the Creative Commons Attribution 4.0 License.
the Creative Commons Attribution 4.0 License.
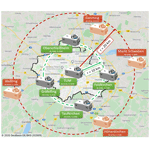
MUCCnet: Munich Urban Carbon Column network
Benno Voggenreiter
Patrick Aigner
Nico Nachtigall
Björn Reger
In order to mitigate climate change, it is crucial to understand urban greenhouse gas (GHG) emissions precisely, as more than two-thirds of the anthropogenic GHG emissions worldwide originate from cities. Nowadays, urban emission estimates are mainly based on bottom-up calculation approaches with high uncertainties. A reliable and long-term top-down measurement approach could reduce the uncertainty of these emission inventories significantly.
We present the Munich Urban Carbon Column network (MUCCnet), the world's first urban sensor network, which has been permanently measuring GHGs, based on the principle of differential column measurements (DCMs), since summer 2019. These column measurements and column concentration differences are relatively insensitive to vertical redistribution of tracer masses and surface fluxes upwind of the city, making them a favorable input for an inversion framework and, therefore, a well-suited candidate for the quantification of GHG emissions.
However, setting up such a stationary sensor network requires an automated measurement principle. We developed our own fully automated enclosure systems for measuring column-averaged CO2, CH4 and CO concentrations with a solar-tracking Fourier transform spectrometer (EM27/SUN) in a fully automated and long-term manner. This also includes software that starts and stops the measurements autonomously and can be used independently from the enclosure system.
Furthermore, we demonstrate the novel applications of such a sensor network by presenting the measurement results of our five sensor systems that are deployed in and around Munich. These results include the seasonal cycle of CO2 since 2015, as well as concentration gradients between sites upwind and downwind of the city. Thanks to the automation, we were also able to continue taking measurements during the COVID-19 lockdown in spring 2020. By correlating the CO2 column concentration gradients to the traffic amount, we demonstrate that our network is capable of detecting variations in urban emissions.
The measurements from our unique sensor network will be combined with an inverse modeling framework that we are currently developing in order to monitor urban GHG emissions over years, identify unknown emission sources and assess how effective the current mitigation strategies are. In summary, our achievements in automating column measurements of GHGs will allow researchers all over the world to establish this approach for long-term greenhouse gas monitoring in urban areas.
- Article
(7102 KB) - Full-text XML
- BibTeX
- EndNote
Climate change is one of the defining issues of our time, and one that affects the entire planet. To reduce greenhouse gas (GHG) emissions effectively, accurate and continuous monitoring systems for local- and regional-scale emissions are a prerequisite.
Especially for urban areas, which contribute to more than 70 % of global fossil fuel CO2 emissions (Gurney et al., 2015) and are therefore hotspots, there is a shortage of accurate emissions assessments. The city emission inventories often underestimate emissions due to unknown emission sources that are not yet included in the inventories (Chen et al., 2020; Plant et al., 2019; McKain et al., 2015).
In recent years, several city networks have been established to improve emission monitoring. These include networks using in situ high-precision instruments (McKain et al., 2015; Bréon et al., 2015; Xueref-Remy et al., 2018; Lamb et al., 2016) and low-cost sensor networks deploying non-dispersive infrared sensors (Kim et al., 2018; Shusterman et al., 2016). In addition, eddy covariance flux tower measurements are used for directly inferring city fluxes (Feigenwinter et al., 2012; Helfter et al., 2011). However, all these approaches involve some challenges when it comes to measuring urban emission fluxes, such as high sensitivity to the boundary layer height dynamics, large variations due to mesoscale transport phenomena or the fact that they can only capture the fluxes of a rather small area.
Column measurements have proven to be a powerful tool for assessing GHG emissions from cities and local sources, because they are relatively insensitive to the dynamics of the boundary layer height and surface fluxes upwind of the city if a differential approach is used (Chen et al., 2016). Therefore, this method has recently been widely deployed for emission studies of cities and local sources using mass balance or other modeling techniques. In St. Petersburg, Makarova et al. (2020) deployed two compact solar-tracking Fourier transform infrared (FTIR) spectrometers (EM27/SUN) and a mass balance approach to study the emissions from the fourth-largest European city. The EM27/SUN spectrometer has been developed by the Karlsruhe Institute of Technology (KIT) in collaboration with Bruker and has been commercially available since 2014 (Gisi et al., 2011, 2012; Hase et al., 2016). Hase et al. (2015) and Zhao et al. (2019) used the measurements of five EM27/SUNs to measure emissions of CO2 and CH4 in Berlin. With a similar sensor configuration, Vogel et al. (2019) studied the Paris metropolitan area and applied the CHIMERE-CAMS model to show that the measured concentration enhancements are mainly due to fossil fuel emissions. Jones et al. (2021) combined measurements from Indianapolis (five EM27/SUNs) with an adapted inverse modeling technique to determine the urban GHG emissions.
Besides these urban studies, column measurements are also used to investigate local sources: Chen et al. (2016) and Viatte et al. (2017) determined the source strength of dairy farms in Chino, California. By combining column measurements with a computational fluid dynamics (CFD) model, Toja-Silva et al. (2017) verified the emission inventory of the largest gas-fired power plant in Munich. With mobile setups, Butz et al. (2017) studied emissions from the volcano Mt. Etna, Luther et al. (2019) quantified the coal mine emissions from upper Silesia and Klappenbach et al. (2015) utilized column measurements on a research vessel for satellite validations above the ocean. However, these studies are all based on the campaign mode and not suited for monitoring the urban emissions permanently. Only TCCON (Total Carbon Column Observing Network; Wunch et al. (2011)) and COCCON (Collaborative Carbon Column Observing Network; Frey et al., 2019; Sha et al., 2020) are measuring the global GHG column concentrations permanently. For this purpose, TCCON uses IFS 125HR spectrometers (resolution: 0.02 cm−1), while COCCON uses calibrated EM27/SUN spectrometers (resolution: 0.5 cm−1). However, both networks focus on detecting GHG background concentrations and are not primarily designed to study urban emissions.
In this paper, we present the Munich Urban Carbon Column network (MUCCnet), the permanent urban GHG network in Munich, which is based on the differential column measurement (DCM) principle and consists of five fully automated FTIR spectrometers. The combination of our sensor network with a suitable modeling framework will build the basis for monitoring urban GHG emissions over years, identifying unknown emission sources, validating satellite-based GHG measurements and assessing the effectiveness of the current mitigation strategies.
As a measurement principle, the DCM method is used (Chen et al., 2016). DCM is an effective approach for determining the emissions of large-area sources using just a small number of stationary ground-based instruments. The basic principle of DCM is illustrated in Fig. 1. The column-averaged concentrations of a gas in the atmosphere are measured upwind and downwind of an emission source, utilizing ground-based FTIR spectrometers that use the sun as a light source. The concentration enhancements between the two stations are caused by the urban emissions. Chen et al. (2016) have shown that the differences between the upwind and downwind column concentrations are relatively insensitive to the boundary layer height and upstream influences. Therefore, DCM in combination with a wind-driven atmospheric transport model can be used to determine emissions.
In order to use the DCM principle for long-term monitoring of the urban GHG emissions, a fully automated measurement system is needed. For this, we developed an electronically controlled enclosure system that includes the related software.
