the Creative Commons Attribution 4.0 License.
the Creative Commons Attribution 4.0 License.
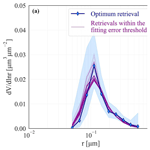
Combined sun-photometer–lidar inversion: lessons learned during the EARLINET/ACTRIS COVID-19 campaign
Anna Gialitaki
Marco Di Paolantonio
Davide Dionisi
Gian Luigi Liberti
Alnilam Fernandes
Artur Szkop
Aleksander Pietruczuk
Daniel Pérez-Ramírez
Maria J. Granados Muñoz
Juan Luis Guerrero-Rascado
Lucas Alados-Arboledas
Diego Bermejo Pantaleón
Juan Antonio Bravo-Aranda
Anna Kampouri
Eleni Marinou
Vassilis Amiridis
Michael Sicard
Adolfo Comerón
Constantino Muñoz-Porcar
Alejandro Rodríguez-Gómez
Salvatore Romano
Maria Rita Perrone
Xiaoxia Shang
Mika Komppula
Rodanthi-Elisavet Mamouri
Argyro Nisantzi
Diofantos Hadjimitsis
Francisco Navas-Guzmán
Alexander Haefele
Dominika Szczepanik
Artur Tomczak
Iwona S. Stachlewska
Livio Belegante
Doina Nicolae
Kalliopi Artemis Voudouri
Dimitris Balis
Athena A. Floutsi
Holger Baars
Linda Miladi
Nicolas Pascal
Oleg Dubovik
Anton Lopatin
The European Aerosol Research Lidar Network (EARLINET), part of the Aerosols, Clouds and Trace gases Research Infrastructure (ACTRIS), organized an intensive observational campaign in May 2020, with the objective of monitoring the atmospheric state over Europe during the COVID-19 lockdown and relaxation period. Besides the standard operational processing of the lidar data in EARLINET, for seven EARLINET sites having collocated sun-photometric observations in the Aerosol Robotic Network (AERONET), a network exercise was held in order to derive profiles of the concentration and effective column size distributions of the aerosols in the atmosphere, by applying the GRASP/GARRLiC (from Generalized Aerosol Retrieval from Radiometer and Lidar Combined data – GARRLiC – part of the Generalized Retrieval of Atmosphere and Surface Properties – GRASP) inversion algorithm. The objective of this network exercise was to explore the possibility of identifying the anthropogenic component and of monitoring its spatial and temporal characteristics in the COVID-19 lockdown and relaxation period. While the number of cases is far from being statistically significant so as to provide a conclusive description of the atmospheric aerosols over Europe during this period, this network exercise was fundamental to deriving a common methodology for applying GRASP/GARRLiC to a network of instruments with different characteristics. The limits of the approach are discussed, in particular the missing information close to the ground in the lidar measurements due to the instrument geometry and the sensitivity of the GRASP/GARRLiC retrieval to the settings used, especially for cases with low aerosol optical depth (AOD) like the ones we show here. We found that this sensitivity is well-characterized in the GRASP/GARRLiC products, since it is included in their retrieval uncertainties.
- Article
(12179 KB) - Full-text XML
-
Supplement
(6787 KB) - BibTeX
- EndNote
Preventive measures, mainly associated with public lockdowns and traffic restrictions, were imposed on a worldwide scale in an attempt to regulate the spread of the COVID-19 pandemic in early 2020. According to the World Health Organization (WHO, 2021), the reduction in anthropic activities affected primary pollutant emissions and hence air quality on a global scale (Venter et al., 2020; Chossière et al., 2021). This unprecedented situation provided the opportunity to study changes in atmospheric composition with respect to the anthropogenic aerosol component, which includes the aerosol particles originating from transportation, domestic heating, industrial facilities and long-range inter-continental transport, among others.
The European Aerosol Research Lidar Network (EARLINET; Pappalardo et al., 2014; Schneider et al., 2000) organized the EARLINET/ACTRIS COVID-19 campaign (https://www.earlinet.org/index.php?id=covid-19, last access: 4 December 2023), with the aim to monitor the atmospheric state over Europe during the COVID-19 lockdown and relaxation period in May 2020. EARLINET is part of the Aerosols, Clouds and Trace gases Research Infrastructure (ACTRIS; https://www.actris.eu/, last access: 4 December 2023) and consists of 33 permanent station members that perform regular lidar measurements, following a predefined schedule for measurements, along with quality-assurance procedures, as these are established by the network. Moreover, the network performs measurements during special atmospheric circumstances (e.g. dust transport; Pappalardo et al., 2009) and/or alerts on hazardous situations (e.g. volcanic eruptions; Pappalardo et al., 2013; Papagiannopoulos et al., 2020). During the 1-month period of the EARLINET/ACTRIS COVID-19 campaign, the network demonstrated its capability to provide the vertical profiling of aerosols and clouds using multi-wavelength lidar measurements, in near real time. In addition to the active remote sensing measurements, passive remote sensing measurements from sun photometers were also acquired during the campaign. The complementary use of active and passive remote sensing sensors has been proven to provide additional capabilities to provide more accurate aerosol profiling and characterization (e.g. Lopatin et al., 2013).
Taking advantage of the data collected during the EARLINET/ACTRIS COVID-19 campaign, a subset of stations with collocated lidar and sun photometers used the synergy of both types of observations in order to investigate the possible reduction in aerosols from anthropogenic activities above Europe due to the COVID-19 lockdown. The characterization of the anthropogenic aerosol component using remote sensing techniques is a challenging task. This is mainly due to the small load of anthropogenic particles in non-heavily polluted areas and/or their mixture with aerosols originating from natural sources, such as windblown dust, marine aerosols or transported smoke. Lidars are the only instruments that can provide detailed vertically resolved profiles of aerosol properties (e.g. Ansmann and Müller, 2005). However, in the lowest part of the planetary boundary layer (PBL) (Kotthaus et al., 2023) where most of the anthropogenic aerosols reside, the detection capabilities of ground-based lidars suffer due to the instrument geometry (Chen et al., 2014; Navas-Guzmán et al., 2011; Wandinger and Ansmann, 2002).
Several lidar studies have highlighted the difficulty of distinguishing between aerosols associated with anthropogenic activities and other species, mainly due to similarities in the optical properties measured by lidars (e.g. Burton et al., 2012, 2013; Groß et al., 2013; Nicolae et al., 2018; Papagiannopoulos et al., 2018). For example, anthropogenic aerosols may be misclassified as biomass burning due to their similar particle extinction-to-backscatter ratio (lidar ratio; S) and Ångström exponent (Å). In Müller et al. (2007) and in Janicka et al. (2017), the spectral dependence of S between 355 and 532 nm was used to distinguish between biomass burning and anthropogenic particles, an option not available when using single-wavelength lidar systems or lidar systems that provide extinction measurements only at a single wavelength. The separation of anthropogenic aerosols and large dust particles can be less complicated when depolarization lidar measurements are available due to the strong depolarization signature of dust (Ansmann et al., 2012; Sugimoto and Lee, 2006; Tesche et al., 2009). Although fine dust presents lower depolarization values (Järvinen et al., 2016; Sakai et al., 2010; Szczepanik et al., 2021), they are still higher than the depolarization values of anthropogenic particles.
The synergy of lidar with sun-photometer measurements provides more advanced retrievals for profiles of particle microphysical properties, as shown for the Lidar-Radiometer Inversion Code (LIRIC) in Chaikovsky et al. (2016), and for the Generalized Aerosol Retrieval from Radiometer and Lidar Combined data (GARRLiC), part of the Generalized Retrieval of Atmosphere and Surface Properties (GRASP), in Lopatin et al. (2013, 2021). Being applied to (most commonly) three-wavelength elastic backscatter lidar data, these algorithms can separate fine and coarse species (LIRIC provides additional separation of spherical and non-spherical coarse particles, though with a common refractive index for all species). Tsekeri et al. (2017) have shown the limitations of these algorithms in complex atmospheric scenes containing anthropogenic particles along with marine and dust particles. A common practice to derive a better characterization is to employ other types of observations (e.g. in situ measurements) or atmospheric models to support the lidar, sun-photometer or combined lidar–sun-photometer analysis. For instance, in Deleva et al. (2019), forecast models and air-mass backward trajectories were used, complementarily to lidar observations, to exclude the possibility of other aerosol types (i.e. dust or smoke) being mixed with a persistent layer of urban pollution found above Sofia, Bulgaria. Newly developed aerosol classification algorithms take this synergy a step further by also making use of land-surface coverage and additional satellite information of active fire regions to avoid the inherent ambiguity in aerosol characterization based on optical properties alone (Mylonaki et al., 2021; Radenz et al., 2021).
Herein, we use a similar synergistic approach in order to derive the microphysical properties of the anthropogenic aerosol component in different European areas. First, we ensure the absence of natural aerosols in the atmospheric scenes: for excluding cases with transported (fine) dust particles, we use lidar depolarization measurements along with air-mass back-trajectory and emission sensitivity analysis. For excluding smoke cases, we utilize a combination of model runs and satellite images in order to identify air masses that were affected by the presence of active fires. Then, we utilize the synergy of lidar and sun-photometer measurements in the advanced inversion scheme of GRASP/GARRLiC that provides columnar and vertically resolved optical and microphysical properties of fine and coarse particles separately. In the absence of natural aerosols in the atmospheric scenes, the retrieved fine particles contain only anthropogenic aerosols. Due to the low aerosol optical depth (AOD) during the campaign, the retrieval uncertainty was found to be high in some cases, particularly for the complex refractive index (Lopatin et al., 2013). Hence, herein we mainly focus on the retrieval of the columnar size distribution and the concentration profile of the anthropogenic particles, excluding the retrieved complex refractive index from our results.
While the objective of the EARLINET/ACTRIS COVID-19 campaign was the monitoring of possible changes in the load and properties of aerosols from anthropogenic activities, the objective of this study is the derivation of a common methodology for applying the GRASP/GARRLiC algorithm to a network of different systems (with different configurations), along with the identification of the issues that should be solved to optimize this process. Coordinated observational activities within the framework of EARLINET have also been organized in the past as part of near-real-time operability demonstrations (D'Amico et al., 2015; Sicard et al., 2015) or during extreme events, such as the Eyjafjallajökull volcano eruption in 2010 (Ansmann et al., 2012; Pappalardo et al., 2013; Sicard et al., 2012). However, these efforts provided only the optical properties of the particles. The present study is – to the best of our knowledge – one of the very few coordinated efforts on the European network level to provide concentration profiles and column-integrated microphysical properties of aerosol particles in the atmosphere (another example is provided in the work of Granados-Muñoz et al., 2016).
The study is organized as follows: in Sect. 2 we present a brief overview of the measurement strategy during the EARLINET/ACTRIS COVID-19 campaign, along with the geographical distribution and the characteristics of the participating EARLINET stations. In Sect. 3 we present the lidar and sun-photometer measurements used to derive the microphysical properties of the anthropogenic particles, along with the atmospheric transport models used to verify the absence of natural aerosols, and the GRASP/GARRLiC retrieval algorithm. Section 4 presents the methodology for applying the GRASP/GARRLiC algorithm on a network level. The retrieved microphysical properties of the anthropogenic particles are presented in Sect. 5. Section 6 presents the issues identified from running GRASP/GARRLiC on a network level, and Sect. 7 summarizes this work and presents the conclusions.
The intensive observational EARLINET/ACTRIS COVID-19 campaign was the result of an EARLINET initiative to detect possible changes in atmospheric composition and atmospheric aerosol load during the COVID-19 pandemic outbreak in Europe. Twenty-one EARLINET stations participated (Fig. 1), performing measurements continuously or twice per day (at 12:00 and 20:00 UTC for 2 h intervals). All stations operated automatic and/or remotely controlled instruments. The lidar measurements were processed and Level-1 and Level-2 data were provided in near real time (ACTRIS ARES Data Centre, 2020b). The Level-1 data include the attenuated backscatter (i.e. the calibrated range-corrected lidar signal), and the Level-2 data include the particle backscatter and extinction coefficients. Potential critical issues of the lidar systems, as well as quality assurance of the signals, were assessed prior to the campaign, using standard quality-assurance procedures applied within EARLINET (Freudenthaler et al., 2018; Belegante et al., 2018). Sun-photometer measurements and related products for aerosol optical and microphysical columnar properties were provided by the global sun-photometric Aerosol Robotic Network (AERONET; Holben et al., 1998).
