the Creative Commons Attribution 4.0 License.
the Creative Commons Attribution 4.0 License.
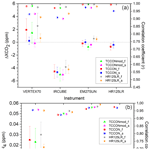
Intercomparison of low- and high-resolution infrared spectrometers for ground-based solar remote sensing measurements of total column concentrations of CO2, CH4, and CO
Martine De Mazière
Justus Notholt
Thomas Blumenstock
Huilin Chen
Angelika Dehn
David W. T. Griffith
Frank Hase
Pauli Heikkinen
Christian Hermans
Alex Hoffmann
Marko Huebner
Nicholas Jones
Rigel Kivi
Bavo Langerock
Christof Petri
Francis Scolas
Qiansi Tu
Damien Weidmann
The Total Carbon Column Observing Network (TCCON) is the baseline ground-based network of instruments that record solar absorption spectra from which accurate and precise column-averaged dry-air mole fractions of CO2 (XCO2), CH4 (XCH4), CO (XCO), and other gases are retrieved. The TCCON data have been widely used for carbon cycle science and validation of satellites measuring greenhouse gas concentrations globally. The number of stations in the network (currently about 25) is limited and has a very uneven geographical coverage: the stations in the Northern Hemisphere are distributed mostly in North America, Europe, and Japan, and only 20 % of the stations are located in the Southern Hemisphere, leaving gaps in the global coverage. A denser distribution of ground-based solar absorption measurements is needed to improve the representativeness of the measurement data for various atmospheric conditions (humid, dry, polluted, presence of aerosol), various surface conditions such as high albedo (>0.4) and very low albedo, and a larger latitudinal distribution. More stations in the Southern Hemisphere are also needed, but a further expansion of the network is limited by its costs and logistical requirements. For this reason, several groups are investigating supplemental portable low-cost instruments. The European Space Agency (ESA) funded campaign Fiducial Reference Measurements for Ground-Based Infrared Greenhouse Gas Observations (FRM4GHG) at the Sodankylä TCCON site in northern Finland aims to characterise the assessment of several low-cost portable instruments for precise solar absorption measurements of XCO2, XCH4, and XCO. The test instruments under investigation are three Fourier transform spectrometers (FTSs): a Bruker EM27/SUN, a Bruker IRcube, and a Bruker Vertex70, as well as a laser heterodyne spectroradiometer (LHR) developed by the UK Rutherford Appleton Laboratory. All four remote sensing instruments performed measurements simultaneously next to the reference TCCON instrument, a Bruker IFS 125HR, for a full year in 2017. The TCCON FTS was operated in its normal high-resolution mode (TCCON data set) and in a special low-resolution mode (HR125LR data set), similar to the portable spectrometers. The remote sensing measurements are complemented by regular AirCore launches performed from the same site. They provide in situ vertical profiles of the target gas concentrations as auxiliary reference data for the column retrievals, which are traceable to the WMO SI standards. The reference measurements performed with the Bruker IFS 125HR were found to be affected by non-linearity of the indium gallium arsenide (InGaAs) detector. Therefore, a non-linearity correction of the 125HR data was performed for the whole campaign period and compared with the test instruments and AirCore. The non-linearity-corrected data (TCCONmod data set) show a better match with the test instruments and AirCore data compared to the non-corrected reference data. The time series, the bias relative to the reference instrument and its scatter, and the seasonal and the day-to-day variations of the target gases are shown and discussed. The comparisons with the HR125LR data set gave a useful analysis of the resolution-dependent effects on the target gas retrieval. The solar zenith angle dependence of the retrievals is shown and discussed. The intercomparison results show that the LHR data have a large scatter and biases with a strong diurnal variation relative to the TCCON and other FTS instruments. The LHR is a new instrument under development, and these biases are currently being investigated and addressed. The campaign helped to characterise and identify instrumental biases and possibly retrieval biases, which are currently under investigation. Further improvements of the instrument are ongoing. The EM27/SUN, the IRcube, the modified Vertex70, and the HR125LR provided stable and precise measurements of the target gases during the campaign with quantified small biases. The bias dependence on the humidity along the measurement line of sight has been investigated and no dependence was found. These three portable low-resolution FTS instruments are suitable to be used for campaign deployment or long-term measurements from any site and offer the ability to complement the TCCON and expand the global coverage of ground-based reference measurements of the target gases.
- Article
(6937 KB) - Full-text XML
- BibTeX
- EndNote
Carbon dioxide (CO2) and methane (CH4) are the two main components of the carbon cycle of the Earth's atmosphere. They absorb and retain heat in the atmosphere, causing global warming. CH4 has a global warming potential of about 28 times greater than CO2 over a 100-year time period. However, it exists in much lower concentrations and has a significantly shorter lifetime compared to CO2. CH4 also plays an important role in atmospheric chemistry by reacting with hydroxyl radicals (OH), thereby reducing the oxidation capacity of the atmosphere and producing ozone (Kirschke et al., 2013). The atmospheric concentration of both these gases has been steadily increasing in recent years caused by anthropogenic activities (Stocker et al., 2013; Dlugokencky and Tans, 2019). The third gas focused on is carbon monoxide (CO). It is a poisonous reactive gas considered to be principally a man-made pollutant. The volatile organic compounds (VOCs) plays an important role in the production of CO. It plays an important role in atmospheric chemistry by reacting with the atmospheric oxidants ozone (O3), the hydroperoxy radical (HO2), and hydroxyl radicals (OH). The lifetime of CO ranges from weeks to months (Novelli et al., 1998). An increase in CO would imply that more OH will be lost through chemical reaction with CO and that less OH will be available for reaction with CH4. Therefore, CO has an indirect but important influence in determining the chemical composition and radiative properties of the atmosphere. Emissions of CO are virtually certain to have a positive radiative forcing; therefore, it is considered an indirect greenhouse gas (Stocker et al., 2013). Continuous monitoring of precise and accurate measurements of these gases is of utmost importance to determine their sources, sinks, and trends. Currently, this is one of the major challenges within climate research which will help in understanding the carbon cycle.
Atmospheric measurements of CO2, CH4, and CO have been performed by in situ surface-based networks for many decades. These have been complemented by sparse aircraft measurement campaigns providing important additional measurements. However, both these measurement types have been performed at only a few locations, and the atmosphere has been sampled non-uniformly. In recent years, satellite-based remote sensing measurements have been able to provide global coverage of these gases. The nadir-looking satellites detecting scattered sunlight in the near-infrared (NIR) spectral region provide the most powerful method for global mapping of these gases. These measurements cover the whole atmospheric column, providing the total column concentrations of the trace gases of interest, and add important measurements to the in situ networks. However, satellite measurements require accurate validation. These accurate reference measurements can be performed from surface-based, airborne (e.g. balloon or aircraft), or already validated satellites. To ensure equal dependency on the measurement parameters, the best validation method for satellite data is to use the total column amounts of the trace gases calculated from the solar absorption measurements performed from the surface and the satellite in the same spectral region. Moreover, the total column observations are much less sensitive to boundary layer effects compared to in situ surface measurements.
The current state-of-the-art validation system for greenhouse gases (GHGs) is the Total Carbon Column Observing Network (TCCON). TCCON is a network of ground-based Fourier transform spectrometers (FTSs), of the type Bruker IFS 125HR, that record solar absorption spectra in the NIR spectral range to retrieve accurate and precise column-averaged abundances of atmospheric constituents including CO2, CH4, and CO amongst other species (Wunch et al., 2011). There are currently about 25 TCCON stations distributed globally, which form the backbone of the validation data set for the GHG-measuring satellites (e.g. GOSAT, OCO-2, Sentinel-5 Precursor) and model comparisons (Inoue et al., 2016; Wunch et al., 2017; Borsdorff et al., 2018; Kivimäki et al., 2019; Ostler et al., 2016; Jing et al., 2018; Kong et al., 2019). The distribution of the TCCON stations currently lacks global coverage, with a majority of its stations located in North America, Europe, and Japan, and currently only five stations in the Southern Hemisphere. The lack of stations close to important source areas and the limited number of stations in general result in an inability to resolve global GHG gradients. Furthermore, for the complete validation of the satellite data set, a denser distribution of ground-based solar absorption measurements is needed to cover geographical gaps and to improve the representativeness of the measurement data for various surface and atmospheric conditions (e.g. high and very low surface albedo, pollution, aerosol presence, humid, dry).
An extension of the TCCON network is limited by high start-up, maintenance, and operational costs, as well as difficulties of campaign-based transportability. The maintenance of the instrument requires skill and experience. All these factors have resulted in the development of a number of cheap and easily deployable instruments for remote sensing measurements of greenhouse gases, mainly driven by scientific research institutes in collaboration with industrial partners. Some of these instruments have been in operation for several years. However, there has been little characterisation, intercomparison, and harmonisation of these new instruments in comparison to the standard instrument used in TCCON, except for the EM27/SUN for which some previous characterisation work has been done (Gisi et al., 2012; Frey et al., 2015; Hedelius et al., 2016, 2017; Frey et al., 2019). These comparisons, however, are mandatory for using these individual data sets independently for science. The EM27/SUN deployed for this campaign is part of the COllaborative Carbon Column Observing Network (COCCON).
For this reason, in 2017, the European Space Agency (ESA) initiated an intercomparison campaign within the project Fiducial Reference Measurements for Ground-Based Infrared Greenhouse Gas observation (FRM4GHG). The campaign was performed in Sodankylä (Finland) with the aim of assessing the performance of different spectrometric instruments for remote sensing of atmospheric trace gases and quantifying their performances regarding precise measurements of column-averaged dry-air volume mole fractions of CO2, CH4, and CO. The instruments were deployed at the meteorological observatory Sodankylä where measurements took place between March and October 2017. The remote sensing measurements were complemented by regular AirCore (Karion et al., 2010) launches from the same site. AirCore measurements provide vertical profiles of the target gas concentrations as auxiliary reference data for the column measurements. The performances of the instruments were compared between themselves and to a reference TCCON instrument. The goal of this campaign was the characterisation of less expensive and more portable FTSs to complement TCCON for the establishment of a wider and denser network.
This paper is organised as follows: Sect. 2 gives a description of the campaign site, the details of the instruments taking part in the campaign, and their evolution. Section 3 gives a description of the measurement strategy that was used to ensure comparable observations. Section 4 gives a description of the data and their availability. Section 5 gives the campaign results, showing the intercomparison results between the TCCON, non-linearity-corrected TCCON (TCCONmod), and AirCore data, as well as results using the AirCore profile as a priori for the FTS retrievals. It also gives the intercomparison results between the test instruments with respect to the reference TCCONmod. The section concludes with a presentation of the intercomparison results of EM27/SUN data processed with PROFFAST (COCCON processing chain) and GFIT (TCCON processing suite), highlighting the code-dependent biases. Section 6 concludes the paper by giving a summary of the results.
2.1 Description of the campaign site
The Finnish Meteorological Institute (FMI) Sodankylä facility was selected as the campaign site as it fulfilled all selection criteria: (i) availability of TCCON measurements at the site, (ii) possibility to launch, retrieve, and analyse AirCore, (iii) infrastructure to host all participating instruments, and (iv) local support by scientists and engineers in the case of problems occurring with the instruments during the campaign. The Sodankylä facility is located above the Arctic Circle in northern Finland (67.3668∘ N, 26.6310∘ E; 188 m a.s.l.) about 6 km south of Sodankylä. Due to the location of the site at a high latitude, measurements are possible for a solar zenith angle (SZA) range between >43 and <90∘. The coverage of high SZAs is important to check the dependence of the air mass on the retrieval results. The air-mass-dependent correction factor applied to the remote sensing data is relevant for measurements at higher SZA. The site is equipped with a stratospheric balloon launch facility. The AirCore system has been operated by FMI to perform regular balloon launches since early September 2013. AirCore and other balloon payloads can be launched within 200 m from the TCCON instrument. In addition, the site also has a mobile system to launch payloads from an upwind site in order to retrieve them in the vicinity of the TCCON site. Upon its recovery, the analysis of the AirCore is done on-site using a Picarro G2401 analyser. Continuous surface in situ measurements of CO2, CH4, and CO are performed from a 50 m tower located 500 m away from the TCCON instrument. Further details on the site can be found in Kivi and Heikkinen (2016). An air-conditioned laboratory container (∼9.1 m long) was set up for the deployment of visiting instruments for the campaign. The laboratory was placed about 30 m south from the building hosting the TCCON instrument.
2.2 Instruments
The TCCON spectrometer, a Bruker IFS 125HR, was the main reference instrument for this campaign. Four low-resolution portable instruments participated in the campaign: a Bruker EM27/SUN, a Bruker Vertex70, a Bruker IRcube, and a homemade laser heterodyne spectroradiometer (LHR). Each of the three Bruker low-resolution instruments is based on a RockSolid™ corner-cube pendulum interferometer. This allows for comparable sampling quality and robustness amongst the instruments. However, the instruments differ in the use of the surrounding imaging optics and their geometric arrangement, which defines the interferometric field of view (FOV) and thus determines the instrumental line shape (ILS) of the respective instrument. The position of the centre burst, which determines the resolution, differs for each instrument. The EM27/SUN records double-sided and the IRcube single-sided interferograms, yielding a maximum resolution of 0.5 cm−1. The Vertex70 records single-sided interferograms giving a maximum resolution of 0.16 cm−1. The number of usable detector positions differs for the three instruments. The EM27/SUN can accommodate two room-temperature (RT) indium gallium arsenide (InGaAs) detectors covering different frequency ranges. Also, the Vertex70 can accommodate two detectors, one InGaAs and a second channel with either a liquid-nitrogen-cooled (LN2) indium antimonide (InSb) or an RT InGaAs detector. The IRcube can only accommodate one InGaAs detector and has no room for a second detector. All instruments used solar trackers with an active feedback loop to track the sun with an accuracy better than 0.1 mrad either with the help of active quadrant diodes or by active camera positioning. All low-resolution test instruments have the advantage that they do not need to be disassembled for transport. A detailed description of the instruments is given in the following subsections, and some of the key features of the instruments, measurement properties, and retrieval strategies during the campaign are listed in Tables 1 and 2.
Table 1List of instruments participating in the FRM4GHG campaign in 2017 and their properties; n/a indicates not applicable.
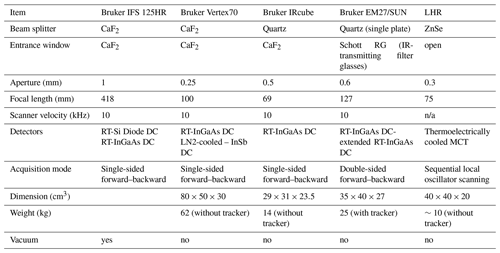
Table 2List of instruments, their measurement properties, and retrieval strategy for the FRM4GHG campaign in 2017.
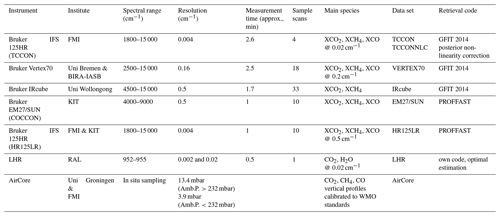
2.2.1 Bruker IFS 125HR
The instrumental and operational setting of the Bruker IFS 125HR in the TCCON mode of operation can be found in detail in Kivi and Heikkinen (2016). The TCCON instrument's operation, maintenance, and data analysis were performed by FMI. The measurements were performed at a spectral resolution of 0.02 cm−1 in a vacuum (<1 hPa) to improve the stability and to reduce water vapour in the system. They were recorded using RT InGaAs and RT silicon (Si) detectors. The recorded signal (interferogram) was stored in DC mode in order to make corrections for the solar intensity variations (Keppel-Aleks et al., 2007). The interferogram upon DC correction was then Fourier-transformed to get the corresponding spectrum. Column abundances of CO2, CH4, CO, N2O, H2O, HDO, O2, and HF were retrieved from the spectra based on the TCCON GFIT retrieval code GGG2014 software version (Wunch et al., 2015). The instrument was also equipped with a liquid-nitrogen-cooled (LN2) InSb detector. This detector enhances the possibilities to expand the wavelength region covered by the instrument (see Table 2) and to retrieve more atmospheric species. In addition to the TCCON and InSb measurements, the instrument was also used to record double-sided DC coupled interferograms at 0.5 cm−1 using the InGaAs detector. These measurements are henceforth called HR125LR. These measurements provide low-resolution data sets from the same TCCON instrument to be compared to the results of the other tested low-resolution instruments. The sequence of measurements was as follows. First, one InGaAs–Si forward–backward scan (standard TCCON measurement) was recorded. Then, two forward–backward HR125LR scans were recorded, and after that was one standard TCCON measurement and two forward–backward HR125LR scans, followed by one InSb forward–backward scan. This cycle was repeated for the whole measurement day. This paper focuses on the measurements performed with only the InGaAs detector (standard TCCON and HR125LR data sets). The instrument was operated in an automated way with the possibility of manual intervention. The ILS characterisation was performed using a HCl (hydrogen chloride) gas cell following the recommendations of TCCON (Hase et al., 2013) using the LINEFIT software (Hase et al., 1999).
