the Creative Commons Attribution 4.0 License.
the Creative Commons Attribution 4.0 License.
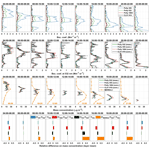
Mass concentration estimates of long-range-transported Canadian biomass burning aerosols from a multi-wavelength Raman polarization lidar and a ceilometer in Finland
Tero Mielonen
Antti Lipponen
Elina Giannakaki
Ari Leskinen
Virginie Buchard
Anton S. Darmenov
Antti Kukkurainen
Antti Arola
Ewan O'Connor
Anne Hirsikko
Mika Komppula
A quantitative comparison study for Raman lidar and ceilometer observations, and for model simulations of mass concentration estimates of smoke particles is presented. Layers of biomass burning aerosol particles were observed in the lower troposphere, at 2 to 5 km height on 4 to 6 June 2019, over Kuopio, Finland. These long-range-transported smoke particles originated from a Canadian wildfire event. The most pronounced smoke plume detected on 5 June was intensively investigated. Optical properties were retrieved from the multi-wavelength Raman polarization lidar PollyXT. Particle linear depolarization ratios (PDRs) of this plume were measured to be 0.08±0.02 at 355 nm and 0.05±0.01 at 532 nm, suggesting the presence of partly coated soot particles or particles that have mixed with a small amount of dust or other non-spherical aerosol type. The layer-mean PDR at 355 nm (532 nm) decreased during the day from ∼0.11 (0.06) in the morning to ∼0.05 (0.04) in the evening; this decrease with time could be linked to the particle aging and related changes in the smoke particle shape properties. Lidar ratios were derived as 47±5 sr at 355 nm and 71±5 sr at 532 nm. A complete ceilometer data processing for a Vaisala CL51 ceilometer is presented from a sensor-provided attenuated backscatter coefficient to particle mass concentration (including the water vapor correction for high latitude for the first time). Aerosol backscatter coefficients (BSCs) were measured at four wavelengths (355, 532, 1064 nm from PollyXT and 910 nm from CL51). Two methods, based on a combined lidar and sun-photometer approach, are applied for mass concentration estimations from both PollyXT and the ceilometer CL51 observations. In the first method, no. 1, we used converted BSCs at 532 nm (from measured BSCs) by corresponding measured backscatter-related Ångström exponents, whereas in the second method, no. 2, we used measured BSCs at each wavelength independently. A difference of ∼12 % or ∼36 % was found between PollyXT and CL51 estimated mass concentrations using method no. 1 or no. 2, showing the potential of mass concentration estimates from a ceilometer. Ceilometer estimations have an uncertainty of ∼50 % in the mass retrieval, but the potential of the data lies in the great spatial coverage of these instruments. The mass retrievals were compared with the Modern-Era Retrospective analysis for Research and Applications, version 2 (MERRA-2) meteorological and aerosol reanalysis. The inclusion of dust (as indicated by MERRA-2 data) in the retrieved mass concentration is negligible considering the uncertainties, which also shows that ceilometer observations for mass retrievals can be used even without exact knowledge of the composition of the smoke-dominated aerosol plume in the troposphere.
- Article
(6378 KB) - Full-text XML
- BibTeX
- EndNote
Wildfires release large amounts of aerosols into the atmosphere, contributing significantly to direct radiative forcing (IPCC 2013, https://www.ipcc.ch/site/assets/uploads/2018/02/WG1AR5_Chapter08_FINAL.pdf, last access: 31 March 2021) and affecting cloud optical properties by acting as cloud condensation nuclei (Yu, 2000) or ice nuclei (Prenni et al., 2012). Biomass burning is the dominant global source for carbonaceous aerosols, including organic and black carbon (Andreae, 2019), which can be transported over thousands of kilometers in the atmosphere (Andreae, 1991; Fromm and Servranckx, 2003; Mielonen et al., 2012; Portin et al., 2012). These smoke plumes can mix with other aerosols (such as dust) originating from regional and local sources (Osborne et al., 2019; Tesche et al., 2009). Long-range transport of particles originating from biomass burning has been recognized as a significant source of tropospheric aerosols at northern latitudes (Generoso et al., 2003), with the most common being smoke from Russia or North America (Müller et al., 2005; Wotawa et al., 2001). The optical properties of smoke particles vary depending on the vegetation fuel types in the source regions and the combustion phase; they also change frequently when dispersing in the air (Reid et al., 2005a, b).
Lidars provide quantitative range-resolved information on atmospheric aerosols. Multi-wavelength Raman lidar, together with its depolarization capability, provides comprehensive information on aerosol optical and microphysical properties (Müller et al., 1999, 2005) and allows the identification of the aerosol type using the intensive optical parameters (Groß et al., 2013; Illingworth et al., 2015). Ground-based lidar networks, such as EARLINET (European Aerosol Research Lidar Network, https://www.earlinet.org, last access: 3 May 2021, Pappalardo et al., 2014), PollyNET (Raman and polarization lidar network, http://picasso.tropos.de, last access: 3 May 2021, Baars et al., 2016), and MPLNET (Micropulse Lidar Network, https://mplnet.gsfc.nasa.gov, last access: 20 July 2021, Welton et al., 2001), have continued to provide observations of clouds and aerosols over large spatial scales. Adam et al. (2020) present a methodology for analyzing the biomass burning events recorded in the EARLINET database and provide a literature review of lidar-derived intensive parameters of biomass burning aerosols (46 reference values from 39 cited papers), including fresh and aged ones. Lidar observations showed that biomass burning aerosols are medium- to highly absorbing particles with an almost spherical shape and small particle size, producing medium to high lidar ratios, low depolarization ratios, and high Ångström exponents (Alados-Arboledas et al., 2011; Amiridis et al., 2009; Baars et al., 2012; Müller et al., 2007; Murayama et al., 2004; Nepomuceno Pereira et al., 2014).
Spaceborne lidars such as CALIOP (Cloud-Aerosol Lidar with Orthogonal Polarization) onboard the CALIPSO (Cloud-Aerosol Lidar and Infrared Pathfinder Satellite Observations) (Winker et al., 2009), and the ADM-Aeolus lidar of the European Space Agency (ESA) (Stoffelen et al., 2005) are complementary to these network observations by providing 3-D aerosol distributions around the globe, which also contribute significantly to the monitoring and documentation of the transport of the smoke (Baars et al., 2021; Kim et al., 2009; Ohneiser et al., 2020).
Numerous studies have investigated the properties of smoke plumes transported from Canadian wildfires to Europe (Ansmann et al., 2018; Fiebig et al., 2003; Hu et al., 2019; Müller et al., 2005). For example, in 2017 a record-breaking Canadian wildfire smoke event was observed over European lidar stations. The arrival of biomass burning smoke layers from this event in August 2017 was first reported by Khaykin et al. (2018). Haarig et al. (2018) present nighttime lidar observations of wildfire smoke aerosols during the event in both tropospheric and stratospheric layers over Leipzig, with lidar ratios of 40–45 sr (355 nm), 65–80 sr (532 nm), 80–95 sr (1064 nm), a low depolarization ratio (<0.03 at 355, 532, 1064 nm) for plumes in the troposphere, and a higher depolarization ratio (0.22 at 355 nm, 0.18 at 532 nm, 0.04 at 1064 nm) for plumes in the stratosphere. Later on, Baars et al. (2019) reported 6 months of observations (from August 2017 to January 2018) of such wildfire smoke aerosols during the episode with a network of 28 EARLINET ground-based lidars in Europe, showing the aerosol properties and the evolution of the smoke layer during the long-range transport. Recently, wildfire smoke layers were measured over the North Pole with a lidar aboard the icebreaker Polarstern during the MOSAiC (Multidisciplinary drifting Observatory for the Study of Arctic Climate) expedition (Engelmann et al., 2021; Ohneiser et al., 2021). However, the spatial resolution remains sparse, as advanced lidars are expensive. Similar observational records over northern Europe are more scarce in the literature.
Several national weather services have built up ceilometer networks for cloud monitoring (e.g., http://ceilometer.fmi.fi, last access: 21 April 2021, Hirsikko et al., 2014; E-Profile (EUMETNET Profiling Programme): https://e-profile.eu, last access: 21 April 2021) with unattended operation on a 24/7 basis. Information from the large number of ceilometers in these networks can fill the gaps between advanced lidar stations. Ceilometers are single-wavelength, eye-safe backscatter lidars, originally designed to determine cloud base heights. Studies (e.g., Wiegner and Geiß, 2012) show that ceilometers can also be used to retrieve the aerosol backscatter coefficient with high accuracy. However, the accuracy of the aerosol extinction coefficient retrieval is sensitive to the estimate of the unknown lidar ratio (LR). Ceilometers typically operate in the near-infrared (1064 nm or 910 nm) but the lidar ratios for different aerosol types have usually been observed and reported only at 532 and 355 nm. Only recently have lidar ratios at 1064 nm been measured by Raman lidar (Haarig et al., 2016).
Ceilometer measurements have been used in several aerosol studies even though the instruments were originally designed to measure cloud heights. From an Arctic station, Mielonen et al. (2013) reported ceilometer observations of biomass burning plume heights from the 2010 Russian wildfires in northern Finland. Tsaknakis et al. (2011) present an intercomparison of lidar and ceilometer measurements under different atmospheric conditions (urban air pollution, biomass burning, and Saharan dust event), showing good agreements in determining the mixing layer height and the attenuated backscatter coefficient. Cazorla et al. (2017) present the implementation of procedures to manage the Iberian Ceilometer Network (ICENET) for monitoring aerosol characterization for near real time, which has been tested during a dust outbreak. Ceilometer measurements of the German Weather Service (DWD) network (http://www.dwd.de/ceilomap, last access: 20 July 2021) were employed to follow the progression of the volcanic ash layer (Emeis et al., 2011) and to visualize the dispersion and temporal development of the North American smoke plumes (Trickl et al., 2015). Vaughan et al. (2018) showed how a dense network of lidars and ceilometers in the UK tracked the evolution of Canadian forest fire smoke. Adam et al. (2016) demonstrated that the operational ceilometer network of the Met Office can also provide valuable information for monitoring pollution events. Huff et al. (2021) demonstrated that ceilometers in the Unified Ceilometer Network (UCN, https://alg.umbc.edu/ucn/, last access: 20 July 2021) can verify and track smoke plume transport from a prescribed fire, in Maryland. Calibrated ceilometer profiles were also used as a tool to evaluate the aerosol forecasts by the European Centre for Medium-Range Weather Forecasts (ECMWF) Integrated Forecasting System aerosol module (IFS-AER) (Flentje et al., 2021). Dionisi et al. (2018) proposed a model-assisted methodology to retrieve key aerosol properties (such as extinction coefficient, surface area, and volume) from ceilometer measurements, under continental conditions; the good performances of that approach suggest that ceilometers can provide quantitative information for operational air quality and meteorological monitoring. In order to analyze to what extent the existing ceilometer infrastructure could do in the case of smoke monitoring, we performed a comparison study using an advanced Raman lidar, a ceilometer, and model data.
On 4–6 June 2019, biomass burning aerosol layers were observed in the lower troposphere over Kuopio, Finland. These smoke particles originated from a Canadian wildfire event. In this study, we present observations of the smoke plume from a multi-wavelength Raman polarization lidar PollyXT and a Vaisala CL51 ceilometer. A combined lidar–photometer approach is presented for estimating mass concentration as a good knowledge of the aerosol mass concentration is required from the aviation safety point of view (Schumann et al., 2011). Based on this approach, we applied two methods in this study: with method no. 1, measured backscatter coefficients were converted to backscatter coefficients at 532 nm by the corresponding measured backscatter-related Ångström exponent and were then applied to estimate the mass concentrations; with method no. 2, mass concentrations were estimated from measured backscatter coefficients at each wavelength (355, 532, 1064 nm from PollyXT and 910 nm from CL51) independently. This study reports, for the first time, a quantitative comparison study of mass concentration estimates of smoke particles, for Raman lidar and ceilometer observations. Moreover, we demonstrate the usefulness of a Vaisala ceilometer to monitor smoke (in terms of quantitative information on the aerosol load) in the troposphere; the potential for mass concentration retrieval from ceilometer observations is also discussed. In addition, the mass retrievals were compared with the Modern-Era Retrospective analysis for Research and Applications, version 2 (MERRA-2) meteorological and aerosol reanalysis.
This paper is organized as follows: in Sect. 2, the measurement site, instrumentation, and data analysis are described. In Sect. 3, the mass estimation methods and results are presented and discussed. The conclusions are given in Sect. 4.
The Vehmasmäki station in Kuopio ( N, E; 190 m a.s.l.), belonging to EARLINET and PollyNET, is a rural site, located ∼18 km from the city center of Kuopio, in eastern Finland. The station has been an operational profiling site since Autumn 2012 and is operated by the Finnish Meteorological Institute (Hirsikko et al., 2014). It is equipped with a ground-based multi-wavelength Raman polarization lidar PollyXT (Baars et al., 2016; Engelmann et al., 2016), Vaisala ceilometers CL31 and CL51, a Doppler lidar, and in situ instruments, next to a 318 m tall mast (for the meteorological observations) during the period. Vehmasmäki is located far from major aerosol sources such as dust or anthropogenic aerosol and the atmosphere is relatively clean.
