the Creative Commons Attribution 4.0 License.
the Creative Commons Attribution 4.0 License.
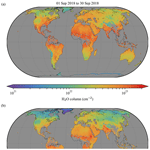
First data set of H2O/HDO columns from the Tropospheric Monitoring Instrument (TROPOMI)
Andreas Schneider
Tobias Borsdorff
Joost aan de Brugh
Franziska Aemisegger
Dietrich G. Feist
Rigel Kivi
Frank Hase
Matthias Schneider
Jochen Landgraf
Global measurements of atmospheric water vapour isotopologues aid to better understand the hydrological cycle and improve global circulation models. This paper presents a new data set of vertical column densities of H2O and HDO retrieved from short-wave infrared (2.3 µm) reflectance measurements by the Tropospheric Monitoring Instrument (TROPOMI) onboard the Sentinel-5 Precursor satellite. TROPOMI features daily global coverage with a spatial resolution of up to 7 km×7 km. The retrieval utilises a profile-scaling approach. The forward model neglects scattering, and strict cloud filtering is therefore necessary. For validation, recent ground-based water vapour isotopologue measurements by the Total Carbon Column Observing Network (TCCON) are employed. A comparison of TCCON δD with ground-based measurements by the Multi-platform remote Sensing of Isotopologues for investigating the Cycle of Atmospheric water (MUSICA) project for data prior to 2014 (where MUSICA data are available) shows a bias in TCCON δD estimates. As TCCON HDO is currently not validated, an overall correction of recent TCCON HDO data is derived based on this finding. The agreement between the corrected TCCON measurements and co-located TROPOMI observations is good with an average bias of molec cm−2 ((1.1±7.2) %) in H2O and molec cm−2 ( %) in HDO, which corresponds to a mean bias of ‰ in a posteriori δD. The bias is lower at low- and mid-latitude stations and higher at high-latitude stations. The use of the data set is demonstrated with a case study of a blocking anticyclone in northwestern Europe in July 2018 using single-overpass data.
- Article
(14260 KB) - Full-text XML
- BibTeX
- EndNote
Atmospheric water vapour represents the strongest natural greenhouse gas and transports a large amount of energy via latent heat; thus, it plays a fundamental role in shaping weather and climate (Kiehl and Trenberth, 1997; Harries, 1997). However, uncertainties in the quantification of the two abovementioned effects are still large and represent one of the key uncertainties in current climate prediction (Stevens and Bony, 2013). Improvement upon current climate prediction requires new observations on a global scale and with a long-term perspective. To this end, satellite observations from space are considered to be the most promising approach (Rast et al., 2014).
Constraints for the hydrological cycle are offered by observations of isotopologues of water vapour. Different equilibrium vapour pressures and diffusion constants of different isotopologues lead to isotopic fractionation whenever a phase change occurs. Isotopic fractionation occurs at the point of phase change, partitioning the heavier and lighter isotopologues, depending on the thermodynamic conditions of the environment. The relative abundance of a heavy isotopologue with respect to the light isotopologue in an air parcel is therefore dependent on the source region's temperature and relative humidity, the source water's isotopic composition as well as the entire transport history of the air parcel, including all evaporation, condensation and mixing events (e.g. Dansgaard, 1964; Craig and Gordon, 1965). This makes measurements of water vapour isotopologues a unique diagnostic of the hydrological cycle (Dansgaard, 1964) and a valuable benchmark for the evaluation and further development of global and regional circulation models (e.g. Joussaume et al., 1984; Hoffmann et al., 1998; Yoshimura et al., 2008; Risi et al., 2010; Pfahl et al., 2012).
The usual notation to describe the isotopological abundance variations is the relative difference of the ratio of the heavy and the light isotopologues, here HDO and H2O, , to a standard abundance ratio RD,std,
(Coplen, 2011). The commonly used standard ratio is Vienna Standard Mean Ocean Water (VSMOW), .
Measurements of atmospheric water vapour isotopologues are not very common. In situ observations are performed from aircrafts and balloons (e.g. Rinsland et al., 1984; Dyroff et al., 2010, 2015; Herman et al., 2014; Sodemann et al., 2017) and on the ground (e.g. Wen et al., 2010; Aemisegger et al., 2012; Bastrikov et al., 2014) using laser spectrometers or cryogenic trapping techniques. Remote sensing instruments exist on the ground and on space- or balloon-based platforms. The former are usually Fourier transform infrared (FTIR) spectrometers. Ground stations are often organised in networks. The largest networks are the Total Carbon Column Observing Network (TCCON, Wunch et al., 2011) and the Network for the Detection of Atmospheric Composition Change (NDACC, De Mazière et al., 2018). The data product of the former includes H2O and HDO, whereas the latter involves water vapour isotopologue measurements retrieved by the Multi-platform remote Sensing of Isotopologues for investigating the Cycle of Atmospheric water (MUSICA) project (Schneider et al., 2016). With respect to satellites, H2O and HDO were first retrieved by Zakharov et al. (2004) using thermal infrared measurements from the Interferometric Monitor for Greenhouse gases (IMG) sensor onboard the Advanced Earth Observing Satellite (ADEOS). Later, this was followed by the Tropospheric Emission Spectrometer (TES) on the Earth Observing System (EOS) Aura satellite (Worden et al., 2006), the Michelson Interferometer for Passive Atmospheric Sounding (MIPAS) onboard the European Space Agency (ESA)'s environmental satellite (ENVISAT) (Steinwagner et al., 2007; Payne et al., 2007), the SCanning Imaging Absorption spectroMeter for Atmospheric CHartographY (SCIAMACHY) instrument on ENVISAT (Frankenberg et al., 2009; Scheepmaker et al., 2015; Schneider et al., 2018), the Infrared Atmospheric Sounding Interferometer (IASI) onboard the MetOP satellites (Herbin et al., 2009; Schneider and Hase, 2011; Schneider et al., 2016; Lacour et al., 2012), the Greenhouse Gases Observing Satellite (GOSAT) (Frankenberg et al., 2013; Boesch et al., 2013) and the Atmospheric Infrared Sounder (AIRS) onboard the NASA Aqua satellite (Worden et al., 2019). The sensitivity of instruments observing in the thermal infrared (IMG, TES, MIPAS, IASI and AIRS) is very different from that of instruments measuring in the short-wave infrared, such as SCIAMACHY and GOSAT. While the former are mainly sensitive in the stratosphere and free troposphere, the latter have good sensitivity in the lower troposphere, including the boundary layer. On 13 October 2017, the Tropospheric Monitoring Instrument (TROPOMI) onboard the Sentinel-5 Precursor (S5P) satellite (Veefkind et al., 2012) was launched. It has a short-wave infrared band in heritage of SCIAMACHY with a spectral range of 2305–2385 nm and a spectral resolution of 0.25 nm, although its signal-to-noise ratio is much better than SCIAMACHY and it has an unprecedented spatial resolution of 7 km×7 km (in the centre of the swath). This work presents a new H2O and HDO column data set from TROPOMI observations starting at first light of the instrument on 9 November 2017. Section 2 introduces the retrieval method. Section 3 presents a ground-based data set to validate the satellite observations against, and the comparison between both data sets is shown in Sect. 4. Section 5 provides a first insight into the data set's use with respect to studying synoptic-scale variability in the atmospheric branch of the water cycle. Finally, the summary of the results and the conclusions are given in Sect. 6.
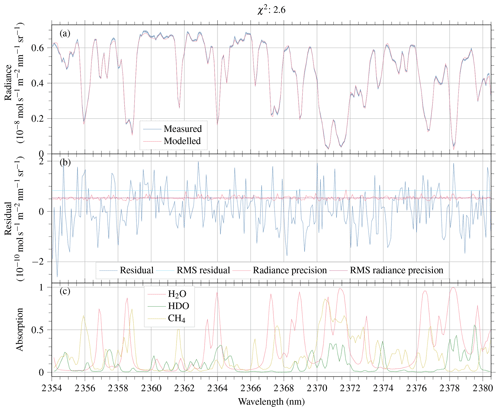
Figure 1(a) Measured radiance (blue) with its precision (light blue shading) and the spectral fit (red) for ground pixel 149 129 in orbit 3969 located near Wollongong, Australia on 20 July 2018. (b) Corresponding residuals (defined as measured minus modelled radiances, in blue) and its root mean square (rms, in cyan), precision of the radiance (in red) and its rms (in purple). (c) Simulated absorption by H2O (red), HDO (green) and CH4 (yellow).
The retrievals are performed with SICOR (short-wave infrared CO retrieval algorithm), which utilises a profile-scaling approach and is described in detail by Scheepmaker et al. (2016), Landgraf et al. (2016) and Borsdorff et al. (2014). In the following, the most important features are summarised and the specific setup is given.