3.1 Hardware
The enclosure system protects the spectrometer inside against harsh weather conditions and other harmful events, such as power or sensor failures. Furthermore, it enables communication between the devices inside the system and allows the host to remotely control the measurements over the internet. Under suitable measuring conditions, such as sunny weather and valid sun elevations, the system automatically starts the measurement process. During the day, the measurements are checked regularly by the enclosure software to detect and solve malfunctions autonomously. When the measuring conditions are no longer suitable, the system stops taking measurements and closes the cover to secure the spectrometer. An operator is informed about any unexpected behavior by email.
3.1.1 Standard edition
The described enclosure is based on our first prototype system, presented in Heinle and Chen (2018), which has been continuously running on the rooftop of the Technical University of Munich (TUM) in Munich's inner city since 2016. This system was developed to semi-automate the measurement process using an EM27/SUN spectrometer over the years. For the permanent urban GHG network, we improved this system to make it more reliable, easier to transport and fully autonomous.
Our new enclosure system is based on a lightweight yet robust aluminum housing (Zarges K470 box, waterproof according to IP54) that we modified for our purposes. The CAD model of this system is shown in Fig. 2. A rotating cover at the top of the housing allows the sunrays to hit the mirrors of the solar tracker at arbitrary azimuth and elevation angles. Every 10∘, a magnet is fixed in the outer cover (see Fig. 3). Reed sensors in the inner cover count these signals so that the relative position of the cover can be computed. Before the cover is opened and after every full rotation, two additional reed sensors indicate the absolute zero position. The target position of the cover is computed automatically depending on the coordinates of the site and the time. Optical rain and direct solar radiation sensors indicate whether the current environmental conditions are suited for measurements.
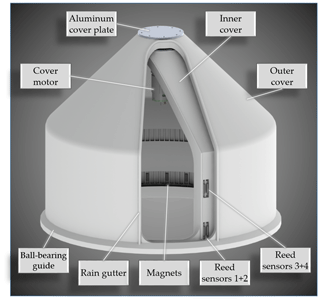
Figure 3CAD model of the newly designed cover (outer and inner one) with a small opening and a steeper slope compared to the first version in Heinle and Chen (2018). With the help of the reed sensors 1 and 2, the relative position of the cover is calculated (in 10∘ steps). The second sensor indicates the direction. The reed sensors 3 and 4 are used to determine the absolute zero position each time before the cover opens.
Signal lamps, push buttons and an emergency stop button can be used to control the basic functions of the enclosure directly at the site. Full control can only be achieved by remote access to the enclosure computer, which is an industrial embedded box PC. In addition to the remote access, the computer is also responsible for controlling the spectrometer and the solar tracker and for storing the interferograms before they are transferred to our retrieval cloud via the internet.
The enclosure system itself is controlled by a Siemens S7-1200 PLC (programmable logic controller) and not by the enclosure computer that runs on a Microsoft Windows operating system. This approach ensures that the safety features – such as rain or power failure detection, cover motor control and temperature control – are separated from the Windows operating system, making the enclosure less error-prone and more fail-safe.
All the additional electronics are placed in the rear part of the enclosure systems and are shown in Fig. 4 in detail. Besides the PLC, we installed an LTE router, a heater, the motor driver, two circuit breakers, surge protection devices and an RCCB (residual current circuit breaker). In addition, new relays were added to the system to be able to reset all error-prone devices – such as the computer, router or PLC – remotely. In order to make the system as lightweight as possible, we replaced the large and heavy thermoelectrical cooler by a cooling fan and a heater, and replaced the lead–acid battery of the UPS (uninterruptible power supply) by capacitor-based energy storage. All the devices inside the system communicate via the two standard protocols TCP/IP and USB.
A photo of one of the four newly developed enclosure systems for the Munich network can be seen in Fig. 5. It shows the measurement setup at our southern site on top of a flat rooftop.
3.1.2 Universal editions
Our enclosure system was originally developed to measure the GHG concentrations in Munich at a latitude of 48.15∘ N. Therefore, the rotating cover that protects the solar tracker from bad weather was designed to enable measurements for all possible solar angles at such a latitude. However, if the enclosure system is used somewhere else in the world, these limitations need to be considered. That is why we designed our new cover so that it can measure solar elevation angles up to about 80∘ and azimuth angles between 30 and 300∘ for setups in the Northern Hemisphere. The asymmetric azimuth angle range is due to the non-centered first mirror of the solar tracker. If the system is used in the Southern Hemisphere, it must be rotated by 180∘ and a setting must be changed in the software. These solar angles cover most places in the world. Furthermore, we adapted some features to overcome challenges such as extreme temperatures and high relative humidity. We developed two of these special editions and tested them at both very low and very high latitudes: one in Uganda next to the Equator and one in Finland next to the polar circle.
As part of the NERC MOYA project, the University of Leicester has been using our enclosure system to measure CH4 emissions from the wetlands north of Jinja, Uganda (latitude: 0.4∘ N), since the beginning of 2020 (Humpage et al., 2019). Quite apart from the significantly higher temperatures and relative humidity than in Munich, the very high solar elevation angles (up to 90∘) are challenging. These high angles are a problem both for the cover of the enclosure as it blocks the sun in such cases and for the solar tracker of the spectrometer. The solar tracker of the EM27/SUN can only measure up to elevation angles of about 85∘. At higher elevations, the control algorithm is no longer stable. Therefore, both the spectrometer and the cover cannot work properly at such high elevation angles.
To overcome this challenge, we tilted the whole enclosure system by a few degrees to simulate the instrument being located at a site with a higher latitude than it actually is. This is done using two state-of-the-art car jacks (see Fig. 6), which can elevate the side of the enclosure that points towards the Equator up to 15∘. As a result, the very low elevation angles can no longer be measured, as the sun is then blocked by the lid of the enclosure, although this is not an issue. This is because the air mass dependency of the slant column cannot be reliably handled by the GFIT retrieval algorithm at these high solar zenith angles (Wunch et al., 2011). Using this unique approach, both the solar tracker and the rotating cover work properly at high elevation angles, which makes this approach suited for locations at low latitudes.
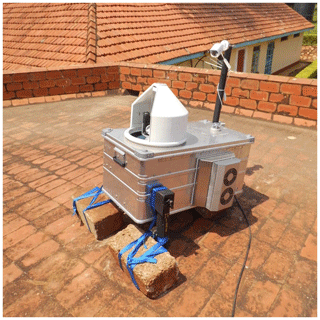
Figure 6Setup of the tropical version of our enclosure in Jinja, Uganda (Latitude: 0.4∘ N). With the help of car jacks the whole system is tilted in order to enable measurements at high elevation levels close to 90∘. Furthermore, the system is equipped with two 150 W thermoelectrical coolers (attached at the two sides of the system) that keep the temperature inside the enclosure constant at 25 ∘C. Photo by Neil Humpage, University of Leicester.
Since the temperature and relative humidity are much higher than in central Europe, the fan and heater normally used are replaced by two 150 W thermoelectrical coolers. They can keep the temperature at a constant level of 25 ∘C under normal weather conditions in Uganda, as well as being able to condense water vapor to reduce the relative humidity inside the system.
Our enclosure is, however, suited to work not only at very low latitudes but also at high ones. To test the system under such conditions, we built another enclosure system for the COCCON site next to the TCCON station in Sodankylä at a latitude of 67.4∘ N (Tu et al., 2020). There, the system has been measuring continuously since 2018, which shows that our system can not only withstand cold winters but is also suitable to measure a large azimuth angle range.
Overall, we developed a system that is universally applicable and can be used for a wide latitude range to enable ground-based GHG measurements worldwide with minimum effort and maximum measurement data.