2.1 Ancillary data
The closest AErosol RObotic NETwork (AERONET, http://aeronet.gsfc.nasa.gov, last access: 3 May 2021) station to the Vehmasmäki site is the Kuopio station ( N, E; 105 m a.s.l.), ∼18 km from Vehmasmäki. The AERONET sun photometers measure the aerosol optical depth (AOD) from 340 to 1640 nm (eight channels) for the total atmospheric column, with an uncertainty from 0.01 to 0.02 (Eck et al., 1999). The AERONET (version 3.0) level 2.0 direct sun products (O'Neill, 2003) and inversion products (Dubovik and King, 2000) were used in this study. These products include spectral AODs, fine-mode and coarse-mode AODs (at 440, 675, 870, and 1020 nm of inversion products or at 500 nm of direct sun products), and fine-mode- and coarse-mode-related volume concentrations in the entire vertical atmospheric column. The volume particle size distribution was retrieved in the range of radius of 0.05–15 µm; the minimum within the size interval from 0.439–0.992 µm was used as a separation point between fine- and coarse-mode particles. A detailed uncertainty analysis was performed by Dubovik et al. (2000), showing low errors in AOD and about 10 %–20 % error in volume concentration.
Temperature and pressure profiles from the GDAS (Global Data Assimilation System, https://www.ready.noaa.gov/gdas1.php, last access: 19 March 2021) database were used for the correction of Rayleigh extinction and backscattering effects for lidar data analysis. Aiming at the observations of water vapor profiles, the most used and well-established measurement method is radiosonde sounding (Wiegner et al., 2019). However, the closest available radiosonde data are from Jokioinen (Finland), located ∼300 km away from the measurement site. Filioglou et al. (2017) reported the inadequate vertical representation of water vapor due to the non-stable atmospheric conditions between two sites when using a radiosonde 100 km away. Thus, the relative humidity profiles from GDAS data were used for the water vapor number density estimations. HYSPLIT (HYbrid Single-Particle Lagrangian Integrated Trajectory, https://ready.arl.noaa.gov/HYSPLIT.php, last access: 19 March 2021) backward trajectories were also analyzed to study the air mass origins. Additionally, the thermal anomalies (fire sources) from Terra and Aqua MODIS data (MODIS, 2019) were synergistically used to locate where the forest fires were occurring. The “Dust score” data provided by AIRS (Atmospheric InfraRed Sounder) were used to determine the occurrences of dust events (https://airs.jpl.nasa.gov, last access: 1 July 2021).
MERRA-2 is a global reanalysis produced with the NASA global Earth System model, GEOS (Goddard Earth Observing System), coupled with the aerosol module GOCART (Goddard Chemistry, Aerosol, Radiation and Transport) (Gelaro et al., 2017) and includes the assimilation of aerosol observations (AOD) from various spaceborne instruments such as MODIS, AVHRR (Advanced Very High Resolution Radiometer), and MISR (Multi-angle Imaging SpectroRadiometer) (Buchard et al., 2017; Randles et al., 2017). It has an approximate horizontal resolution of and 72 hybrid-eta level levels from the surface to 0.01 hPa. Every 3 h, MERRA-2 produces vertical profiles of aerosol mass mixing ratio for five aerosol species (dust; sea salt – SS; black and organic carbon – BC and OC; and sulfate – SU) from which lidar optical parameters, such as aerosol extinction and backscattering coefficients, can be calculated.
2.2 PollyXT lidar
The multi-wavelength Raman polarization lidar PollyXT has three emission wavelengths (355, 532, 1064 nm) and 12 detection channels, including a far-range receiver unit with eight channels (355, 387, 407, 532, 607, 1064 nm, and two depolarization channels at 355 and 532 nm), and a near-range receiver unit with four channels (355, 387, 532, and 607 nm). The initial spatial and time resolution is 7.5 m and 30 s, respectively. The laser beams are tilted to an off-zenith angle of 5∘ to avoid specular reflections from horizontally aligned ice crystals. For the calculation of optical properties in this study, the profiles were temporally averaged at 2 h intervals and smoothed with a vertical gliding averaging window length of 11 bins (a vertical range of ∼82 m).
Data processing methods of Raman lidars are well established. When the signal-to-noise ratio is high enough for the received signals at inelastic Raman-shifted wavelengths (387 and 607 nm), which is mainly during the nighttime, profiles of extinction and backscatter coefficients at 355 and 532 nm can be derived independently using the Raman inversion technique (Ansmann et al., 1992). Otherwise, the Klett–Fernald method (Fernald, 1984; Klett, 1981) is applied using the elastic signals to retrieve the backscatter coefficients. The relative uncertainties are in the range of 5 %–10 % for backscatter coefficients and depolarization ratios at 355 and 532 nm (Ansmann et al., 1992; Baars et al., 2012). The backscatter coefficient retrieval at 1064 nm may be possible with a relative uncertainty of 15 % using only an elastic signal by assuming a proper lidar ratio. The lidar ratios at 355 and 532 nm are measured with a typical relative uncertainty of ∼20 % when the inelastic measurements are good enough. Higher uncertainties in lidar ratio at 1064 nm (∼30 %) should be considered (Haarig et al., 2018). Further details on the instrument setup, principle, and error propagation can be found in Engelmann et al. (2016).
2.3 Ceilometer and data processing
The Vaisala CL51 ceilometer used in this study is a commercial elastic backscatter lidar originally intended for measuring cloud base heights. It operates at 910 nm with an initial temporal resolution of 10 s and range resolution of 10 m and was tilted to an angle of 12–13∘ from the vertical. The horizontal distances between the laser beams of CL51 and PollyXT are of the order of ∼700 m at 5 km, which is considered negligible in this study. The signal-to-noise ratio (SNR) for raw CL51 backscatter signals above the boundary layers is weak; hence some temporal averaging and vertical smoothing were required when performing further analysis. In this study, CL51 signals were smoothed with a vertical gliding averaging window length of seven bins (a vertical range of ∼70 m). The profiles were temporally averaged at 10 min intervals for the time–height cross section quick look and at 2 h intervals to calculate the optical properties.
Kotthaus et al. (2016) states that background corrections are needed for some ceilometer firmware versions. Following the method proposed in Kotthaus et al. (2016), range histograms of observations from CL51 on clear-sky days were analyzed. The results show that the background corrections are not needed for the CL51 data from Kuopio station because the CL51 operates with a specific firmware version which is recommended by E-Profile and ACTRIS (the Aerosol, Clouds and Trace Gases Research Infrastructure, https://www.actris.eu, last access: 3 May 2021).
The instrument constant is not accurately calibrated in absolute terms for many of the ceilometers in the network (http://ceilometer.fmi.fi, last access: 3 May 2021) because the main application is the cloud base height detection, in which a correct instrument constant is not required. Different calibration procedures (e.g., relative and/or absolute calibration) have been proposed and applied in the literature. The stratocumulus cloud technique (O'Connor et al., 2004) is the most appropriate for the CL51 sensor which was used in this study and recommended by E-Profile for this sensor type. Stratocumulus cloud cases in 2019 were analyzed, and five good cases from April to September 2019 were found. A calibration factor of 1.75±0.03 was derived with small standard deviation during the 6 months, showing that the CL51 system is quite stable. A relative uncertainty on the instrument constant within 10 % should be considered, as the calibration approach contains a range of possible multiple-scattering factors.
Wiegner and Gasteiger (2015) report that the ceilometer signal must be corrected for water vapor if emitting wavelengths around 910 nm are used. They show that the error in the backscatter coefficient retrieval can be in the order of 20 % for midlatitudes, and more than 50 % for the tropics, if water vapor absorption is ignored. We performed similar simulations in Kuopio station, following the method given in Wiegner and Gasteiger (2015). The water vapor number densities were calculated from the relative humidity and the temperature profiles from GDAS data. The water vapor absorption cross sections in the spectral range between 900 and 920 nm were simulated based on the HITRAN (Rothman et al., 2005) database, which covers a wide range between 10−28 and 10−21 cm2. Wiegner and Gasteiger (2015) state that the annual variability of pressure and temperature has no significant influence on the water vapor absorption cross sections. It is possible to use the tabulated mean absorption cross section to calculate an approximative water vapor transmission with a high accuracy (the inherent error of the squared water vapor transmissions is <0.3 %; more details are given in Sect. 4 in Wiegner and Gasteiger, 2015). For the CL51, we assumed a Gaussian shape of the spectrum, with the central wavelength (λ0) of 910 nm and a full width at half maximum of 3.4 nm, as specified by Vaisala. Thus, the water vapor transmission can be estimated, and the effective water vapor transmission was applied to the ceilometer signals for the water vapor corrections (equations and more details can be found in Wiegner and Gasteiger, 2015). One example is given in Fig. 1:
- i.
Using the forward integration method, the retrieved particle backscatter coefficients without water vapor correction (β∗) were much lower than the ones with water vapor correction (β) (Fig. 1c). This underestimate increased with height in the boundary layer and by ∼40 % for the elevated layer.
- ii.
Using the backward integration method, neglecting that the water vapor led to an overestimate, which increased with the distance from the chosen reference height. An overestimate of ∼50 % can be found near the ground for the given example. Nevertheless, a much smaller error (∼6 %) was found for the elevated layer using this method compared to the forward integration method.
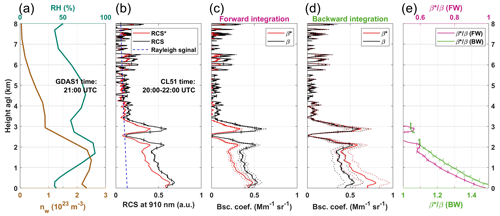
Figure 1Example of water vapor corrections on 2 h averaged ceilometer data on 5 June 2019 (20:00–22:00 UTC). (a) Relative humidity (RH, teal) and water vapor number density (nw, brown) from GDAS data at 21:00 UTC. (b) Range-corrected signal at 910 nm, without (RCS∗, red) or with (RCS, black) water vapor correction and the hypothetical Rayleigh signal at 910 nm (dashed blue). (c) Retrieved particle backscatter coefficients: β∗ without (red) and β with (black) water vapor correction, using forward (FW) integration Klett solution. (d) Same as (c) but application of the backward (BW) integration. (e) Ratio of the retrieved β∗ and β, when using forward integration (magenta) or backward integration (green). The horizontal lines illustrate the uncertainty range due to wrong assumptions of the central wavelength λ0±2 nm. The uncertainties in backscatter coefficients of the analytical solution were shown by dashed lines.
The uncertainty range due to wrong assumptions of λ0±2 nm is given by the horizontal lines in Fig. 1. The uncertainties in the backscatter coefficients of the analytical solution are also shown by dashed lines. Bedoya-Velásquez et al. (2021) applied a water vapor correction method to the CL51 ceilometer measurements, based on the one proposed by Wiegner and Gasteiger (2015). They also studied the sensitivity of the aerosol retrievals to the use of modeled temperature and absolute humidity from HYSPLIT to correct water vapor absorption instead of the co-located Microwave radiometer measurements: this leads to errors of up to 9 % and 2.2 % in the pre-processed range-corrected signals and in particle backscatter coefficients, respectively. Thus, an extra uncertainty should be considered as GDAS data were used for the water vapor correction. We cannot quantify the error in GDAS temperature. Nevertheless, PollyXT-measured relative humidity (RH) values were applied for the comparison, and good agreements were found. The relative difference in the squared effective water vapor transmissions using RH profiles of GDAS or PollyXT is less than 2 % for the case in Fig. 1. The input uncertainty in water vapor transmissions due to the use of modeled input was not taken into account in this study, as there was no means of quantifying the value. As the water vapor contribution cannot be neglected at Kuopio during summer, the water vapor corrections have been applied to CL51 data in this study.
The retrieval methods for deriving the backscatter coefficient from ceilometers are quite mature (Wiegner et al., 2014; Wiegner and Geiß, 2012). Under favorable conditions, a relative error of the backscatter coefficient on the order of 10 % seems feasible with a careful calibration by applying the forward integration. By contrast, significant temporal averaging of ceilometer data is required for performing a Rayleigh calibration, as the detection of molecular signals is intrinsically very difficult. Binietoglou et al. (2011) propose a two-step approach, resulting in promising agreement compared to their lidar PEARL (Potenza EARLINET Raman lidar). The uncertainty of the backscatter coefficient could be in the range of 20 %–30 % using the backward integration. The advantage of the forward algorithm is that calibration is required only occasionally, and it is not affected by the low SNR in the upper troposphere. However, the accuracy in deriving extinction coefficients is limited due to the unknown LR at 910 or 1064 nm and its uncertainties. In particular the presence of multi-layered aerosol distributions (with different aerosol types) may introduce more uncertainties. In addition, the uncertainty due to neglecting the water vapor increased with the distance from the chosen reference height. In this study, we applied the Klett method (Wiegner et al., 2014) by defining the reference height as close as to the layer of interest so that the error propagation (due to uncertainties of LR and water vapor transmission) would be minimized for that layer. Characteristic LR values for aerosol types are often measured at 355 or 532 nm; it is only recently that Haarig et al. (2016) measured the LR at 1064 nm and reported values of 80–95 sr for aged biomass burning smoke (Haarig et al., 2018). A value of 82 sr for LR, as measured at 1064 nm (82±27 sr in Haarig et al., 2018), was assumed as being appropriate for use at 910 nm in this study.