Using the spectral window from 2354.0 to 2380.5 nm (Scheepmaker et al., 2016), the algorithm fits the total columns of H2O, HDO, CH4 and CO as well as a Lambertian surface albedo in the form of a Legendre polynomial of order 1. The isotopologue H218O is included in the forward model but not fitted. A priori profiles of water vapour are adapted from the European Centre for Medium-Range Weather Forecasts (ECMWF) analysis product. As the ECMWF data product does not distinguish between individual isotopologues, H2O, HDO and H218O profiles are obtained from the water vapour profile by scaling it with the respective average relative natural abundances. That implicitly corresponds to a prior of δD of 0 ‰. A priori profiles of CH4 and CO are taken from TM5 simulations (Krol et al., 2005). Scattering cross-sections are taken from HITRAN 2016 (Gordon et al., 2017). The forward model ignores scattering, so that strict filtering for clear-sky scenes is necessary. To this end, co-located measurements from the Visible Infrared Imaging Radiometer Suite (VIIRS) instrument onboard the Suomi National Polar-orbiting Partnership (S-NPP) satellite, which flies in formation with S5P, are used (Siddans, 2016). The cloud cover threshold is 1 % for both the inner field of view and the outer field of view. Moreover, soundings with a high aerosol load are filtered out by a two-band filter as introduced by Scheepmaker et al. (2016) and Hu et al. (2018), which in the present configuration requires that the ratio of retrieved methane in bands with weak and strong absorption (2310–2315 and 2363–2373 nm respectively) is between 0.94 and 1.06. Furthermore, scenes with a solar zenith angle greater than 75∘ are discarded because they are prone to errors due to more scattering and diffraction effects, which are not covered well by the forward model, and due to typically low radiances, meaning low signal-to-noise ratios.
An exemplary spectral fit and the resulting residuals (which are defined as measured minus modelled radiances) are shown in Fig. 1. The root-mean-square (rms) residual (cyan horizontal line in Fig. 1b) is in the order of the rms uncertainty of the radiance (purple horizontal line in Fig. 1b).
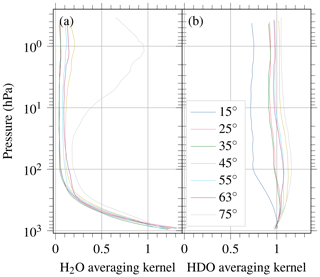
Figure 2Examples of column averaging kernels for (a) H2O and (b) HDO for different solar zenith angles in orbit 4924 on 25 September 2018.
The sensitivity of a retrieved column to changes in a given altitudinal region is described by the column averaging kernel (Rodgers, 2000). The ideal averaging kernel is unity at all altitudes, but in practice the sensitivity changes with height. Figure 2 depicts examples of column averaging kernels for different solar zenith angles. The sensitivity for the two isotopologues are significantly different. For H2O, the highest sensitivity is in the lowest layer (where most water vapour typically resides) and decreases with increasing altitude. The sensitivity in the stratosphere is small; however, the amount of water vapour in this altitudinal region is very small and contributes little to the total column. The sensitivity of HDO does not deviate as much from unity as that of H2O. In the lower troposphere it increases slightly with increasing altitude before reaching a maximum depending on the solar zenith angle, above which it decreases. The differences in the column averaging kernel are due to the different absorption strengths of the two isotopologues and mean that a posteriori δD is sensitive to the profile shapes, particularly of the main isotopologue H2O; this is due to the fact that the averaging kernel for H2O deviates considerably from unity at higher altitudes.
Strong et al. (2019)Kivi et al. (2014)Wunch et al. (2018)Deutscher et al. (2015)Notholt et al. (2014)Hase et al. (2015)Té et al. (2014)Warneke et al. (2019)Wennberg et al. (2017)Morino et al. (2018c)Wennberg et al. (2016)Morino et al. (2018a)Iraci et al. (2016)Wennberg et al. (2014)Wennberg et al. (2015)Kawakami et al. (2014)Morino et al. (2018b)Griffith et al. (2014)Sherlock et al. (2014); Pollard et al. (2019)To validate the TROPOMI retrievals, ground-based Fourier transform infrared (FTIR) measurements are used. HDO is a product of NDACC-MUSICA (Barthlott et al., 2017) and TCCON (Wunch et al., 2015). NDACC-MUSICA provides two products: type 1 is the direct retrieval output, and type 2 contains a posteriori processed output that reports the optimal estimation of (H2O, δD) pairs; here the type 2 product is used because it is recommended for isotopologue analyses (Barthlott et al., 2017). Seven stations exist in both networks: Eureka (Barthlott et al., 2016; Strong et al., 2019), Ny Ålesund (Barthlott et al., 2016; Notholt et al., 2017), Bremen (Barthlott et al., 2016; Notholt et al., 2014), Karlsruhe (Barthlott et al., 2016; Hase et al., 2015), Izaña (Barthlott et al., 2016; Blumenstock et al., 2017), Wollongong (Barthlott et al., 2016; Griffith et al., 2014) and Lauder (Barthlott et al., 2016; Sherlock et al., 2014; Pollard et al., 2019). This allows for comparison of the TCCON and NDACC-MUSICA (here type 2) data products, which reveals a large difference in δD of 58 ‰ on average (which corresponds to a mean relative difference of −30 %) when co-locating with a maximal time difference of 1 h. An example for Wollongong is plotted in Fig. 3. A comparison between MUSICA and TCCON was also performed by Weaver (2019), who compared the MUSICA type 1 product with TCCON and found a bias in δD of 40 ‰ on average.
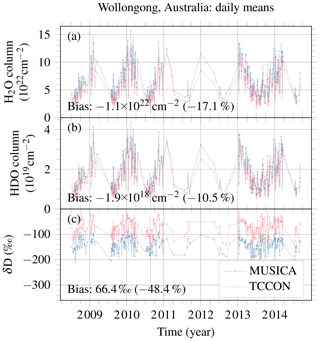
Figure 3Time series of daily averages of H2O (a), HDO (b) and a posteriori δD (c) of the NDACC-MUSICA type 2 (blue crosses) and TCCON (red pluses) data products at Wollongong, Australia. The bias in δD without daily averaging is 65.8 ‰ (−47.4 %).
MUSICA is explicitly created for isotopologue studies, and δD profiles have been validated against aircraft measurements in an altitudinal range between 2 and 7 km during a dedicated campaign in summer 2013 (Schneider et al., 2015, 2016; Dyroff et al., 2015). However, data are only available until 2014; thus, there is no temporal overlap with TROPOMI which was launched in October 2017. TCCON H2O total columns are calibrated with in situ measurements (mainly radiosondes); a so-called aircraft correction factor of 1.0183 is applied to match the reference (Wunch et al., 2015). However, TCCON HDO is currently not verified; thus, no correction factor is applied to it. Therefore, it is assumed that TCCON HDO has to be corrected.
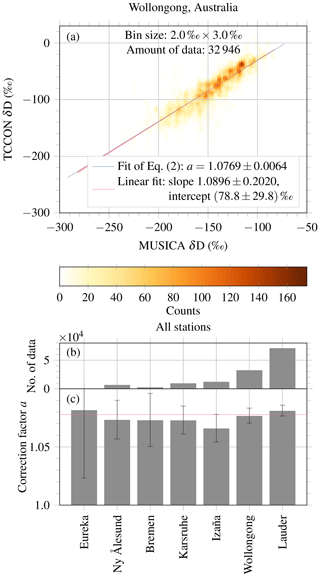
Figure 4(a) Exemplary correlation histogram of TCCON δD vs. MUSICA δD for Wollongong, Australia. The blue line shows the result of a fit of Eq. (2), giving the correction factor for TCCON HDO. The red line shows a linear fit of slope and intercept, and the red shading represents the confidence interval computed using the bootstrap method. (b) Number of co-located measurements for all stations in both networks. (c) Fit results of correction factors for individual stations. The red line corresponds to the error-weighted average over all stations, a=1.0778.
In order to correct for the discrepancy, the idea is to scale TCCON HDO to match MUSICA δD. Scaling HDO by a factor a, i.e. cHDO↦a cHDO, is equivalent to the linear transformation
in δD. Figure 4a depicts a correlation histogram of TCCON δD vs. MUSICA δD for the Wollongong station. Here, the relation between MUSICA and TCCON is described to a large degree by a simple scaling of the column. The result of a fit of Eq. (2) to the data is plotted as a blue line, giving the scaling factor for the TCCON HDO column. To demonstrate that this approach does not involve intercept issues, a linear fit of slope and intercept (red line) as well as the confidence interval computed using the bootstrap method (i.e. by fitting a randomly reduced data set 10 000 times, shown using red shading) has also been plotted in the figure. Both the slope and the offset are similar to the approach using Eq. (2), and the latter lies within the confidence band of the former. The bar chart in Fig. 4c visualises fit results for all stations in both networks. It shows that the correction factor does not change much between stations. The large difference in fit error is mostly due to the large difference in the amount of data (Fig. 4b). Thus, it is meaningful to scale HDO at all TCCON stations by the error-weighted average correction factor a=1.0778 in order to correct TCCON's bias in HDO and, in turn, δD.
