the Creative Commons Attribution 4.0 License.
the Creative Commons Attribution 4.0 License.
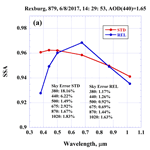
Employing relaxed smoothness constraints on imaginary part of refractive index in AERONET aerosol retrieval algorithm
Alexander Sinyuk
Brent N. Holben
Thomas F. Eck
David M. Giles
Ilya Slutsker
Oleg Dubovik
Joel S. Schafer
Alexander Smirnov
Mikhail Sorokin
In the Aerosol Robotic Network (AERONET) retrieval algorithm, smoothness constraints on the imaginary part of the refractive index provide control of retrieved spectral dependence of aerosol absorption by preventing the inversion code from fitting the noise in optical measurements and thus avoiding unrealistic oscillations of retrievals with wavelength. The history of implementation of the smoothness constraints in the AERONET retrieval algorithm is discussed. It is shown that the latest version of the smoothness constraints on the imaginary part of refractive index, termed standard and employed by Version 3 of the retrieval algorithm, should be modified to account for strong variability of light absorption by brown-carbon-containing aerosols in UV through mid-visible parts of the solar spectrum. In Version 3 strong spectral constraints were imposed at high values of the Ångström exponent (440–870 nm) since black carbon was assumed to be the primary absorber, while the constraints became increasingly relaxed as aerosol exponent deceased to allow for wavelength dependence of absorption for dust aerosols. The new version of the smoothness constraints on the imaginary part of the refractive index assigns different weights to different pairs of wavelengths, which are the same for all values of the Ångström exponent. For example, in the case of four-wavelength input, the weights assigned to short-wavelength pairs (440–675, 675–870 nm) are small so that smoothness constraints do not suppress natural spectral variability of the imaginary part of the refractive index. At longer wavelengths (870–1020 nm), however, the weight is 10 times higher to provide additional constraints on the imaginary part of refractive index retrievals of aerosols with a high Ångström exponent due to low sensitivity to aerosol absorption for longer channels at relatively low aerosol optical depths. The effect of applying the new version of smoothness constraints, termed relaxed, on retrievals of single-scattering albedo is analyzed for case studies of different aerosol types: black- and brown-carbon-containing fine mode aerosols, mineral dust coarse mode aerosols, and urban industrial fine mode aerosol. It is shown that for brown-carbon-containing aerosols employing the relaxed smoothness constraints resulted in significant reduction in retrieved single-scattering albedo and spectral residual errors (compared to standard) at the short wavelengths. For example, biomass burning smoke cases showed a reduction in single-scattering albedo and spectral residual error at 380 nm of ∼ 0.033 and ∼ 17 %, respectively, for the Rexburg site and ∼ 0.04 and ∼ 12.7 % for the Rimrock site, both AERONET sites in Idaho, USA. For a site with very high levels of black-carbon-containing aerosols (Mongu, Zambia), the effect of modification in the smoothness constraints was minor. For mineral dust aerosols at small Ångström exponent values (Mezaira site, UAE), the spectral constraint on the imaginary part of the refractive index was already relaxed in Version 3; therefore the new relaxed constraint results in minimal change. In the case of weakly absorbing urban industrial aerosols at the GSFC site, there are significant changes in retrieved single-scattering albedo using relaxed assumption, especially reductions at longer wavelengths: ∼ 0.016 and ∼ 0.02 at 875 and 1020 nm, respectively, for 440 nm aerosol optical depth (AOD) ∼ 0.3. The modification of smoothness constraints on the imaginary part of the refractive index has a minor effect on retrievals of other aerosol parameters such as the real part of the refractive index and parameters of the aerosol size distribution. The implementation of the relaxed smoothness constraints on the imaginary part of the refractive index in the next version of the AERONET inversion algorithm will produce significant impacts at some sites in short wavelength channels (380 and 440 nm) for some biomass burning smoke cases with significant brown carbon content and possibly in mid-visible channels (500 and 675 nm) to near-infrared channels (870 to 1020 nm) for some urban industrial aerosol types. However, most differences in single-scattering albedo retrievals between those applying the new relaxed constraint and the standard constraint will be within the uncertainty of the single-scattering albedo retrievals, depending on the level of aerosol optical depth, Ångström exponent, brown carbon content and wavelength.
- Article
(4946 KB) - Full-text XML
- BibTeX
- EndNote
The Aerosol Robotic Network (AERONET) (Holben et al., 1998) of globally distributed ground-based sun–sky radiometers provides measurements of total atmospheric column spectral aerosol optical depth (AOD; see the list of abbreviations in Appendix A) and inversion algorithm retrievals of column-integrated aerosol particle size distribution (PSD) and complex index of refraction (CIR). The AOD measurements have been frequently utilized for satellite validation purposes (Sayer et al., 2018, 2019; Levy et al., 2013, 2015; Holzer-Popp et al., 2013; Lyapustin et al., 2018; Kahn et al., 2010; Limbacher and Kahn, 2019; Ahn et al., 2014; Choi et al., 2018) plus as input to the inversion algorithm along with directional sky radiances over a range of scattering angles. The AOD is measured at high accuracy (Eck et al., 1999; ∼ 0.01 in visible and near infrared and ∼ 0.02 in the UV), thereby providing a strong bound on the inversion results, especially since the sky radiance uncertainty combined with extraterrestrial flux uncertainty is ∼ 5 % (Sinyuk et al., 2020). The retrieved aerosol parameters are often used in development of satellite retrieval algorithms which must sometimes assume some aerosol optical and physical properties (Remer et al., 2005; Lyapustin et al., 2018). The retrievals of aerosol parameters are performed by the AERONET aerosol inversion algorithm which was developed by Dubovik and King (2000) and refined by Dubovik et al. (2006) with the addition of non-spherical (spheroidal) scattering. The latest version of the algorithm employed in AERONET Version 3 (V3) is described in detail in Sinyuk et al. (2020). The data quality assurance procedures and cloud screening of AOD in V3 are presented in Giles et al. (2019). The standard AERONET aerosol retrieval product is obtained by inverting measurements taken at four standard wavelengths: 440, 675, 875 and 1020 nm. However, the V3 aerosol retrieval algorithm can invert extended sets of wavelengths including the UV at 380 nm (Sinyuk et al., 2020). Absorption at 380 nm is particularly important as this is the wavelength range in which satellite observations and algorithms are able to retrieve atmospheric column absorption from existing (Jethva et al., 2014) and future satellite sensors (Werdell at al., 2019). The unique utility of measurements in UV for satellite remote sensing is related to increased sensitivity to aerosol absorption due to absorption of molecular scattering by aerosols (e.g., Torres et al., 1998). In addition to PSD parameters and spectral CIR, which are directly retrieved by the aerosol retrieval algorithm, other aerosol characteristics such as single-scattering albedo (SSA), absorption optical depth, asymmetry parameter, lidar and depolarization ratios are calculated from the retrieved aerosol parameters.
In general, inverted measurements have limited information content, resulting in non-uniqueness of the solution and high sensitivity to random measurement errors. In the worst-case scenario, an inversion fits the measurement noise (overfitting of the data), causing non-realistic oscillation in retrieved functions (e.g., Twomey, 1977). To prevent overfitting of the data, additional constraints on the retrieved functions should be imposed. These constraints usually restrict the norm of the solution or the norm of its derivatives (e.g., Dubovik, 2004; Aster et al., 2013) and are called smoothness constraints. The strength of the smoothness constraints should be selected in an optimal way as to prevent non-uniqueness of solution and overfitting of the measurements and yet not to overconstrain and suppress the natural variability of retrieved functions. In addition, stronger smoothness constraints should be optimally imposed at those ranges of solution variability which are not sufficiently constrained by the measurements. For example, for high-Ångström-exponent (AE) aerosols (fine-mode-dominated) measurement sensitivity to the imaginary part of the refractive index (IPRI) at longer wavelengths is limited due to low AOD at these wavelengths. The AERONET aerosol retrieval algorithm employs two types of smoothness constraints: (1) size dependence of PSD is constrained by restricting the norm of the third derivatives, and (2) the spectral dependencies of the real and imaginary parts of the refractive index are constrained by restricting that of the first derivatives (Dubovik and King, 2000).
The IPRI is one of the key retrieved aerosol parameters largely defining SSA which plays the central role in estimation of aerosol radiative forcing and atmospheric heating (Haywood and Boucher, 2000; Jacobson, 2001; Bond et al., 2013; Myhre et al., 2013). The optimal selection of the IPRI smoothness constraints and its effect on retrieved aerosol parameters is the subject of this paper. Throughout the development of the AERONET aerosol retrieval algorithm different implementations of the IPRI smoothness constraint were employed. The latest V3 implementation imposes weak smoothness constraints on the spectral dependence of the IPRI for low-AE aerosols and strong smoothness constraints for high-AE aerosols with linear interpolation on AE between these two cases. The first assumption works reasonably well for coarse-mode-dominated mineral dust aerosols which exhibit high IPRI spectral variability in UV to mid-visible parts of the solar spectrum, and the second assumption is suitable for aerosols whose chemical composition is dominated by black carbon (BC) exhibiting a spectrally flat imaginary index of refraction (Bond and Bergstrom, 2006; Kirchstetter et al., 2004). However, for high-AE aerosols containing brown carbon (BrC), the assumption of the strong IPRI smoothness constraints is not always suitable due to possible strong spectral variability of absorption by these aerosols at short wavelengths (mid-visible through the UV) (e.g., Kirchstetter and Thatcher, 2012).
