the Creative Commons Attribution 4.0 License.
the Creative Commons Attribution 4.0 License.
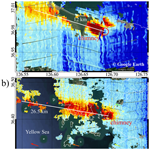
Highly resolved mapping of NO2 vertical column densities from GeoTASO measurements over a megacity and industrial area during the KORUS-AQ campaign
Gyo-Hwang Choo
Kyunghwa Lee
Hyunkee Hong
Ukkyo Jeong
Wonei Choi
Scott J. Janz
The Korea–United States Air Quality (KORUS-AQ) campaign is a joint study between the United States National Aeronautics and Space Administration (NASA) and the South Korea National Institute of Environmental Research (NIER) to monitor megacity and transboundary air pollution around the Korean Peninsula using airborne and ground-based measurements. Here, tropospheric nitrogen dioxide (NO2) slant column density (SCD) measurements were retrieved from Geostationary Trace and Aerosol Sensor Optimization (GeoTASO) L1B data during the KORUS-AQ campaign (2 May to 10 June 2016). The retrieved SCDs were converted to tropospheric vertical column densities using the air mass factor (AMF) obtained from a radiative transfer calculation with trace gas profiles and aerosol property inputs simulated with the Community Multiscale Air Quality (CMAQ) model and surface reflectance data obtained from the Moderate Resolution Imaging Spectroradiometer (MODIS). For the first time, we examine highly resolved (250 m × 250 m resolution) tropospheric NO2 over the Seoul and Busan metropolitan regions and the industrial region of Anmyeon. We reveal that the maximum NO2 vertical column densities (VCDs) were 4.94×1016 and 1.46×1017 molec. cm−2 at 09:00 and 15:00 LT over Seoul, respectively, 6.86×1016 and 4.89×1016 molec. cm−2 in the morning and afternoon over Busan, respectively, and 1.64×1016 molec. cm−2 over Anmyeon. The VCDs retrieved from the GeoTASO airborne instrument were correlated with those obtained from the Ozone Monitoring Instrument (OMI) (r=0.48), NASA's Pandora Spectrometer System (r=0.91), and NO2 mixing ratios obtained from in situ measurements (r=0.07 in the morning, r=0.26 in the afternoon over the Seoul, and r>0.56 over Busan). Based on our results, GeoTASO is useful for identifying NO2 hotspots and their spatial distribution in highly populated cities and industrial areas.
- Article
(12602 KB) - Full-text XML
- BibTeX
- EndNote
Nitrogen dioxide (NO2) is one of the most important atmospheric trace gases and plays a key role in aerosol production and tropospheric ozone photochemistry (Boersma et al., 2004; Richter et al., 2005). Furthermore, high concentrations of NO2 in the atmosphere have adverse effects on human health, such as respiratory infections and associated symptoms (Brauer et al., 2002; Latza et al., 2009).
The main sources of NO2 in the atmosphere are fossil fuel combustion from vehicles and thermal power plants, lightning, and biogenic soil processes. Furthermore, NO2 concentrations are highly correlated with population size (Lamsal et al., 2013). The implementation of emission control technology and environmental regulation has led to a decrease in surface NO2 concentrations in western Europe, the United States, and Japan in the last few decades (Richter et al., 2005). The concentration of NO2 in major metropolitan cities in South Korea and China is over 3 times larger than over similarly sized cities in Europe and the United States, despite NO2 concentrations decreasing in China and South Korea (de Foy et al., 2016; Choo et al., 2020).
To date, several low-orbit spaceborne sensors, such as the Global Ozone Monitoring Experiment (GOME) (Burrows et al., 1999), the Scanning Imaging Spectrometer for Atmospheric Cartography (SCIAMACHY) (Burrows et al., 1995), the Ozone Monitoring Instrument (OMI) (Levelt et al., 2006), GOME-2 (Callies et al., 2000), and the Tropospheric Monitoring Instrument (TROPOMI) (Veefkind et al., 2012), have monitored atmospheric ozone and its precursors, including NO2 and formaldehyde (HCHO) as a proxy for volatile organic compounds (VOCs). Furthermore, the Geostationary Environment Monitoring Spectrometer (GEMS) (Choi et al., 2018; Kim et al., 2020), which was launched on 18 February 2020, will form a constellation of geostationary satellites, including the upcoming Tropospheric Emission: Monitoring of Pollution (TEMPO) (Zoogman et al., 2017) and Sentinel-4 platforms, to continuously observe the air quality of the Northern Hemisphere during the day.
NO2 retrievals from spaceborne hyperspectral measurements are typically conducted using the differential optical absorption spectroscopy (DOAS) method (Platt and Stutz, 2008) to first retrieve the view-dependent slant column density (SCD), and then radiative transfer models are used to determine the vertical column density (VCD) using an air mass factor (AMF) correction. Previous and ongoing spaceborne instruments use various radiative transfer codes and model input assumptions to calculate NO2 AMF values at coarse spatial resolution. Because AMF weighting has a large impact on NO2 retrievals using the DOAS method, it is important to use model input assumptions that most accurately match viewing and atmospheric conditions. Several studies have demonstrated the sensitivity of AMF calculations to inaccurate model input parameters (e.g., a priori NO2 vertical profile and aerosol properties) and a priori data (cloud information and surface reflectance) (Leitão et al., 2010; Hong et al., 2017; Lorente et al., 2017; Boersma et al., 2018). NO2 retrievals have also been consistently conducted based on surface remote sensing measurements, including the Multi-Axis DOAS (MAX-DOAS), Système D'Analyse par Observations Zènithales (SAOZ) spectrometer (Pastel et al., 2014), and Pandora (Herman et al., 2009) systems. These ground-based measurements can be used as validation references for both airborne and spaceborne measurements.
NO2 retrievals from airborne remote sensing instruments, such as the Geostationary Coast and Air Pollution Event (GEO-CAPE) Airborne Simulator (GCAS) (Kowalewski and Janz, 2014), the Heidelberg Airborne Imaging DOAS Instrument (HAIDI) (General et al., 2014), the Geostationary Trace gas and Aerosol Sensor Optimization (GeoTASO) (Leitch et al., 2014), the Airborne Prism Experiment (APEX; Popp et al., 2012), the Airborne Imaging DOAS instrument for Measurements of Atmospheric Pollution (AirMAP; Meier et al., 2017; Schönhardt et al., 2015), the Small Whiskbroom Imager for atmospheric compositioN monitorinG (SWING; Merlaud et al., 2018), and the Spectrolite Breadboard Instrument (SBI; Vlemmix et al., 2017; Tack et al., 2019), have also been performed to identify local emission sources and obtain highly resolved horizontal NO2 distributions.
Observations using airborne measurements have an advantage as they enable the observation of horizontal distributions of trace gases at resolutions higher than those of space-based satellites and provide data over a wider area than those of ground-based observations. For example, Nowlan et al. (2018) retrieved tropospheric NO2 VCDs over Houston, Texas, during the Deriving Information on Surface Conditions from Column and Vertically Resolved Observations Relevant to Air Quality (DISCOVER-AQ) campaign and identified a high correlation with data retrieved from Pandora. Popp et al. (2012) also presented the morning and afternoon NO2 spatial distribution in Zurich, Switzerland, using APEX. Tack et al. (2017) have conducted high-resolution mapping of NO2 over three Belgium cities (Antwerp, Brussels, and Liège) using APEX, and Judd et al. (2020) and Tack et al. (2021) compared NO2 VCDs retrieved from GCAS/GeoTASO and APEX with those obtained from TROPOMI over New York City and over Antwerp and Brussels, respectively. Merlaud et al. (2013) observed NO2 VCDs in Turceni over Romania using SWING mounted on an uncrewed aerial vehicle (UAV) during the Airborne Romanian Measurements of Aerosols and Trace gases (AROMAT) campaign. These existing NO2 retrievals using airborne measurements have been useful in constraining regional air quality models due to the highly resolved source identification and the ability to tie these results to ground-based observations.
This work focuses on airborne NO2 retrievals from GeoTASO. This instrument was developed by Ball Aerospace to reduce mission risk for UV-Vis air quality measurements from geostationary orbit for the GEMS and TEMPO missions (Leitch et al., 2014). The retrieval of NO2, SO2, and HCHO observed from GeoTASO L1B data using DOAS and principal component analysis (PCA) (Wold et al., 1987) was conducted through the DISCOVER-AQ and Korea–United States Air Quality (KORUS-AQ) campaigns (Nowlan et al., 2016; Judd et al., 2018; Choi et al., 2020; Chong et al., 2020). The KORUS-AQ campaign was a joint study organized from May to June 2016 between the National Institute of Environmental Research (NIER) and National Aeronautics and Space Administration (NASA) to monitor megacity air pollution and transboundary pollution and to prepare for geostationary satellite (i.e., GEMS, TEMPO, and Sentinel-4) air quality observability (of trace gases and aerosols).
Although surface NO2 concentrations in South Korea are the high due to high population density, high traffic volumes, and many industrial complexes and thermal power plants, and although NO2 retrieval studies using airborne and ground measurements in North America, Europe, China, and Japan exist, data for South Korea remain limited. The specific objectives of this study are as follows: to retrieve tropospheric NO2 vertical column data using GeoTASO measurements over polluted regions of the Seoul and Busan metropolitan areas and the Anmyeon industrial region of the Korean Peninsula; to estimate NO2 VCD uncertainties using error propagation accounting for spectral fitting errors and AMF uncertainties associated with input data errors, including aerosol optical depth (AOD), single scattering albedo (SSA), aerosol peak height (APH), and surface reflectance (SR); and to compare NO2 VCDs retrieved from GeoTASO and those obtained from OMI and ground-based Pandora instruments, as well as surface in situ measurements.
2.1 Campaign area
The Korean Peninsula, located on the Asia-Pacific coast, has a complex atmospheric environment due to local emissions and long-range transport under appropriate weather conditions (Jeong et al., 2017; NIER and NASA, 2020; Choo et al., 2020). Seoul, the capital of South Korea, and its metropolitan area are densely populated, while power plants and industrial activities on the northwestern coast emit relatively large amounts of pollutants. The KORUS-AQ campaign conducted three-dimensional observations, including ground-based remote, aircraft, and satellite observations, and air quality modeling to understand the complex air quality and interpret the observations of GEMS launched in 2020. The KORUS-AQ campaign period was from 2 May to 10 June 2016. During the KORUS-AQ campaign, air pollutants were conducted using the GeoTASO on board the NASA Langley Research Center B200 aircraft to monitor air quality and long-range transport of pollutants over the Korean Peninsula (NIER and NASA, 2020). The GeoTASO observations were conducted 30 times in 23 d out of 40 d. Most observations were made once or twice a day. Each flight was planned and conducted on a day when the weather conditions were fine and flight hours were approximately 2–4 h. We show the average values of GeoTASO flight information, such as flight time, altitude, speed, solar zenith angle (SZA), and viewing zenith angle (VZA) for the dates retrieved for NO2 VCD; aerosol properties (AOD, SSA) extracted from the Community Multiscale Air Quality (CMAQ) model; and cloud fraction and SR extracted from the Moderate Resolution Imaging Spectroradiometer (MODIS) in Table 1. Flight information about the date of the aircraft observation can be found at http://www-air.larc.nasa.gov/missions/korus-aq/docs/KORUS-AQ_Flight_Summaries_ID122.pdf (last access: 26 October 2022). Figure 1 indicates the flight routes of B200 and the tropospheric NO2 VCD obtained from the OMI during the campaign period. The observations were concentrated in the metropolitan areas of Seoul and Busan and the industrial area of Anmyeon, with an average flight altitude of ∼8.5 km during KORUS-AQ.