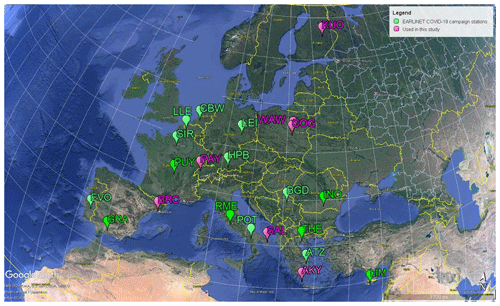
Figure 1The 21 EARLINET stations that participated in the COVID-19 campaign. The stations that were used for the characterization of the anthropogenic aerosol component in Europe are shown in pink, and the rest of the stations are in green. Payerne station data were also used in the present analysis, though the station did not participate in the campaign. The three-letter code name of each station can be found on the EARLINET web page: https://www.earlinet.org/index.php?id=105 (last access: 4 December 2023). Map created using © Google Earth.
In the present analysis we used measurements from 6 out of the 21 EARLINET stations participating in the campaign (pink symbols in Fig. 1) and Payerne station (EARLINET station not participating in the campaign) based on the following:
-
the collocation criteria of the sun-photometer and lidar measurements (i.e. a maximum of a 30 min time difference and 1 km spatial difference between the sun-photometer and lidar measurements);
-
the full-overlap height of the lidar measurements (different for each system, as shown in Table 3, with a maximum value of 1 km), which marks the lowest height of trustworthy profiles, since below this height the lidar backscattered signal is only partially collected from the receiving telescope (e.g. Wandinger and Ansmann, 2002).
A list with the characteristics of the stations participating with data in the current study is given in Table 1, including the EARLINET and AERONET station names, the latitude and longitude, the altitude, and the type of surrounding area (i.e. rural, suburban, urban, background and industrial).
Table 1EARLINET and AERONET stations used for the characterization of the anthropogenic aerosol component in Europe, during the EARLINET COVID-19 campaign: name, location (lat, long), altitude above sea level (a.s.l.) and type of surrounding area.
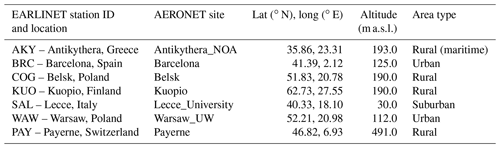
Regarding the selected cases, we first selected all cloud-free cases for which the sun-photometer and lidar measurements had ±30 min difference, and we filtered out the cases with a presence of fine natural aerosol particles in the atmosphere (i.e. smoke and dust particles). For excluding cases with transported (fine) dust particles, we used the modelling tools described in Sect. 3.3, along with lidar measurements of the volume and particle linear depolarization ratio (VLDR and PLDR, respectively), indicating non-spherical particles (i.e. dust particles), as discussed in Sect. 3.1 (VLDR takes into account both molecules and aerosol particles in the atmosphere, whereas PLDR takes into account only the aerosol particles). For excluding smoke cases, we identified air masses that were affected by the presence of active fires by examining the back trajectories of the air masses (Sect. 3.3) in combination with the “Fires and Thermal Anomalies” product from the VIIRS instrument on board the Suomi NPP satellite. As shown in Table 2, the selected cases that contain mostly anthropogenic particles comprise 40 % of the available cloud-free cases for the EARLINET stations used in the analysis.
Table 2The total number of measurements during the EARLINET/ACTRIS COVID-19 campaign and the selected cases presented in this study: the total number of cases with Level-2.0 (quality assured) lidar backscatter profiles in EARLINET database; the total number of sun-photometer Level-1.5 inversions from the AERONET database; cloud-free lidar–sun-photometer collocated cases; and selected cases, containing mostly anthropogenic particles.
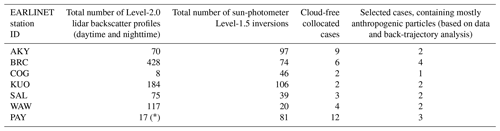
* Only Level-1.0 profiles.
3.1 Lidar data
EARLINET lidars used in this study employ Nd:YAG lasers that emit radiation pulses at a low repetition rate (usually 20 Hz), recording the light backscattered from the constituents of the atmosphere (i.e. molecules, aerosols, clouds). The outgoing laser radiation is linearly polarized and emitted simultaneously at multiple wavelengths (i.e. 355, 532, 1064 nm), as shown in Table 3. Moreover, many of those systems also employ Raman extinction channels at 387 and 607 nm to measure light backscattered from N2 molecules, and they provide profiles of the particle extinction coefficient at 355 and 532 nm, respectively (Ansmann et al., 1990). Finally, the cross-polarized backscattered light (with respect to the emitted light polarization) is typically recorded at 355 and/or 532 nm, to derive profiles of the VLDR and PLDR. These profiles are used for identifying non-spherical particles in the atmospheric column (e.g. dust particles), which depolarize the backscattered laser light. Table 3 also reports the operation mode of the lidars during the campaign (i.e. continuous or measuring twice per day) and the system full-overlap height.
Table 3Characteristics of the lidar and sun-photometer measurements used for the characterization of the anthropogenic aerosol component in Europe, during the EARLINET/ACTRIS COVID-19 campaign: EARLINET station ID, lidar name, operation mode, wavelength of measured backscattered light, wavelength of Raman measurements (for extinction products), depolarization measurements, full-overlap height above ground level (a.g.l.) and wavelengths of sun-photometer measurements. The specific wavelengths used for the GRASP/GARRLiC analysis for each station are highlighted in bold.
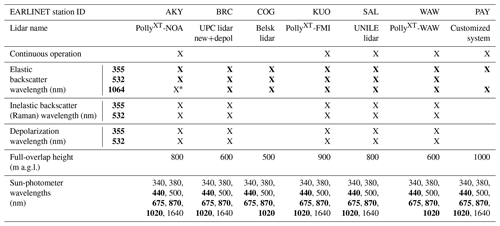
* Not operational during the campaign.
An example of the lidar measurements is shown for the continuous measurements provided by the PollyXT-NOA lidar located at the Antikythera EARLINET station during the period of the campaign. Specifically, the attenuated backscatter coefficient at 532 nm is shown in Fig. 2a, and the VLDR at 532 nm is shown in Fig. 2b. The VLDR profile was used in our analysis along with atmospheric transport model simulations to identify dust layers in the column (Fig. 2b).
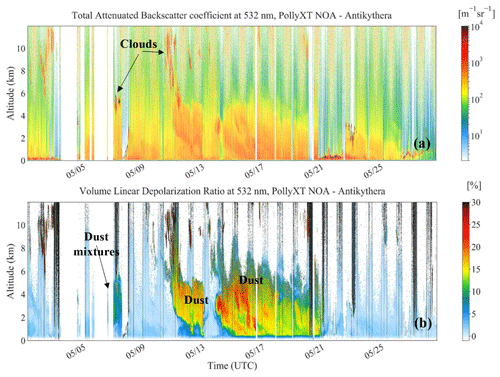
Figure 2Time–height plots of continuous lidar measurements from the PollyXT-NOA lidar at the Antikythera (AKY) EARLINET station in Greece, for the whole period of the EARLINET/ACTRIS COVID-19 campaign (May 2020). (a) Attenuated backscatter coefficient at 532 nm. (b) VLDR at 532 nm. Altitude is provided in kilometres above sea level. The time format along the x axes is month/day.
In this study, we utilize two types of EARLINET lidar product:
- a.
Level-1 data of the pre-processed, range-corrected signal at 355, 532 and 1064 nm, used as inputs in the GRASP/GARRLiC algorithm (Sect. 4.1);
- b.
Level-2 data of the particle backscatter and extinction coefficients at 355, 532 and 1064 nm, used for comparison with the corresponding products from the GRASP/GARRLiC algorithm (Sect. 4.2).
The majority of the Level-1 and Level-2 lidar products are processed with the Single Calculus Chain (SCC) algorithm (D'Amico et al., 2016; Mattis et al., 2016). The SCC is an automatic analysis tool for lidar data, developed within EARLINET, that ensures the homogeneity and quality of adverse lidar system data and facilitates the processing of lidar measurements in a fully automatic way. Only the observations from the Payerne station are processed with an in-house-developed algorithm, since the signals at 1064 nm were acquired with a ceilometer. To ensure sufficient noise suppression in the lidar signals, a time interval of 2 h and a vertical smoothing window of 60 m are selected for temporal and spatial averaging, respectively. Data with a low signal-to-noise ratio (i.e. relative error of the lidar signals >50 %) are not used in the current analysis.
The Level-1 data are derived by the SCC pre-processing module (ELPP module) that corrects the measured lidar signals for dead-time effects, trigger delay, background subtraction and overlap, as described in detail in D'Amico et al. (2015, 2016). The ELPP output files also contain the statistical uncertainty of the Level-1 data, along with the molecular atmosphere profiles, calculated using either radio-sounding or atmospheric numerical weather prediction models (e.g. from the Weather Research and Forecasting (WRF) Model; Marinou et al., 2019).
The Level-2 data are provided by the SCC ELDA module (Mattis et al., 2016) and include profiles of the particle backscatter and extinction coefficient (the latter is available mainly for nighttime measurements), along with the VLDR and PLDR (used as an indication of the presence of non-spherical particles in this analysis). The optical property profiles are typically calculated either with the Klett–Fernald method (Fernald, 1984; Klett, 1981) or with the iterative method (Di Girolamo et al., 1995) and for some lidars with the Raman method (Ansmann et al., 1990). The latter method is more frequently applied during the nighttime in the absence of strong background radiation. ELDA module also provides the statistical uncertainty in Level-2 data.
3.2 Sun-photometer data
The EARLINET stations that participated with measurements in this study were equipped with Cimel sun photometers, part of the global sun-photometer network AERONET. In the absence of clouds and during the daytime, Cimel sun photometers perform direct-sun irradiance measurements every 15 min and almucantar measurements of sky radiance every hour. Regular calibration procedures are employed within AERONET to assure high-accuracy retrievals, with residuals of 0.01 % and 5 % for direct-sun and sky-radiance measurements, respectively. Sun and sky radiances at 440, 675, 870 and 1020 nm are used to derive the AERONET products of the size distribution, complex refractive index and sphericity fraction of the aerosol particles along the atmospheric column (Dubovik and King, 2000; Dubovik et al., 2006). Additionally, the phase function, asymmetry factor, single-scattering albedo and absorption aerosol optical depth are provided at the same wavelengths.
The sun-photometer data used in the current study are as follows:
- a.
Level-1.5 data of direct-sun and sky-radiance measurements at 440, 675, 870 and 1020 nm, as input into the GRASP/GARRLiC retrieval (Sect. 4.1);
- b.
Level-1.5 data of the particle volume size distribution, for evaluating the corresponding results from the GRASP/GARRLiC retrieval (Sect. 4.2).
3.3 Atmospheric models
In order to identify the aerosol sources during the EARLINET/ACTRIS COVID-19 campaign, we used atmospheric model simulations that helped us, along with lidar measurements, to exclude fine natural aerosol particles (dust and smoke) and focus only on the anthropogenic aerosol component. This was done through the identification of the origin of the aerosol particles using the FLEXible PARTicle dispersion model (FLEXPART; Pisso et al., 2019), the BSC-DREAM dust model (Nickovic et al., 2001), and the Navy Aerosol Analysis and Prediction System (NAAPS). FLEXPART is a Lagrangian transport and dispersion model, which we used to identify the sources of the observed atmospheric layers, as shown for example in Fig. 4. The BSC-DREAM dust model predicts the atmospheric life cycles of desert dust. The NAAPS model produces forecasts of three-dimensional aerosol concentrations of sulfate, dust and smoke aerosols in the troposphere.
3.4 GRASP/GARRLiC retrieval algorithm
The GRASP/GARRLiC retrieval algorithm performs a statistically optimized inversion for both lidar and sun-photometer measurements, based on a multi-term least-squares method, also taking into account a priori information about the aerosol properties (Lopatin et al., 2013, 2021). An example of a priori knowledge is the minimum and maximum values of the retrieved parameters (derived from the physically expected range of values for the parameters of atmospheric aerosols (e.g. Dubovik et al., 2002); Table S2 in the Supplement). Another example is the smoothing constraints imposed on the retrieved volume size distributions or on the spectral variability in the retrieved refractive index. The input of GRASP/GARRLiC consists of the pre-processed, range-corrected, normalized lidar signals (see Appendix A), along with the total optical depth (TOD) and the sky radiances at 440, 675, 870 and 1020 nm acquired from the sun photometer. The lidar signals may be at one or more wavelengths; in this study we utilized the measurements at 355, 532 and 1064 nm wherever they were available (see Table 3).
Amongst others, the retrieved parameters include the columnar aerosol total volume size distribution; the columnar spectral complex refractive index at 440, 670, 870 and 1020 nm; and the profiles of aerosol concentration at 60 altitude levels (considering a homogeneous layer of constant concentration from the surface to the full-overlap lidar height), for both fine and coarse aerosol modes (see more details in Lopatin et al., 2013, 2021). Thus, for the fine-mode aerosols investigated here, the size distribution changes with height in terms of aerosol concentration, but its shape is constant with height. Moreover, the aerosols' spectral refractive index (i.e. composition) does not change with height. For each parameter the retrieval uncertainty is provided (Herrera et al., 2022) or can be calculated from GRASP/GARRLiC outputs (see Appendix B).