This paper describes the modification of V3 assumptions on the IPRI smoothness constraints (standard, STD) to accommodate a priori information on spectral variability of the IPRI for different aerosol types. Section 2 discusses the history of implementation of the IPRI smoothness constraints in the AERONET aerosol retrieval algorithm and presents the new implementation, which is termed relaxed smoothness constraints (REL). In Sect. 3 we describe the effect of the REL smoothness constraints on SSA retrievals by analyzing case studies for different aerosol types. Comparisons of aerosol parameters retrieved by the AERONET aerosol retrieval algorithm using both REL and STD assumptions on the IPRI smoothness constraints are presented in Sect. 4. Section 5 shows examples of comparison of both REL and STD SSA retrievals to SSA derived from in situ measurements. The summary and conclusions are presented in Sect. 6.
The AERONET aerosol retrieval algorithm, like that of GRASP (Dubovik et al., 2011, 2021), is based on the multi-term least-squares method (LMS) approach, which was developed over the years by Oleg Dubovik with co-authors (Dubovik et al., 1995; Dubovik and King, 2000; Dubovik, 2004; Dubovik et al., 2011, 2021). The multi-term LSM concept allows flexible incorporation of very general a priori constraints on retrieved parameters, with emphasis on smoothness constraints in particular. Smoothness constraints limit variability of retrieved functions such as aerosol size distribution and spectral dependence of refractive index by using a priori information on their derivatives. From a formal point of view (e.g.,, Dubovik et al., 2021), the smoothness constraints are related to the limited values of derivatives of retrieved functions, i. e. with deviations of their mth derivatives from zero:
For the vector of unknowns , which is a discrete representation of continuous function f, the condition (1) can be formulated as a linear system of equations
where Gm is the Jacobian matrix of mth derivatives, which in the discrete case is approximated by the matrix of mth finite differences estimated at point a. 0∗ is the zero vector, representing the fact that a priori estimates of the corresponding derivatives are equal to zero. are the errors reflecting uncertainty in the knowledge of the deviation of the retrieved function f from the assumed simple functions such as constant (m = 1), straight line (m = 2), parabola (m = 3), etc. Under the assumption that the values in Eq. (2) are normally distributed with covariance matrix Cg, smoothness constraints can be easily included in the general framework of the multi-term LMS technique to obtain the iterative solution for vector a. For the case of a standard set of AERONET observations (spectral AOD, spectral sky radiances) and retrieved aerosol parameters (PSD, CIR), the solution is as follows:
In Eq. (3) , where represents vectors of measurements of AOD (k = 1) and sky radiances (k = 2), and represents the measurements fit at the pth iteration. k = 1, 2 represents Jacobian matrices at the pth iteration of the function fk in the vicinity of ap, and Wk denotes weighting matrices related to corresponding covariance matrices Ck by
where εk is the first diagonal element of Ck, and represents Lagrange multipliers for different types of measurements and represents Lagrange multiplier for smoothness constraints for size distribution (n = 1) and real (n = 2) and imaginary parts (n = 3) of the complex index of refraction. Jacobian matrices are the matrices of the first derivatives of the measurements with respect to retrieved parameters, and covariance matrices are diagonal matrices with elements equal to the accuracy (variances) of the measurements and/or a priori estimates. Ωn denotes smoothness matrices and is defined as
The values of Lagrange multipliers in Eq. (3) determine the strength of the corresponding smoothness constraints. Their selection is described in detail in Dubovik and King (2000) and Dubovik (2004), where it is shown that theoretical values of the Lagrange multiplier can be expressed as a function of the norm of the mth derivatives. In practice, however, it was found both convenient and justifiable to determine the values of the Lagrange multiplier for the smoothness constraints empirically based on external information on the variability of the retrieved functions. For example, if external information suggests weak variability of the refractive index with wavelength, the value of the Lagrange multiplier can be adjusted to force spectral dependence of the refractive index to be spectrally flat. From other hand, if substantial spectral variability is expected, the strength of the smoothness constraint should be relaxed.
The primary goal of smoothness constraints on the IPRI is to provide control of retrieved spectral dependence of aerosol absorption by preventing an excessive destabilizing effect of measurement noise on the solution and thus avoiding unrealistic oscillations of retrievals with wavelength. Also, they help in stabilizing retrievals of absorption of radiation for high-AE aerosols at long wavelengths by constraining its spectral dependence based on a priori information. The long wavelengths in these high-AE cases often have AOD that is too low to contain sufficient information content on particle absorption.
The AERONET aerosol retrieval algorithm employs constraints of the first derivatives of the IPRI wavelength dependence (m = 1) in which case these constraints relate the values of the IPRI at neighboring pairs of wavelengths. Throughout the course of algorithm development, different approximations were used for Lagrange multipliers while assuming equal weights to all the pairs of wavelengths and thus replacing weighing matrices Wm,n with unit matrices. In version 1 (V1) of the AERONET database, it was assumed that the IPRI for all the aerosol types is spectrally flat. This was physically justified by the flat spectral dependence of the principal fine mode aerosol absorber, black carbon (BC), in this wavelength range. Therefore, the strong smoothness constraints were used with the value of equal to 10−1. In AERONET versions 2 and 3 (V2 and V3) it was realized that such strong smoothness constraints suppress the natural spectral variability of absorption of dust aerosols (low AE), especially for the short wavelength visible (440 nm), but still assumed flat spectral independence of the IPRI for fine mode aerosols. The Lagrange parameter was linearly interpolated by AE (440–870 nm) between “pure” dust (AE = 0.001, ) and “pure” fine mode aerosol (AE = 2.5, ).
After incorporating the vector radiative transfer model SORD (Korkin et al., 2017) in the V3 aerosol retrieval algorithm (Sinyuk et al., 2020), it became possible to invert an extended set of wavelengths including in the near UV (380 nm). In this case however, the assumption on spectral independence of the IPRI for fine mode aerosols might not be very realistic for the high-AE aerosols containing BrC with strong UV absorption (e.g., Mok et al., 2016). Therefore, the V3 assumption for the IPRI smoothness constraints should be modified by relaxing its strength at short wavelengths but keeping larger values at longer wavelengths where measurement signal is low, thus making strength of the smoothness constraints spectrally dependent. This can be accomplished by adjusting the elements of the weight matrix in Eq. (5) by using smaller weights for short-wavelength pairs and the larger ones for those of longer wavelengths.
In the new assumption of the IPRI smoothness constraints, which we term “relaxed smoothness constraints” (REL), the value of in Eq. (3) is kept the same as for the pure dust in V2 and V3 (10−6). The diagonal elements of the weighting matrix are equal to 1 for wavelength pairs, which, for example, in the case of six-wavelength input are 380–440, 440–500, 500–675 and 675–870 nm. However, for the 870–1020 nm pair, the diagonal element of the weighting matrix is equal to 10, thus making the effective value of . The performance of this assumption is tested for different aerosol types in the following sections.
In this section, the effect of incorporating the relaxed smoothness constraint in the AERONET aerosol retrieval algorithm on SSA retrievals is analyzed for different aerosol types: brown- and black-carbon-containing aerosols, desert dust, and urban industrial aerosols.
3.1 Aerosols containing brown and black carbon
The light-absorbing aerosols that are produced from combustion (e.g., from biomass burning and fossil fuels) are typically classified as black carbon or brown carbon (e.g., Adler et al., 2019; Bond and Bergstrom, 2006). BC absorbs radiation across the entire UV–near-infrared spectrum, exhibiting little to no spectral selectivity (the IPRI is spectrally flat). BrC possess a strong wavelength-dependent absorption that peaks in the UV spectral region and declines though the visible part of the spectrum (e.g., Kirchstetter and Thatcher, 2012).
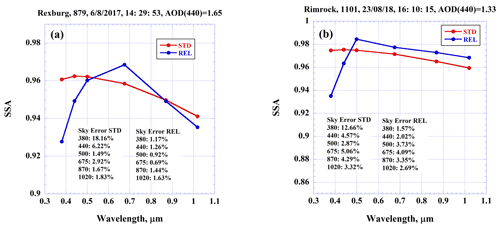
Figure 1SSA retrieved using V3 assumptions on the IPRI smoothness constants (STD) and that of relaxed constraints (REL): (a) Rexburg (AOD(440) = 1.65, AE = 1.74) and (a) Rimrock (AOD(440) = 1.33, AE = 1.79) AERONET sites.