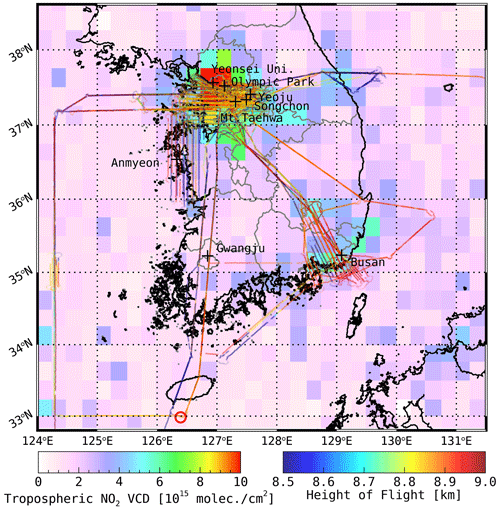
Figure 1Flight paths of the NASA LaRC B200 aircraft carrying GeoTASO and the average tropospheric NO2 VCDs obtained from OMI gridded to a 0.25∘ × 0.25∘ horizontal grid during the KORUS-AQ campaign period. The color of each line represents flight height. In this period, the GeoTASO observations focused on megacities (Seoul and Busan) and an industrial complex area (Anmyeon) with high tropospheric NO2 concentrations. The reference spectrum for spectral fitting is obtained from the radiation data over Jeju Island (marked with a red circle).
Table 1Summary of information on the dates when NO2 VCD was retrieved during the KORUS-AQ period (LT = UTC+ 9 h). The average values of GeoTASO data sets for flight characteristics, aerosol properties, geometric information, and cloud information are given
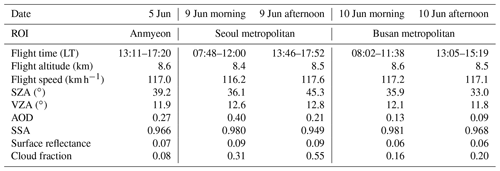
As shown in Fig. 1, GeoTASO observations were conducted focusing on highly NO2-polluted regions in the Seoul and Busan metropolitan areas and the Anmyeon region during the KORUS-AQ campaign. The Seoul metropolitan area (Seoul Special City, Gyeonggi Province, and Incheon City) is one of the most densely populated areas worldwide, with a population of approximately 20 million in 2016. Busan is the second-largest city in South Korea, with a population of approximately 3.4 million in 2016. Anmyeon is located southwest of Seoul, with petrochemical complexes, steel mills, and thermal power stations in the area. The background color in Fig. 1 represents the average NO2 VCD obtained from the OMI during the KORUS-AQ campaign period, showing over 1×1016 molec. cm−2 over the Seoul metropolitan area. The OMI data were obtained with the Level 2.0 OMNO2 version 3.0 and downloaded from the NASA Earthdata search (http://search.earthdata.nasa.gov/search/, last access: 19 January 2023). We calculated the arithmetic means of tropospheric NO2 VCDs, like Choo et al. (2020), to obtain the grid data (0.25∘ × 0.25∘) during the KORUS-AQ period. The average tropospheric NO2 VCD data were excluded from 30 May to 9 Jun 2016, when the OMI data did not exist during the campaign period.
2.2 Pandora
NO2 VCDs retrieved from the GeoTASO were validated using those from NASA's Pandora Spectrometer system. The Pandora spectrometer is a hyper-spectrometer that can provide direct sun measurements of UV-Vis spectra (280–525 nm with a full width at half maximum (FWHM) of 0.6 nm) for observing atmospheric trace gases. During the KORUS-AQ, eight Pandora instruments monitored NO2 and ozone (O3) VCD as depicted by plus symbols in Fig. 1. The retrieved data are available on the KORUS-AQ pages of NASA's Goddard Space Flight Center website (https://avdc.gsfc.nasa.gov/pub/DSCOVR/Pandora/DATA/KORUS-AQ/, last access: 19 October 2022). We compared NO2 VCDs obtained from five Pandora measurements (Busan university: 35.24∘ N, 129.08∘ E; Olympic park: 37.52∘ N, 127.13∘ E: Songchon: 37.41∘ N, 127.56∘ E; Yeoju: 37.34∘ N, 127.49∘ E; Yonsei University: 37.56∘ N, 126.93∘ E) within 0.05∘ and 30 min with those from GeoTASO. Because NO2 has a short atmospheric lifetime, especially during the summer (Shah et al., 2020), its spatial and temporal distributions vary notably. A detailed description of Pandora's operation during the KORUS-AQ campaign has previously been reported (Herman et al., 2018; Spinei et al., 2018).
2.3 Ground-based in situ NO2 measurement
Although the basic physical quantity of VCD and the surface mixing ratio from in situ measurements are different, comparison of their spatiotemporal variations provides useful information for deriving surface air quality from airborne instruments (e.g., Jeong and Hong, 2021a, b). In this study, we compare the NO2 VCDs (molec. cm−2) retrieved from GeoTASO to surface mixing ratios measured by ground-based in situ monitoring network over South Korea (i.e., Air-Korea, a national real-time air quality network; https://www.airkorea.or.kr/, last access: 19 January 2023). The instruments use the chemiluminescence method (Kley and McFarland, 1980), and approximately 400 air quality monitoring sites in South Korea are registered in the system, providing hourly surface NO2 concentrations. We compared NO2 VCDs retrieved from GeoTASO within 0.5 km and 30 min with NO2 concentrations obtained from Air-Korea.
2.4 GeoTASO measurements
NO2 VCDs were retrieved from the L1B radiance dataset (version: V02y) obtained using GeoTASO during the KORUS-AQ campaign. The NASA Goddard Space Flight Center conducted the L1B radiance calibration, which included offset and smear correction, gain matching, amplifier cross-talk correction, dark rate correction, integration normalization, sensitivity derivation, wavelength registration, geo-registration, nonlinearity correction, and ground pixel geolocation (Kowalewski et al., 2017; Chong et al., 2020). The detailed specifications of GeoTASO are listed in Table 2 (Nowlan et al., 2016).
2.4.1 NO2 slant column density retrieval
Figure 2 indicates the flowchart for retrieving the tropospheric NO2 VCD from the GeoTASO. We first retrieved NO2 SCDs using the DOAS method (Platt, 1994). Nonlinear least-squares minimization was used to retrieve the NO2 SCDs, which minimizes the difference between the measured optical depth and the modeled value in QDOAS software (Eq. 1; Danckaert et al., 2012):
where I(λ) is the measured earthshine radiance at wavelength λ; I0 is the reference radiance from the reference sector (ocean south of Jeju Island denoted with the red circle in Fig. 1; 32.983∘ N, 126.392∘ E) at 09:00 LT on 1 May 2016. The Community Multiscale Air Quality (CMAQ) modeling system data indicated that the NO2 VCD from the surface to 50 hPa over this reference sector on this day was 6.75×1015 molec. cm−2, and the mean of total NO2 VCD obtained from the OMI during the KOURS-AQ period was 4.77×1015 molec. cm−2 with a standard deviation of 1.33×1015 molec. cm−2. We also confirmed the stability of NO2 distribution over this area using the TROPOMI offline data from 2019 to 2020. In this period, the NO2 VCD from the TROPOMI was 4.81×1015 molec. cm−2 with a standard deviation of 0.43×1015 molec. cm−2. The NO2 VCD used as a reference sector obtained from CMAQ was mainly dominated by stratospheric NO2 VCD. However, stratospheric NO2 VCD has a relatively lower value compared to tropospheric NO2 VCD. The ρj represents the SCD of each species j; represents the differential gas phase absorption cross section convolved with the Gaussian distribution function (GDF) with GeoTASO FWHM (the UV and VIS range were 0.34–0.49 nm and 0.70–1.00 nm, respectively; Nowlan et al., 2016) at wavelength λ of species j, respectively.
We used the measured radiances at the reference sector to calculate differential slant column density (dSCD) over the whole domain of the GetoTASO measurements. CMAQ calculation over the reference sector (i.e., 6.75×1015 molec. cm−2) was adopted as the reference SCD (SC0), which is added to all dSCD values to convert to the SCD. The reference sector is known as a background area but is occasionally affected by the long-range transport of NO2 from upwind areas. Considering the standard deviation of the OMI measurements accounts for such effects during the measurement period, we estimate the maximum uncertainties of the SC0 can be calculated from this value (i.e., 1.33×1015 molec. cm−2) in addition to the difference in the mean values between CMAQ and OMI (i.e., 1.98×1015 molec. cm−2). Therefore, our best estimate of the uncertainty of the SC0 is the root of the sum of squares of these values (i.e., 2.38×1015 molec. cm−2).
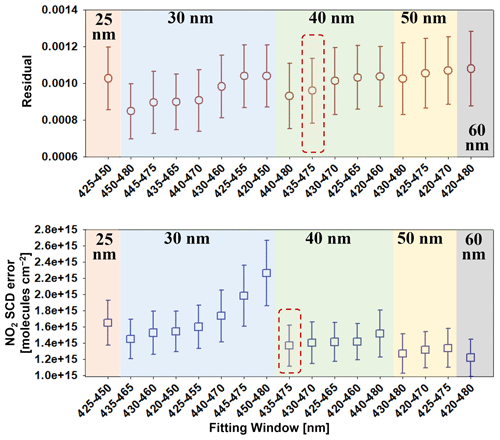
Figure 3Residuals and NO2 SCD errors of 17 spectral fitting window candidates (17 May 2016, with an across-track number of 15).
The spectral fitting window was selected based on the sensitivity test with 17 fitting window candidates from 420 to 480 nm with a fitting window length from 25 to 60 nm. Spectral fitting residuals and NO2 SCD errors have been investigated for 17 spectral fitting window candidates (Fig. 3).
In terms of the residual, when the NO2 fitting window includes a wavelength region less than 430 nm it has a larger residual compared to the case where it does not. The higher residual can include the more noise signals that cannot be calculated mathematically, which can become an uncertainty for the NO2 SCD retrievals. Therefore, we excluded the fitting window, which includes wavelengths less than 430 nm for the GeoTASO NO2 retrievals during the KORUS-AQ campaign. In the case of the NO2 SCD error, it was confirmed that the longer the fitting window length was, the lower the NO2 SCD error appeared regardless of including the wavelength region less than 430 nm. Therefore, for the stable NO2 SCD retrieval, an appropriate spectral fitting window needs to be selected that can minimize the residual with a moderate length of the fitting window. To find the optimal fitting window, we set the threshold value based on the above results: residual <0.001, NO2 SCD error molec. cm−2, and the length of fitting window >30 nm. Following this, the fitting window of 435–475 nm was selected for the GeoTASO NO2 retrievals during the KORUS-AQ campaign. To determine the wavelength registration more accurately in the narrow fitting window, additional wavelength calibration of the spectra for each of the 33 across-track pixels was performed using a high-resolution solar reference spectrum (Kurucz solar spectrum) (Chance and Kurucz, 2010) with the GDF. The absorption cross sections of NO2 (Vandaele et al., 1998), O3 (Bogumil et al., 2000), H2O (Rothman et al., 2010), and the Ring effect as pseudo-absorbers (Chance and Spurr, 1997) were used to construct the model equation, while B(λ), R(λ), A(λ), and N(λ) are the broad absorption of trace gases, extinction by Mie and Rayleigh scattering, variation in the spectral sensitivity of the detector or spectrograph, and noise, respectively, which were accounted for by an eighth-order polynomial. An example of the spectral fitting results is presented in Fig. 4.
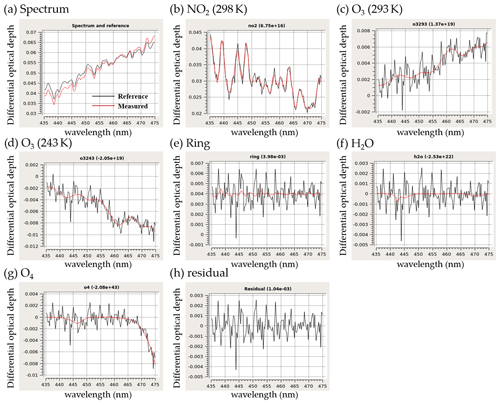
Figure 4An example of the spectral fitting results of NO2 retrievals from GeoTASO during the KORUS-AQ campaign (at Gangnam, Seoul on 9 June 2016). The red and black line in panel (a) represent the measured and reference spectrum, respectively. Panels (b) to (h) depict examples of spectral fitting results of (b) NO2, (c) O3 (293 K), (d) O3 (243 K), (e) Ring effect, (f) H2O, and (g) O4, where red and black lines are the absorption cross section of the target species and the fitting residual plus the absorption of the target species, respectively. Panel (h) indicates the fitting residual of this example.
