the Creative Commons Attribution 4.0 License.
the Creative Commons Attribution 4.0 License.
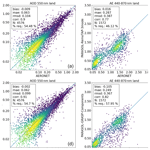
Algorithm evaluation for polarimetric remote sensing of atmospheric aerosols
Pavel Litvinov
Guangliang Fu
Cheng Chen
Oleg Dubovik
From a passive satellite remote sensing point of view, the richest set of information on aerosol properties can be obtained from instruments that measure both intensity and polarization of backscattered sunlight at multiple wavelengths and multiple viewing angles for one ground pixel. However, it is challenging to exploit this information at a global scale because complex algorithms are needed with many fit parameters (aerosol and land/ocean reflection), based on online radiative transfer models. So far, two such algorithms have demonstrated this capability at a global scale: the Generalized Retrieval of Atmosphere and Surface Properties (GRASP) algorithm and the Remote sensing of Trace gas and Aerosol Products (RemoTAP) algorithm. In this paper, we present a detailed comparison of the most recent versions of RemoTAP and GRASP. We evaluate both algorithms for synthetic observations, for real PARASOL (Polarization and Anisotropy of Reflectances for Atmospheric Science coupled with Observations from a Lidar) observations against AERONET (Aerosol Robotic Network) for common pixels, and for global PARASOL retrievals for the year 2008. For the aerosol optical depth (AOD) over land, both algorithms show a root mean square error (RMSE) of 0.10 (at 550 nm). For single scattering albedo (SSA), both algorithms show a good performance in terms of RMSE (0.04), but RemoTAP has a smaller bias (0.002) compared to GRASP (0.021). For the Ångström exponent (AE), GRASP has a smaller RMSE (0.367) than RemoTAP (0.387), mainly caused by a small overestimate of AE at low values (large particles). Over ocean both algorithms perform very well. For AOD, RemoTAP has an RMSE of 0.057 and GRASP an even smaller RMSE of 0.047. For AE, the RMSEs of RemoTAP and GRASP are 0.285 and 0.224, respectively. Based on the AERONET comparison, we conclude that both algorithms show very similar overall performance, where both algorithms have stronger and weaker points. For the global data products, we find a root mean square difference (RMSD) between RemoTAP and GRASP AOD of 0.12 and 0.038 over land and ocean, respectively. The largest differences occur over the biomass burning region in equatorial Africa. The global mean values are virtually unbiased with respect to each other. For AE the RMSD between RemoTAP and GRASP is 0.33 over land and 0.23 over ocean. For SSA, we find much better agreement over land (bias = −0.01, RMSD = 0.043 for retrievals with AOD > 0.2) than over ocean (bias = 0.053, RMSD = 0.074). As expected, the differences increase towards low AOD, over both land and ocean. We also compared the GRASP and RemoTAP AOD and AE products against MODIS. For AOD over land, the agreement of either GRASP or RemoTAP with MODIS is worse than the agreement between the two PARASOL algorithms themselves. Over ocean, the agreement is very similar among the three products for AOD. For AE, the agreement between GRASP and RemoTAP is much better than the agreement of both products with MODIS. The agreement of the latest product versions with each other and with AERONET improved significantly compared to the previous version of the global products of GRASP and RemoTAP. The results demonstrate that the dedicated effort in algorithm development for multi-angle polarimetric (MAP) aerosol retrievals still leads to substantial improvement of the resulting aerosol products, and this is still an ongoing process.
- Article
(18519 KB) - Full-text XML
- BibTeX
- EndNote
Terrestrial aerosol is a complex mixture of liquid, solid, or mixed-phase particles emitted by natural and anthropogenic sources. Aerosols affect Earth’s climate as they scatter and absorb solar radiation and act as condensation nuclei for cloud droplets and ice crystals. Substantial changes in anthropogenic aerosol emissions in the industrial age occurred. The overall increase in aerosol emissions has led to a cooling of Earth’s atmosphere, compensating for part of the temperature increase imposed by anthropogenic greenhouse gas emissions (Andreae et al., 2005). Prevailing uncertainty in the (effective) radiative forcing (ERF) from anthropogenic aerosol emissions continues to limit the accuracy of estimates of global climate sensitivity to changes in greenhouse gas concentrations, as clearly stated in the 6th Assessment Report by the Intergovernmental Panel on Climate Change (IPCC-AR6; Forster and et al., 2021). This uncertainty severely hampers future predictions of climate change. There have been significant changes in aerosol emissions in the past 2 decades. Over Europe and the USA aerosol emissions have declined since the 1990s. Over East Asia, there was an increase in aerosol emissions until 2010 and a decline afterwards. Over south Asia, aerosol emissions are continuing to increase (Forster and et al., 2021). Overall, reductions in global aerosol emissions are expected in the next decades, improving air quality but also likely leading to a further warming of the atmosphere. For aerosol–radiation interactions (ARIs) substantial uncertainties exist related to insufficient knowledge on aerosol absorption (J. Li et al., 2022). However, aerosol–cloud interactions (ACIs) represent the most uncertain contribution to the total aerosol ERF (Bellouin et al., 2020). By acting as cloud condensation nuclei (CCN), aerosols affect the cloud droplet number concentration (Nd) and consequently the cloud albedo (Twomey, 1974; Quaas et al., 2020), causing the radiative forcing due to ACIs (RFaci). Subsequently, rapid adjustments take place in, e.g., cloud fraction and liquid water path that result from an initial change in Nd (Gryspeerdt et al., 2020). A key step in quantifying RFaci (and subsequent rapid adjustments) is to quantify the sensitivity of Nd to the number concentration of cloud active aerosol, which depends mostly on the (dry) size distribution (Dusek et al., 2006; Hasekamp et al., 2019a).
To improve our understanding of the effect of aerosols on climate, weather, and air quality, measurements of aerosol chemical composition, size distribution, refractive index, optical properties like aerosol optical depth (AOD) and single scattering albedo (SSA), and the aerosol height profile are of crucial importance. It has been demonstrated by various studies that multi-angle polarimetric (MAP) measurements are needed to provide information about detailed aerosol properties like size distribution, refractive index, and SSA, in addition to the AOD. The only space-based MAP instrument that has provided a multi-year data set (2005–2013) in the past has been the French POLDER-3 instrument on the PARASOL (Polarization and Anisotropy of Reflectances for Atmospheric Science coupled with Observations from a Lidar) mission (hereafter simply referred to as PARASOL). Now space agencies realize the large potential of MAP instrumentation (Dubovik et al., 2019): in the 2020s several such instruments will be launched, e.g., SPEXone (Hasekamp et al., 2019a) and HARP-2 on PACE by NASA in 2024 (Werdell et al., 2019), 3MI on METOP-SG by ESA in 2025 (Fougnie et al., 2018), a MAP instrument on the CO2 Monitoring Mission by ESA in 2026, and a MAP instrument on the Atmosphere Observing System (AOS) mission by NASA in 2028. To cope with the increased information content on aerosols of MAP instrumentation and to assess the climatic effect of aerosols, new tools for retrieval need to be (further) developed. So far, this development has lagged behind the instrument development, which is the reason for the under-exploitation of the existing PARASOL data sets.