2.2.2 Bruker EM27/SUN
The EM27/SUN spectrometer was developed by the Karlsruhe Institute of Technology (KIT) in cooperation with Bruker starting in 2011 (Gisi et al., 2012). The spectrometer has been available as a commercial item from Bruker since 2014, and an additional channel for CO detection was assigned in 2016 (Hase et al., 2016). Today more than 40 units are already being operated by working groups around the globe (Frey et al., 2019). The EM27/SUN used during the campaign was provided by KIT. The EM27/SUN records double-sided DC coupled interferograms making an average of 10 scans in about 58 s at a spectral resolution of 0.5 cm−1. A double-sided recording of the interferograms largely reduces the sensitivity to residual phase error. The measurements were performed using an RT InGaAs detector (5500–11 000 cm−1) and a DC coupled wavelength-extended RT InGaAs detector (4000–5500 cm−1) (Hase et al., 2016). In this extended configuration, the EM27/SUN covers the same spectral region as TCCON and encompasses the spectral section as observed by the TROPOspheric Monitoring Instrument (TROPOMI) (Hasekamp et al., 2019; Landgraf et al., 2018). Spectra were generated from raw interferograms using the preprocessor tool developed by KIT in the framework of the COCCON-PROCEEDS project funded by the European Space Agency (ESA). Column abundances of CO2, CH4, CO, H2O, and O2 were retrieved from the resulting spectra using the PROFFAST retrieval code. PROFFAST is a code for retrieving trace gas amounts from low-resolution solar absorption spectra. It was developed on behalf of the ESA in order to provide an open-source and freely available code (without any licensing restrictions) as required by the growing COCCON user community, e.g. for TROPOMI validation work. It is a least-squares fitting algorithm, which adjusts the trace gas amounts by scaling atmospheric a priori profiles. The retrievals are performed on spectra generated with the included PREPROCESS tool. This tool produces spectra from the measured DC coupled EM27/SUN interferograms. It includes a DC correction of the interferogram, a dedicated phase correction scheme for double-sided interferograms, and several quality control tests (e.g. testing for the presence of out-of-band artefacts). The lookup table for cross sections used by PROFFAST is created on the basis of HITRAN spectroscopic line lists: for H2O, CH4, and N2O, HITRAN 2008 line lists are used (in the case of H2O including some minor empirical adjustments); for CO2 and CO, HITRAN 2012 line lists are used. PROFFAST uses the solar line list compiled by Geoff Toon, JPL, for GGG2014. In contrast to the TCCON GGG2014 processing, the empirical air-mass-independent and air-mass-dependent post-calibrations are applied species-wise including molecular oxygen. Thereby, the Xair equivalent provided by PROFFAST is on average normalised to unity, while it remains an uncalibrated intermediate result in GGG2014, which calibrates only the Xgas results. The PROFFAST approach of calibrating Xair is transparent for users, as the calibration factors can be directly related to deviations of the spectroscopic band intensities, and gives the user a more sensitive diagnostic tool at hand, as air-mass-dependent artefacts in the reported quantity are also reduced. The XCO2 and XCH4 products are bias-corrected based on the extensive COCCON development. The bias correction is only done for the EM27/SUN and not for any other test data sets. The PROFFAST and the PREPROCESSOR tools can be downloaded from the KIT web page at http://www.imk-asf.kit.edu/english/3225.php (last access: 10 July 2020). The characterisation of the ILS was performed using an open-path measurement as described in Frey et al. (2015). The solar tracker of the EM27/SUN is attached to the body of the spectrometer. It was operated outside the FRM4GHG laboratory container at ambient conditions for the whole campaign period. This mode of deployment showed the capability of the instrument to be operated even under harsh campaign conditions. The day-to-day instrument operation was performed by KIT with local support from FMI for some measurement days. Once deployed, the instrument operation is automated. The EM27/SUN was supported by a pressure sensor and a GPS sensor for accurate timekeeping and position acquisition.
2.2.3 Bruker Vertex70
The Vertex70 spectrometer was purchased from Bruker to take part in the campaign. It records single-sided DC coupled interferograms making an average of two scans in about 17.3 s at a spectral resolution of 0.2 cm−1. The intensity of the interferogram varies during the scan, and the incident angle on the two interferometer mirrors of the pendulum changes during the scan due to the large optical path covered by the pendulum drive, leading to self-apodisation. Both these factors were taken into account while performing the retrieval. Several scans were co-added for one measurement (∼2.5 min) with a comparable signal-to-noise ratio (SNR) to the reference TCCON measurements. The Vertex70 has the advantage of accommodating and measuring with two detectors covering a wide spectral range. An extended RT InGaAs detector (3500–15 000 cm−1) and an LN2-cooled InSb detector (2500–10 000 cm−1) were used. This paper focuses on the measurements performed with only the InGaAs detector. The GFIT retrieval code was used to analyse the measured spectra and retrieve column abundances of CO2, CH4, CO, H2O, and O2. The characterisation of the ILS was performed using an HCl gas cell similar to TCCON. The Vertex70 was operated from inside the dedicated FRM4GHG air-conditioned laboratory container regulated at about 20 ∘C, with the solar beam being fed to the instrument using a homemade BIRA-IASB solar tracker mounted on top of the container. The distance between the solar tracker and the spectrometer was 3 m. The tracking of the sun was performed using a camera-based active feedback option. The instrument operation was automated using the BARCOS system (Neefs et al., 2007) and a homemade automated control unit system built by BIRA-IASB with the possibility of a manual intervention at any time. The solar tracker was equipped with sun intensity and rain detection sensors, which facilitated the automatic opening and closing of the solar tracker cover depending on the weather conditions. This facilitated performing atmospheric measurements on every occasion with good weather conditions. The data analysis was performed by the University of Bremen, and maintenance was shared between BIRA-IASB and the University of Bremen.
2.2.4 Bruker IRcube
The IRcube is a compact portable FTS manufactured by Bruker Optics. It records single-sided DC coupled interferograms using an RT-extended InGaAs detector (4500–15 000 cm−1) making an average of 33 scans (17 forward and 16 backward) in about 1.7 min at a spectral resolution of 0.5 cm−1. It has an internal full angle FOV of 72 mrad. The novel design of the IRcube for this field campaign was the use of a fibre-optic feed from an independent solar tracker (STR-21G, Eko Instruments Co., Ltd. of Japan) mounted on top of the FRM4GHG laboratory container to receive the solar beam. A 50 cm focal length F/5 telescope (glass lens) focuses the solar beam onto a 20 m long, 600 µm core fibre with a numerical aperture of 0.22. This defines the external FOV on the solar disc at 1.2 mrad. The coupling of light from the optical fibre to the IRcube was chosen to optically match the input optics of the IRcube as closely as possible by coupling the power from the fibre-optic cable to the spectrometer so that the signal-to-noise ratio is comparable to TCCON, while avoiding unwanted spectral features that are present in NIR optical fibres. There is a limited range of numerical apertures commercially available, out of which the best compromise for the IRcube with good spectral characteristics was the low-OH Thorlabs FG550LEC. A glass lens and aperture in front of the IRcube refocus the solar beam from the fibre into the entrance aperture (0.5 mm). A small part of the main beam reflected from the CaF2 entrance window was used to monitor the solar radiation for cloud filtering. The IRcube can be housed anywhere within the length of the fibre-optic cable (here 20 m). This design concept is of significant importance for certain applications for which the spectrometer can be placed far away from the solar tracker, e.g. inside a weather proof enclosure. During this campaign the IRcube was set up by the University of Wollongong inside the FRM4GHG container, and the operation of both the tracker and IRcube was automatic. The characterisation of the ILS was performed using an open-path measurement similar to the procedures followed for the EM27/SUN. The data analysis was performed by the University of Wollongong using the GFIT retrieval code.
2.2.5 Laser heterodyne spectroradiometer (LHR)
The LHR is a research instrument developed by the Spectroscopy Group of the Space Science and Technology Department of the Rutherford Appleton Laboratory (RAL) (Weidmann et al., 2007; Tsai et al., 2012; Hoffmann et al., 2016). The principle of operation is similar to that of a heterodyne radio receiver; however, the LHR operates in the mid-infrared region of the spectrum. The benefits of such an approach to spectroscopy include (i) high spectral resolution (up to >500 000 resolving power), (ii) ideally shot-noise-limited radiometric noise, (iii) intrinsic narrow FOV, and (iv) scalability down to ultra-miniaturised packages through optical integration.
Compared to the laboratory instrument reported in Hoffmann et al. (2016), the LHR was re-engineered to the requirements of the FRM4GHG campaign with the following modifications: (i) the optical path was reworked to bring the instrument package down to cm3. (ii) A secondary laser channel (to be equipped in future) was integrated. (iii) A thermoelectrically cooled mercury cadmium telluride (HgCdTe) photodiode for photomixing was installed to avoid LN2 usage. (iv) A solar disc imager was installed for FOV monitoring and optional solar tracking operations. (v) Acquisition as well as instrument control hardware and software were integrated to allow full unattended operation, except for switch-on and switch-off procedures.
The LHR was installed inside the FRM4GHG container and operated under ambient conditions. The incoming solar beam had a 12 mm diameter and was side-sampled from the BIRA-IASB solar tracker. The LHR has no entrance window. Inside the instrument, the incoming beam is split into a transmitted mid-infrared component for heterodyning and a visible component for solar imaging. To that end, a germanium (Ge) long-wave infrared bandpass filter is used. To carry out the fine spectral analysis, the incoming mid-IR field is superimposed with that of an optical local oscillator by a zinc selenide (ZnSe) beam splitter. The local oscillator consists of a continuously tunable semiconductor laser source, in this case a quantum cascade laser, operating in the narrow spectral range between 952 and 955 cm−1 (v1←v3 CO2 band) optimised through prior analysis for atmospheric state retrieval information. The spectra were resolved through the local oscillator continuous frequency tuning. The superimposed atmospheric and local oscillator beams are mixed onto the high-speed photodiode, effectively transposing the middle infrared spectral information into the radio-frequency (RF) domain. The spectral resolution is determined by electronic filters. For the FRM4GHG campaign, the spectral resolution was set to 0.02 cm−1. Each spectrum was recorded over 30 s. The start and stop operation of the LHR was performed manually by the local support staff at the measurement site. A typical atmospheric spectrum showing the CO2 window as measured by the LHR can be seen in Fig. 6 in Hoffmann et al. (2016). The data analysis was performed by the RAL team using the optimum estimation atmospheric retrieval method, in which the Reference Forward Model was used (Dudhia, 2017).
2.2.6 AirCore
The AirCore is a novel innovative technique to sample high-altitude profiles of atmospheric concentrations of trace gases. A detailed description of the technique can be found in Karion et al. (2010). The AirCore system used for this campaign was originally built by the University of Groningen (RUG) and was further developed together with the Finnish Meteorological Institute (FMI). The total length of the AirCore is 100 m. It consists of two types of stainless-steel tubing with outer diameters of and . The vertical resolution of measurements from the AirCore is 13.4 mbar for ambient pressures between the surface and 232 mbar, and it is 3.9 mbar for ambient pressures lower than 232 mbar. A custom-made data logger by FMI was used to record the temperature and ambient pressure of the AirCore tubing. An automatic valve was developed and installed prior to the campaign, which closed the inlet valve of the AirCore system upon landing. The AirCore was packed in a styrofoam box to protect it from damage during landing, with its inlet valve protruding through the styrofoam box. Magnesium perchlorate (Mg(ClO4)2) was used as a dryer in the AirCore. The AirCore package includes tubing, connectors, valves, a data logger, and a box. The air volume of the AirCore is approximately 1400 mL. The AirCore was launched hanging on a 3000 g meteorological balloon (Totex TX3000). The payload included a Vaisala RS92-SGPL radiosonde (Dirksen et al., 2014), an iridium and GPS–GSM positioning device, and a lightweight transponder. The balloon burst after reaching the ceiling height (typically about 30–35 km). A large parachute was used to slow down the descent speed of the AirCore while a tracking system located its position. Upon landing, the AirCore was recovered and brought to the laboratory to obtain mole fractions of CO2, CH4, and CO with a Picarro G2401-m cavity ring-down spectrometer (CRDS). The precision and accuracy for CO2, CH4, and CO are 0.05 ppm and 0.1 ppm, 0.5 ppb and 1 ppb, and 8 ppb and 3 ppb, respectively. An orifice (Sapphire, Type A, size 0.18 mm) was placed between the pump and the analyser to achieve a constant flow of 40 mL min−1. The sample was analysed starting from the stratospheric part (the closed end) to minimise the diffusion. Before each flight, the AirCore was flushed with dry air from a fill cylinder for several hours. This procedure dries the inner surface of the AirCore and fills it with air of known mole fractions. The mole fraction of CO in the fill cylinder was ∼12 ppm. The fill air was used as an indicator of air mixing and as a diagnostic tool. Radiosonde (Vaisala RS92-SGPL) ambient pressure, temperature, and AirCore temperature were available for each AirCore flight. AirCore vertical profiles were retrieved based on the measured time series of mole fractions and the recorded in-flight information, e.g. coil temperature, ambient pressure, and ambient altitude, using a custom-made retrieval software by RUG.
2.2.7 In situ
The in situ measurements used for this work were provided by the FMI. The concentrations of CO2, CH4, and CO were measured on a 50 m tower at three levels (2, 22, and 48 m) above the surface using a Picarro G2401 system. More information about the site can be found on the web page at http://fmiarc.fmi.fi/index.php (last access: 1 October 2019).
3.1 Measurement set-up
The campaign took place between March and October 2017. The site is located at high latitude; therefore, it was not possible to measure beyond this period due to the high solar zenith angle (SZA). Solar measurements were recorded between sunrise and sunset, depending on the SZA limits set by the local scene and weather conditions (cloud, fog, and strong winds). The FMI team monitored the operation of the instruments during the campaign period. Depending on the weather conditions, all spectrometers performed as many measurements as possible to improve the measurement statistics. The measurements preformed helped to observe the diurnal variation of the target gases. The campaign began with an initial blind intercomparison phase during which the instruments were operated with the optimised settings best known to their PIs to get a good SNR comparable to the TCCON instrument. The measurements performed by the different remote sensing instruments were submitted to the chosen referee BIRA-IASB.
The intercomparison study of the blind phase showed that the Vertex70 instrument was not optimised and needed a modification. The aperture was reduced on 6 July 2017 such that the beam diameter changed from 40 mm to 20 mm, reducing the intensity of the light reaching the detector. This helped to reduce the scatter in the retrieved column values by ensuring the operation of the instrument in the linear region of the detector. This configuration was used until almost the end of the measurement period when the aperture stop was further reduced with an iris to 9 mm. However, we did not have any solar measurements with this setting due to unsuitable weather conditions.
The IRcube did not have to undergo any internal modifications; however, an optical fibre which was broken on 23 March 2017 was replaced in April 2017, and the measurements resumed as of 25 April 2017. The first optical fibre used for the IRcube was an ultra-low-OH silica optical fibre from Polymicron Technologies, part FIA8008801100 with a numerical aperture of 0.22 and a core diameter of 800 µm. Due to a long delivery time of this optical fibre, a replacement optical fibre, as discussed in Sect. 2.2.4, was ordered and used from the end of April 2017.
The EM27/SUN was operated without any modifications during the whole campaign period. The exact dates of all performed modifications are shown in Table 3.
A total of 10 AirCore launches were performed during the campaign, and these were used as an in situ reference data set to better understand the intercomparison of the remote sensing data. Further details are discussed in Sect. 5.2 and 5.3.
3.2 Instrument characterisation
All teams performed a full functionality test of their respective instruments and accessories before shipping and upon arrival at the campaign site in Sodankylä. The functionality test included quality checks and performing ILS measurements of the instruments. These measurements serve as a reference to check the effects (if any) of transport on the instrumental properties and to ensure nominal operation in the case of new set-ups. During the campaign all teams performed ILS measurements when possible to monitor the long-term stability of the participating instruments. The modulation efficiency of the TCCON instrument at the maximum optical path difference (OPD) was <1.02 with a phase error in the range of ±2 mrad throughout the year. The modulation efficiency of the EM27/SUN at the maximum OPD was about 1.02 with a phase error in the range between −3 and 1 mrad throughout the year. The modulation efficiency of the Vertex70 before shipping and upon arrival at the Sodankylä site was about 0.935 at 4.5 cm OPD, and the phase error was changing between −16 and −36 mrad. The modulation efficiency improved significantly from 0.935 to about 0.973, and the phase error improved to about −13 mrad after the modification of the Vertex70 with the introduction of the additional aperture. The IRcube has a modulation efficiency of about 0.95 with the phase error in the range between −5 and +1.5 mrad. A summary of the ILS properties of the FTS is given in Table 3. The ILS of the LHR was determined by the radio-frequency (RF) filter characteristics used to limit the detector bandwidth and hence the spectral resolution of the instrument and is therefore an inherent property of the instrument. A detailed description of the ILS validation of the LHR with C2H4 gas cell measurements can be found in a technical document by Hoffmann et al. (2017). None of the instruments showed any sign of degradation of the instrumental properties during the whole campaign.
The raw measurements (level 0 data) from all participating remote sensing instruments are made publicly available at https://doi.org/10.18758/71021040 (Sha et al., 2018). The atmospheric concentration of the trace gases (level 2 data) together with the auxiliary data are made publicly available at https://doi.org/10.18758/71021048 (Sha et al., 2019). All data sets and the documentation are also made publicly available via the project web page (http://frm4ghg.aeronomie.be, last access: 10 July 2020) and via the ESA Atmospheric Validation Data Centre (EVDC).
5.1 Intercomparison data
Sodankylä is located within the Arctic Circle; therefore, solar measurements with sufficiently low SZA are only possible from the beginning of March to the end of October. During the months of September and October we had a mostly overcast sky. Only 3 d of measurements were possible with the TCCON instrument during this period. However, these measurements were recorded with SZA >75∘.
Based on the measurement capabilities by the individual instruments, the groups were asked to provide some or preferably all of the following parameters: measurement day and time; ground pressure; total column amounts of O2, H2O, CO2, CH4, and CO; and column-averaged dry-air mole fraction of the gas (Xgas) values for XCO2, XCH4, and XCO. Xgas is defined by the following equation:
where 0.2095 is the dry-air O2 mole fraction.
For the Fourier transform infrared (FTIR) instruments the column-averaged dry-air mole fraction of dry air (Xair) was also submitted. Xair is dependent on the total column amounts of measured oxygen, surface pressure, and water vapour. It is calculated following Eq. (3) described in Wunch et al. (2015). Xair is a measure of the instrument's performance and is used by TCCON to examine station-to-station biases. Ideally, the Xair values should be 1 for measurements of total column amounts of oxygen with accurate spectroscopy, surface pressure, and water vapour retrievals. Typical Xair values for TCCON measurements are 0.98, which is because of a 2 % bias in the O2 spectroscopy. A summary of the data sets and the corresponding retrieval methods is provided in Table 2. The spectrometers used an identical set of ground–pressure data collected at the Sodankylä site for the retrieval. The Xgas values, which were calculated using GFIT, were scaled to the WMO standards using the calibration factors used by TCCON and as discussed in Wunch et al. (2015). The recent values of the correction factors (air-mass-dependent correction factor, ADCF, and air-mass-independent correction factor, AICF) for the respective gases were taken from Table 4 in Wunch et al. (2015). The scaling factors for the Xgas values, which were calculated using PROFFAST for the EM27/SUN, are discussed in detail in Frey et al. (2015).
All interventions performed on the respective instruments and as discussed in Sect. 3.1 are marked in the time series plots with vertical lines and colours corresponding to the respective instrument. The dates are given in Table 3. In the following sections the intercomparison results will be shown, the long-term stability will be discussed, and cases in which clear deviations of the retrieval results from the participating instruments with respect to the reference data set are observed will be explained.