Figure 1 shows two examples of SSA retrieved from six spectral channel measurements at 380, 440, 500, 675, 870 and 1020 nm taken at AERONET sites where aerosols from biomass burning were present during the time of observations: Rexburg, Idaho, USA (43∘ N, 111∘ W), on 6 August 2017 (Fig. 1a) and Rimrock, Idaho, USA (46∘ N, 116∘ W), on 23 August 2018 (Fig. 1b). The AE was high for both of these cases, AE (440–870 nm) = 1.74 for the Rexburg case and AE = 1.79 for the Rimrock retrieval case, thereby imposing strong constraints on spectral IPRI in the V3 or STD retrievals. It is noted that the AOD was very high for both of these cases (Rimrock: 1.65 at 440 nm and 0.35 1020 nm; Rexburg: 1.32 at 440 nm and 0.27 at 1020 nm), thereby providing a large aerosol signal at most wavelengths and thus enabling very accurate retrievals of absorption information (Sinyuk et al., 2020). High-AOD events dominated by fine mode particles (high AE) in the northern US Rocky Mountain region in June–October are dominated by biomass burning emissions. Sky radiances at 440 to 1020 nm for these measurements were calibrated using an integrating sphere (e.g., Holben et al., 1998) while the vicarious method (e.g., Li et al., 2008) was used for calibration at 380 nm since the sphere does not provide high enough radiance output in the UV. Figure 1 displays two types of SSA retrievals: the first, depicted as STD, was obtained by inversion using the V3 assumption on the IPRI smoothness constraints, and the second, depicted as REL, is the result of inversion employing the new assumption on spectral IPRI while all other aspects remain the same. Two major features are displayed in Fig. 1: a significant decrease in SSA retrieved using REL at the shortest wavelengths (380 and 440 nm) and a reduction in corresponding residual error values at these channels. The residual is the root mean square difference between the measured sky radiances and those computed based on the retrieved aerosol parameters. For example, the reduction in SSA in the Rexburg case is ∼ 0.033 and ∼ 0.013 at 380 and 440 nm, respectively, while at longer wavelengths, the difference in SSA retrieved using REL and STD assumptions is less than 0.01. The spectral dependence of SSA retrieved using the REL assumption is qualitatively consistent with that of BrC absorption (e.g., Mok et at al., 2016). The absolute reduction (difference between the STD and the REL residual errors) in residual values in the Rexburg case is ∼ 17 % and ∼ 5 % at 380 and 440 nm, respectively, implying more accurate SSA retrievals obtained using the REL assumption. The retrieved spectral dependence of SSA and residuals in the Rimrock case exhibit similar behavior: ∼ 0.04 and ∼ 0.012 decrease in SSA at 380 and 440 nm, respectively, with correspondent reductions in spectral residuals of ∼ 11.1 % and ∼ 2.55 %. Figure 1 shows that in both cases the SSA absolute differences at 675 nm are comparable to that at 440 nm, which only can partly be explained by BrC absorption due to its decrease with wavelength. Another possible reason for that is the larger uncertainty at 675 nm than at other wavelengths in the extraterrestrial solar spectrum as discussed in Sinyuk et al. (2020).
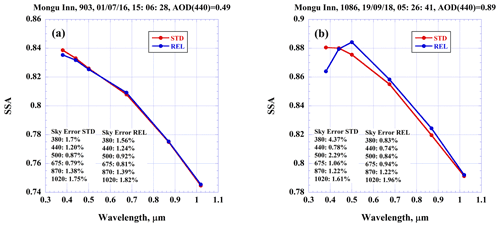
Figure 2SSA retrieved using V3 assumptions on the IPRI smoothness constants (STD) and that of relaxed constraints (REL) at Mongu Inn AERONET site: (a) AOD(440) = 0.49, AE = 1.85 and (b) AOD(440) = 0.89, AE = 1.97.
Figure 2 shows SSA retrieved at the Mongu Inn AERONET site in Zambia (15∘ S, 23∘ E) for cases with two different values of AOD measured at 440 nm: 0.49 (Fig. 2a) and 0.89 (Fig. 2b). The AE is high for both of these cases (1.85 and 1.97, respectively), therefore resulting in strong constraints in the spectral IPRI in the STD retrievals. The light absorption by aerosol at this site in the July–October burning season (Eck et al., 2003) is dominated by BC due to savanna burning with significant flaming phase combustion production of BC (Ward et al., 1996). Therefore inversions using STD and REL assumptions should result in similar retrievals, assuming BC dominates over BrC absorption. This is indeed the case as can be seen from Fig. 2. For the lower-AOD case, the SSA and residual values corresponding to both STD and REL assumptions are very close with SSA absolute difference below 0.0033 and that of residual values less than 0.14 %. For the higher-AOD case, the spectral dependencies show similar behavior except for 380 nm, where the difference in SSA and residual values is ∼ 0.017 and 3.6 %, respectively. This increase in aerosol absorption at 380 nm can be explained by the presence of BrC in addition to BC in aerosol composition for this biomass burning event. Indeed Kirchstetter et al. (2004) measured a significant BrC absorption signature in biomass burning smoke from savanna burning in southern Africa.
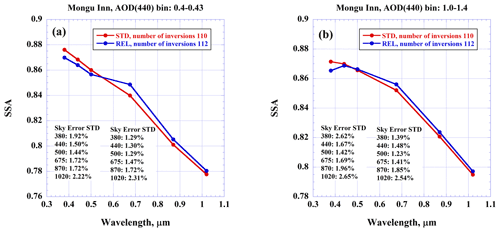
Figure 3SSA averaged over AOD(440) bins for the Mongu Inn AERONET site: (a) 0.4–0.43 and (b) 1.0–1.4.
The above example illustrates that for biomass burning aerosols dominated by BC, the BrC also may be present in aerosol composition, with its relative concentration possibly increasing with AOD magnitude. To check this assumption, statistics of retrieved SSA and spectral residuals at the Mongu Inn site were generated by averaging them over narrow AOD bins. Figure 3 shows averaged SSA and spectral residual values for two bins in AOD: 0.4–0.43 (Fig. 3a) and 1.0–1.4 (Fig. 3b). As can be seen, for the lower-AOD bin, the average SSA retrieved with the REL assumption does not show any indication of BrC presence and is very close to that of STD retrieval, with a maximum difference of ∼ 0.009 at 675 nm. This difference, as before, can be attributed in part to larger uncertainty in the solar spectrum at this wavelength. Spectral residual errors are very close for both types of inversion, with the difference under 1 %. Figure 3b, on the other hand, shows a slight increase in aerosol absorption at 380 nm as retrieved by inversion using the REL assumption, with differences of ∼ 0.006 and ∼ 1.2 % for SSA and residual values, respectively. The same type of statistics were generated for the Rimrock site and are shown in Fig. 4. It shows averaged SSA and spectral residual values for two AOD bins: 0.5–0.53 (Fig. 4a) and 1.0–1.4 (Fig. 4b). A higher-AOD-magnitude bin for lower AOD for this site was selected because statistics for the 0.4–0.43 bin was not representative due to small sample size. Figure 4 shows that biomass burning at Rimrock shows strong BrC absorption at shorter wavelengths for AOD magnitude higher than 0.5 while, according to Fig. 3b, for Mongu Inn BrC absorption at 380 nm is noticeable only for AOD magnitude higher than 1 (an analysis for the 0.5–0.53 AOD bin for Mongu did not show any increase in 380 nm absorption). The magnitude of BrC absorption and its strength relative to BC absorption (from mid-visible to UV) varies significantly for biomass burning aerosols potentially dependent on several factors including fuel types and moisture content, relative strengths of the phase of combustion (flaming versus smoldering), fire intensity, and aging processes of the aerosols (Lewis et al., 2008; Di Lorenzo et al., 2017; Wong et al., 2019).
3.2 Desert dust aerosols
For desert dust aerosols REL and STD retrievals of SSA are expected to be similar due to similarity between REL and V3 (or STD) assumptions and very weak constraints on spectral IPRI for low AE in both. Figure 5 shows SSA and spectral residual values retrieved at the Mezaira, UAE (23∘ N, 53∘ E) AERONET site for two individual cases of AOD: 0.44 (Fig. 5a) and 1.45 (Fig. 5b). The AE(440–870) was 0.24 for the case shown in Fig. 5a and 0.20 for the case in Fig. 5b; therefore both are cases dominated by coarse mode dust (Reid et al., 2008; Eck et al., 2008). As expected, SSA retrieved using REL and STD assumptions is very similar, with differences below 0.002 and 0.004 for AOD 0.44 and 1.45, respectively. The difference between spectral residuals is within half a percent. The decrease in SSA at the shorter visible and UV wavelengths is primarily due to absorption from iron oxide content in mineral dust (Di Biagio et al., 2019). The similarity between SSA retrieved using REL and STD assumptions also holds for SSA averaged over AOD bins. Figure 6 shows averaged SSA and spectral residuals for two AOD bins: 0.4–0.43 (Fig. 6a) and 1.0–1.4 (Fig. 6b). The SSA differences are below 0.0006 and 0.0015 and therefore insignificant for lower and higher AOD, respectively, with the differences in spectral residual values within 0.5 %.
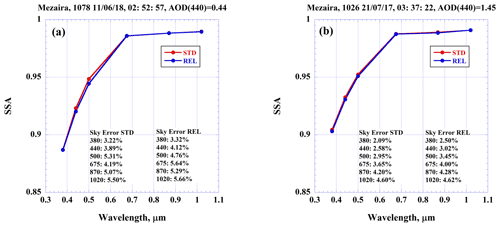
Figure 5SSA retrieved using V3 assumptions on the IPRI smoothness constants (STD) and that of relaxed constraints (REL) at the Mezaira AERONET site: (a) AOD(440) = 0.44, AE = 0.24 and (b) AOD(440) = 1.45, AE = 0.2.