There are currently a number of aerosol retrieval algorithms available for the exploitation of MAP measurements. The first algorithms to generate the operational PARASOL products were using lookup tables (LUTs) based on a limited number of standard aerosol models (a combination of size distribution and refractive index) and omit measurements that have a considerable contribution from land/ocean reflection (Deuzé et al., 2001; Herman et al., 1997). Retrievals from PARASOL measurements using LUT approaches have so far hardly demonstrated added value compared to single-viewing-angle radiometer retrievals from, e.g., MODIS. In order to make full use of the information contained in MAP measurements, full inversion approaches are needed that consider a continuous space of aerosol microphysical properties (size distribution, refractive index) instead of using standard aerosol models and that properly account for land or ocean reflection by retrieving land or ocean parameters simultaneously with aerosol properties. Examples of such algorithms are the Generalized Retrieval of Aerosol and Surface Properties (GRASP) algorithm (Dubovik et al., 2011, 2014, 2021; Chen et al., 2020), the Remote sensing of Trace gas and Aerosol Products (RemoTAP) algorithm (Hasekamp et al., 2011; Fu and Hasekamp, 2018; Fu et al., 2020; Lu et al., 2022), the Jet Propulsion Laboratory (JPL) algorithm (Xu et al., 2017, 2018), the Microphysical Aerosol Properties from Polarimetry (MAPP) algorithm (Stamnes et al., 2018), and the Multi-Angular Polarimetric Ocean coLor (MAPOL) algorithm (Gao et al., 2021a, b, 2022, 2023). Of the full inversion approaches only the RemoTAP and GRASP algorithms have demonstrated this capability using real data at a global scale. These are the two algorithms that are evaluated in the present work.
Already important scientific advancement has been made based on retrieval products from the GRASP and RemoTAP algorithms. For example, data assimilation and inverse modeling studies have been performed for GRASP (Chen et al., 2018, 2019) and RemoTAP (Tsikerdekis et al., 2021, 2023), making use explicitly of unique MAP information on size and absorption by aerosols. Also, the data products have been used for the model evaluation of aerosol absorption and quantifying the direct radiative effect of aerosols (Lacagnina et al., 2016, 2017; Chen et al., 2022b), as well as the radiative forcing due to aerosol–cloud interactions (Hasekamp et al., 2019b). Both algorithms have already shown good performance against ground-based measurements from the Aerosol Robotic Network (AERONET) (Chen et al., 2020; Lacagnina et al., 2017; Schutgens et al., 2021; Zhang et al., 2021), although also limitations of the products were identified in the validation studies (e.g., bias at low AOD for both products).
In this paper, we present a detailed comparison of the most recent versions of RemoTAP and GRASP. We evaluate both algorithms for synthetic observations, for real PARASOL observations against AERONET for common pixels, and for global PARASOL retrievals for the year 2008. Through this comparison, we identify aspects of both data products with high fidelity and aspects that need improvement. Section 2 describes the data and methods used in this study, Sect. 3 shows a comparison between the RemoTAP and GRASP forward models and a comparison of the retrievals from simulated observations, and Sect. 4 shows a comparison of the GRASP and RemoTAP data products against AERONET and each other. Finally, Sect. 5 concludes the paper.
In this section, we summarize the main characteristics of the RemoTAP and GRASP algorithms and describe experiments with synthetic data and the validation and comparison approach for retrievals from real PARASOL observations.
2.1 Retrieval algorithms
The RemoTAP algorithm has been developed at SRON Netherlands Institute for Space Research. It combines the capability for aerosol retrieval from MAP measurements (Hasekamp et al., 2011; Wu et al., 2015, 2016; Fu and Hasekamp, 2018; Fu et al., 2020) with the capability to retrieve trace gas columns from spectrometer measurements in the near-infrared and shortwave-infrared bands (Hasekamp and Butz, 2008; Butz et al., 2009, 2011) into one algorithm (Lu et al., 2022). The latest version of RemoTAP is described by Lu et al. (2022) for retrievals over land, whereas the ocean model for retrievals over ocean is described by Fan et al. (2019). RemoTAP is based on the iterative fitting of a linearized radiative transfer model to the measurements of intensity and polarization of light reflected by the Earth's atmosphere and surface. It has large flexibility in the definition of parameters to be retrieved and allows retrievals over land, ocean, and clouds. The RemoTAP software has a strong tradition in the application to PARASOL measurements, as well as airborne measurements of the Research Scanning Polarimeter (RSP), Spectropolarimeter for Planetary EXploration (SPEX) airborne instrument, and the Airborne Multi-angle SpectroPolarimeter Imager (airMSPI). RemoTAP is also planned to be used for operational processing of the data from SPEXone on PACE. Main characteristics of the algorithm are summarized in Appendix A.
The GRASP algorithm has been developed and maintained by the Laboratoire d'Optique Atmosphérique (LOA)/CNRS of the University of Lille and the GRASP SAS company. GRASP is a new-generation algorithm developed for deriving extensive aerosol and surface properties from diverse space-borne and ground-based instruments, as well as their synergy (Dubovik et al., 2014, 2019, 2021). In applications for MAP measurements, the algorithm retrieves a set of aerosol and surface parameters simultaneously using the measurements at all wavelengths, all angles, and all states of polarization. GRASP retrieval is based on statistical optimization in the frame of the multi-term least-squares method concept (Dubovik et al., 2021) that allows the use of multiple constraints simultaneously. In addition, GRASP is designed to perform multi-pixel inversion where statistically optimized retrieval is implemented for a large group of satellite pixels (Dubovik et al., 2011). This allows us to improve retrieval by using additional a priori constraints on spatial or temporal variability in any retrieved parameter in different pixels. Moreover, the GRASP algorithm is highly flexible and versatile in the modeling of aerosol and surface properties and can be adapted to various situations with different information content in the measurements (Dubovik et al., 2021; Litvinov et al., 2011; Lopatin et al., 2013, 2021; Chen et al., 2020, 2022a; L. Li et al., 2022). The open-source software and documentation are available at https://www.grasp-open.com/ (last access: 23 February 2024). Main characteristics of the algorithm are summarized in Appendix A.