5.2 Detector non-linearity effects
The reference measurements performed with the Bruker IFS 125HR during the campaign in 2017 are found to be affected by the non-linearity of the InGaAs detector. The non-linearity was identified towards the very end of the campaign in 2017 while checking the interferogram signal measured by the TCCON and comparing it to the EM27/SUN. The detector non-linearity is dependent on the photon load incident on the detector and influences the Xgas values dependent on the signal strength of the measurements. The non-linearity being a signal-dependent function, it can be avoided by keeping the signal level within the linear domain of the detector. To test the non-linearity, a metal grid was placed in the parallel light beam at the entrance port to reduce the signal by about 20 %. Figure 1 shows two spectra measured with the standard TCCON configuration with no grid (red) and with a grid (black) placed in the parallel light beam. These spectra cover the complete spectral regions measured by the detector and are zoomed in to highlight the signal of the out-of-band spectral regions. The non-linearity effect leads to out-of-band artefacts in the spectrum falsely indicating the presence of energy where the detector is insensitive. The signal between 0 cm−1 and the lower cutoff of the detector at 4000 cm−1 as well as the signal between the upper cutoff at about 12 000 cm−1 and the end of the detector bandpass at about 16 000 cm−1 show non-zero values for the no-grid case, indicating that the measurements performed were affected by the detector non-linearity. However, the measurements performed with the reduced intensity by introducing the grid in the parallel beam do not show such high out-of-band intensities. The lower-wavenumber out-of-band region shows only noise values, and the higher-wavenumber region close to the detector bandpass shows values which are higher than the noise but much lower than the signal of the standard measurements. These higher values can be explained by the presence of unintended double passing of the infrared beam in the interferometer that occurs if some radiation is reflected back from the detector system. The presence of the signal, as a result of this double passing, is superimposed onto the non-linearity artefact of the detector in this wavenumber region, which makes this spectral region unusable for the determination of non-linearity. The high signal in the out-of-band spectral regions confirms that the TCCON measurements performed during 2017 are affected by the detector non-linearity. A correction method has been developed based on the method described in Hase (2000, chap. 5); it has been tested and applied to the TCCON data. The results of this are shown in Appendix A. The non-linearity-corrected TCCON data are henceforth referred to as TCCONmod in this paper. The AirCore measurements performed during the campaign were used to compare with the TCCON and TCCONmod data sets. These results are discussed further in the next section.
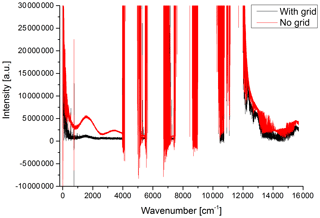
Figure 1Standard spectrum (red) recorded with the Bruker IFS 125HR at the Sodankylä TCCON facility. Spectrum (black) recorded with a grid placed in the parallel optical light path, showing a reduction of the non-linearity features in the out-of-band spectral regions. For comparison, the maximum intensity of both spectra (not visible in the plot) have been normalised to the same value.
Intercomparison results of the Xgas calculated from AirCore relative to the TCCON and TCCONmod data set
AirCore measurements performed in 2017 at the Sodankylä site are listed in Table 4. The retrieval of the TCCON and TCCONmod data set was performed using the TCCON a priori. The daily a priori files were automatically generated during the GFIT run. In addition, the tool to generate the daily TCCON a priori for any given location is available using a stand-alone programme via a DOI link provided by Toon and Wunch (2017). The AirCore measurements are in situ measurements of the targeted species calibrated to the WMO scale and serve as a better reference for the vertical profile of the measured species. However, the AirCore profiles are limited to a vertical sampling height of about 25–30 km depending on the ceiling height reached by the launching balloon. Given this height limitation, the AirCore profiles cover only a part of the atmosphere relative to the TCCON a priori profile, which covers a larger range starting from the site altitude up to 70 km. The lowermost layer of an AirCore profile is contaminated as the sampled air of the lowermost part of the atmosphere gets mixed with the reference push gas. The push gas is needed to let the sampled air pass through the analyser. The in situ measurements performed at 2 m of height above ground level at a nearby forest measurement site of the Finnish Meteorological Institute were used to substitute the concentrations of the lowermost layer of the measured AirCore profile. The AirCore profile above the topmost measured layer was further extended by a scaled TCCON a priori profile to cover the missing profile information up to 70 km of altitude. This is equivalent to a filling of <5 % of the total column above the top height of an AirCore measurement. The modified profile constructed using the ground-based in situ measurement, AirCore measurement, and scaled TCCON a priori profile for 3 sample days on 24 April, 15 May, and 28 August 2017 is shown in Fig. 2. The figure shows the measured AirCore profiles (blue rectangles), the a priori profiles from the GFIT run (black plus), the tower mast measurements (green rectangle), and the extended AirCore profiles (red circles) for 3 d. These 3 d were chosen to show the variability of the a priori profile during the different seasons at the Sodankylä site. Panels (a), (d), and (g) represent the data plotted for XCO2; panels (b), (e), and (h) represent the plots for XCH4, and panels (c), (f), and (i) represent the plots for XCO as a function of the altitude for 24 April (a–c), 15 May (d–f), and 28 August 2017 (g–i), respectively.
Table 4AirCore flight performed during the FRM4GHG campaign in 2017 at the Sodankylä TCCON site. Date format: dd/mm/yyyy.
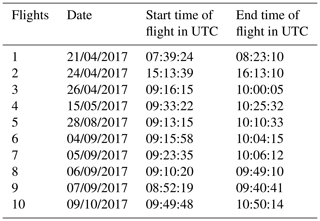
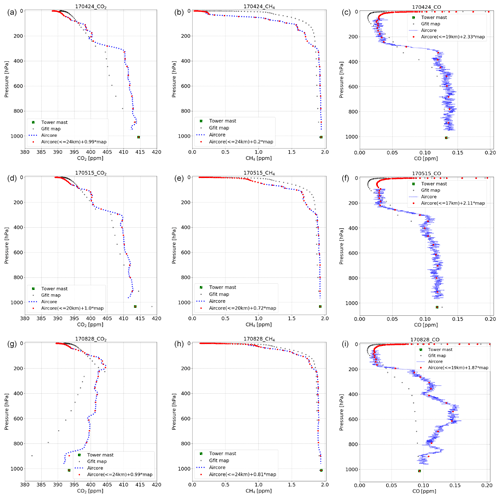
Figure 2AirCore profile, GFIT map profile, AirCore extended profile, and tower mast measurements are plotted for XCO2 (a, d, g), XCH4 (b, e, h), and XCO (c, f, i) as a function of altitude for the following: 24 April 2017 with launch time at 15:13:39 UTC and landing time at 16:13:10 UTC (a–c); 15 May 2017 with launch time at 09:33:22 UTC and landing time at 10:25:32 UTC (d–f); and 28 August 2017 with launch time at 09:13:15 UTC and landing time at 10:10:33 UTC (g–i).
The Xgas values are calculated directly from the modified AirCore profiles by using the TCCON averaging kernels (AKs). These Xgas values are then used to compare to the Xgas values retrieved from the standard TCCON and the non-linearity-corrected TCCON data sets. Any difference in the intercomparison results is a direct reflection of the difference between the measured AirCore profile and the ground-based in situ data relative to the TCCON a priori for the same altitude coverage. The time corresponding to 90 % of the profile (starting at the top of the atmosphere) acquisition time is taken as the AirCore time stamp for the intercomparison of the Xgas values. A 3 h time window around the AirCore measurement time was used as the coincidence limit. All Xgas values from TCCON data and TCCONmod data in this time window were averaged and taken as the coincident data sets for the intercomparison. The 3 h time window was selected for the remote sensing measurement as it is a good representation of the AirCore measurements. Reducing the time window resulted in the reduction of co-located measurement days, and increasing the time window introduced the true variability of the atmospheric state in the remote sensing data.
Table 5Statistics of the intercomparison results for AirCore vs. TCCON and non-linearity-corrected TCCON data sets for measurements performed in 2017 with SZA <75∘ for the TCCON measurements. The values provided are the mean bias ± the standard deviation and the correlation coefficient (r).

The mean bias, the standard deviation of the difference, and the correlation coefficient of the Xgas values calculated from the AirCore relative to the TCCON and the TCCONmod are shown in Table 5. The XCO2 mean bias between AirCore and TCCON is 0.47 ppm with a standard deviation of 0.66 ppm and a correlation coefficient of 0.994. The mean bias is reduced significantly to −0.03 ppm for the intercomparison between the AirCore and the TCCONmod. The standard deviation of the difference is very similar; however, the correlation coefficient improved slightly for the TCCONmod. This shows that the XCO2 values from the TCCONmod data set are a better representation of the true atmospheric state.
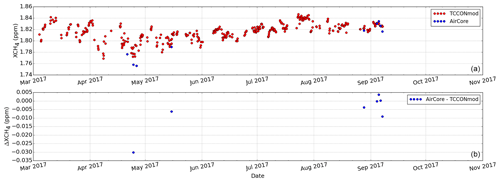
Figure 3Time series of XCH4 retrievals using non-linearity-corrected TCCON and AirCore measurements (a) and bias plot in absolute unit (b) plotted for measurements performed in 2017 at SZA <75∘.
The XCH4 mean bias between the AirCore and the TCCONmod increases to −0.007 ppm compared to the mean bias of −0.004 ppm between AirCore and TCCON. The scatter remains the same, with an improvement in the correlation for the TCCONmod. The improvement in the correlation indicates that the TCCONmod data are a better representation of the true atmospheric state. The increase in the mean bias is due to the difference in the TCCON a priori profiles used for the retrieval relative to the true atmospheric profiles. Figure 3a shows the time series of a 30 min averaged TCCONmod XCH4 data set and XCH4 calculated from the AirCore measurements. Panel (b) shows the difference in the XCH4 bias. The large difference between the two data sets in April is due to the difference between the a priori from the true atmospheric state. The bias is significantly reduced for all later AirCore measurement days.
The XCO mean bias between AirCore and TCCONmod is slightly reduced to 6.25 ppb compared to the mean bias of 6.4 ppb between AirCore and TCCON. The scatter is almost the same, with very similar correlation coefficients. The CO retrieval from the AirCore has a large uncertainty. As a result, the impact due to the change of the data set from the TCCON to the TCCONmod is within the uncertainty budget of the AirCore measurements.
The direct intercomparison results of the Xgas calculated from AirCore relative to the TCCON and non-linearity-corrected TCCON data sets clearly indicate that the non-linearity-corrected data set gives Xgas amounts which are closer to the AirCore amounts and hence closer to our best estimate of the true atmospheric conditions. We will therefore use the TCCONmod data set as our reference data set for further intercomparison studies in the main section of our paper. However, in Appendix B we also show the intercomparison results of the low-resolution measurements relative to the standard TCCON product, which is not yet non-linearity-corrected.
5.3 Intercomparison results using AirCore as a priori profile
The extended AirCore vertical profiles for the targeted gases derived from the AirCore flights have been fed as input a priori profiles for the retrieval of the respective gases from the measurements performed with the remote sensing instruments on the respective days. The retrieval results with the modified AirCore profiles have been given the suffix “AC” at the end of the instrument name. As the remote sensing instruments covered a larger range of SZAs on 15 May and 28 August than on 24 April, those 2 d were selected for the intercomparison study. In order to make the intercomparison, data from each instrument were sorted and all data within the time interval of a 5 min sequence were averaged and associated with the respective start time of the bin. The time stamp of the reference data set (e.g. TCCONmod) was matched with the same time stamp as the other instruments to find the coincident data pairs, which were used for the difference and the correlation calculation.
5.3.1 XCO2 intercomparison results
The intercomparison results for XCO2 retrieved using the TCCON a priori and modified AirCore a priori for the TCCONmod and EM27/SUN data sets are shown in Fig. 4. Panels (a–d) show the results for measurements performed on 15 May and on 28 August 2017, respectively. The same plots for the Vertex70 and the IRcube are shown in Fig. A4. The difference between the TCCON a priori and the modified AirCore a priori profiles is relatively small on 15 May compared to the high difference of the profiles on 28 August (see Fig. 2). This implies that the TCCON a priori is closer to the true atmospheric state on 15 May than on 28 August. As a result, the difference between the standard retrievals from each instrument using the TCCON a priori and the retrievals using the modified AirCore a priori is smaller on 15 May compared to the difference on 2 August. The retrieval results for all instruments for the measurements on 28 August show a bias between the TCCON a priori and the modified AirCore a priori retrievals. The bias shows a strong dependency of the retrieval on the SZA of the measurements. This is due to the TCCON CO2 AK dependence on the SZA as seen in Fig. 6 of Hedelius et al. (2016). With these AKs the a priori information is very relevant. The AirCore a priori is in principle the closest a priori to the truth. When applying the AirCore a priori and doing the retrieval we see that the air-mass dependence is much reduced.
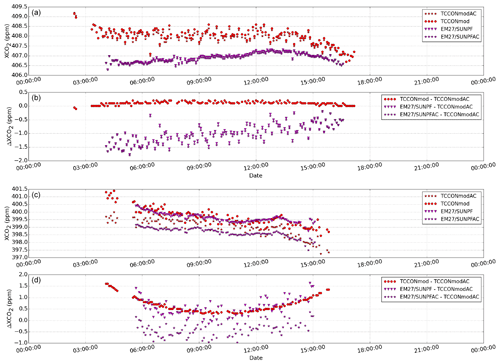
Figure 4(a) XCO2 plotted for TCCONmod and EM27/SUN retrievals with the TCCON a priori and with a modified a priori (calculated using in situ, AirCore, and TCCON map files; labelled with AC at the end) for measurements performed on 15 May 2017 at Sodankylä. Panel (b) shows the difference between the two retrievals in absolute units. Panels (c) and (d) show the same plots as mentioned above for measurements performed on 28 August 2017 at Sodankylä.
For example, the AK values for CO2 for lower altitudes are >1 for measurements performed at higher SZA, which means that the retrieval will overcompensate for any overestimation or underestimation of the a priori: if the a priori is underestimating the lower partial column values in comparison to the true atmospheric state, then these will be overestimated by the retrieval in the total column amount and vice versa; if the a priori overestimates the lower partial columns, then the retrieval will underestimate their contribution to the total column amount. Similar reasoning is applicable to the case in which the AK <1 for lower SZA measurements, typically at local noon. From Fig. 2 we can see that the TCCON a priori underestimates values during the summer months, and therefore the SZA dependence in the bias (TCCONmod – TCCONmodAC) in Fig. 4 can be explained from the shape of the AK; it is higher for the 28 August measurements compared to the 15 May measurements. The intercomparison plots also show the scatter of the retrieval results from the individual instruments for 2 d. The EM27/SUN shows a lower scatter compared to the TCCONmod due to the low noise resulting from the averaging of the individual measurements. Within the period of 5 min, it is possible to average five measurements for the EM27/SUN data set, whereas a maximum of only two measurements is possible for the TCCONmod data set. The Vertex70 measurements on 15 May were performed before the instrument modifications. As a result, a high bias relative to the TCCONmod was seen. This bias is not present for the measurements performed after the instrument modification on 28 August. The scatter in the IRcube and Vertex70 is comparable to the TCCONmod due to the averaging of the similar number of measurements within the 5 min time interval.
5.3.2 XCH4 intercomparison results
The intercomparison results for XCH4 retrieved using the TCCON a priori and modified AirCore a priori for the TCCONmod and EM27/SUN data sets are shown in Fig. 5. Panels (a–d) show the results for measurements performed on 15 May and 28 August 2017. The same plots for the Vertex70 and the IRcube are shown in Fig. A5. The difference between the TCCON a priori and the modified AirCore a priori profiles of CH4 is the highest for 24 April, followed by 15 May, and the smallest for 28 August (see Fig. 2). The vertical distribution of the CH4 concentration during the winter and spring period is poorly modelled by the TCCON a priori tool. The a priori during the summer is in better agreement with the AirCore measurements as seen for the 28 August profiles. As a result, the difference between the standard retrievals from each instrument using the TCCON a priori and the retrievals using the modified AirCore a priori is smaller for 28 August than for 15 May.
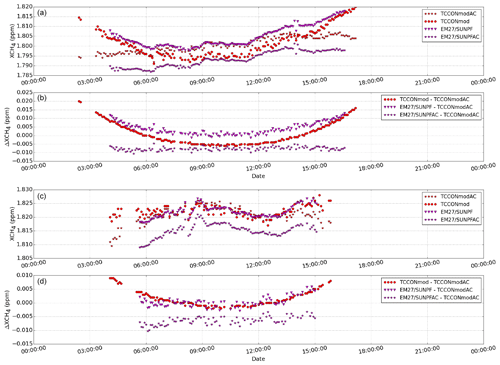
Figure 5(a) XCH4 plotted for TCCONmod and EM27/SUN retrievals with the TCCON a priori and with a modified a priori (calculated using in situ, AirCore, and TCCON map files; labelled with AC at the end) for measurements performed on 15 May 2017 at Sodankylä. Panel (b) shows the difference between the two retrievals in absolute units. Panels (c) and (d) show the same plots as mentioned above for measurements performed on 28 August 2017 at Sodankylä.
The TCCON CH4 AK dependence as a function of the SZA is shown in Fig. 6 of Hedelius et al. (2016). The AK values are >1 for measurements at a lower SZA, which means that the retrieval overestimates the contribution from all layers above 10 km. However, the AK values are <1 for measurements with SZA >65∘, which means that the retrieval underestimates the contribution from all layers above 10 km. The TCCONmodAC results are higher than the TCCONmod results for the lower SZA values and vice versa. This effect is stronger for the retrieval results for 15 May compared to the results of 28 August when the TCCON a priori is closer to the AirCore a priori. The retrieval results for the 15 May measurements for all instruments show a bias between the TCCON a priori and the modified AirCore a priori. The bias shows a strong dependency of the retrieval on the SZA. The EM27 and SUNAC results show a small bias compared to the EM27/SUN. The difference plot shows that the change in the retrieved XCH4 values with the modified AirCore a priori has the same sign compared to the TCCONmod. The same feature is also seen in the Vertex70 and IRcube results. The bias for 28 August is largely reduced compared to that of 15 May. The small remaining bias is due to the difference in the a priori and the AK of the instruments. The AK for the low-resolution instrument, e.g. the EM27/SUN, is shown in the top row of Fig. 6 in Hedelius et al. (2016).