A limitation of the algorithm is the assumption of a fixed atmospheric profile (future versions of the algorithm are planned to properly address this issue). The fixed atmospheric profile may not adequately provide the backscatter and extinction profiles of the molecules in the atmosphere (i.e. similar issues are identified in Mona et al., 2009). Moreover, the gas absorption is also neglected within the channels. In this study only the elastic backscattering of the lidar signal is modelled (the GRASP algorithm provides the capability to utilize Raman and depolarization lidar signals as well).
4.1 Methodology for applying GRASP/GARRLiC on a network level
The methodology for applying the GRASP/GARRLiC algorithm on a network level is based on a two-step approach, first optimizing the parameters used to run the GRASP/GARRLiC retrieval and derive the “optimum retrieval” (Sect. 4.1.1) and then evaluating the robustness of the optimum retrieval through evaluating its sensitivity to the initial guess (Sect. 4.1.2).
4.1.1 First step: optimizing the setting parameters of GRASP/GARRLiC retrieval and deriving the optimum retrieval
In the first step of our methodology, we define the appropriate combination of the setting parameters for running the GRASP/GARRLiC retrieval. More specifically, we optimize the a priori smoothing constraints used in order to limit unrealistic oscillations (spikes) in the retrieved parameters. The smoothing is defined by (a) the order of finite difference of the function used to characterize the degree of non-linearity of (i) the volume size distribution, (ii) the spectral dependence of the refractive index and (iii) the height dependence of the concentration profile, for fine and coarse particles, and (b) the corresponding Lagrange multipliers that determine the contributions of the smoothing to the solution (Dubovik and King, 2000; Dubovik et al., 2011).
The definition of the optimum combination of the setting parameters is not a trivial task, and it may vary, e.g. for different atmospheric states. In the framework of this study we tried to derive a “global” optimum combination of the setting parameters that could be used for all cases, tailored to each EARLINET station. This analysis was not conclusive, in part due to the low AOD of the case studies available in the current analysis (with AOD values lower than 0.15 at 500 nm for fine particles), resulting in less robust retrievals. Thus, herein, we derive the optimum combination of the setting parameters for each case and for each EARLINET station separately. The derivation is done by running multiple retrievals using different combinations for the values of the a priori smoothing constraints. Table 4 shows the combinations of the values used for each a priori smoothing constraint for the case studies from the different stations. The range of values used is determined empirically for the specific cases so that the retrieved properties present various degrees of smoothing. Table S1 shows the optimum combination of the setting parameters selected for each case study. We should note that although we are interested in the retrieval of the fine mode, we need to optimize the smoothing constraints of the coarse mode as well, since they affect the retrieval of the fine-mode properties.
Table 4List of the a priori smoothing constraints and the corresponding range of values used for finding the optimum combination for the cases studied herein, for each EARLINET station. The selected optimum combination of the smoothing constraints is provided in Table S1. OFD is the order of finite difference, LM is the Lagrange multiplier, FM is the fine mode and CM is the coarse mode.
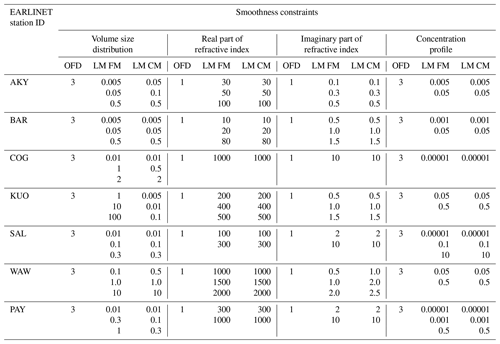
The “optimum combination” for the setting parameters is the one providing the optimum retrieval, considering the fitting of the measurements and the realistic values and oscillations of the retrieved parameters: the multiple GRASP/GARRLiC runs that are executed for each case using different combinations of the a priori smoothing constraints provide an ensemble of solutions, from which we first select the “acceptable solutions”. These are the solutions that have residuals within the uncertainty of the observations, i.e. 0.01 for TOD, 5 % for sky radiances and 30 %–50 % (depending on the station) for the lidar–ceilometer signals. (We should note that the uncertainty in the lidar observations is considered here to be constant with height, although in reality, the uncertainty is height-dependent.) The optimum retrieval is then selected from the ensemble of the acceptable solutions, based on the requirements to have the least possible smoothing imposed and present no unphysical oscillations in any of the retrieved parameters. The latter criterion cannot be strictly quantified and is evaluated in a purely qualitative way and thus is mostly subjective, based on scientific experience.
To demonstrate our methodology, we show here an example of a case study from the Antikythera station on 25 May 2020. Figure 3a shows the time–height evolution of the total attenuated backscatter coefficient at 532 nm, from 12:00 to 17:00 UTC, which shows that the majority of the aerosol load is found (from the ground) up to almost 4 km. The low PLDR values, ranging between 0.02 and 0.04 at 532 nm (not shown here), reveal that the particles are close to spherical; thus no dust particles are present. The FLEXPART source–receptor analysis in Fig. 4 shows that the air masses that arrive in Antikythera on this day follow north-eastern and north-western routes and that, for a total of 5 d preceding the observations, they mostly reside above urban areas and the sea. Consequently, it is expected that the atmospheric column consists of a mixture of marine and anthropogenic particles. The marine particles are considered to be mainly coarse particles and thus are expected to have a minimum effect on the load of the fine mode, which consists mainly of anthropogenic particles. According to the collocated sun-photometer measurements, the AOD at 500 nm does not exceed the value of 0.15 and the fine aerosol fraction is higher than 75 % (Fig. 3b). All of the above advocates for the presence of (mostly) fine-mode particles, which are considered here to be the anthropogenic component we aim to retrieve.
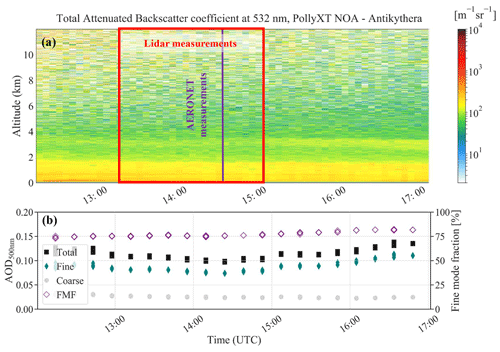
Figure 3(a) The total attenuated backscatter coefficient at 532 nm, for the case study at the Antikythera EARLINET station on 25 May 2020 at 12:00–17:00 UTC. Altitude is provided in kilometres above sea level. The time window of the lidar measurements used for the GRASP/GARRLiC retrieval presented herein is marked with the red rectangle. The time of the collocated measurements from the sun photometer used in the retrieval is shown with a purple line. (b) The collocated AOD at 500 nm for all particles (total; black squares), fine particles (green diamonds) and coarse particles (grey circles), provided by AERONET. The fine-mode fraction is also shown (purple diamonds).
The lidar measurements used as input for the GRASP/GARRLiC retrieval are time averages of the elastic lidar signals at 355 and 532 nm between 13:15 and 15:00 UTC (indicated with the red rectangle in Fig. 3a), achieving in this way sufficient noise suppression. The sky radiances and the TOD measurements of the sun photometer are taken at 14:25 UTC (purple line in Fig. 3a) in order to overlap with the lidar observations.
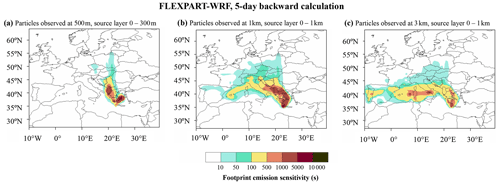
Figure 4The 5 d backward FLEXPART-WRF calculation of footprint emission sensitivity in seconds for the particles that arrived at Antikythera EARLINET station on 25 May 2020 (14:25 UTC) at receptor heights (a) 500 m, (b) 1 km and (c) 3 km. The footprint emission sensitivity represents the residence time – the total time an air parcel spent over a certain surface and below the reception height – and is a first hint of the type of the aerosol load of the air parcel. The longer an air parcel resides close to the surface, the more likely it will acquire the aerosol footprint of the surface.
We used the lidar and sun-photometer measurements from this case study, and we applied the first step of our methodology: we ran ∼ 2500 retrievals of GRASP/GARRLiC, using ∼ 2500 combinations of a priori smoothing constraints, with values shown for the case study AKY in Table 4. The retrievals that fitted the measurements within the uncertainty of the observations (i.e. 0.01 for TOD, 5 % for sky radiances and 30 % for the lidar signals) are considered the acceptable solutions for this case. Figure 5 shows an example of the fitting with GRASP/GARRLiC of the lidar and sun-photometer observations (i.e. the total optical depth, sky radiance and lidar signals) for one of the acceptable solutions (size distributions and concentration profiles), which is shown in Fig. 6. Finally, the optimum retrieval is selected from amongst the acceptable solutions, based on the requirement to have the least smoothing imposed and for the absence of unphysical oscillations in the retrieved parameters. The latter is evaluated in a purely qualitative way, as discussed above. The optimum retrieval for the volume size distribution and the concentration profile of the anthropogenic component at the Antikythera EARLINET station on 25 May 2020, along with the corresponding retrieval uncertainty, is shown in Fig. 6 (blue line). The values of the a priori smoothing constraints used for the optimum retrieval are provided in Table 5.
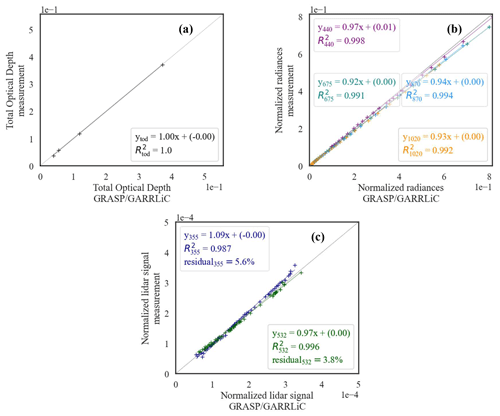
Figure 5Example of the GRASP/GARRLiC fitting of the lidar and sun-photometer observations, for the case study from the Antikythera station on 25 May 2020: (a) the TOD at 440, 675, 870 and 1020 nm; (b) the normalized sky radiances at 440, 675, 870 and 1020 nm; and (c) the normalized, range-corrected signals at 355 and 532 nm.
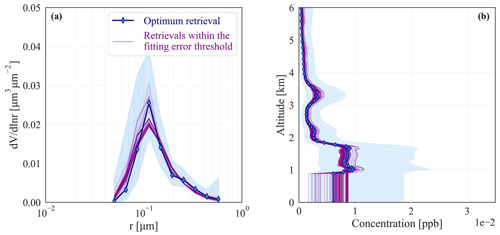
Figure 6The (a) volume size distribution and (b) volume concentration profile of the anthropogenic component for the case study from the Antikythera station on 25 May 2020 (altitude in km a.s.l.). For both plots, purple lines represent all the solutions derived with variable a priori smoothing constraints for which the retrieval residuals fall within the uncertainty of the measurements; thus they are acceptable solutions. The blue line represents the optimum solution, provided with its retrieval uncertainty (blue-shaded area).
Table 5The a priori smoothing constraints used for the retrieval of the volume size distribution, the spectral refractive index and the concentration profile of the anthropogenic component at the Antikythera station on 25 May 2020. OFD is the order of finite difference, LM is the Lagrange multiplier, fm is the fine mode and cm is the coarse mode.

A notable feature in Fig. 6 is that all of the acceptable solutions are within the retrieval uncertainty of the optimal solution. This indicates that the variability in the solutions due to the smoothing constraints used to run the GRASP/GARRLiC retrieval is already provided in the GRASP/GARRLiC product, as part of the retrieval uncertainty of the solution. This conclusion holds for all the case studies investigated herein.
4.1.2 Second step: sensitivity of the GRASP/GARRLiC optimum retrieval to initial guess
In the second step we examine the stability of the optimum solution with respect to the values adopted as the initial guess for the retrieval. Although this step does not constitute a thorough investigation of the stability of the retrieval, it provides a first quality check. Specifically, we generate ∼ 100 (random) initial guesses and we run the retrieval using the optimum combination of the a priori smoothing constraints defined in step 1. In order for the optimum solution to be characterized as stable, the retrievals performed with the random initial guesses should fall within its retrieval uncertainty. The range of values used for the initial guess of the different aerosol parameters (Table S2) covers the physically expected range of values for atmospheric aerosols (e.g. Dubovik et al., 2002). (Note that the minimum and maximum values shown in Table S2 are also used in the retrieval as a priori assumptions of the minimum and maximum values of the derived parameters.) Figure 7 shows the results of step 2 for the optimum solution for the case study from the Antikythera EARLINET station on 25 May 2020. All the retrievals that are calculated with different first guesses fall within the retrieval uncertainty of the optimum solution and thus verify its robustness.