3.2 Software
For controlling and automating the enclosure system, we developed two independent software programs: ECon and Pyra. The purpose of ECon is to control all safety and enclosure features that are monitored by the PLC, whereas Pyra is used to control the spectrometer and take measurements automatically. Pyra also includes a user interface (UI) through which the operator can set all parameters and observe the current state of the system.
3.2.1 Enclosure control (ECon)
The enclosure control software ECon was already a part of the first enclosure version (Heinle and Chen, 2018). There, a microcontroller program is used to control the enclosure features, such as opening and closing the rotating cover, analyzing the rain sensor data, powering the spectrometer and monitoring the UPS. For the new version, we separated these safety operations from the measurement-related software that is running on a Windows computer to make these features fail-safe. As the microcontroller is replaced by a PLC in the new version, the ECon software needed to be renewed as well.
ECon is structured as a sequence control that loops through the main program, which is grouped into several functions, over and over again. These functions include, for example, the detection of any alarm caused by the UPS, encoder or power failures; the request of the current solar azimuth angle and the control of the cover motor; and other outputs such as relays or signal lamps.
The most safety-relevant function is the control of the cover motor. The program is structured in such a way that closing the cover is prioritized in any condition. Even in the event of a reed sensor failure, the program will make sure that the cover closes correctly by evaluating the sensor signals, which are implemented redundantly.
Furthermore, ECon monitors whether the ethernet connections to the computer, spectrometer and internet are working properly. If any malfunction is detected, the program automatically restarts the spectrometer, computer or router, depending on the kind of failure, by briefly interrupting the power supply to the respective device using relays. This approach ensures a minimum requirement of human interactions if malfunctions occur, which is particularly beneficial for operating very remote sites.
To keep the temperature within a predefined range, ECon also controls the temperature inside the enclosure by powering either the heater or the fan, depending on the actual and the given nominal temperature.
3.2.2 Automation software (Pyra)
In order to control the measurements of the spectrometers automatically, it was necessary to develop software that covers all the tasks that a human operator normally does to perform the measurements. We decided to use Python as the programming language to develop both the automation software and a user interface that allows an operator to set all necessary parameters and observe the current state of the system. The program runs all the time on each enclosure computer and serves as a juncture between the spectrometer, enclosure system and operator. Since the measurements are based on the spectral analysis of the sun, we have named the program Pyra, which is a combination of the programming language Python and the name of the Egyptian sun god Ra.
The manufacturer Bruker provides the EM27/SUN spectrometers with the two independent software components OPUS and CamTracker, to control both the spectrometer itself and the camera-based solar tracker that is attached to the spectrometer. Pyra does not replace these two software elements but provides the possibility to start, stop and control them automatically. Besides these necessary tasks, Pyra also monitors the operating system and the spectrometer to detect malfunctions such as insufficient disk space or non-working connections. Furthermore, it evaluates whether the environmental conditions are suited for measurements and logs each event to a file.
Pyra has four different operating modes: the manual one, in which the operator can start and stop the measurements with just one click; two semi-automated modes, in which Pyra starts and stops the measurements based on either a defined time or the solar zenith angle (SZA) range; and the fully automated mode. In the latter, Pyra evaluates the direct solar radiation sensor data and combines them with the SZA information calculated online to start and stop the measurements whenever the environmental conditions are suitable.
A more detailed description about the features of Pyra can be found in Appendix A.
Although Pyra was developed to automate the process of EM27/SUN spectrometers that are operated in our enclosure system, it can also be used without this system or in a different shelter. In this case, only the fully automated mode no longer works, as the information from the direct solar radiation sensor is not available. However, all the other modes work, which leads to less human effort and more reliable measurements.
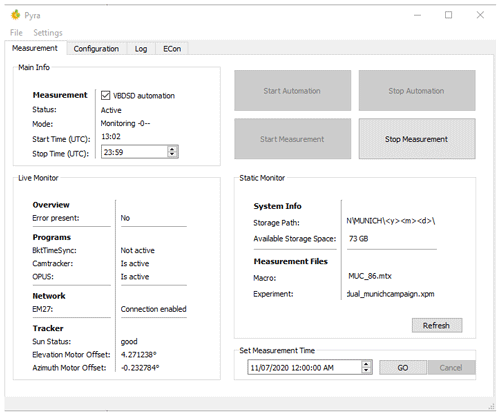
Figure 7User interface for the control software, Pyra. In total there are four different tabs (Measurement, Configuration, Log and Enclosure control) that can be selected. In this image, the measurement tab is shown.
All the aforementioned features of Pyra are combined in a common user interface (see Fig. 7). It is a clear and handy interface that allows any operator to make all the necessary settings for performing automated measurements using EM27/SUN spectrometers. In total, there are three Pyra tabs (measurement, configuration and log) and one ECon tab, which we also included in this user interface. The ECon tab allows us to control the PLC that operates the enclosure system (for details, see Sect. 3.2.1). Thus, the program itself runs not on the enclosure computer but on the PLC, which makes the safety-related features fail-safe. As the PLC does not provide its own graphical user interface, we decided to include these functions – such as controlling the cover motor, heater, fan, relays etc. – in the Pyra UI as well. For that, the Python library snap7 is used, which makes it possible to communicate with a Siemens S7 PLC using an ethernet connection.
3.2.3 Automated retrieval process
For a fully automated greenhouse gas observation network, not only the measurements need to be autonomous; the data processing also needs to be autonomous. Therefore, we automated the data processing chain as well.
At the end of a measurement day, each enclosure computer automatically uploads all the interferograms and weather data via an SSH connection to our Linux cloud server at the Leibniz Supercomputing Center in Garching. After about 5 d, when the a priori vertical pressure profiles from NCEP (National Centers for Environmental Prediction) are available, the retrieval algorithm converts the information from the interferograms into concentrations. The retrieval algorithm used is GGG2014 (Wunch et al., 2015), which is also used to retrieve all the TCCON data. We applied the standard TCCON parameters, including the air-mass-independent correction factors. The spectral windows for retrieving diverse gas species are slightly modified according to the EGI setup (Hedelius et al., 2016).
We tested the automated network consisting of five spectrometers in a measurement campaign in August 2018 (Dietrich et al., 2019), before the permanent network was installed in September 2019. In addition, our first enclosure system has been permanently measuring on the university rooftop since 2016.
4.1 Test campaign – Munich, August 2018
After building a total of five enclosure systems, we established the first fully automated GHG sensor network based on the differential column measurement principle (Chen et al., 2016) in a 1-month measurement campaign in Munich.
To test our enclosure systems and the network configuration, we borrowed spectrometers from KIT and the German Aerospace Center (DLR). In addition to our long-term operating station in the inner city, we set up a system in each compass direction (see red shaded enclosure systems in Fig. 8). A distance of approximately 20 km was selected between the downtown station and each outer station, to ensure that the outer stations are not directly affected by the city emissions if they are located upwind.