5.3.3 XCO intercomparison results
The intercomparison results for XCO retrieved using the TCCON a priori and modified AirCore a priori for the TCCONmod and EM27/SUN data sets are shown in Fig. 6. Panels (a–d) show the results for measurements performed on 15 May and 28 August 2017. The same plots for the Vertex70 are shown in Fig. A6. The TCCON a priori and modified AirCore a priori profiles of CO for 3 d in 2017 are shown in Fig. 2. The AirCore-measured CO profiles are provided for altitudes up to 17 km and in some cases as high as 19 km. The AirCore profile measured on 28 August captured a large signal in the troposphere, but it is not seen in the TCCON a priori. The TCCON CO prior is a representation of the climatology, so it will generally not capture pollution events. The difference in the profiles in the stratosphere is the largest for 24 April, followed by 15 May, and the difference is the smallest for 28 August. As a result, the difference between the standard retrievals using the TCCON a priori and the retrievals using the modified AirCore a priori is slightly higher for 15 May than for 28 August. The TCCON CO AK dependence as a function of the SZA is shown in Fig. 6 of Hedelius et al. (2016). The AK contribution to the retrieval results is underestimated (AK values <1) for layers below 5 km and overestimated for layers above 5 km with AK values >1, even increasing up to or above 2 for higher layers. The bias dependence on SZA is significant for measurements performed only at high SZA. The TCCONmodAC XCO retrievals show a constant bias relative to the TCCONmod XCO retrievals for most of the SZA, and the deviation is seen only for measurements performed at the high SZAs. The EM27/SUN and the Vertex70 results also show a slight dependency of the XCO retrieved using the TCCON a priori and the modified AirCore a priori on the measurements performed at a high SZA and a constant bias for measurements performed at a low SZA.
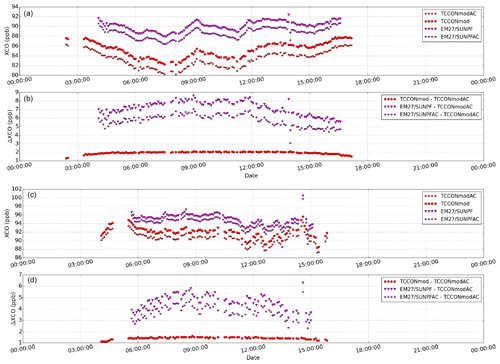
Figure 6(a) XCO plotted for TCCONmod and EM27/SUN retrievals with the TCCON a priori and with a modified a priori (calculated using in situ, AirCore, and TCCON map files; labelled with AC at the end) for measurements performed on 15 May 2017 at Sodankylä. Panel (b) shows the difference between the two retrievals in absolute units. Panels (c) and (d) show the same plots as mentioned above for measurements performed on 28 August 2017 at Sodankylä.
5.4 Methodology for the intercomparisons of the remote sensing data
The data acquisition of the level 2 products was different for each instrument (see Table 2 for details). In order to make the intercomparison, data from each instrument were sorted, and all data within the time interval of a 5 min sequence were averaged and associated with the respective start time of the bin. The time stamp of the reference data set (e.g. TCCONmod) was matched with the same time stamp as the other instruments to find the coincident data pairs, which were used for the difference and the correlation calculation. The TCCON and the low-resolution instruments showed a strong air-mass dependence for measurements with SZA >75∘; these data were therefore not included in this study. Filtering these data removed only a very limited fraction of the data set (about 5 % for EM27/SUN and LHR, about 10 % for IRcube, and about 13 % for Vertex70). Statistical values were computed from the coincident data set to obtain the bias, scatter, and seasonal variation of the individual instruments with respect to a reference data set from the Bruker IFS 125HR. A linear regression line was fitted to the correlation data set for each gas. The slope, intercept, correlation coefficient, and standard error are shown in the respective correlation plots.
5.5 Intercomparisons with reference TCCONmod data
The intercomparison results with the TCCONmod data as a reference and data from other low-resolution remote sensing instruments are discussed in this section species by species. All instruments performed the retrievals following their standard procedure and using the TCCON a priori as the common prior. The statistical values for the intercomparison results (mean of the bias, the standard deviation of the difference, and the Pearson correlation coefficient) are given in Table 6 and plotted in Fig. 11.
Table 6Statistics of the intercomparison results for LHR, Vertex70, IRcube, and EM27/SUN vs. non-linearity-corrected TCCON for measurements performed in 2017 with SZA <75∘. The values provided are the mean bias ± the standard deviation and the correlation coefficient (r). The statistics in the first four rows cover the full year of measurements in 2017, while those in the last four rows are for the measurements performed between 6 July and 12 September 2017.
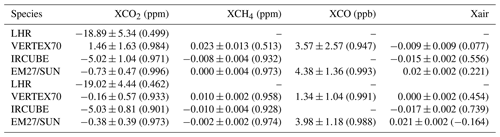
5.5.1 XCO2 intercomparison results
The time series of the coincident XCO2 values measured during the year 2017 by each test instrument and the reference TCCONmod are shown in Fig. 7a. The corresponding differences relative to the TCCONmod are shown in panel (b). The correlation plots between the test instruments and the TCCONmod are shown in Fig. 7c–f. The measured XCO2 values are high during the early winter and low during the summer season, which represents the annual seasonal cycle at the site. All instruments captured the annual summer drawdown.
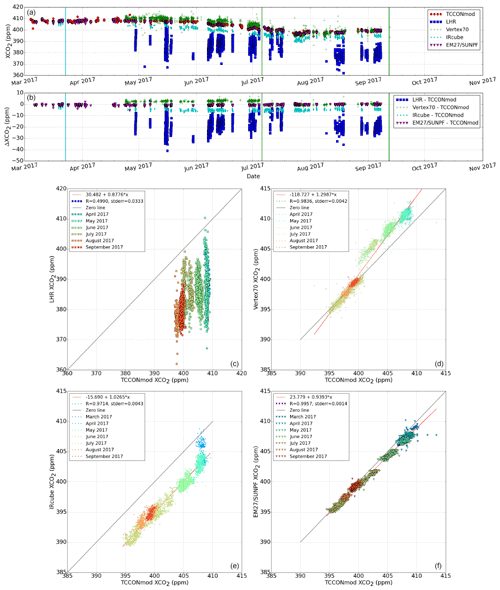
Figure 7Time series of XCO2 retrievals for TCCONmod, LHR, Vertex70, IRcube, and EM27/SUN using the standard procedure with the TCCON a priori for measurements performed at Sodankylä in 2017 (a). The difference of XCO2 time series for each instrument relative to the reference TCCONmod results (b). The correlation plots of XCO2 from LHR, Vertex70, IRcube, and EM27/SUN instruments vs. TCCONmod for all measurements with SZA <75∘: (c) LHR vs. TCCONmod; (d) Vertex70 vs. TCCONmod; (e) IRcube vs. TCCONmod; (f) EM27/SUN vs. TCCONmod. The colours represent the measurements performed during the different months of the year.
Amongst the test FTS instruments, the EM27/SUN has the lowest mean bias of −0.73 ppm with a standard deviation of 0.47 ppm and a very high correlation coefficient of 0.996. The difference plot (Fig. 7b) and the correlation plot (Fig. 7f) show a small seasonal dependency of the bias relative to the TCCONmod.
The correlation plot in Fig. 7e shows a step change in the XCO2 values for the IRcube in March as a result of the replacement of the optical fibre, which caused a change in the ILS of the instrument. The IRcube data show high bias and have a small seasonal dependency. This may be because of the poorly defined ILS due to compact short-focal-length optics or detector non-linearity.
The Vertex70 has also shown a step change relative to the TCCONmod since its modification in July 2017. The data set after the instrument modification shows a significant reduction in scatter and bias compared to the earlier data from the campaign. As a result, data from the period between 6 July and 12 September 2017 are compared separately to characterise the behaviour of the Vertex70 relative to the TCCONmod and the other test instruments. The statistics for the data for the selected period are shown in the lower part of Table 6 and are plotted in Fig. 11. The data from the Vertex70 show a significant reduction in bias from 1.46 to −0.16 ppm and the standard deviation from 1.63 to 0.57 ppm, while the correlation coefficient still remained high. The Vertex70 and EM27/SUN measurements are comparable to each other for this period. The mean bias and the standard deviation of the other instruments are quite similar for the July–September period compared to the full year. However, due to the limited data set, the correlation coefficient is slightly poorer for the shorter period.
The LHR instrument is in its developmental phase and measured only CO2 and H2O. XCO2 data were found to be affected by two clearly different noise processes: a high-frequency random error, mostly determined by detector noise, was found ranging from 2 to 5 ppm (one sigma) depending on the instrument SNR. On top of this random error, large slowly varying diurnal biases were observed to be up to ∼10 ppm. With all biases included and averaged, the biases against the TCCONmod for the full year were found to be ppm. These biases were found to be inherent to the re-engineered LHR instrument in contrast to the better controlled laboratory one (Hoffmann et al., 2016). They are under study; some instrumental ones have been identified to stem from laser optical feedback and laser excess noise, producing a variable offset in the heterodyne demodulated signal.
5.5.2 XCH4 intercomparison results
The time series of the coincident XCH4 values measured during the year 2017 by each test instrument and the reference TCCONmod (panel a), the corresponding differences relative to the TCCONmod (panel b), and the correlation plots (panels c–e) are shown in Fig. 8. XCH4 values are high during the late winter, followed by a dip during the spring and further rise during the summer period. The annual cycle can be seen for the TCCONmod, EM27/SUN, and IRcube measurements. The Vertex70 data, after the instrument modification in July, are also representative of the TCCONmod data set.
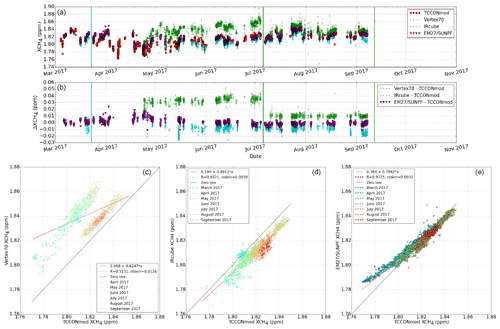
Figure 8Time series of XCH4 retrievals for TCCONmod, Vertex70, IRcube, and EM27/SUN using the standard procedure with the TCCON a priori for measurements performed at Sodankylä in 2017 (a) and the difference of XCH4 time series for each instrument relative to the reference TCCONmod results (b). The correlation plots of XCH4 from Vertex70, IRcube, and EM27/SUN instruments vs. TCCONmod for all measurements with SZA <75∘: (c) Vertex70 vs. TCCONmod; (d) IRcube vs. TCCONmod; (e) EM27/SUN vs. TCCONmod. The colours represent the measurements performed during the different months of the year.
The EM27/SUN has the lowest mean bias of zero with a standard deviation of 0.004 ppm and a correlation coefficient of 0.973. The difference plot in Fig. 8b shows that both the EM27/SUN and IRcube have a high bias of about 0.01 ppm with respect to the TCCONmod in the period between early March and the end of May. Also, the correlation plots relative to the TCCONmod shown in Fig. 8d and e for the IRcube and the EM27/SUN show the monthly deviation very clearly.
The Vertex70 data show a step change in bias of about 0.03 ppm and a significant reduction in the measurement standard deviation after the instrument modification. The statistical values for all instruments between 6 July and 12 September 2017 are shown in Table 6 and are plotted in Fig. 11. The Vertex70 data have a bias of 0.01 ppm, the IRcube data have a bias of −0.01 ppm, and the EM27/SUN data have a bias of −0.002 ppm relative to the TCCONmod. The positive bias of the Vertex70 still remains after the instrument modification and the annual cycle is also captured. The standard deviations of the measurements from the three test instruments are comparable.
5.5.3 XCO intercomparison results
Carbon monoxide is measured by the TCCON, Vertex70, and EM27/SUN instruments. The time series of the coincident XCO values measured during the year 2017 by these instruments (panel a), the corresponding differences (panel b), and the correlation plots (panels c, d) relative to the TCCONmod are shown in Fig. 9. XCO values during the start of the measurement period in late winter are high, followed by a dip during summer and rising values during the late summer period. The annual cycle is seen by all instruments.
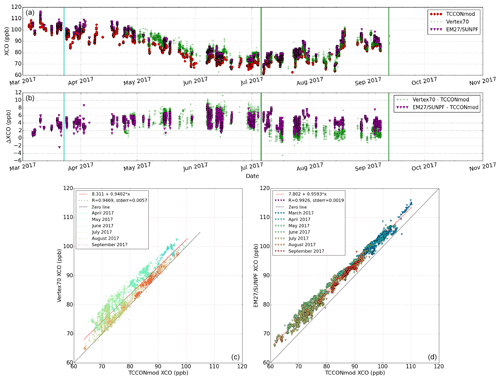
Figure 9Time series of XCO retrievals for TCCONmod, Vertex70, and EM27/SUN using the standard procedure with the TCCON a priori for measurements performed at Sodankylä in 2017 (a) and the difference of XCO time series for each instrument relative to the reference TCCONmod results (b). The correlation plots of XCO from Vertex70 and EM27/SUN instruments vs. TCCONmod for all measurements with SZA <75∘: (c) Vertex70 vs. TCCONmod; (d) EM27/SUN vs. TCCONmod. The colours represent the measurements performed during the different months of the year.
The EM27/SUN has a mean bias of 4.38 ppb with a standard deviation of 1.36 ppb and a high correlation coefficient of 0.993. The difference plot shows that the bias is seasonally dependent with high scatter during the summer period due to measurements with large SZA variation performed on long summer days.
The Vertex70 data show a significant improvement in the scatter after the instrument modification. The statistics showing the mean, standard deviation, and correlation coefficient are given in the bottom part of Table 6. The Vertex70 result has a high correlation coefficient of 0.991 for the period after the instrument modification. The scatter and outliers are reduced; the comparison shows a mean bias of 1.34 ppb and standard deviation of 1.04 ppb relative to the TCCONmod data set.
5.5.4 Xair intercomparison results
Xair values were submitted by all FTIR instruments. The time series of the coincident Xair values for the year 2017 for the instruments are shown in Fig. 10a, and the corresponding differences relative to the TCCONmod are shown in panel (b). Ideally, Xair being the scaled ratio of the surface pressure divided by the retrieved total column of oxygen values should be 1. Any difference relative to the ideal case is an indicator for the instrument and retrieval code performance and the spectroscopy.
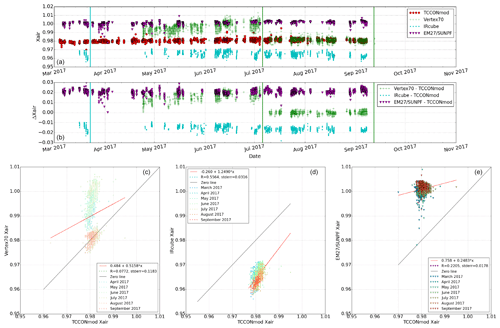
Figure 10Time series of Xair for TCCONmod, Vertex70, IRcube, and EM27/SUN using the standard procedure with the TCCON a priori for measurements performed at Sodankylä in 2017 (a) and the difference of Xair time series for each instrument relative to the reference TCCONmod results (b). The correlation plots of Xair from Vertex70, IRcube, and EM27/SUN instruments vs. TCCONmod for all measurements with SZA <75∘: (c) Vertex70 vs. TCCONmod; (d) IRCUBE vs. TCCONmod; (e) EM27/SUN vs. TCCONmod. The colours represent the measurements performed during the different months of the year.
The Xair values of the Vertex70 show two distinct groups due to the instrument modification in July. After the instrument modification the scatter in the Xair values is significantly reduced. The EM27/SUN shows a slightly lower scatter compared to the IRcube for the full year. However, the scatter of the EM27/SUN, Vertex70, and IRcube is similar for the shorter time period. There is a small offset relative to the TCCONmod. However, the small offset in bias is less important than a stable Xair over the long time series. The correlation plots between the test instruments and the TCCONmod are shown in Fig. 10c–e. Panels (c) and (d) show that the spread of the Xair values on the y axis (representing Vertex70 and IRcube, respectively) is higher than those on the x axis (representing TCCONmod). Panel (e) shows that the spread on the x axis (representing TCCONmod) is similar to the spread on the y axis (representing EM27/SUN) except for a few outliers. The EM27/SUN shows the smallest air-mass dependence, whereas the Vertex70 and the IRcube show decreasing Xair values with an increasing SZA similar to the TCCONmod. This may reflect the difference between the GFIT and PROFFAST results and the use of the different spectroscopic line list as standardly used by the TCCON and COCCON communities.
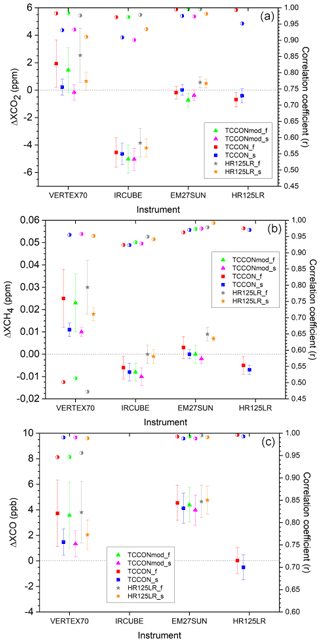
Figure 11(a) XCO2 bias plotted for each instrument relative to non-linearity-corrected TCCON (full year – green triangle, short period – magenta triangle), relative to TCCON (full year – red box, short period – blue box), and relative to HR125LR (full year – grey star, short period – orange star). The correlation coefficients for the respective data set are plotted as half-filled circles and correspond to the right-hand y axis. The XCH4 and XCO biases for each instrument are plotted in panels (b) and (c), respectively. A horizontal dashed line at zero is overlaid on each plot to help in the interpretation of the results.
The Xgas biases between the low-resolution test instruments and the TCCONmod data sets as a reference may be due to effects such as different responses to a priori profiles, interfering species in the retrieval windows, or different averaging kernels. Furthermore, it is important to note that TCCON uses a network-wide constant scaling factor to scale its Xgas values to the WMO standards. The scaling factors specific to each gas for TCCON was determined from several measurement campaigns in which vertically distributed measurements of the gases were performed from airborne platforms using WMO-calibrated instruments. The EM27/SUN uses species-dependent scaling factors for XCO2 and XCH4, which were calculated from long-term intercomparison measurements performed at the Karlsruhe TCCON site. However, no such instrument-specific calibration factors were applied for the other instruments or for the XCO results from the EM27/SUN measurements. This also contributes to the residual bias observed in this intercomparison result. The biases purely due to resolution differences are addressed by performing low-resolution measurements with the same TCCON instrument. These data are then used for an intercomparison relative to the TCCON and for the intercomparison with other low-resolution test instruments. Further details of the intercomparison results are given in Appendix C and D, respectively.
5.6 Humidity dependencies of bias
The presence of water vapour lines in the retrieval windows can lead to errors in the determination of the Xgas values unless they are fitted well in the forward model. It is therefore necessary to check the influence of the water vapour lines for retrievals performed with the low-resolution instruments. Sodankylä is not the most humid TCCON site. The maximum XH2O measured by the TCCON is <6000 ppm during the summer period. In comparison, the TCCON site at Darwin, which is a relatively humid site, shows maximum measured XH2O of <10 000 ppm during the summer period. The year 2017 was relatively dry: the range of XH2O measured at the Sodankylä site was between 500 and 4500 ppm. A detailed discussion of the bias dependence on the humidity present along the measurement line of sight is presented in Appendix F. The results show that the Xgas values derived from the low-resolution instruments during the campaign period showed no dependencies on the humidity along the measurement line of sight.