From 4 to 6 June 2019, several lofted aerosol particle layers were detected with PollyXT (near-real-time quick looks are publicly accessible at the PollyNET website: http://picasso.tropos.de, last access: 3 May 2021). The AERONET sun photometer observed enhanced AOD values for these days: the total AOD at 500 nm ranged from 0.24 to 0.42, whereas the mean values for the previous week and the following week were both below 0.08.
In this study, we focus on the observations on 5 June, when the most pronounced aerosol layers were detected. The range-corrected signals (RCSs) at 1064 nm from PollyXT and at 910 nm from CL51 on 5–6 June are presented in Fig. 2. A dense lofted aerosol layer was highlighted by the enhanced lidar signals, located at ∼5 km in the morning and descending to ∼2 km in the evening; this layer is defined as SPoI (smoke plume of interest). Two faint lofted thin layers were also detected below this layer in the morning. Our in situ pollen measurements (more information about pollen instruments can be found in Bohlmann et al., 2021) shows high pine pollen loading at the ground (highest 2 h pollen concentrations were ∼3000 m−3 on 5 June and ∼7000 m−3 on 6 June). The pollen particles were well mixed in the boundary layer (below 2 km), causing strong backscattering together with a high depolarization ratio at 532 nm with a clear diurnal cycle. Although also of interest, the analysis of the pollen layer is beyond the scope of this paper.
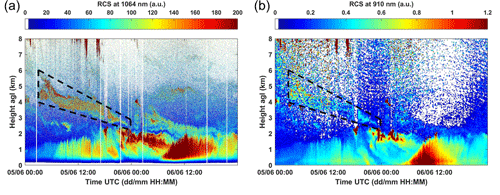
Figure 2Time–height cross section of range-corrected signal (RCS) (a) at 1064 nm of PollyXT and (b) at 910 nm of the CL51 ceilometer on 5 and 6 June 2019 over Vehmasmäki station. Time is given in UTC, and height is above ground. Initial PollyXT data were used with temporal and vertical resolutions of 30 s and 7.5 m, respectively. CL51 data were smoothed with temporal and vertical resolutions of 10 min and 70 m, respectively. The SPoI (smoke plume of interest) is inside the black box with dashed lines.
As shown in Fig. 3, the AERONET (level 2.0 aerosol spectral deconvolution algorithm (SDA) products) fine-mode AOD fraction on 5 June was higher than 93 %, and the Ångström exponent at 500–1020 nm (380–500 nm) varied between 1.4 and 1.8 (0.8 and 1.1), indicating the presence of fine particles in the atmospheric column. The coarse-mode AOD slightly increased during the daytime (always below 0.02) on 5 June, which can be interpreted as the pollen-related contribution to optical depth in the boundary layer. The higher coarse-mode AODs on 6 June could also be a consequence of higher pollen contributions. As a consequence, ceilometer signals were almost totally attenuated above 3 km on 6 June (Fig. 2b).
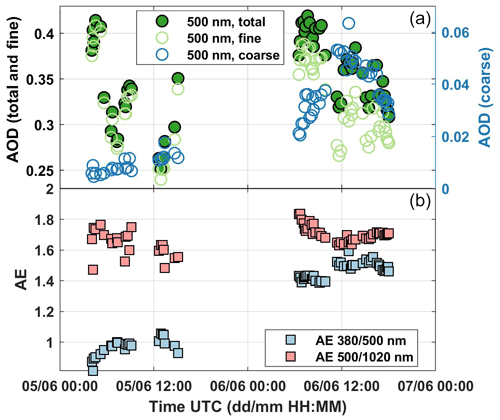
Figure 3AERONET sun-photometer observations (in Kuopio station, on 5 and 6 June 2019, http://aeronet.gsfc.nasa.gov, last access: 3 May 2021) of (a) 500 nm aerosol optical depth (level 2.0 data) and (b) Ångström exponents (AEs) computed from the optical depths measured at 380, 500, and 1020 nm. The fine-mode-related (for particle with diameters <1 µm) and coarse-mode-related aerosol optical depth (diameters >1 µm) are also shown (a, level 2.0 aerosol spectral deconvolution algorithm (SDA) products).
The backward trajectory analysis was performed using the HYSPLIT model. The analysis shows that particles in the SPoI had traveled about 7 d from the forest fire sources (MODIS, 2019) in western Canada to northern Europe (Fig. 4). The AIRS dust score map (https://airs.jpl.nasa.gov/map/, last access: 1 July 2021) also showed some dust presence in North America on 30 May.
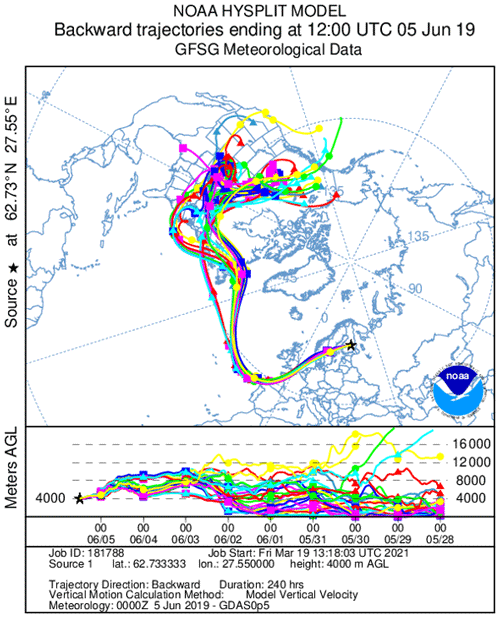
Figure 4Ten-day backward trajectories from the HYSPLIT model (in ensemble type), ending at 12:00 UTC on 5 June 2019 for Kuopio, Finland. The end location of the air mass is at 4 km a.g.l. in the SPoI (smoke plume of interest).
3.1 Optical properties
The pronounced smoke layer, i.e., the SPoI shown in the black box in Fig. 2, had a layer depth of ∼1.8 km in the early morning and became much thinner when descending at night. The PollyXT-derived AOD at 355 nm (532 nm) of this layer decreased from 0.21 (0.13) in the morning to 0.04 (0.02) in the night.
Layer-mean values of optical properties of the SPoI were derived and are given in Table 1. Two-hour time-averaged and vertically smoothed (with a smoothing window of ∼82 m) lidar profiles were used in order to increase the SNR. LR retrievals at 355 nm were available for the whole day, providing layer-mean values of 47±5 sr, which remained quite constant during the period. LR retrievals at 532 nm were only possible after sunset (after 18:00 UTC), resulting in layer-mean values of 71±5 sr. The 532 nm lidar ratio for aged smoke is larger than the 355 nm lidar ratio, in contrast to urban haze and fresh smoke, where the 355 nm lidar ratio is typically larger than the 532 nm lidar ratio (e.g., Nicolae et al., 2013; Pereira et al., 2014). Actually, as stated by Ansmann et al. (2021) and references therein, this characteristic ratio in LR, i.e., , is not produced by any other aerosol type and allows a clear identification of aged smoke.
Table 1Optical properties (lidar ratio; particle linear depolarization ratios – PDRs; backscatter- or extinction-related Ångström exponent – BAE or EAE) of biomass burning aerosols. Layer-mean values of the SPoI (smoke plume of interest) and the standard deviations are given. Optical properties and the effective radius (Reff) found in the literature on aged forest fire smoke aerosols observed in the troposphere are also given for comparisons. The source regions of these smoke aerosols are all Canada and/or North America.
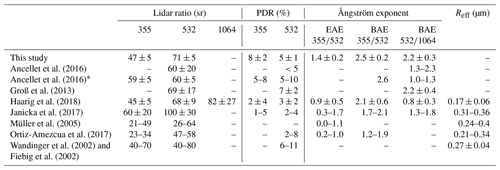
∗ Biomass burning mixing with a small amount of dust.
The backscatter coefficients of aged wildfire smoke show a clear and strong wavelength dependence for both 355–532 nm and 532–1064 nm wavelength ranges; the backscatter-related Ångström exponent (BAE) between 355 and 532 nm (between 532 and 1064 nm) shows high values of ∼2.5 (∼2.2). Nonetheless, the wavelength dependence of the extinction coefficient for the 355–532 nm spectral range is much weaker, with an extinction-related Ångström exponent (EAE) of ∼1.4. Nicolae et al. (2013) state that the EAE can be used for identifying the evolution of aging processes of biomass burning aerosol, as it decreased from 2 for fresh to ∼1.4–0.5 for aged biomass burning aerosols. The microphysical analysis was not performed in this study; yet the measured EAE would be related to the effective radius of ∼0.23 µm when considering the relationship between EAE and effective radius of forest fires smoke reported by Müller et al. (2005) (see Fig. 6 in that paper). This estimated effective radius value is consistent with those of aged smoke aerosols reported in the literature (Table 1). The AERONET sun-photometer Ångström exponent at 380–500 nm on 5 June showed lower values than lidar EAE at 355–532 nm; possible cirrus contamination could partly explain this as sun-photometer data are for the total atmospheric column. Note that the lidar's EAE was only available for nighttime (between 5 and 6 June).
The smoke particles caused slightly enhanced particle linear depolarization ratios (PDRs) at 355 nm (532 nm) with a mean value of 0.08±0.02 (0.05±0.01) in the smoke layer, suggesting the presence of partly coated soot particles or particles that have mixed with a small amount of dust or other non-spherical aerosol type. The layer-mean PDR at 355 nm (532 nm) decreased during the day, from ∼0.11 (0.06) in the morning to ∼0.05 (0.04) in the evening. The decrease in the PDR with time could be linked to the particle aging and related changes in the smoke particle shape properties, as stated by Baars et al. (2019). The relative humidity (RH) profiles from GDAS data showed low values in the lower atmosphere (<60 % below 6 km) before 15:00 UTC and even lower RH (<40 %) at the SPoI altitude. RH increased slightly in the evening. The signal in the 407 nm Raman-shifted channel was used to determine the water vapor mixing ratio profile during the nighttime, showing that the layer-mean RH changed from ∼27 % at 19:00 to ∼38 % at 23:00, which was associated with the advection of a moister air mass with a water vapor mixing ratio close to 1–3 g kg−1. The smoke particles were dry and then captured water vapor in the atmosphere during the evening. The decreasing temperature and increasing RH also increase the probability that smoke particles become glassy. The depolarization ratios of aged biomass burning aerosols (originating from Canada and/or North America) reported in the literature (Table 1) range from 0.01 to 0.11 (0.01 to 0.08) at 532 nm (355 nm). More information on the aged smoke from other regions can be found in the literature review by Adam et al. (2020; see the Supplement).
3.2 Mass concentration estimation
Ansmann et al. (2011) present a combined lidar–photometer method that enables the retrieval of the vertical profiles of ash and non-ash (fine-mode) particle mass concentration (m). It is based on the mass density (ρ) of the considered particles (mainly from the literature), the volume-to-extinction conversion factors cv (from the literature or computed from AERONET sun-photometer products), the backscatter coefficient (BSC, β) (from lidar measurements), and the lidar ratio (LR) (from Raman lidar measurements or assumptions depending on aerosol types), following the equation
where a indicates the aerosol type and λ the wavelength. cv,a uses the temporal mean value within a given time period to convert particle extinction coefficients into particle volume concentrations:
The column particle volume concentration v and corresponding optical thickness τ for aerosol component a are obtained from AERONET sun-photometer products.
This approach was applied to both PollyXT and CL51 data to estimate the mass concentration profiles for biomass burning aerosols in the SPoI. Adapting the methods described by Ansmann et al. (2021), we applied two methods in this study:
- Method no. 1:
mass concentrations were estimated from the measured backscatter coefficients which were converted to 532 nm, using the corresponding measured backscatter-related Ångström exponent. The volume-to-extinction conversion factors at 532 nm from the literature were applied (currently the only available wavelength for the smoke factor in the literature).
- Method no. 2:
mass concentrations were estimated from measured backscatter coefficients at each wavelength of 355, 532, 1064, and 910 nm. The volume-to-extinction conversion factors were evaluated at corresponding wavelengths using AERONET data.
In this study, we assume that both methods can be applied appropriately, and the limitations and sources of uncertainties of method no. 2 will be discussed in Sect. 3.2.2. The recommendation on the chosen method will be discussed later.
We assume that there are only biomass burning aerosols in the SPoI. Values for the smoke particle density vary in the literature (Chen et al., 2017; Li et al., 2016; Reid et al., 2005a) but should be in the range of 1.0–1.3 g cm−3 (Ansmann et al., 2021). In this study, a particle density ρs of 1.3 g cm−3 was used for the biomass burning particles. In the SPoI, the backscatter coefficient of smoke particles is assumed to be equal to the total particle backscatter coefficient (βs=βtotal). Figure 5a shows the lidar-derived backscatter coefficients at 355 (blue), 532 (green), and 1064 nm (red) from PollyXT and at 910 nm (black) from CL51 for 2 h time-averaged lidar profiles on 5 June 2019. Signals were smoothed with vertical gliding averaging window lengths of 11 bins for PollyXT and 7 bins for CL51. The peak value of backscatter coefficients in the SPoI reached ∼5 at 355 nm in the morning (06:00–08:00 UTC) and reduced to ∼3 at 355 nm at night (22:00–24:00 UTC).