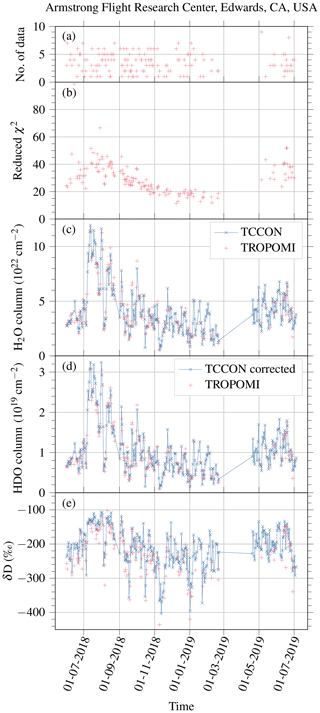
Figure 5Time series of daily averages of corrected TCCON measurements (blue crosses) and co-located TROPOMI observations (red pluses) at Edwards station (35.0∘ N, 117.9∘ W, 700 m a.s.l.). Shown are (a) the number of individual observations per day, (b) the reduced χ2, (c) the H2O columns, (d) the HDO columns and (e) the a posteriori δD.
For validation, TROPOMI observations are co-located with TCCON measurements with a radius of 30 km, a maximal altitude difference of 500 m, a field of view of 45∘ in the FTIR viewing direction and a maximal time difference of 2 h. Here, the TCCON HDO data are corrected according to the approach presented in the previous section. Table 1 gives an overview of all stations used. Other stations have too few (less than 5 d) co-located measurements and have therefore not been included in the validation study. No altitude correction is applied here. The mentioned co-location criterion for altitude is used to ensure that no bias due to the large height difference between the station and satellite ground pixel is introduced (cf. Schneider et al., 2018). For each station, daily averages are computed over all co-located measurements. Figure 5 shows an exemplary time series for Edwards station. The co-located observations of H2O and HDO agree very well, and the agreement in δD is also good, with more scatter and a small bias. Corresponding correlation plots are depicted in Fig. 6. Figure 6a and b confirm the excellent agreement in H2O and HDO with Pearson correlation coefficients of 0.98 and 0.99 respectively, and a corresponding correlation coefficient of 0.96 for δD. The average difference between TROPOMI and TCCON defines the bias. In δD, a small bias is plain in the correlation plot and amounts to −22 ‰.
Figure 7 depicts the validation statistics for all TCCON stations. The correlation in H2O and HDO is high for all stations. In δD, the correlation is high except for a low correlation of 0.37 at Saga station, where the amount of data is very small, the variability in δD is small, but H2O and HDO vary considerably. Figure 8 shows the biases. At low- and mid-latitude (<54∘) stations the bias is as low as molec cm−2 (corresponding to a relative bias of (0.6±5.7) %) in H2O and molec cm−2 ( %) in HDO, which corresponds to ‰ (4.7±6.8 %) in a posteriori δD. At these stations the bias in δD ranges between about −30 ‰ and +15 ‰. At high-latitude stations it can be as high as −45 ‰ to −60 ‰. Possible reasons for these high biases are higher relative biases in H2O and/or HDO at these relatively dry locations. At high-latitude, retrievals are generally challenging due to high solar zenith angles and low albedos which lead to low signal-to-noise ratios. The average bias over all stations is or (1.1±7.2) % in H2O, or % in HDO, and ‰ or (5.6±6.7) % in a posteriori δD. This is good considering that δD is very sensitive to small errors in H2O or HDO.
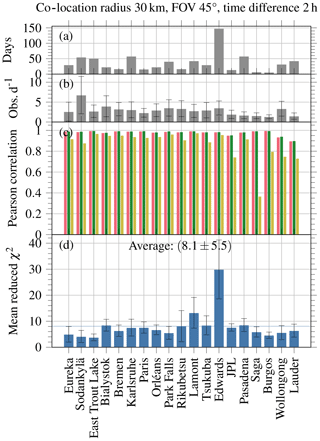
Figure 7Statistics of the validation for all TCCON stations. (a) Number of days with co-located measurements. (b) Average number of co-located TROPOMI observations per day and its standard deviation. (c) Pearson correlation coefficient for H2O (red), HDO (green) and δD (yellow). (d) Average reduced χ2 and its standard error; the blue line visualises the average over all stations.
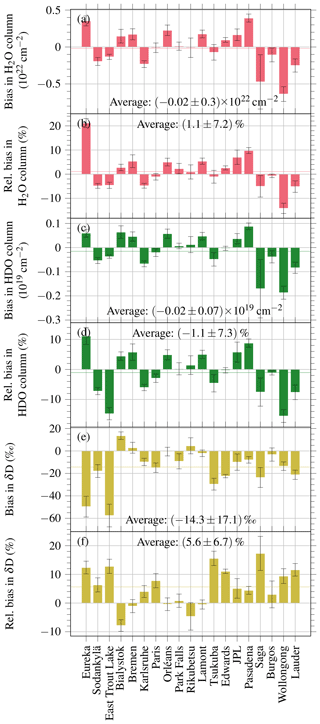
Figure 8Biases for all TCCON stations. (a) Bias in H2O and its standard error. (b) Relative bias in H2O and its standard error. (c) Bias in HDO and its standard error. (d) Relative bias in HDO and its standard error. (e) Bias in a posteriori δD and its standard error. (f) Relative bias in a posteriori δD and its standard error. The horizontal line in all panels visualises the average over all stations.
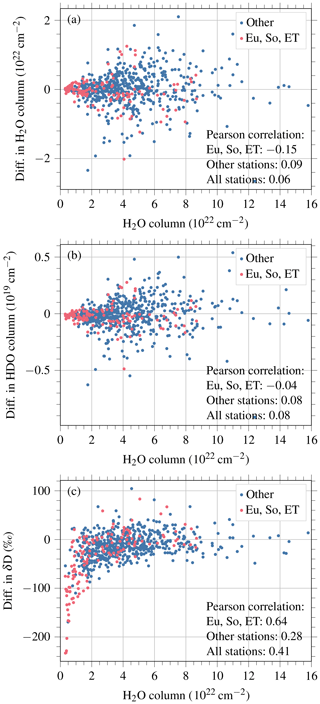
Figure 9Dependence of the difference (TROPOMI – TCCON) of H2O (a), HDO (b) and a posteriori δD (c) on the TROPOMI H2O column. Data from the high-latitude stations Eureka (Eu), Sodankylä (So) and East Trout Lake (ET) are marked using red, and those from the other stations are marked using blue.
Figure 9 shows how differences in TROPOMI observations and corrected TCCON measurements depend on the H2O column. For H2O and HDO there is no such dependence, and the Pearson correlations are very low: 0.06 and 0.08 respectively. In a posteriori δD there is no dependence for low- and mid-latitude stations (correlation coefficient of 0.28). At the high-latitude stations Eureka, Sodankylä and East Trout Lake (marked using red in Fig. 9), a large range of H2O columns show no dependence, but at H2O columns below molec cm−2 (i.e. in dry conditions), the differences between TROPOMI and TCCON increase and do depend on the H2O column. This is the main reason for the high biases at these stations, as discussed in the previous paragraph. As mentioned above, retrievals at low albedos and high solar zenith angles are generally challenging. Whether the dependence of the difference in δD on the H2O column in dry conditions at high latitudes is due to co-location errors or due to the retrieval is still unclear and has to be examined in future research.
An illustration of the TROPOMI retrievals on the global and monthly scale is depicted in Fig. 10 for September 2018. There are no data over the oceans because water is too dark in the short-wave infrared and glint measurements are not taken into account. The data gaps in tropical regions are due to persistent clouds. The data quality in terms of noise is significantly better than for a multiyear average of SCIAMACHY observations (cf. Schneider et al., 2018, Fig. 7). In the spatial distribution shown in Fig. 10 the major isotopic effects formulated by Dansgaard (1964) can be recognised. The general latitudinal gradient due to the temperature dependence of the fractionation effects and progressive rain out of heavy isotopologues, the so-called latitudinal effect, is clearly visible. The continental effect of depletion due to the rain out of the heavy isotopologue is visible on all continents, including Australia. The altitude effect, which describes depletion above high ground due to lower temperature and increasing rain out, can be seen, for example, over the Andes and the Himalayas.