3.3 Urban industrial aerosols
Urban industrial aerosols are defined as aerosol originating primarily from fossil fuel combustion in populated industrial regions (Eck et al., 1999). For this analysis the GSFC, Maryland, USA (38∘ N, 76∘ W), AERONET site located near Washington, DC, was selected. Note that this site has relatively high SSA when compared to other urban industrial sites (Dubovik et al., 2002; Giles et al., 2012), due to relatively low BC content. Figure 7 shows two cases of spectral SSA retrievals corresponding to two different values of 440 nm AOD: 0.33 (Fig. 7a) and 0.49 (Fig. 7b). The AE(440–870 nm) for the case in Fig. 7a was 1.74 and for Fig. 7b was 1.63; therefore these high AE values resulted in strong constraint on IPRI being applied in the V3 or STD assumption. The AOD cases with lower magnitudes were selected for this site due to the lower average 440 nm AOD level at GSFC (0.186) compared to the considered AERONET sites (e.g., 0.427 for Mongu Inn and 0.36 for Mezaira). In addition, according to Sinyuk et al. (2020), average SSA uncertainties at 440 nm estimated at GSFC for AOD (440) = 0.3 are ∼ 0.03, which is similar to the AERONET threshold of AOD(440) = 0.4 with SSA(440) uncertainty of 0.03 for Level 2. Figure 7 shows that employing the REL smoothness constraint assumption for IPRI reduces the values of spectral residuals in both AOD cases, implying that the STD constraint was restricting spectral variability of the IPRI (SSA) for this aerosol type. The absolute SSA differences at the four standard AERONET sky radiance measurement channels, 440, 675, 870 and 1020 nm, for the first case are 0.0045, 0.0074, 0.0163 and 0.021, respectively, which is within average uncertainties estimated in Sinyuk et al. (2020) for this site at AOD 0.3 using the STD assumption: 0.028, 0.034, 0.043 and 0.048. For the higher-AOD case the SSA differences are smaller than that for lower-AOD case and within the average uncertainties of Sinyuk et al. (2020) (in parentheses) estimated at AOD 0.5: 0.0074 (0.019), 0.0162 (0.023), 0.0154 (0.029) and 0.0135 (0.033). The SSA differences at 380 nm are slightly larger than those at 440 nm: 0.0052 and 0.013 for Fig. 7a and b, respectively. The SSA difference at 500 nm is in between those at 440 and 675 nm.
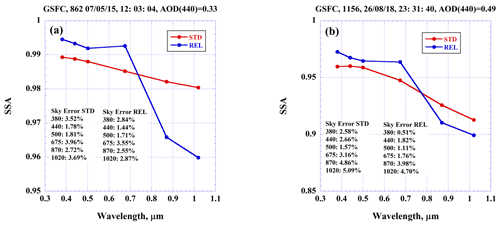
Figure 7SSA retrieved using V3 assumptions, depicted as STD, and that of REL on the IPRI smoothness constants at the GSFC AERONET site: (a) AOD(440) = 0.33, AE = 1.74 and (b) AOD(440) = 0.49, AE = 1.63.
Figure 8 shows spectral dependencies of retrieved SSA at the GSFC site averaged over narrow AOD bins: 0.3–0.33 (Fig. 8a) and 0.5–0.53 (Fig. 8b). As in the example cases analyzed before, one can see a reduction in spectral residuals for the REL assumption and similar spectral behavior of retrieved SSA. Also, both Figs. 7 and 8 demonstrate similar features in spectral behavior of SSA retrieved using the REL assumption, one of which is a slight drop in SSA values at 500 nm. The relative magnitude of this drop depends on the SSA value at 675 nm, which was largely suppressed by the STD assumption (larger residual value at 675 nm) while at 500 nm both STD and REL residuals are lower than at the neighboring 675 nm and similar to each other. One of the possible reasons for this non-smooth spectral behavior of retrieved SSA can be explained by spectrally non-uniform (in both magnitude and sign) sky radiance calibration coefficients which will have a stronger effect on retrievals for weaker smoothness constraints. Figure 9 shows results of Fig. 7a computed with SSA retrieved by inverting sky radiances which were vicariously calibrated using the technique of Li et al. (2008). In this vicarious method the field of view (FOV) at 870 nm was computed from sphere radiance calibration along with the highly accurate sun calibration plus extraterrestrial solar irradiance. Then the FOV was subsequently utilized to determine sky radiance calibration for all other wavelengths by assuming a constant FOV for all wavelengths. Implemented in such a way, the vicarious calibration approach can potentially eliminate spectral non-uniformity of calibration as well as eliminate dependence on the solar spectrum at wavelengths other than 870 nm. Figure 9 shows that inverting vicariously calibrated sky radiances produced smoother spectral dependence of SSA, eliminating the slight decrease in SSA at 500 nm. Notably, all of the SSA plots for the GSFC site (Figs. 7, 8 and 9) show a marked difference in spectral slope from less than 675 nm to greater than 675 nm. This is likely a result of BrC absorption in the shorter wavelengths from the organic carbon aerosol component present in emissions from fossil fuel combustion.
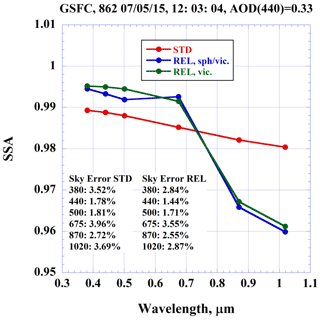
Figure 9Same as Fig. 7a with the addition of SSA retrieved from vicariously calibrated sky radiances, depicted by “REL, vic”. The abbreviation “REL, sph./vic.” refers to SSA retrieved by inverting sky radiances calibrated using the integrated sphere at all the channels except 380 nm, which was calibrated using the vicarious method.
The comparison of retrievals obtained using REL vs. STD assumptions on the IPRI smoothness constraints is presented for the Rexburg, Mongu, Mezaira and GSFC AERONET sites, and results are summarized in Tables 1 through 3. For parameters characterizing aerosol absorption, SSA comparisons are presented instead of IPRI due to its widespread use in climate research. In a manner similar to that of Sinyuk et al. (2020), for SSA and the real part of the refractive index (RPRI), this analysis is done for three bins in 440 nm AOD, which provides comprehensive comparisons by considering different levels of sensitivity to adjustment in the IPRI smoothness constraints. For PSD parameters, two bins in 440 nm AOD are used due to much higher stability and the small uncertainty of aerosol size distribution retrievals. All the tables display mean values and standard deviations of the difference between STD and REL aerosol retrievals.
Table 1Average values and standard deviations (in parentheses) of the difference in SSA retrievals from inversions using STD and REL assumptions on IPRI smoothness constraints. The difference is defined as REL minus STD.
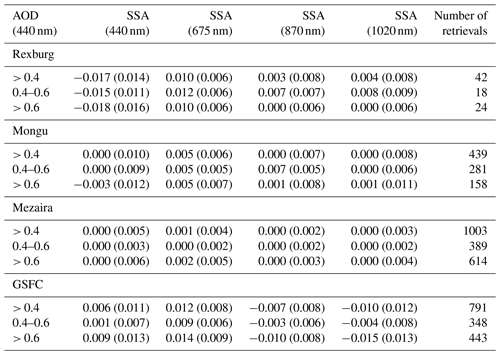
Table 2Average values and standard deviations (in parentheses) of the difference in the real part of refractive index (RPRI) retrievals from inversions using STD and REL assumptions on IPRI smoothness constraints. The difference is defined as REL minus STD.
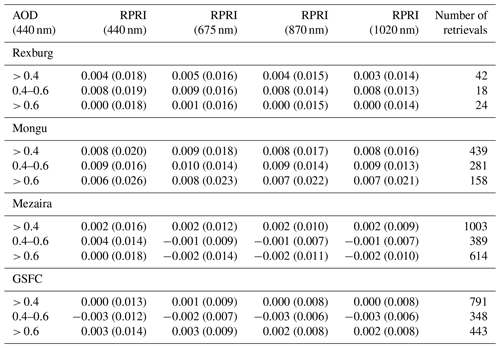
Table 3Average values and standard deviations (in parentheses) of the difference in volume median radius (VMR) in microns and width of particle size distribution (STD) retrievals from inversions using STD and REL assumptions on IPRI smoothness constraints. The difference is defined as REL minus STD.
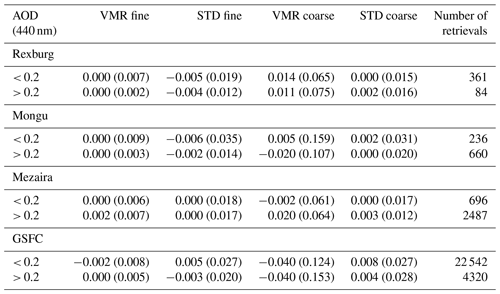
For Rexburg, the largest difference in SSA retrievals, shown in Table 1, is at 440 nm, with no clear dependence on the level of AOD at 440 nm. The average difference (over AOD levels) of −0.0167 constitutes ∼ 50 % of the SSA uncertainty at 440 nm estimated in Sinyuk et al. (2020) for biomass burning aerosols (for a different site however, Mongu). The SSA differences decreased as wavelength increased with the value of ∼ 0.01 at 675 nm and the values at 870 and 1020 nm below 0.01, which is consistent with the spectral behavior of BrC absorption. The differences in the retrieved real part of the refractive index are shown in Table 2. Since assumptions on smoothness constraints for RPRI were not modified, the observed differences are small, significantly smaller than variability in PRRI retrievals. Table 3 shows that retrieved PSD parameters for fine mode aerosol are practically the same for STD and REL inversions while the differences for volume median radius (VMR) for coarse mode aerosol are 0.014 and 0.01 µm for 440 nm AOD smaller and larger than 0.2, respectively. These differences in VMR for coarse mode are not significant considering a rather small coarse mode optical contribution for this fine-mode-dominated total AOD, plus the large size of these coarse mode particles (VMR typically > 3 µm).