5.7 Intercomparison of EM27/SUN data processed with PROFFAST and GFIT
So far, the EM27/SUN (COCCON unit) tested in the framework of the campaign has been investigated using the procedures as recommended by the COCCON network, including the consideration of the individual instrumental line shape (ILS) characterisation and the use of the PREPROCESS and PROFFAST processing chain. This seems appropriate because otherwise the steps of the established and previously tested procedure for operating the EM27/SUN within COCCON would be skipped.
On the other hand, the separation of instrumental from processing effects provides additional insights and allows us to estimate the performance of the spectrometer and the processing chain independently. For this purpose, a short comparison between PREPROCESS and PROFFAST versus the GFIT processing suite as used and validated for TCCON is provided in this section. The EM27/SUN GGG interferogram processing suite version 2014 developed by Hedelius and Wennberg (2017) was used for processing the EM27/SUN data.
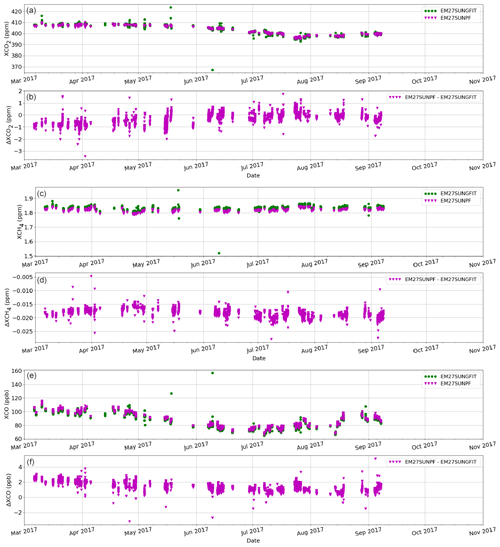
Figure 12Time series of XCO2 (a), XCH4 (c), and XCO (e) retrieved from EM27/SUN measurements processed following the COCCON recommendations (EM27SUNPF) and using GFIT (EM27SUNGFIT) for measurements performed at Sodankylä in 2017. The difference of XCO2 (b), XCH4 (d), and XCO (f) time series for the EM27SUNPF relative to the EM27SUNGFIT analysis as a reference.
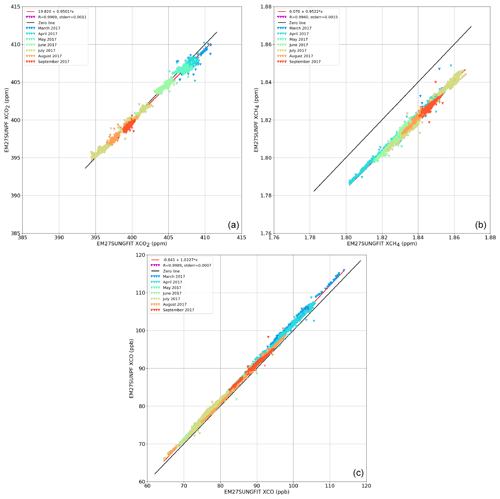
Figure 13Correlation plots for XCO2 (a), XCH4 (b), and XCO (c) retrieved from EM27/SUN measurements processed following the COCCON recommendations (EM27SUNPF) and using GFIT (EM27SUNGFIT) for measurements performed at Sodankylä in 2017.
Table 7Statistics of the intercomparison results for EM27/SUN and non-linearity-corrected TCCON with different retrieval procedures for measurements performed in 2017 with SZA <75∘. The values provided are the mean bias ± the standard deviation and the correlation coefficient (r).

The time series of XCO2, XCH4, and XCO processed following the COCCON recommendations (labelled EM27SUNPF) and using GFIT (labelled EM27SUNGFIT) as well as their respective differences and correlation are shown in Figs. 12 and 13, respectively. The biases between the two approaches (EM27SUNPF – EM27SUNGFIT) are listed in the first row of Table 7. The COCCON (EM27SUNPF) XCO2 is biased low with respect to GFIT by about 0.29 ppm, the XCH4 is biased low by 18 ppb, and the XCO is biased high by about 1.3 ppb. On most days the intra-day random variability (or scatter) is similar for both analyses, but the GFIT analysis includes a larger number of outliers from the daily means (Fig. 12). No consistent reduction of calibration biases with respect to TCCON is achieved by applying GFIT instead of PROFFAST. A detailed study of the code differences is needed to understand the differences and is beyond the scope of this paper. Apart from this, the bias in the correlation is very stable between the two codes without e.g. noticeable air-mass-dependent artefacts or inter-annual drifts. The a priori profile shapes recommended by TCCON are also applied by COCCON, so the smoothing error should largely cancel out, as both codes predict very similar column sensitivities.
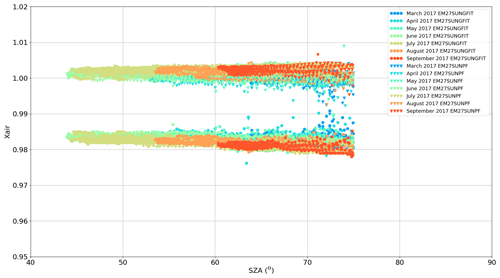
Figure 14Xair plotted with respect to the measurement solar zenith angle for retrievals performed with EM27/SUN data following COCCON recommendations (EM27SUNPF) and using GFIT (EM27SUNGFIT) for measurements performed at Sodankylä in 2017.
The air-mass dependency of Xair retrieved with either code is shown in Fig. 14. The Xair data product is not directly comparable, as PROFFAST applies both an air-mass-independent and an air-mass-dependent correction on Xair. The Xair values for EM27SUNPF are therefore around 1. This is done to exploit this important diagnostic tool in an optimal manner (while GFIT only calibrates the Xgas products for the target gases) – excursions due to instrumental issues can obviously be detected easier in a calibrated Xair product. Moreover, the definition of Xair in PROFFAST differs from GFIT, as the spectroscopically derived air mass is in the nominator and the pressure derived from the in situ measurement is in the denominator, which is the opposite of the convention used in GFIT. Therefore, an excursion towards elevated values in PROFFAST is equivalent to a depression in the value reported by GFIT. The comparison between the two codes looks plausible, as we find the expected larger bias and a stronger air-mass dependency in the uncalibrated GFIT Xair. The calibration chosen in PROFFAST seems to be accurate, with a slight high bias of the order of 0.2 %.
Table 7 presents the biases between the low-resolution results achieved with either PROFFAST or GFIT and the TCCON reference (rows 2 and 3). GFIT applied to the EM27/SUN provides a smaller bias in XCO2 (PROFFAST is biased low by about 0.7 ppm; GFIT is biased low by about 0.4 ppm), while it shows a higher bias in XCH4 (GFIT is biased high by 19 ppb, while no detectable bias is found in the PROFFAST data) and smaller bias in XCO (PROFFAST is biased high by about 4.4 ppb; GFIT is biased high by about 3 ppb).
In summary, the code comparison suggests an excellent performance of the COCCON processing chain. We believe that remaining biases with respect to TCCON can be reduced by further careful adjustment of the calibration factors used in PROFFAST. This work of tying COCCON to TCCON has already been taken up and will be based on several COCCON instruments operated near different TCCON stations in order to minimise the impact of residual instrument or station-specific biases. We are also planning for the realisation of a COCCON travel standard in this context. Based on our results, we recommend using the COCCON workflow for the processing of raw data collected with the EM27/SUN spectrometer.
The FRM4GHG campaign was successfully executed by comparing four portable remote sensing instruments against the reference TCCON instrument at the Sodankylä site during the year 2017. The EM27/SUN was set up every day at the ambient temperature and pressure and was operated without configuration changes during the whole campaign. The other low-resolution FTIR and the LHR were operated from inside a dedicated temperature-controlled container. The instruments needed optimisation and behaved better with a low bias and a high correlation relative to the TCCON instrument afterwards.
In the course of the campaign not only the Vertex70, IRcube, and LHR instruments were improved but also the TCCON instrument by detecting and correcting non-linearity of the detector response. Detecting this issue by comparison with the EM27/SUN shows the potential of this instrument as a travelling standard for TCCON.
The intercomparison results using AirCore profiles as a priori provided interesting insights into the FTS retrievals, its sensitivity to the resolution, and the averaging kernels. The AirCore profiles also showed differences relative to the TCCON a prioris and the resulting biases in the retrievals of the target species. The Xgas calculated from AirCore compared to the TCCON and the non-linearity-corrected TCCON (TCCONmod) data sets show that the latter data set is a better representation of the true atmospheric state.
The EM27/SUN Xgas biases relative to the TCCONmod data were low for the target species except for the high XCH4 bias during the March–May period, which is due to the difference in the sensitivity of the high- and low-resolution instruments and the a prioris not matching well with the actual profile shape. The EM27/SUN results include an instrument-specific bias correction for XCO2 and XCH4 using scaling factors, which was determined independently prior to this study from long-term intercomparison measurements performed at the Karlsruhe TCCON site. It may be that the scaling factor is not optimal for the current location and is also contributing to the bias. This needs to be verified for comparison measurements performed at other TCCON locations. The EM27/SUN Xgas values show high precision and good correlation relative to the reference data sets.
The IRcube Xgas values show relatively high biases, which are related to the possible dependence of the signal level on the extended InGaAs detector known to have non-linearity characteristics. The ILS of the IRcube is also less ideal compared to other larger instruments due to the compact short-focal-length optics. The impact of the ILS on the biases is being further investigated. However, the comparison shows low scatter and a good correlation relative to the TCCONmod data.
The Vertex70 was equipped with an extended InGaAs detector, which led to identifiable non-linearity effects. The optical path was modified by introducing an aperture stop to avoid saturation and operate in the linear region of the detector to improve the ILS. The bias of the Xgas values, the standard deviation of the difference, and the correlation of the modified Vertex70 instrument relative to the TCCONmod data were significantly lower after this instrument modification and comparable to the EM27/SUN results relative to the TCCONmod data.
The LHR was a new instrument deployed for testing during this campaign. It showed large scatter and large biases with a strong diurnal variation relative to the TCCONmod and other FTS instruments. The LHR data for the 2017 campaign are not yet able to provide meaningful geophysical information. However, this comparison has proven to be invaluable to characterise and understand the instrumental biases and possibly the retrieval biases. Both aspects are currently under investigation and improvements are being developed.
The intercomparison results showed that the non-linearity-corrected TCCON data gave a better match to the low-resolution instruments. The standard deviation of the bias and the correlation coefficient are similar for the target species for the non-linearity-corrected TCCON data relative to the standard TCCON data.
The intercomparison results of the EM27/SUN data processed with the COCCON processing chain showed excellent performance in comparison to the retrieval results from the GFIT processing suite. We recommend the COCCON workflow for the processing of raw data collected with the EM27/SUN spectrometer.
The intercomparison results of the low-resolution measurements performed with the Bruker IFS 125HR relative to the standard TCCON and other low-resolution instruments provided a useful analysis of the resolution-dependent effects on the Xgas retrieved for the target gases. The low-resolution measurements performed with the Bruker IFS 125HR also helped to determine that the high bias in the XCH4 during the March–May period was caused by the resolution difference and the corresponding different sensitivities to the vertical profile shape as seen in the averaging kernels.
The air-mass dependence of the retrievals is an effect of the software and spectroscopy. The EM27/SUN and the HR125LR results retrieved with PROFFAST do not show SZA dependence for species to which an air-mass correction factor was applied. Both these data sets were retrieved using PROFFAST. All other results show SZA dependence to some degree. The correction for the SZA dependence is a long-standing and ongoing issue for TCCON that is relevant to all instruments. In order to minimise the effect of the SZA, measurements with SZA <75∘ were used for the intercomparison of the different data sets.
The bias dependence on the humidity along the measurement line of sight was investigated for each target species. However, no dependence was found.
The EM27/SUN, the IRcube, the modified Vertex70, and the HR125LR provided stable and precise measurements of the target gases during the campaign. The portable low-resolution instruments can be used for campaigns or long-term measurements from any site and complement the TCCON network. The Xgas measurements from these instruments will be of similar quality as the TCCON Xgas data.
The TCCON measurements performed during the campaign in 2017 are found to be affected by the non-linearity of the InGaAs detector used for the measurements. A correction method has been developed based on the method described in Hase (2000, chap. 5) to correct the signal in the interferogram domain using the following equation:
where I is the original interferogram and I′ is the non-linearity-corrected interferogram. The coefficients a, b, and c are determined for each measurement by minimising the cost function. The cost function is defined as the ratio of the signal sum in the out-of-band regions covering 100–3600 and 14 200–15 750 cm−1 to the signal sum for the in-band spectral region covering 4100–9700 cm−1. The individual correction functions are plotted in Fig. A1 as red curves, their mean is plotted as blue open circles, and the black line is the fourth-order polynomial fit of the mean with the coefficient values of a=0.00138, b=0.00302, and c=0.0166. The coefficients of the fitted curve representing the averaged correction function are used to correct the non-linearity of all TCCON measurements. Figure A2a and c show the original and the non-linearity-corrected spectra for the measurement day of 6 September 2017. The colours of the spectrum depend on the interferogram maximum signal at the centre burst. The highest values represented by dark red are for measurements performed with the highest solar intensity during noontime, and the measurements performed with the lowest solar intensity are represented by blue as the minimum. Figure A2b and d show the original and the non-linearity-corrected spectra for the out-of-band region between 100 and 3600 cm−1. The signal reduction in the out-of-band spectral region clearly shows that the non-linearity correction worked for the spectra. In order to quantify the effect of the non-linearity correction on the Xgas values, explicit results are shown in detail for 6 September 2017. Figure A3a shows the retrieved XCO2 values from the original spectra (red) and the non-linearity-corrected spectra (black). Panels (b) and (c) show the difference in parts per million (ppm) and the relative difference in percentage for the individual measurements. The non-linearity correction is dependent on the signal intensity of the recorded interferograms. The interferograms with less signal intensity are affected less compared to the signal with high intensity. The maximum of the correction is therefore applied for the measurements with the highest signal during noontime, and the minimum correction is needed for measurements with the lowest signal when the sun is near the horizon.
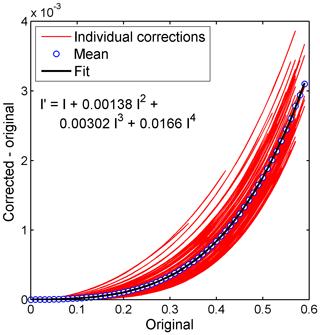
Figure A1Plot showing the difference of the corrected interferograms – original interferograms vs. the original interferograms. The individual corrections are plotted in red, the mean value is plotted as blue open circles, and the black line is the fit.
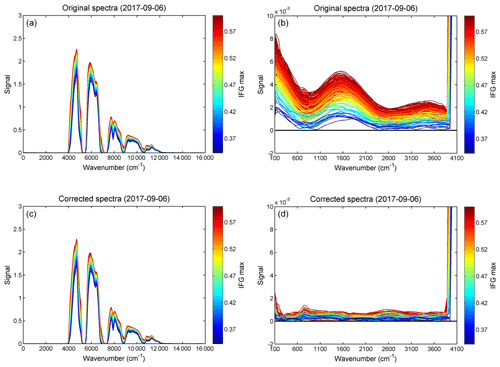
Figure A2Original (a) and non-linearity-corrected (c) spectra; zoom of the out-of-band spectral region (100–3600 cm−1) with the original spectra (b) and non-linearity-corrected (d) spectra from the Bruker IFS 125HR at the Sodankylä TCCON facility. The colour of the spectrum depends on the interferogram maximum signal at the centre burst. The highest values corresponding to dark red are recorded during noontime when the signal is the highest.
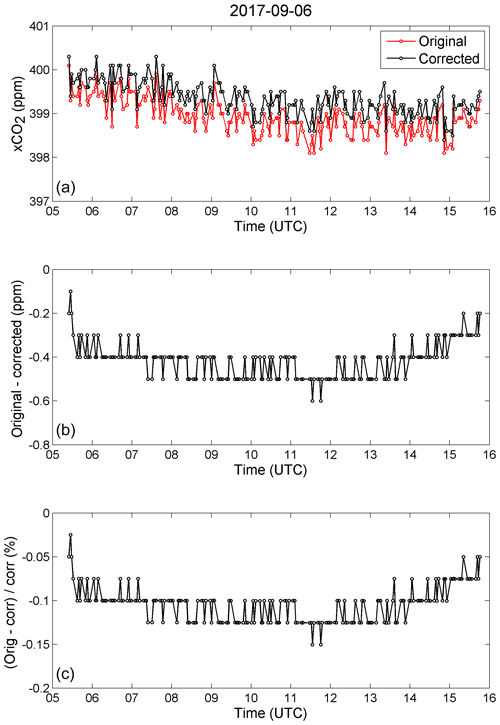
Figure A3(a) Plot showing the original (red) and non-linearity-corrected (black) XCO2 values for 1 d of measurements performed on 6 September 2017 by the Bruker 125HR Sodankylä TCCON instrument. Panel (b) shows the difference between the original and the corrected XCO2 values. Panel (c) shows the relative difference (original − corrected) ∕ corrected in percentage for the XCO2 values.
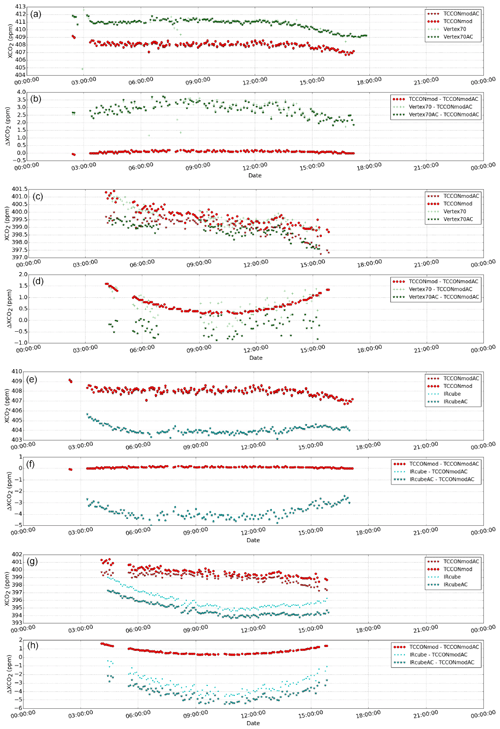
Figure A4XCO2 values plotted for TCCONmod and Vertex70 retrievals with the TCCON a priori and with a modified a priori (calculated using in situ, AirCore, and TCCON map files; labelled with AC at the end) are shown in panel (a), and their difference is shown in panel (b) for measurements performed on 15 May 2017 at Sodankylä. Panels (c) and (d) show the same plots for 28 August 2017. The same plots for TCCONmod and IRcube retrievals for measurements performed on 15 May 2017 are shown in panels (e) and (f), and those for 28 August 2017 are shown in panels (g) and (h).