2.4.2 NO2 AMF calculation
AMF, the ratio of SCD to VCD, can be calculated using the scattering weight (ω) and shape factor (S) (Palmer et al., 2001) in Eq. (2)–(5):
where AMFG represents the geometric AMF, IB is the earthshine radiance, τ is the optical depth, α is the absorption cross section, and n is the number density of the absorber. NO2 AMF was calculated using a linearized pseudo-spherical scalar and vector discrete ordinate radiative transfer model (VLIDORT, version 2.6; Spurr and Christi, 2014). Aerosol properties, such as AOD, SSA, APH, and a priori NO2 vertical profile information, were simulated using the CMAQ, and surface reflectivity was obtained from MODIS (Collection 6). The SR products, MCD43A3, available at a 500 m spatial resolution, provide an estimate of the surface spectral reflectance including MODIS bands 1 through 7. Here, MODIS band 3 (459–479 nm) was used because this band is the closest the wavelength (455 nm) used in the calculation of AMF in this study. APH was assumed to be the peak height of the aerosol extinction coefficient simulated in CMAQ, and the aerosol profile applied GDF based on APH (Hong et al., 2017). For pixels without reflectance information, AMF was not calculated. The products were corrected for atmospheric conditions, such as aerosol, gases, and Rayleigh scattering. In previous studies (Lamsal et al., 2017; Nowlan et al., 2018; Judd et al., 2019; Chong et al., 2020), an AMF was described for both above and below aircraft altitude is used to convert NO2 SCDs to VCDs using Eqs. (6)–(8).
where AMF↑ and AMF↓ are AMF above and below aircraft, respectively, and NO2 VCD↑ represents NO2 VCD above the aircraft obtained from a chemical transport model (CTM). However, here we calculated the NO2 VCD↓ by dividing NO2 SCDs by AMF↓ as the CMAQ only simulates the troposphere (surface to 50 hPa). However, as the stratospheric and free tropospheric NO2 (NO2 VCD↑) column densities over megacities and industrial areas are much lower than tropospheric NO2 column densities (Valks et al., 2011), we assume that the uncertainties in the AMF without considering the upper atmosphere are negligible in this study.
2.5 Chemical model description
Vertical profiles from CMAQ (Byun and Ching, 1999; Byun and Schere, 2006), a CTM, were used to calculate AMFs. The CMAQ simulations were conducted with a horizontal resolution of 15×15 km and had 27 vertical layers from the surface to 50 hPa. The meteorological fields were prepared using the advanced research Weather Research and Forecasting (WRF) Advanced Research WRF (ARW) Model (Skamarock et al., 2008). Anthropogenic emissions were generated based on the KORUS v5.0 model (Woo et al., 2012), and biogenic emissions were simulated using the Model of Emissions of Gases and Aerosols from Nature (MEGAN v2.1; Guenther et al., 2006, 2012). Besides anthropogenic and biogenic emissions, the Fire Inventory from NCAR (FINN; Wiedinmyer et al., 2006, 2011) was used to update the pyrogenic emission fields.
The CMAQ AOD was calculated by integrating the aerosol extinction coefficient (Qext), which is the sum of scattering (Qsca) and absorption (Qabs) coefficients over all vertical layers (z) as follows:
where ωij indicates SSA of particulate species i for the particulate mode (or size bin) j, βij denotes the mass extinction efficiency, fij(RH) is the hygroscopicity factor according to the relative humidity (RH), and [C]ij is the concentration of particulate species. CMAQ SSA is defined as the ratio of the integrated Qsca to AOD, and NO2 vertical profiles were obtained from NO2 concentrations at each vertical layer by conducting CMAQ simulations. Details of the model descriptions and calculations of optical properties are given by Lee et al. (2020) and Malm and Hand (2007).
3.1 NO2 VCD retrieval
3.1.1 Seoul metropolitan region
We show the final NO2 VCDs from 250 m spatial resolution. Because of NO2 VCD, we selected the dates observed in both the morning and afternoon during the KORUS-AQ period over the Seoul metropolitan area, Busan, and Anmyeon. The retrieved dates for NO2 VCDs were 5, 9, and 10 June 2016.
The population of the Seoul metropolitan region is approximately 20 million, which is approximately 40 % of the total population of South Korea. It is rare to obtain high-resolution horizontal NO2 VCD distributions using airborne measurements in the morning and afternoon, especially in Asian megacities. Figure 5 indicates tropospheric NO2 VCDs over Seoul on 9 June 2016, at 09:00 and 15:00 LT. Because of an issue with the imaging systems, enlarged views (Figs. 5–8) present a slightly stripy appearance from the GeoTASO observation (Nowlan et al., 2016; Chong et al., 2020).
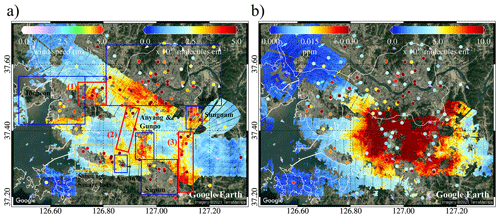
Figure 5Tropospheric NO2 VCD in the Seoul metropolitan region on 9 June 2016 retrieved from GeoTASO (a) at 09:00 and (b) at 15:00 LT. The red boxes represent expressways (counterclockwise from left to right, (1) Gyeongin Expressway, (2) Seohaean Expressway, and (3) Gyeongbu Expressway), the orange box indicates the industrial complex, and the blue boxes indicate the major cities (Seoul, Incheon, Suwon, Bucheon, Anyang, Gunpo, Sungnam, and Ansan) of the Seoul metropolitan region. The colors of the circles depict the NO2 surface mixing ratio obtained from Air-Korea. The colored arrows indicate the wind direction and speed at 1000 hPa over the Seoul metropolitan region obtained via the Unified Model (UM) simulations (the background RGB image is from Google Earth; https://www.google.com/maps/, last access: 19 January 2023).
In the morning, NO2 VCDs retrieved from GeoTASO were highly correlated with expressways (red boxes in Fig. 5), such as the Gyeongin, Seohaean, and Gyeongbu expressways, and over major cities with heavy traffic, such as Seoul, Bucheon, Ansan, Anyang, and Suwon. GeoTASO observed NO2 VCD values that were 3 times higher ( molec. cm−2) in these areas compared to the surrounding rural areas. High NO2 VCD values above 6×1016 molec. cm−2 were observed above the Gyeongin Expressway, which has very heavy traffic in a relatively short section, and the Gunpo Complex Logistics zone, where diesel vehicle traffic is also high. The main NO2 source regions and the regions where high NO2 VCD values were observed were highly consistent at 09:00 LT because the wind speed at this time – as obtained from the Unified Model (UM)-based Regional Data Assimilation and Prediction System (RDAPS) of the Korea Meteorological Administration (KMA) – was as low as 0.1 ms−1, and the average wind direction was 84.7∘ at 1000 hPa over Seoul metropolitan region. The average daily traffic volume of these expressways exceeds 150 000 vehicles, and the total number of vehicles registered in these major cities is >6 000 000, with an average daily mileage per car per day of over 38 km. Detailed information on these cities and expressways is listed in Tables 3 and 4. Based on the level of vehicular traffic, combustion using gasoline and diesel engines leads to high overall emissions of NO2 in the Seoul metropolitan region (Kendrick et al., 2015).
Table 3The population, number of registered vehicles, and average mileage per car per day of the major cities in the Seoul and Busan metropolitan region obtained from the Korean Statistical Information Service (https://kosis.kr/eng, last access: 25 March 2022).
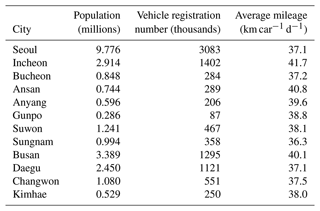
Table 4Daily average traffic volume on the Gyeongin, Gyeongbu, and Seohaean expressways obtained using the Traffic Monitoring System (https://www.road.re.kr, last access: 28 February 2022).
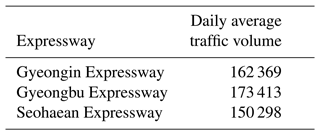
Compared to the data of the morning, the average wind speed and wind direction were 1.7 ms−1 and 284.5∘ at 1000 hPa in the afternoon, and the afternoon had extremely high tropospheric NO2 VCD values (exceeding 5×1016 molec. cm−2) in most of the Seoul metropolitan region, including rural areas, whereas the NO2 mixing ratio (MR) obtained from Air-Korea decreases in the afternoon. According to Tzortziou et al. (2018), similar results were retrieved from the Pandora site in Seoul, with higher afternoon NO2 VCDs than in the morning. This result is because the amount of NO2 produced by chemical conversion of nitric oxide (NO) by O3 and VOCs in the atmosphere, along with NOx generated by regional emissions (traffic) in the Seoul metropolitan region, is greater than the amount lost by photolysis and transport to nearby areas (Herman et al., 2018). Furthermore, the increase in tropospheric NO2 VCD in the afternoon is likely due to the accumulation and dispersion of NO2 according to the height of the change in the planetary boundary layer (Ma et al., 2013).
3.1.2 Industrial and power plant regions in Anmyeon
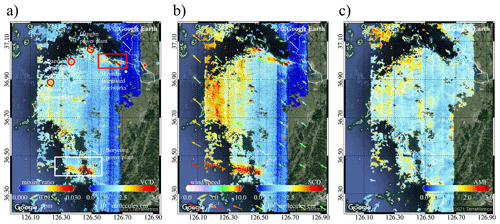
Figure 6(a) Tropospheric NO2 VCD and (b) NO2 SCD retrieved from GeoTASO and (c) NO2 AMF at native resolution (250 m) calculated using VLIDORT over Anmyeon in South Korea on 5 June 2016. The colored arrows indicate wind speed and wind direction at 850 hPa from the Unified Model (UM) simulations. The red circles and rectangle in (a) represent the major NO2 emission sources, such as steelworks and power plants (background RGB image is from Google Earth; https://www.google.com/maps/, last access: 19 January 2023).
The high spatial resolution of the tropospheric NO2 VCD from GeoTASO over the Anmyeon industrial region, where many industrial facilities and several power plants are distributed, is shown in Fig. 6. Figure 6a and b indicate tropospheric NO2 VCD and NO2 SCD retrieved from GeoTASO L1B data, respectively, between 13:00 and 17:00 LT on 5 June 2016. Figure 6c depicts the calculated AMF of NO2 from native resolution over the domain. GeoTASO observations detected the following moderate and strong NO2 emission sources in this area: (1) Boryeong power plant, (2) Hyundai integrated steelworks, (3) Dangjin power plant, (4) Daesan petrochemical complex, and (5) Taean power plant. High NO2 VCD values ( molec. cm−2) were observed over steel mill works, petrochemical complexes, and power plants, whereas values were comparatively low ( molec. cm−2) over small cities with populations of less than 0.1 million, including Seosan, Dangjin, and Boryeong, and the Seohaean Expressway. In 2016, the annual NOx emissions from the Hyundai steelworks and the Dangjin and Boryeong power plants were approximately 10.3, 11.9, and 16.8 kt yr−1, respectively. The NOx emission rates of major industrial facilities in the Anmyeon region are shown in Table 5.
Table 5NOx emission rates in 2016 from major industrial facilities in the Anmyeon region obtained from the Continuous Emission Monitoring System of the Korea Environment Corporation (https://www.stacknsky.or.kr/eng/index.html, last access: 25 March 2022).