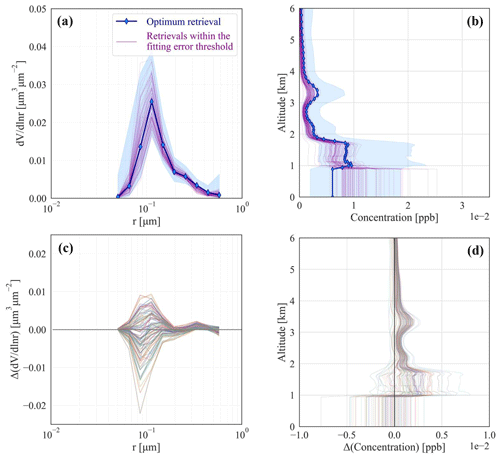
Figure 7Test for the robustness of the optimum solution in Fig. 6, for the case study from the Antikythera EARLINET station on 25 May 2020: (a) the size distribution and (b) the concentration profile of the anthropogenic component. The optimum solution for panels (a) and (b) is shown with blue, whereas the solutions calculated using different initial guesses are shown with purple. The retrieval uncertainty of the optimum solution is shown with blue shading. The absolute difference of each solution calculated with variable initial guesses, with respect to the optimum solution, is shown in panel (c) for the size distribution and panel (d) for the concentration profile. The altitude in concentration profiles is provided in kilometres above sea level.
An interesting feature in Fig. 7 is the absolute difference of each solution calculated with variable initial guesses, with respect to the optimum solution, for the concentration profile. As shown in Fig. 7d, the underestimation (overestimation) of the concentration profile within the lidar overlap region, due to the missing lidar information in this region, results in the overestimation (underestimation) of the concentration profile at higher heights. This highlights the fact that the algorithm tries to compensate for the over-/underestimation of the concentration profile below the overlap region and preserve a constant value for the total aerosol concentration. The latter is provided mainly from the sun-photometer measurements, which contain the information of the total column.
4.2 Comparison of GRASP/GARRLiC results with SCC and AERONET products
A qualitative evaluation of the retrieved microphysical and optical properties of the anthropogenic particles is done through their comparison with the corresponding products of the volume size distribution from AERONET and the extinction and backscatter coefficient profiles derived from SCC with the Klett method.
An example of the comparison of the retrieved volume size distribution with the AERONET product is provided for the case study from the Antikythera station on 25 May 2020 in Fig. 8, showing a good agreement within the retrieval uncertainty of the GRASP/GARRLiC product. In principle, the information content of lidar measurements on the aerosol size distribution is low compared to the one contained in the sky radiances measured from the sky–sun photometer. Therefore, we do not expect the addition of the lidar measurements in the retrieval to have a strong impact on the derived size distribution (this does not mean though that we expect no impact, as discussed in Sect. 5).
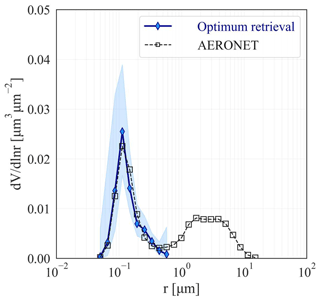
Figure 8Evaluation of the retrieved volume size distribution (blue line) of the anthropogenic component, with the AERONET volume size distribution (black line with squares), for the case study from the Antikythera EARLINET station on 25 May 2020.
Since we consider only daytime measurements, the SCC particle extinction coefficient profiles are calculated using the particle backscatter coefficient profiles derived from ELDA and a constant predefined lidar ratio (S) value, which is characteristic for each specific scene, ranging between 45 and 55 sr, with an uncertainty of 10 sr. The range of 35–65 sr covers most of the LR values reported for “pollution” aerosols at 355 and 532 nm, as shown in Floutsi et al. (2023). The extinction coefficient uncertainty is calculated through error propagation that includes both the uncertainty in the backscatter coefficient (provided directly by ELDA) and the assumed uncertainty in the predefined S value. The GRASP/GARRLiC backscatter and extinction coefficients are calculated from the retrieved microphysical properties, as shown in Eqs. (1) and (2), using the retrieved volume concentration profile (VC), AOD (τ) and lidar ratio (SG), for fine (f) (i.e. anthropogenic) and coarse (c) particles. Here the superscript G is used to denote GRASP/GARRLiC retrieved properties, z to denote altitude and λ to denote wavelength.
The uncertainty in the particle backscatter and extinction coefficients retrieved from GRASP/GARRLiC is calculated as shown in Appendix A.
Figure 9 provides an example comparison between the extinction and backscatter coefficient profiles of GRASP/GARRLiC and SCC products, for the case study from the Antikythera station on 25 May 2020. The comparison shows a good agreement within the retrieval uncertainties of the SCC and GRASP/GARRLiC products. Both the particle backscatter and extinction profiles at 355 nm exhibit larger differences compared to the respective profiles at 532 nm. This is expected since the molecular-scattering contribution is higher in the shorter wavelengths, and thus, the effect of an assumed constant atmospheric profile in the GRASP/GARRLiC retrieval is more prominent in the UV.
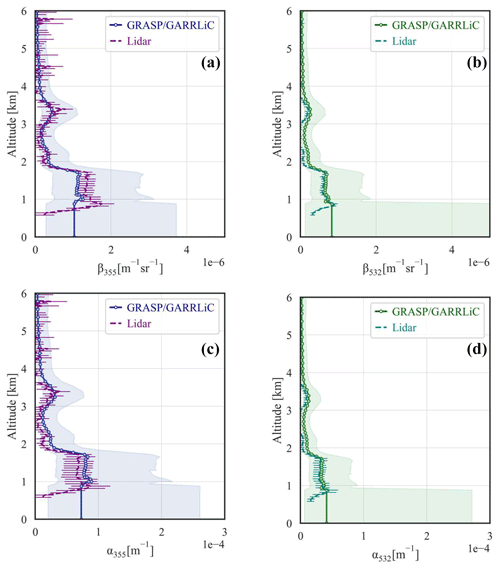
Figure 9Evaluation of the backscatter (a, b) and extinction (c, d) coefficient profiles at 355 and 532 nm, derived from GRASP/GARRLiC (solid lines with circles), against the SCC corresponding products (dashed lines), for the case study from the Antikythera EARLINET station on 25 May 2020, 13:15–15:00 UTC. The altitude is provided in kilometres above sea level.
The GRASP/GARRLiC retrievals of the volume size distribution and concentration profile of the anthropogenic component above Europe during the relaxation period of the COVID-19 lockdown are provided in Figs. 10 and 11, respectively. For the size distribution, the evaluation with the corresponding AERONET product is also included in each plot. The differences between GRASP/GARRLiC and AERONET retrievals are larger than 15 % (i.e. the retrieval uncertainty of the AERONET product provided by Dubovik et al. (2000) for water-soluble particles with radii in the range of 0.1–7 µm) for a number of cases analysed. Due to the low number of cases, the results are not statistically significant enough to characterize the atmospheric state over Europe during the COVID-19 lockdown and relaxation period. The results for the concentration profiles (Fig. 11) present a wide range of concentrations, along with large retrieval uncertainties. We should also note that they depend on the different overlap of the different lidar systems.
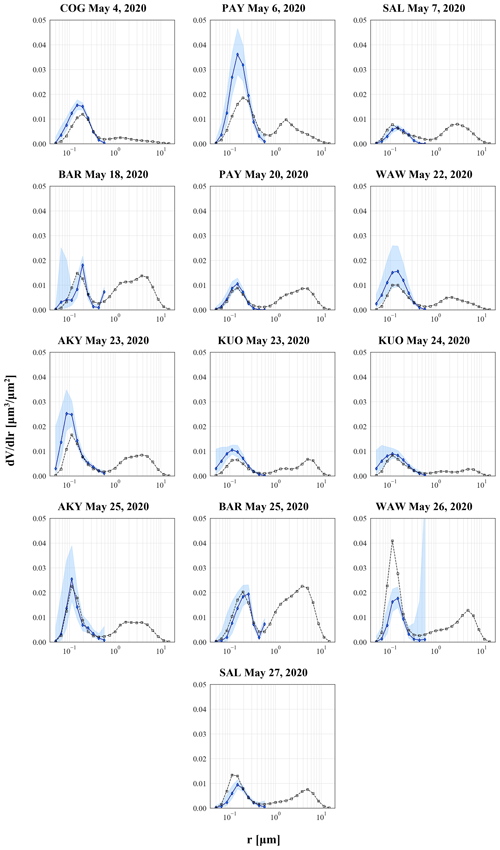
Figure 10The retrieved volume size distributions of the anthropogenic component over Europe (blue line), during the lockdown and relaxation period of May 2020 due to the COVID-19 pandemic. The shaded blue area represents the GRASP/GARRLiC retrieval uncertainty for the volume size distribution. The AERONET size distribution used for the evaluation of the results is also provided for each case (black line with squares).
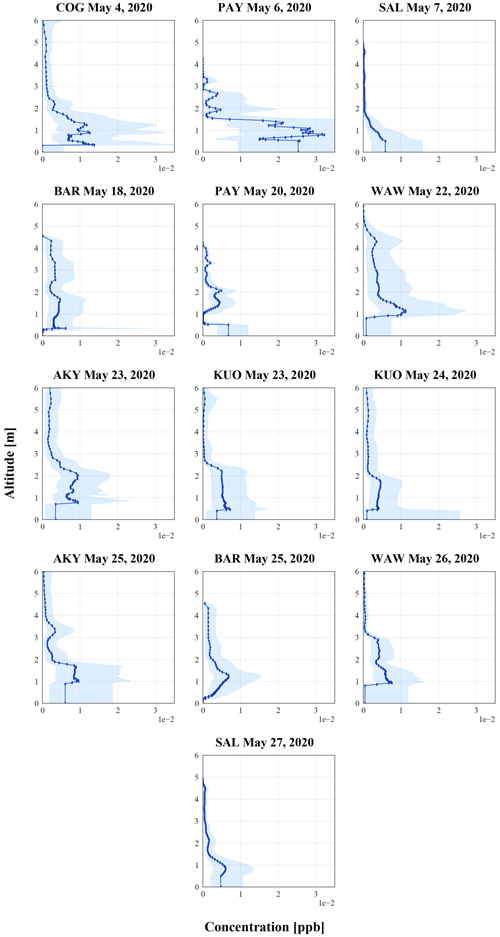
Figure 11The retrieved concentration profiles of the anthropogenic component over Europe (blue line), during the lockdown and relaxation period of May 2020 due to the COVID-19 pandemic. The altitude is provided in kilometres above sea level. The shaded blue area represents the retrieval uncertainty of GRASP/GARRLiC for the concentration profiles.
Details about the atmospheric state for each case study used in our analysis based on the lidar range-corrected signal quicklooks and the transport model simulations, along with the evaluation of the retrieved particle backscatter and extinction coefficient with the corresponding SCC products, are provided in the Supplement.
Figures 12c, 13 and 14 show histograms of the retrieved centre-of-mass (COM) height of the layer, the volume concentration and the effective radius of the anthropogenic component over Europe, during the COVID-19 lockdown and the relaxation period. COM was calculated from the retrieved concentration profile using the following equation (Siomos et al., 2017):
where z and c correspond to altitude and concentration, respectively.
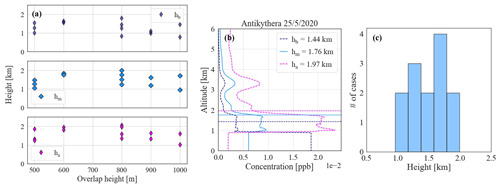
Figure 12(a) Scatterplot of the centre of mass (COM) of the anthropogenic component, as derived from GRASP/GARRLiC concentration profiles, with respect to the full-overlap height of the different lidar systems. hm (blue) represents COM as derived from the concentration profile without accounting for the retrieval uncertainty, ha (pink) represents COM as derived from the concentration profile taking into account the maximum value above the full-overlap height and the minimum value below the full-overlap height, and hb (dark blue) represents COM as derived from the concentration profile taking into account the minimum value above the full-overlap height and the maximum value below the full-overlap height. Detailed calculations of hm, ha and hb for each case study are included in the Supplement. (b) Example of hm (blue), ha (pink) and hb (dark blue) profiles, for the case study from the Antikythera EARLINET station on 25 May 2020. The altitude is provided in kilometres above sea level. (c) Histogram of hm COM values of the anthropogenic component concentration profile for all cases studied herein.