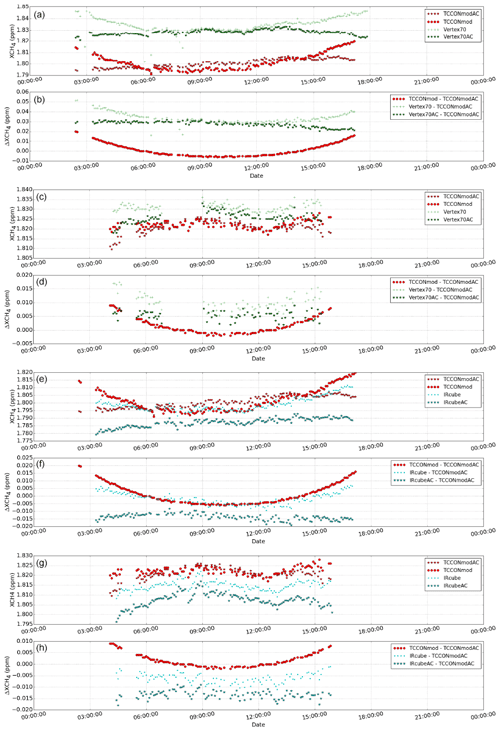
Figure A5XCH4 values plotted for TCCONmod and Vertex70 retrievals with the TCCON a priori and with a modified a priori (calculated using in situ, AirCore, and TCCON map files; labelled with AC at the end) are shown in panel (a), and their difference is shown in panel (b) for measurements performed on 15 May 2017 at Sodankylä. Panels (c) and (d) show the same plots for 28 August 2017. The same plots for TCCONmod and IRcube retrievals for measurements performed on 15 May 2017 are shown in panels (e) and (f), and those for 28 August 2017 are shown in panels (g) and (h).
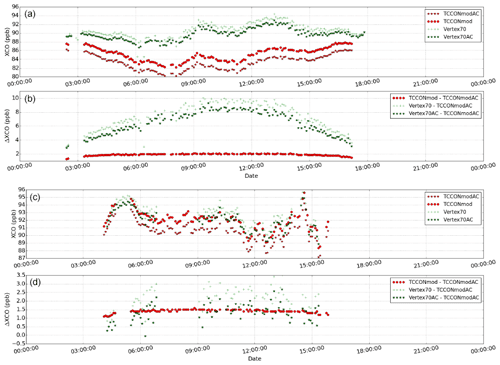
Figure A6XCO values plotted for TCCONmod and Vertex70 retrievals with the TCCON a priori and with a modified a priori (calculated using in situ, AirCore, and TCCON map files; labelled with AC at the end) are shown in panel (a), and their difference is shown in panel (b) for measurements performed on 15 May 2017 at Sodankylä. Panels (c) and (d) show the same plots for 28 August 2017.
In this section the intercomparison results of the low-resolution test instruments are shown in comparison to the standard TCCON data. The mean bias, the standard deviation of the difference, and the correlation coefficient between the individual low-resolution data sets and the TCCON for the full year of measurements in 2017 and for the period between 6 July and 12 September 2017 are shown in Table B1 and are plotted in Fig. 11. The comparison of the shorter time period has been made in order to check the statistics relative to the period during which the Vertex70 was operated with improved settings.
Table B1Statistics of intercomparison results for LHR, Vertex70, IRcube, and EM27/SUN vs. TCCON for measurements performed in 2017 with SZA <75∘. The values provided are the mean bias ± the standard deviation and the correlation coefficient (r). The statistics in the first four rows cover the full year of measurements in 2017, while those in the last four rows are for the measurements performed between 6 July and 12 September 2017.
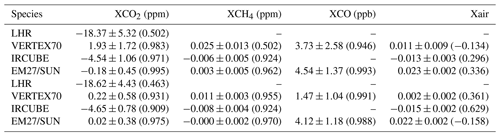
B1 XCO2 intercomparison results
The mean bias changed by −0.5 ppm with a standard deviation change of 0.23 ppm for the intercomparison between the TCCON and the TCCONmod data sets for the full measurement period. The intercomparison of the low-resolution measurements with TCCONmod and TCCON as a reference also shows similar changes in the mean bias values. The standard deviation of the difference and the correlation coefficient remained similar for the intercomparison with TCCONmod compared to TCCON. As an example, the mean bias values for the EM27/SUN changed from −0.18 to −0.73 ppm, the standard deviation of the difference changed from 0.45 to 0.47 ppm, and the correlation coefficient changed from 0.995 to 0.996 for the comparison relative to TCCON; the latter values are for the comparison relative to TCCONmod.
B2 XCH4 intercomparison results
The mean bias changed by −0.003 ppm with a standard deviation change of 0.001 ppm for the intercomparison between the TCCON and the TCCONmod data sets for the full measurement period. The intercomparison of the low-resolution measurements with TCCONmod and TCCON as a reference also shows similar changes in the mean bias values. The standard deviation of the difference and the correlation coefficient remained similar or improved slightly for the intercomparison with TCCONmod compared to TCCON. As an example, the mean bias values for the EM27/SUN changed from 0.003 to 0.000 ppm, the standard deviation of the difference changed from 0.005 to 0.004 ppm, and the correlation coefficient changed from 0.962 to 0.973 for the comparison relative to TCCON; the latter values are for the comparison relative to TCCONmod.
B3 XCO intercomparison results
The mean bias changed by −0.14 ppm with a standard deviation change of 0.08 ppm for the intercomparison between the TCCON and the TCCONmod data sets for the full measurement period. The intercomparison of the low-resolution measurements with TCCONmod and TCCON as a reference also shows similar changes in the mean bias values. The standard deviation of the difference and the correlation coefficient remained similar for the intercomparison with TCCONmod compared to TCCON. As an example, the mean bias values for the EM27/SUN changed from 4.54 to 4.38 ppb, the standard deviation of the difference changed from 1.37 to 1.36 ppb, and the correlation coefficient remained the same at 0.993 for the comparison relative to TCCON; the latter values are for the comparison relative to TCCONmod.
The above results show the difference of the TCCON data relative to the TCCONmod and the intercomparison with respect to the low-resolution data sets. This difference of the TCCON data has to be taken into account when using the official TCCON data set until the non-linearity-corrected results are uploaded. The standard deviation of the difference and the correlation coefficient remained similar or improved slightly for the target species for the TCCONmod case. The TCCONmod data set is a better representation of the true atmospheric signal. Having this method implemented and tested for 1 year of data during this campaign will help in dealing with many years of historic TCCON data measured at the Sodankylä site. The HR125LR data were compromised by the non-linearity in a similar way as the high-resolution TCCON spectra.
The Bruker IFS 125HR was configured to record regular low-resolution measurements (spectral resolution of 0.5 cm−1) together with the standard TCCON measurements (spectral resolution of 0.02 cm−1). The low-resolution measurements are henceforth referred to as HR125LR. The low-resolution measurements (HR125LR data set), which are similar double-sided interferograms as the EM27/SUN, were processed by the KIT group using PROFFAST. The results were post-processed in the same way as the results of the EM27/SUN. The comparison results with TCCON data as a reference and HR125LR data recorded with the same instrument are discussed in this section species by species.
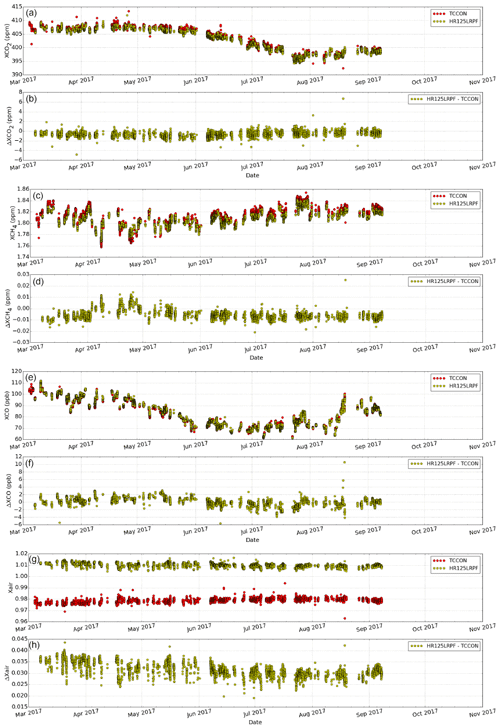
Figure C1Time series of XCO2 (a), XCH4 (c), XCO (e), and Xair (g) retrievals for TCCON and HR125LR using the standard procedure with the TCCON a priori for measurements performed at Sodankylä in 2017. The difference of XCO2 (b), XCH4 (d), XCO (f), and Xair (h) time series for HR125LR relative to the reference TCCON results.
Table C1Statistics of the intercomparison results for HR125LR vs. TCCON for measurements performed in 2017 with SZA <75∘. The values provided are the mean bias ± the standard deviation and the correlation coefficient (r). The statistics in the first row cover the full year of measurements in 2017, while those in the second row cover the measurements performed between 6 July and 12 September 2017.

The time series of the coincident XCO2, XCH4, XCO, and Xair values measured during the year 2017 for the HR125LR and the reference TCCON instrument are shown in Fig. C1a, c, e, and g. The corresponding differences for each species relative to the TCCON data are shown in panels (b), (d), (f), and (h) respectively. The mean bias, the standard deviation of the difference, and the correlation coefficient between HR125LR and TCCON data sets for the full year of measurements in 2017 and those for the period between 6 July and 12 September 2017 are given in Table C1 and are plotted in Fig. 11. The shorter time period was chosen in order to compare the results with the improved Vertex70 instrument.
C1 XCO2 comparison results
The seasonal cycle, including the summer drawdown of CO2, was captured well by the HR125LR. The mean bias for the full year and the shorter period of measurements are −0.69 and −0.4 ppm, respectively. The relatively small difference in the bias for the two timescales indicates that the bias is quite constant over the year. The high bias of −0.69 ppm may be due to the choice of the constant scaling factor used for the calculation of the XCO2 values for the HR125LR data set. The same calibration factors as used by the EM27/SUN were used for the scaling of gases retrieved from the HR125LR measurements. However, these calibration factors are specific to the EM27/SUN and therefore may not be accurate for the HR125LR. This is the reason for the high bias, which is understood and not a problem as long as it remains constant and does not vary over the season. The difference plot (Fig. C1b) shows that there is a quite constant bias over the whole period with no seasonal dependencies. The standard deviation of the difference (0.53 ppm) and the correlation coefficient (0.993) between the HR125LR and the TCCON data sets are very similar to those when comparing the EM27/SUN and the modified Vertex70 data sets relative to the TCCON data. This implies similar behaviour of the above-mentioned low-resolution instruments.
C2 XCH4 comparison results
The seasonal cycle of CH4 is well captured by the HR125LR except for the March–May period. The mean bias for the full year is −0.005 ppm with a standard deviation of −0.004 ppm and a correlation coefficient of 0.975. The difference plot of the XCH4 values (see Fig. C1d) shows a relatively high bias during the March–May period. This feature is also seen in the intercomparison results between other low-resolution instruments and the TCCONmod (see Fig. 8d). The reason for this is the difference in resolution and the column averaging kernel (AK) between the TCCON and the low-resolution instruments. During the March–May period the TCCON a priori profiles show large differences to the AirCore profiles (see Fig. 2d–f); the latter give a better representation of the true atmospheric state. The AK represents the sensitivity of the retrieved total column to the true partial column profile. An AK value of 1 for all altitudes is the ideal case, which implies perfect sensitivity for the whole atmosphere. In such a case the retrieved total column represents the true atmospheric state. An AK value of <1 or >1 for a given altitude implies that the retrieval underestimates or overestimates the contribution from that particular layer in the total column calculation budget, respectively. The TCCON AK for CH4 as a function of the SZA is shown in Fig. 6 of Hedelius et al. (2016). The AKs for the high SZAs at the lower layers are underestimating and those above the troposphere are overestimating the contribution. Any deviation of the CH4 a priori profile from the true atmospheric state will affect the retrieval results with the higher SZA (mostly during the winter seasons) more compared to those with the lower SZA. The deviation of the TCCON a priori from the true profile in combination with the overestimation of the retrieval values due to AKs >1 for high SZAs during the spring season is the reason for the high bias for the March–May period. This is further discussed in detail in Sect. 5.3. The mean bias of the shorter time period is −0.007 ppm with a standard deviation of 0.002 ppm and a correlation coefficient of 0.97. The bias difference and the low standard deviation for the shorter period are due to the selected data set being outside the March–May period, which does not cover the high values. The standard deviation of the difference between the HR125LR and TCCON data is very similar to those when comparing the EM27/SUN, IRcube, and modified Vertex70 data sets relative to the TCCON data. This again implies similar behaviour of the low-resolution instruments.
C3 XCO comparison results
The seasonal cycle of CO is captured well by the HR125LR. The mean bias for the full year is 0.03 ppb with a standard deviation of 1.02 ppb and a correlation coefficient of 0.996. The values for the shorter time period are similar to those of the full year. However, a slight seasonal dependency is seen. The bias is due to the choice of the scaling factor used for the calculation of the XCO values. The TCCON AK for most of the atmospheric layers overestimates its contribution to the retrieval results. However, the concentration of CO in the atmosphere decreases rapidly with increasing altitude, implying that the contribution in the total column is low and not strongly dependent on the SZA of the measurements. The intercomparison results of the low-resolution data set from all instruments relative to the TCCON data show that their performance was very similar in relation to the standard deviation of the difference and the correlation coefficient relative to the TCCON data.
C4 Xair comparison results
The Xair values for the HR125LR over the whole year are constant with a mean bias of 0.03 relative to the TCCON data and a standard deviation of 0.003. The mean bias for the shorter time period is the same as that for the full year. As the Xair values should be constant over the year, there is no correlation of Xair values expected between the two data sets. This is seen for the shorter time period. The complete time period shows a slightly negative correlation between the two data sets. The constant Xair values over the longer time period show that the performance of the Bruker IFS 125HR operated in the low-resolution mode was stable.
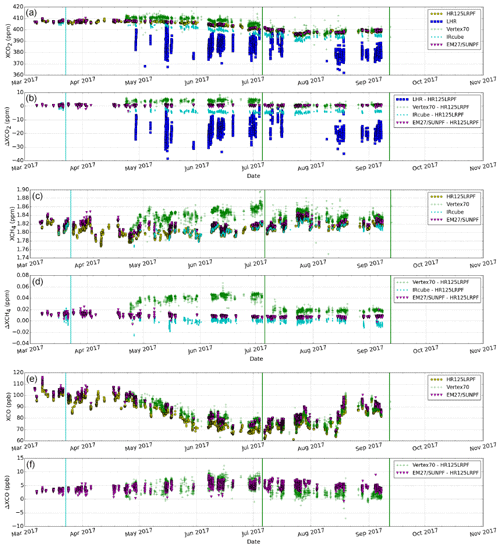
Figure D1Time series of XCO2 (a), XCH4 (c), and XCO (e) retrievals for HR125LR, LHR, Vertex70, IRcube, and EM27/SUN using the standard procedure with the TCCON a priori for measurements performed at Sodankylä in 2017. The suffix PF indicates that the retrievals were performed with PROFFAST code. The difference of XCO2 (b), XCH4 (d), and XCO (f) time series for the test instruments relative to the HR125LR results.
Table D1Statistics of the intercomparison results for LHR, Vertex70, IRcube, and EM27/SUN vs. HR125LR for measurements performed in 2017 with SZA <75∘. The values provided are the mean bias ± the standard deviation and the correlation coefficient (r). The statistics in the first four rows cover the full year of measurements in 2017, while those in the last four rows are for the measurements performed between 6 July and 12 September 2017.
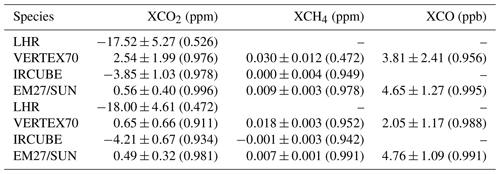
In this section we discuss the intercomparison results between the HR125LR as a reference in relation to other low-resolution remote sensing instruments species by species. The time series of the coincident XCO2, XCH4, and XCO values for the HR125LR and the other test instruments are shown in Fig. D1a, c, and e. The corresponding differences relative to the HR125LR are shown in panels (b), (d), and (f), respectively. The mean bias, standard deviation of the difference, and correlation coefficient between the individual instruments and the HR125LR for the full year of measurements in 2017 and that for the shorter time period are given in Table D1 and are plotted in Fig. 11.
The mean biases of the target species for the test instruments (see Table D1) are close to the difference of the biases of the species in Table B1 minus the biases in Table C1 for the full year of 2017 and for the shorter time period. The Vertex70 shows a significant improvement of the bias, scatter, and correlation coefficient for the intercomparison results during the shorter time period performed after the instrument modification.
D1 XCO2 intercomparison results
The EM27/SUN and the IRcube show slight improvement, while the modified Vertex70 shows a slight degradation of the standard deviation of the difference and the correlation coefficient for the intercomparison results relative to the HR125LR compared to TCCON for the full year and the shorter time period (see Tables D1 and B1). The scatter of the LHR instrument is very high, and it is the dominating component of the intercomparison with other reference instruments.
D2 XCH4 intercomparison results
The high bias observed in the March–May period for the intercomparison of the test instruments with the TCCON instrument is not seen in the intercomparison results of the test instruments with the HR125LR. This indicates that the high resolution and the AK of TCCON are the cause of the large bias during this period when the TCCON a priori is further away from the true atmospheric state. The standard deviation of the difference and the correlation coefficient improved for the EM27/SUN comparison. The IRcube has no significant bias, and the scatter and the correlation coefficient also improved for the HR125LR intercomparison in relation to the TCCON intercomparison. The modified Vertex70 has the same standard deviation of the difference and a similar correlation coefficient for the HR125LR compared to the TCCON intercomparison results.
D3 XCO intercomparison results
The EM27/SUN results for the full period and the Vertex70 results for the short period show similar values for the standard deviation of the difference and the correlation coefficient for the intercomparison results relative to the HR125LR compared to the TCCON results.