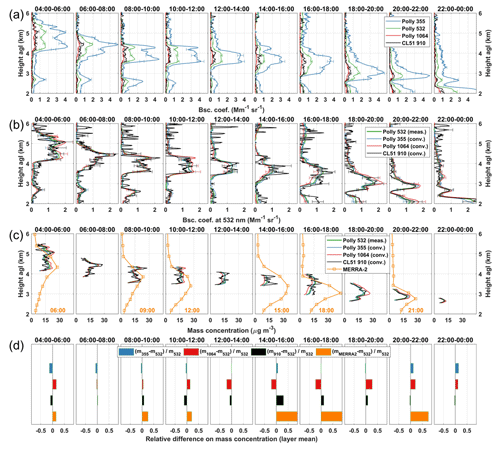
Figure 5(a) Lidar-derived backscatter coefficients (BSC) at 355 (blue), 532 (green), and 1064 nm (red) from PollyXT and at 910 nm (black) from CL51. (b) BSCs at 532 nm: measured at 532 nm (meas.) or converted (conv.) from measured BSCs at other wavelengths. (c) Estimated mass concentration profiles for the SPoI (smoke plume of interest) using BSCs in (b), based on parameters in Table 2, method no. 1. Mass concentrations from MERRA-2 model are also shown in orange color with corresponding time given at the bottom right of each panel. (d) Relative differences in the mass concentrations (denoted as m) estimated from measured and converted BSCs and of the MERRA-2 model, using the one from measured BSC at 532 nm as the reference. Two-hour time-averaged lidar profiles are used, with the time slot (UTC) on 5 June 2019 given at the top of each panel. The horizontal lines (in a, b) illustrate the uncertainty range. The uncertainties in mass concentrations (in c) are discussed in Sect. 3.2.1.
Table 2Parameters required for the mass concentration retrieval using two methods. The smoke mass density and lidar ratio at 532 nm are common parameters required for both methods no. 1 and no. 2.
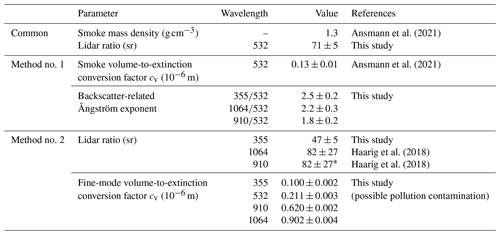
∗ LR values measured at 1064 nm are used for LR at 910 nm.
3.2.1 Method no. 1: based on BAE and the conversion factor from the literature
Ansmann et al. (2021) recommended the used of m as the conversion factor at 532 nm for the smoke observations far away from fire regions. In this section, we used this recommended smoke conversion factor of 0.13 (denoted as ). The BAEs between a wavelength λ (which can be 355 or 1064 nm of PollyXT or 910 nm of CL51) and 532 nm (of PollyXT) were derived (Eq. 3) for the SPoI (shown in Table 2) using the measured BSCs. These measured backscatter coefficients were converted to the wavelength of 532 nm, denoted as , following Eq. (4); the profiles of these converted backscatter coefficients are given in Fig. 5b. The mass concentration can be thus estimated by Eq. (5), as the product of , the smoke lidar ratio at 532 nm (LR532), the conversion factor at 532 nm, and the smoke particle densities (Table 2). The mass concentration profiles of the SPoI, retrieved from measured backscatter coefficients at four wavelengths based on method no. 1, are given in Fig. 5c.
The peak value of the mass concentrations of ∼23.5 (27.5) µg m−3 estimated from the backscatter coefficients at 532 nm (910 nm) was found at 06:00–08:00 UTC. If we take the mass concentration estimated from the BSC at 532 nm as the reference, good agreements are found between the mass concentrations estimated from BSCs at different wavelengths (Fig. 5d). The mean values of the relative differences were around 8 %, 12 %, and 18 % for the estimations from BSCs at 355, 910, and 1064 nm, respectively. Comparing 532 and 355 nm mass estimates, better agreement was found during the daytime (08:00–20:00 UTC), with a difference <6 %. Nonetheless, considering the 532 and 910 nm estimates, the best agreement was found at 06:00–08:00 and 20:00–24:00 UTC, with a difference <3 %, whereas the worst agreement of ∼30 % was found at 14:00–16:00 UTC. Larger differences between the 910 and 1064 nm estimates were found, with a mean relative difference of ∼28 % and a highest value of ∼64 % at 14:00–16:00 UTC.
In Table 3, the uncertainties in the input parameters and the estimated mass concentrations are listed. We assume an uncertainty of 20 % in the smoke mass density (Ansmann et al., 2021). The uncertainties in backscatter coefficients at different wavelengths and the lidar ratio at 532 nm follow from the discussions in Sect. 2. The conversion factor and lidar ratio at 532 nm are required as input, with assumed uncertainties of 10 % (given in Ansmann et al., 2021) and 20 % (see Sect. 2.2), respectively. The uncertainties in BAE between different wavelength pairs and in were obtained by error propagations to Eqs. (3) and (4). Note that the standard deviations of BAE from our measurements (Table 2) show lower values than their uncertainties. Finally, after applying the law of error propagation to Eq. (5), we expect an overall uncertainty in the mass concentration estimates of 32 %–45 %. The highest uncertainty of 45 % was found when using the ceilometer method, mainly due to the higher uncertainty of 20 % in the backscatter coefficient retrieval.
Table 3Relative uncertainties in the input parameters and in the retrieved products (in italics). The uncertainty origins are given for input parameters and denoted as follows: R – Raman measurement available; E – only elastic measurement for the retrieval; L – literature; A – assumption. The uncertainty in the smoke mass density (ρ) was assumed to be 20 % as in Ansmann et al. (2021). Different retrieval information (R or E) is available at each wavelength with a different system (PollyXT or CL51); thus different uncertainties in the backscatter coefficients (β) and lidar ratio (LR) are considered. The uncertainty in the smoke volume-to-extinction conversion factor (cv) was assumed as 10 % for both methods, as given in Ansmann et al. (2021). The relative uncertainties in the mass concentration (m), backscatter-related Ångström exponent (BAE), and converted backscatter coefficient () are obtained by the error propagation applied to Eqs. (1)–(5).
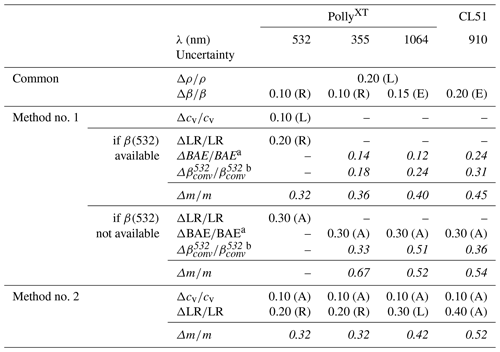
a Wavelength pair of λ and 532. b Converted backscatter coefficient at 532 nm from λ.
However, the lidar measurements at 532 nm are not always co-located, especially for numerous ceilometer stations. For those cases, the lidar ratio at 532 nm and the BAEs (or color ratios) should be assumed, thus with higher uncertainties. We can assume uncertainties of 30 % in lidar ratio at 532 nm and 30 % in BAEs for all wavelength pairs; thus, the uncertainty for the estimated mass concentrations will be over 50 % (Table 3). For the smoke particles, extended overviews of observed wavelength dependencies of backscatter coefficients can be found in Burton et al. (2012) and Adam et al. (2020).
3.2.2 Method no. 2: BSC at each wavelength and conversion factors from site
Method no. 1 is recommended when the measurements at 532 nm are additionally available or the BAE (or backscatter color ratio) can be reasonably assumed. Nevertheless, here we suggest a second method, in which mass concentrations were estimated from measured backscatter coefficients at several wavelengths independently, and the measurement at one single wavelength (e.g., for elastic lidars and ceilometers) is required as input for each estimate. This method no. 2 is recommended in regions with the pure aerosol type (dust, smoke, marine, etc.) condition, where the conversion factor can be evaluated with high accuracy. The mass estimations of the SPoI from measured backscatter coefficients at each wavelength are compared in this section.
Since AERONET inversion products of level 2.0 were not available on 5 June 2019, the AERONET products for the observations on 6 June 2019 (three distributions at 06:33, 07:10, and 14:41) were used to compute the volume-to-extinction conversion factors at different wavelengths for fine-mode particles (cv). The fine-mode fraction on 6 June was a bit lower than on 5 June (Fig. 3) but still higher than 90 % before 10:00 UTC and around 87 % in the afternoon (11:00–16:00). From the size distribution, the separation points between fine- and coarse-mode particles were found as ∼0.576 µm (the size classes 1–10 were considered for fine-mode aerosols). Here, the assumption is made that the photometer-derived fine particles are mainly smoke particles. Both the Dubovik approach (Dubovik et al., 2006; Dubovik and King, 2000) and the O'Neill method (2003) were applied at the wavelength of 532 nm, resulting in similar values for this factor (∼0.1 % difference). For wavelengths of 355, 910, and 1064 nm, only the Dubovik approach was applied. The mean conversion factors at four wavelengths are given in Table 2 (method no. 2) together with their standard deviations.
The estimated conversion factor value at 532 nm of m is higher than what we used in the previous section, with the difference ( m) larger than the uncertainty. This value is higher than the values for both fresh and aged smoke observations (from 0.13±0.01 to m) at several AERONET stations reported in Ansmann et al. (2021). However, Ansmann et al. (2012) also applied a high value of m for the mass concentration retrieval of smoke aerosols (fine mode) when studying lofted layers containing desert dust and biomass burning smoke. It is hard to distinguish between smoke and urban haze aerosols, as they are often small (with sizes up to about 1 µm in radius) and quasi-spherical aerosols. Further, the characteristic conversion factors are in a similar value range. For examples, Ansmann et al. (2011) reported a conversion factor of m for central European haze; Mamali et al. (2018) found a factor of m for continental/pollution particles over Cyprus; Mamouri et al. (2017) computed a factor of m for continental aerosol pollution over Germany.
Air mass sources of aerosols on 6 June were investigated by the backward trajectory analysis (HYSPLIT model). It shows that some of the particles were coming from the forest fire in the Canada region, while part of them were transported from Poland where urban haze could have been mixed with smoke aerosols (e.g., Fig. 6). The aerosol subtype products (version 4.20) from CALIPSO when the orbit was passing over Poland on 3 June (orbit from UTC 11:44 to 11:58) and 4 June (orbit from UTC 01:18 to 01:31) indicate the presence of polluted continental/smoke and polluted dust.
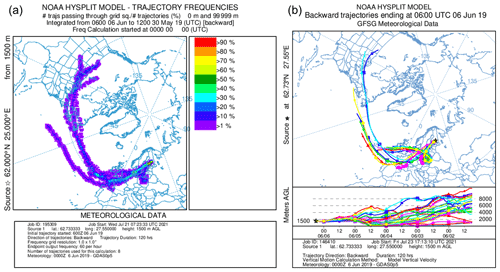
Figure 6Five-day backward trajectories from the HYSPLIT model (a) in frequency option, and (b) in ensemble option, ending at 06:00 UTC on 6 June 2019 for Kuopio, Finland. The end location of the air mass is at 1.5 km a.g.l. in the range-transported plume.
Consequently, it is possible that European pollution was mixed with Canadian smoke aerosols on 6 June in the fine-mode particles. Hence, the retrieved conversion factors cannot perfectly describe the smoke. However, in this section we still assume these factors reflect the smoke so as to do the comparison analysis of estimated mass concentration from PollyXT and CL51.
The mass concentrations were derived from the product of the BSCs at four wavelengths, their respective smoke lidar ratios, the related conversion factors, and the smoke particle densities (Table 2, Eq. 1). The estimated mass concentration profiles are given in Fig. 7a, based on the lidar-derived BSCs at 355, 532, 910, or 1064 nm, independently.
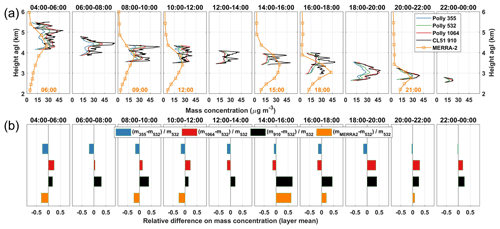
Figure 7(a) Estimated mass concentration profiles for the SPoI (smoke plume of interest), based on parameters in Table 2, method no. 2, using corresponding measured backscatter coefficients (BSCs, Fig. 5a). Mass concentrations from the MERRA-2 model are also shown in orange color with the corresponding time given at the bottom right of each panel. The uncertainties are discussed in Sect. 3.2.2. (b) Relative differences in the mass concentrations (denoted as m) estimated from measured BSCs and the MERRA-2 model, using the one from measured BSC at 532 nm as the reference. Two-hour time-averaged lidar profiles are used, with the time slot (UTC) on 5 June 2019 given at the top of each panel.
The peak value of the mass concentrations estimated from the BSCs at 532 nm reached ∼38 µg m−3 at 06:00–08:00 UTC, higher than the one estimated from method no. 1 because of the bigger conversion factor. The relative differences in the mass concentrations estimated from the BSCs at different wavelengths were analyzed (Fig. 7b). Similarly, we take the mass concentration estimated from the BSCs at 532 nm (which is the wavelength most often used in earlier studies) as a reference and found an underestimate when using BSCs at 355 nm, with a mean bias of ∼15 % and a peak bias of ∼25 % at 04:00–06:00 UTC; the best agreement was found for nighttime measurements (20:00–24:00 UTC) with a bias <5 %. Nevertheless, an overestimate was found for the mass concentration estimated from the BSCs at 910 nm, with a mean bias of ∼36 %, a peak bias of ∼68 % at 14:00–16:00 UTC, and a minimum bias of ∼14 % at 10:00–12:00 UTC. The overestimate for CL51-derived mass concentrations could be due to an overestimate of LR at 910 nm, since we used LR at 1064 nm in the calculations. In addition, big differences (with a mean value of ∼42 %) were found between the CL51-derived mass concentrations and the ones estimated from the PollyXT-derived BSCs at 1064 nm; the highest discrepancy, of ∼95 %, was found at 14:00–16:00 and ∼75 % at 16:00–18:00 UTC, whereas better agreements were found at 04:00–06:00, 10:00–12:00, and 18:00–24:00, with a bias <7 %.