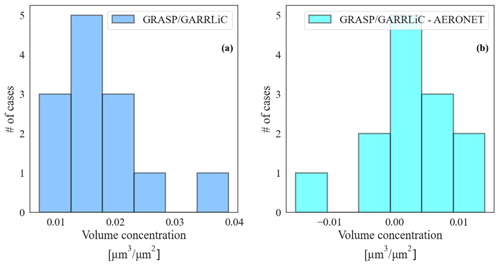
Figure 13(a) Histogram of the volume concentration of the anthropogenic aerosol component, as retrieved from GRASP/GARRLiC. (b) Histogram of the absolute difference of panel (a) from the volume concentration of the fine mode from AERONET.
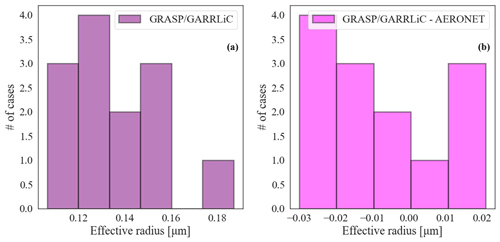
Figure 14(a) Histogram of the mean effective radius of the anthropogenic aerosol component, as retrieved from GRASP/GARRLiC. (b) Histogram of the absolute difference of panel (a) from the effective radius of the fine mode from AERONET.
Figure 12a and b illustrate the effect on the COM calculation of the limited information below the full-overlap region, for different overlap heights. In order to investigate this effect, we construct three different concentration profiles for calculating COM (see example in Fig. 12b for the case study from the Antikythera EARLINET station on 25 May 2020): hm (blue) is the concentration profile without accounting for its retrieval uncertainty, ha (pink) is the concentration profile composed of the maximum values above the full-overlap height and the minimum values below the full-overlap height. hb (dark blue) is the concentration profile composed of the minimum values above the full-overlap height and the maximum values below the full-overlap height. These profiles are provided for each case in the Supplement, and COM calculated for each profile is plotted against the overlap height in Fig. 12a. Figure 12a shows that for the different cases herein, as well as different overlap heights, hm has values of 1.25–1.75 km with a median value of 1.5 km, ha has a median value of 1.61 km and hb has a median value of 1.27 km. We see that there is no clear dependence of the calculated COM on the overlap height and that the mean value and standard deviation of COM calculated from the hm concentration profile (Fig. 12c) characterizes well the distribution of values derived for ha and hb.
The volume concentration is ∼ 0.01–0.03 µm3 µm−2, with a median value of ∼ 0.015 µm3 µm−2 (Fig. 13a), and the retrieved values are mostly similar to the corresponding AERONET products (Fig. 13b).
The retrieved effective radius is ∼ 0.1–0.15 µm (Fig. 14a). Figure 14b shows that the retrieved values are lower than the AERONET products for most of the cases used in our analysis (i.e. we compare the retrieved effective radius with the effective radius of the fine-mode “REff-F” in the “size distribution parameters” inversion product of AERONET). This may be attributed to higher information content, compared to AERONET, for the fine particles included in the GRASP/GARRLiC retrieval due to the presence of lidar measurements at 355 nm. Another possible reason is the way the molecular contribution to the signal is represented in the forward model, since a constant density profile for gases is adopted. Given the spectral dependence of the molecular-scattering properties, the signal at 355nm should be the one that is more affected by this assumption and, consequently, the retrieval of the smaller particles for GRASP/GARRLiC. Thus, in terms of the size distribution, an incorrect estimation of the molecular-scattering contribution is likely to affect the retrieved size distribution of fine particles.
Figure 15 shows the scatterplots of the differences (GRASP/GARRLiC − AERONET) of the retrieved effective radius vs. the retrieved volume concentration, along with the corresponding normalized differences. The plots show that the differences are anti-correlated, with the lower values of the effective radius retrieved from GRASP/GARRLiC being associated with higher values of retrieved volume concentrations. This anti-correlation is not easy to interpret.
The use of the GRASP/GARRLiC retrieval to assess the possible changes in aerosol particles related to anthropogenic activities above Europe during the EARLINET/ACTRIS COVID-19 campaign is to our knowledge one of the very few network exercises dedicated to deriving profiles of particle microphysics above Europe. During this study, we identified the case studies (Sect. 2), data and modelling tools providing auxiliary information (Sect. 3), as well as the methodological tools that are required to derive the microphysical properties of aerosols from a combination of lidar and sun-photometer measurements on a network level (Sect. 4). This process also involved the identification of the issues that a network study like this entails. These issues are grouped by topics regarding the selection of the study cases, the aspects of the inversion approach and the evaluation of the results, and they are discussed in the following subsections.
6.1 Selection of the study cases
The spatial and temporal collocation criteria applied to the selection of suitable case studies are a maximum of 30 min acquisition time difference and 1 km spatial distance between the sun-photometer and lidar measurements (Sect. 2). These fixed threshold selection criteria are based on empirical knowledge of the optimum time and spatial difference between the different measurement datasets (i.e. Papagiannopoulos et al., 2016) and need to be re-evaluated for each station in the network in order to take into account the effective spatio-temporal variability in the aerosols' properties. In principle, the spatio-temporal variability is expected to depend on the local geographical characteristics of the site, as well as on the atmospheric conditions.
Moreover, some case studies were not used in the analysis, due to the high full-overlap height (>1 km) of the corresponding lidar measurements. The lack of information in the lowermost atmospheric layers is a critical issue, given the typical vertical distribution of aerosols, and especially for the anthropogenic aerosols that are investigated herein. However, by selecting only the systems with full-overlap below 1 km, we only reduced the sensitivity to the assumptions adopted to handle this issue. A discussion on the impact of the missed information in this range of the atmosphere is provided in Sect. 6.2.
6.2 Aspects of the inversion approach
The experience during this campaign highlighted a strong dependence of the solution on the smoothing constraints used to perform the inversion. In Sect. 4 we present a detailed analysis of our methodological approach to defining the optimum smoothing constraints based on objective criteria, but we recognize that there are a few aspects that could be addressed in addition. First, the definition could also be based on a synthetic dataset for which the solution is known. Moreover, there should be a dedicated study on whether it is possible to have a unique setting of constraints for the whole network or to define the settings based on the characteristics of the measurements (e.g. aerosol type, load, vertical distribution, temporal variability).
Regarding the sensitivity of the retrieval to the first guess used for the inversion, it was found to be not that significant compared to the corresponding sensitivity of the smoothing constraints. Although herein we have used the first guess provided as the default option for GRASP/GARRLiC retrieval, it may be preferable to define a first guess closer to the solution based on climatological data and/or previous days' solutions.
We should highlight again that although the GRASP/GARRLiC retrieval was found to be sensitive to both setting parameters (i.e. smoothing constraints and first guess), the resulting variability was well-described by the retrieval uncertainty of the solution, as provided by the algorithm. Thus, the uncertainty provided for the GRASP/GARRLiC product also accounts for the uncertainty due to the sensitivity of the algorithm to different setting parameters.
Moreover, the retrieval also depends on the input dataset, and issues may arise from the way the low-quality data are handled. Herein we removed the low-quality data (i.e. lidar signals with noise >50 % or cases where the sky radiances from the sun photometer were recorded at a small number of viewing angles) from the input dataset (losing the information completely), and for all other data we accounted for their quality through the covariance matrix of the observations. In future studies we plan to screen out low-quality observations that do not provide any information on the retrieval but to incorporate the information of the low-quality data that contain useful information for the retrieval (providing their quality through the covariance matrix). The definition of the corresponding quality thresholds will be derived through inversion of synthetic datasets of measurements. Regarding the covariance matrix, herein we assumed it to be diagonal, with a constant relative uncertainty for each type of observation (i.e. AOD, radiances and lidar measurements). GRASP/GARRLiC provides the capability to use a height-dependent non-diagonal covariance matrix for the lidar signals (Herrera et al., 2022). In future studies we should also take into account realistic uncertainties for the lidar measurements, instead of a constant relative uncertainty, although these are not always easy to characterize.
Similarly to the removal of the low-quality data, we have also removed all measurements below the full-overlap height. In this scenario GRASP/GARRLiC uses a homogeneous layer of constant concentration to characterize the missing information of the vertical distribution from the lidar signals at these heights. In cases where the real vertical distribution is different, this may introduce discrepancies with the photometer measurements, which are sensitive to the vertical distribution in the lowermost atmospheric level. In future studies we should try to estimate the overlap function independently (Sasano et al., 1979; Tomine et al., 1989; Dho et al., 1997; Wandinger and Ansmann, 2002; Guerrero-Rascado et al., 2010; Di Paolantonio et al., 2022) and to use the lidar signals that are corrected for the overlap effect as input in GRASP/GARRLiC. A different solution could also be for GRASP/GARRLiC to allow different vertical distributions for the overlap heights, for example an exponential distribution with a given scale height. This would give a range of solutions and somehow, also considering the uncertainty estimated by GRASP/GARRLiC, sensitivity to the approach adopted to handle the missing lidar information. Finally, in principle, GRASP/GARRLiC may be upgraded to retrieve the overlap function, including it in the forward model and in the state vector. The information for the retrieval may be provided utilizing the temporal multipixel approach (Lopatin et al., 2021) through providing a set of profiles with variability in the aerosol vertical distribution, assuming a constant instrument setup (and thus a constant overlap function).
Finally, a potential critical issue in the current forward model of GRASP/GARRLiC is the molecular-scattering representation. The forward model assumes a standard atmospheric density model to estimate the molecular-scattering contribution to the measured signal at the different wavelengths. Given the range of wavelengths of the signals analysed, the assumption of a constant molecular scattering is likely to generate artefacts. At low AODs this is likely to provide artefacts as compared to the use of more precise temperature and pressure profiles. A user-provided atmospheric profile is planned to be included in the future in GRASP/GARRLiC.
6.3 Evaluation of results
Section 4 provides a description of the methodology followed for the evaluation of the results adopting two approaches:
-
consistency between simulated and measured signals (Sect. 4.1),
-
soundness of the retrieved geophysical results (Sect. 4.2).
When evaluating the results it is necessary to define a quantitative metric to interpret the relevance of obtained differences. In the absence of a known solution, the only way to obtain such a metric is by comparing the simulated to the measured signals. Although such convergence can also be obtained with solutions with low geophysical soundness, this constitutes the limit of the available information in the measurements in the absence of auxiliary information.
Regarding the comparison of our results with the products from EARLINET SCC and AERONET, this may imply that there is no added value in the synergetic use of observations. However, the EARLINET SCC products are derived using the Klett–Fernald algorithm, which is an independent method as it does not depend on the forward model or the numerical-inversion approach utilized within GRASP/GARRLiC and thus can be considered an evaluation for the latter. For the columnar properties, although AERONET and GRASP algorithms share many similarities, the additional information coming from the lidar signals is missing from the stand-alone sun-photometer inversions. In future studies we plan to augment the datasets used for the evaluation of our results, using, for example, (ground-based and/or airborne) in situ measurements.
Within the framework of the EARLINET/ACTRIS COVID-19 campaign, a subset of simultaneous and collocated AERONET data were selected to be processed with the GRASP/GARRLiC algorithm in order to provide additional information with respect to the standard EARLINET products, such as aerosol columnar size distribution and concentration profiles. In principle, such additional information was planned to be used to identify anthropogenic aerosols and to monitor their spatial and temporal variability during the COVID-19 lockdown and relaxation period of May 2020.
By applying selection criteria, first to the compliance of the station (i.e. collocation with AERONET measurements) and successively to the quality of the measurements (i.e. overlap height criteria, cloud-free atmospheres), and minimizing the contribution of natural aerosols in the fine mode (i.e, fine desert dust and smoke particles), there was a drastic reduction in the data availability. Subsequently, the obtained results have a low representativity and cannot be used to depict properly the spatio-temporal variability in aerosol composition during the COVID-19 lockdown and relaxation period over Europe. Nevertheless, we proceeded with the network exercise to apply GRASP/GARRLiC to measurements performed by lidar systems with different technical characteristics, located in different regions, with the aim to identify the fundamental and propaedeutic issues relating to the use of GRASP/GARRLiC on the network level. This exercise is particularly helpful for defining the limitations of applying GRASP/GARRLiC on a network level, since the low-load conditions during the COVID-19 lockdown and relaxation period impose a stringent test on the algorithm's performance.
First, in addition to the input measurements and associated uncertainties, GRASP/GARRLiC results depend on the setting parameters used to run the retrieval (e.g. smoothing constraints and first guesses). Although the sensitivity of the results to the smoothing parameters is higher than it is to the first guess used, the resulting variability in the solution due to both is included in the GRASP/GARRLiC product as part of the estimated retrieval uncertainty. This is true for the solutions that reproduce the measurements within the measurement uncertainty. Thus, finding the optimum setting parameters for GRASP/GARRLiC retrieval may be non-trivial, especially since they seem to depend on the different stations within the network and on different atmospheric conditions. Even so, reproducing the measurements (although using different setting parameters) is sufficient for finding the solution, since in this case the variability due to the use of different setting parameters is included in the retrieval uncertainty of the solution.