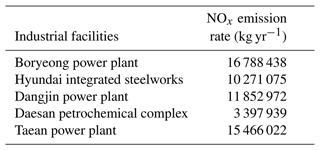
Figure 6 shows high NO2 concentrations of the main industrial facilities in the Anmyeon region, where the combustion of fossil fuel in factories and thermal power plants leads to high emissions (Prasad et al., 2012). Due to relatively sparse distribution over rural areas, the Air-Korea measurements did not detect the major NO2 plume as shown in Fig. 6a. Thus, airborne remote sensing systems, such as GeoTASO, can effectively complement ground-based networks for monitoring minor and major NOx emissions, particularly over these remote industrial regions.
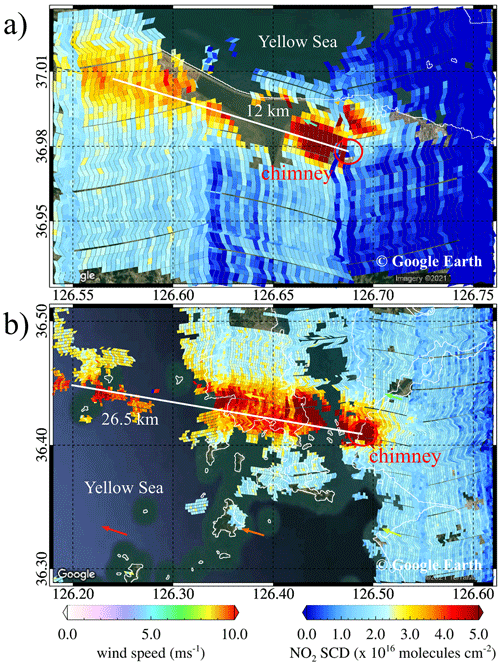
Figure 7Enlarged view of GeoTASO tropospheric NO2 SCD observation over (a) the Hyundai steel works, indicated by the red box in Fig. 6, and (b) the Boryeong power plant, indicated by the white box in Fig. 6. The arrows represent the wind direction and speed at 850 hPa from the Unified Model (UM) simulations (the background RGB image is from Google Earth; https://www.google.com/maps/, last access: 19 January 2023).
The GeoTASO data captured not only NOx emissions from the chimneys of steelworks and power plants but also its transport by the wind. Figure 7a and b show enlarged views of tropospheric NO2 SCD retrieved using GeoTASO over the Hyundai steelworks (red box in Fig. 6) and the Boryeong power plant (white box in Fig. 6). The arrows in Fig. 7 represent the prevailing wind direction and speed from RDAPS. NO2 emitted from the chimneys of these sites was transported to the Yellow Sea, traveling distances of over 26.5 km at speeds of approximately 6 ms−1. According to Chong et al. (2020), similar results were found for SO2 emitted and transported from these sites.
3.1.3 Busan metropolitan region
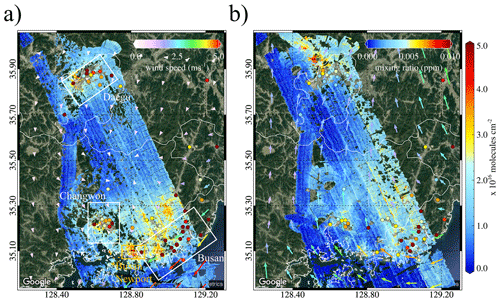
Figure 8Tropospheric NO2 VCD in the Busan metropolitan region in the (a) morning and (b) afternoon of 10 June 2016. The wind speed (color scale) and wind direction (arrows) at 1000 hPa pressure level were obtained from the Unified Model (UM) simulations. The white boxes represent the major cities of Busan, Daegu, and Changwon. The orange box represents Busan New Port (the background RGB image is from Google Earth; https://www.google.com/maps/, last access: 19 January 2023).
Figure 8a and b show tropospheric NO2 VCD retrieved from the GeoTASO L1B data over the Busan metropolitan region on 10 June 2016 in the morning (between 08:00 and 11:00 LT) and afternoon (between 13:00 and 16:00 LT), respectively. The arrows in Fig. 8 indicate the wind speed and wind direction at 1000 hPa obtained from the UM-RDAPS, with the average wind speed and wind direction of 0.9 ms−1 and 55.4∘ and 1.9 ms−1 and 147.0∘, respectively, in the morning and afternoon. High NO2 VCDs were observed above urban areas, ports, industrial complexes, and the inter-city road between Busan and Changwon. Like the Seoul metropolitan region, combustion using gasoline and diesel engines is estimated to contribute to the high NOx emission. In the morning, NO2 VCDs were high (approximately 3×1016 molec. cm−2) in the major cities and especially around Busan New Port, with values exceeding 7×1016 molec. cm−2. In comparison, in the mountainous regions between Daegu and Busan, the NO2 VCD values were less than 1×1016 molec. cm−2 during the same period. The spatial distribution of tropospheric NO2 VCDs was similar in the Seoul metropolitan regions, with high values over major cities and roads (compare Figs. 5 and 8). In Busan, fossil fuel combustion in use by both road vehicles and ships is likely to contribute to the NOx emissions. In the afternoon, unlike the Seoul metropolitan region, tropospheric NO2 VCD over Busan decreased by over 3×1016 molec. cm−2, which also corresponds with NO2 MR data obtained from the Air-Korea sites. Detailed information on these cities is listed in Table 3.
3.2 Error estimation
The accuracy of the NO2 VCD retrieval using the DOAS method depends on both the AMF calculation and the spectral fitting error of the SCD retrieval. Retrieval errors of the NO2 VCD were estimated using error propagation analysis as expressed in Eq. (12):
where εVCD is the total error of NO2 VCD. The error of NO2 SCD (εSCD) is obtained from the spectral fitting error of NO2 SCD via the DOAS spectral fitting. εAMF indicates the error of NO2 AMF caused by uncertainties in the model input parameters for AMF calculation. Uncertainties in aerosol properties (AOD, SSA, and APH) and SR for the radiative transfer model (RTM) calculations are the major factors affecting NO2 AMF accuracy (Boersma et al., 2004; Leitão et al., 2010; Hong et al., 2017). Therefore, in this present study we quantified the NO2 AMF errors (εAMF) due to uncertainties in the input parameters independent of each other using Eq. (13):
where are partial derivatives of NO2 AMF regarding the input parameters (χi), σχi represents the uncertainty of the χi. The σ of AOD, SSA, SR, and APH are assumed to be 30 % (Ahn et al., 2014), 0.04 (Jethva et al., 2014), 0.005 + 0.05 × SR (EOS Land Validation; https://landval.gsfc.nasa.gov, last access: 22 July 2022), and 1 km (Fishman et al., 2012), respectively, in this study. To derive , the true χi is input to the RTM to simulate “true” NO2 AMF. For the AOD, SSA, APH, and SR, perturbed NO2 AMF was simulated using RTM with χi±σχi. ∂χi denotes the difference between the “center” χi and χi±σχi, and ∂AMF is the difference between the “center” NO2 AMF (AMFcentre) simulated with “center” input values and the perturbed NO2 AMF (AMFperturbed) simulated using the perturbed input parameters χi±σχi (i.e., the original input parameters modified by the uncertainty). The simulation for calculating the εAMF was conducted using the input parameters on 9 June 2016.
Table 6Total NO2 VCD caused by uncertainties in NO2 SCD and NO2 AMF (the average for the flight on 9 June 2016).
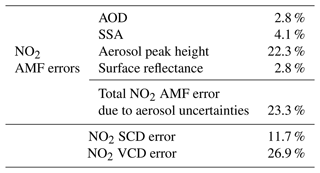
Table 6 lists the estimated NO2 VCD error on 9 June 2016 for each source based on the error propagation method. The error estimation was conducted for the pixels where the root-mean-square residual is <0.001 and NO2 VCD > 5 × 1015 molec. cm−2 since NO2 SCD precision is reported to be highly decreased in low NO2 conditions (Hong et al., 2017). The total NO2 VCD error was 26.9 %, with a high portion of NO2 AMF error. The NO2 SCD error was calculated to be 11.7 %, showing the importance of accurate DOAS spectral fitting for deriving NO2 SCD. The total AMF error due to uncertainties in the input parameters was calculated to be 23.3 %. Among model input parameters, the effect of APH on NO2 AMF becomes high (22.3 %), indicating the importance of accurate aerosol profile information. APH sensitivity affects NO2 AMF because aerosols lead to multiple scattering effects near the surface where trace gases and aerosols are well mixed, and the light absorption of trace gases is due to the increasing light path (Castellanos et al., 2015; Hong et al., 2017). APH has the potential to be the most important input parameter in the Asia region where high loadings of aerosol plumes persist throughout the year. The NO2 AMF calculation errors due to uncertainties in SSA and AOD were 4.1 % and 2.8 %, respectively. The NO2 AMF calculation error due to uncertainties in aerosol optical properties (SSA and AOD) appears to be smaller than those in a previous study (Leitão et al., 2010). The smaller effect of the aerosol properties can be explained by the moderate aerosol loading (AOD = 0.40) on the day of flight day. The NO2 AMF errors become larger under high AOD conditions. The smallest effect of SR was found on NO2 AMF calculation error, which was calculated based on the uncertainty of the SR of the satellite-based product (MODIS). Therefore, it may be an unrealistic number for the airborne NO2 AMF calculation. Once the uncertainty of airborne-based SR is provided, considering its measurement geometry and finer spatial resolution, more realistic airborne-based NO2 AMF calculation error due to uncertainties in SR can be estimated. The a priori NO2 profile shape can also be a factor that causes a calculation error for the NO2 AMF, as reported in previous studies (Leitão et al., 2010, Meier et al., 2017; Hong et al., 2017). Therefore, it is necessary to calculate the contribution of the shape of the a priori NO2 profile to the accuracy of NO2 AMF in the future. Moreover, the resulting uncertainties in input parameters of a GeoTASO ground pixel need to be considered by combining the initial uncertainties of CTM and satellite-based products and by using the variability of the parameters within the respective CTM (AOD, SSA, and APH) and satellite (SR) grid box. If values such as SR are assumed constant over larger areas, the fundamental spatial variability in these data increases the uncertainty of the AMF and hence of the determined NO2 VCD on the respective finer spatial scale. In addition, the uncertainty from the assumption on the SC0 and the uncertainty from ignoring the NO2 above the aircraft in the AMF calculations both need to be considered in the error analysis. This analysis should be considered in a further study.
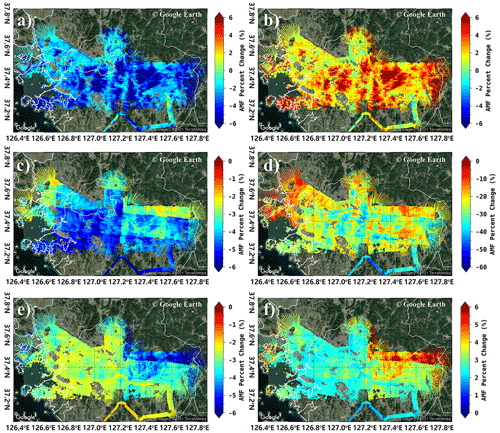
Figure 9Percent change between AMF calculated using the CMAQ model simulation and those using (a) 30 % lower AOD, (b) 30 % higher AOD, (c) 0.04 lower SSA, and (d) 1 km higher APH compared to the model outputs. The percentage change for AMF calculated using MODIS data and those using (e) SR lower SR and (f) 0.005 + 0.05 × SR higher SR are also shown (the background RGB image is from Google Earth; https://www.google.com/maps/, last access: 19 January 2023).