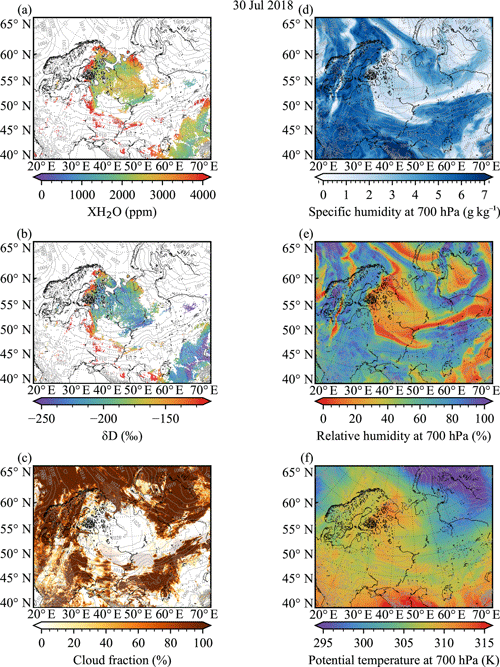
Figure 11TROPOMI single-overpass results for H2O column (a) and δD (b) over Europe on 30 July 2018; VIIRS cloud fraction on the same day (c); specific humidity (d), relative humidity (e), and potential temperature (f) at 700 hPa from the ECMWF analysis product over Europe at 12:00 UTC on 30 July 2018. The 700 hPa level is chosen for the thermodynamic variables because it reflects the large-scale conditions in the lower troposphere above the continental boundary layer. The overlaying contours in all panels show mean sea-level pressure from ECMWF at 12:00 UTC with a contour line distance of 2 hPa.
To demonstrate the quality and the possibilities of the new data set of water vapour isotopologues from TROPOMI, a case study using single-overpass results over Europe on 30 July 2018 is presented in Fig. 11. The summer 2018 was one of the hottest and driest in central and northern Europe (Copernicus Climate Service, 2018; Gubler et al., 2018) with forest fires in Scandinavia, dry fields and low river stages all over the central and northern parts of the continent. The reason for this exceptionally hot and dry summer was the presence of a high-pressure system over northern Europe that blocked the otherwise predominant westerly moist flow from the North Atlantic. Synoptic-scale atmospheric blocking situations can lead to hot temperature extremes due to adiabatic warming of the descending air in the core of the anticyclone (Pfahl and Wernli, 2012). The descending vertical motion favours clear-sky conditions and, thus, further contributes to surface warming via radiative effects in the centre of the anticyclone (Trigo et al., 2004). In particular, the end of July 2018 was characterised by a stationary blocking anticyclone extending over the entire troposphere over northwestern Russia and Scandinavia. This blocking led to large-scale descent and to a divergent flow near the surface in its core, resulting in clear-sky conditions over northwestern Russia and Finland (see Fig. 11c). The isotopic signature of the blocking anticyclone in Fig. 11b reflects this synoptic flow configuration with low δD signals of between −250 ‰ and −200 ‰ in the centre of the anticyclone. The depleted total column vapour in this region is due to the large-scale subsidence transporting depleted (Fig. 11b) and dry (Fig. 11d) upper tropospheric air towards lower levels. The near-surface divergent wind exports more enriched freshly evaporated moisture that is taken up near the surface towards the edges of the blocking. The anticyclone area is characterised by clear skies (Fig. 11c) with low specific humidity (1–3 g kg−1 at 700 hPa, Fig. 11d), low relative humidity (10 %–30 % at 700 hPa, Fig. 11e) and high potential temperature associated with the dry subsiding (adiabatically warming) air masses (Fig. 11f). The dry low-level outflow encounters moister and warmer air at the edge of the surface anticyclone, leading to a very strong horizontal gradient of specific and relative humidity (Fig. 11d, e) in the lower troposphere. As a consequence, the warm moist air is forced to rise, localised instabilities occur, and isolated convective cells develop leading to condensation and the formation of a ring of clouds around the blocking anticyclone. A distinct arc-like feature of enriched total column water vapour at the edge of the anticyclone can be distinguished and is slightly displaced from the first clouds in the northwest (Fig. 11b). Turbulent mixing and convection that inject more enriched, freshly evaporated moisture advected with the large-scale flow from marine environments (Barents Sea, North Sea and Black Sea) could be the reason for this interesting enriched ring-like water vapour isotopologue pattern. A very depleted cloud-free area south of the Ob River with δD values below −250 ‰ (Fig. 11b) might be connected to anomalously strong subsidence of northerly continental air masses.
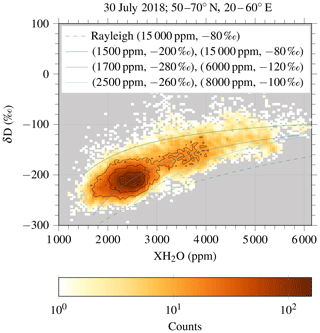
Figure 12Two-dimensional histogram of all TROPOMI observations in the 50–70∘ N, 20–60∘ E area on 30 July 2018 (colour-coded). The black contours show the 25 %, 50 % and 75 % levels of the cumulative density. The dashed green curve represents a Rayleigh fractionation process, and the solid green, blue and cyan curves represent idealised mixing processes as specified by the legend.
The large range of measured δD and H2O mixing ratios during the northeastern European blocking on 30 July 2018 (Fig. 11a, b) becomes apparent in Fig. 12, where a two-dimensional histogram and the cumulative density of the TROPOMI data in the 50–70∘ N, 20–60∘ E region is shown along with two different types of idealised air mass transformation scenarios (coloured lines). These simple idealised scenarios are frequently used in the literature to guide the interpretation of stable isotope measurements in (H2O, δD) diagrams (e.g. Rozanski and Sonntag, 1982; Worden et al., 2007; Noone, 2012). The first scenario, illustrated by the dashed green line in Fig. 12, is that an air parcel with a humidity of 15 000 ppm and ‰ (typical for the continental boundary layer in this northerly continental region, Bastrikov et al., 2014) experienced moist adiabatic ascent with condensation following a Rayleigh process (dashed green line; Rayleigh, 1902; Dansgaard, 1964). The progressive decrease of δD with a decreasing water vapour mixing ratio would thus be due to preferential condensation of HDO compared with H2O and the subsequent removal of hydrometeors by precipitation. The dashed green Rayleigh curve in Fig. 12 shows a behaviour that is different from the TROPOMI data points. Given the clear-sky conditions and the subsidising movement of the air masses within the blocking anticyclone, the assumptions needed for a Rayleigh distillation process are hardly fulfilled. The second scenario, illustrated by the solid green, blue, and cyan lines in Fig. 12, is that two air parcels with distinct humidity and δD are mixed due to turbulent and convective mixing to yield different blends that follow the so-called mixing lines in the (H2O, δD) space. The highest density of observed blocking anticyclone points retrieved by TROPOMI is located in the region spanned by the green and the cyan mixing lines in the (H2O, δD) space. The 25 %, 50 % and 75 % contours of the cumulative density of points are aligned with the blue mixing line. This suggests that a two end-member mixing process describes the data much better than an idealised Rayleigh process (dashed green line in Fig. 12). In this particular synoptic situation, this corresponds to the moistening of a subsidising air mass from the mid troposphere.
In future work, the nature and occurrence of these features should be analysed in more detail, including a catalogue of different continental blocking events with observations from TROPOMI.
Apart from investigations on the water cycle dynamics associated with continental blockings, many other dynamically interesting contexts exist where TROPOMI could present an important added value for further investigations. These comprise, among others, the region of the heat low over the Sahara (e.g. Schneider et al., 2015; González et al., 2016; Lacour et al., 2017) or continental regions upstream of cold air surges leading to events of strong ocean evaporation along the warm ocean western boundary currents (Aemisegger and Papritz, 2018; Aemisegger and Sjolte, 2018).
This work presents a new data set of H2O and HDO columns retrieved from TROPOMI short-wave infrared observations. Scattering is ignored in the forward model so that a strict cloud filtering is necessary, which is performed with co-located VIIRS measurements. The data quality is such that single overpasses yield meaningful results, which is a huge step forward compared with previous missions like SCIAMACHY.
For validation of the TROPOMI data product, particular attention must be paid to the reference data sets. At this stage, there are two data products of ground-based observations of the HDO total column available, provided by the TCCON and NDACC-MUSICA networks. Comparing these two data products for stations in both networks reveals a large bias between the ground-based products of 58 ‰ on average in δD. NDACC-MUSICA was decidedly developed for water vapour isotopologue studies and is validated in δD with aircraft measurements; however, data are only available until 2014. TCCON provides recent data with temporal overlap with TROPOMI observations, and its H2O total column data product is validated against in situ measurements; however, its HDO data product is not verified. In order to obtain a suitable validation data set, TCCON HDO columns are scaled by a factor of 1.0778 to match the MUSICA δD over the common observation time period.
Using a co-location radius of 30 km, a maximal altitude difference of 500 m, a field of view of 45∘ and a maximal time difference of 2 h, a good agreement is found between corrected TCCON measurements and co-located TROPOMI observations. The mean bias is molec cm−2 ((1.1±7.2) %) for H2O, molec cm−2 ( %) for HDO and ‰ ((5.6±6.7) %) for δD. At low- and mid-latitude stations the bias in δD ranges between about −30 ‰ and +15 ‰, whereas at high-latitude stations it can be as high as −45 ‰ to −60 ‰. Retrievals at high latitudes are challenging due to long light paths and low albedos.