Second, from the point of view of input observations, a main issue is the overlap function. This may be handled, in principle, by modifying GRASP/GARRLiC to retrieve the overlap function from the synergy of lidar and photometer data. In the future, the requirement of ACTRIS for overlap of <300 m will reduce the impact of this issue. Moreover, we did not exploit the full information provided by the EARLINET lidars (i.e. Raman and depolarization measurements in Table 3), although GRASP provides this capability.
Third, concerning the GRASP/GARRLiC forward model, a potential source of uncertainty has been identified in the constant atmospheric density profile adopted. The next GRASP versions are planned to include the capability of changing the atmospheric profile by the user.
Fourth, the impact of combining the sun-photometer measurements with lidar measurements with GRASP/GARRLiC is evaluated by comparing the obtained GRASP/GARRLiC products against the ones derived from single-instrument measurements, namely the AERONET and the EARLINET SCC products. We expect that the lidar information does not significantly influence the retrieval of aerosol columnar microphysical properties. This is observed for several cases; however there are a few cases where a change in the size distribution, in particular of the smaller particles, is obtained combining the instruments with respect to the sun-photometer-only (AERONET) product. A possible explanation may be the additional spectral information introduced by the lidar measurements at 355 nm, or it may be a bias due to the assumption of a constant atmospheric density profile.
It should be noted that the above conclusions are obtained considering the capability of reproducing correctly the input measurements. For an ill-posed problem, optimizing the inversion based uniquely on the capability to reproduce the input measurements should be further supported by evaluation of the results with independent datasets (e.g. in situ data) and/or by performing numerical exercises based on synthetic measurements.
Recognizing the value of the homogenization of the GRASP/GARRLiC retrieval within the framework of ACTRIS, AERIS/ICARE has been developing a new tool for automatic GRASP/GARRLiC retrievals, utilizing combined measurements of EARLINET lidars and AERONET sun photometers. The issue of the “optimum setting parameters” for each station has not been solved as of yet for the automated retrieval. Currently, the same parameters are used for all stations. Moreover, at this stage, the evaluation of the results is done through visual inspection, e.g. for unphysical oscillations, whereas evaluation with SCC products may be included in the future.
Overall, this study highlights the potential of utilizing GRASP/GARRLiC on a network level, with the aim of the effective characterization of the atmospheric state with respect to the microphysical properties of aerosol particles and their concentration profiles. It also identifies the associated issues that require a more thorough investigation of their impacts or new developments to the algorithm, along with recognizing the need to acquire independent datasets for the evaluation of the results or to perform ad hoc studies with synthetic datasets.
A1 Normalization of range-corrected lidar signals
The actual number of points in the range-corrected lidar signals used herein varies with the selection of maximum and minimum altitude as well as the vertical resolution used during signal pre-processing in the SCC. For the EARLINET COVID-19 campaign the pre-processing vertical resolution and maximum altitude of the profiles were selected to be at 60 m and 15 km respectively, for all stations, while minimum altitude varies depending on the full-overlap height of each system. The latter was carefully defined prior to the campaign, based on quality-assurance tests performed on each system following Freudenthaler et al. (2018). This procedure led to range-corrected signals of approximately 350–500 altitude points.
This number had to be scaled down before the signals were used as input to the GRASP/GARRLiC retrieval in order to
- i.
reduce the excessively high number of retrieved parameters, since the altitude points of the retrieved concentration profiles are the same as the altitude points of the range-corrected lidar signals;
- ii.
reduce noise contamination of lidar signals at high altitudes;
- iii.
decrease calculation time.
The procedure followed is described in Sect. A1, as well as in Lopatin et al. (2013), as “decimation of lidar signals in logarithmic scale over altitude”, and additional details can be found on the GRASP-OPEN web page (https://www.grasp-open.com, last access: 4 December 2023).
A2 Lidar signal decimation and normalization
In order to move to a logarithmic altitude/range scale and reduce lidar signal and lidar altitude vectors to points in a logarithmically equidistant manner, we first define each profile point (hi) in a logarithmic scale ():
The logarithmic-scale step Δz can be calculated from min () and max () altitude as
with Nz being the total number of logarithmically equidistant points in the profile (herein Nz=60).
Then, each logarithmic altitude point (hk) can be calculated as
Finally, for each hk value the lidar signal profile and lidar altitude vector are averaged as follows:
where n is the total number of points inside logarithmic altitude ranges and Ak denotes the lidar signal profile.
In order to account for discrepancies between different instruments, the last step is to normalize the lidar signals between min and max altitude following
Equations (B1) and (B2) provide the formulas utilized for the calculation of GRASP/GARRLiC backscatter and extinction coefficient uncertainty.
where k denotes the fine or coarse mode.
As a first-order approximation, the standard deviation of the logarithms can be treated as the relative error so that we finally obtain
where i denotes the extinction (a) or backscatter (β) coefficient profile.
All the available EARLINET lidar data during the COVID-19 pandemic are published under https://doi.org/10.21336/gen.w3w1-j222 (ACTRIS ARES Data Centre, 2020a). AERONET L1.5 retrievals used for result validation are publicly available at the following web page: https://aeronet.gsfc.nasa.gov/ (NASA GSFC, 2023).
The supplement related to this article is available online at: https://doi.org/10.5194/amt-16-6025-2023-supplement.
AT and AG organized and coordinated the work presented herein and structured the manuscript. AT, AG, MDP, DD, GLL, AF, AS, AP, DPR, MJGM and JLGR formulated the methodology for running GRASP/GARRLiC on a network level (with guidance from AL) and performed the GRASP/GARRLiC retrievals presented herein. MDP, DD, GLL and ISS provided valuable guidance on the structure and content of the manuscript. AK, AS and DPR performed the atmospheric model simulations. AT, AG, AF, AS, AP, EM, VA, MS, AC, CMP, ARG, SR, MRP, XS, MK, RF, WK, DS and ISS provided the high-quality lidar and sun-photometer measurements used herein. All authors contributed with comments on the manuscript and reviewed the final version.
At least one of the (co-)authors is a member of the editorial board of Atmospheric Measurement Techniques. The peer-review process was guided by an independent editor, and the authors also have no other competing interests to declare.
Publisher’s note: Copernicus Publications remains neutral with regard to jurisdictional claims made in the text, published maps, institutional affiliations, or any other geographical representation in this paper. While Copernicus Publications makes every effort to include appropriate place names, the final responsibility lies with the authors.
This article is part of the special issue “Quantifying the impacts of stay-at-home policies on atmospheric composition and properties of aerosol and clouds over the European regions using ACTRIS related observations (ACP/AMT inter-journal SI)”. It is not associated with a conference.
We are grateful to EARLINET (https://www.earlinet.org/, last access: 12 April 2023) and ACTRIS (https://www.actris.eu, last access: 12 April 2023) for the data collection, calibration, processing and dissemination. The authors would like to acknowledge the use of GRASP inversion algorithm software (http://www.grasp-open.com, last access: 4 December 2023) in this work. We thank the AERONET team and all principal investigators (PIs) and co-investigators (Co-Is) and their staff for establishing and maintaining the 21 AERONET sites used in this investigation and making the data available for the community. We would like to acknowledge COST Action HARMONIA (International network for harmonization of atmospheric aerosol retrievals from ground-based photometers), CA2119, supported by COST (European Cooperation in Science and Technology). This research was supported by data and services obtained from the PANhellenic GEophysical observatory of Antikythera (PANGEA) of the National Observatory of Athens (NOA). Limassol station acknowledges the H2020 Widespread Teaming project EXCELSIOR – ERATOSTHENES: EXcellence Research Centre for Earth SurveiLlance and Space-Based MonItoring Of the EnviRonment (https://excelsior2020.eu/, last access: 4 December 2023). The EXCELSIOR project has received funding from the European Union's Horizon 2020 research and innovation programme under grant agreement no. 857510; from the government of the Republic of Cyprus through the Directorate General for European Programmes, Coordination and Development; and from the Cyprus University of Technology. Kuopio station acknowledges the support of the Academy of Finland (projects nos. 310312 and 329216). Granada station acknowledges the Spanish national project nos. PID2020-120015RB-100, PID2020-117825GB-C21, PID2020-117825GB-C22 and PID2021-128008OB-I00 funded by MCIN/AEI/10.13039/501100011033/FEDER “A way of making Europe”; project nos. A-RNM-430-UGR20, PP2022.PP.34, P20-0136 and P18-RT-3820; H2020-MSCA-RISE-2017 “GRASP-ACE”; ACTRIS IMP; ATMO-ACCESS (grant agreement no. 101008004); REALISTIC (grant agreement no. 101086690); AEROMOST (Junta de Andalucía, ProExcel_00204); the Scientific Unit of Excellence “Earth System” (UCE-PP2017-02); and the national infrastructure programmes EQC2019-006192-P and EQC2019-006423-P. The INOE team acknowledges the Core Program within the National Research Development and Innovation Plan 2022–2027, carried out with the support of MCID, project no. PN 23 05.
This research was financially supported by D-TECT (grant agreement no. 725698) funded by the European Research Council (ERC) under the European Union's Horizon 2020 research and innovation programme and by PANGEA4CalVal (grant agreement no. 101079201) funded by the European Union . The Barcelona site has been funded by the REALISTIC project (grant agreement no. 101086690) under the European Union's Horizon Widera 2022 Talents programme. The Warsaw site has been supported by the European Commission H2020 (ACTRIS IMP (grant no. 871115)). The Belsk site has been supported by the National Science Centre, Poland (grant no. 2021/41/B/ST10/03660).
This paper was edited by Simone Lolli and reviewed by two anonymous referees.
ACTRIS ARES Data Centre: ACTRIS Aerosol Remote Sensing COVID-19 campaign data of May 2020, ACTRIS ARES Data Centre [data set], https://doi.org/10.21336/gen.w3w1-j222, 2020a.
ACTRIS ARES Data Centre: ACTRIS Aerosol Remote Sensing COVID-19 campaign data of May 2020, ACTRIS ARES Data Centre [data set], https://doi.org/10.21336/gen.xmbc-tj86, 2020b.
Ansmann, A. and Müller, D.: Lidar and Atmospheric Aerosol Particles, in: Lidar. Springer Series in Optical Sciences, Vol. 102, edited by: Weitkamp, C., Springer, New York, NY, https://doi.org/10.1007/0-387-25101-4_4, 2005.
Ansmann, A., Riebesell, M., and Weitkamp, C.: Measurement of atmospheric aerosol extinction profiles with a Raman lidar, Opt. Lett., 15, 746, https://doi.org/10.1364/OL.15.000746, 1990.
Ansmann, A., Seifert, P., Tesche, M., and Wandinger, U.: Profiling of fine and coarse particle mass: case studies of Saharan dust and Eyjafjallajökull/Grimsvötn volcanic plumes, Atmos. Chem. Phys., 12, 9399–9415, https://doi.org/10.5194/acp-12-9399-2012, 2012.
Belegante, L., Bravo-Aranda, J. A., Freudenthaler, V., Nicolae, D., Nemuc, A., Ene, D., Alados-Arboledas, L., Amodeo, A., Pappalardo, G., D'Amico, G., Amato, F., Engelmann, R., Baars, H., Wandinger, U., Papayannis, A., Kokkalis, P., and Pereira, S. N.: Experimental techniques for the calibration of lidar depolarization channels in EARLINET, Atmos. Meas. Tech., 11, 1119–1141, https://doi.org/10.5194/amt-11-1119-2018, 2018.
Burton, S. P., Ferrare, R. A., Hostetler, C. A., Hair, J. W., Rogers, R. R., Obland, M. D., Butler, C. F., Cook, A. L., Harper, D. B., and Froyd, K. D.: Aerosol classification using airborne High Spectral Resolution Lidar measurements – methodology and examples, Atmos. Meas. Tech., 5, 73–98, https://doi.org/10.5194/amt-5-73-2012, 2012.
Burton, S. P., Ferrare, R. A., Vaughan, M. A., Omar, A. H., Rogers, R. R., Hostetler, C. A., and Hair, J. W.: Aerosol classification from airborne HSRL and comparisons with the CALIPSO vertical feature mask, Atmos. Meas. Tech., 6, 1397–1412, https://doi.org/10.5194/amt-6-1397-2013, 2013.
Chaikovsky, A., Dubovik, O., Holben, B., Bril, A., Goloub, P., Tanré, D., Pappalardo, G., Wandinger, U., Chaikovskaya, L., Denisov, S., Grudo, J., Lopatin, A., Karol, Y., Lapyonok, T., Amiridis, V., Ansmann, A., Apituley, A., Allados-Arboledas, L., Binietoglou, I., Boselli, A., D'Amico, G., Freudenthaler, V., Giles, D., Granados-Muñoz, M. J., Kokkalis, P., Nicolae, D., Oshchepkov, S., Papayannis, A., Perrone, M. R., Pietruczuk, A., Rocadenbosch, F., Sicard, M., Slutsker, I., Talianu, C., De Tomasi, F., Tsekeri, A., Wagner, J., and Wang, X.: Lidar-Radiometer Inversion Code (LIRIC) for the retrieval of vertical aerosol properties from combined lidar/radiometer data: development and distribution in EARLINET, Atmos. Meas. Tech., 9, 1181–1205, https://doi.org/10.5194/amt-9-1181-2016, 2016.