For Mongu, the differences in SSA are very small (Table 1) as expected due to weak spectral dependence of the IPRI for BC-dominated aerosols, and this is the assumption applied in the STD retrievals. SSA differences at 440 nm are smaller than 0.0001 for the first two 440 nm AOD bins. At the same time, the difference for 440 nm AOD greater than 0.6 is larger with the value of ∼ 0.003, which may be indicative of BrC presence for this larger aerosol loading. Tables 2 and 3 show the mean difference in RPRI and PSD parameters, respectively. As in the Rexburg case, the difference in RPRI retrievals is very small: 0.01 and lower. The retrieved PSD parameters are also in very close agreement for STD and REL retrievals for both fine and coarse modes.
For Mezaira (UAE), the results of the REL–STD comparison are shown in Tables 1, 2 and 3. Due to similarity of REL and STD smoothness constraint assumptions for coarse mode aerosols, all three tables show very small and insignificant differences in the retrieved aerosol parameters. Table 1 shows that the difference in SSA retrievals is smaller than 0.0001, except at 675 nm where the difference is smaller than 0.002. The differences in RPRI retrievals are below 0.005 for all the wavelengths' AOD levels. The difference in retrieved PSD parameters is also small, with the largest difference (0.02 µm) in coarse VMR for AOD greater than 0.2, which is significantly smaller than the standard deviation of retrievals for this parameter for this site (0.36 µm).
Table 1 shows the differences in SSA retrievals for the GSFC AERONET site. The main feature as is displayed in Table 1 is the increase in differences with increasing AOD level at all wavelengths. If the increase is defined as the difference between average SSA values corresponding to the third (> 0.6) and second (0.4–0.6) AOD bins, the values are the following: 0.008, 0.005, 0.007 and 0.011 for 440, 675, 870 and 1020 nm, respectively. This increase in REL–STD differences at higher AOD can be explained by stronger smoothing of the spectral dependence of IPRI by the STD constraints for larger AOD. This is illustrated by the different degree of reduction in spectral residual errors for different AOD values at 440 nm. For example, Fig. 7 shows that reductions in spectral residuals at 380 nm are 0.68 % and 2.07 % for AOD = 0.33 and 0.49, respectively, illustrating stronger suppression of spectral dependence of IPRI at higher AOD. Also, like in the case studies, the 675 comparison exhibits the largest difference, which can be at least partially explained by the largest uncertainty in extraterrestrial solar flux at this wavelength (Sinyuk et al., 2020) as well as possibly by spectrally non-uniform uncertainty in sky radiance calibration coefficients. Table 2 shows a comparison of RPRI retrievals. The agreement between STD and REL retrievals is very good, with a mean difference below 0.003. Table 3 also demonstrates a very good agreement in retrieved PSD parameters for this site, similar to all other sites examined.
In this section SSA retrieved from AERONET observations is compared to SSA determined from in situ measurements collected during the DRAGON-MD (Distributed Regional Aerosol Gridded Observational Network-Maryland) experiment in 2011 (Holben et al., 2018). The SSA values are derived from in situ measurements made during aircraft vertical profiles of scattering and absorption coefficients at 550 nm. For each profile, 1 s sampled values of scattering coefficient measurements at 450, 550 and 700 nm from the nephelometer and absorption coefficient measurements at 470, 532 and 660 nm from the particle soot absorption photometer were provided, both from dried air samples. At 550 nm, an additional scattering measurement at ambient relative humidity allowed for the calculation of an ambient SSA (rather than dried aerosol) that is more suitable for comparison with the SSAs derived from AERONET radiance measurements. In order to produce a column SSA value to compare with AERONET, the 1 s SSA aircraft measurements were averaged for the duration of the profile sampling after weighting the values according to aerosol loading (Schafer et al., 2014). The analysis of Schafer et al. (2014) had shown that SSA retrieved by AERONET (interpolated to 550 nm) was on average 0.011 lower than the values derived from in situ profiles. In this section we compare SSA derived from AERONET observations using both the STD and REL assumptions on the IPRI smoothness constraints to SSA determined from in situ measurements. The comparison is done for several temporary AERONET sites which were set up during the DRAGON 2011 campaign in Maryland, USA: Aldino, Beltsville, Essex and Fair Hill. The type of aerosols that dominated the selected sites are similar to that at GSFC and can also be defined as urban industrial. All the comparisons are made for 440 nm AOD larger than 0.3 due to higher sensitivity to aerosol absorption for this aerosol type (Sinyuk et al., 2020).
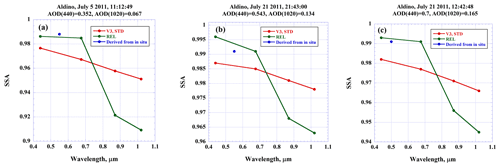
Figure 10Comparison of SSA retrieved by V3 aerosol inversion code employing the STD assumption on IPRI smoothness constraints and SSA retrieved by inversion code using the REL assumption on SSA derived from in situ measurements at Aldino AERONET site: (a) 440 nm AOD = 0.352, (b) 440 nm AOD = 0.543 and (c) 440 nm AOD = 0.7.
Figure 10 shows comparisons of SSA retrieved at the Aldino site for three levels of 440 nm AOD: 0.352, 0.543 and 0.7. All three cases show that the values of SSA retrieved by the V3 inversion code employing the STD assumption on the IPRI smoothness constraints are lower than SSA derived from in situ measurements, which is consistent with the conclusion of Schafer et al. (2014). On the other hand, the SSA values retrieved from the inversion code employing the REL constraint assumption are closer to in situ measured SSA. For the lowest 440 nm AOD value, Fig. 10a, the differences between in situ SSA and that retrieved using STD and REL (in parentheses) assumptions are 0.0158 (0.0024). For intermediate, Fig. 10b, and the largest, Fig. 10c, 440 nm AOD the corresponding deviations are 0.0035 (−0.0027) and 0.011 (−0.001).
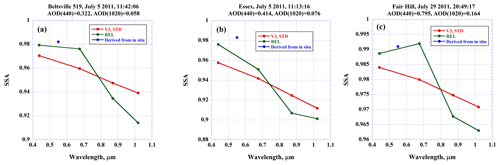
Figure 11Comparison of SSA retrieved by V3 aerosol inversion code employing the STD assumption on IPRI smoothness constraints and SSA retrieved by inversion code using the REL assumption on SSA derived from in situ measurements: (a) Beltsville AERONET site, 440 nm AOD = 0.322, (b) Essex AERONET site, 440 nm AOD = 0.414 and (c) Fair Hill AERONET site, 440 nm AOD = 0.795.
Figure 11 shows SSA comparisons for three AERONET sites: Beltsville (440 nm AOD = 0.322), Essex (440 nm AOD = 0.414) and Fair Hill (440 nm AOD = 0.795). As in the Aldino cases, the SSA values retrieved from inversions using REL assumptions on IPRI smoothness constraints are closer to SSA values derived from in situ measurements than those retrieved by the V3 (STD assumption) inversion code: 0.0166 (0.004) for Beltsville, 0.032 (0.018) for Essex and 0.009 (0.0008) for Fair Hill.
The above examples demonstrate that the bias in SSA comparison reported in Schafer et al. (2014) may be due at least in part to the strong IPRI smoothness constraints which restricted spectral variability of IPRI for this urban industrial aerosol type. Therefore, it is expected that analysis based on SSA retrievals from inversions employing the REL assumption on the IPRI smoothness constraints will result in smaller bias. However, it must be considered that these differences are well within the uncertainty error bars of both the in situ measured SSA and the AERONET retrieved SSA (regardless of the constraint type on the IPRI). Therefore the “improvement” in agreement when applying the REL constraint on the IPRI may not have much significance.
A modification of the assumption on the smoothness constraints of the spectral variation in IPRI employed by V3 of the AERONET aerosol retrieval algorithm is presented and discussed. This modification is termed relaxed due to the weaker strength of this new smoothness constraint. It prevents oversmoothing of the spectral dependence of the IPRI for different aerosol types including high-AE aerosols (fine mode dominated) containing BrC. The modification employs spectrally dependent smoothness constraints, which are implemented by assigning different weights to different pairs of wavelengths. The weight 10−6 is assigned to shorter wavelengths, while weight 10−5 is assigned to the 870–1020 nm pair. This larger weight provides a stronger constraint for longer wavelengths where sensitivity to aerosol absorption is limited for high-AE aerosols due to low AOD.