The use of the new data set is demonstrated in a case study of an atmospheric blocking event with a single TROPOMI overpass over northeastern Europe on 30 July 2018. Depleted air masses are found in the core of the anticyclone due to subsidence transporting upper tropospheric air towards lower levels. At the edge of the anticyclone a ring of enriched air is observed. A climatological study on the water vapour isotopic signature of continental summer blocking events could provide promising insights into the atmospheric water cycling associated with such systems that frequently lead to heat waves and hot temperature extremes. This case study shows the quality of the new data set and the added value for isotopologue studies, enabling studies on a day-by-day basis with high spatial resolution over continental regions.
Due to the restrictive filter for clear-sky scenes, the data coverage is limited. To improve on this, cloudy-sky retrievals over low clouds will be considered in a future study by using a forward model that accounts for scattering. Moreover, a calibration and validation of the TCCON HDO product is necessary. Additionally, it would be beneficial if recent NDACC-MUSICA data became available. Finally, an improvement in the consistency between the networks would be very valuable.
The TROPOMI HDO data set from this study is available for download at ftp://ftp.sron.nl/open-access-data-2/TROPOMI/tropomi/hdo/9_1/ (last access: 20 December 2019). MUSICA data are available from ftp://ftp.cpc.ncep.noaa.gov/ndacc/MUSICA/ (last access: 20 December 2019) and via https://doi.org/10.5281/zenodo.48902 (Barthlott et al., 2016). TCCON data are available from the TCCON Data Archive as follows:
-
https://doi.org/10.14291/tccon.ggg2014.izana01.r1 (Blumenstock et al., 2017);
-
https://doi.org/10.14291/tccon.ggg2014.bialystok01.r1/1183984 (Deutscher et al., 2015);
-
https://doi.org/10.14291/tccon.ggg2014.wollongong01.r0/1149291 (Griffith et al., 2014);
-
https://doi.org/10.14291/tccon.ggg2014.karlsruhe01.r1/1182416 (Kawakami et al., 2014);
-
https://doi.org/10.14291/tccon.ggg2014.sodankyla01.r0/1149280 (Kivi et al., 2014);
-
https://doi.org/10.14291/tccon.ggg2014.tsukuba02.r2 (Morino et al., 2018a);
-
https://doi.org/10.14291/tccon.ggg2014.burgos01.r0 (Morino et al., 2018b);
-
https://doi.org/10.14291/tccon.ggg2014.rikubetsu01.r2 (Morino et al., 2018c);
-
https://doi.org/10.14291/tccon.ggg2014.bremen01.r0/1149275 (Notholt et al., 2014);
-
https://doi.org/10.14291/tccon.ggg2014.nyalesund01.r0/1149278 (Notholt et al., 2017);
-
https://doi.org/10.14291/tccon.ggg2014.lauder03.r0 (Pollard et al., 2019);
-
https://doi.org/10.14291/tccon.ggg2014.lauder02.r0/1149298 (Sherlock et al., 2014);
-
https://doi.org/10.14291/tccon.ggg2014.eureka01.r3 (Strong et al., 2019);
-
https://doi.org/10.14291/tccon.ggg2014.paris01.r0/1149279 (Té et al., 2014);
-
https://doi.org/10.14291/tccon.ggg2014.orleans01.r0/1149276 (Warneke et al., 2019);
-
https://doi.org/10.14291/tccon.ggg2014.jpl02.r1/1330096 (Wennberg et al., 2014);
-
https://doi.org/10.14291/tccon.ggg2014.pasadena01.r1/1182415 (Wennberg et al., 2015);
-
https://doi.org/10.14291/tccon.ggg2014.lamont01.r1/1255070 (Wennberg et al., 2016);
-
https://doi.org/10.14291/tccon.ggg2014.parkfalls01.r1 (Wennberg et al., 2017); and
-
https://doi.org/10.14291/tccon.ggg2014.easttroutlake01.r1 (Wunch et al., 2018).
AS, TB, JadB and JL undertook the TROPOMI HDO retrievals and the analysis. FA carried out the case study in Sect. 5. DGF aided in the search for the cause of discrepancies between (uncorrected) TCCON HDO and TROPOMI HDO. RK and FH provided TCCON data. MS provided MUSICA data and TCCON data. All authors discussed the results and commented on the paper.
The authors declare that they have no conflict of interest.
Plots/data contain modified Copernicus Sentinel data, processed by SRON.
This work was supported by the ESA Living Planet Fellowship project Water vapour Isotopologues from TROPOMI (WIFT). The TROPOMI data processing was carried out on the Dutch National e-infrastructure with the support of the SURF Cooperative. The MUSICA project has been funded by the European Research Council under the European Community's Seventh Framework Programme (FP7/2007–2013)/ERC under grant agreement number 256961. Karlsruhe Institute of Technology acknowledges BMWi for funding TCCON data analysis and delivery via a DLR project (grant no 50EE1711A). The TCCON project for the Tsukuba site is supported in part by the GOSAT series project. Nicholas Deutscher, David Griffith, Laura T. Iraci, Isamu Morino, Justus Notholt, Christof Petri, Dave Pollard, Kei Shiomi, Kimberly Strong, Yao Té, Thorsten Warneke, Paul Wennberg and Debra Wunch provided TCCON data.
This research has been supported by the European Space Agency; SRON Netherlands Institute for Space Research (grant no. 4000125587/18/I-NS).
This paper was edited by John Worden and reviewed by Christian Frankenberg and one anonymous referee.
Aemisegger, F. and Papritz, L.: A Climatology of Strong Large-Scale Ocean Evaporation Events. Part I: Identification, Global Distribution, and Associated Climate Conditions, J. Climate, 31, 7287–7312, https://doi.org/10.1175/JCLI-D-17-0591.1, 2018. a
Aemisegger, F. and Sjolte, J.: A Climatology of Strong Large-Scale Ocean Evaporation Events. Part II: Relevance for the Deuterium Excess Signature of the Evaporation Flux, J. Climate, 31, 7313–7336, https://doi.org/10.1175/JCLI-D-17-0592.1, 2018. a
Aemisegger, F., Sturm, P., Graf, P., Sodemann, H., Pfahl, S., Knohl, A., and Wernli, H.: Measuring variations of δ18O and δ2H in atmospheric water vapour using two commercial laser-based spectrometers: an instrument characterisation study, Atmos. Meas. Tech., 5, 1491–1511, https://doi.org/10.5194/amt-5-1491-2012, 2012. a
Barthlott, S., Schneider, M., Hase, F., Blumenstock, T, Mengistu Tsidu, G., Grutter de la Mora, M., Strong, K., Notholt, J., Mahieu, E., Jones, N., and Smale, D.: The ground-based MUSICA dataset: Tropospheric water vapour isotopologues (H216O, H218O and HD16O) as obtained from NDACC/FTIR solar absorption spectra, https://doi.org/10.5281/zenodo.48902, Zenodo, 2016. a, b, c, d, e, f, g, h
Barthlott, S., Schneider, M., Hase, F., Blumenstock, T., Kiel, M., Dubravica, D., García, O. E., Sepúlveda, E., Mengistu Tsidu, G., Takele Kenea, S., Grutter, M., Plaza-Medina, E. F., Stremme, W., Strong, K., Weaver, D., Palm, M., Warneke, T., Notholt, J., Mahieu, E., Servais, C., Jones, N., Griffith, D. W. T., Smale, D., and Robinson, J.: Tropospheric water vapour isotopologue data (H216O, H218O, and HD16O) as obtained from NDACC/FTIR solar absorption spectra, Earth Syst. Sci. Data, 9, 15–29, https://doi.org/10.5194/essd-9-15-2017, 2017. a, b
Bastrikov, V., Steen-Larsen, H. C., Masson-Delmotte, V., Gribanov, K., Cattani, O., Jouzel, J., and Zakharov, V.: Continuous measurements of atmospheric water vapour isotopes in western Siberia (Kourovka), Atmos. Meas. Tech., 7, 1763–1776, https://doi.org/10.5194/amt-7-1763-2014, 2014. a, b
Blumenstock, T., Hase, F., Schneider, M., García, O. E., and Sepúlveda, E.: TCCON data from Izaña (ES), Release GGG2014.R1, https://doi.org/10.14291/tccon.ggg2014.izana01.r1, 2017. a, b