Chossière, G. P., Xu, H., Dixit, Y., Isaacs, S., Eastham, S. D., Allroggen, F., Speth, R. L., and Barrett, S. R. H.: Air pollution impacts of COVID-19–related containment measures, Science Advances, 7, eabe1178, https://doi.org/10.1126/sciadv.abe1178, 2021.
Chen, H., Chen, S., Zhang, Y., Chen, H., Guo, P., and Chen, B.: Experimental determination of Raman lidar geometric form factor combining Raman and elastic return, Opt. Commun., 332, 296–300, https://doi.org/10.1016/j.optcom.2014.07.025, 2014.
D'Amico, G., Amodeo, A., Baars, H., Binietoglou, I., Freudenthaler, V., Mattis, I., Wandinger, U., and Pappalardo, G.: EARLINET Single Calculus Chain – overview on methodology and strategy, Atmos. Meas. Tech., 8, 4891–4916, https://doi.org/10.5194/amt-8-4891-2015, 2015.
D'Amico, G., Amodeo, A., Mattis, I., Freudenthaler, V., and Pappalardo, G.: EARLINET Single Calculus Chain – technical – Part 1: Pre-processing of raw lidar data, Atmos. Meas. Tech., 9, 491–507, https://doi.org/10.5194/amt-9-491-2016, 2016.
Deleva, A. D., Krasteva, E. N., Vulkova, L. A. and Dreischuh, T. N.: Thick anthropogenic aerosol layers detected by LIDAR above Sofia, AIP Conf. Proc., 2075, 130019, https://doi.org/10.1063/1.5091304, 2019.
Di Girolamo, P., Gagliardi, R. V., Pappalardo, G., Spinelli, N., Velotta, R., and Berardi, V.: Two wavelength lidar analysis of stratospheric aerosol size distribution, J. Aerosol Sci., 26, 989–1001, https://doi.org/10.1016/0021-8502(95)00025-8, 1995.
Di Paolantonio, M., Dionisi, D., and Liberti, G. L.: A semi-automated procedure for the emitter–receiver geometry characterization of motor-controlled lidars, Atmos. Meas. Tech., 15, 1217–1231, https://doi.org/10.5194/amt-15-1217-2022, 2022.
Dho, S. W., Park, Y. J., and Kong, H. J.: Experimental determination of a geometric form factor in a lidar equation for an inhomogeneous atmosphere, Appl. Optics, 36, 6009–6010, https://doi.org/10.1364/AO.36.006009, 1997.
Dubovik, O. and King, M. D.: A flexible inversion algorithm for retrieval of aerosol optical properties from Sun and sky radiance measurements, J. Geophys. Res.-Atmos., 105, 20673–20696, https://doi.org/10.1029/2000JD900282, 2000.
Dubovik, O., Smirnov, A., Holben, B., King, M., Kaufman, Y., Eck, T., and Slutsker, I.: Accuracy assessments of aerosol optical properties retrieved from Aerosol Robotic Network (AERONET) Sun and sky radiance measurements, J. Geophys. Res.-Atmos., 105, 9791–9806, https://doi.org/10.1029/2000jd900040, 2000.
Dubovik, O., Holben, B., Eck, T. F., Smirnov, A., Kaufman, Y. J., King, M. D., Tanré, D., and Slutsker, I.: Variability of Absorption and Optical Properties of Key Aerosol Types Observed in Worldwide Locations, J. Atmos. Sci., 59, 590–608, https://doi.org/10.1175/1520-0469(2002)059<0590:VOAAOP>2.0.CO;2, 2002.
Dubovik, O., Sinyuk, A., Lapyonok, T., Holben, B. N., Mishchenko, M., Yang, P., Eck, T. F., Volten, H., Muñoz, O., Veihelmann, B., Sorokin, M., and Slutsker, I.: Application of spheroid models to account for aerosol particle nonsphericity in remote sensing of desert dust, J. Geophys. Res.-Atmos., 111, D11208, https://doi.org/10.1029/2005JD006619, 2006.
Dubovik, O., Herman, M., Holdak, A., Lapyonok, T., Tanré, D., Deuzé, J. L., Ducos, F., Sinyuk, A., and Lopatin, A.: Statistically optimized inversion algorithm for enhanced retrieval of aerosol properties from spectral multi-angle polarimetric satellite observations, Atmos. Meas. Tech., 4, 975–1018, https://doi.org/10.5194/amt-4-975-2011, 2011.
Fernald, F. G.: Analysis of atmospheric lidar observations: Some comments, Appl. Optics, 23, 652–653, https://doi.org/10.1364/AO.23.000652, 1984.
Floutsi, A. A., Baars, H., Engelmann, R., Althausen, D., Ansmann, A., Bohlmann, S., Heese, B., Hofer, J., Kanitz, T., Haarig, M., Ohneiser, K., Radenz, M., Seifert, P., Skupin, A., Yin, Z., Abdullaev, S. F., Komppula, M., Filioglou, M., Giannakaki, E., Stachlewska, I. S., Janicka, L., Bortoli, D., Marinou, E., Amiridis, V., Gialitaki, A., Mamouri, R.-E., Barja, B., and Wandinger, U.: DeLiAn – a growing collection of depolarization ratio, lidar ratio and Ångström exponent for different aerosol types and mixtures from ground-based lidar observations, Atmos. Meas. Tech., 16, 2353–2379, https://doi.org/10.5194/amt-16-2353-2023, 2023.
Freudenthaler, V., Linné, H., Chaikovski, A., Rabus, D., and Groß, S.: EARLINET lidar quality assurance tools, Atmos. Meas. Tech. Discuss. [preprint], https://doi.org/10.5194/amt-2017-395, in review, 2018.
Groß, S., Esselborn, M., Weinzierl, B., Wirth, M., Fix, A., and Petzold, A.: Aerosol classification by airborne high spectral resolution lidar observations, Atmos. Chem. Phys., 13, 2487–2505, https://doi.org/10.5194/acp-13-2487-2013, 2013.
Granados-Muñoz, M. J., Navas-Guzmán, F., Guerrero-Rascado, J. L., Bravo-Aranda, J. A., Binietoglou, I., Pereira, S. N., Basart, S., Baldasano, J. M., Belegante, L., Chaikovsky, A., Comerón, A., D'Amico, G., Dubovik, O., Ilic, L., Kokkalis, P., Muñoz-Porcar, C., Nickovic, S., Nicolae, D., Olmo, F. J., Papayannis, A., Pappalardo, G., Rodríguez, A., Schepanski, K., Sicard, M., Vukovic, A., Wandinger, U., Dulac, F., and Alados-Arboledas, L.: Profiling of aerosol microphysical properties at several EARLINET/AERONET sites during the July 2012 ChArMEx/EMEP campaign, Atmos. Chem. Phys., 16, 7043–7066, https://doi.org/10.5194/acp-16-7043-2016, 2016.
Guerrero-Rascado, J. L., Costa, M. J., Bortoli, D., Silva, A. M., Lyamani, H., and Alados-Arboledas, L.: Infrared lidar overlap function: an experimental determination, Opt. Express, 18, 2035020369, https://doi.org/10.1364/OE.18.020350, 2010.
Herrera, M. E., Dubovik, O., Torres, B., Lapyonok, T., Fuertes, D., Lopatin, A., Litvinov, P., Chen, C., Benavent-Oltra, J. A., Bali, J. L., and Ristori, P. R.: Estimates of remote sensing retrieval errors by the GRASP algorithm: application to ground-based observations, concept and validation, Atmos. Meas. Tech., 15, 6075–6126, https://doi.org/10.5194/amt-15-6075-2022, 2022.
Holben, B. N., Eck, T. F., Slutsker, I., Tanré, D., Buis, J. P., Setzer, A., Vermote, E., Reagan, J. A., Kaufman, Y. J., Nakajima, T., Jankowiak, I., and Smirnov, A.: AERONET – A federated instrument network and data archive for aerosol characterization, Remote Sens. Environ., 66, 1–16, https://doi.org/10.1016/S0034-4257(98)00031-5, 1998.
Janicka, L., Stachlewska, I. S., Veselovskii, I., and Baars, H.: Temporal variations in optical and microphysical properties of mineral dust and biomass burning aerosol derived from daytime Raman lidar observations over Warsaw, Poland, Atmos. Environ., 169, 162–174, https://doi.org/10.1016/j.atmosenv.2017.09.022, 2017.
Järvinen, E., Kemppinen, O., Nousiainen, T., Kociok, T., Möhler, O., Leisner, T., and Schnaiter, M.: Laboratory investigations of mineral dust near-backscattering depolarization ratios, J. Quant. Spectrosc. Ra., 178, 192–208, https://doi.org/10.1016/j.jqsrt.2016.02.003, 2016.
Klett, J. D.: Stable analytical inversion solution for processing lidar returns, Appl. Optics, 20, 211–220, https://doi.org/10.1364/AO.20.000211, 1981.
Kotthaus, S., Bravo-Aranda, J. A., Collaud Coen, M., Guerrero-Rascado, J. L., Costa, M. J., Cimini, D., O'Connor, E. J., Hervo, M., Alados-Arboledas, L., Jiménez-Portaz, M., Mona, L., Ruffieux, D., Illingworth, A., and Haeffelin, M.: Atmospheric boundary layer height from ground-based remote sensing: a review of capabilities and limitations, Atmos. Meas. Tech., 16, 433–479, https://doi.org/10.5194/amt-16-433-2023, 2023.
Lopatin, A., Dubovik, O., Chaikovsky, A., Goloub, P., Lapyonok, T., Tanré, D., and Litvinov, P.: Enhancement of aerosol characterization using synergy of lidar and sun-photometer coincident observations: the GARRLiC algorithm, Atmos. Meas. Tech., 6, 2065–2088, https://doi.org/10.5194/amt-6-2065-2013, 2013.
Lopatin, A., Dubovik, O., Fuertes, D., Stenchikov, G., Lapyonok, T., Veselovskii, I., Wienhold, F. G., Shevchenko, I., Hu, Q., and Parajuli, S.: Synergy processing of diverse ground-based remote sensing and in situ data using the GRASP algorithm: applications to radiometer, lidar and radiosonde observations, Atmos. Meas. Tech., 14, 2575–2614, https://doi.org/10.5194/amt-14-2575-2021, 2021.
Marinou, E., Tesche, M., Nenes, A., Ansmann, A., Schrod, J., Mamali, D., Tsekeri, A., Pikridas, M., Baars, H., Engelmann, R., Voudouri, K.-A., Solomos, S., Sciare, J., Groß, S., Ewald, F., and Amiridis, V.: Retrieval of ice-nucleating particle concentrations from lidar observations and comparison with UAV in situ measurements, Atmos. Chem. Phys., 19, 11315–11342, https://doi.org/10.5194/acp-19-11315-2019, 2019.
Mattis, I., D'Amico, G., Baars, H., Amodeo, A., Madonna, F., and Iarlori, M.: EARLINET Single Calculus Chain – technical – Part 2: Calculation of optical products, Atmos. Meas. Tech., 9, 3009–3029, https://doi.org/10.5194/amt-9-3009-2016, 2016.
Mona, L., Pappalardo, G., Amodeo, A., D'Amico, G., Madonna, F., Boselli, A., Giunta, A., Russo, F., and Cuomo, V.: One year of CNR-IMAA multi-wavelength Raman lidar measurements in coincidence with CALIPSO overpasses: Level 1 products comparison, Atmos. Chem. Phys., 9, 7213–7228, https://doi.org/10.5194/acp-9-7213-2009, 2009.
Müller, D., Ansmann, A., Mattis, I., Tesche, M., Wandinger, U., Althausen, D., and Pisani, G.: Aerosol-type-dependent lidar ratios observed with Raman lidar, J. Geophys. Res., 112, D16202, https://doi.org/10.1029/2006JD008292, 2007.
Mylonaki, M., Giannakaki, E., Papayannis, A., Papanikolaou, C.-A., Komppula, M., Nicolae, D., Papagiannopoulos, N., Amodeo, A., Baars, H., and Soupiona, O.: Aerosol type classification analysis using EARLINET multiwavelength and depolarization lidar observations, Atmos. Chem. Phys., 21, 2211–2227, https://doi.org/10.5194/acp-21-2211-2021, 2021.
NASA GSFC: AERONET (AErosol RObotic NETwork) program, https://aeronet.gsfc.nasa.gov/ (last access: 4 December 2023), 2023.