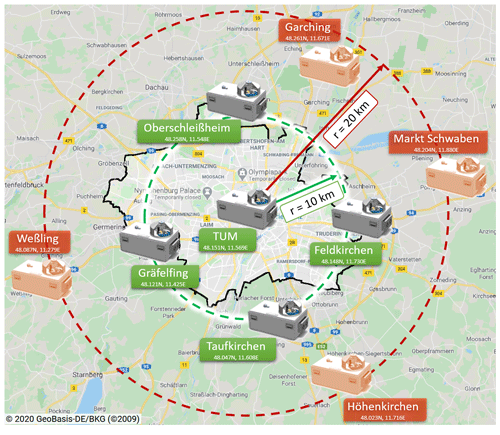
Figure 8Map of the greater Munich area together with the two different sensor network setups that have been implemented. The urban area itself (indicated by the black border) is largely contained within the inner green dashed circle in the center, which represents the current setup of the stationary network. The light red shaded sensor systems, together with the center station, represent the setup during our 2018 summer campaign. Both setups are characterized by a center station and a station in each compass direction to measure the inflow and outflow of GHG concentrations under arbitrary wind conditions. Map data are from © Google Maps.
Thanks to the automation, we were able to take measurements on each of the 25 sunny days in August, both weekdays and weekends, mostly from very early in the morning to late evening (approx. 07:00 to 20:00 CEST). This kept human interactions to a minimum and restricted them mostly to setting up and disassembling the enclosure systems on the rooftops that we used as measurement sites. Therefore, this campaign was characterized by a very small effort as well as a very high data volume. These results are the desired outcomes of such campaigns and are also the foundation for using this kind of setup for a permanent urban GHG observation network.
4.2 Permanent Munich GHG network setup
Although the configuration of the outer stations in the August 2018 campaign was well suited for capturing the background concentrations, this setup cannot be used to determine the emissions of the city center of Munich separately from its outer surroundings. Instead, the greater Munich area emissions are captured as well. As our focus is emissions from the city itself, we decided to go closer to the city boundaries for our permanent sensor network. The distance between the downtown station and each outer station was halved to 10 km (see green enclosure systems in Fig. 8). Thus, the outer stations are located approximately at the city boundaries of Munich. The second benefit of such a dense sensor setup is that it can be better used for validating concentration gradients measured by satellites. Due to the unique dataset of our sensor network, the NASA satellites OCO-2 (Crisp et al., 2017) and OCO-3 (Eldering et al., 2019) have been measuring CO2 concentrations over Munich in the target mode since spring 2020. The area OCO-2 can cover over Munich in this mode is approximately 21 km × 13 km. As the satellite trajectory is not exactly aligned on the north–south axis, the distance of 10 km between the inner and outer stations is optimal for capturing the urban concentration gradients.
In addition to the relocation, the enclosure systems were slightly improved based on the experiences from the August 2018 campaign. In particular, this includes the addition of a direct solar radiation sensor in order to start and stop the measurements depending on the actual weather conditions. Furthermore, we replaced the three borrowed spectrometers with our own ones so that all five instruments can measure long-term.
All in all, we were able to set up MUCCnet, the first permanent urban column concentration network for GHGs, in September 2019 using our own five spectrometers. Since this date, we have been measuring not only the absolute GHG concentration trend of Munich but also the city gradients, which will be used to determine the urban GHG emissions in Munich over the years, as well as to find unknown emission sources.
Since 2015, we have been continuously measuring the GHG concentrations in Munich with at least one instrument. Over time, the amount of data has increased as we have improved our automation and used more and more instruments.
5.1 Seasonal cycle
In Fig. 9, we show the measurement curve of our downtown station over the first 5 years of measurements. In order to display the seasonal cycle, we use a sinusoidal function of the form
with the parameters a to d to be fitted. One can clearly see the globally rising trend in CO2 (about 2.4 ppm yr−1), as well as the seasonal cycle over the 5-year period.
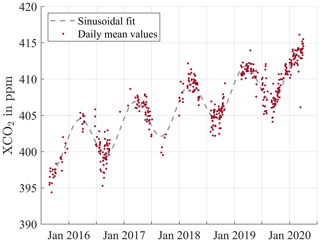
Figure 9Daily mean values of the CO2 measurements from the downtown station in Munich. The concentrations follow the globally rising trend. Furthermore, the seasonal cycle with lower concentrations in summer and higher concentrations in winter is clearly visible for the 5-year period shown.
Although the entire period from fall 2015 to summer 2020 is covered, some times within this range yield a much greater volume of data than others. These high-density data clusters represent our campaigns in summer 2017 and 2018. A further hot spot can be detected in fall 2016, when the first version of our enclosure system (Heinle and Chen, 2018) was established and intensively tested in the semi-automated mode. Since summer 2019, the fully automated enclosure system has been measuring whenever the weather conditions are suitable, which results in a very high and dense data volume.
In total, we have measured on 498 d throughout the last 5 years. Of these, only days with continuous measurements of at least 1 h are taken into account. The ratio of measurement days compared to non-measurement days is about 17 % for the time period before summer 2019. Once full automation was established, this ratio increased to about 52 %, which shows the great benefit of our fully automated sensor network approach. In this calculation all days are taken into account, regardless of whether the measuring conditions were good or bad.
5.2 Side-by-side and urban gradient comparison
The results in the previous section show that our automation works and that we are able to gather a lot of GHG measurement data. The final goal of our network is, however, to quantify the urban emissions. For that, the gradients between the single stations need to be analyzed. As the concentration enhancements of column-averaged dry-air mole fractions are quite small for an urban emission source, it is absolutely necessary to calibrate the instruments regularly. In addition to the calibration of absolute concentration values during measurements next to the TCCON station in Karlsruhe, the relative comparison between the single instrument is even more decisive. Therefore, we calibrate all instruments regularly with respect to our defined standard represented by the instrument ma61. Figure 10 shows the setup of this kind of side-by-side measurement day, where five automated sensor systems measure next to each other on our university roof.
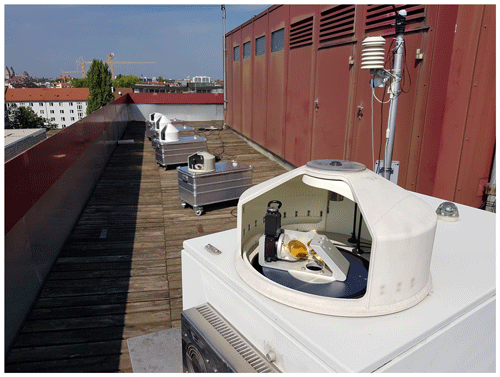
Figure 10Calibration measurements of all our five sensor systems on the roof of our institute's building. One can see four slightly different versions of our enclosure systems.
For each instrument and gas species, a constant correction factor f (see Table B1) is determined to convert the raw concentration value craw to the corrected concentration value ccorr using linear scaling:
As an absolute reference value, we will use the instrument that was calibrated at a TCCON station most recently. So far, the most recent correction value determined by Frey et al. (2019) before shipping the instruments from Bruker to Munich has been used (see Table B2).
Figure 11 shows the CH4 gradients of a standard measurement day on a Saturday during Oktoberfest 2019. It indicates that our sensor network can detect the differences in CH4 concentrations well, which allows us to determine the urban emissions using these measurements as an input. Furthermore, one can see that our automated network allows us to measure not only on weekdays but also on weekends from early morning to evening, without the need for human resources.
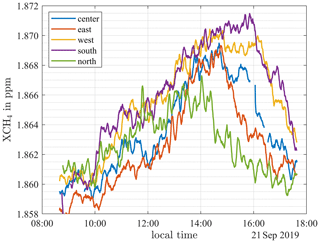
Figure 11CH4 measurement values (5 min average) from all five stations during our Oktoberfest 2019 campaign on 21 September 2019. The concentration gradients between the single stations are clearly visible, which indicates the presence of strong CH4 sources in the city.