The effect of these REL smoothness constraints on retrievals of SSA was analyzed for different aerosol types: BrC- and BC-containing aerosols, mineral dust, and urban industrial aerosols. Analyses have shown that the modification of the IPRI smoothness constraints mainly affects SSA retrieved for BrC-containing biomass burning aerosols, resulting in reduction of both SSA and spectral residuals at shorter wavelengths (mid-visible to the UV). For example, for SSA retrieved for specific cases at the Rexburg and Rimrock AERONET sites (in Idaho) for biomass burning aerosol, the reduction in SSA and residual at 380 nm are 0.033 and ∼ 17 % and 0.04 and ∼ 11.1 %, respectively. The reduction in sky radiance residual for these cases implies more accurate SSA retrievals with the new REL assumption since the computed sky radiances closely match the measured values. For mineral dust and BC-dominated aerosols the effect of modified assumptions on IPRI smoothness constraints is very small except for high-AOD cases for BC-dominated aerosols, which show a slight increase in absorption at 380 nm. This can be explained by an increase in BrC in aerosol composition for high-AOD biomass burning events dominated by BC as the primary aerosol absorber (Mongu, Zambia). The REL assumption on the IPRI smoothness constraints results in changes of SSA and spectral residual for urban industrial aerosols, especially reduced SSA at the longest wavelengths. For example, the reduction in retrieved SSA is ∼ 0.016 and ∼ 0.02 at 875 and 1020 nm, respectively, for 440 nm AOD ∼ 0.3 at GSFC.
For urban industrial aerosols at GSFC, retrieved SSA exhibited non-smooth spectral behavior in the vicinity of 500 nm. It was assumed that this behavior can be explained, in part, by the spectral non-uniformity (in both magnitude and sign) of sky radiance calibration coefficients and spectral variation in uncertainty of the assumed extraterrestrial irradiance. To check this assumption, SSA spectral dependence was retrieved by inverting vicariously calibrated sky radiances. Vicarious calibration employs field of view determined empirically by matching vicarious and sphere calibrated radiances at the 870 nm spectral channel. Designed in such a way, it minimizes spectral non-uniformity of calibration as well as dependence on the solar spectrum at wavelengths other than 870 nm. Inversion of the vicariously calibrated sky radiances produced smoother spectral dependence of SSA without an anomaly at 500 nm.
The average differences between aerosol parameters retrieved using STD and REL assumptions are presented and analyzed for four AERONET sites: Rexburg, Mongu, Mezaira and GSFC. The analysis confirmed the results of the case study analysis for SSA: the largest difference is observed for BrC-containing biomass burning aerosols (Rexburg) and urban industrial aerosols (GSFC). The average differences for SSA retrieved at these sites are within U27 uncertainties estimated in Sinyuk et al. (2020). For mineral dust (Mezaira) and BC-containing aerosols (Mongu) the average SSA differences are small due to similarity in STD and REL assumptions for dust cases and due to similarity in BC spectral variation in IPRI to the STD assumption for the high-BC-content cases. Comparisons of the RPRI and PSD parameters showed very close agreements because assumptions on smoothness constraints for these parameters were not modified.
SSA retrieved from AERONET observations using both STD and REL assumptions were compared to SSA determined from in situ measurements collected from aircraft profiles during the DRAGON-MD experiment. The comparisons were done for four temporary AERONET sites set up during the experiment and showed closer agreement between SSA retrieved using the REL assumption and in situ SSA than between in situ SSA and SSA retrieved using the STD constraint. This can possibly be explained by the fact that the STD assumption employed in V3 resulted in over-smoothing of the retrieved IPRI.
The implementation of the relaxed smoothness constraints on the imaginary part of the refractive index in the next version of the AERONET inversion algorithm will produce significant impacts at some sites, with changes up to ±0.033 and ±0.013 in short wavelength channels (380 and 440 nm) for some biomass burning smoke cases with significant BrC content and possibly up to ±0.015 in mid-visible channels (500 and 675 nm) to near-IR channels (870 to 1020 nm) for some urban industrial aerosol types while still being mostly within the uncertainty of the AERONET SSA retrievals, depending on AOD level, Ångström exponent and wavelength. For mineral dust aerosols the impact will be insignificant, while for biomass burning aerosol dominated by BC the changes will be relatively small. The application of this new REL constraint on the spectral variation in the IPRI is being planned for future retrievals in Version 4 of the AERONET database. These retrievals will therefore have increased sensitivity to absorption by BrC in fine-mode-dominated aerosol than is currently available in the Version 3 database. Additionally, future retrievals with the added wavelengths of 380 and 500 nm will enable more accurate and robust monitoring of BrC absorption in fine mode aerosols and iron oxides in coarse mode dust aerosols.
Abbreviation | Term |
AOD | Aerosol optical depth |
AE | Ångström exponent |
BrC | Brown carbon |
BC | Black carbon |
CIR | Complex index of refraction |
IPRI | Imaginary part of refractive index |
LMS | Least-squares method |
PSD | Particle size distribution |
RPRI | Real part of refractive index |
REL | Relaxed smoothness constraints |
STD | Standard smoothness constraints |
SSA | Single-scattering albedo |
VMR | Volume median radius |
V1 | Version 1 of AERONET aerosol retrieval algorithm |
V2 | Version 2 of AERONET aerosol retrieval algorithm |
V3 | Version 3 of AERONET aerosol retrieval algorithm |
Retrieval code is available on demand from aliaksandr.sinyuk-1@nasa.gov and/or david.m.giles@nasa.gov. The version of the code used in this paper is not operational yet and will be a part of upcoming Version 4 of the AERONET aerosol retrieval algorithm, which will be released in 2024.
Research data are available on demand from aliaksandr.sinyuk-1@nasa.gov and/or david.m.giles@nasa.gov. The data used are not yet a part of the AERONET aerosol retrieval product and will be part of upcoming Version 4 of the AERONET aerosol retrieval algorithm, which will be released in 2024.
The development of relaxed smoothness constraints for the AERONET aerosol retrieval algorithm is the result of joint effort of the members of the AERONET team (listed as ASi, BNH, TFE, DMG, IS, JSS, ASm and MS) as well as researchers from outside the project (OD). Individual contributions can be summarized as follows. ASi modified AERONET retrieval code by incorporating relaxed smoothness along with OD, who developed the theoretical basis. BNH, TFE, DMG, IS, JSS, ASm and MS contributed to discussions during AERONET team meetings.
At least one of the (co-)authors is a member of the editorial board of Atmospheric Measurement Techniques. The peer-review process was guided by an independent editor, and the authors also have no other competing interests to declare.
Publisher's note: Copernicus Publications remains neutral with regard to jurisdictional claims in published maps and institutional affiliations.
The AERONET project at NASA GSFC is supported by the Earth Observing System Project Science Office cal–val, Radiation Sciences Program at NASA headquarters, and various field campaigns. Resources supporting this work were provided by the NASA High-End Computing (HEC) Program through the NASA Center for Climate Simulation (NCCS) at the Goddard Space Flight Center.
This paper was edited by Alexander Kokhanovsky and reviewed by three anonymous referees.
Ahn, C., Torres, O., and Jethva, H.: Assessment of OMI near-UV aerosol optical depth over land, J. Geophys. Res.-Atmos., 119, 2457–2473, https://doi.org/10.1002/2013JD020188, 2014.
Alder, J., Wagner, N., L., Lamb, K. D., Manfred, K., M., Schwarz, J., P., Franchin, A., Middlebrook, A., M., Washenfelder, R., A., Womack, C., C., Yokelson, R., J., and Murphy, D., M.: Evidence in biomass burning smoke aerosol with properties intermediate between brown and black carbon, Aerosol Sci. Tech., 53, 976–989, 2019.
Aster, R. C., Borchers, B., and Thurber, C. H.: Parameter estimation and inverse problems, 2nd edn., Elsevier Inc., ISBN 978-0128100929, 2013.
Bond, T. C. and Bergstrom, R. W.: Light absorption by carbonaceous particles: An investigative review, Aerosol Sci. Tech., 40, 27–67, 2006.
Bond, T. C., Doherty, S. J., Fahey, D. W., Forster, P., M., Berntsen, T., DeAngelo, B. J., Flanner, M. J., Ghan, S., Karcher, B., Koch, D., Kinne, S., Kondo, Y., Quinn, P. K., Sarofim, M. C., Schulz, M. G., Schulz, M., Venkataraman, C., Zhang, H., Zhang, S., Bellouin, N., Guttikunda, S. K., Hopke, P. K., Jacobson, M. Z., Kaiser, J. W., Klimont, Z., Lohmann, U., Schwartz, J. P., Shindell, D., Storelvmo, T., Warren, S. G., and Zender, C. S.: Bounding the role of black carbon in the climate system: A scientific assessment, J. Geophys. Res.-Atmos., 118, 5380–5552, https://doi.org/10.1002/jgrd.50171, 2013.
Choi, M., Kim, J., Lee, J., Kim, M., Park, Y.-J., Holben, B., Eck, T. F., Li, Z., and Song, C. H.: GOCI Yonsei aerosol retrieval version 2 products: an improved algorithm and error analysis with uncertainty estimation from 5-year validation over East Asia, Atmos. Meas. Tech., 11, 385–408, https://doi.org/10.5194/amt-11-385-2018, 2018.