In this study, we also investigated the spatial distribution of AMF calculation errors associated with uncertainties in aerosol properties (AOD, SSA, and APH) and SR. The percent change in NO2 AMF (AMFpercent_change) was calculated on each spatial pixel using Eq. (14). Figure 9a and b indicate the percentage change error between the calculated AMFs using the CMAQ AOD data with 30 % lower (Fig. 9a) and 30 % higher (Fig. 9b) values, respectively. The AMF decreased and increased by up to 10 % with decreasing and increasing AOD, respectively, in the Seoul metropolitan region. We estimated that, under low aerosol loading conditions, an increase in AOD near the surface leads to an increase in the scattering probability within the surface layer with high NO2 concentrations. Figure 9c indicates the percent change error between the calculated AMFs using CMAQ SSA data with a 0.04 lower value. The AMF decreased with decreasing SSA because the absorption of light increased. APH was also found to greatly affect the accuracy of the AMF calculations (Fig. 9d). The APH uncertainty of 1 km decreased the AMFs with an average AMFpercent_change of −25 % on the flight day. On the pixels where AOD > 0.6 in particular, the average AMFpercent_change was found to be −26 %, whereas this value was −27 % on the pixels where AOD > 0.4, showing the combined effect of aerosol loading and aerosol profile shape on the NO2 AMF calculations. Figure 9e and f indicate the percentage change error between the calculated AMFs using the MODIS SR data with 0.005 + 0.05 × SR lower (Fig. 9e) and 0.005 + 0.05 × SR higher (Fig. 9f) values, respectively. The AMF decreased by approximately 3 % when the SR decreases, with the opposite occurring when it increased.
3.3 Validation of NO2 VCDs retrieved from GeoTASO
The tropospheric NO2 VCDs retrieved from GeoTASO L1B data (NO2,G) were compared with those obtained from OMI total NO2 VCDs (NO2,O) and Pandora (NO2,P). The NO2,O were only available for 10 June during the campaign period. Therefore, we compared only 48 NO2,G and NO2,O data points within a radius of 20 km and 30 min, which yielded a correlation coefficient of 0.48 with a slope of 0.13 (Fig. 10a). To validate this data, all NO2,G within a radius 20 km of the OMI center coordinate were averaged.
The NO2 values are relatively low, as GeoTASO observation is conducted in a region with low NO2 compared to the Seoul metropolitan region, and the overpass time of OMI is approximately 13:30 LT when NO2 decreased. The low slope value is because the OMI with low spatial resolution does not reflect the spatial NO2 inhomogeneity in the pixel.
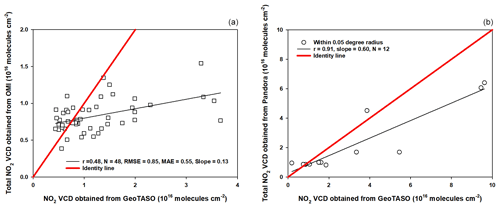
Figure 10Scatter plots of (a) NO2 VCD retrieved from GeoTASO and total NO2 VCD obtained from OMI and (b) total NO2 VCD obtained from Pandora and NO2 VCD retrieved from GeoTASO, respectively.
To compare NO2,G data, we made a comparison with total NO2 VCD obtained from the Pandora system (NO2,P) during the KORUS-AQ campaign period. NO2,P obtained from Busan University, Olympic Park, Songchon, Yeoju, and Yonsei University Pandora sites on 5, 9, and 10 June were used for the GeoTASO validation (Fig. 1). NO2,G and NO2,P columns at these sites are compared in Fig. 11. To compare NO2,G and NO2,P, we used averaged NO2,G retrieved from 16 across-track runs with the smallest viewing zenith angle possible and averaged 30 min NO2 data obtained from Pandora measurements within a radius of approximately 0.05∘. NO2,G and NO2,P were correlated (R=0.91, with a slope of 0.60); however, when NO2,P was lower than 1×1016 molec. cm−2, the correlation coefficient between NO2,G and NO2,P was <0.1. The weak correlation at low NO2 levels most likely reflects differences in viewing geometries and the horizontal inhomogeneity of the measured NO2 between Pandora and GeoTASO. Furthermore, Pandora and GeoTASO can be used for the NO2 validation of geostationary satellites, such as GEMS. However, because the number of Pandora measurements is limited in this campaign, we have difficulty validating NO2 retrieved from GeoTASO under various conditions. Many ground-based remote sensing measurements are needed to validate GEMS under various conditions.
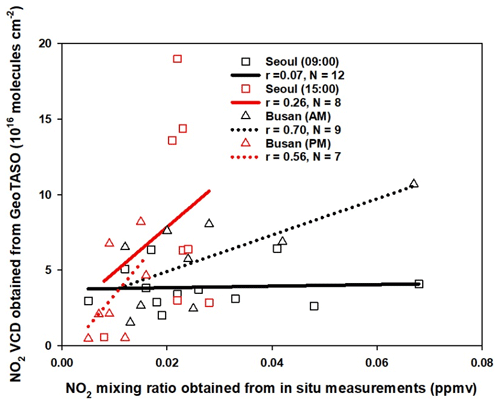
Figure 11Scatter plot of the NO2 VCDs retrieved from GeoTASO and the NO2 surface mixing ratio obtained from Air-Korea. The black and red squares represent the NO2 data at 09:00 and 15:00 LT in the Seoul metropolitan region, respectively. The black and red triangles represent those in the morning and afternoon over Busan, respectively.
To compare the spatiotemporal distribution of NO2 VCDs retrieved from GeoTASO, NO2,G was compared with surface spatial patterns and NO2,G was compared with NO2,A for GeoTASO data within a radius of approximately 0.05 km and 30 min (Fig. 11). To compare NO2,G and NO2,A, we used averaged NO2,G retrieved from 16 across-track runs and averaged 30 min within a radius of 0.05∘. Because in situ measurements provide NO2 volume mixing ratio (VMR) (NO2,A) (ppmv) once per hour, NO2,A of the nearest time is used to compare with NO2,G. The correlation coefficient (R) between NO2,G (molec. cm−2) and NO2,A at 09:00 and 15:00 LT in the Seoul metropolitan region was 0.07 and 0.26, respectively. When using only roadside station data from Air-Korea, the R value for the morning increased to 0.72, which implies GeoTASO is more sensitive to emissions from NO2 source areas, such as roadsides (Fig. 5). During the comparison there were large differences in the morning and afternoon. These results were identified because synoptic meteorology played an important role from 1 to 10 June 2016 (Choi et al., 2019). As described by Judd et al. (2018), the spatial distribution for NO2 VCDs appears to reflect the emission source in local industrialized regions and transportation in the morning with relatively weak winds. NO2 concentration often increases in the late morning, indicating that the emission process proceeds faster than the NO2 removal process. As the planetary boundary layer height (PBLH) increases in the early afternoon and surface NO2 is mixed through a deeper PBLH, the NO2 VCDs distribution showed a wider increase in most of the Seoul metropolitan area, while the column amounts continue to increase (Judd et al., 2018).
When comparing NO2 VCDs with surface NO2 concentrations, it should be highlighted that it is a nonlinear relationship between NO2,G and NO2,A. Although it may vary depending on weather conditions, high NO2 VCDs from airborne observations can sometimes be detected with low surface NO2 concentrations. When exhaust gases emitted from industrial facilities occur at a certain altitude (stacks or chimneys), NO2,G shows high NO2 VCDs, but NO2,A may be observed to have a low concentration. Unfortunately, in the Anmyeon industrial region, NO2,G and NO2,A could not be compared due to spatial restrictions because the distribution of ground observation stations is concentrated in metropolitan areas.
In the Busan metropolitan area, the R value of the NO2,G and NO2,A data had a correlation coefficient greater than 0.56. This reflects the more even horizontal distribution of NO2 in the afternoon, when diffusion from the source areas had occurred. However, for a more accurate comparison, NO2 VCD data should be converted to NO2 MR based on mixing layer height, temperature, and pressure profile data (Kim et al., 2017; Qin et al., 2017; Jeong and Hong, 2021a). However, because the number of Pandora and satellite data are limited in this campaign, we had difficulties in validating NO2 retrieved from GeoTASO under various conditions. Because ground-based, airborne, and spaceborne remote sensing measurements have their own advantages and disadvantages, it is recommended that a comprehensive observation campaign involving all of ground-based, airborne, and spaceborne measurements should be conducted continuously for the upcoming new era of geostationary environmental satellites.
For the first time, we have retrieved NO2 VCD data using airborne GeoTASO observations over the Seoul metropolitan region, one of the most populous cities worldwide, the Busan metropolitan region, the second-largest city in South Korea, and Anmyeon, an area with thermal power plants and industrial complexes. By retrieving NO2 data using GeoTASO L1B radiance, it was possible to observe the spatial distribution of NO2 in these metropolitan and industrial regions. In the morning, tropospheric NO2 VCD in Seoul showed a strong horizontal gradient between rural and urban areas. In urban areas, tropospheric NO2 VCD was high, with values exceeding 3×1016 molec. cm−2; in rural areas, values were typically below 1×1016 molec. cm−2. Extremely high values over 10×1016 molec. cm−2 were also observed in both rural and urban areas. In Anmyeon, GeoTASO observations showed that NO2 is mainly emitted from the chimneys of industrial complexes and thermal power plants and subsequently transported by wind approximately 26.5 km to the Yellow Sea on the western coast of the Korean Peninsula. In the Busan metropolitan region, in the morning tropospheric NO2 VCDs showed a pattern similar to the Seoul metropolitan region, with high values above the inter-city road. However, unlike Seoul, tropospheric NO2 VCDs in Busan decreased in the afternoon due to different local weather conditions.
To compare the data retrieved from the GeoTASO system, we compared NO2,G with NO2,O obtained from the OMI, NO2,A obtained from Air-Korea, and NO2,P obtained from the Pandora observation system. When the distance between two observations was below 20 km or 0.05∘ within 30 min, the correlation coefficients were relatively high (R=0.48, and 0.91, respectively). However, the correlation between NO2,G and NO2,A over the Seoul metropolitan region was extremely weak (R=0.07) in the morning because of the more pronounced NO2 horizontal gradient.
The GeoTASO system successfully observed NO2 VCDs with high horizontal spatial resolution for both metropolitan and industrial regions. This demonstrates that airborne remote sensing measurements from GeoTASO, similar to GCAS, APEX, and others, can be an effective tool for the validation of trace gases retrieved from environmental satellites, including the OMI, TROPOMI, and GOME-2; these systems can obtain high-resolution measurements over relatively wide areas. However, to validate geostationary environmental satellites with higher spatiotemporal resolutions, such as the GEMS, TEMPO, and Sentinel-4, additional validation strategies are needed. Based on error estimation, it can be concluded that aerosol properties are relevant and should be determined and that NO2 vertical profile retrievals should be performed using, for example, Lidar, MAX-DOAS, and sondes. This is important because the accuracy of aerosol properties, surface reflectance values, and the NO2 vertical profiles affects the accuracy of AMF calculations (Leitão et al., 2010; Hong et al., 2017; Lorente et al., 2017; Boersma et al., 2018). Furthermore, as we observed in the Seoul metropolitan area, observations taken closer together using ground-based remote sensing systems and in situ measurements are needed as NO2 displays large horizontal gradients, especially in the morning.
The whole code was developed in Interactive Data Language (IDL) environment. Code from this study (IDL scripts) is available from the corresponding author upon request to choo4616@korea.kr.
KORUS-AQ campaign data are distributed from the NASA LaRC data archive, and they are available at https://www-air.larc.nasa.gov/cgi-bin/ArcView/korusaq (last access: 10 February 2022; NASA, 2022).
GHC and HH designed and implemented the research. KL provided the CTM data. GHC developed the code for model runs and performed the RTM simulations. HH and UJ contributed to the analysis of ground-based data. GHC and WC conducted the sensitivity test. GHC, KL, HH, UJ, WC, and JJS revised and edited the paper. HH, UJ, and WC provided constructive comments. All authors contributed to this work.
The contact author has declared that none of the authors has any competing interests.