In Fig. 12, we show the CO2 concentration enhancements above the background concentration for the four outer-city stations depending on the wind direction. For this purpose, we use an ultrasonic wind sensor (Gill WindObserver II) on a roof in the inner city of Munich (48.148∘ N, 11.573∘ E, 24 m a.g.l.). To determine the background concentration, we use the data from all of our measurement stations and determine the lowest measurement point for each time step. Afterwards, a moving average with a window size of 4 h is used to smooth the curve as we assume that the background concentration must not change rapidly. For each station, a polar histogram shows where the concentration enhancements originate and how frequent they are. In contrast to a standard wind rose, the different colors indicate the strength of the concentration enhancement; yellow means low and red high enhancement. The wind speed is displayed by the distance of the respective cell to the center point of each circle.
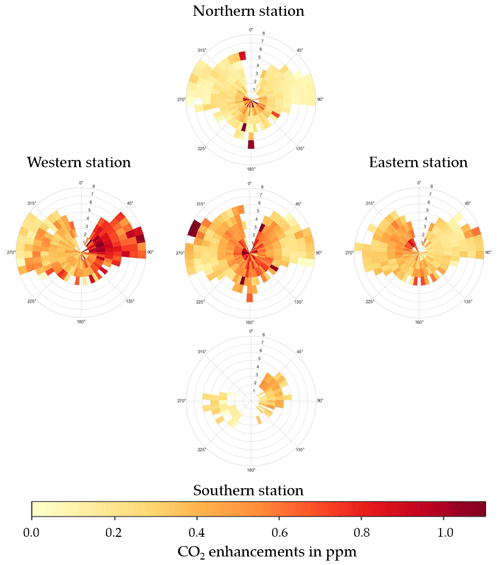
Figure 12Concentration enhancements over the background for each of the five stations displayed as a polar histogram. The CO2 enhancements are represented by the different colors (low (yellow) to red (high)). The wind direction is indicated by the location of the respective cells in the circle, and the wind speed by the distance of the cells to the center point.
One can see clearly that for all four stations the enhancements are higher towards the city. For the eastern station, for example, the highest enhancements, indicated by the reddish color, are located in the west. These results indicate that the captured GHGs are mainly generated in the city and that our network is able to detect and quantify such urban emitters. Due to technical issues, not all stations started their measurements at the same time. Therefore, the data volume collected at the southern station, which started no earlier than May 2020, is much smaller. An overview of when each station started its measurements in the permanent network, including the data collected so far, is shown in Table 1.
5.3 Influences of the COVID-19 lockdown on urban concentration gradients
Thanks to the automation, we took measurements throughout the COVID-19 lockdown in spring 2020, which resulted in a unique dataset showing the influence of such a drastic event on the urban GHG gradients of a city like Munich. Figure 13 displays the gradients between the measurements of the inner-city station and the background concentrations (cf. Sect. 5.2).
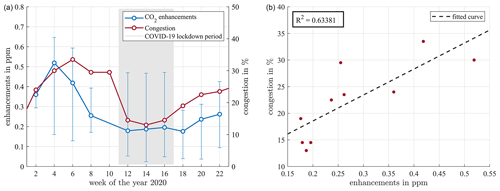
Figure 13Correlations between the CO2 enhancements over the background measured at our inner-city station in Munich and the traffic amount represented by the congestion rate (a: time series; b: regression plot). The time period includes the COVID-19 lockdown in spring 2020. We show the median trend of all column concentration gradients clustered into biweekly bins. The error bars show the 1σ standard deviation of all enhancements within the respective 2-week period. Traffic data are from © 2020 TomTom International BV.
All concentration gradients are clustered into biweekly bins. In Fig. 13, the median of these bins is displayed as the blue curve. The error bars indicate the 1σ standard deviation of these biweekly distributions. In addition, volume of traffic in Munich is displayed in red using the congestion rate provided by TomTom International BV. Furthermore, the COVID-19 lockdown period is shown as the grey shaded area.
The plot demonstrates that the lockdown had a significant impact on traffic flow. The CO2 enhancements show a similar pattern throughout the first half of the year 2020. Based on the regression plot, there seems to be a correlation between the reduced traffic volume and the lower CO2 enhancements (R2=0.63). Both curves first decrease and then increase again after the strict restrictions were gradually loosened.
However, our statistical approach, which uses about 100 000 measurement points, shows large variations in the CO2 enhancements for the single bins. Such high variations are, however, not concerning as the approach does not take into account wind speed and direction, for example. Furthermore, the assumption of homogeneously distributed emissions sources does not reflect the truth, and photosynthetic effects are not considered. Therefore, it can only serve as a first indication of how the emissions were reduced during the lockdown period. In the future, we will apply more sophisticated modeling approaches to quantify the emissions.
We present the world's first permanent urban GHG column network consisting of five compact solar-tracking spectrometer systems distributed in and around Munich (MUCCnet). We developed the hardware and software to establish this kind of a fully automated GHG sensor network for quantifying large-area emission sources, such as cities. Both the enclosure system and the related Python program for automating the measurement process can be used by the community to build up similar sensor networks in cities worldwide. Also, COCCON would benefit greatly from this kind of automated system, as the current approach of operating EM27/SUN spectrometers in this network still requires man power on site to start up measurements and to protect the spectrometer from adverse meteorological conditions. Permanent and long-term observations will help to improve the understanding of the global carbon cycle.
With our sensor systems, we carried out several test campaigns between 2016 and 2019 and finally set up the permanent urban GHG sensor network based on the differential column methodology in fall 2019. The results show the advantages of this kind of automated network, such as very high data volume, low personnel effort and high data quality. Due to the very frequent measurements that were taken independent of the day of the week or the season, this study shows that our network can effectively detect both the globally rising trend of CO2 concentrations and the seasonal cycle.
The final goal of this kind of network is the quantification of urban GHG emissions. For that, the concentration gradients between the downwind and upwind stations are decisive, as they represent the anthropogenic emissions superimposed with biological processes. Our results indicate that these gradients can be captured clearly with our sensor setup. Additional analyses, including wind information, show that the city causes these enhancements.
Furthermore, the network can be used to validate GHG satellites in a unique way, as not only absolute values but also concentration gradients can be compared. Since spring 2020, the NASA OCO-2 and OCO-3 satellites have been measuring urban CO2 concentration gradients in Munich using the spatially highly resolved target mode in a recurring pattern to compare the satellite measurements with our ground-based ones.
With the benefit of full automation, we were also able to measure concentration gradients during the COVID-19 lockdown period in spring 2020. The results show a possible correlation between the CO2 column concentration gradients and the traffic amount, both of which appear to be drastically affected by the lockdown.
In order to quantify the Munich GHG emissions, we are currently developing an atmospheric transport model based on Bayesian inversion. This kind of modeling framework will help us quantify Munich's GHG emissions in the future and find correlations with parameters such as time of the day, season and weather conditions. Furthermore, we will use our rich dataset to detect and quantify unknown GHG emission sources.
In summary, this study provides the framework for establishing a permanent GHG sensor network to determine urban concentration gradients using column measurements over a wide range of latitudes. The characteristics of the hardware presented here – such as high reliability, ease of use and low operating costs – form the basis for it to become a new standard for monitoring urban GHG concentrations.