Di Biagio, C., Formenti, P., Balkanski, Y., Caponi, L., Cazaunau, M., Pangui, E., Journet, E., Nowak, S., Andreae, M. O., Kandler, K., Saeed, T., Piketh, S., Seibert, D., Williams, E., and Doussin, J.-F.: Complex refractive indices and single-scattering albedo of global dust aerosols in the shortwave spectrum and relationship to size and iron content, Atmos. Chem. Phys., 19, 15503–15531, https://doi.org/10.5194/acp-19-15503-2019, 2019.
Di Lorenzo, R. A., Washenfelder, R. A., Attwood, A. R., Guo, H., Xu, L., Ng, N. L., Weber, R. J., Baumann, K., Edgerton, E., and Young, C. J.: Molecular-Size-Separated Brown Carbon Absorption for Biomass-Burning Aerosol at Multiple Field Sites, Environ. Sci. Technol., 51, 3128–3137, https://doi.org/10.1021/acs.est.6b06160, 2017.
Dubovik, O.: Optimization of numerical inversion in photopolarimetric remote sensing, in: Photopolarimetry in remote sensing, edited by: Videen, G., Yatskiv, Y., and Mishchenko, M., Kluwer Academic Publisher, Dordecht, the Netherlands, 65–106, https://doi.org/10.1007/1-4020-2368-5_3, 2004.
Dubovik, O. and King, M.: A flexible inversion algorithm for retrieval of aerosol optical properties from Sun and sky radiance measurements, J. Geophys. Res., 105, 20763–20696, 2000.
Dubovik, O., Holben, B. N., Eck, T. F., Smirnov, A., Kaufman, Y. J., King M. D., Tanre, D., and Slutsker, I.: Variability of absorption and optical properties of key aerosol types observed in worldwide locations, J. Atmos. Sci., 59, 590–608, 2002.
Dubovik, O., Sinyuk, A., Lapyonok, T., Holben, B. N., Mishchenko, M., Yang, P., Eck, T. F., Volten, H., Munoz, O., Veihelmann, B., van der Zande, W. J., Leon, J. F., Sorokin, M., and Slutsker, I.: Application of spheroid models to account for aerosol particle nonsphericity in remote sensing of desert dust, J. Geophys. Res., 111, D11208, https://doi.org/10.1029/2005JD006619, 2006.
Dubovik, O., Herman, M., Holdak, A., Lapyonok, T., Tanré, D., Deuzé, J. L., Ducos, F., Sinyuk, A., and Lopatin, A.: Statistically optimized inversion algorithm for enhanced retrieval of aerosol properties from spectral multi-angle polarimetric satellite observations, Atmos. Meas. Tech., 4, 975–1018, https://doi.org/10.5194/amt-4-975-2011, 2011.
Dubovik, O., Fuertes, D., Litvinov, P., Lopatin, A., Lapyonok, T., Dubovik, I., Xu, F., Ducos, F., Chen, C., Torres, B., Derimian, Y., Li, L., Herreras-Giralda, M., Herrera, M., Karol, Y., Matar, C., Schuster, G., Espinosa, R., Puthukkudy, A., Li, Z., Fischer, J., Preusker, R., Cuesta, Y., Kreuter, A., Cede, A., Aspetsberger, M., Marth, D., Bindreiter, L., Hangler, A., Lanzinger, V., Holter, C., and Federspiel, C.: A comprehensive description of Multi-term LSM for applying multiple a priori constraints in problems of atmospheric remote sensing: GRASP algorithm, concept, and applications, Front. Remote, Sens., 2, 706851, https://doi.org/10.3389/frsen.2021.706851, 2021.
Dubovik, O. V., Lapyonok, T. V., and Oshchepkov, S. L.: Improved technique for data inversion: Optical sizing of multicomponent aerosols, Appl. Opt., 34, 8422–8435, 1995.
Eck, T. F., Holben, B. N., Reid, J. S., Dubovik, O., Smirnov, A., O'Neill, N. T., Slutsker, I., and Kinne, S.: Wavelength dependence of the optical depth of biomass burning, urban, and desert dust aerosols, J. Geophys. Res., 104, 31333–31349, 1999.
Eck, T. F., Holben, B. N., Ward, D. E., Mukelabai, M. M., Dubovik, O., Smirnov, A., Schafer, J. S., Hsu, N. C., Piketh, S. J., Queface, A., and Roux, J. L.: Variability of biomass burning aerosol optical characteristics in southern Africa during the SAFARI 2000 dry season campaign and a comparison of single scattering albedo estimates from radiometric measurements, J. Geophys. Res.-Atmos., 108, 8477, https://doi.org/10.1029/2002JD002321, 2003.
Eck, T. F., Holben, B. N., Reid, J. S., Sinyuk, A., Dubovik, O., Smirnov, A., Giles, D., O'Neill, N. T., Tsay, S. C., Ji, Q., and Al Mandoos, A.: Spatial and temporal variability of column-integrated aerosol optical properties in the southern Arabian Gulf and United Arab Emirates in summer, J. Geophys. Res.-Atmos., 113, D01204, https://doi.org/10.1029/2007JD008944, 2008.
Giles, D. M., Holben, B. N., Eck, T. F., Sinyuk, A., Smirnov, A., Slutsker, I., Dickerson, R. R., Thompson, A. M., and Schsfer, J. S.: An analysis of AERONET aerosol absorption properties and classifications representative of aerosol source regions, J. Geophys. Res., 117, D17203, https://doi.org/10.1029/2012JD018127, 2012.
Giles, D. M., Sinyuk, A., Sorokin, M. G., Schafer, J. S., Smirnov, A., Slutsker, I., Eck, T. F., Holben, B. N., Lewis, J. R., Campbell, J. R., Welton, E. J., Korkin, S. V., and Lyapustin, A. I.: Advancements in the Aerosol Robotic Network (AERONET) Version 3 database – automated near-real-time quality control algorithm with improved cloud screening for Sun photometer aerosol optical depth (AOD) measurements, Atmos. Meas. Tech., 12, 169–209, https://doi.org/10.5194/amt-12-169-2019, 2019.
Haywood, J. and Boucher, O.: Estimates of the direct and indirect radiative forcing due to tropospheric aerosols: A review, Rev. Geophys., 38, 513–543, 2000.
Holben, B. N., Eck, T. F., Slutsker, I., Tanre, D., Buis, J. P., Setzer A., Vermore, E., Reagan, J. A., Kaufman, Y. J., Nakajima, T., Lavenu, F., Jankowiak, I., and Smirnov, A.: AERONET- a federal instrument network and data archive for aerosol characterization, Remote Sens. Environ., 66, 1–16, 1998.
Holben, B. N., Kim, J., Sano, I., Mukai, S., Eck, T. F., Giles, D. M., Schafer, J. S., Sinyuk, A., Slutsker, I., Smirnov, A., Sorokin, M., Anderson, B. E., Che, H., Choi, M., Crawford, J. H., Ferrare, R. A., Garay, M. J., Jeong, U., Kim, M., Kim, W., Knox, N., Li, Z., Lim, H. S., Liu, Y., Maring, H., Nakata, M., Pickering, K. E., Piketh, S., Redemann, J., Reid, J. S., Salinas, S., Seo, S., Tan, F., Tripathi, S. N., Toon, O. B., and Xiao, Q.: An overview of mesoscale aerosol processes, comparisons, and validation studies from DRAGON networks, Atmos. Chem. Phys., 18, 655–671, https://doi.org/10.5194/acp-18-655-2018, 2018.
Holzer-Popp, T., de Leeuw, G., Griesfeller, J., Martynenko, D., Klüser, L., Bevan, S., Davies, W., Ducos, F., Deuzé, J. L., Graigner, R. G., Heckel, A., von Hoyningen-Hüne, W., Kolmonen, P., Litvinov, P., North, P., Poulsen, C. A., Ramon, D., Siddans, R., Sogacheva, L., Tanre, D., Thomas, G. E., Vountas, M., Descloitres, J., Griesfeller, J., Kinne, S., Schulz, M., and Pinnock, S.: Aerosol retrieval experiments in the ESA Aerosol_cci project, Atmos. Meas. Tech., 6, 1919–1957, https://doi.org/10.5194/amt-6-1919-2013, 2013.
Jacobson, M. Z.: Strong radiative heating due to the mixing state of black carbon in atmospheric aerosols, Nature, 409, 695–697, 2001.
Jethva, H., Torres, O., and Ahn, C.: Global assessment of OMI aerosol single-scattering albedo using ground-based AERONET inversion, J. Geophys. Res.-Atmos., 119, 9020–9040, https://doi.org/10.1002/2014JD021672, 2014.
Kahn, R. A., Gaitley, B. J., Garay, M. J., Diner, D. J., Eck, T. F., Smirnov, A., and Holben, B. N.: Multiangle Imaging SpectroRadiometer global aerosol product assessment by comparison with the Aerosol Robotic Network, J. Geophys. Res., 115, D23209, https://doi.org/10.1029/2010JD014601, 2010.
Kirchstetter, T. W. and Thatcher, T. L.: Contribution of organic carbon to wood smoke particulate matter absorption of solar radiation, Atmos. Chem. Phys., 12, 6067–6072, https://doi.org/10.5194/acp-12-6067-2012, 2012.