Both RemoTAP and GRASP algorithms are so-called “full physics” algorithms that iteratively fit a radiative transfer model to observations of intensity and polarization of scattered/reflected light. However, there are also important differences between RemoTAP and GRASP.
-
Inversion approach. GRASP uses a multi-pixel approach where multiple ground pixels (in time and space) are used at the same time in the inversion procedure (Dubovik et al., 2011, 2014, 2021), imposing smoothness constraints on the temporal and spatial changes in surface and aerosol properties. All GRASP retrievals in this paper have been performed using the multi-pixel approach. RemoTAP uses a single-pixel approach where a separate inversion is applied for each ground pixel.
-
State vector definition. GRASP uses an aerosol description based on five fixed-size modes (three for the fine and two for the coarse mode). The GRASP refractive index for each mode is represented as the internal mixture of several chemical components (Li et al., 2019). Here, the refractive index for fine mode is represented as the internal mixture of the black and brown carbon (BC and BrC), quartz (fine-mode dust), and soluble components. The refractive index of the coarse mode is internal mixture of iron oxides, quartz, and soluble components. For both modes the soluble component mimics sulfate, ammonium nitrate, or sea salt dissolved in water. RemoTAP uses an aerosol description based on three modes where effective radius reff and effective variance veff of each mode are retrieved. As in GRASP, the refractive index is described by a mixture of chemical components, but the contribution of each component is retrieved independently.
-
Forward model. Both GRASP and RemoTAP use the same pre-calculated tables (kernels) of the single scattering aerosol optical properties, based on Mie, T-matrix, and geometrical optics computations for spherical and spheroidal particles in a wide range of the complex refractive indices, non-sphericity, and size parameters (Dubovik et al., 2006). The kernels are presented on a fixed grid of the size parameter () taken at the wavelength of 340 nm. To do calculations for an arbitrary wavelength, RemoTAP uses a fixed grid of the size parameter so that the tabulated values do not have to be interpolated (but the size distribution has to be computed for a different radius grid for different wavelengths). GRASP on the other hand uses a fixed radius grid of the size distribution, which means that the tabulated optical properties have to be interpolated for each different wavelength. The radiative transfer solvers used by RemoTAP (Landgraf et al., 2002; Hasekamp and Landgraf, 2002, 2005; Schepers et al., 2014) and GRASP (Lenoble et al., 2007; Waquet and Herman, 2019; Herreras-Giralda et al., 2022) are also different, but this has a minor impact.
-
Measurement vector. RemoTAP includes (sun-normalized) intensity I together with the degree of linear polarization (DoLP), which is defined as . The assumed (1σ) uncertainty is 1 % for I and 0.007 (absolute) for DoLP. GRASP includes (sun-normalized) intensity I together with the Stokes fractions and . The assumed uncertainty is 1 % for I and 0.002 (absolute) for q and u. The assumed measurement uncertainties for both GRASP and RemoTAP are likely underestimated (Fougnie et al., 2007; Snik et al., 2014), which results in χ2 values that are larger than 1.
-
Data filtering. GRASP applies cloud screening from PARASOL based on Zeng et al. (2011) prior to the retrievals and applies a posteriori filter-removing retrievals with a pixel-level minimum relative fit residual > 3 % over land and > 10 % over ocean. RemoTAP applies a cloud screening based on MODIS, keeping only cloud fractions < 0.20, prior to the retrieval and applies a posteriori filter-removing pixels for which χ2>5. The a posteriori filter based on χ2 provides an additional cloud filter (Stap et al., 2015). The different filtering approaches between GRASP and RemoTAP can partly compensate for the different assumed measurement uncertainties.
The latest version of RemoTAP (Lu et al., 2022) used in this paper is substantially different from the previous version used for global PARASOL processing (Lacagnina et al., 2017). The most important difference from the previous version is the three-mode aerosol description compared to the two-mode description used previously. Other differences include a more extended lookup table for first-guess retrievals, the description of the refractive index by a contribution from different chemical components, and the use of a new model for the ocean body contribution (Fan et al., 2019). The version of GRASP, referred to as GRASP-CC, is based on the recently developed chemical component approach (Li et al., 2019; Zhang et al., 2021), while the previous global versions of GRASP (Chen et al., 2020) were based on the GRASP “optimized”, “high precision”, and “models” approaches, referred to as GRASP-O, GRASP-HP, and GRASP-M. The validation results of Chen et al. (2020) suggest that GRASP-M provides the most accurate AOD, while the most accurate detailed aerosol microphysical properties are provided by GRASP-HP. At the same time, the total AOD from the GRASP-HP product had an issue of non-negligible biases for low-AOD cases, which suggested a general difficulty in retrieving many free parameters when the information content is low (Chen et al., 2020). In that respect, the latest GRASP-CC approach uses a reduced set of parameters but more parameters than in the GRASP-M approach. In the present paper we also shortly summarize the validation results of the previous RemoTAP and GRASP products mentioned above in order to compare with the latest versions.
2.2 Setup for synthetic comparison
For a comparison between the forward models of RemoTAP and GRASP we use a set of aerosol properties of Table 1 and surface properties of Table 2. We have chosen a typical bi-modal size distribution, with refractive index representative of organic carbon, where we vary the AOD of the fine and coarse modes. So, different size distributions are accounted for by different contributions of the fine and coarse modes. We do not expect the forward model comparison to depend much on the refractive index because the optical properties of the refractive index (in the Mie and T-matrix code) are treated the same in GRASP and RemoTAP. For the geometries, we use PARASOL geometries from overpasses of the AERONET stations of Table 3, which represent a wide variety of geometries. The next step is to compare synthetic retrievals of RemoTAP and GRASP. For this purpose we created two sets of synthetic measurements that are as realistic as possible and not consistent with assumptions made in both retrieval codes:
-
RemoTAP-ECHAM is based on aerosol microphysical properties from simulations by the ECHAM-HAM aerosol climate model. ECHAM-HAM provides the mass mixing ratio in different vertical layers of the atmosphere of different aerosol species (sulfate, organic carbon, black carbon, dust, sea salt) in seven different size modes: nucleation soluble (NS), Aitken soluble (KS), accumulation soluble (AS), coarse soluble (CS), Aitken insoluble (KI), accumulation insoluble (AI), and coarse insoluble (CI). Based on the composition we can compute the refractive index for each mode. The seven-mode aerosol description is different from the standard setup of the RemoTAP algorithm (three modes with different refractive indices) and also from the standard GRASP algorithm (five modes with a mixture of different chemical components for the refractive index). For surface BRDF (bidirectional reflectance distribution function) and BPDF (bidirectional polarization distribution function) properties and AOD we use PARASOL-retrieved values over the AERONET sites of Table 3. The solar and viewing geometries are based on PARASOL geometries for overpasses of these AERONET stations. The corresponding measurements are generated by the RemoTAP forward model.