Publisher's note: Copernicus Publications remains neutral with regard to jurisdictional claims in published maps and institutional affiliations.
Pandora data were obtained from the KORUS-AQ home page of NASA's Goddard Space Flight Center (https://avdc.gsfc.nasa.gov/pub/DSCOVR/Pandora/DATA/KORUS-AQ/, last access: 22 July 2022). Ground-based NO2 MR data were obtained from Air-Korea (http://www.airkorea.or.kr/web/detailViewDown?pMENU_NO=125/, last access: 16 February 2022). The authors would like to thank the KORUS-AQ campaign team for providing the GeoTASO and Pandora data.
This research has been supported by the National Institute of Environmental Research (grant no. NIER-2021-01-01-100).
This paper was edited by Andreas Richter and reviewed by two anonymous referees.
Ahn, C., Torres, O., and Jethva, H., Assessment of OMI near‐UV aerosol optical depth over land, J. Geophys. Res.-Atmos., 119, 2457–2473, 2014.
Boersma, K. F., Eskes, H. J., and Brinksma, E. J.: Error analysis for tropospheric NO2 retrieval from space: ERROR ANALYSIS FOR TROPOSPHERIC NO2, J. Geophys. Res., 109, D04311, https://doi.org/10.1029/2003JD003962, 2004.
Boersma, K. F., Eskes, H. J., Richter, A., De Smedt, I., Lorente, A., Beirle, S., van Geffen, J. H. G. M., Zara, M., Peters, E., Van Roozendael, M., Wagner, T., Maasakkers, J. D., van der A, R. J., Nightingale, J., De Rudder, A., Irie, H., Pinardi, G., Lambert, J.-C., and Compernolle, S. C.: Improving algorithms and uncertainty estimates for satellite NO2 retrievals: results from the quality assurance for the essential climate variables (QA4ECV) project, Atmos. Meas. Tech., 11, 6651–6678, https://doi.org/10.5194/amt-11-6651-2018, 2018.
Bogumil, K., Orphal, J., and Burrows, J. P.: Temperature dependent absorption cross sections of O3, NO2, and other atmospheric trace gases measured with the SCIAMACHY spectrometer, Proceedings of the ERS-Envisat-Symposium, Goteborg, Sweden, 2000.
Brauer, M., Hoek, G., Van Vliet, P., Meliefste, K., Fischer, P. H., Wijga, A., Koopman, L. P., Neijens, H. J., Gerritsen, J., Kerkhof, M., Heinrich, J., Bellander, T., and Brunekreef, B.: Air Pollution from Traffic and the Development of Respiratory Infections and Asthmatic and Allergic Symptoms in Children, Am. J. Respir. Crit. Care Med., 166, 1092–1098, https://doi.org/10.1164/rccm.200108-007OC, 2002.
Burrows, J. P., Hölzle, E., Goede, A. P. H., Visser, H., and Fricke, W.: SCIAMACHY – scanning imaging absorption spectrometer for atmospheric chartography, Acta Astronaut., 35, 445–451, https://doi.org/10.1016/0094-5765(94)00278-T, 1995.
Burrows, J. P., Weber, M., Buchwitz, M., Rozanov, V., Ladstätter-Weißenmayer, A., Richter, A., DeBeek, R., Hoogen, R., Bramstedt, K., Eichmann, K.-U., Eisinger, M., and Perner, D.: The Global Ozone Monitoring Experiment (GOME): Mission Concept and First Scientific Results, J. Atmos. Sci., 56, 151–175, https://doi.org/10.1175/1520-0469(1999)056<0151:TGOMEG>2.0.CO;2, 1999.
Byun, D. W. and Ching, J. K. S.: Science algorithms of the EPA Models-3 Community Multiscale Air Quality (CMAQ) Modeling System, U.S. EPA/600/R-99/030, 1999.
Byun, D. and Schere, K. L.: Review of the Governing Equations, Computational Algorithms, and Other Components of the Models-3 Community Multiscale Air Quality (CMAQ) Modeling System, Appl. Mech. Rev., 59, 51, https://doi.org/10.1115/1.2128636, 2006.
Callies, J., Corpaccioli, E., Eisinger, M., Hahne, A., and Lefebvre, A.: GOME-2-Metop's second-generation sensor for operational ozone monitoring, ESA Bull, 102, 28–36, 2000.
Castellanos, P., Boersma, K. F., Torres, O., and de Haan, J. F.: OMI tropospheric NO2 air mass factors over South America: effects of biomass burning aerosols, Atmos. Meas. Tech., 8, 3831–3849, https://doi.org/10.5194/amt-8-3831-2015, 2015.
Chance, K. and Kurucz, R. L.: An improved high-resolution solar reference spectrum for earth's atmosphere measurements in the ultraviolet, visible, and near infrared, J. Quant. Spectrosc. Ra. Trans., 111, 1289–1295, https://doi.org/10.1016/j.jqsrt.2010.01.036, 2010.
Chance, K. V. and Spurr, R. J. D.: Ring effect studies: Rayleigh scattering, including molecular parameters for rotational Raman scattering, and the Fraunhofer spectrum, Appl. Opt., 36, 5224, https://doi.org/10.1364/AO.36.005224, 1997.
Choi, S., Lamsal, L. N., Follette-Cook, M., Joiner, J., Krotkov, N. A., Swartz, W. H., Pickering, K. E., Loughner, C. P., Appel, W., Pfister, G., Saide, P. E., Cohen, R. C., Weinheimer, A. J., and Herman, J. R.: Assessment of NO2 observations during DISCOVER-AQ and KORUS-AQ field campaigns, Atmos. Meas. Tech., 13, 2523–2546, https://doi.org/10.5194/amt-13-2523-2020, 2020.
Choi, W. J., Moon, K.-J., Yoon, J., Cho, A., Kim, S. K., Lee, S., Ko, D. H., Kim, J., Ahn, M. H., Kim, D.-R., Kim, S.-M., Kim, J.-Y., Nicks, D., and Kim, J.-S.: Introducing the geostationary environment monitoring spectrometer, J. Appl. Remote Sens., 12, 1, https://doi.org/10.1117/1.JRS.12.044005, 2018.
Choi, M., Lim, H., Kim, J., Lee, S., Eck, T. F., Holben, B. N., Garay, M. J., Hyer, E. J., Saide, P. E., and Liu, H.: Validation, comparison, and integration of GOCI, AHI, MODIS, MISR, and VIIRS aerosol optical depth over East Asia during the 2016 KORUS-AQ campaign, Atmos. Meas. Tech., 12, 4619–4641, https://doi.org/10.5194/amt-12-4619-2019, 2019.
Chong, H., Lee, S., Kim, J., Jeong, U., Li, C., Krotkov, N. A., Nowlan, C. R., Al-Saadi, J. A., Janz, S. J., Kowalewski, M. G., Ahn, M.-H., Kang, M., Joiner, J., Haffner, D. P., Hu, L., Castellanos, P., Huey, L. G., Choi, M., Song, C. H., Han, K. M., and Koo, J.-H.: High-resolution mapping of SO2 using airborne observations from the GeoTASO instrument during the KORUS-AQ field study: PCA-based vertical column retrievals, Remote Sens. Environ., 241, 111725, https://doi.org/10.1016/j.rse.2020.111725, 2020.
Choo, G.-H., Seo, J., Yoon, J., Kim, D.-R., and Lee, D.-W.: Analysis of long-term (2005–2018) trends in tropospheric NO2 percentiles over Northeast Asia, Atmos. Pollut. Res., 11, 1429–1440, https://doi.org/10.1016/j.apr.2020.05.012, 2020.
Danckaert, T., Fayt, C., Van Roozendael, M., De Smedt, I., Letocart, V., Merlaud, A., and Pinardi, G.: QDOAS Software user manual, Belgian Institute for Space Aeronomy, 1–117, 2016.
de Foy, B., Lu, Z., and Streets, D. G.: Satellite NO2 retrievals suggest China has exceeded its NOx reduction goals from the twelfth Five-Year Plan, Sci. Rep., 6, 35912, https://doi.org/10.1038/srep35912, 2016.
Fishman, J., Iraci, L., Al-Saadi, J., Chance, K., Chavez, F., Chin, M., Coble, P., Davis, C., DiGiacomo, P., Edwards, D., Eldering, L., Goes, J., Herman, J., Hu, C., Jacob, D. J., Jordan, C., Kawa, S. R., Key, R., Liu, X., Lohrenz, S., Mannino, A., Natraj, V., Neil, D., Neu, J., Newchruch, M., Pickering, K., Salisbury, J., Sosik, H., Subramaniam, A., Tzortziou, M., Wang, J., and Wang, M.: The United States’ next generation of atmospheric composition and coastal ecosystem measurements: NASA’s Geostationary Coastal and Air Pollution Events (GEO-CAPE) Mission, B. Am. Meteorol. Soc., 93, 1547–1566, 2012.
General, S., Pöhler, D., Sihler, H., Bobrowski, N., Frieß+, U., Zielcke, J., Horbanski, M., Shepson, P. B., Stirm, B. H., Simpson, W. R., Weber, K., Fischer, C., and Platt, U.: The Heidelberg Airborne Imaging DOAS Instrument (HAIDI) – a novel imaging DOAS device for 2-D and 3-D imaging of trace gases and aerosols, Atmos. Meas. Tech., 7, 3459–3485, https://doi.org/10.5194/amt-7-3459-2014, 2014.
Guenther, A., Karl, T., Harley, P., Wiedinmyer, C., Palmer, P. I., and Geron, C.: Estimates of global terrestrial isoprene emissions using MEGAN (Model of Emissions of Gases and Aerosols from Nature), Atmos. Chem. Phys., 6, 3181–3210, https://doi.org/10.5194/acp-6-3181-2006, 2006.
Guenther, A. B., Jiang, X., Heald, C. L., Sakulyanontvittaya, T., Duhl, T., Emmons, L. K., and Wang, X.: The Model of Emissions of Gases and Aerosols from Nature version 2.1 (MEGAN2.1): an extended and updated framework for modeling biogenic emissions, Geosci. Model Dev., 5, 1471–1492, https://doi.org/10.5194/gmd-5-1471-2012, 2012.
Herman, J., Cede, A., Spinei, E., Mount, G., Tzortziou, M., and Abuhassan, N.: NO2 column amounts from ground-based Pandora and MFDOAS spectrometers using the direct-sun DOAS technique: Intercomparisons and application to OMI validation, J. Geophys. Res., 114, D13307, https://doi.org/10.1029/2009JD011848, 2009.
Herman, J., Spinei, E., Fried, A., Kim, J., Kim, J., Kim, W., Cede, A., Abuhassan, N., and Segal-Rozenhaimer, M.: NO2 and HCHO measurements in Korea from 2012 to 2016 from Pandora spectrometer instruments compared with OMI retrievals and with aircraft measurements during the KORUS-AQ campaign, Atmos. Meas. Tech., 11, 4583–4603, https://doi.org/10.5194/amt-11-4583-2018, 2018.
Hong, H., Lee, H., Kim, J., Jeong, U., Ryu, J., and Lee, D.: Investigation of Simultaneous Effects of Aerosol Properties and Aerosol Peak Height on the Air Mass Factors for Space-Borne NO2 Retrievals, Remote Sens., 9, 208, https://doi.org/10.3390/rs9030208, 2017.
Jeong, U., and Hong, H.: Assessment of tropospheric concentrations of NO2 from the TROPOMI/Sentinel-5 Precursor for the estimation of long-term exposure to surface NO2 over South Korea, Remote Sens., 13, 1877, https://doi.org/10.3390/rs13101877, 2021a.
Jeong, U. and Hong, H.: Comparison of total column and surface mixing ratio of carbon monoxide derived from the TROPOMI/Sentinel-5 Precursor with In-Situ measurements from extensive ground-based network over South Korea, Remote Sens., 13, 3987, https://doi.org/10.3390/rs13193987, 2021b.