Boesch, H., Deutscher, N. M., Warneke, T., Byckling, K., Cogan, A. J., Griffith, D. W. T., Notholt, J., Parker, R. J., and Wang, Z.: HDO∕H2O ratio retrievals from GOSAT, Atmos. Meas. Tech., 6, 599–612, https://doi.org/10.5194/amt-6-599-2013, 2013. a
Borsdorff, T., Hasekamp, O. P., Wassmann, A., and Landgraf, J.: Insights into Tikhonov regularization: application to trace gas column retrieval and the efficient calculation of total column averaging kernels, Atmos. Meas. Tech., 7, 523–535, https://doi.org/10.5194/amt-7-523-2014, 2014. a
Copernicus Climate Service: online, available at: https://climate.copernicus.eu/dry-and-warm-spring-and-summer, (last access: 21 May 2019), 2018. a
Coplen, T. B.: Guidelines and recommended terms for expression of stable-isotope-ratio and gas-ratio measurement results, Rapid Commun. Mass Spectrom., 25, 2538–2560, https://doi.org/10.1002/rcm.5129, 2011. a
Craig, H. and Gordon, L. I.: Deuterium and oxygen 18 variations in the ocean and the marine atmosphere, in: Stable Isotopes in Oceanographic Studies and Paleotemperatures, edited by: Tongiorgi, E., Laboratorio di geologia nucleare, Pisa, 9–130, 1965. a
Dansgaard, W.: Stable isotopes in precipitation, Tellus, 16, 436–468, https://doi.org/10.3402/tellusa.v16i4.8993, 1964. a, b, c, d
De Mazière, M., Thompson, A. M., Kurylo, M. J., Wild, J. D., Bernhard, G., Blumenstock, T., Braathen, G. O., Hannigan, J. W., Lambert, J.-C., Leblanc, T., McGee, T. J., Nedoluha, G., Petropavlovskikh, I., Seckmeyer, G., Simon, P. C., Steinbrecht, W., and Strahan, S. E.: The Network for the Detection of Atmospheric Composition Change (NDACC): history, status and perspectives, Atmos. Chem. Phys., 18, 4935–4964, https://doi.org/10.5194/acp-18-4935-2018, 2018. a
Deutscher, N. M., Notholt, J., Messerschmidt, J., Weinzierl, C., Warneke, T., Petri, C., and Grupe, P.: TCCON data from Bialystok (PL), Release GGG2014.R1, https://doi.org/10.14291/tccon.ggg2014.bialystok01.r1/1183984, 2015. a, b
Dyroff, C., Fütterer, D., and Zahn, A.: Compact diode-laser spectrometer ISOWAT for highly sensitive airborne measurements of water-isotope ratios, Appl. Phys. B, 98, 537–548, https://doi.org/10.1007/s00340-009-3775-6, 2010. a
Dyroff, C., Sanati, S., Christner, E., Zahn, A., Balzer, M., Bouquet, H., McManus, J. B., González-Ramos, Y., and Schneider, M.: Airborne in situ vertical profiling of HDO∕H216O in the subtropical troposphere during the MUSICA remote sensing validation campaign, Atmos. Meas. Tech., 8, 2037–2049, https://doi.org/10.5194/amt-8-2037-2015, 2015. a, b
Frankenberg, C., Yoshimura, K., Warneke, T., Aben, I., Butz, A., Deutscher, N., Griffith, D., Hase, F., Notholt, J., Schneider, M., Schrijver, H., and Röckmann, T.: Dynamic Processes Governing Lower-Tropospheric HDO/H2O Ratios as Observed from Space and Ground, Science, 325, 1374–1377, https://doi.org/10.1126/science.1173791, 2009. a
Frankenberg, C., Wunch, D., Toon, G., Risi, C., Scheepmaker, R., Lee, J.-E., Wennberg, P., and Worden, J.: Water vapor isotopologue retrievals from high-resolution GOSAT shortwave infrared spectra, Atmos. Meas. Tech., 6, 263–274, https://doi.org/10.5194/amt-6-263-2013, 2013. a
González, Y., Schneider, M., Dyroff, C., Rodríguez, S., Christner, E., García, O. E., Cuevas, E., Bustos, J. J., Ramos, R., Guirado-Fuentes, C., Barthlott, S., Wiegele, A., and Sepúlveda, E.: Detecting moisture transport pathways to the subtropical North Atlantic free troposphere using paired H2O−δD in situ measurements, Atmos. Chem. Phys., 16, 4251–4269, https://doi.org/10.5194/acp-16-4251-2016, 2016. a
Gordon, I., Rothman, L., Hill, C., Kochanov, R., Tan, Y., Bernath, P., Birk, M., Boudon, V., Campargue, A., Chance, K., Drouin, B., Flaud, J.-M., Gamache, R., Hodges, J., Jacquemart, D., Perevalov, V., Perrin, A., Shine, K., Smith, M.-A., Tennyson, J., Toon, G., Tran, H., Tyuterev, V., Barbe, A., Császár, A., Devi, V., Furtenbacher, T., Harrison, J., Hartmann, J.-M., Jolly, A., Johnson, T., Karman, T., Kleiner, I., Kyuberis, A., Loos, J., Lyulin, O., Massie, S., Mikhailenko, S., Moazzen-Ahmadi, N., Müller, H., Naumenko, O., Nikitin, A., Polyansky, O., Rey, M., Rotger, M., Sharpe, S., Sung, K., Starikova, E., Tashkun, S., Auwera, J. V., Wagner, G., Wilzewski, J., Wcisło, P., Yu, S., and Zak, E.: The HITRAN2016 molecular spectroscopic database, J. Quant. Spectrosc. Ra., 203, 3–69, https://doi.org/10.1016/j.jqsrt.2017.06.038, 2017. a
Griffith, D. W., Velazco, V. A., Deutscher, N. M., Paton-Walsh, C., Jones, N. B., Wilson, S. R., Macatangay, R. C., Kettlewell, G. C., Buchholz, R. R., and Riggenbach, M. O.: TCCON data from Wollongong (AU), Release GGG2014.R0, https://doi.org/10.14291/tccon.ggg2014.wollongong01.r0/1149291, 2014. a, b, c
Gubler, S., Scherrer, S., Bader, S., Burgstall, A., Casanueva, A., Duguay-Tetzlaff, A., Gehrig, R., Kotlarski, S., and Spirig, C.: Hitze und Trockenheit im Sommerhalbjahr 2018 – eine klimatologische Übersicht, Fachbericht MeteoSchweiz 272, MeteoSchweiz, available at: https://www.meteoschweiz.admin.ch/content/dam/meteoswiss/de/service-und-publikationen/Publikationen/doc/Fachbericht_TrockenheitHitze_2018_final_d.pdf, (last access: 7 June 2019), 2018. a
Harries, J. E.: Atmospheric radiation and atmospheric humidity, Q. J. Roy. Meteor. Soc., 123, 2173–2186, https://doi.org/10.1002/qj.49712354402, 1997. a
Hase, F., Blumenstock, T., Dohe, S., Groß, J., and Kiel, M.: TCCON data from Karlsruhe (DE), Release GGG2014.R1, https://doi.org/10.14291/tccon.ggg2014.karlsruhe01.r1/1182416, 2015. a, b
Herbin, H., Hurtmans, D., Clerbaux, C., Clarisse, L., and Coheur, P.-F.: H216O and HDO measurements with IASI/MetOp, Atmos. Chem. Phys., 9, 9433–9447, https://doi.org/10.5194/acp-9-9433-2009, 2009. a
Herman, R. L., Cherry, J. E., Young, J., Welker, J. M., Noone, D., Kulawik, S. S., and Worden, J.: Aircraft validation of Aura Tropospheric Emission Spectrometer retrievals of HDO∕H2O, Atmos. Meas. Tech., 7, 3127–3138, https://doi.org/10.5194/amt-7-3127-2014, 2014. a
Hoffmann, G., Werner, M., and Heimann, M.: Water isotope module of the ECHAM atmospheric general circulation model: A study on timescales from days to several years, J. Geophys. Res., 103, 16871–16896, https://doi.org/10.1029/98JD00423, 1998. a
Hu, H., Landgraf, J., Detmers, R., Borsdorff, T., Aan de Brugh, J., Aben, I., Butz, A., and Hasekamp, O.: Toward Global Mapping of Methane With TROPOMI: First Results and Intersatellite Comparison to GOSAT, Geophys. Res. Lett., 45, 3682–3689, https://doi.org/10.1002/2018GL077259, 2018. a
Iraci, L. T., Podolske, J. R., Hillyard, P. W., Roehl, C., Wennberg, P. O., Blavier, J.-F., Landeros, J., Allen, N., Wunch, D., Zavaleta, J., Quigley, E., Osterman, G. B., Albertson, R., Dunwoody, K., and Boyden, H.: TCCON data from Edwards (US), Release GGG2014.R1, https://doi.org/10.14291/tccon.ggg2014.edwards01.r1/1255068, 2016. a
Joussaume, S., Sadourny, R., and Jouzel, J.: A general circulation model of water isotope cycles in the atmosphere, Nature, 311, 24–29, https://doi.org/10.1038/311024a0, 1984. a