-
GRASP-AERONET is based on AERONET retrievals for the five stations of Table 3. AERONET retrieves the aerosol size distribution in 22 bins, as well as one size-independent, wavelength-dependent complex refractive index. The 22-bin size distribution is inconsistent with the assumptions in both RemoTAP and GRASP. For surface BRDF–BPDF and geometry we use the values from PARASOL overpasses as above. The corresponding measurements are generated by the GRASP forward model.
We apply both RemoTAP and GRASP-CC to both sets of synthetic measurements.
Table 1Aerosol properties used for forward model comparisons. RRI: real refractive index; IRI: imaginary refractive index.
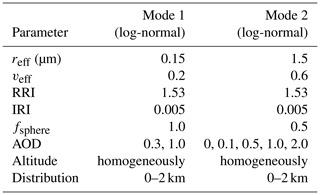
2.3 Validation and comparison approach for PARASOL retrievals
We performed retrievals from PARASOL Collection 3 (C3) level-1 data for the year 2008 by both RemoTAP and GRASP-CC and validated the results with AERONET Version 3 Level 2.0 data (https://aeronet.gsfc.nasa.gov/, last access: 23 February 2024). Also, we performed a global comparison of retrieved properties. Both RemoTAP and GRASP-CC perform retrievals at the native PARASOL pixel size of 6 km × 6 km. For validation with AERONET, we consider 3×3 pixels centered over all available AERONET sites in 2008. Only comparisons were performed if AERONET, GRASP-CC, and RemoTAP were available. We performed validation for the AOD and SSA at 550 nm (AOD550 nm; SSA550 nm), as well as the Ångström exponent (AE) between 440 and 870 nm. Here, it should be noted that the AOD (and hence AE) AERONET product is based on direct sun measurements achieving high AOD accuracy (± 0.01–± 0.02), whereas the SSA is based on the inversion of diffuse sky measurements which rely on several retrieval assumptions, leading to a moderate accuracy of ± 0.03 (Dubovik et al., 2000). Also, inversion level-2 products are strongly filtered on many quality criteria and are only available when AOD (440 nm) > 0.4. This significantly reduces the number of SSA comparisons with AERONET. For the different aerosol properties we compare the mean retrieved value of the nine PARASOL pixels corresponding to an overpass to the mean AERONET value within ± 1 h of the overpass. We evaluate the differences between the PARASOL retrievals and AERONET against the requirements formulated by the Global Climate Observing System (GCOS). For AOD the GCOS requirement is that the AOD error should be smaller than 0.03 % or 10 % (whichever is greater). For AERONET validation, this requirement has been modified in the Aerosol CCI (Climate Change Initiative) study (Popp et al., 2016) to 0.04 % or 10 % to also take into account the uncertainty in AERONET AOD. For SSA the GCOS requirement is that the error should be smaller than 0.03. This requirement is not modified for AERONET evaluation given that the 0.03 requirement is considered already loose (Popp et al., 2016). As a validation metric we use the fraction of retrievals with an “error” (defined as the difference between retrieved value and corresponding AERONET value) smaller than the corresponding requirement. Although for AE no requirement has been formulated, we report the fraction of retrievals with an AE error smaller than 0.2.
For the global comparison between RemoTAP and GRASP products we first grid both products on a 0.1° by 0.1° grid and perform the comparison of all common grid cells. Finally, we compare both RemoTAP and GRASP-CC AOD against a merged MODIS Deep Blue (MODIS-DB) (Hsu et al., 2013) and Dark Target (MODIS-DT) (Levy et al., 2013) product and the AE against MODIS-DT (land and ocean). MODIS Collection 6.1 aerosol products (MYD04L2) from the DT and DB algorithms were acquired from the AERIS ICARE Data and Services Center (http://www.icare.univ-lille.fr, last access: 23 February 2024), where the unchanged NASA MODIS data are redistributed.
3.1 Forward model comparison
Figure 1 shows the forward model comparisons between RemoTAP and GRASP. The bias and standard deviation of the differences in radiance are mostly below 2 % and for DoLP mostly below 0.005. There is a dependence on wavelength of the differences: for radiance the differences are larger at 490 and 565 nm, and for DoLP the differences are larger at 670 nm (up to 0.01 for some angles). The wavelength dependence of the differences can most likely be explained by differences in the way optical aerosol properties are computed from micro-physical properties using the tabulated Mie, T-matrix, and geometric optics calculations because GRASP and RemoTAP use different methods to interpolate wavelength and particle size. Overall, the comparison between the GRASP and RemoTAP forward models looks reasonably good, and the differences are in general smaller than the measurement uncertainty for PARASOL: of 2 % for radiance (Fougnie et al., 2007) and 0.01–0.02 for DoLP (Snik et al., 2014). Below we will investigate the importance of the forward model differences by performing RemoTAP retrievals on synthetic measurements created by the GRASP forward model and vice versa.
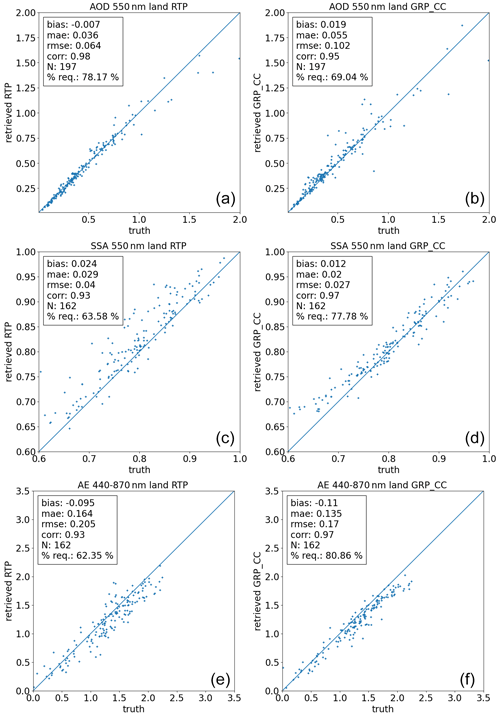
Figure 2Synthetic retrieval results for the “RemoTAP-ECHAM” synthetic measurements created by the RemoTAP forward model. Shown are the results for RemoTAP (a, c, e) and GRASP-CC (b, d, f). Properties shown (from top to bottom) are the AOD, SSA, and AE. AOD results have been filtered for RemoTAP χ2 < 1 and GRASP-CC minimum residual < 3 % (keeping 197 out of 202 retrievals). AE and SSA results have been additionally filtered for AOD > 0.2.