Jeong, U., Kim, J., Lee, H., and Lee, Y. G.: Assessing the effect of long-range pollutant transportation on air quality in Seoul using the conditional potential source contribution function method, Atmos. Environ., 150, 33–44, https://doi.org/10.1016/j.atmosenv.2016.11.017, 2017.
Jethva, H., Torres, O., and Ahn, C., Global assessment of OMI aerosol single‐scattering albedo using ground‐based AERONET inversion, J. Geophys. Res.-Atmos., 119, 9020–9040, 2014.
Judd, L. M., Al-Saadi, J. A., Valin, L. C., Pierce, R. B., Yang, K., Janz, S. J., Kowalewski, M. G., Szykman, J. J., Tiefengraber, M., and Mueller, M.: The Dawn of Geostationary Air Quality Monitoring: Case Studies From Seoul and Los Angeles, Front. Environ. Sci., 6, 85, https://doi.org/10.3389/fenvs.2018.00085, 2018.
Judd, L. M., Al-Saadi, J. A., Janz, S. J., Kowalewski, M. G., Pierce, R. B., Szykman, J. J., Valin, L. C., Swap, R., Cede, A., Mueller, M., Tiefengraber, M., Abuhassan, N., and Williams, D.: Evaluating the impact of spatial resolution on tropospheric NO2 column comparisons within urban areas using high-resolution airborne data, Atmos. Meas. Tech., 12, 6091–6111, https://doi.org/10.5194/amt-12-6091-2019, 2019.
Judd, L. M., Al-Saadi, J. A., Szykman, J. J., Valin, L. C., Janz, S. J., Kowalewski, M. G., Eskes, H. J., Veefkind, J. P., Cede, A., Mueller, M., Gebetsberger, M., Swap, R., Pierce, R. B., Nowlan, C. R., Abad, G. G., Nehrir, A., and Williams, D.: Evaluating Sentinel-5P TROPOMI tropospheric NO2 column densities with airborne and Pandora spectrometers near New York City and Long Island Sound, Atmos. Meas. Tech., 13, 6113–6140, https://doi.org/10.5194/amt-13-6113-2020, 2020.
Kendrick, C. M., Koonce, P., and George, L. A.: Diurnal and seasonal variations of NO, NO2 and PM2.5 mass as a function of traffic volumes alongside an urban arterial, Atmos. Environ., 122, 133–141, https://doi.org/10.1016/j.atmosenv.2015.09.019, 2015.
Kim, D., Lee, H., Hong, H., Choi, W., Lee, Y., and Park, J.: Estimation of Surface NO2 Volume Mixing Ratio in Four Metropolitan Cities in Korea Using Multiple Regression Models with OMI and AIRS Data, Remote Sens., 9, 627, https://doi.org/10.3390/rs9060627, 2017.
Kim, J., Jeong, U., Ahn, M.-H., Kim, J. H., Park, R. J., Lee, H., Song, C. H., Choi, Y.-S., Lee, K.-H., Yoo, J.-M., Jeong, M.-J., Park, S. K., Lee, K.-M., Song, C.-K., Kim, S.-W., Kim, Y. J., Kim, S.-W., Kim, M., Go, S., Liu, X., Chance, K., Chan Miller, C., Al-Saadi, J., Veihelmann, B., Bhartia, P. K., Torres, O., Abad, G. G., Haffner, D. P., Ko, D. H., Lee, S. H., Woo, J.-H., Chong, H., Park, S. S., Nicks, D., Choi, W. J., Moon, K.-J., Cho, A., Yoon, J., Kim, S., Hong, H., Lee, K., Lee, H., Lee, S., Choi, M., Veefkind, P., Levelt, P. F., Edwards, D. P., Kang, M., Eo, M., Bak, J., Baek, K., Kwon, H.-A., Yang, J., Park, J., Han, K. M., Kim, B.-R., Shin, H.-W., Choi, H., Lee, E., Chong, J., Cha, Y., Koo, J.-H., Irie, H., Hayashida, S., Kasai, Y., Kanaya, Y., Liu, C., Lin, J., Crawford, J. H., Carmichael, G. R., Newchurch, M. J., Lefer, B. L., Herman, J. R., Swap, R. J., Lau, A. K. H., Kurosu, T. P., Jaross, G., Ahlers, B., Dobber, M., McElroy, C. T., and Choi, Y.: New Era of Air Quality Monitoring from Space: Geostationary Environment Monitoring Spectrometer (GEMS), B. Am. Meteorol. Soc., 101, E1–E22, https://doi.org/10.1175/BAMS-D-18-0013.1, 2020.
Kley, D. and McFarland, M.: Chemiluminescence detector for NO and NO2, Atmos. Technol., 12, 63–69, 1980.
Kowalewski, M. G. and Janz, S. J.: Remote sensing capabilities of the GEO-CAPE airborne simulator, SPIE Optical Engineering + Applications, San Diego, California, United States, 92181I, https://doi.org/10.1117/12.2062058, 2014.
Kowalewski, M. G., Janz, S., Al-Saadi, J. A., Good, W., Ruppert, L., and Cole, J.: GeoTASO instrument characterization and level1b radiance product generation, in: Proceedings of the 1st KORUS-AQ Science Team Meeting, Jeju, South Korea, 27 February–3 March 2017, 13 pp., 2017.
Lamsal, L. N., Martin, R. V., Parrish, D. D., and Krotkov, N. A.: Scaling Relationship for NO2 Pollution and Urban Population Size: A Satellite Perspective, Environ. Sci. Technol., 47, 7855–7861, https://doi.org/10.1021/es400744g, 2013.
Lamsal, L. N., Janz, S. J., Krotkov, N. A., Pickering, K. E., Spurr, R. J. D., Kowalewski, M. G., Loughner, C. P., Crawford, J. H., Swartz, W. H., and Herman, J. R.: High-resolution NO2 observations from the Airborne Compact Atmospheric Mapper: Retrieval and validation, J. Geophys. Res.-Atmos., 122, 1953–1970, https://doi.org/10.1002/2016JD025483, 2017.
Latza, U., Gerdes, S., and Baur, X.: Effects of nitrogen dioxide on human health: Systematic review of experimental and epidemiological studies conducted between 2002 and 2006, Int. J. Hygiene Environ. Health, 212, 271–287, https://doi.org/10.1016/j.ijheh.2008.06.003, 2009.
Lee, K., Yu, J., Lee, S., Park, M., Hong, H., Park, S. Y., Choi, M., Kim, J., Kim, Y., Woo, J.-H., Kim, S.-W., and Song, C. H.: Development of Korean Air Quality Prediction System version 1 (KAQPS v1) with focuses on practical issues, Geosci. Model Dev., 13, 1055–1073, https://doi.org/10.5194/gmd-13-1055-2020, 2020.
Leitão, J., Richter, A., Vrekoussis, M., Kokhanovsky, A., Zhang, Q. J., Beekmann, M., and Burrows, J. P.: On the improvement of NO2 satellite retrievals – aerosol impact on the airmass factors, Atmos. Meas. Tech., 3, 475–493, https://doi.org/10.5194/amt-3-475-2010, 2010.
Leitch, J. W., Delker, T., Good, W., Ruppert, L., Murcray, F., Chance, K., Liu, X., Nowlan, C., Janz, S. J., Krotkov, N. A., Pickering, K. E., Kowalewski, M., and Wang, J.: The GeoTASO airborne spectrometer project, SPIE Optical Engineering + Applications, San Diego, California, United States, 92181H, https://doi.org/10.1117/12.2063763, 2014.
Levelt, P. F., van den Oord, G. H. J., Dobber, M. R., Malkki, A., Huib Visser, Johan de Vries, Stammes, P., Lundell, J. O. V., and Saari, H.: The ozone monitoring instrument, IEEE Trans. Geosci. Remote Sensing, 44, 1093–1101, https://doi.org/10.1109/TGRS.2006.872333, 2006.
Lorente, A., Folkert Boersma, K., Yu, H., Dörner, S., Hilboll, A., Richter, A., Liu, M., Lamsal, L. N., Barkley, M., De Smedt, I., Van Roozendael, M., Wang, Y., Wagner, T., Beirle, S., Lin, J.-T., Krotkov, N., Stammes, P., Wang, P., Eskes, H. J., and Krol, M.: Structural uncertainty in air mass factor calculation for NO2 and HCHO satellite retrievals, Atmos. Meas. Tech., 10, 759–782, https://doi.org/10.5194/amt-10-759-2017, 2017.
Ma, J. Z., Beirle, S., Jin, J. L., Shaiganfar, R., Yan, P., and Wagner, T.: Tropospheric NO2 vertical column densities over Beijing: results of the first three years of ground-based MAX-DOAS measurements (2008–2011) and satellite validation, Atmos. Chem. Phys., 13, 1547–1567, https://doi.org/10.5194/acp-13-1547-2013, 2013.
Malm, W. C. and Hand J. L.: An examination of the physical and optical properties of aerosols collected in the IMPROVE program, Atmos. Environ., 41, 3407–3427, https://doi.org/10.1016/j.atmosenv.2006.12.012, 2007.
Merlaud, A., Constantin, D., Mingireanu, F., Mocanu, I., Maes, J., Fayt, C., Voiculescu, M., Murariu, G., Georgescu, L., and Van Roozendael, M.: Small whiskbroom imager for atmospheric composition monitoring (SWING) from an unmanned aerial vehicle (UAV), in: Proceedings of the 21st ESA Symposium on European Rocket & Balloon Programmes and related Research, Thun, Switzerland, 9–13, June, 2013.
Meier, A. C., Schönhardt, A., Bösch, T., Richter, A., Seyler, A., Ruhtz, T., Constantin, D.-E., Shaiganfar, R., Wagner, T., Merlaud, A., Van Roozendael, M., Belegante, L., Nicolae, D., Georgescu, L., and Burrows, J. P.: High-resolution airborne imaging DOAS measurements of NO2 above Bucharest during AROMAT, Atmos. Meas. Tech., 10, 1831–1857, https://doi.org/10.5194/amt-10-1831-2017, 2017.
Merlaud, A., Tack, F., Constantin, D., Georgescu, L., Maes, J., Fayt, C., Mingireanu, F., Schuettemeyer, D., Meier, A. C., Schönardt, A., Ruhtz, T., Bellegante, L., Nicolae, D., Den Hoed, M., Allaart, M., and Van Roozendael, M.: The Small Whiskbroom Imager for atmospheric compositioN monitorinG (SWING) and its operations from an unmanned aerial vehicle (UAV) during the AROMAT campaign, Atmos. Meas. Tech., 11, 551–567, https://doi.org/10.5194/amt-11-551-2018, 2018.
NASA: Airborne Science Data for Atmosperic Composition, KORUSAQ_2016, NASA LaRC data archive [data set], https://www-air.larc.nasa.gov/cgi-bin/ArcView/korusaq, last access: 10 February 2022.
National Institute of Environmental Research (NIER) and National Aeronautics and Space Administration (NASA): KORUS-AQ Final Science Synthesis Report, https://espo.nasa.gov/sites/default/files/documents/5858211.pdf (last access: 27 June 2022), 2020.
Nowlan, C. R., Liu, X., Leitch, J. W., Chance, K., González Abad, G., Liu, C., Zoogman, P., Cole, J., Delker, T., Good, W., Murcray, F., Ruppert, L., Soo, D., Follette-Cook, M. B., Janz, S. J., Kowalewski, M. G., Loughner, C. P., Pickering, K. E., Herman, J. R., Beaver, M. R., Long, R. W., Szykman, J. J., Judd, L. M., Kelley, P., Luke, W. T., Ren, X., and Al-Saadi, J. A.: Nitrogen dioxide observations from the Geostationary Trace gas and Aerosol Sensor Optimization (GeoTASO) airborne instrument: Retrieval algorithm and measurements during DISCOVER-AQ Texas 2013, Atmos. Meas. Tech., 9, 2647–2668, https://doi.org/10.5194/amt-9-2647-2016, 2016.