Kawakami, S., Ohyama, H., Arai, K., Okumura, H., Taura, C., Fukamachi, T., and Sakashita, M.: TCCON data from Saga (JP), Release GGG2014.R0, https://doi.org/10.14291/tccon.ggg2014.saga01.r0/1149283, 2014. a, b
Kiehl, J. T. and Trenberth, K. E.: Earth's Annual Global Mean Energy Budget, B. Am. Meteorol. Soc., 78, 197–208, https://doi.org/10.1175/1520-0477(1997)078<0197:EAGMEB>2.0.CO;2, 1997. a
Kivi, R., Heikkinen, P., and Kyrö, E.: TCCON data from Sodankylä (FI), Release GGG2014.R0, https://doi.org/10.14291/tccon.ggg2014.sodankyla01.r0/1149280, 2014. a, b
Krol, M., Houweling, S., Bregman, B., van den Broek, M., Segers, A., van Velthoven, P., Peters, W., Dentener, F., and Bergamaschi, P.: The two-way nested global chemistry-transport zoom model TM5: algorithm and applications, Atmos. Chem. Phys., 5, 417–432, https://doi.org/10.5194/acp-5-417-2005, 2005. a
Lacour, J.-L., Risi, C., Clarisse, L., Bony, S., Hurtmans, D., Clerbaux, C., and Coheur, P.-F.: Mid-tropospheric δD observations from IASI/MetOp at high spatial and temporal resolution, Atmos. Chem. Phys., 12, 10817–10832, https://doi.org/10.5194/acp-12-10817-2012, 2012. a
Lacour, J.-L., Flamant, C., Risi, C., Clerbaux, C., and Coheur, P.-F.: Importance of the Saharan heat low in controlling the North Atlantic free tropospheric humidity budget deduced from IASI δD observations, Atmos. Chem. Phys., 17, 9645–9663, https://doi.org/10.5194/acp-17-9645-2017, 2017. a
Landgraf, J., aan de Brugh, J., Scheepmaker, R., Borsdorff, T., Hu, H., Houweling, S., Butz, A., Aben, I., and Hasekamp, O.: Carbon monoxide total column retrievals from TROPOMI shortwave infrared measurements, Atmos. Meas. Tech., 9, 4955–4975, https://doi.org/10.5194/amt-9-4955-2016, 2016. a
Morino, I., Matsuzaki, T., and Horikawa, M.: TCCON data from Tsukuba (JP), 125HR, Release GGG2014.R2, https://doi.org/10.14291/tccon.ggg2014.tsukuba02.r2, 2018a. a, b
Morino, I., Velazco, V. A., Hori, A., Uchino, O., and Griffith, D. W.: TCCON data from Burgos, Ilocos Norte (PH), Release GGG2014.R0, https://doi.org/10.14291/tccon.ggg2014.burgos01.r0, 2018b. a, b
Morino, I., Yokozeki, N., Matsuzaki, T., and Horikawa, M.: TCCON data from Rikubetsu (JP), Release GGG2014.R2, https://doi.org/10.14291/tccon.ggg2014.rikubetsu01.r2, 2018c. a, b
Noone, D.: Pairing Measurements of the Water Vapor Isotope Ratio with Humidity to Deduce Atmospheric Moistening and Dehydration in the Tropical Midtroposphere, J. Climate, 25, 4476–4494, https://doi.org/10.1175/JCLI-D-11-00582.1, 2012. a
Notholt, J., Petri, C., Warneke, T., Deutscher, N. M., Palm, M., Buschmann, M., Weinzierl, C., Macatangay, R. C., and Grupe, P.: TCCON data from Bremen (DE), Release GGG2014.R0, https://doi.org/10.14291/tccon.ggg2014.bremen01.r0/1149275, 2014. a, b, c
Notholt, J., Warneke, T., Petri, C., Deutscher, N. M., Weinzierl, C., Palm, M., and Buschmann, M.: TCCON data from Ny Ålesund, Spitsbergen (NO), Release GGG2014.R0, https://doi.org/10.14291/tccon.ggg2014.nyalesund01.r0/1149278, 2017. a, b
Payne, V. H., Noone, D., Dudhia, A., Piccolo, C., and Grainger, R. G.: Global satellite measurements of HDO and implications for understanding the transport of water vapour into the stratosphere, Q. J. Roy. Meteor. Soc., 133, 1459–1471, https://doi.org/10.1002/qj.127, 2007. a
Pfahl, S. and Wernli, H.: Quantifying the relevance of atmospheric blocking for co-located temperature extremes in the Northern Hemisphere on (sub-)daily time scales, Geophys. Res. Lett., 39, L12807, https://doi.org/10.1029/2012GL052261, 2012. a
Pfahl, S., Wernli, H., and Yoshimura, K.: The isotopic composition of precipitation from a winter storm – a case study with the limited-area model COSMOiso, Atmos. Chem. Phys., 12, 1629–1648, https://doi.org/10.5194/acp-12-1629-2012, 2012. a
Pollard, D. F., Robinson, J., and Shiona, H.: TCCON data from Lauder (NZ), Release GGG2014.R0, https://doi.org/10.14291/tccon.ggg2014.lauder03.r0, 2019. a, b, c
Rast, M., Johannessen, J., and Mauser, W.: Review of Understanding of Earth's Hydrological Cycle: Observations, Theory and Modelling, Surv. Geophys., 35, 491–513, https://doi.org/10.1007/s10712-014-9279-x, 2014. a
Rayleigh, J. W. S.: On the distillation of binary mixtures, Philos. Mag., 4, 521–537, https://doi.org/10.1080/14786440209462876, 1902. a
Rinsland, C. P., Goldman, A., Devi, V. M., Fridovich, B., Snyder, D. G. S., Jones, G. D., Murcray, F. J., Murcray, D. G., Smith, M. A. H., Seals, R. K., Coffey, M. T., and Mankin, W. G.: Simultaneous stratospheric measurements of H2O, HDO, and CH4 from balloon-borne and aircraft infrared solar absorption spectra and tunable diode laser laboratory spectra of HDO, J. Geophys. Res., 89, 7259–7266, https://doi.org/10.1029/JD089iD05p07259, 1984. a
Risi, C., Bony, S., Vimeux, F., and Jouzel, J.: Water-stable isotopes in the LMDZ4 general circulation model: Model evaluation for present-day and past climates and applications to climatic interpretations of tropical isotopic records, J. Geophys. Res., 115, D12118, https://doi.org/10.1029/2009JD013255, 2010. a
Rodgers, C. D.: Inverse methods for atmospheric sounding: theory and practice, vol. 2 of Series on atmospheric, oceanic and planetary physics, World Scientific, Singapore, 2000. a
Rozanski, K. and Sonntag, C.: Vertical distribution of deuterium in atmospheric water vapour, Tellus, 34, 135–141, https://doi.org/10.3402/tellusa.v34i2.10795, 1982. a
Scheepmaker, R. A., Frankenberg, C., Deutscher, N. M., Schneider, M., Barthlott, S., Blumenstock, T., Garcia, O. E., Hase, F., Jones, N., Mahieu, E., Notholt, J., Velazco, V., Landgraf, J., and Aben, I.: Validation of SCIAMACHY HDO∕H2O measurements using the TCCON and NDACC-MUSICA networks, Atmos. Meas. Tech., 8, 1799–1818, https://doi.org/10.5194/amt-8-1799-2015, 2015. a
Scheepmaker, R. A., aan de Brugh, J., Hu, H., Borsdorff, T., Frankenberg, C., Risi, C., Hasekamp, O., Aben, I., and Landgraf, J.: HDO and H2O total column retrievals from TROPOMI shortwave infrared measurements, Atmos. Meas. Tech., 9, 3921–3937, https://doi.org/10.5194/amt-9-3921-2016, 2016. a, b, c
Schneider, A., Borsdorff, T., aan de Brugh, J., Hu, H., and Landgraf, J.: A full-mission data set of H2O and HDO columns from SCIAMACHY 2.3 µm reflectance measurements, Atmos. Meas. Tech., 11, 3339–3350, https://doi.org/10.5194/amt-11-3339-2018, 2018. a, b, c
Schneider, M. and Hase, F.: Optimal estimation of tropospheric H2O and δD with IASI/METOP, Atmos. Chem. Phys., 11, 11207–11220, https://doi.org/10.5194/acp-11-11207-2011, 2011. a
Schneider, M., González, Y., Dyroff, C., Christner, E., Wiegele, A., Barthlott, S., García, O. E., Sepúlveda, E., Hase, F., Andrey, J., Blumenstock, T., Guirado, C., Ramos, R., and Rodríguez, S.: Empirical validation and proof of added value of MUSICA's tropospheric δD remote sensing products, Atmos. Meas. Tech., 8, 483–503, https://doi.org/10.5194/amt-8-483-2015, 2015. a, b
Schneider, M., Wiegele, A., Barthlott, S., González, Y., Christner, E., Dyroff, C., García, O. E., Hase, F., Blumenstock, T., Sepúlveda, E., Mengistu Tsidu, G., Takele Kenea, S., Rodríguez, S., and Andrey, J.: Accomplishments of the MUSICA project to provide accurate, long-term, global and high-resolution observations of tropospheric {H2O,δD} pairs – a review, Atmos. Meas. Tech., 9, 2845–2875, https://doi.org/10.5194/amt-9-2845-2016, 2016. a, b, c