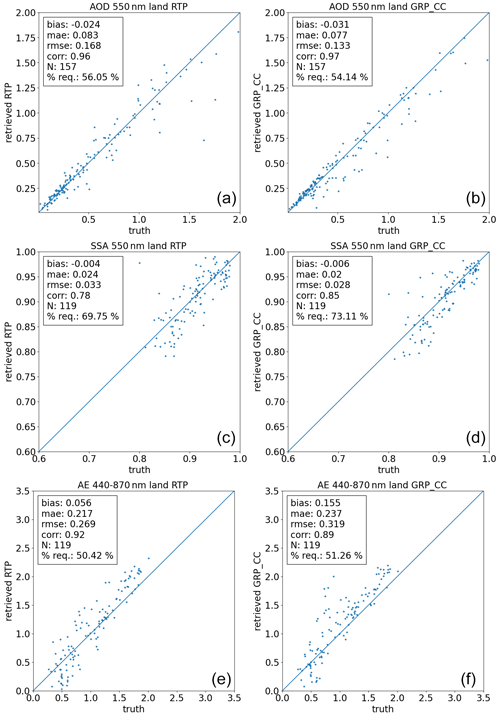
Figure 3Synthetic retrieval results for the “GRASP-AERONET” synthetic measurements created by the RemoTAP forward model. Shown are the results for RemoTAP (a, c, e) and GRASP-CC (b, d, f). Properties shown (from top to bottom) are the AOD, SSA, and AE. Results have been filtered for RemoTAP χ2 < 1 and GRASP-CC minimum residual < 3 % (keeping 157 out of 202 retrievals). AE and SSA results have been additionally filtered for AOD > 0.2.
3.2 Synthetic retrievals
In reality, the assumed aerosol description in the retrieval will typically be different from the real aerosol properties. Therefore, it is important to investigate the performance of the algorithms for synthetic measurements created with a more extended and realistic set of aerosol properties than assumed in the retrieval. At the same time, it is important to evaluate the effect of differences due to differences in the forward models of GRASP and RemoTAP. Therefore, the “RemoTAP-ECHAM” measurements have been created with the RemoTAP forward model, and the “GRASP-AERONET” measurements have been created with the GRASP forward model (see above).
Figure 2 shows the results for the RemoTAP-ECHAM synthetic measurements created by the RemoTAP forward model. For AOD, GRASP-CC retrievals have a root mean square error (RMSE) and mean absolute error (MAE) of 0.10 % and 0.055 % and are 69 % within the GCOS–CCI requirement, whereas RemoTAP has a smaller RMSE and MAE of 0.064 and 0.036 and a larger fraction (78.2 %) within the GCOS requirement. For SSA, GRASP-CC shows the best performance with an RMSE and MAE of 0.027 and 0.024 (77.8 % within GCOS–CCI), while RemoTAP has an RMSE and MAE of 0.04 and 0.029 (63.6 % within GCOS). For AE, GRASP-CC also has a smaller RMSE and MAE (0.17 and 0.135) than RemoTAP (0.21 and 0.164). Figure 3 shows the results for the GRASP-AERONET synthetic measurements created by the GRASP forward model. For AOD, GRASP-CC has an RMSE and MAE of 0.13 % and 0.077 % and 54.1 % within GCOS–CCI requirements, whereas RemoTAP has an RMSE and MAE of 0.17 % and 0.083 % and 56.1 % within GCOS–CCI requirements. For SSA, GRASP-CC has an RMSE and MAE of 0.028 % and 0.02 % with 73.1 % of retrievals within GCOS–CCI requirements and a bias of −0.006. RemoTAP has an RMSE and MAE of 0.033 and 0.024 and a fraction of retrievals within GCOS–CCI requirements (69.8 %) but a similar bias (−0.004). For AE RemoTAP has a smaller RMSE and MAE in the GRASP-AERONET synthetic measurements (RMSE and MAE of 0.269 and 0.217 versus 0.319 and 0.237). To conclude, we see that for AOD the best performance is obtained for the algorithm whose forward model has been used to generate the synthetic measurements. For SSA, GRASP-CC has a smaller RMSE and MAE for both sets of synthetic measurements. For AE, interestingly GRASP-CC has a smaller RMSE and MAE for the RemoTAP-ECHAM synthetic measurements, and RemoTAP has a smaller RMSE and MAE for the GRASP-AERONET synthetic measurements. However, overall the difference in performance for synthetic measurements is small, and both RemoTAP and GRASP-CC have demonstrated robustness against aerosol assumptions and forward model differences and are capable of performing accurate retrievals under different circumstances.
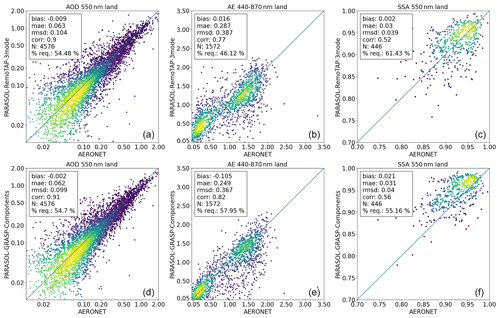
Figure 4Validation of RemoTAP (a, b, c) and GRASP-CC (d, e, f) retrievals over land with AERONET for AOD (a, d), AE (b, e), and SSA (c, f) at 550 nm.
Table 6Validation metrics of the previous RemoTAP (Lacagnina et al., 2017) and GRASP-HP and GRASP-M (Chen et al., 2020) PARASOL products. Note that for SSA the validation was not separated in retrievals over land and ocean because not enough data points are available for comparison. The validation has been performed for the year 2006 and a spatial gridding of 0.2° because the RemoTAP global product is only available for this year and spatial gridding. The difference in gridding explains the different values for RMSE than those found by Chen et al. (2020), where a spatial gridding of 0.1° was used.