The uncertainties in the input parameters and the estimated mass concentrations of this method no. 2 are listed in Table 3. The uncertainties in the conversion factors from the standard deviation in Table 2 are very small due to the limited sample number; thus 0.10 was used as proposed in Ansmann et al. (2021). The uncertainties in backscatter coefficients and lidar ratios at each wavelength follow from the discussion in Sect. 2. Uncertainties in the lidar ratio at ceilometer wavelengths are much larger, particularly as we applied the lidar ratio value measured at 1064 nm to the ceilometer wavelength of 910 nm. Thus, we assume an uncertainty of 40 % in the ceilometer lidar ratio. The overall uncertainties in the mass concentration estimates are of about 30 %–50 %, with the highest uncertainty of 52 % when using ceilometer measurements.
As can be seen in Table 3, when applying method no. 2, the uncertainty in mass concentration estimations is slightly lower using measured BSCs at 355 nm, whereas higher uncertainties were found when using measured BSCs at 1064 and 910 nm. The main reason lies in the high uncertainties in lidar ratios at 1064 and 910 nm. Hence, when the lidar ratio can be measured or properly estimated and the conversion factor can be estimated under the pure aerosol type condition, method no. 2 is recommended. Otherwise, method no. 1 can be applied by using properly estimated BAEs or color ratios.
The good agreement between mass concentrations derived from PollyXT and CL51 measurements in this study shows the potential of mass concentration estimates from ceilometers. However, when deriving parameters such as mass concentration from ceilometers, the applied parameters (i.e., the BAE532,910 value for method no. 1 or the LR910 and cv,910 values for method no. 2) and their uncertainties should always be carefully evaluated and provided, as the accuracy of the retrieved mass concentration depends primarily on the accuracy of the parameters that are not derived from the ceilometer observations.
3.3 Comparison with MERRA-2 model – wildfire smoke and dust aerosol mixture
The mass concentrations from MERRA-2 model data are used for the comparison with the lidar retrievals. An interesting feature in the MERRA-2 simulation results is the presence of dust in the SPoI. The contribution of dust to the total AOD is very low (much lower than the carbon optical depth), indicating that the dust particles are in the fine mode. However, the dust contribution to the total mass concentration is non-negligible. Low values of the lidar-derived depolarization ratio suggest no significant presence of non-spherical particles, but in principle, a small amount of dust could be mixed with the smoke. It is possible that there are biomass burning aerosols and fine dust aerosols in the SPoI, as only fine dust particles should be able to remain in the atmosphere for long enough to be transported from North America to Kuopio. Furthermore, the air masses in SPoI passed by the area in North America where dust was present (shown by the AIRS data).
In this section, the MERRA-2 mass concentrations were compared with the mass concentrations estimated from the PollyXT backscatter coefficients at 532 nm (from method no. 1, Fig. 5c, and method no. 2, Fig. 7a). Note that the main difference in PollyXT-estimated mass concentrations from two methods is due to the different conversion factor values (Table 2); thus the mass concentrations estimated from BSCs at 532 nm using method no. 1 are ∼40 % lower than method no. 2. When the PollyXT estimates from method no. 1 were used as the reference, good consistencies were found in the morning (at 06:00, 09:00, and 12:00 UTC), with overestimations (<30 %) of MERRA-2 mass concentrations, whereas large discrepancies were found in the afternoon, with high overestimations of ∼160 % at 15:00 UTC and ∼90 % at 18:00 UTC. If the PollyXT estimates from method no. 2 were used as the reference, good consistencies were also found in the morning (at 06:00, 09:00, and 12:00 UTC), but with underestimations (<30 %), and a large overestimation of ∼63 % was found at 15:00 UTC. At 15:00 UTC, the MERRA-2 simulated dust mass concentration fraction is more than half of the MERRA-2 simulated total mass concentration. It is good to keep in mind that both observations and simulations have significant uncertainties. The presence of cirrus cloud in the upper atmosphere during the day may also have some impacts on MODIS AOD, which is assimilated by the MERRA-2 model.
In order to check how the inclusion of dust (as indicated by MERRA-2) would affect the mass concentration estimations, we assume that there was a mixture of wildfire smoke and fine dust aerosol in the SPoI. The POLIPHON (polarization-lidar photometer networking) method (Mamouri and Ansmann, 2014, 2017) was applied to separate fine dust (particles with radius <500 nm) and biomass burning aerosols for the SPoI. Here we used the PollyXT retrieved particle backscatter coefficient and particle linear depolarization ratio profiles at 532 nm because the uncertainty in the 355 nm particle depolarization ratios is much larger (Mamouri and Ansmann, 2017). The depolarization ratios at 532 nm of smoke and fine dust particles were assumed to be 0.03 (Haarig et al., 2018) and 0.16 (Sakai et al., 2010). The fine dust and smoke extinction coefficients were obtained by multiplying the backscatter coefficients with their respective lidar ratios as follows: Ansmann et al. (2019) report that the typical dust lidar ratio is 40 sr at 532 nm, and lidar ratios for fine- and coarse-mode dust were assumed to be the same. For smoke particles, we took the lidar ratio of 71 sr, which was retrieved during our nighttime measurements. For the fine dust, the conversion factor cv,d of m (Ansmann et al., 2019) and the particle density of 2.6 g cm−3 (Ansmann et al., 2012) were used. For smoke particles, 1.3 g cm−3 was used as the particle density (Ansmann et al., 2021; Reid et al., 2005a), whereas the conversion factors cv,s of m (method no. 1) and m (method no. 2) were both applied. These parameters are reported in Table 4. The fine dust and smoke particle mass concentrations were derived using Eq. (1). For the example given in Fig. 8, the fine dust contributes ∼13 % to the extinction in the SPoI, whereas its mass concentration contributes ∼32 % (method no. 1) or ∼23 % (method no. 2) to the total mass concentration. However, the derived total mass concentration considering a fine dust and smoke mixture is only ∼18 % (method no. 1) or ∼4 % (method no. 2) higher than one assuming smoke particles only. The inclusion of a dust mixture results in slightly higher estimated mass concentration values, with a negligible difference considering the uncertainties.
Table 4Parameters required for the mass concentration retrieval, considering fine dust and smoke mixture.
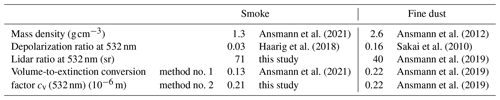
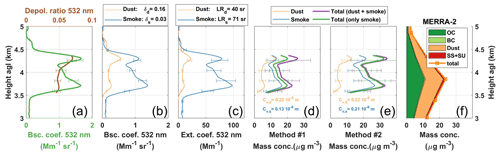
Figure 8Lidar products obtained from PollyXT measurements on 5 June 2019, 08:00–10:00 UTC (2 h signal average). (a) Measured 532 nm total particle backscatter coefficient (green) and particle linear depolarization ratio (brown). (b) Particle backscatter coefficients (BSCs) for fine dust (orange) and smoke (blue) particles, obtained with the POLIPHON method. (c) Respective fine dust and smoke extinction coefficients (EXTs) obtained by multiplying the BSCs (in b) with the lidar ratios. (d, e) The fine dust (orange) and smoke (blue) particle mass concentrations derived from the EXT profiles (in c), by using parameters in Table 4 for method no. 1 (d) and no. 2 (e). The total mass concentrations of fine dust and smoke mixture (purple) or of only smoke particles (green) are shown. (f) Mass concentrations of organic carbon (OC, dark green), black carbon (BC, light green), dust (orange), sea salt, and sulfate (SS+SU, red) from the MERRA-2 model at 09:00 UTC on 5 June. The total mass concentration profile is also given by orange squares. The horizontal lines illustrate the uncertainty range.
We have also performed POLIPHON considering a coarse-mode dust mixture; higher (∼20 %–30 %) total mass concentrations were retrieved but they were still within the uncertainty range. The aged smoke aerosols may also introduce enhanced depolarization ratios. If we use a larger value (e.g., 0.05) instead of 0.03 as the smoke depolarization ratio in POLIPHON, the dust impacts on the mass concentration estimations are even smaller. Hence, the mass estimations of the SPoI considering only smoke are good enough even if the plume contains a small amount of dust.
A similar conclusion can also be applied to ceilometer observations. It is not possible to perform the aerosol separation using ceilometer data alone, as no depolarization information is available at this wavelength. For this instrument, only one aerosol type should always be assumed in the layer of interest, which then imparts an additional bias when estimating the mass concentration. However, we have shown in this section that ceilometer observations for mass retrievals can be used even without an exact knowledge of the composition of the smoke plume in the troposphere.
On 4–6 June 2019, aerosol layers arising from biomass burning were observed in the lower troposphere between 2–5 km in altitude over Kuopio, Finland. Enhanced backscattered signals were detected by both a multi-wavelength Raman polarization lidar PollyXT and a Vaisala CL51 ceilometer. The HYSPLIT backward trajectory analysis and MODIS fire data suggested that these long-range-transported smoke particles originated from a Canadian wildfire event. An AERONET sun photometer located in Kuopio observed enhanced AOD values in concert with high Ångström exponents, indicating the presence of fine-mode dominant aerosols in the atmospheric column.
The most pronounced smoke plume, defined as SPoI (smoke plume of interest), detected on 5 June was intensively investigated. Lidar ratios were derived from the Raman lidar, as 47±5 sr at 355 nm and 71±5 sr at 532 nm, showing that the aerosols of biomass burning origin in the SPoI were medium- to highly absorbing particles. Particle linear depolarization ratios in this layer were measured as 0.08±0.02 at 355 nm and 0.05±0.01 at 532 nm; which could indicate the presence of irregular-shaped aged smoke particles and/or mixing with a small amount of fine dust particles. Complete processing steps for Vaisala CL51 ceilometer data analysis were firstly reported in this study. The water vapor correction was analyzed and applied at a high latitude for the first time, showing that water vapor absorption cannot be neglected for high-latitude stations during summer. Two methods, based on a combined lidar and sun-photometer approach (based on AERONET products), were applied to both PollyXT and CL51 data for estimating mass concentrations: with method no. 1, measured backscatter coefficients were converted to backscatter coefficients at 532 nm by the corresponding measured backscatter-related Ångström exponent and then applied to estimate the mass concentrations; with method no. 2, mass concentrations were estimated from measured backscatter coefficients at each wavelength (355, 532, 1064 nm from PollyXT and 910 nm from CL51) independently. A difference of ∼12 % or ∼36 % was found between PollyXT and CL51 estimated mass concentrations using method no. 1 or no. 2, showing that ceilometers are potential tools for mass concentration retrievals with ∼50 % uncertainty but with great spatial coverage. The retrieved mass concentration profiles were also compared with MERRA-2 aerosol profiles, where we considered and analyzed two scenarios in the SPoI – (1) only smoke particles and (2) a mixture of fine dust and smoke aerosols – and reported these with the corresponding uncertainties. The inclusion of dust in the retrieved mass concentration is negligible considering the uncertainties, which indicates that ceilometer observations for mass retrievals can be used even without an exact knowledge of the composition of the smoke-dominated aerosol plume in the troposphere. We demonstrated the potential of the Vaisala CL51 ceilometer to contribute to atmospheric aerosol research in the vertical profile (e.g., to monitor smoke in the troposphere) from a sensor-provided attenuated backscatter coefficient to particle mass concentration.
The data for this paper are available from the authors upon request.
XS analyzed the data and wrote the paper with contributions from the co-authors. XS, TM, AnL, AH, and MK conceptualized the study. EG and AA helped in the validation of lidar and AERONET data analysis. ArL provided and assured the quality of the in situ measurements. VB and AD provided and assured the quality of the MERRA-2 data. AK carried out the simulations for the water vapor absorption cross sections. MK and EO ran the lidars and collected the observational data. EO and AH helped with ceilometer data analysis. All authors were involved in the interpretation of the results and reviewing and editing the paper.
The contact author has declared that neither they nor their co-authors have any competing interests.
Publisher's note: Copernicus Publications remains neutral with regard to jurisdictional claims in published maps and institutional affiliations.
The authors acknowledge the use of imagery from the NASA Worldview application (https://worldview.earthdata.nasa.gov, last access: 3 May 2021), part of the NASA Earth Observing System Data and Information System (EOSDIS). We thank the NOAA Air Resources Laboratory (ARL) for the provision of the HYSPLIT transport and dispersion model used in this publication. The authors acknowledge the MODIS Science, Processing and Data Support Teams for producing and providing MODIS data. The authors acknowledge the AIRS research team for the AIRS observation data and the NASA Langley Research Center Atmospheric Sciences Data Center for the data processing and distribution of CALIPSO products. The authors thank Stephanie Bohlmann and Anu-Maija Sundström for fruitful discussions.