To control the spectrometer program OPUS, we use the Microsoft Windows technology dynamic data exchange (DDE), which is also supported by OPUS. It is a protocol for exchanging data based on the client–server model and allows us to send requests, such as starting a measurement or loading a specific setting file, to OPUS. With the help of DDE, combined with an MTX macro file for OPUS, Pyra can start recurring measurements of the spectrometer. The necessary settings are stored in an XPM experiment file and are loaded into the program in the same way.
Communication with the solar tracker program CamTracker is simpler, as this program's settings no longer need to be changed after the initialization. Therefore, we asked the manufacturer Bruker to implement an autostart option for the tracker. Whenever CamTracker is called with this option, the solar tracker automatically aligns its two mirrors with the calculated live position of the sun and enables the tracking of the sun. Once the program has been terminated, the tracker automatically moves back to its parking position.
In order to detect malfunctions, Pyra is equipped with several live monitoring functions. It monitors whether the two programs OPUS and CamTracker are still running correctly every 0.2 s. If they are not, it automatically restarts the non-working program to proceed with the measurements. Furthermore, the log files of CamTracker are read continuously, which allows us to detect automatically if the solar tracker is not tracking the sun correctly anymore, for example. Such a behavior is quite common as the solar tracker uses a camera-based approach to follow the sun over the course of the day. In cloudy conditions, the algorithm sometimes mistakenly detects objects other than the sun, resulting in incorrect tracking. In such a case, the tracking is restarted using the calculated position of the sun at the given coordinates and time. In addition to trying to solve the error automatically, Pyra also sends an error notification email to an operator, whose email address can be defined in the settings.
Table B1Scaling factors of the side-by-side measurements with reference to our standard instrument ma61 for CO2 and CH4.
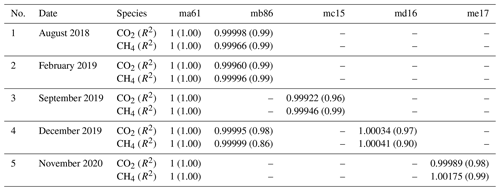
Table B2Scaling factors according to Frey et al. (2019) of our five EM27/SUN instruments with respect to the reference EM27/SUN (S/N 037) at KIT.
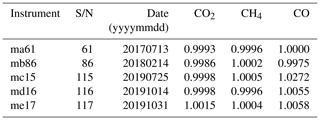
The Python software Pyra and the measurement data can be provided by the authors upon request.
The measurement data are also available at http://atmosphere.ei.tum.de/ (Dietrich et al., 2021).
FD and JC conceived the study and developed the concept; FD led the hardware and software development as well as the setup of the sensor network. FD, BV and BR built the enclosure systems. PA, BV and FD developed the software Pyra. FD and JC performed the measurements. FD, JC and NN analyzed the measurement data. FD and JC wrote the manuscript.
The authors declare that they have no conflict of interest.
We thank Ludwig Heinle for developing the first version of a semi-automated enclosure system; Frank Hase for testing and calibrating the instruments prior to the delivery and for providing us with two spectrometers each for our August 2018 and Oktoberfest 2019 campaign; André Butz for providing us with his EM27/SUN in our August 2018 campaign; Jacob Hedelius for his support in all matters concerning the GFIT retrieval algorithm; Stephan Hachinger for helping us regarding the automated retrieval process on the Linux cloud; Jonathan Franklin, Taylor Jones, Andreas Luther and Ralph Kleinscheck for their support during our measurement campaigns; Neil Humpage and Harmut Boesch for testing our enclosure system in Uganda; Martin Wild, Norbert Tuschl, Abdurahim Bingöl, Sebastian Zunterer and Bernhard Obermaier for manufacturing the enclosure systems; Markus Garhammer and Mark Wenig for providing us with meteorological data; First Mayor Andreas Janson (Feldkirchen) and the municipalities of Gräfelfing, Markt Schwaben, Oberschleißheim and Taufkirchen as well as Lothar Lauterbach from the ARCONE Technology Center Höhenkirchen, who allowed us to use their rooftops as measurement sites; and our students Andreas Forstmaier, Adrian Wenzel, Nikolas Hars, Jared Matzke, Yiming Zhao, Xu Hang, Dingcong Lu, Xiao Bi and Michal Wedrat for their help during the campaigns and the network setup as well as programming helpful automation scripts and supporting the CAD model. TUM is grateful to Stefan Schwietzke and Daniel Zavala-Araiza for helpful conversation in their role as part of the Office of the Chief Scientist of the Climate and Clean Air Coalition Methane Science Studies (MSS), which are funded by the Environmental Defense Fund, the European Commission, the companies of the Oil and Gas Climate Initiative, and the United Nations Environment Programme. TUM is additionally grateful for invitations to participate in workshops hosted by UNEP in the context of the Methane Science Studies.
This research has been supported by the Deutsche Forschungsgemeinschaft (DFG, German Research Foundation) (grant nos. CH 1792/2-1, INST 95/1544). Jia Chen is supported by the Technical University of Munich – Institute for Advanced Study, funded by the German Excellence Initiative and the European Union Seventh Framework Programme under grant agreement number 291763.
This work was supported by the German Research Foundation (DFG) and the Technical University of Munich (TUM) in the framework of the Open Access Publishing Program.
This paper was edited by Markus Rapp and reviewed by David Griffith and one anonymous referee.
Bréon, F. M., Broquet, G., Puygrenier, V., Chevallier, F., Xueref-Remy, I., Ramonet, M., Dieudonné, E., Lopez, M., Schmidt, M., Perrussel, O., and Ciais, P.: An attempt at estimating Paris area CO2 emissions from atmospheric concentration measurements, Atmos. Chem. Phys., 15, 1707–1724, https://doi.org/10.5194/acp-15-1707-2015, 2015. a
Butz, A., Dinger, A. S., Bobrowski, N., Kostinek, J., Fieber, L., Fischerkeller, C., Giuffrida, G. B., Hase, F., Klappenbach, F., Kuhn, J., Lübcke, P., Tirpitz, L., and Tu, Q.: Remote sensing of volcanic CO2, HF, HCl, SO2, and BrO in the downwind plume of Mt. Etna, Atmos. Meas. Tech., 10, 1–14, https://doi.org/10.5194/amt-10-1-2017, 2017. a