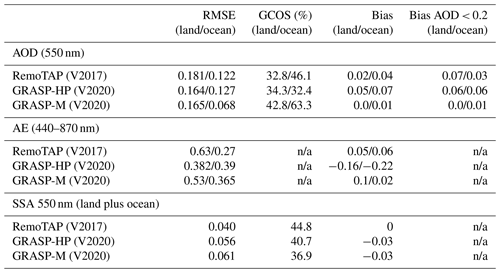
4.1 Validation with AERONET
4.1.1 Retrievals over land
Figure 4 shows the validation of AOD550 nm, AE, and SSA550 nm against AERONET for both RemoTAP and GRASP-CC retrievals over land. Metrics for AOD validation for different AOD ranges can be found in Table 4 and metrics for SSA, AE, and fine- and coarse-mode AOD in Table 5. Only common pixels are included in this validation. For comparison, we also show validation results of the previous versions of RemoTAP (Lacagnina et al., 2017) and GRASP (GRASP-HP and GRASP-M) (Chen et al., 2020) in Table 6. Although these validation results correspond to a different year (2006) and a different spatial gridding (0.2° versus 0.1°), we can clearly see that the latest versions of RemoTAP and GRASP(-CC) have substantially improved compared to the earlier version(s). The latest algorithm versions used in the present study show closer agreement with AERONET for AOD and SSA. For AE, the present version of GRASP (CC) shows similar agreement to AERONET as the previous (HP) version, but RemoTAP improved significantly, removing a large positive bias at small AOD, also reported by Tsikerdekis et al. (2023). If we compare the performance between RemoTAP and GRASP-CC, we see that for AOD both algorithms show very similar performance to AERONET, both in terms of MAE and RMSE and bias but also for the fraction of the retrievals within GCOS–CCI requirements. For AE, GRASP-CC still has a smaller RMSE because RemoTAP still shows a small overestimate at low AE values and an underestimate at large values, although the difference in RMSE with GRASP-CC is very small. The comparison of fine- and coarse-mode AOD confirms the better performance of GRASP-CC for aerosol size retrieval. For SSA, RemoTAP shows a smaller bias against AERONET than GRASP-CC. The RMSE for SSA is comparable for both RemoTAP and GRASP-CC, and the fraction of retrievals within the GCOS–CCI requirements is 61.4 % for RemoTAP and 55.2 % for GRASP-CC.
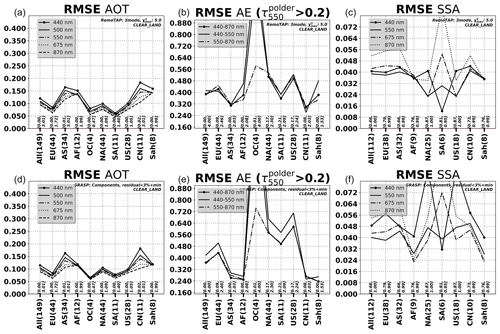
Figure 5RMSE for different regions/countries for AOD (a, d), AE (b, e), and SSA (c, f). Upper panels correspond to RemoTAP retrievals and lower panels to GRASP-CC retrievals. Metrics are shown for all stations (all), Europe (EU), Asia (AS), Africa (AF), Oceania (OC), South America (SA), North America (NA), USA (US), China (CN), and the Sahara (Sah).
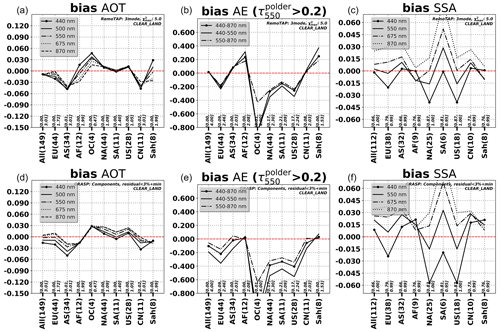
Figure 6Bias for different regions for AOD (a, d), AE (b, e), and SSA (c, f). Upper panels correspond to RemoTAP retrievals and lower panels to GRASP-CC retrievals.
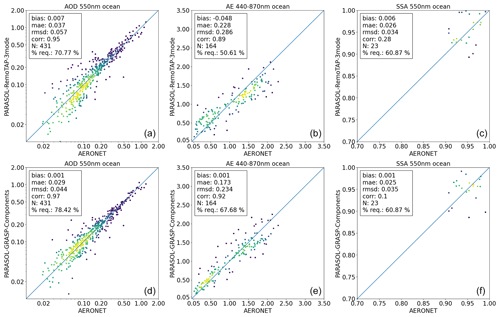
Figure 7Validation of RemoTAP (a, b, c) and GRASP-CC (d, e, f) retrievals over ocean with AERONET for AOD (a, d), AE (b, e), and SSA (c, f) at 550 nm.
Figures 5 and 6 show the RMSE and bias of AOD, AE, and SSA, respectively, for different regions/countries: Europe (EU), Asia (AS), Africa (AF), Oceania (OC), South America (SA), China (CN), and the Sahara (Sah). In AOD, we see many similarities between RemoTAP and GRASP-CC in the regional dependence of RMSE, with large RMSE (in absolute sense) over Asia, China, and the Sahara for both algorithms, which are regions with large AOD. Low values for RMSE are found over Europe and South and North America. Over Oceania, RemoTAP has larger RMSE than GRASP-CC (especially at smaller wavelengths). Looking at the bias, RemoTAP has a smaller bias over Europe, Asia, and China and a larger bias over Oceania and the Sahara. Both algorithms have a similar bias over Africa (although opposite in sign), North America, South America, and the USA. The regional dependence for RMSE and bias for AE shows a similar pattern to that of AOD for both RemoTAP and GRASP-CC, with the exception that GRASP-CC, like RemoTAP, also shows large RMSE and bias over Oceania, while for AOD this region was only problematic for RemoTAP. Further, we see from Figs. 5 and 6 that overall the AE behaves similarly for different wavelength pairs, although the pair 550–870 nm shows smaller RMSE and bias over Oceania. For SSA we see that GRASP-CC shows larger variation in RMSE over regions than RemoTAP. For bias, GRASP-CC also shows larger variation over region than RemoTAP, but there is a clear correlation between the two algorithms. We also see that both RemoTAP and GRASP-CC show worse performance for SSA at 870 nm than the other wavelengths, which is most likely caused by the fact that AOD at 870 nm is typically small.
4.1.2 Retrievals over ocean
Figure 7 shows the validation of AOD550nm, AE, and SSA550 nm against AERONET observations for coastal stations for both RemoTAP and GRASP-CC retrievals (common pixels) over ocean. It should be noted that in coastal regions the ocean (body) reflection is more complex than over the open ocean, which may be expected to result in less-accurate aerosol retrievals. Metrics for AOD validation for different AOD ranges can be found in Table 7 and metrics for SSA, AE, and fine- and coarse-mode AOD in Table 8. As expected, the performance for AOD and AE of both algorithms over ocean is better than over land, and both algorithms perform very well against AERONET. We also note that GRASP-CC has a larger GCOS fraction and smaller MAE, RMSE, and bias for AOD than RemoTAP, although the differences are small (≤0.01). Zooming in to different AOD ranges (Table 7), we see the same general behavior. For AE, both RemoTAP and GRASP-CC show a tendency to underestimate AE for larger values. Like for AOD, GRASP-CC shows smaller MAE, RMSE, and bias than RemoTAP. For SSA, the agreement with AERONET is similar to RemoTAP and GRASP-CC, but the number of co-locations is too small to draw further conclusions. Comparing the validation results over ocean to those of the previous versions of the RemoTAP and GRASP products in Table 6, we can see that the RMSE of the RemoTAP AOD improved by about a factor of 2 and the GRASP-CC product improved by even more compared to the previous GRASP-HP product and by about 30 % compared to the GRASP-M product. Also the bias of RemoTAP (overall and for AOD smaller than 0.2) and the bias of GRASP improved compared to the previous GRASP-HP product. For AE, GRASP-CC improved considerably, while the RemoTAP performance for AE stays similar to the previous product version. Like over land, GRASP-CC has a smaller RMSE for AE over ocean, and this is consistent with the results for fine- and coarse-mode AOD.