This research has been supported by the National Emergency Supply Agency (decision no. 19078). Tero Mielonen's work was supported by the Academy of Finland (grant no. 308292).
This paper was edited by Ulla Wandinger and reviewed by three anonymous referees.
Adam, M., Turp, M., Horseman, A., Ordóñez, C., Buxmann, J., and Sugier, J.: From Operational Ceilometer Network to Operational Lidar Network, EPJ Web Conf., 119, 27007, https://doi.org/10.1051/EPJCONF/201611927007, 2016.
Adam, M., Nicolae, D., Stachlewska, I. S., Papayannis, A., and Balis, D.: Biomass burning events measured by lidars in EARLINET – Part 1: Data analysis methodology, Atmos. Chem. Phys., 20, 13905–13927, https://doi.org/10.5194/acp-20-13905-2020, 2020.
Alados-Arboledas, L., Müller, D., Guerrero-Rascado, J. L., Navas-Guzmán, F., Pérez-Ramírez, D., and Olmo, F. J.: Optical and microphysical properties of fresh biomass burning aerosol retrieved by Raman lidar, and star-and sun-photometry, Geophys. Res. Lett., 38, L01807, https://doi.org/10.1029/2010GL045999, 2011.
Amiridis, V., Balis, D. S., Giannakaki, E., Stohl, A., Kazadzis, S., Koukouli, M. E., and Zanis, P.: Optical characteristics of biomass burning aerosols over Southeastern Europe determined from UV-Raman lidar measurements, Atmos. Chem. Phys., 9, 2431–2440, https://doi.org/10.5194/acp-9-2431-2009, 2009.
Ancellet, G., Pelon, J., Totems, J., Chazette, P., Bazureau, A., Sicard, M., Di Iorio, T., Dulac, F., and Mallet, M.: Long-range transport and mixing of aerosol sources during the 2013 North American biomass burning episode: analysis of multiple lidar observations in the western Mediterranean basin, Atmos. Chem. Phys., 16, 4725–4742, https://doi.org/10.5194/acp-16-4725-2016, 2016.
Andreae, M. O.: Biomass burning: Its history, use and distribution and its impact on environmental quality and global climate, in: Global Biomass Burning: Atmospheric, Climatic and Biospheric Implications, edited by: Levine, J. S., MIT Press, Cambridge, Mass., 3–21, 1991.
Andreae, M. O.: Emission of trace gases and aerosols from biomass burning – an updated assessment, Atmos. Chem. Phys., 19, 8523–8546, https://doi.org/10.5194/acp-19-8523-2019, 2019.
Ansmann, A., Wandinger, U., Riebesell, M., Weitkamp, C., and Michaelis, W.: Independent measurement of extinction and backscatter profiles in cirrus clouds by using a combined Raman elastic-backscatter lidar, Appl. Optics, 31, 7113–7131, https://doi.org/10.1364/AO.31.007113, 1992.
Ansmann, A., Tesche, M., Seifert, P., Groß, S., Freudenthaler, V., Apituley, A., Wilson, K. M., Serikov, I., Linné, H., Heinold, B., Hiebsch, A., Schnell, F., Schmidt, J., Mattis, I., Wandinger, U., and Wiegner, M.: Ash and fine-mode particle mass profiles from EARLINET-AERONET observations over central Europe after the eruptions of the Eyjafjallajökull volcano in 2010, J. Geophys. Res., 116, D00U02, https://doi.org/10.1029/2010JD015567, 2011.
Ansmann, A., Seifert, P., Tesche, M., and Wandinger, U.: Profiling of fine and coarse particle mass: case studies of Saharan dust and Eyjafjallajökull/Grimsvötn volcanic plumes, Atmos. Chem. Phys., 12, 9399–9415, https://doi.org/10.5194/acp-12-9399-2012, 2012.
Ansmann, A., Baars, H., Chudnovsky, A., Mattis, I., Veselovskii, I., Haarig, M., Seifert, P., Engelmann, R., and Wandinger, U.: Extreme levels of Canadian wildfire smoke in the stratosphere over central Europe on 21–22 August 2017, Atmos. Chem. Phys., 18, 11831–11845, https://doi.org/10.5194/acp-18-11831-2018, 2018.
Ansmann, A., Mamouri, R.-E., Hofer, J., Baars, H., Althausen, D., and Abdullaev, S. F.: Dust mass, cloud condensation nuclei, and ice-nucleating particle profiling with polarization lidar: updated POLIPHON conversion factors from global AERONET analysis, Atmos. Meas. Tech., 12, 4849–4865, https://doi.org/10.5194/amt-12-4849-2019, 2019.
Ansmann, A., Ohneiser, K., Mamouri, R.-E., Knopf, D. A., Veselovskii, I., Baars, H., Engelmann, R., Foth, A., Jimenez, C., Seifert, P., and Barja, B.: Tropospheric and stratospheric wildfire smoke profiling with lidar: mass, surface area, CCN, and INP retrieval, Atmos. Chem. Phys., 21, 9779–9807, https://doi.org/10.5194/acp-21-9779-2021, 2021.
Baars, H., Ansmann, A., Althausen, D., Engelmann, R., Heese, B., Müller, D., Artaxo, P., Paixao, M., Pauliquevis, T., and Souza, R.: Aerosol profiling with lidar in the Amazon Basin during the wet and dry season, J. Geophys. Res.-Atmos., 117, D21201, https://doi.org/10.1029/2012JD018338, 2012.
Baars, H., Kanitz, T., Engelmann, R., Althausen, D., Heese, B., Komppula, M., Preißler, J., Tesche, M., Ansmann, A., Wandinger, U., Lim, J.-H., Ahn, J. Y., Stachlewska, I. S., Amiridis, V., Marinou, E., Seifert, P., Hofer, J., Skupin, A., Schneider, F., Bohlmann, S., Foth, A., Bley, S., Pfüller, A., Giannakaki, E., Lihavainen, H., Viisanen, Y., Hooda, R. K., Pereira, S. N., Bortoli, D., Wagner, F., Mattis, I., Janicka, L., Markowicz, K. M., Achtert, P., Artaxo, P., Pauliquevis, T., Souza, R. A. F., Sharma, V. P., van Zyl, P. G., Beukes, J. P., Sun, J., Rohwer, E. G., Deng, R., Mamouri, R.-E., and Zamorano, F.: An overview of the first decade of PollyNET: an emerging network of automated Raman-polarization lidars for continuous aerosol profiling, Atmos. Chem. Phys., 16, 5111–5137, https://doi.org/10.5194/acp-16-5111-2016, 2016.
Baars, H., Ansmann, A., Ohneiser, K., Haarig, M., Engelmann, R., Althausen, D., Hanssen, I., Gausa, M., Pietruczuk, A., Szkop, A., Stachlewska, I. S., Wang, D., Reichardt, J., Skupin, A., Mattis, I., Trickl, T., Vogelmann, H., Navas-Guzmán, F., Haefele, A., Acheson, K., Ruth, A. A., Tatarov, B., Müller, D., Hu, Q., Podvin, T., Goloub, P., Veselovskii, I., Pietras, C., Haeffelin, M., Fréville, P., Sicard, M., Comerón, A., Fernández García, A. J., Molero Menéndez, F., Córdoba-Jabonero, C., Guerrero-Rascado, J. L., Alados-Arboledas, L., Bortoli, D., Costa, M. J., Dionisi, D., Liberti, G. L., Wang, X., Sannino, A., Papagiannopoulos, N., Boselli, A., Mona, L., D'Amico, G., Romano, S., Perrone, M. R., Belegante, L., Nicolae, D., Grigorov, I., Gialitaki, A., Amiridis, V., Soupiona, O., Papayannis, A., Mamouri, R.-E., Nisantzi, A., Heese, B., Hofer, J., Schechner, Y. Y., Wandinger, U., and Pappalardo, G.: The unprecedented 2017–2018 stratospheric smoke event: decay phase and aerosol properties observed with the EARLINET, Atmos. Chem. Phys., 19, 15183–15198, https://doi.org/10.5194/acp-19-15183-2019, 2019.
Baars, H., Radenz, M., Floutsi, A. A., Engelmann, R., Althausen, D., Heese, B., Ansmann, A., Flament, T., Dabas, A., Trapon, D., Reitebuch, O., Bley, S., and Wandinger, U.: Californian Wildfire Smoke Over Europe: A First Example of the Aerosol Observing Capabilities of Aeolus Compared to Ground-Based Lidar, Geophys. Res. Lett., 48, e2020GL092194, https://doi.org/10.1029/2020GL092194, 2021.
Bedoya-Velásquez, A. E., Herreras-Giralda, M., Román, R., Wiegner, M., Lefebvre, S., Toledano, C., Huet, T., and Ceolato, R.: Ceilometer inversion method using water-vapor correction from co-located microwave radiometer for aerosol retrievals, Atmos. Res., 250, 105379, https://doi.org/10.1016/J.ATMOSRES.2020.105379, 2021.
Binietoglou, I., Amodeo, A., D'Amico, G., Giunta, A., Madonna, F., Mona, L., and Pappalardo, G.: Examination of possible synergy between lidar and ceilometer for the monitoring of atmospheric aerosols, Proc. SPIE, 8182, 818209, https://doi.org/10.1117/12.897530, 2011.
Bohlmann, S., Shang, X., Vakkari, V., Giannakaki, E., Leskinen, A., Lehtinen, K. E. J., Pätsi, S., and Komppula, M.: Lidar depolarization ratio of atmospheric pollen at multiple wavelengths, Atmos. Chem. Phys., 21, 7083–7097, https://doi.org/10.5194/acp-21-7083-2021, 2021.
Buchard, V., Randles, C. A., da Silva, A. M., Darmenov, A., Colarco, P. R., Govindaraju, R., Ferrare, R., Hair, J., Beyersdorf, A. J., Ziemba, L. D., and Yu, H.: The MERRA-2 aerosol reanalysis, 1980 onward. Part II: Evaluation and case studies, J. Climate, 30, 6851–6872, https://doi.org/10.1175/JCLI-D-16-0613.1, 2017.
Burton, S. P., Ferrare, R. A., Hostetler, C. A., Hair, J. W., Rogers, R. R., Obland, M. D., Butler, C. F., Cook, A. L., Harper, D. B., and Froyd, K. D.: Aerosol classification using airborne High Spectral Resolution Lidar measurements – methodology and examples, Atmos. Meas. Tech., 5, 73–98, https://doi.org/10.5194/amt-5-73-2012, 2012.
Cazorla, A., Casquero-Vera, J. A., Román, R., Guerrero-Rascado, J. L., Toledano, C., Cachorro, V. E., Orza, J. A. G., Cancillo, M. L., Serrano, A., Titos, G., Pandolfi, M., Alastuey, A., Hanrieder, N., and Alados-Arboledas, L.: Near-real-time processing of a ceilometer network assisted with sun-photometer data: monitoring a dust outbreak over the Iberian Peninsula, Atmos. Chem. Phys., 17, 11861–11876, https://doi.org/10.5194/acp-17-11861-2017, 2017.
Chen, J., Li, C., Ristovski, Z., Milic, A., Gu, Y., Islam, M. S., Wang, S., Hao, J., Zhang, H., He, C., Guo, H., Fu, H., Miljevic, B., Morawska, L., Thai, P., LAM, Y. F., Pereira, G., Ding, A., Huang, X., and Dumka, U. C.: A review of biomass burning: Emissions and impacts on air quality, health and climate in China, Sci. Total Environ., 579, 1000–1034, https://doi.org/10.1016/j.scitotenv.2016.11.025, 2017.
Dionisi, D., Barnaba, F., Diémoz, H., Di Liberto, L., and Gobbi, G. P.: A multiwavelength numerical model in support of quantitative retrievals of aerosol properties from automated lidar ceilometers and test applications for AOT and PM10 estimation, Atmos. Meas. Tech., 11, 6013–6042, https://doi.org/10.5194/amt-11-6013-2018, 2018.
Dubovik, O. and King, M. D.: A flexible inversion algorithm for retrieval of aerosol optical properties from Sun and sky radiance measurements, J. Geophys. Res.-Atmos., 105, 20673–20696, https://doi.org/10.1029/2000JD900282, 2000.
Dubovik, O., Smirnov, A., Holben, B. N., King, M. D., Kaufman, Y. J., Eck, T. F., and Slutsker, I.: Accuracy assessments of aerosol optical properties retrieved from Aerosol Robotic Network (AERONET) Sun and sky radiance measurements, J. Geophys. Res.-Atmos., 105, 9791–9806, https://doi.org/10.1029/2000JD900040, 2000.
Dubovik, O., Sinyuk, A., Lapyonok, T., Holben, B. N., Mishchenko, M., Yang, P., Eck, T. F., Volten, H., Muñoz, O., Veihelmann, B., van der Zande, W. J., Leon, J.-F., Sorokin, M., and Slutsker, I.: Application of spheroid models to account for aerosol particle nonsphericity in remote sensing of desert dust, J. Geophys. Res., 111, D11208, https://doi.org/10.1029/2005JD006619, 2006.
Eck, T. F., Holben, B. N., Reid, J. S., Dubovik, O., Smirnov, A., O'Neill, N. T., Slutsker, I., and Kinne, S.: Wavelength dependence of the optical depth of biomass burning, urban, and desert dust aerosols, J. Geophys. Res.-Atmos., 104, 31333–31349, https://doi.org/10.1029/1999JD900923, 1999.