Chen, J., Viatte, C., Hedelius, J. K., Jones, T., Franklin, J. E., Parker, H., Gottlieb, E. W., Wennberg, P. O., Dubey, M. K., and Wofsy, S. C.: Differential column measurements using compact solar-tracking spectrometers, Atmos. Chem. Phys., 16, 8479–8498, https://doi.org/10.5194/acp-16-8479-2016, 2016. a, b, c, d, e
Chen, J., Dietrich, F., Maazallahi, H., Forstmaier, A., Winkler, D., Hofmann, M. E. G., Denier van der Gon, H., and Röckmann, T.: Methane emissions from the Munich Oktoberfest, Atmos. Chem. Phys., 20, 3683–3696, https://doi.org/10.5194/acp-20-3683-2020, 2020. a
Crisp, D., Pollock, H. R., Rosenberg, R., Chapsky, L., Lee, R. A. M., Oyafuso, F. A., Frankenberg, C., O'Dell, C. W., Bruegge, C. J., Doran, G. B., Eldering, A., Fisher, B. M., Fu, D., Gunson, M. R., Mandrake, L., Osterman, G. B., Schwandner, F. M., Sun, K., Taylor, T. E., Wennberg, P. O., and Wunch, D.: The on-orbit performance of the Orbiting Carbon Observatory-2 (OCO-2) instrument and its radiometrically calibrated products, Atmos. Meas. Tech., 10, 59–81, https://doi.org/10.5194/amt-10-59-2017, 2017. a
Dietrich, F., Chen, J., Reger, B., Matzke, J., Forstmaier, A., Bi, X., Luther A., Frey, M., Hase, F., and Butz, A.: First fully-automated differential column network for measuring GHG emissions tested in Munich, EGU General Assembly 2019, Vienna, Austria, 7–12 April 2019, EGU2019–13327, https://doi.org/10.13140/RG.2.2.26867.17441, 2019. a
Dietrich, F., Rissmann, M., Makowski, M., and Chen, J.: Column Greenhouse Gas Concentrations, available at: http://atmosphere.ei.tum.de, last access: 8 February 2021. a
Eldering, A., Taylor, T. E., O'Dell, C. W., and Pavlick, R.: The OCO-3 mission: measurement objectives and expected performance based on 1 year of simulated data, Atmos. Meas. Tech., 12, 2341–2370, https://doi.org/10.5194/amt-12-2341-2019, 2019. a
Feigenwinter, C., Vogt, R., and Christen, A.: Eddy Covariance Measurements Over Urban Areas, in: Eddy Covariance: A Practical Guide to Measurement and Data Analysis, edited by Aubinet, M., Vesala, T., and Papale, D., Springer Atmospheric Sciences, Springer Netherlands, Dordrecht, https://doi.org/10.1007/978-94-007-2351-1_16, 377–397, 2012. a
Frey, M., Sha, M. K., Hase, F., Kiel, M., Blumenstock, T., Harig, R., Surawicz, G., Deutscher, N. M., Shiomi, K., Franklin, J. E., Bösch, H., Chen, J., Grutter, M., Ohyama, H., Sun, Y., Butz, A., Mengistu Tsidu, G., Ene, D., Wunch, D., Cao, Z., Garcia, O., Ramonet, M., Vogel, F., and Orphal, J.: Building the COllaborative Carbon Column Observing Network (COCCON): long-term stability and ensemble performance of the EM27/SUN Fourier transform spectrometer, Atmos. Meas. Tech., 12, 1513–1530, https://doi.org/10.5194/amt-12-1513-2019, 2019. a, b, c
Gisi, M., Hase, F., Dohe, S., and Blumenstock, T.: Camtracker: a new camera controlled high precision solar tracker system for FTIR-spectrometers, Atmos. Meas. Tech., 4, 47–54, https://doi.org/10.5194/amt-4-47-2011, 2011. a
Gisi, M., Hase, F., Dohe, S., Blumenstock, T., Simon, A., and Keens, A.: XCO2$-measurements with a tabletop FTS using solar absorption spectroscopy, Atmos. Meas. Tech., 5, 2969–2980, https://doi.org/10.5194/amt-5-2969-2012, 2012. a
Gurney, K. R., Romero-Lankao, P., Seto, K. C., Hutyra, L. R., Duren, R., Kennedy, C., Grimm, N. B., Ehleringer, J. R., Marcotullio, P., Hughes, S., Pincetl, S., Chester, M. V., Runfola, D. M., Feddema, J. J., and Sperling, J.: Climate change: Track urban emissions on a human scale, Nature, 525, 179–181, https://doi.org/10.1038/525179a, 2015. a
Hase, F., Frey, M., Blumenstock, T., Groß, J., Kiel, M., Kohlhepp, R., Mengistu Tsidu, G., Schäfer, K., Sha, M. K., and Orphal, J.: Application of portable FTIR spectrometers for detecting greenhouse gas emissions of the major city Berlin, Atmos. Meas. Tech., 8, 3059–3068, https://doi.org/10.5194/amt-8-3059-2015, 2015. a
Hase, F., Frey, M., Kiel, M., Blumenstock, T., Harig, R., Keens, A., and Orphal, J.: Addition of a channel for XCO observations to a portable FTIR spectrometer for greenhouse gas measurements, Atmos. Meas. Tech., 9, 2303–2313, https://doi.org/10.5194/amt-9-2303-2016, 2016. a
Hedelius, J. K., Viatte, C., Wunch, D., Roehl, C. M., Toon, G. C., Chen, J., Jones, T., Wofsy, S. C., Franklin, J. E., Parker, H., Dubey, M. K., and Wennberg, P. O.: Assessment of errors and biases in retrievals of , , XCO, and from a 0.5 cm−1 resolution solar-viewing spectrometer, Atmos. Meas. Tech., 9, 3527–3546, https://doi.org/10.5194/amt-9-3527-2016, 2016. a
Heinle, L. and Chen, J.: Automated enclosure and protection system for compact solar-tracking spectrometers, Atmos. Meas. Tech., 11, 2173–2185, https://doi.org/10.5194/amt-11-2173-2018, 2018. a, b, c, d
Helfter, C., Famulari, D., Phillips, G. J., Barlow, J. F., Wood, C. R., Grimmond, C. S. B., and Nemitz, E.: Controls of carbon dioxide concentrations and fluxes above central London, Atmos. Chem. Phys., 11, 1913–1928, https://doi.org/10.5194/acp-11-1913-2011, 2011. a
Humpage, N., Boesch, H., Dietrich, F., and Chen, J.: Testing an automated enclosure system for a ground-based greenhouse gas remote sensing spectrometer; application to the validation of Sentinel-5 Precursor carbon monoxide and methane, in: Copernicus Sentinel-5 Precursor Validation Team Workshop, 11–14 November 2019, Frascati (Rome), Italy, https://doi.org/10.13140/RG.2.2.18535.80808, 2019. a
Jones, T. S., Franklin, J. E., Chen, J., Dietrich, F., Hajny, K. D., Paetzold, J. C., Wenzel, A., Gately, C., Gottlieb, E., Parker, H., Dubey, M., Hase, F., Shepson, P. B., Mielke, L. H., and Wofsy, S. C.: Assessing Urban Methane Emissions using Column Observing Portable FTIR Spectrometers and a Novel Bayesian Inversion Framework, Atmos. Chem. Phys. Discuss. [preprint], https://doi.org/10.5194/acp-2020-1262, in review, 2021. a
Kim, J., Shusterman, A. A., Lieschke, K. J., Newman, C., and Cohen, R. C.: The BErkeley Atmospheric CO2 Observation Network: field calibration and evaluation of low-cost air quality sensors, Atmos. Meas. Tech., 11, 1937–1946, https://doi.org/10.5194/amt-11-1937-2018, 2018. a
Klappenbach, F., Bertleff, M., Kostinek, J., Hase, F., Blumenstock, T., Agusti-Panareda, A., Razinger, M., and Butz, A.: Accurate mobile remote sensing of XCO2 and XCH4 latitudinal transects from aboard a research vessel, Atmos. Meas. Tech., 8, 5023–5038, https://doi.org/10.5194/amt-8-5023-2015, 2015. a