Nowlan, C. R., Liu, X., Janz, S. J., Kowalewski, M. G., Chance, K., Follette-Cook, M. B., Fried, A., González Abad, G., Herman, J. R., Judd, L. M., Kwon, H.-A., Loughner, C. P., Pickering, K. E., Richter, D., Spinei, E., Walega, J., Weibring, P., and Weinheimer, A. J.: Nitrogen dioxide and formaldehyde measurements from the GEOstationary Coastal and Air Pollution Events (GEO-CAPE) Airborne Simulator over Houston, Texas, Atmos. Meas. Tech., 11, 5941–5964, https://doi.org/10.5194/amt-11-5941-2018, 2018.
Palmer, P. I., Jacob, D. J., Chance, K., Martin, R. V., Spurr, R. J. D., Kurosu, T. P., Bey, I., Yantosca, R., Fiore, A., and Li, Q.: Air mass factor formulation for spectroscopic measurements from satellites: Application to formaldehyde retrievals from the Global Ozone Monitoring Experiment, J. Geophys. Res., 106, 14539–14550, https://doi.org/10.1029/2000JD900772, 2001.
Pastel, M., Pommereau, J.-P., Goutail, F., Richter, A., Pazmiño, A., Ionov, D., and Portafaix, T.: Construction of merged satellite total O3 and NO2 time series in the tropics for trend studies and evaluation by comparison to NDACC SAOZ measurements, Atmos. Meas. Tech., 7, 3337–3354, https://doi.org/10.5194/amt-7-3337-2014, 2014.
Platt, U.: Differential absorption spectroscopy (DOAS), Chem. Anal. Series, 127, 27–83, 1994.
Platt, U. and Stutz, J.: Differential absorption spectroscopy, in: Differential Optical Absorption Spectroscopy, Springer, Berlin, Heidelberg, 135–174, ISBN 978-3-540-21193-8. 2008.
Popp, C., Brunner, D., Damm, A., Van Roozendael, M., Fayt, C., and Buchmann, B.: High-resolution NO2 remote sensing from the Airborne Prism EXperiment (APEX) imaging spectrometer, Atmos. Meas. Tech., 5, 2211–2225, https://doi.org/10.5194/amt-5-2211-2012, 2012.
Prasad, A. K., Singh, R. P., and Kafatos, M.: Influence of coal-based thermal power plants on the spatial–temporal variability of tropospheric NO2 column over India, Environ. Monit. Assess., 184, 1891–1907, https://doi.org/10.1007/s10661-011-2087-6, 2012.
Qin, K., Rao, L., Xu, J., Bai, Y., Zou, J., Hao, N., Li, S., and Yu, C.: Estimating Ground Level NO2 Concentrations over Central-Eastern China Using a Satellite-Based Geographically and Temporally Weighted Regression Model, Remote Sens., 9, 950, https://doi.org/10.3390/rs9090950, 2017.
Richter, A., Burrows, J. P., Nüß, H., Granier, C., and Niemeier, U.: Increase in tropospheric nitrogen dioxide over China observed from space, Nature, 437, 129–132, https://doi.org/10.1038/nature04092, 2005.
Rothman, L. S., Gordon, I. E., Barber, R. J., Dothe, H., Gamache, R. R., Goldman, A., Perevalov, V. I., Tashkun, S. A., and Tennyson, J.: HITEMP, the high-temperature molecular spectroscopic database, J. Quant. Spectrosc. Ra. Transf., 111, 2139–2150, 2010.
Schönhardt, A., Altube, P., Gerilowski, K., Krautwurst, S., Hartmann, J., Meier, A. C., Richter, A., and Burrows, J. P.: A wide field-of-view imaging DOAS instrument for two-dimensional trace gas mapping from aircraft, Atmos. Meas. Tech., 8, 5113–5131, https://doi.org/10.5194/amt-8-5113-2015, 2015.
Shah, V., Jacob, D. J., Li, K., Silvern, R. F., Zhai, S., Liu, M., Lin, J., and Zhang, Q.: Effect of changing NOx lifetime on the seasonality and long-term trends of satellite-observed tropospheric NO2 columns over China, Atmos. Chem. Phys., 20, 1483–1495, https://doi.org/10.5194/acp-20-1483-2020, 2020.
Skamarock, W., Klemp, J., Dudhia, J., Gill, D., Barker, D., Wang, W., Huang, X.-Y., and Duda, M.: A Description of the Advanced Research WRF Version 3, NCAR technical note: a description of the advanced research WRF version 3. Boulder, Colorado: National Center for Atmospheric Research, https://doi.org/10.5065/D68S4MVH, 2008.
Spinei, E., Whitehill, A., Fried, A., Tiefengraber, M., Knepp, T. N., Herndon, S., Herman, J. R., Müller, M., Abuhassan, N., Cede, A., Richter, D., Walega, J., Crawford, J., Szykman, J., Valin, L., Williams, D. J., Long, R., Swap, R. J., Lee, Y., Nowak, N., and Poche, B.: The first evaluation of formaldehyde column observations by improved Pandora spectrometers during the KORUS-AQ field study, Atmos. Meas. Tech., 11, 4943–4961, https://doi.org/10.5194/amt-11-4943-2018, 2018.
Spurr, R. and Christi, M.: On the generation of atmospheric property Jacobians from the (V)LIDORT linearized radiative transfer models, J. Quant. Spectrosc. Ra. Transf., 142, 109–115, https://doi.org/10.1016/j.jqsrt.2014.03.011, 2014.
Tack, F., Merlaud, A., Iordache, M.-D., Danckaert, T., Yu, H., Fayt, C., Meuleman, K., Deutsch, F., Fierens, F., and Van Roozendael, M.: High-resolution mapping of the NO2 spatial distribution over Belgian urban areas based on airborne APEX remote sensing, Atmos. Meas. Tech., 10, 1665–1688, https://doi.org/10.5194/amt-10-1665-2017, 2017.
Tack, F., Merlaud, A., Meier, A. C., Vlemmix, T., Ruhtz, T., Iordache, M.-D., Ge, X., van der Wal, L., Schuettemeyer, D., Ardelean, M., Calcan, A., Constantin, D., Schönhardt, A., Meuleman, K., Richter, A., and Van Roozendael, M.: Intercomparison of four airborne imaging DOAS systems for tropospheric NO2 mapping – the AROMAPEX campaign, Atmos. Meas. Tech., 12, 211–236, https://doi.org/10.5194/amt-12-211-2019, 2019.
Tack, F., Merlaud, A., Iordache, M.-D., Pinardi, G., Dimitropoulou, E., Eskes, H., Bomans, B., Veefkind, P., and Van Roozendael, M.: Assessment of the TROPOMI tropospheric NO2 product based on airborne APEX observations, Atmos. Meas. Tech., 14, 615–646, https://doi.org/10.5194/amt-14-615-2021, 2021.
Tzortziou, M., Parker, O., Lamb, B., Herman, J., Lamsal, L., Stauffer, R., and Abuhassan, N.: Atmospheric Trace Gas (NO2 and O3) Variability in South Korean Coastal Waters, and Implications for Remote Sensing of Coastal Ocean Color Dynamics, Remote Sens., 10, 1587, https://doi.org/10.3390/rs10101587, 2018.
Valks, P., Pinardi, G., Richter, A., Lambert, J.-C., Hao, N., Loyola, D., Van Roozendael, M., and Emmadi, S.: Operational total and tropospheric NO2 column retrieval for GOME-2, Atmos. Meas. Tech., 4, 1491–1514, https://doi.org/10.5194/amt-4-1491-2011, 2011.
Vandaele, A. C., Hermans, C., Simon, P. C., Carleer, M., Colin, R., Fally, S., Mérienne, M. F., Jenouvrier, A., and Coquart, B.: Measurements of the NO2 absorption cross-section from 42 000 cm−1 to 10 000 cm−1 (238–1000 nm) at 220 K and 294 K, J. Quant. Spectrosc. Ra. Transf., 59, 171–184, https://doi.org/10.1016/S0022-4073(97)00168-4, 1998.
Veefkind, J. P., Aben, I., McMullan, K., Förster, H., de Vries, J., Otter, G., Claas, J., Eskes, H. J., de Haan, J. F., Kleipool, Q., van Weele, M., Hasekamp, O., Hoogeveen, R., Landgraf, J., Snel, R., Tol, P., Ingmann, P., Voors, R., Kruizinga, B., Vink, R., Visser, H., and Levelt, P. F.: TROPOMI on the ESA Sentinel-5 Precursor: A GMES mission for global observations of the atmospheric composition for climate, air quality and ozone layer applications, Remote Sens. Environ., 120, 70–83, https://doi.org/10.1016/j.rse.2011.09.027, 2012.
Vlemmix, T., Ge, X. (., de Goeij, B. T. G., van der Wal, L. F., Otter, G. C. J., Stammes, P., Wang, P., Merlaud, A., Schüttemeyer, D., Meier, A. C., Veefkind, J. P., and Levelt, P. F.: Retrieval of tropospheric NO2 columns over Berlin from high-resolution airborne observations with the spectrolite breadboard instrument, Atmos. Meas. Tech. Discuss. [preprint], https://doi.org/10.5194/amt-2017-257, in review, 2017.
Wiedinmyer, C., Quayle, B., Geron, C., Belote, A., McKenzie, D., Zhang, X., O'Neill, S., and Wynne, K. K.: Estimating emissions from fires in North America for air quality modeling, Atmos. Environ., 40, 3419–3432, https://doi.org/10.1016/j.atmosenv.2006.02.010, 2006.
Wiedinmyer, C., Akagi, S. K., Yokelson, R. J., Emmons, L. K., Al-Saadi, J. A., Orlando, J. J., and Soja, A. J.: The Fire INventory from NCAR (FINN): a high resolution global model to estimate the emissions from open burning, Geosci. Model Dev., 4, 625–641, https://doi.org/10.5194/gmd-4-625-2011, 2011.
Wold, S., Esbensen, K., and Geladi, P.: Principal component analysis, Chemometr. Intell. Lab., 2, 37–52, https://doi.org/10.1016/0169-7439(87)80084-9, 1987.
Woo, J.-H., Choi, K.-C., Kim, H. K., Baek, B. H., Jang, M., Eum, J.-H., Song, C. H., Ma, Y.-I., Sunwoo, Y., Chang, L.-S., and Yoo, S. H.: Development of an anthropogenic emission processing system for Asia using SMOKE, Atmos. Environ., 58, 5–13, https://doi.org/10.1016/j.atmosenv.2011.10.042, 2012.
Zoogman, P., Liu, X., Suleiman, R. M., Pennington, W. F., Flittner, D. E., Al-Saadi, J. A., Hilton, B. B., Nicks, D. K., Newchurch, M. J., Carr, J. L., Janz, S. J., Andraschko, M. R., Arola, A., Baker, B. D., Canova, B. P., Chan Miller, C., Cohen, R. C., Davis, J. E., Dussault, M. E., Edwards, D. P., Fishman, J., Ghulam, A., González Abad, G., Grutter, M., Herman, J. R., Houck, J., Jacob, D. J., Joiner, J., Kerridge, B. J., Kim, J., Krotkov, N. A., Lamsal, L., Li, C., Lindfors, A., Martin, R. V., McElroy, C. T., McLinden, C., Natraj, V., Neil, D. O., Nowlan, C. R., O'Sullivan, E. J., Palmer, P. I., Pierce, R. B., Pippin, M. R., Saiz-Lopez, A., Spurr, R. J. D., Szykman, J. J., Torres, O., Veefkind, J. P., Veihelmann, B., Wang, H., Wang, J., and Chance, K.: Tropospheric emissions: Monitoring of pollution (TEMPO), J. Quant. Spectrosc. Ra. Transf., 186, 17–39, https://doi.org/10.1016/j.jqsrt.2016.05.008, 2017.