4.2 Comparison of global data products
We performed global processing with RemoTAP and GRASP-CC and compared the gridded data products (0.1 × 0.1°). Both RemoTAP and GRASP-CC provide a similar number of valid retrievals after data filtering. Also, we perform a comparison with MODIS data products.
4.2.1 Comparison of AOD
Figure 8 shows maps of the mean AOD for GRASP-CC and RemoTAP, respectively, and a map of the mean differences. Overall, GRASP-CC and RemoTAP show the same AOD pattern with high AOD over the Sahara (dust) and equatorial Africa (biomass burning) and the outflow of dust and biomass burning aerosol over the Atlantic Ocean. Also, high AOD values are retrieved over polluted areas in east Asia and the Ganges valley. Also interesting is the high mean AOD over Siberia, which is related to boreal forest fires. If we look at the differences between RemoTAP and GRASP-CC, for most of the globe the differences are small. Exceptions are the Sahara and the Arabian Peninsula, where RemoTAP AOD is higher by 0.05–0.10, related to differences in retrieved BPDF (see Sect. 4.4) and equatorial Africa, where RemoTAP AOD is lower than GRASP-CC by almost 0.15. Also notable is the difference (especially in relative sense) over the most southern part of the ocean where RemoTAP retrieves lower AOD than GRASP-CC.
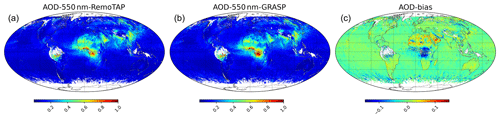
Figure 8Annual mean AOD for (a) RemoTAP and (b) GRASP-CC and (c) the bias between them (RemoTAP−GRASP).
Figure 9 shows scatter plots of the RemoTAP AOD versus the GRASP-CC AOD at 550 nm and separately for retrievals over land and ocean. Also, a histogram of the differences is shown. Over land, the root mean square difference (RMSD) is 0.12, and the difference in the mean (RemoTAP−GRASP) is 0.01. The RMSD and bias are larger at 443 nm (0.14 and 0.026, respectively) and comparable at 865 nm (not shown). Over ocean, the RMSD is 0.038 nm and the bias is −0.008. The differences are larger at 443 nm and smaller at 865 nm (not shown).
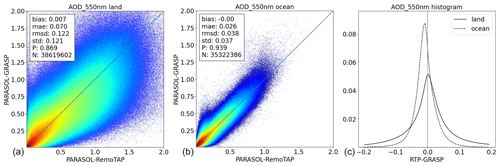
Figure 9AOD (550 nm) scatter plots (GRASP-CC versus RemoTAP) for retrievals over land (a) and ocean (b) and a histogram of the differences (c), where the dashed line corresponds to retrievals over ocean and the solid line to retrievals over land.
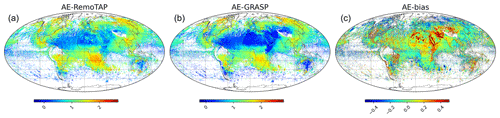
Figure 10Annual mean AE for (a) RemoTAP and (b) GRASP-CC and (c) the bias between them (RemoTAP−GRASP). Only retrievals with AOD550nm>0.2 are included.
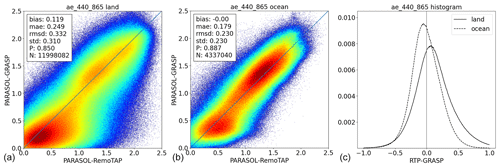
Figure 11AE scatter plots (GRASP-CC versus RemoTAP) for retrievals over land (a) and ocean (b) and a histogram of the differences (c), where the dashed line corresponds to retrievals over ocean and the solid line to retrievals over land. Only retrievals with AOD550nm>0.2 are included.
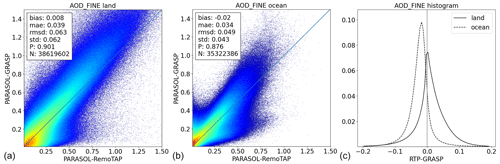
Figure 13Fine-mode AOD (550 nm) scatter plots (GRASP-CC versus RemoTAP) for retrievals over land (a) and ocean (b) and a histogram of the differences (c), where the dashed line corresponds to retrievals over ocean and the solid line to retrievals over land.
4.2.2 Comparison of AE and fine- and coarse-mode AOD
Figure 10 shows maps of the mean AE (440–865 nm) for GRASP-CC and RemoTAP and the map of the mean differences. These maps include only retrievals for cases where AOD550nm>0.2. The AE maps for RemoTAP and GRASP-CC both show a very similar overall pattern. Small AE values (larger sizes) occur over desert and over the open ocean and correspond mostly to situations dominated by dust or sea salt. Larger AE values (smaller sizes) occur over areas with anthropogenic pollution (Asia, Europe, North and South America) and over areas with biomass burning (southern Africa, South America, Indonesia, Australia). The most important differences between RemoTAP and GRASP-CC arise over the Sahara and Middle East, where RemoTAP retrieves higher AE than GRASP-CC, which is consistent with the AERONET comparison showing overestimated AE values for big particles provided by RemoTAP. On the other hand, the maximum differences (where RemoTAP can be 0.5 higher than GRASP-CC) are not expected from the AERONET comparison.
Figure 11 shows scatter plots of the RemoTAP AE versus the GRASP-CC AE and separately for retrievals over land and ocean. Only retrievals with AOD > 0.2 are included. Also, a histogram of the differences is shown. Over land, the RMSD is 0.33, while over ocean the RMSD is 0.23. Over land, the agreement is very good for larger values of AE (small particle size) and a bit worse for lower AE values (large particles size), where RemoTAP retrieves larger AE. Over ocean, the agreement is very good for both low and high AE values.