Lamb, B. K., Cambaliza, M. O. L., Davis, K. J., Edburg, S. L., Ferrara, T. W., Floerchinger, C., Heimburger, A. M. F., Herndon, S., Lauvaux, T., Lavoie, T., Lyon, D. R., Miles, N., Prasad, K. R., Richardson, S., Roscioli, J. R., Salmon, O. E., Shepson, P. B., Stirm, B. H., and Whetstone, J.: Direct and Indirect Measurements and Modeling of Methane Emissions in Indianapolis, Indiana, Environ. Sci. Technol., 50, 8910–8917, https://doi.org/10.1021/acs.est.6b01198, 2016. a
Luther, A., Kleinschek, R., Scheidweiler, L., Defratyka, S., Stanisavljevic, M., Forstmaier, A., Dandocsi, A., Wolff, S., Dubravica, D., Wildmann, N., Kostinek, J., Jöckel, P., Nickl, A.-L., Klausner, T., Hase, F., Frey, M., Chen, J., Dietrich, F., Nȩcki, J., Swolkień, J., Fix, A., Roiger, A., and Butz, A.: Quantifying CH4 emissions from hard coal mines using mobile sun-viewing Fourier transform spectrometry, Atmos. Meas. Tech., 12, 5217–5230, https://doi.org/10.5194/amt-12-5217-2019, 2019. a
Makarova, M. V., Alberti, C., Ionov, D. V., Hase, F., Foka, S. C., Blumenstock, T., Warneke, T., Virolainen, Y., Kostsov, V., Frey, M., Poberovskii, A. V., Timofeyev, Y. M., Paramonova, N., Volkova, K. A., Zaitsev, N. A., Biryukov, E. Y., Osipov, S. I., Makarov, B. K., Polyakov, A. V., Ivakhov, V. M., Imhasin, H. Kh., and Mikhailov, E. F.: Emission Monitoring Mobile Experiment (EMME): an overview and first results of the St. Petersburg megacity campaign-2019, Atmos. Meas. Tech. Discuss. [preprint], https://doi.org/10.5194/amt-2020-87, in review, 2020. a
McKain, K., Down, A., Raciti, S. M., Budney, J., Hutyra, L. R., Floerchinger, C., Herndon, S. C., Nehrkorn, T., Zahniser, M. S., Jackson, R. B., Phillips, N., and Wofsy, S. C.: Methane emissions from natural gas infrastructure and use in the urban region of Boston, Massachusetts, P. Natl. Acad. Sci. USA, 112, 1941–1946, https://doi.org/10.1073/pnas.1416261112, 2015. a, b
Plant, G., Kort, E. A., Floerchinger, C., Gvakharia, A., Vimont, I., and Sweeney, C.: Large Fugitive Methane Emissions From Urban Centers Along the U.S. East Coast, Geophys. Res. Lett., 46, 8500–8507, https://doi.org/10.1029/2019GL082635, 2019. a
Sha, M. K., De Mazière, M., Notholt, J., Blumenstock, T., Chen, H., Dehn, A., Griffith, D. W. T., Hase, F., Heikkinen, P., Hermans, C., Hoffmann, A., Huebner, M., Jones, N., Kivi, R., Langerock, B., Petri, C., Scolas, F., Tu, Q., and Weidmann, D.: Intercomparison of low- and high-resolution infrared spectrometers for ground-based solar remote sensing measurements of total column concentrations of CO2, CH4, and CO, Atmos. Meas. Tech., 13, 4791–4839, https://doi.org/10.5194/amt-13-4791-2020, 2020. a
Shusterman, A. A., Teige, V. E., Turner, A. J., Newman, C., Kim, J., and Cohen, R. C.: The BErkeley Atmospheric CO2 Observation Network: initial evaluation, Atmos. Chem. Phys., 16, 13449–13463, https://doi.org/10.5194/acp-16-13449-2016, 2016. a
Toja-Silva, F., Chen, J., Hachinger, S., and Hase, F.: CFD simulation of CO2 dispersion from urban thermal power plant: Analysis of turbulent Schmidt number and comparison with Gaussian plume model and measurements, J. Wind Eng. Ind. Aerod., 169, 177–193, https://doi.org/10.1016/j.jweia.2017.07.015, 2017. a
Tu, Q., Hase, F., Blumenstock, T., Kivi, R., Heikkinen, P., Sha, M. K., Raffalski, U., Landgraf, J., Lorente, A., Borsdorff, T., Chen, H., Dietrich, F., and Chen, J.: Intercomparison of atmospheric CO2 and CH4 abundances on regional scales in boreal areas using Copernicus Atmosphere Monitoring Service (CAMS) analysis, COllaborative Carbon Column Observing Network (COCCON) spectrometers, and Sentinel-5 Precursor satellite observations, Atmos. Meas. Tech., 13, 4751–4771, https://doi.org/10.5194/amt-13-4751-2020, 2020. a
Viatte, C., Lauvaux, T., Hedelius, J. K., Parker, H., Chen, J., Jones, T., Franklin, J. E., Deng, A. J., Gaudet, B., Verhulst, K., Duren, R., Wunch, D., Roehl, C., Dubey, M. K., Wofsy, S., and Wennberg, P. O.: Methane emissions from dairies in the Los Angeles Basin, Atmos. Chem. Phys., 17, 7509–7528, https://doi.org/10.5194/acp-17-7509-2017, 2017. a
Vogel, F. R., Frey, M., Staufer, J., Hase, F., Broquet, G., Xueref-Remy, I., Chevallier, F., Ciais, P., Sha, M. K., Chelin, P., Jeseck, P., Janssen, C., Té, Y., Groß, J., Blumenstock, T., Tu, Q., and Orphal, J.: XCO2 in an emission hot-spot region: the COCCON Paris campaign 2015, Atmos. Chem. Phys., 19, 3271–3285, https://doi.org/10.5194/acp-19-3271-2019, 2019. a
Wunch, D., Toon, G. C., Blavier, J. F., Washenfelder, R. A., Notholt, J., Connor, B. J., Griffith, D. W., Sherlock, V., and Wennberg, P. O.: The total carbon column observing network, Philos. T. R. Soc. A, 369, 2087–112, 2011. a, b
Wunch, D., Toon, G. C., Sherlock, V., Deutscher, N. M., Liu, X., Feist, D. G., and Wennberg, P. O.: Documentation for the 2014 TCCON Data Release, CaltechDATA, https://doi.org/10.14291/tccon.ggg2014.documentation.R0/1221662, 2015. a
Xueref-Remy, I., Dieudonné, E., Vuillemin, C., Lopez, M., Lac, C., Schmidt, M., Delmotte, M., Chevallier, F., Ravetta, F., Perrussel, O., Ciais, P., Bréon, F.-M., Broquet, G., Ramonet, M., Spain, T. G., and Ampe, C.: Diurnal, synoptic and seasonal variability of atmospheric CO2 in the Paris megacity area, Atmos. Chem. Phys., 18, 3335–3362, https://doi.org/10.5194/acp-18-3335-2018, 2018. a
Zhao, X., Marshall, J., Hachinger, S., Gerbig, C., Frey, M., Hase, F., and Chen, J.: Analysis of total column CO2 and CH4 measurements in Berlin with WRF-GHG, Atmos. Chem. Phys., 19, 11279–11302, https://doi.org/10.5194/acp-19-11279-2019, 2019. a
- Abstract
- Introduction
- Measurement principle
- Measurement system
- Network setup
- Results
- Conclusion
- Appendix A: Pyra – software features
- Appendix B: EM27/SUN calibration factors
- Code and data availability
- Author contributions
- Competing interests
- Acknowledgements
- Financial support
- Review statement
- References
- Abstract
- Introduction
- Measurement principle
- Measurement system
- Network setup
- Results
- Conclusion
- Appendix A: Pyra – software features
- Appendix B: EM27/SUN calibration factors
- Code and data availability
- Author contributions
- Competing interests
- Acknowledgements
- Financial support
- Review statement
- References