Emeis, S., Forkel, R., Junkermann, W., Schäfer, K., Flentje, H., Gilge, S., Fricke, W., Wiegner, M., Freudenthaler, V., Groß, S., Ries, L., Meinhardt, F., Birmili, W., Münkel, C., Obleitner, F., and Suppan, P.: Measurement and simulation of the 16/17 April 2010 Eyjafjallajökull volcanic ash layer dispersion in the northern Alpine region, Atmos. Chem. Phys., 11, 2689–2701, https://doi.org/10.5194/acp-11-2689-2011, 2011.
Engelmann, R., Kanitz, T., Baars, H., Heese, B., Althausen, D., Skupin, A., Wandinger, U., Komppula, M., Stachlewska, I. S., Amiridis, V., Marinou, E., Mattis, I., Linné, H., and Ansmann, A.: The automated multiwavelength Raman polarization and water-vapor lidar PollyXT: the neXT generation, Atmos. Meas. Tech., 9, 1767–1784, https://doi.org/10.5194/amt-9-1767-2016, 2016.
Engelmann, R., Ansmann, A., Ohneiser, K., Griesche, H., Radenz, M., Hofer, J., Althausen, D., Dahlke, S., Maturilli, M., Veselovskii, I., Jimenez, C., Wiesen, R., Baars, H., Bühl, J., Gebauer, H., Haarig, M., Seifert, P., Wandinger, U., and Macke, A.: Wildfire smoke, Arctic haze, and aerosol effects on mixed-phase and cirrus clouds over the North Pole region during MOSAiC: an introduction, Atmos. Chem. Phys., 21, 13397–13423, https://doi.org/10.5194/acp-21-13397-2021, 2021.
Fernald, F. G.: Analysis of atmospheric lidar observations: some comments, Appl. Optics, 23, 652–653, 1984.
Fiebig, M., Petzold, A., Wandinger, U., Wendisch, M., Kiemle, C., Stifter, A., Ebert, M., Rother, T., and Leiterer, U.: Optical closure for an aerosol column: Method, accuracy, and inferable properties applied to a biomass-burning aerosol and its radiative forcing, J. Geophys. Res.-Atmos., 107, LAC 12-1–LAC 12-15, https://doi.org/10.1029/2000JD000192, 2002.
Fiebig, M., Stohl, A., Wendisch, M., Eckhardt, S., and Petzold, A.: Dependence of solar radiative forcing of forest fire aerosol on ageing and state of mixture, Atmos. Chem. Phys., 3, 881–891, https://doi.org/10.5194/acp-3-881-2003, 2003.
Filioglou, M., Nikandrova, A., Niemelä, S., Baars, H., Mielonen, T., Leskinen, A., Brus, D., Romakkaniemi, S., Giannakaki, E., and Komppula, M.: Profiling water vapor mixing ratios in Finland by means of a Raman lidar, a satellite and a model, Atmos. Meas. Tech., 10, 4303–4316, https://doi.org/10.5194/amt-10-4303-2017, 2017.
Flentje, H., Mattis, I., Kipling, Z., Rémy, S., and Thomas, W.: Evaluation of ECMWF IFS-AER (CAMS) operational forecasts during cycle 41r1–46r1 with calibrated ceilometer profiles over Germany, Geosci. Model Dev., 14, 1721–1751, https://doi.org/10.5194/gmd-14-1721-2021, 2021.
Fromm, M. D. and Servranckx, R.: Transport of forest fire smoke above the tropopause by supercell convection, Geophys. Res. Lett., 30, 1542, https://doi.org/10.1029/2002GL016820, 2003.
Gelaro, R., McCarty, W., Suárez, M. J., Todling, R., Molod, A., Takacs, L., Randles, C. A., Darmenov, A., Bosilovich, M. G., Reichle, R., Wargan, K., Coy, L., Cullather, R., Draper, C., Akella, S., Buchard, V., Conaty, A., da Silva, A. M., Gu, W., Kim, G. K., Koster, R., Lucchesi, R., Merkova, D., Nielsen, J. E., Partyka, G., Pawson, S., Putman, W., Rienecker, M., Schubert, S. D., Sienkiewicz, M., and Zhao, B.: The modern-era retrospective analysis for research and applications, version 2 (MERRA-2), J. Climate, 30, 5419–5454, https://doi.org/10.1175/JCLI-D-16-0758.1, 2017.
Generoso, S., Bréon, F.-M., Balkanski, Y., Boucher, O., and Schulz, M.: Improving the seasonal cycle and interannual variations of biomass burning aerosol sources, Atmos. Chem. Phys., 3, 1211–1222, https://doi.org/10.5194/acp-3-1211-2003, 2003.
Groß, S., Esselborn, M., Weinzierl, B., Wirth, M., Fix, A., and Petzold, A.: Aerosol classification by airborne high spectral resolution lidar observations, Atmos. Chem. Phys., 13, 2487–2505, https://doi.org/10.5194/acp-13-2487-2013, 2013.
Haarig, M., Engelmann, R., Ansmann, A., Veselovskii, I., Whiteman, D. N., and Althausen, D.: 1064 nm rotational Raman lidar for particle extinction and lidar-ratio profiling: cirrus case study, Atmos. Meas. Tech., 9, 4269–4278, https://doi.org/10.5194/amt-9-4269-2016, 2016.
Haarig, M., Ansmann, A., Baars, H., Jimenez, C., Veselovskii, I., Engelmann, R., and Althausen, D.: Depolarization and lidar ratios at 355, 532, and 1064 nm and microphysical properties of aged tropospheric and stratospheric Canadian wildfire smoke, Atmos. Chem. Phys., 18, 11847–11861, https://doi.org/10.5194/acp-18-11847-2018, 2018.
Hirsikko, A., O'Connor, E. J., Komppula, M., Korhonen, K., Pfüller, A., Giannakaki, E., Wood, C. R., Bauer-Pfundstein, M., Poikonen, A., Karppinen, T., Lonka, H., Kurri, M., Heinonen, J., Moisseev, D., Asmi, E., Aaltonen, V., Nordbo, A., Rodriguez, E., Lihavainen, H., Laaksonen, A., Lehtinen, K. E. J., Laurila, T., Petäjä, T., Kulmala, M., and Viisanen, Y.: Observing wind, aerosol particles, cloud and precipitation: Finland's new ground-based remote-sensing network, Atmos. Meas. Tech., 7, 1351–1375, https://doi.org/10.5194/amt-7-1351-2014, 2014.
Hu, Q., Goloub, P., Veselovskii, I., Bravo-Aranda, J.-A., Popovici, I. E., Podvin, T., Haeffelin, M., Lopatin, A., Dubovik, O., Pietras, C., Huang, X., Torres, B., and Chen, C.: Long-range-transported Canadian smoke plumes in the lower stratosphere over northern France, Atmos. Chem. Phys., 19, 1173–1193, https://doi.org/10.5194/acp-19-1173-2019, 2019.
Huff, A. K., Kondragunta, S., Zhang, H., Laszlo, I., Zhou, M., Caicedo, V., Delgado, R., and Levy, R.: Tracking Smoke from a Prescribed Fire and Its Impacts on Local Air Quality Using Temporally Resolved GOES-16 ABI Aerosol Optical Depth (AOD), J. Atmos. Ocean. Tech., 38, 963–976, https://doi.org/10.1175/JTECH-D-20-0162.1, 2021.
Illingworth, A. J., Barker, H. W., Beljaars, A., Ceccaldi, M., Chepfer, H., Clerbaux, N., Cole, J., Delanoë, J., Domenech, C., Donovan, D. P., Fukuda, S., Hirakata, M., Hogan, R. J., Huenerbein, A., Kollias, P., Kubota, T., Nakajima, T., Nakajima, T. Y., Nishizawa, T., Ohno, Y., Okamoto, H., Oki, R., Sato, K., Satoh, M., Shephard, M. W., Velázquez-Blázquez, A., Wandinger, U., Wehr, T., and van Zadelhoff, G.-J.: The EarthCARE Satellite: The Next Step Forward in Global Measurements of Clouds, Aerosols, Precipitation, and Radiation, B. Am. Meteorol. Soc., 96, 1311–1332, https://doi.org/10.1175/BAMS-D-12-00227.1, 2015.
IPCC: Climate Change 2013: The Physical Science Basis. Contribution of Working Group I to the Fifth Assessment Report of the Intergovernmental Panel on Climate Change, edited by: Stocker, T. F., Qin, D., Plattner, G.-K., Tignor, M., Allen, S. K., Boschung, J., Nauels, A., Xia, Y., Bex, V., and Midgley, P. M., Cambridge University Press, Cambridge, United Kingdom and New York, NY, USA, 2013.
Janicka, L., Stachlewska, I. S., Veselovskii, I., and Baars, H.: Temporal variations in optical and microphysical properties of mineral dust and biomass burning aerosol derived from daytime Raman lidar observations over Warsaw, Poland, Atmos. Environ., 169, 162–174, https://doi.org/10.1016/j.atmosenv.2017.09.022, 2017.
Khaykin, S. M., Godin-Beekmann, S., Hauchecorne, A., Pelon, J., Ravetta, F., and Keckhut, P.: Stratospheric Smoke With Unprecedentedly High Backscatter Observed by Lidars Above Southern France, Geophys. Res. Lett., 45, 1639–1646, https://doi.org/10.1002/2017GL076763, 2018.
Kim, S.-W., Chazette, P., Dulac, F., Sanak, J., Johnson, B., and Yoon, S.-C.: Vertical structure of aerosols and water vapor over West Africa during the African monsoon dry season, Atmos. Chem. Phys., 9, 8017–8038, https://doi.org/10.5194/acp-9-8017-2009, 2009.
Klett, J. D.: Stable analytical solution for processing lidar returns, Appl. Optics, 20, 211–220, 1981.
Kotthaus, S., O'Connor, E., Münkel, C., Charlton-Perez, C., Haeffelin, M., Gabey, A. M., and Grimmond, C. S. B.: Recommendations for processing atmospheric attenuated backscatter profiles from Vaisala CL31 ceilometers, Atmos. Meas. Tech., 9, 3769–3791, https://doi.org/10.5194/amt-9-3769-2016, 2016.
Li, C., Hu, Y., Chen, J., Ma, Z., Ye, X., Yang, X., Wang, L., Wang, X., and Mellouki, A.: Physiochemical properties of carbonaceous aerosol from agricultural residue burning: Density, volatility, and hygroscopicity, Atmos. Environ., 140, 94–105, https://doi.org/10.1016/j.atmosenv.2016.05.052, 2016.
Mamali, D., Marinou, E., Sciare, J., Pikridas, M., Kokkalis, P., Kottas, M., Binietoglou, I., Tsekeri, A., Keleshis, C., Engelmann, R., Baars, H., Ansmann, A., Amiridis, V., Russchenberg, H., and Biskos, G.: Vertical profiles of aerosol mass concentration derived by unmanned airborne in situ and remote sensing instruments during dust events, Atmos. Meas. Tech., 11, 2897–2910, https://doi.org/10.5194/amt-11-2897-2018, 2018.
Mamouri, R. E. and Ansmann, A.: Fine and coarse dust separation with polarization lidar, Atmos. Meas. Tech., 7, 3717–3735, https://doi.org/10.5194/amt-7-3717-2014, 2014.
Mamouri, R.-E. and Ansmann, A.: Potential of polarization/Raman lidar to separate fine dust, coarse dust, maritime, and anthropogenic aerosol profiles, Atmos. Meas. Tech., 10, 3403–3427, https://doi.org/10.5194/amt-10-3403-2017, 2017.
Mielonen, T., Portin, H., Komppula, M., Leskinen, A., Tamminen, J., Ialongo, I., Hakkarainen, J., Lehtinen, K. E. J., and Arola, A.: Biomass burning aerosols observed in Eastern Finland during the Russian wildfires in summer 2010 – Part 2: Remote sensing, Atmos. Environ., 47, 279–287, https://doi.org/10.1016/j.atmosenv.2011.07.016, 2012.
Mielonen, T., Aaltonen, V., Lihavainen, H., Hyvärinen, A.-P., Arola, A., Komppula, M., and Kivi, R.: Biomass Burning Aerosols Observed in Northern Finland during the 2010 Wildfires in Russia, Atmosphere, 4, 17–34, https://doi.org/10.3390/ATMOS4010017, 2013.
MODIS: MODIS Collection 6 NRT Hotspot/Active Fire Detections MCD14DL, Earthdata [data set], https://doi.org/10.5067/FIRMS/MODIS/MCD14DL.NRT.006, 2019.
Müller, D., Wandinger, U., and Ansmann, A.: Microphysical particle parameters from extinction and backscatter lidar data by inversion with regularization: theory, Appl. Optics, 38, 2346–2357, https://doi.org/10.1364/AO.38.002346, 1999.
Müller, D., Mattis, I., Wandinger, U., Ansmann, A., Althausen, D., and Stohl, A.: Raman lidar observations of aged Siberian and Canadian forest fire smoke in the free troposphere over Germany in 2003: Microphysical particle characterization, J. Geophys. Res., 110, D17201, https://doi.org/10.1029/2004JD005756, 2005.
Müller, D., Ansmann, A., Mattis, I., Tesche, M., Wandinger, U., Althausen, D., and Pisani, G.: Aerosol–type–dependent lidar ratios observed with Raman lidar, J. Geophys. Res.-Atmos., 112, D16202, https://doi.org/10.1029/2006JD008292, 2007.