The intercomparison results show the Xgas dependence on the resolution of the instrument, the averaging kernels, and the a priori. The low-resolution measurements helped to determine that the high bias in the XCH4 during the March–May period was caused by the resolution difference and its sensitivity to the different averaging kernels.
The TCCON Xgas values are known to be affected by the SZA during the measurements. In this section we check the SZA dependence of the low-resolution instruments with respect to the TCCON and HR125LR data sets.
E1 XCO2 intercomparison results
The XCO2 biases as a function of the measurement SZA for the low-resolution test instruments relative to the TCCON and HR125LR for all measurements in 2017 are shown in Figs. E1 and E2, respectively.
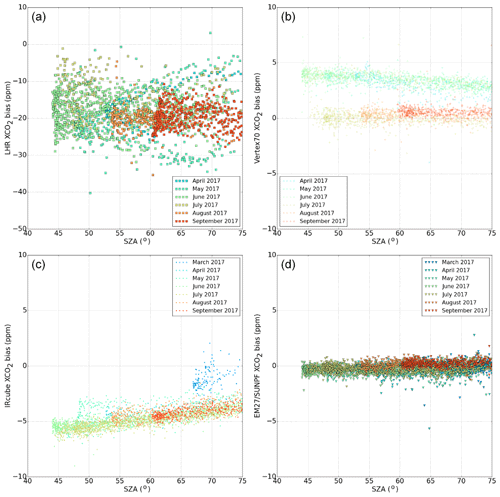
Figure E1XCO2 bias relative to TCCON for each instrument plotted with respect to the solar zenith angle: LHR (a), Vertex70 (b), IRcube (c), EM27/SUN (d). The colours represent the measurement performed during the different months of the year.
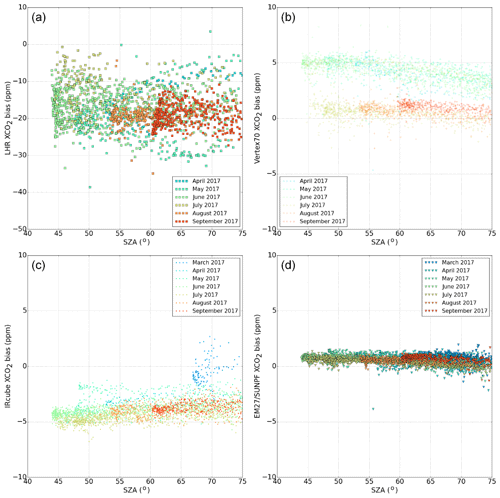
Figure E2XCO2 bias relative to HR125LR for each instrument plotted with respect to the solar zenith angle: LHR (a), Vertex70 (b), IRcube (c), EM27/SUN (d). The colours represent the measurement performed during the different months of the year.
Panel (a) in the two figures shows no SZA dependence of the bias for the LHR. The scatter is very high, and the dominant instrumental biases mask any possible SZA dependence.
The plots for the Vertex70 are shown in Figs. E1b and E2b. The TCCON comparison results show no SZA dependence of the bias after the instrument modification, which implies that the two instruments have the same SZA dependence. However, the HR125LR comparison results show decreasing bias values for an increasing SZA of the measurements. This implies that the XCO2 values retrieved from the HR125LR measurements have a smaller SZA dependency compared to the Vertex70.
The plots for the IRcube and the EM27/SUN are shown in panels (c) and (d). The IRcube bias shows an increase with SZA relative to the TCCON data set and is quite constant relative to the HR125LR data set. The EM27/SUN bias shows a slight increase with SZA relative to the TCCON, and it shows a rather constant bias relative to the HR125LR. This implies that the XCO2 values retrieved from the HR125LR measurements show a similar SZA dependence compared to the retrieval results for the EM27/SUN and the IRcube.
The retrievals for the HR125LR and the EM27/SUN were performed by PROFFAST, and the retrievals of other instruments were performed by GFIT. The data sets for the HR125LR, the IRcube, and EM27/SUN were all measured at the same spectral resolution of 0.5 cm−1, whereas the data set for the Vertex70 was measured at a higher spectral resolution of 0.2 cm−1, and that for the TCCON was measured at 0.02 cm−1. From the plots it can be seen that the SZA dependency of the retrievals is related to the spectral resolution and the AK of the instruments. This explains the decrease in the standard deviation of the bias for the EM27/SUN from 0.45 to 0.4 ppm, while the correlation coefficient improved slightly; the first values are intercomparison results relative to the TCCON, and the latter values are relative to the HR125LR. For the IRcube the standard deviation of the bias decreased from 1.06 to 1.03 ppm, while the correlation coefficient improved from 0.971 to 0.978. For the modified Vertex70 we see an increase in the standard deviation of the bias from 0.582 to 0.657 ppm, while the correlation coefficient decreased from 0.931 to 0.911.
E2 XCH4 intercomparison results
The XCH4 biases as a function of the measurement SZA for the low-resolution test instruments relative to the TCCON and the HR125LR for all measurements in 2017 are shown in Fig. E3.
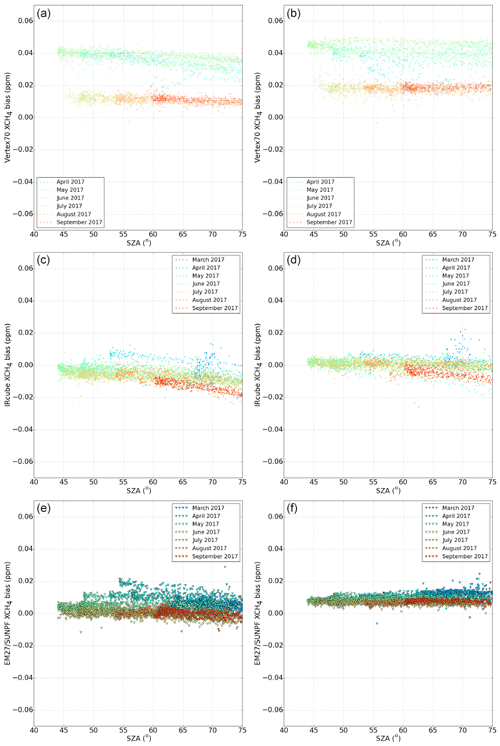
Figure E3XCH4 bias relative to TCCON (a, c, e) and relative to HR125LR (b, d, f) for each instrument plotted with respect to the solar zenith angle: Vertex70 (a, b), IRcube (c, d), EM27/SUN (e, f). The colours represent the measurement performed during the different months of the year.
The Vertex70 XCH4 biases are shown in panels (a) and (b). The TCCON comparison results show a slight decrease in the bias values for an increasing SZA of the measurements, whereas the bias remains constant with an increasing SZA for the HR125LR comparison for the measurements performed after the instrument modification. The correlation coefficient and the scatter of the bias for the two comparison results are very similar.
The plots for the IRcube are shown in panels (c) and (d). The IRcube bias shows a stronger dependence on SZA, leading to a slightly poorer correlation coefficient of 0.924 for the TCCON comparison relative to a correlation coefficient of 0.949 for the HR125LR comparison. The standard deviation of the difference is slightly better for the HR125LR comparison.
Panel (e) shows a dependence of the bias as a function of the SZA for the EM27/SUN comparison relative to the TCCON. Panel (f), however, does not show any dependence of the EM27/SUN comparison relative to the HR125LR. The correlation coefficient of the TCCON comparison was 0.962 and improved slightly to 0.978 for the HR125LR comparison. The scatter in the bias is slightly low, leading to a significant improvement of the standard deviation of the difference from 0.005 ppm for the TCCON to 0.003 ppm for the HR125LR.
E3 XCO intercomparison results
The XCO biases as a function of the measurement SZA for the Vertex70 and the EM27/SUN relative to the TCCON and the HR125LR for all measurements in 2017 are shown in Fig. E4.
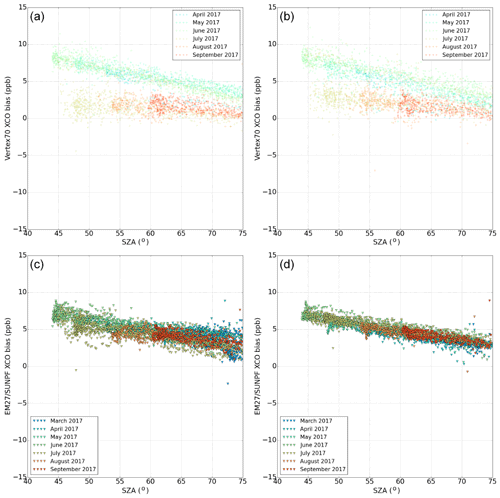
Figure E4XCO bias relative to TCCON (a, c) and relative to HR125LR (b, d) for each instrument plotted with respect to the solar zenith angle: Vertex70 (a, b) and EM27/SUN (c, d). The colours represent the measurement performed during the different months of the year.
The Vertex70 comparison results relative to the TCCON show a slight decrease in the bias with an increasing SZA of the measurements performed after the instrument modification. However, the bias increases slightly with an increasing SZA of the measurement for the HR125LR comparison. The standard deviation of the bias changed from 1.04 to 1.17 ppb, whereas the correlation coefficient changed from 0.991 to 0.988 when comparing the Vertex70 results relative to TCCON and relative to HR125LR. This shows that the Vertex70 comparison relative to the TCCON is slightly better than that of the HR125LR.
The EM27/SUN comparison results show a larger bias dependency on the SZA for the TCCON relative to the HR125LR comparison. The standard deviation of the bias decreased from 1.37 to 1.27 ppb, and the correlation coefficient improved from 0.993 to 0.995 for the results relative to the HR125LR compared to the TCCON.
This shows that the Vertex70 comparison with the TCCON shows better results, whereas the EM27/SUN shows better results in comparison with the HR125LR.
E4 Xair intercomparison results
The Xair values as a function of the SZA of the measurements for the low-resolution instruments and the TCCON for all measurements in 2017 are shown in Fig. E5. The plots show the SZA dependence of the retrieved oxygen column for the performed measurements. The EM27/SUN and the HR125LR show no SZA dependence. However, the TCCON, Vertex70, and IRcube show a decreasing Xair value for an increasing SZA of the measurements.
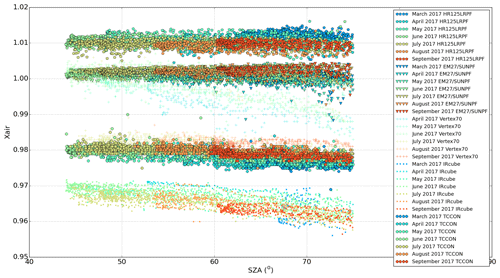
Figure E5Xair plotted for HR125LR, EM27/SUN, Vertex70, IRcube, and TCCON with respect to the measurement solar zenith angle. The colours represent the measurement performed during the different months of the year.
The EM27/SUN and the HR125LR results retrieved with PROFFAST do not show SZA dependence for species to which an air-mass correction factor, which was previously determined, was applied except for carbon monoxide to which no correction was applied. The other instruments show an SZA dependence to some degree. In order to minimise the effect of the SZA, measurements with an SZA <75∘ should be used for the instruments.
In this section the Xgas bias dependence on the humidity present along the measurement line of sight is discussed.
F1 XCO2 intercomparison results
The XCO2 bias as a function of the humidity along the measurement line of sight for the low-resolution test instruments relative to the HR125LR for all measurements in 2017 is shown in Fig. F1.
Panel (b) shows no bias dependency on the humidity for the Vertex70. The scatter in the bias shows a significant reduction after the instrument modification.
The IRcube bias plot is shown in panel (c). The measurements in March, which were performed with the original optic fibre, show a high scatter in the values. The scatter is reduced after the change of the fibre and no humidity dependency of the bias is seen.
Panel (d) shows the bias plot for the EM27/SUN. The measurements in March show a higher scatter in the bias values compared to the rest of the year; however, no dependency on the humidity is seen.
Panel (a) shows no bias dependency on the humidity for the LHR. However, owing to the instrumental biases the scatter is very high.
This demonstrates that the XCO2 values retrieved from the low-resolution instruments have no dependencies on the humidity in the line of sight of the measurements.
F2 XCH4 intercomparison results
The XCH4 bias as a function of the humidity along the measurement line of sight for the low-resolution test instruments relative to the HR125LR for all measurements in 2017 is shown in Fig. F2.
Panel (a) shows no bias dependency on the humidity for the Vertex70. Also, here we see a significant reduction in the scatter in the bias values after the instrument modification.
Panel (b) shows no dependency of the bias on the humidity for the IRcube. Also, here we see high scatter before the change of the fibre.
Panel (c) shows the bias for the EM27/SUN. The scatter in the bias values during the dry period is slightly higher compared to the measurements from other months, but there is no dependency on the humidity seen. The high scatter of the bias during the dry period (March–May) may be due to the difference of the a prioris compared to the true atmospheric state. This demonstrates that the XCH4 retrieved from the low-resolution instruments has no dependencies on the humidity in the line of sight of the measurements.
F3 XCO intercomparison results
The XCO bias as a function of the humidity along the measurement line of sight for the low-resolution test instruments relative to the HR125LR for all measurements in 2017 is shown in Fig. F2.
Panel (d) shows no bias dependency on the humidity for the Vertex70. A reduction in the scatter of the bias values after the instrument modification is also seen here.
Panel (e) shows the bias plot for the EM27/SUN. The scatter in the values is high; however, no dependency on the humidity can be seen. This demonstrates that the XCO retrieved from the low-resolution instruments has no dependencies on the humidity in the line of sight of the measurements.
F4 Xair intercomparison results
The Xair values as a function of the humidity along the measurement line of sight for the low-resolution test instruments, the HR125LR, and the TCCON for all measurements in 2017 are shown in Fig. F3. The figure shows the humidity dependency of the retrieved oxygen column for the measurements performed. The Vertex70 and the IRcube show a significant reduction in the scatter of the Xair values after the instrument modification. The low-resolution instruments show no dependencies on the humidity.
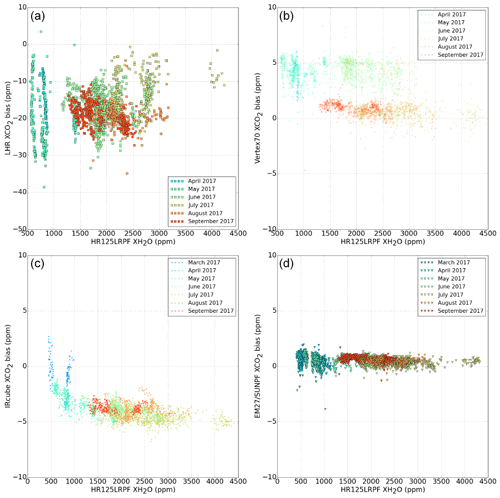
Figure F1XCO2 bias relative to HR125LR for each instrument plotted with respect to the total column water vapour retrieved by the reference HR125LR measurements: LHR (a), Vertex70 (b), IRcube (c), EM27/SUN (d). The colours represent the measurement performed during the different months of the year.
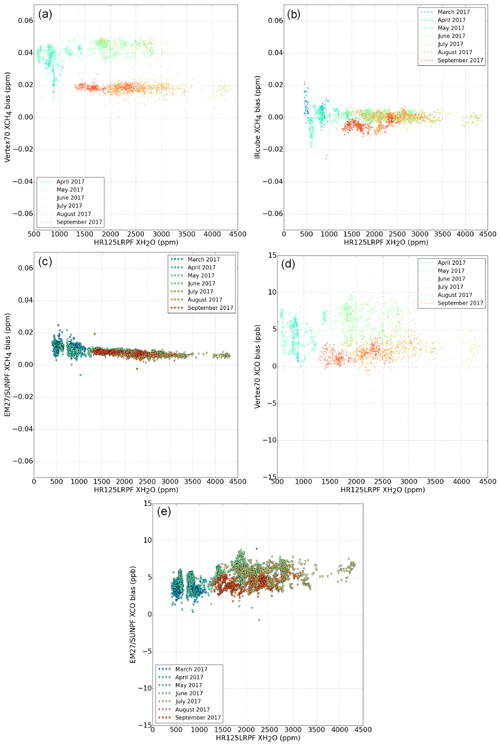
Figure F2XCH4 bias relative to HR125LR for Vertex70 (a), IRcube (b), and EM27/SUN (c). XCO bias relative to HR125LR for Vertex70 (d) and EM27/SUN (e) plotted with respect to the total column water vapour retrieved by the reference HR125LR measurements. The colours represent the measurement performed during the different months of the year.
The raw measurements (level 0) are available at https://doi.org/10.18758/71021040 (Sha et al., 2018). The atmospheric concentrations of the trace gases (level 2) are available at https://doi.org/10.18758/71021048 (Sha et al., 2019).
MKS, MDM, and JN designed the study. MKS wrote the paper and produced the intercomparison analysis and results with input from all authors. RK and PH operated the TCCON station and provided data; they also provided local support in the operation of all other instruments. QT, FH, and TB were involved with the operation and provided data from the EM27/SUN. CP provided EM27/SUN data processed with GFIT. NJ and DWTG were involved with the operation and provided data from the IRcube. CP, CH, FS, MKS, BL, MDM, and JN were involved with the operation and provided data from the Vertex70. AH and MH designed, built, developed, and deployed the LHR. AH improved the retrieval algorithm to derive data from the LHR. DW technically supervised the tasks associated with the LHR development and operation, as well as contributing to the paper, particularly in the LHR section. HC, RK, and PH were involved with the operation and provided data from the AirCore. AD contributed to the discussions and interpretation of the data. All authors read the paper and provided comments.
The authors declare that they have no conflict of interest.
The authors wish to thank the instrument operators and the scientists doing the retrieval for the delivery of the data, which were used for the intercomparison discussed in this paper. We thank Tuomas Laurila and Juha Hatakka from FMI for providing in situ measurements from the 50 m tower at the site and for providing critical instrumentation needed for the AirCore analysis, including a gas analyser, calibration gases, and fill gases. We thank Nicholas Kumps for checking on the automatic operation and data recording of the Vertex70 instrument. KIT would like to thank the FMI staff and their student Elina Paulus for supporting the operation of the EM27/SUN spectrometer. The UK team also wishes to acknowledge support from the Centre of Earth Observation Instrumentation (CEOI) and the UK Space Agency (UKSA). We thank Paolo Castracane for his contribution during the project meetings and discussions.
This research has been supported by the European Space Agency (grant no. ESA-IPL-POE-LG-cl-LE-2015-1129).
This paper was edited by Dominik Brunner and reviewed by Ruediger Lang and one anonymous referee.