Kirchstetter, T. W., Novakov, T., and Hobbs, P. V.: Evidence that the spectral dependence of light absorption by aerosols is affected by organic carbon, J. Geophys. Res.-Atmos., 109, D21208, https://doi.org/10.1029/2004JD004999, 2004.
Korkin, S., Lyapustin, A., Sinyuk, A., Holben, B. N., and Kokhanovsky, A.: Vector radiative transfer code SORD: Performance analysis and quick start guide, J. Quant. Spectrosc. Radiat. Transfer., 200, 295–310, 2017.
Levy, R. C., Mattoo, S., Munchak, L. A., Remer, L. A., Sayer, A. M., Patadia, F., and Hsu, N. C.: The Collection 6 MODIS aerosol products over land and ocean, Atmos. Meas. Tech., 6, 2989–3034, https://doi.org/10.5194/amt-6-2989-2013, 2013.
Levy, R. C., Munchak, L. A., Mattoo, S., Patadia, F., Remer, L. A., and Holz, R. E.: Towards a long-term global aerosol optical depth record: applying a consistent aerosol retrieval algorithm to MODIS and VIIRS-observed reflectance, Atmos. Meas. Tech., 8, 4083–4110, https://doi.org/10.5194/amt-8-4083-2015, 2015.
Lewis, K., Arnott, W. P., Moosmuller, H., and Wold, C. E.: Strong spectral variation of biomass smoke light absorption and single scattering albedo observed with a novel dual-wavelength photoacoustic instrument, J. Geophys. Res., 113, D16203, https://doi.org/10.1029/2007JD009699, 2008.
Li, Z., Blarel, L., Podvin, T., Goloub, P., Buis, J.-P., and Morel, J.-P.: Transferring the calibration of direct solar irradiance to diffuse-sky radiance measurements for CIMEL Sun-sky radiometers, Appl. Optics, 47, 1368–1377, 2008.
Limbacher, J. A. and Kahn, R. A.: Updated MISR over-water research aerosol retrieval algorithm – Part 2: A multi-angle aerosol retrieval algorithm for shallow, turbid, oligotrophic, and eutrophic waters, Atmos. Meas. Tech., 12, 675–689, https://doi.org/10.5194/amt-12-675-2019, 2019.
Lyapustin, A., Wang, Y., Korkin, S., and Huang, D.: MODIS Collection 6 MAIAC algorithm, Atmos. Meas. Tech., 11, 5741–5765, https://doi.org/10.5194/amt-11-5741-2018, 2018.
Mok, J., Krotkov, N. A., Arola, A., Torres, O., Jethva, H., Andrade, M., Labow, G., Eck, T. F., Li, Z., Dickerson, R. R., Stenchikov, G. L., Osipov, S., and Ren, X.: Impact of brown carbon from biomass burning on surface UV and ozone photochemistry in the Amazon Basin, Sci. Rep., 6, 36940, https://doi.org/10.1038/srep36940, 2016.
Myhre, G., Samset, B. H., Schulz, M., Balkanski, Y., Bauer, S., Berntsen, T. K., Bian, H., Bellouin, N., Chin, M., Diehl, T., Easter, R. C., Feichter, J., Ghan, S. J., Hauglustaine, D., Iversen, T., Kinne, S., Kirkevåg, A., Lamarque, J.-F., Lin, G., Liu, X., Lund, M. T., Luo, G., Ma, X., van Noije, T., Penner, J. E., Rasch, P. J., Ruiz, A., Seland, Ø., Skeie, R. B., Stier, P., Takemura, T., Tsigaridis, K., Wang, P., Wang, Z., Xu, L., Yu, H., Yu, F., Yoon, J.-H., Zhang, K., Zhang, H., and Zhou, C.: Radiative forcing of the direct aerosol effect from AeroCom Phase II simulations, Atmos. Chem. Phys., 13, 1853–1877, https://doi.org/10.5194/acp-13-1853-2013, 2013.
Reid, J. S., Reid, E. A., Walker, A., Piketh, S., Cliff, S., Al Mandoos, A., Tsay, S. C., and Eck, T. F.: Dynamics of southwest Asian dust particle size characteristics with implications for global dust research, J. Geophys. Res.-Atmos., 113, D14212, https://doi.org/10.1029/2007JD009752, 2008.
Remer, L. A., Kaufman, Y. J., Tanr , D., Mattoo, S., Chu, D. A., Martins, J. V., Li, R., Ichoku, C., Levy, R. C., Kleidman, R. G., Eck, T. F., Vermote, E., and Holben, B. N.: The MODIS Aerosol Algorithm, Products, and Validation, J. Atmos. Sci., 62, 947–973, https://doi.org/10.1175/JAS3385.1, 2005.
Sayer, A. M., Hsu, N. C., Lee, J., Bettenhausen, C., Kim, W. V., and Smirnov, A.: Satellite Ocean Aerosol Retrieval (SOAR) algorithm extension to S-NPP VIIRS as part of the “Deep Blue” aerosol project, J. Geophys. Res.-Atmos., 123, 380–400, https://doi.org/10.1002/2017JD027412, 2018.
Sayer, A. M., Hsu, N. C., Lee, J., Kim, W. V., and Dutcher, S. T.: Validation, stability, and consistency of MODIS collection 6.1 and VIIRS version 1 Deep Blue aerosol data over land, J. Geophys. Res.-Atmos., 124, 4658–4688, https://doi.org/10.1029/2018JD029598, 2019.
Schafer, J. S., Eck, T. F., Holben, B. N., Thornhill, K. L., Anderson, B. E., Sinyuk, A., Giles, D. M., Winstead, E. L., Ziemba, L. D., Beyersdorf, A. J., Kenny, P. R., Smirnov, A., and Slutsker, I.: Intercomparison of aerosol single scattering albedo derived from AERONET surface radiometers and LARGE in situ aircraft profiles during the 2011 DRAGON-MD and DISCOVER-AQ experiments, J. Geophys. Res.-Atmos., 119, 7439–7452, https://doi.org/10.1002/2013JD021166, 2014.
Sinyuk, A., Holben, B. N., Eck, T. F., Giles, D. M., Slutsker, I., Korkin, S., Schafer, J. S., Smirnov, A., Sorokin, M., and Lyapustin, A.: The AERONET Version 3 aerosol retrieval algorithm, associated uncertainties and comparisons to Version 2, Atmos. Meas. Tech., 13, 3375–3411, https://doi.org/10.5194/amt-13-3375-2020, 2020.
Torres, O., Bhartia, P. K., Herman, J. R., Ahmod, Z., and Gleason, J.: Derivation of aerosol properties from satellite measurements of backscattered ultraviolet radiation: Theoretical basis, J. Geophys. Res., 103, 17099–17110, 1998.
Twomey, S.: Introduction to mathematics of inversion in in remote sensing and indirect measurements, Elsevier Inc., ISBN 9781483289564, 1977.
Ward, D. E., Hao, W. M., Susott, R. A., Babbitt, R. E., Shea, R. W., Kaufman, J. B., and Justice, C. O.: Effect of fuel composition on combustion efficiency and emission factors for African savanna ecosystems, J. Geophys. Res., 101, 23569–23576, 1996.
Werdell, P. J., Behrenfeld, M. J., Bontempi, P. S., Boss, E., Cairns, B., Davis, G. T., Franz, B. A., Gliese, U. B., Gorman, E. T., Hasekamp, O., Knobelspiesse, K. D., Mannino, A., Martins, J. V., McClain, C. R., Meister, G., and Remer, L. A.: The Plankton, Aerosol, Cloud, ocean Ecosystem (PACE) mission: Status, science, advances, B. Am. Meteorol. Soc., 100, 1775–1794, https://doi.org/10.1175/BAMS-D-18-0056.1, 2019.
Wong, J. P. S., Tsagkaraki, M., Tsiodra, I., Mihalopoulos, N., Violaki, K., Kanakidou, M., Sciare, J., Nenes, A., and Weber, R. J.: Atmospheric evolution of molecular-weight-separated brown carbon from biomass burning, Atmos. Chem. Phys., 19, 7319–7334, https://doi.org/10.5194/acp-19-7319-2019, 2019.
- Abstract
- Introduction
- Theory
- Effect of relaxed smoothness constraints for the IPRI on SSA retrievals
- Comparison of aerosol parameters retrieved using STD and REL assumptions from four-channel inversion
- Comparison of SSA retrieved using STD and REL assumptions to in situ measurements
- Summary and conclusions
- Appendix A: List of abbreviations used in paper
- Code availability
- Data availability
- Author contributions
- Competing interests
- Disclaimer
- Acknowledgements
- Review statement
- References
- Abstract
- Introduction
- Theory
- Effect of relaxed smoothness constraints for the IPRI on SSA retrievals
- Comparison of aerosol parameters retrieved using STD and REL assumptions from four-channel inversion
- Comparison of SSA retrieved using STD and REL assumptions to in situ measurements
- Summary and conclusions
- Appendix A: List of abbreviations used in paper
- Code availability
- Data availability
- Author contributions
- Competing interests
- Disclaimer
- Acknowledgements
- Review statement
- References