Navas-Guzmán, F., Guerrero-Rascado, J. L., and Alados-Arboledas, L.: Retrieval of the lidar overlap function using Raman signals, Óptica Pura y Aplicada, 44, 71–75, 2011.
Nickovic, S., Kallos, G., Papadopoulos, A., and Kakaliagou, O.: A model for prediction of desert dust cycle in the atmosphere, J. Geophys. Res., 106, 18113–18130, https://doi.org/10.1029/2000JD900794, 2001.
Nicolae, D., Vasilescu, J., Talianu, C., Binietoglou, I., Nicolae, V., Andrei, S., and Antonescu, B.: A neural network aerosol-typing algorithm based on lidar data, Atmos. Chem. Phys., 18, 14511–14537, https://doi.org/10.5194/acp-18-14511-2018, 2018.
Papagiannopoulos, N., Mona, L., Alados-Arboledas, L., Amiridis, V., Baars, H., Binietoglou, I., Bortoli, D., D'Amico, G., Giunta, A., Guerrero-Rascado, J. L., Schwarz, A., Pereira, S., Spinelli, N., Wandinger, U., Wang, X., and Pappalardo, G.: CALIPSO climatological products: evaluation and suggestions from EARLINET, Atmos. Chem. Phys., 16, 2341–2357, https://doi.org/10.5194/acp-16-2341-2016, 2016.
Papagiannopoulos, N., Mona, L., Amodeo, A., D'Amico, G., Gumà Claramunt, P., Pappalardo, G., Alados-Arboledas, L., Guerrero-Rascado, J. L., Amiridis, V., Kokkalis, P., Apituley, A., Baars, H., Schwarz, A., Wandinger, U., Binietoglou, I., Nicolae, D., Bortoli, D., Comerón, A., Rodríguez-Gómez, A., Sicard, M., Papayannis, A., and Wiegner, M.: An automatic observation-based aerosol typing method for EARLINET, Atmos. Chem. Phys., 18, 15879–15901, https://doi.org/10.5194/acp-18-15879-2018, 2018.
Papagiannopoulos, N., D'Amico, G., Gialitaki, A., Ajtai, N., Alados-Arboledas, L., Amodeo, A., Amiridis, V., Baars, H., Balis, D., Binietoglou, I., Comerón, A., Dionisi, D., Falconieri, A., Fréville, P., Kampouri, A., Mattis, I., Mijić, Z., Molero, F., Papayannis, A., Pappalardo, G., Rodríguez-Gómez, A., Solomos, S., and Mona, L.: An EARLINET early warning system for atmospheric aerosol aviation hazards, Atmos. Chem. Phys., 20, 10775–10789, https://doi.org/10.5194/acp-20-10775-2020, 2020.
Pappalardo G., Bosenberg J., Amodeo A., Ansmann A., Apituley A., Arboledas L. A., Balis D., Bockmann C., Chaikovsky A., Comeron A., D'Amico G., Freudenthaler V., Grigorov I., Hansen G., Linnè H., Kinne S., Mattis I., Mona L., Mueller D., Mitev V., Nicolae D., Papayannis A., Perrone M. R., Pietruczuk A., Pujadas M., Putaud J.-P., Ravetta F., Rizi V., Simeonov V., Spinelli N., Trickl T., Wandinger U., and Wiegner M.: Earlinet: The European aerosol research lidar network for the aerosol climatology on continental scale AIP Conf. Proc., 1100, 189–192, https://doi.org/10.1063/1.3116946, 2009.
Pappalardo, G., Mona, L., D'Amico, G., Wandinger, U., Adam, M., Amodeo, A., Ansmann, A., Apituley, A., Alados Arboledas, L., Balis, D., Boselli, A., Bravo-Aranda, J. A., Chaikovsky, A., Comeron, A., Cuesta, J., De Tomasi, F., Freudenthaler, V., Gausa, M., Giannakaki, E., Giehl, H., Giunta, A., Grigorov, I., Groß, S., Haeffelin, M., Hiebsch, A., Iarlori, M., Lange, D., Linné, H., Madonna, F., Mattis, I., Mamouri, R.-E., McAuliffe, M. A. P., Mitev, V., Molero, F., Navas-Guzman, F., Nicolae, D., Papayannis, A., Perrone, M. R., Pietras, C., Pietruczuk, A., Pisani, G., Preißler, J., Pujadas, M., Rizi, V., Ruth, A. A., Schmidt, J., Schnell, F., Seifert, P., Serikov, I., Sicard, M., Simeonov, V., Spinelli, N., Stebel, K., Tesche, M., Trickl, T., Wang, X., Wagner, F., Wiegner, M., and Wilson, K. M.: Four-dimensional distribution of the 2010 Eyjafjallajökull volcanic cloud over Europe observed by EARLINET, Atmos. Chem. Phys., 13, 4429–4450, https://doi.org/10.5194/acp-13-4429-2013, 2013.
Pappalardo, G., Amodeo, A., Apituley, A., Comeron, A., Freudenthaler, V., Linné, H., Ansmann, A., Bösenberg, J., D'Amico, G., Mattis, I., Mona, L., Wandinger, U., Amiridis, V., Alados-Arboledas, L., Nicolae, D., and Wiegner, M.: EARLINET: towards an advanced sustainable European aerosol lidar network, Atmos. Meas. Tech., 7, 2389–2409, https://doi.org/10.5194/amt-7-2389-2014, 2014.
Pisso, I., Sollum, E., Grythe, H., Kristiansen, N. I., Cassiani, M., Eckhardt, S., Arnold, D., Morton, D., Thompson, R. L., Groot Zwaaftink, C. D., Evangeliou, N., Sodemann, H., Haimberger, L., Henne, S., Brunner, D., Burkhart, J. F., Fouilloux, A., Brioude, J., Philipp, A., Seibert, P., and Stohl, A.: The Lagrangian particle dispersion model FLEXPART version 10.4, Geosci. Model Dev., 12, 4955–4997, https://doi.org/10.5194/gmd-12-4955-2019, 2019.
Radenz, M., Seifert, P., Baars, H., Floutsi, A. A., Yin, Z., and Bühl, J.: Automated time–height-resolved air mass source attribution for profiling remote sensing applications, Atmos. Chem. Phys., 21, 3015–3033, https://doi.org/10.5194/acp-21-3015-2021, 2021.
Sakai, T., Nagai, T., Zaizen, Y., and Mano, Y.: Backscattering linear depolarization ratio measurements of mineral, sea-salt, and ammonium sulfate particles simulated in a laboratory chamber, Appl. Optics, 49, 4441, https://doi.org/10.1364/AO.49.004441, 2010.
Sasano, Y., Shimizu, H., Takeuchi, N., and Okuda, M.: Geometrical form factor in the laser radar equation: an experimental determination, Appl. Optics, 18, 3908–3910, https://doi.org/10.1364/AO.18.003908, 1979.
Schneider, J., Balis, D., Böckmann, C., Bösenberg, J., Calpini, B., Chaikovsky, A. P., Comeron, A., Flamant, P., Freudenthaler, V., Hågård, A., Vaughan, G., and Visconti, G.: European aerosol research lidar network to establish an aerosol climatology (EARLINET), J. Aerosol Sci., 31, 592–593, 2000.
Sicard, M., Guerrero-Rascado, J. L., Navas-Guzmán, F., Preißler, J., Molero, F., Tomás, S., Bravo-Aranda, J. A., Comerón, A., Rocadenbosch, F., Wagner, F., Pujadas, M., and Alados-Arboledas, L.: Monitoring of the Eyjafjallajökull volcanic aerosol plume over the Iberian Peninsula by means of four EARLINET lidar stations, Atmos. Chem. Phys., 12, 3115–3130, https://doi.org/10.5194/acp-12-3115-2012, 2012.
Sicard, M., D'Amico, G., Comerón, A., Mona, L., Alados-Arboledas, L., Amodeo, A., Baars, H., Baldasano, J. M., Belegante, L., Binietoglou, I., Bravo-Aranda, J. A., Fernández, A. J., Fréville, P., García-Vizcaíno, D., Giunta, A., Granados-Muñoz, M. J., Guerrero-Rascado, J. L., Hadjimitsis, D., Haefele, A., Hervo, M., Iarlori, M., Kokkalis, P., Lange, D., Mamouri, R. E., Mattis, I., Molero, F., Montoux, N., Muñoz, A., Muñoz Porcar, C., Navas-Guzmán, F., Nicolae, D., Nisantzi, A., Papagiannopoulos, N., Papayannis, A., Pereira, S., Preißler, J., Pujadas, M., Rizi, V., Rocadenbosch, F., Sellegri, K., Simeonov, V., Tsaknakis, G., Wagner, F., and Pappalardo, G.: EARLINET: potential operationality of a research network, Atmos. Meas. Tech., 8, 4587–4613, https://doi.org/10.5194/amt-8-4587-2015, 2015.
Siomos, N., Balis, D. S., Poupkou, A., Liora, N., Dimopoulos, S., Melas, D., Giannakaki, E., Filioglou, M., Basart, S., and Chaikovsky, A.: Investigating the quality of modeled aerosol profiles based on combined lidar and sunphotometer data, Atmos. Chem. Phys., 17, 7003–7023, https://doi.org/10.5194/acp-17-7003-2017, 2017.
Sugimoto, N. and Lee, C. H.: Characteristics of dust aerosols inferred from lidar depolarization measurements at two wavelengths, Appl. Optics, 45, 7468–7474, https://doi.org/10.1364/AO.45.007468, 2006.
Szczepanik, D. M., Stachlewska, I. S., Tetoni, E., and Althausen, D.: Properties of Saharan Dust Versus Local Urban Dust–A Case Study, Earth Space Science, 8, e2021EA001816, https://doi.org/10.1029/2021EA001816, 2021.
Tesche, M., Ansmann, A., Müller, D., Althausen, D., Engelmann, R., Freudenthaler, V., and Groß, S.: Vertically resolved separation of dust and smoke over Cape Verde using multiwavelength Raman and polarization lidars during Saharan Mineral Dust Experiment 2008, J. Geophys. Res., 114, D13202, https://doi.org/10.1029/2009JD011862, 2009.
Tomine, K., Hirayama, C., Michimoto, K., and Takeuchi, N.: Experimental determination of the crossover function in the laser radar equation for days with a light mist, Appl. Optics, 28, 2194–2195, https://doi.org/10.1364/AO.28.002194, 1989.
Tsekeri, A., Lopatin, A., Amiridis, V., Marinou, E., Igloffstein, J., Siomos, N., Solomos, S., Kokkalis, P., Engelmann, R., Baars, H., Gratsea, M., Raptis, P. I., Binietoglou, I., Mihalopoulos, N., Kalivitis, N., Kouvarakis, G., Bartsotas, N., Kallos, G., Basart, S., Schuettemeyer, D., Wandinger, U., Ansmann, A., Chaikovsky, A. P., and Dubovik, O.: GARRLiC and LIRIC: strengths and limitations for the characterization of dust and marine particles along with their mixtures, Atmos. Meas. Tech., 10, 4995–5016, https://doi.org/10.5194/amt-10-4995-2017, 2017.
Venter, Z. S., Aunan, K., Chowdhury, S., and Lelieveld, J.: COVID-19 lockdowns cause global air pollution declines, P. Natl. Acad. Sci. USA, 117, 18984–18990. https://doi.org/10.1073/pnas.2006853117, 2020.
Wandinger, U. and Ansmann, A.: Experimental determination of the lidar overlap profile with raman lidar, Appl. Optics, 41, 511–514, https://doi.org/10.1364/AO.41.000511, 2002.
WHO: WMO air quality and climate bulletin released for Clean Air Day, https://public-old.wmo.int/en/our-mandate/focus-areas/environment/air_quality (last access: 8 December 2023), 2021.
- Abstract
- Introduction
- The EARLINET/ACTRIS COVID-19 campaign
- Data and tools
- Methodology
- Results
- Issues identified from running GRASP/GARRLiC on a network level
- Summary and conclusions
- Appendix A
- Appendix B: Calculation of the GRASP/GARRLiC retrieval uncertainties for the retrieved aerosol backscatter and extinction coefficients
- Data availability
- Author contributions
- Competing interests
- Disclaimer
- Special issue statement
- Acknowledgements
- Financial support
- Review statement
- References
- Supplement
- Abstract
- Introduction
- The EARLINET/ACTRIS COVID-19 campaign
- Data and tools
- Methodology
- Results
- Issues identified from running GRASP/GARRLiC on a network level
- Summary and conclusions
- Appendix A
- Appendix B: Calculation of the GRASP/GARRLiC retrieval uncertainties for the retrieved aerosol backscatter and extinction coefficients
- Data availability
- Author contributions
- Competing interests
- Disclaimer
- Special issue statement
- Acknowledgements
- Financial support
- Review statement
- References
- Supplement