Borsdorff, T., aan de Brugh, J., Hu, H., Hasekamp, O., Sussmann, R., Rettinger, M., Hase, F., Gross, J., Schneider, M., Garcia, O., Stremme, W., Grutter, M., Feist, D. G., Arnold, S. G., De Mazière, M., Kumar Sha, M., Pollard, D. F., Kiel, M., Roehl, C., Wennberg, P. O., Toon, G. C., and Landgraf, J.: Mapping carbon monoxide pollution from space down to city scales with daily global coverage, Atmos. Meas. Tech., 11, 5507–5518, https://doi.org/10.5194/amt-11-5507-2018, 2018. a
Dirksen, R. J., Sommer, M., Immler, F. J., Hurst, D. F., Kivi, R., and Vömel, H.: Reference quality upper-air measurements: GRUAN data processing for the Vaisala RS92 radiosonde, Atmos. Meas. Tech., 7, 4463–4490, https://doi.org/10.5194/amt-7-4463-2014, 2014. a
Dlugokencky, E. and Tans, P.: Trends in Atmospheric Carbon Dioxide and Methane, available at: http://www.esrl.noaa.gov/gmd/ccgg/trends/, last access: 1 October 2019. a
Dudhia, A.: The Reference Forward Model (RFM), J. Quant. Spectrosc. Ra., 186, 243–253, https://doi.org/10.1016/j.jqsrt.2016.06.018, 2017. a
Frey, M., Hase, F., Blumenstock, T., Groß, J., Kiel, M., Mengistu Tsidu, G., Schäfer, K., Sha, M. K., and Orphal, J.: Calibration and instrumental line shape characterization of a set of portable FTIR spectrometers for detecting greenhouse gas emissions, Atmos. Meas. Tech., 8, 3047–3057, https://doi.org/10.5194/amt-8-3047-2015, 2015. a, b, c
Frey, M., Sha, M. K., Hase, F., Kiel, M., Blumenstock, T., Harig, R., Surawicz, G., Deutscher, N. M., Shiomi, K., Franklin, J. E., Bösch, H., Chen, J., Grutter, M., Ohyama, H., Sun, Y., Butz, A., Mengistu Tsidu, G., Ene, D., Wunch, D., Cao, Z., Garcia, O., Ramonet, M., Vogel, F., and Orphal, J.: Building the COllaborative Carbon Column Observing Network (COCCON): long-term stability and ensemble performance of the EM27/SUN Fourier transform spectrometer, Atmos. Meas. Tech., 12, 1513–1530, https://doi.org/10.5194/amt-12-1513-2019, 2019. a, b
Gisi, M., Hase, F., Dohe, S., Blumenstock, T., Simon, A., and Keens, A.: XCO2-measurements with a tabletop FTS using solar absorption spectroscopy, Atmos. Meas. Tech., 5, 2969–2980, https://doi.org/10.5194/amt-5-2969-2012, 2012. a, b
Hase, F.: Inversion von Spurengasprofilen aus hochaufgelöstenbodengebundenen FTIR-Messungen in Absorption, PhD thesis, available at: http://www.imk-asf.kit.edu/downloads/bod/disshase.pdf (last access: 1 October 2019), 2000. a, b
Hase, F., Blumenstock, T., and Paton-Walsh, C.: Analysis of the instrumental line shape of high-resolution Fourier transform IR spectrometers with gas cell measurements and new retrieval software, Appl. Optics, 38, 3417–3422, https://doi.org/10.1364/AO.38.003417, 1999. a
Hase, F., Drouin, B. J., Roehl, C. M., Toon, G. C., Wennberg, P. O., Wunch, D., Blumenstock, T., Desmet, F., Feist, D. G., Heikkinen, P., De Mazière, M., Rettinger, M., Robinson, J., Schneider, M., Sherlock, V., Sussmann, R., Té, Y., Warneke, T., and Weinzierl, C.: Calibration of sealed HCl cells used for TCCON instrumental line shape monitoring, Atmos. Meas. Tech., 6, 3527–3537, https://doi.org/10.5194/amt-6-3527-2013, 2013. a
Hase, F., Frey, M., Kiel, M., Blumenstock, T., Harig, R., Keens, A., and Orphal, J.: Addition of a channel for XCO observations to a portable FTIR spectrometer for greenhouse gas measurements, Atmos. Meas. Tech., 9, 2303–2313, https://doi.org/10.5194/amt-9-2303-2016, 2016. a, b
Hasekamp, O., Lorente, A., Hu, H., Butz, A., aan de Brugh, J., and Landgraf, J.: Algorithm Theoretical Baseline Document for Sentinel-5 Precursor: Methane Retrieval, available at: https://sentinel.esa.int/documents/247904/2476257/Sentinel-5P-TROPOMI-ATBD-Methane-retrieval, last access: 1 October 2019. a
Hedelius, J. and Wennberg, P.: EM27/SUN GGG interferogram processing suite, CaltechDATA, https://doi.org/10.22002/D1.306, 2017. a
Hedelius, J. K., Viatte, C., Wunch, D., Roehl, C. M., Toon, G. C., Chen, J., Jones, T., Wofsy, S. C., Franklin, J. E., Parker, H., Dubey, M. K., and Wennberg, P. O.: Assessment of errors and biases in retrievals of X, X, XCO, and X from a 0.5 cm−1 resolution solar-viewing spectrometer, Atmos. Meas. Tech., 9, 3527–3546, https://doi.org/10.5194/amt-9-3527-2016, 2016. a, b, c, d, e, f
Hedelius, J. K., Parker, H., Wunch, D., Roehl, C. M., Viatte, C., Newman, S., Toon, G. C., Podolske, J. R., Hillyard, P. W., Iraci, L. T., Dubey, M. K., and Wennberg, P. O.: Intercomparability of X and X from the United States TCCON sites, Atmos. Meas. Tech., 10, 1481–1493, https://doi.org/10.5194/amt-10-1481-2017, 2017. a
Hoffmann, A., Macleod, N. A., Huebner, M., and Weidmann, D.: Thermal infrared laser heterodyne spectroradiometry for solar occultation atmospheric CO2 measurements, Atmos. Meas. Tech., 9, 5975–5996, https://doi.org/10.5194/amt-9-5975-2016, 2016. a, b, c, d
Hoffmann, A., Macleod, N. A., Huebner, M., and Weidmann, D.: LHR ILS Validation for FRM4GHG campaign, Tech. rep., Spectroscopy Group, Earth Observation & Technology Department, Space Science & Technology Department, Rutherford Appleton Laboratory, available at: http://frm4ghg.aeronomie.be/ProjectDir/documents/ILS_parameters/LHR_ILS_validation.pdf (last access: 1 October 2019), 2017. a
Inoue, M., Morino, I., Uchino, O., Nakatsuru, T., Yoshida, Y., Yokota, T., Wunch, D., Wennberg, P. O., Roehl, C. M., Griffith, D. W. T., Velazco, V. A., Deutscher, N. M., Warneke, T., Notholt, J., Robinson, J., Sherlock, V., Hase, F., Blumenstock, T., Rettinger, M., Sussmann, R., Kyrö, E., Kivi, R., Shiomi, K., Kawakami, S., De Mazière, M., Arnold, S. G., Feist, D. G., Barrow, E. A., Barney, J., Dubey, M., Schneider, M., Iraci, L. T., Podolske, J. R., Hillyard, P. W., Machida, T., Sawa, Y., Tsuboi, K., Matsueda, H., Sweeney, C., Tans, P. P., Andrews, A. E., Biraud, S. C., Fukuyama, Y., Pittman, J. V., Kort, E. A., and Tanaka, T.: Bias corrections of GOSAT SWIR XCO2 and XCH4 with TCCON data and their evaluation using aircraft measurement data, Atmos. Meas. Tech., 9, 3491–3512, https://doi.org/10.5194/amt-9-3491-2016, 2016. a
Jing, Y., Wang, T., Zhang, P., Chen, L., Xu, N., and Ma, Y.: Global Atmospheric CO2 Concentrations Simulated by GEOS-Chem: Comparison with GOSAT, Carbon Tracker and Ground-Based Measurements, Atmosphere, 9, 175, https://doi.org/10.3390/atmos9050175, 2018. a
Karion, A., Sweeney, C., Tans, P., and Newberger, T.: AirCore: An Innovative Atmospheric Sampling System, J. Atmos. Ocean. Tech., 27, 1839–1853, https://doi.org/10.1175/2010JTECHA1448.1, 2010. a, b
Keppel-Aleks, G., Toon, G. C., Wennberg, P. O., and Deutscher, N. M.: Reducing the impact of source brightness fluctuations on spectra obtained by Fourier-transform spectrometry, Appl. Optics, 46, 4774–4779, https://doi.org/10.1364/AO.46.004774, 2007. a
Kirschke, S., Bousquet, P., Ciais, P., Saunois, M., Canadell, J. G., Dlugokencky, E. J., Bergamaschi, P., Bergmann, D., Blake, D. R., Bruhwiler, L., Cameron-Smith, P., Castaldi, S., Chevallier, F., Feng, L., Fraser, A., Heimann, M., Hodson, E. L., Houweling, S., Josse, B., Fraser, P. J., Krummel, P. B., Lamarque, J.-F., Langenfelds, R. L., Le Quéré, C., Naik, V., O’Doherty, S., Palmer, P. I., Pison, I., Plummer, D., Poulter, B., Prinn, R. G., Rigby, M., Ringeval, B., Santini, M., Schmidt, M., Shindell, D. T., Simpson, I. J., Spahni, R., Steele, L. P., Strode, S. A., Sudo, K., Szopa, S., van der Werf, G. R., Voulgarakis, A., van Weele, M., Weiss, R. F., Williams, J. E., and Zeng, G.: Three decades of global methane sources and sinks, Nat. Geosci., 6, 813–823, https://doi.org/10.1038/ngeo1955, 2013. a
Kivi, R. and Heikkinen, P.: Fourier transform spectrometer measurements of column CO2 at Sodankylä, Finland, Geosci. Instrum. Method. Data Syst., 5, 271–279, https://doi.org/10.5194/gi-5-271-2016, 2016. a, b
Kivimäki, E., Lindqvist, H., Hakkarainen, J., Laine, M., Sussmann, R., Tsuruta, A., Detmers, R., Deutscher, N. M., Dlugokencky, E. J., Hase, F., Hasekamp, O., Kivi, R., Morino, I., Notholt, J., Pollard, D. F., Roehl, C., Schneider, M., Sha, M. K., Velazco, V. A., Warneke, T., Wunch, D., Yoshida, Y., and Tamminen, J.: Evaluation and Analysis of the Seasonal Cycle and Variability of the Trend from GOSAT Methane Retrievals, Remote Sensing, 11, 882, https://doi.org/10.3390/rs11070882, 2019. a
Kong, Y., Chen, B., and Measho, S.: Spatio-Temporal Consistency Evaluation of XCO2 Retrievals from GOSAT and OCO-2 Based on TCCON and Model Data for Joint Utilization in Carbon Cycle Research, Atmosphere, 10, 354, https://doi.org/10.3390/atmos10070354, 2019. a
Landgraf, J., aan de Brugh, J., Scheepmaker, R. A., Borsdorff, T., Houweling, S., and Hasekamp, O.: Algorithm Theoretical Baseline Document for Sentinel-5 Precursor: Carbon Monoxide Total Column Retrieval, available at: https://sentinel.esa.int/documents/247904/2476257/Sentinel-5P-TROPOMI-ATBD-Carbon-Monoxide-Total-Column-Retrieval (last access: 1 October 2019), 2018. a
Neefs, E., Mazière, M. D., Scolas, F., Hermans, C., and Hawat, T.: BARCOS, an automation and remote control system for atmospheric observations with a Bruker interferometer, Rev. Sci. Instrum., 78, 035109, https://doi.org/10.1063/1.2437144, 2007. a
Novelli, P. C., Masarie, K. A., and Lang, P. M.: Distributions and recent changes of carbon monoxide in the lower troposphere, J. Geophys. Res.-Atmos., 103, 19015–19033, https://doi.org/10.1029/98JD01366, 1998. a
Ostler, A., Sussmann, R., Patra, P. K., Houweling, S., De Bruine, M., Stiller, G. P., Haenel, F. J., Plieninger, J., Bousquet, P., Yin, Y., Saunois, M., Walker, K. A., Deutscher, N. M., Griffith, D. W. T., Blumenstock, T., Hase, F., Warneke, T., Wang, Z., Kivi, R., and Robinson, J.: Evaluation of column-averaged methane in models and TCCON with a focus on the stratosphere, Atmos. Meas. Tech., 9, 4843–4859, https://doi.org/10.5194/amt-9-4843-2016, 2016. a
Sha, M. K., De Mazière, M., Notholt, J., Blumenstock, T., Chen, H., Griffith, D., Hase, F., Heikkinen, P., Hoffmann, A., Huebner, M., Jones, N., Kivi, R., Petri, C., Tu, Q., Warneke, T., and Weidmann, D.: FRM4GHG raw dataset from the Sodankylä campaign, Royal Belgian Institute for Space Aeronomy, https://doi.org/10.18758/71021040, 2018. a, b
Sha, M. K., De Mazière, M., Notholt, J., Blumenstock, T., Chen, H., Griffith, D., Hase, F., Heikkinen, P., Hoffmann, A., Huebner, M., Jones, N., Kivi, R., Petri, C., Tu, Q., Warneke, T., and Weidmann, D.: FRM4GHG level2 dataset from the Sodankylä campaign, Royal Belgian Institute for Space Aeronomy, https://doi.org/10.18758/71021048, 2019. a, b
Stocker, T. F., Qin, D., Plattner, G.-K., Alexander, L., Allen, S., Bindoff, N., Bréon, F.-M., Church, J., Cubasch, U., Emori, S., Forster, P., Friedlingstein, P., Gillett, N., Gregory, J., Hartmann, D., Jansen, E., Kirtman, B., Knutti, R., Kumar, K. K., Lemke, P., Marotzke, J., Masson-Delmotte, V., Meehl, G., Mokhov, I., Piao, S., Ramaswamy, V., Randall, D., Rhein, M., Rojas, M., Sabine, C., Shindell, D., Talley, L., Vaughan, D., and Xie, S.-P.: Technical Summary, in: Climate Change 2013: The Physical Science Basis. Contribution of Working Group I to the Fifth Assessment Report of the Intergovernmental Panel on Climate Change, edited by: Stocker, T. F., Qin, D., Plattner, G.-K., Tignor, M., Allen, S. K., Boschung, J., Nauels, A., Xia, Y., Bex, V., and Midgley, P. M., Cambridge University Press, Cambridge, UK and New York, NY, USA, 2013. a, b
Toon, G. C. and Wunch, D.: A stand-alone a priori profile generation tool for GGG2014 release, CaltechDATA, https://doi.org/10.14291/TCCON.GGG2014.PRIORS.R0/1221661, 2017. a
Tsai, T. R., Rose, R. A., Weidmann, D., and Wysocki, G.: Atmospheric vertical profiles of O3, N2O, CH4, CCl2F2, and H2O retrieved from external-cavity quantum-cascade laser heterodyne radiometer measurements, Appl. Optics, 51, 8779–8792, https://doi.org/10.1364/AO.51.008779, 2012. a
Weidmann, D., Reburn, W. J., and Smith, K. M.: Ground-based prototype quantum cascade laser heterodyne radiometer for atmospheric studies, Rev. Sci. Instrum., 78, 073107, https://doi.org/10.1063/1.2753141, 2007. a
Wunch, D., Toon, G. C., Blavier, J.-F. L., Washenfelder, R. A., Notholt, J., Connor, B. J., Griffith, D. W. T., Sherlock, V., and Wennberg, P. O.: The Total Carbon Column Observing Network, Philos. T. Roy. Soc. A, 369, 2087–2112, https://doi.org/10.1098/rsta.2010.0240, 2011. a
Wunch, D., Toon, G. C., Sherlock, V., Deutscher, N. M., Liu, C., Feist, D. G., and Wennberg, P. O.: The Total Carbon Column Observing Network's GGG2014 Data Version, Tech. rep., Carbon Dioxide Information Analysis Center, Oak Ridge National Laboratory, Oak Ridge, Tennessee, USA, https://doi.org/10.14291/tccon.ggg2014.documentation.R0/1221662, 2015. a, b, c, d
Wunch, D., Wennberg, P. O., Osterman, G., Fisher, B., Naylor, B., Roehl, C. M., O'Dell, C., Mandrake, L., Viatte, C., Kiel, M., Griffith, D. W. T., Deutscher, N. M., Velazco, V. A., Notholt, J., Warneke, T., Petri, C., De Maziere, M., Sha, M. K., Sussmann, R., Rettinger, M., Pollard, D., Robinson, J., Morino, I., Uchino, O., Hase, F., Blumenstock, T., Feist, D. G., Arnold, S. G., Strong, K., Mendonca, J., Kivi, R., Heikkinen, P., Iraci, L., Podolske, J., Hillyard, P. W., Kawakami, S., Dubey, M. K., Parker, H. A., Sepulveda, E., García, O. E., Te, Y., Jeseck, P., Gunson, M. R., Crisp, D., and Eldering, A.: Comparisons of the Orbiting Carbon Observatory-2 (OCO-2) X measurements with TCCON, Atmos. Meas. Tech., 10, 2209–2238, https://doi.org/10.5194/amt-10-2209-2017, 2017. a
- Abstract
- Introduction
- Measurements at Sodankylä and campaign instrumentation
- Description of the measurement strategy to ensure comparable observations
- Data description
- Campaign results
- Summary and outlook
- Appendix A: Non-linearity of the Sodankylä TCCON InGaAs detector
- Appendix B: Effect of the non-linearity on the Xgas values of TCCON
- Appendix C: Comparisons between TCCON and low-resolution measurements performed with Bruker IFS 125HR
- Appendix D: Intercomparisons with HR125LR data
- Appendix E: SZA dependencies of bias
- Appendix F: Xgas bias dependence on the humidity
- Data availability
- Author contributions
- Competing interests
- Acknowledgements
- Financial support
- Review statement
- References
- Abstract
- Introduction
- Measurements at Sodankylä and campaign instrumentation
- Description of the measurement strategy to ensure comparable observations
- Data description
- Campaign results
- Summary and outlook
- Appendix A: Non-linearity of the Sodankylä TCCON InGaAs detector
- Appendix B: Effect of the non-linearity on the Xgas values of TCCON
- Appendix C: Comparisons between TCCON and low-resolution measurements performed with Bruker IFS 125HR
- Appendix D: Intercomparisons with HR125LR data
- Appendix E: SZA dependencies of bias
- Appendix F: Xgas bias dependence on the humidity
- Data availability
- Author contributions
- Competing interests
- Acknowledgements
- Financial support
- Review statement
- References