimonte, D., Vandemark, D., Feng, H., Schuster, G., Fabbri, B. E., Kaitala, S., and Seppälä, J.: AERONET-OC: A Network for the Validation of Ocean Color Primary Products, J. Atmos. Ocean. Tech., 26, 1634–1651, https://doi.org/10.1175/2009JTECHO654.1, 2009. a, b, c