Murayama, T., Müller, D., Wada, K., Shimizu, A., Sekiguchi, M., and Tsukamoto, T.: Characterization of Asian dust and Siberian smoke with multi-wavelength Raman lidar over Tokyo, Japan in spring 2003, Geophys. Res. Lett., 31, 1–5, https://doi.org/10.1029/2004GL021105, 2004.
Nepomuceno Pereira, S., Preißler, J., Guerrero-Rascado, J. L., Silva, A. M., and Wagner, F.: Forest fire smoke layers observed in the free troposphere over Portugal with a multiwavelength Raman lidar: Optical and microphysical properties, Sci. World J., 2014, 421838, https://doi.org/10.1155/2014/421838, 2014.
Nicolae, D., Nemuc, A., Müller, D., Talianu, C., Vasilescu, J., Belegante, L., and Kolgotin, A.: Characterization of fresh and aged biomass burning events using multiwavelength Raman lidar and mass spectrometry, J. Geophys. Res.-Atmos., 118, 2956–2965, https://doi.org/10.1002/jgrd.50324, 2013.
O'Connor, E. J., Illingworth, A. J., and Hogan, R. J.: A technique for autocalibration of cloud lidar, J. Atmos. Ocean. Tech., 21, 777–786, https://doi.org/10.1175/1520-0426(2004)021<0777:ATFAOC>2.0.CO;2, 2004.
O'Neill, N. T.: Spectral discrimination of coarse and fine mode optical depth, J. Geophys. Res., 108, 4559, https://doi.org/10.1029/2002JD002975, 2003.
Ohneiser, K., Ansmann, A., Baars, H., Seifert, P., Barja, B., Jimenez, C., Radenz, M., Teisseire, A., Floutsi, A., Haarig, M., Foth, A., Chudnovsky, A., Engelmann, R., Zamorano, F., Bühl, J., and Wandinger, U.: Smoke of extreme Australian bushfires observed in the stratosphere over Punta Arenas, Chile, in January 2020: optical thickness, lidar ratios, and depolarization ratios at 355 and 532 nm, Atmos. Chem. Phys., 20, 8003–8015, https://doi.org/10.5194/acp-20-8003-2020, 2020.
Ohneiser, K., Ansmann, A., Engelmann, R., Ritter, C., Chudnovsky, A., Veselovskii, I., Baars, H., Gebauer, H., Griesche, H., Radenz, M., Hofer, J., Althausen, D., Dahlke, S., and Maturilli, M.: Siberian fire smoke in the High-Arctic winter stratosphere observed during MOSAiC 2019–2020, Atmos. Chem. Phys. Discuss. [preprint], https://doi.org/10.5194/acp-2021-117, in review, 2021.
Ortiz-Amezcua, P., Guerrero-Rascado, J. L., Granados-Muñoz, M. J., Benavent-Oltra, J. A., Böckmann, C., Samaras, S., Stachlewska, I. S., Janicka, L., Baars, H., Bohlmann, S., and Alados-Arboledas, L.: Microphysical characterization of long-range transported biomass burning particles from North America at three EARLINET stations, Atmos. Chem. Phys., 17, 5931–5946, https://doi.org/10.5194/acp-17-5931-2017, 2017.
Osborne, M., Malavelle, F. F., Adam, M., Buxmann, J., Sugier, J., Marenco, F., and Haywood, J.: Saharan dust and biomass burning aerosols during ex-hurricane Ophelia: observations from the new UK lidar and sun-photometer network, Atmos. Chem. Phys., 19, 3557–3578, https://doi.org/10.5194/acp-19-3557-2019, 2019.
Pappalardo, G., Amodeo, A., Apituley, A., Comeron, A., Freudenthaler, V., Linné, H., Ansmann, A., Bösenberg, J., D'Amico, G., Mattis, I., Mona, L., Wandinger, U., Amiridis, V., Alados-Arboledas, L., Nicolae, D., and Wiegner, M.: EARLINET: towards an advanced sustainable European aerosol lidar network, Atmos. Meas. Tech., 7, 2389–2409, https://doi.org/10.5194/amt-7-2389-2014, 2014.
Pereira, S. N., Preißler, J., Guerrero-Rascado, J. L., Silva, A. M., and Wagner, F.: Forest fire smoke layers observed in the free troposphere over Portugal with a multiwavelength Raman lidar: optical and microphysical properties., Sci. World J., 2014, 421838, https://doi.org/10.1155/2014/421838, 2014.
Portin, H., Mielonen, T., Leskinen, A., Arola, A., Pärjälä, E., Romakkaniemi, S., Laaksonen, A., Lehtinen, K. E. J., and Komppula, M.: Biomass burning aerosols observed in Eastern Finland during the Russian wildfires in summer 2010 – Part 1: In-situ aerosol characterization, Atmos. Environ., 47, 269–278, https://doi.org/10.1016/j.atmosenv.2011.10.067, 2012.
Prenni, A. J., DeMott, P. J., Sullivan, A. P., Sullivan, R. C., Kreidenweis, S. M., and Rogers, D. C.: Biomass burning as a potential source for atmospheric ice nuclei: Western wildfires and prescribed burns, Geophys. Res. Lett., 39, L11805, https://doi.org/10.1029/2012GL051915, 2012.
Randles, C. A., da Silva, A. M., Buchard, V., Colarco, P. R., Darmenov, A., Govindaraju, R., Smirnov, A., Holben, B., Ferrare, R., Hair, J., Shinozuka, Y., and Flynn, C. J.: The MERRA-2 aerosol reanalysis, 1980 onward. Part I: System description and data assimilation evaluation, J. Climate, 30, 6823–6850, https://doi.org/10.1175/JCLI-D-16-0609.1, 2017.
Reid, J. S., Koppmann, R., Eck, T. F., and Eleuterio, D. P.: A review of biomass burning emissions part II: intensive physical properties of biomass burning particles, Atmos. Chem. Phys., 5, 799–825, https://doi.org/10.5194/acp-5-799-2005, 2005a.
Reid, J. S., Eck, T. F., Christopher, S. A., Koppmann, R., Dubovik, O., Eleuterio, D. P., Holben, B. N., Reid, E. A., and Zhang, J.: A review of biomass burning emissions part III: intensive optical properties of biomass burning particles, Atmos. Chem. Phys., 5, 827–849, https://doi.org/10.5194/acp-5-827-2005, 2005b.
Rothman, L. S., Jacquemart, D., Barbe, A., Benner, D. C., Birk, M., Brown, L. R., Carleer, M. R., Chackerian, C., Chance, K., Coudert, L. H., Dana, V., Devi, V. M., Flaud, J. M., Gamache, R. R., Goldman, A., Hartmann, J. M., Jucks, K. W., Maki, A. G., Mandin, J. Y., Massie, S. T., Orphal, J., Perrin, A., Rinsland, C. P., Smith, M. A. H., Tennyson, J., Tolchenov, R. N., Toth, R. A., Vander Auwera, J., Varanasi, P., and Wagner, G.: The HITRAN 2004 molecular spectroscopic database, J. Quant. Spectrosc. Ra., 96, 139–204, https://doi.org/10.1016/j.jqsrt.2004.10.008, 2005.
Sakai, T., Nagai, T., Zaizen, Y., and Mano, Y.: Backscattering linear depolarization ratio measurements of mineral, sea-salt, and ammonium sulfate particles simulated in a laboratory chamber, Appl. Optics, 49, 4441–4449, https://doi.org/10.1364/AO.49.004441, 2010.
Schumann, U., Weinzierl, B., Reitebuch, O., Schlager, H., Minikin, A., Forster, C., Baumann, R., Sailer, T., Graf, K., Mannstein, H., Voigt, C., Rahm, S., Simmet, R., Scheibe, M., Lichtenstern, M., Stock, P., Rüba, H., Schäuble, D., Tafferner, A., Rautenhaus, M., Gerz, T., Ziereis, H., Krautstrunk, M., Mallaun, C., Gayet, J.-F., Lieke, K., Kandler, K., Ebert, M., Weinbruch, S., Stohl, A., Gasteiger, J., Groß, S., Freudenthaler, V., Wiegner, M., Ansmann, A., Tesche, M., Olafsson, H., and Sturm, K.: Airborne observations of the Eyjafjalla volcano ash cloud over Europe during air space closure in April and May 2010, Atmos. Chem. Phys., 11, 2245–2279, https://doi.org/10.5194/acp-11-2245-2011, 2011.
Stoffelen, A., Pailleux, J., Källén, E., Vaughan, J. M., Isaksen, L., Flamant, P., Wergen, W., Andersson, E., Schyberg, H., Culoma, A., Meynart, R., Endemann, M., and Ingmann, P.: The Atmospheric Dynamics Mission for Global Wind Field Measurement, B. Am. Meteorol. Soc., 86, 73–88, https://doi.org/10.1175/BAMS-86-1-73, 2005.
Tesche, M., Ansmann, A., Müller, D., Althausen, D., Engelmann, R., Freudenthaler, V., and Groß, S.: Vertically resolved separation of dust and smoke over Cape Verde using multiwavelength Raman and polarization lidars during Saharan Mineral Dust Experiment 2008, J. Geophys. Res., 114, D13202, https://doi.org/10.1029/2009JD011862, 2009.
Trickl, T., Vogelmann, H., Flentje, H., and Ries, L.: Stratospheric ozone in boreal fire plumes – the 2013 smoke season over central Europe, Atmos. Chem. Phys., 15, 9631–9649, https://doi.org/10.5194/acp-15-9631-2015, 2015.
Tsaknakis, G., Papayannis, A., Kokkalis, P., Amiridis, V., Kambezidis, H. D., Mamouri, R. E., Georgoussis, G., and Avdikos, G.: Inter-comparison of lidar and ceilometer retrievals for aerosol and Planetary Boundary Layer profiling over Athens, Greece, Atmos. Meas. Tech., 4, 1261–1273, https://doi.org/10.5194/amt-4-1261-2011, 2011.
Vaughan, G., Draude, A. P., Ricketts, H. M. A., Schultz, D. M., Adam, M., Sugier, J., and Wareing, D. P.: Transport of Canadian forest fire smoke over the UK as observed by lidar, Atmos. Chem. Phys., 18, 11375–11388, https://doi.org/10.5194/acp-18-11375-2018, 2018.
Wandinger, U., Müller, D., Böckmann, C., Althausen, D., Matthias, V., Bösenberg, J., Weiß, V., Fiebig, M., Wendisch, M., Stohl, A., and Ansmann, A.: Optical and microphysical characterization of biomass-burning and industrial-pollution aerosols from multiwavelength lidar and aircraft measurements, J. Geophys. Res.-Atmos., 107, 8125, https://doi.org/10.1029/2000JD000202, 2002.
Welton, E. J., Campbell, J. R., Spinhirne, J. D., and Scott III, V. S.: Global monitoring of clouds and aerosols using a network of micropulse lidar systems, Proc. SPIE, 4153, 151, https://doi.org/10.1117/12.417040, 2001.
Wiegner, M. and Gasteiger, J.: Correction of water vapor absorption for aerosol remote sensing with ceilometers, Atmos. Meas. Tech., 8, 3971–3984, https://doi.org/10.5194/amt-8-3971-2015, 2015.
Wiegner, M. and Geiß, A.: Aerosol profiling with the Jenoptik ceilometer CHM15kx, Atmos. Meas. Tech., 5, 1953–1964, https://doi.org/10.5194/amt-5-1953-2012, 2012.
Wiegner, M., Madonna, F., Binietoglou, I., Forkel, R., Gasteiger, J., Geiß, A., Pappalardo, G., Schäfer, K., and Thomas, W.: What is the benefit of ceilometers for aerosol remote sensing? An answer from EARLINET, Atmos. Meas. Tech., 7, 1979–1997, https://doi.org/10.5194/amt-7-1979-2014, 2014.
Wiegner, M., Mattis, I., Pattantyús-Ábrahám, M., Bravo-Aranda, J. A., Poltera, Y., Haefele, A., Hervo, M., Görsdorf, U., Leinweber, R., Gasteiger, J., Haeffelin, M., Wagner, F., Cermak, J., Komínková, K., Brettle, M., Münkel, C., and Pönitz, K.: Aerosol backscatter profiles from ceilometers: validation of water vapor correction in the framework of CeiLinEx2015, Atmos. Meas. Tech., 12, 471–490, https://doi.org/10.5194/amt-12-471-2019, 2019.
Winker, D. M., Vaughan, M. A., Omar, A., Hu, Y., Powell, K. A., Liu, Z., Hunt, W. H., and Young, S. A.: Overview of the CALIPSO mission and CALIOP data processing algorithms, J. Atmos. Ocean. Tech., 26, 2310, https://doi.org/10.1175/2009JTECHA1281.1, 2009.
Wotawa, G., Novelli, P. C., Trainer, M., and Granier, C.: Inter-annual variability of summertime CO concentrations in the Northern Hemisphere explained by boreal forest fires in North America and Russia, Geophys. Res. Lett., 28, 4575–4578, https://doi.org/10.1029/2001GL013686, 2001.
Yu, S.: Role of organic acids (formic, acetic, pyruvic and oxalic) in the formation of cloud condensation nuclei (CCN): A review, Atmos. Res., 53, 185–217, https://doi.org/10.1016/S0169-8095(00)00037-5, 2000.