Sherlock, V., Connor, B., Robinson, J., Shiona, H., Smale, D., and Pollard, D. F.: TCCON data from Lauder (NZ), 125HR, Release GGG2014.R0, https://doi.org/10.14291/tccon.ggg2014.lauder02.r0/1149298, 2014. a, b, c
Siddans, R.: S5P-NPP Cloud Processor ATBD, Tech. rep., Rutherford Appleton Laboratory, available at: http://www.tropomi.eu/sites/default/files/files/S5P-NPPC-RAL-ATBD-0001_NPP-Clouds_v1p0p0_20160212.pdf (last access: 20 December 2019), 2016. a
Sodemann, H., Aemisegger, F., Pfahl, S., Bitter, M., Corsmeier, U., Feuerle, T., Graf, P., Hankers, R., Hsiao, G., Schulz, H., Wieser, A., and Wernli, H.: The stable isotopic composition of water vapour above Corsica during the HyMeX SOP1 campaign: insight into vertical mixing processes from lower-tropospheric survey flights, Atmos. Chem. Phys., 17, 6125–6151, https://doi.org/10.5194/acp-17-6125-2017, 2017. a
Steinwagner, J., Milz, M., von Clarmann, T., Glatthor, N., Grabowski, U., Höpfner, M., Stiller, G. P., and Röckmann, T.: HDO measurements with MIPAS, Atmos. Chem. Phys., 7, 2601–2615, https://doi.org/10.5194/acp-7-2601-2007, 2007. a
Stevens, B. and Bony, S.: What Are Climate Models Missing?, Science, 340, 1053–1054, https://doi.org/10.1126/science.1237554, 2013. a
Strong, K., Roche, S., Franklin, J. E., Mendonca, J., Lutsch, E., Weaver, D., Fogal, P. F., Drummond, J. R., Batchelor, R., and Lindenmaier, R.: TCCON data from Eureka (CA), Release GGG2014.R3, https://doi.org/10.14291/tccon.ggg2014.eureka01.r3, 2019. a, b, c
Té, Y., Jeseck, P., and Janssen, C.: TCCON data from Paris (FR), Release GGG2014.R0, https://doi.org/10.14291/tccon.ggg2014.paris01.r0/1149279, 2014. a, b
Trigo, R. M., Trigo, I. F., DaCamara, C. C., and Osborn, T. J.: Climate impact of the European winter blocking episodes from the NCEP/NCAR Reanalyses, Clim. Dynam., 23, 17–28, https://doi.org/10.1007/s00382-004-0410-4, 2004. a
Veefkind, J., Aben, I., McMullan, K., Förster, H., de Vries, J., Otter, G., Claas, J., Eskes, H., de Haan, J., Kleipool, Q., van Weele, M., Hasekamp, O., Hoogeveen, R., Landgraf, J., Snel, R., Tol, P., Ingmann, P., Voors, R., Kruizinga, B., Vink, R., Visser, H., and Levelt, P.: TROPOMI on the ESA Sentinel-5 Precursor: A GMES mission for global observations of the atmospheric composition for climate, air quality and ozone layer applications, Remote Sens. Environ., 120, 70–83, https://doi.org/10.1016/j.rse.2011.09.027, 2012. a
Warneke, T., Messerschmidt, J., Notholt, J., Weinzierl, C., Deutscher, N. M., Petri, C., and Grupe, P.: TCCON data from Orléans (FR), Release GGG2014.R0, https://doi.org/10.14291/tccon.ggg2014.orleans01.r0/1149276, 2019. a, b
Weaver, D.: Water Vapour Measurements in the Canadian High Arctic, phdthesis, University of Toronto, available at: http://hdl.handle.net/1807/95942 (last access: 20 December 2019), 2019. a
Wen, X.-F., Zhang, S.-C., Sun, X.-M., Yu, G.-R., and Lee, X.: Water vapor and precipitation isotope ratios in Beijing, China, J. Geophys. Res., 115, D01103, https://doi.org/10.1029/2009JD012408, 2010. a
Wennberg, P. O., Roehl, C. M., Blavier, J.-F., Wunch, D., and Allen, N. T.: TCCON data from Jet Propulsion Laboratory (US), 2011, Release GGG2014.R1, https://doi.org/10.14291/tccon.ggg2014.jpl02.r1/1330096, 2014. a, b
Wennberg, P. O., Wunch, D., Roehl, C. M., Blavier, J.-F., Toon, G. C., and Allen, N. T.: TCCON data from Caltech (US), Release GGG2014.R1, https://doi.org/10.14291/tccon.ggg2014.pasadena01.r1/1182415, 2015. a, b
Wennberg, P. O., Wunch, D., Roehl, C. M., Blavier, J.-F., Toon, G. C., and Allen, N. T.: TCCON data from Lamont (US), Release GGG2014.R1, https://doi.org/10.14291/tccon.ggg2014.lamont01.r1/1255070, 2016. a, b
Wennberg, P. O., Roehl, C. M., Wunch, D., Toon, G. C., Blavier, J.-F., Washenfelder, R., Keppel-Aleks, G., Allen, N. T., and Ayers, J.: TCCON data from Park Falls (US), Release GGG2014.R1, https://doi.org/10.14291/tccon.ggg2014.parkfalls01.r1, 2017. a, b
Worden, J., Bowman, K., Noone, D., Beer, R., Clough, S., Eldering, A., Fisher, B., Goldman, A., Gunson, M., Herman, R., Kulawik, S. S., Lampel, M., Luo, M., Osterman, G., Rinsland, C., Rodgers, C., Sander, S., Shephard, M., and Worden, H.: Tropospheric Emission Spectrometer observations of the tropospheric HDO∕H2O ratio: Estimation approach and characterization, J. Geophys. Res., 111, D16309, https://doi.org/10.1029/2005JD006606, 2006. a
Worden, J., Noone, D., Bowman, K., The Tropospheric Emission Spectrometer, and data contributors: Importance of rain evaporation and continental convection in the tropical water cycle, Nature, 445, 528–532, https://doi.org/10.1038/nature05508, 2007. a
Worden, J. R., Kulawik, S. S., Fu, D., Payne, V. H., Lipton, A. E., Polonsky, I., He, Y., Cady-Pereira, K., Moncet, J.-L., Herman, R. L., Irion, F. W., and Bowman, K. W.: Characterization and evaluation of AIRS-based estimates of the deuterium content of water vapor, Atmos. Meas. Tech., 12, 2331–2339, https://doi.org/10.5194/amt-12-2331-2019, 2019. a
Wunch, D., Toon, G. C., Blavier, J.-F. L., Washenfelder, R. A., Notholt, J., Connor, B. J., Griffith, D. W. T., Sherlock, V., and Wennberg, P. O.: The Total Carbon Column Observing Network, Philos. T. Roy. Soc. A, 369, 2087–2112, https://doi.org/10.1098/rsta.2010.0240, 2011. a
Wunch, D., Toon, G. C., Sherlock, V., Deutscher, N. M., Liu, X., Feist, D. G., and Wennberg, P. O.: The Total Carbon Column Observing Network's GGG2014 Data Version, Tech. rep., TCCON, https://doi.org/10.14291/tccon.ggg2014.documentation.R0/1221662, 2015. a, b
Wunch, D., Mendonca, J., Colebatch, O., Allen, N. T., Blavier, J.-F., Roche, S., Hedelius, J., Neufeld, G., Springett, S., Worthy, D., Kessler, R., and Strong, K.: TCCON data from East Trout Lake, SK (CA), Release GGG2014.R1, https://doi.org/10.14291/tccon.ggg2014.easttroutlake01.r1, 2018. a, b
Yoshimura, K., Kanamitsu, M., Noone, D., and Oki, T.: Historical isotope simulation using Reanalysis atmospheric data, J. Geophys. Res., 113, D19108, https://doi.org/10.1029/2008JD010074, 2008. a
Zakharov, V. I., Imasu, R., Gribanov, K. G., Hoffmann, G., and Jouzel, J.: Latitudinal distribution of the deuterium to hydrogen ratio in the atmospheric water vapor retrieved from IMG/ADEOS data, Geophys. Res. Lett., 31, L12104, https://doi.org/10.1029/2004GL019433, 2004. a
- Abstract
- Introduction
- Retrieval method
- Ground-based FTIR data sets
- Validation of TROPOMI retrievals
- Demonstration of applications of the data set
- Summary and conclusions
- Data availability
- Author contributions
- Competing interests
- Disclaimer
- Acknowledgements
- Financial support
- Review statement
- References
- Abstract
- Introduction
- Retrieval method
- Ground-based FTIR data sets
- Validation of TROPOMI retrievals
- Demonstration of applications of the data set
- Summary and conclusions
- Data availability
- Author contributions
- Competing interests
- Disclaimer
- Acknowledgements
- Financial support
- Review statement
- References