When using a lower AOD threshold of 0.1 (not shown), the agreement gets worse with RMSD values of 0.48 and 0.36 over land and ocean, respectively. Over land, the overall scatter of the data increases compared to the higher AOD threshold, while over ocean we also see some specific cases where RemoTAP retrieves a small AE (close to 0) and GRASP-CC retrieves values up to 1.5. To investigate the dependence of AE difference on AOD in more detail, Fig. 12 shows the AE difference as a function of AOD. We can see the AE difference depends strongly on AOD. Over land, there is a large positive bias (0.4) at AOD = 0.10 which decreases gradually to 0 at AOD = 0.4. Over ocean, there is a bias of −0.45 at AOD = 0.05 which decreases more rapidly with AOD than over land. The strong dependence of AE difference on AOD results from the fact that at small AOD, the AE calculation is very sensitive to even small errors in AOD at the different wavelengths.
Figure 13 shows the comparison of the fine-mode AOD and Fig. 14 of the coarse-mode AOD. Over land, the RMSD for the fine-mode AOD is smaller than for the coarse-mode AOD (RMSD 0.063 versus 0.107). This means that for the total AOD, most of the differences can be explained by differences in the coarse-mode AOD. Further, we see that for the fine-mode AOD RemoTAP retrieves smaller values than GRASP-CC towards higher AOD, while for the coarse mode the opposite is observed. For retrievals over ocean, the RMSD for the coarse-mode AOD is smaller than for the fine mode. Further, for the fine mode RemoTAP is systematically smaller than GRASP-CC (−0.02 bias), while for the coarse mode RemoTAP is larger (0.016 bias) such that the total AOD bias between RemoTAP and GRASP-CC is very small.
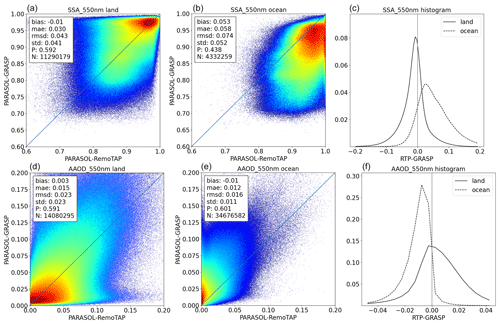
Figure 16SSA and AAOD (550 nm) scatter plots (GRASP-CC versus RemoTAP) for retrievals over land (a, d) and ocean (b, e) and a histogram of the differences (c, f), where the dashed line corresponds to retrievals over ocean and the solid line to retrievals over land.
4.2.3 Comparison of SSA and absorbing aerosol optical depth (AAOD)
Figure 15 shows maps of the mean SSA (550 nm) for GRASP-CC and RemoTAP, as well as the bias. Overall, the maps show similar patterns, but there are also important differences. In general, RemoTAP retrieves a higher SSA over ocean and lower SSA over land. Larger differences occur over equatorial Africa (biomass burning region) where RemoTAP retrieves a significantly higher (>0.10 difference) SSA than GRASP-CC over ocean but also over land (in contrast to other land regions). The difference is especially apparent over ocean. Also, over India and the Indian ocean there are notable differences in SSA between RemoTAP and GRASP-CC. Here, over land RemoTAP retrieves a mean SSA of 0.90, while GRASP-CC retrieves 0.95. Over ocean, the difference is opposite: RemoTAP retrieves an SSA of 0.95–1, while GRASP-CC retrieves 0.90. Both RemoTAP and GRASP-CC show an unexpected sharp transition between land and ocean in this region. A similar pattern can be seen at the west coast of the USA.
Figure 16 shows scatter plots of the RemoTAP SSA versus the GRASP-CC SSA at 550 nm and separately for retrievals over land and ocean. Also, a histogram of the differences is shown. Over land, the RMSD is 0.043, whereas the overall bias is <0.01. There is some compensation between different areas, as is apparent from the world map, but overall, the agreement can be considered good over land. Over ocean the differences are substantially larger, with an RMSD and bias of 0.074 and 0.053, respectively. Here, RemoTAP retrieves a higher SSA than GRASP-CC, as was already seen from the world map. As noted above, the AERONET SSA validation over ocean does not have sufficient points to indicate whether the difference is caused by errors in RemoTAP or GRASP-CC. Clearly, there is a need for more SSA validation points over ocean. Figure 16 also shows the comparison of AAOD. Over land, the overall bias is relatively small (0.003), but there is considerable scatter between RemoTAP and GRASP-CC. Over ocean, there is a clear bias between RemoTAP and GRASP-CC where GRASP-CC retrieves higher AAOD than RemoTAP. This is expected because GRASP-CC retrieves a smaller SSA than RemoTAP over ocean and comparable AOD, which should result in a higher AAOD.
Figure 17 shows the SSA difference (RemoTAP−GRASP) as a function of AOD (mean of RemoTAP and GRASP-CC) for retrievals over land and ocean. Over land, we see that for AOD < 0.15 the difference between RemoTAP and GRASP-CC is largest where the mean difference reaches −0.10 for the lowest AOD values. The SSA difference decreases (in absolute sense) rapidly till AOD = 0.15 where the SSA difference is −0.01 and slowly decreases further to higher AOD. Despite the fact that on average the RemoTAP SSA is lower than the GRASP-CC SSA, we see that for a substantial number of retrievals the GRASP-CC SSA is higher. These retrievals correspond mostly to biomass burning retrievals (see above).
4.3 Summary of global aerosol comparison
Table 9RMSD and bias between GRASP and RemoTAP for global retrievals for the year 2008 (Jan–Nov) for the properties AOD, SSA, and AE. The table shows both the comparison of the new products and the previous versions of the RemoTAP (Lacagnina et al., 2017) and GRASP (Chen et al., 2020) products.
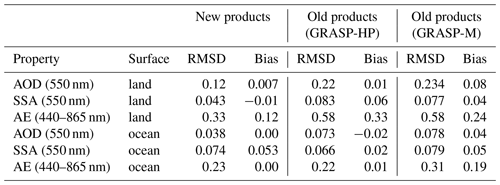
Table 9 shows a summary of the global comparison between GRASP-CC and RemoTAP for 2008. For reference, also the global comparison of the old RemoTAP and GRASP (-HP and -M) products is shown (for 2006 and at 0.2° spatial gridding). We can see that for AOD the agreement between GRASP-CC and RemoTAP improved significantly, with a reduction in RMSD by about a factor of 2. For AE over land, both the RMSD and bias improved by about a factor of 2, while for AE over ocean the agreement stays similar to the previous product versions. For SSA, the agreement over land is reasonable and significantly improved compared to the previous product versions. Over ocean, the agreement is poor with a bias of 0.053 (RemoTAP retrieves a higher SSA than GRASP-CC), and the agreement did not improve compared to the previous product versions.
4.4 Land surface properties
Table 10 shows the RMSD and bias for the comparison of the isotropic BRDF parameters (see Sect. B1) between RemoTAP and GRASP-CC at different wavelengths. We can see that RemoTAP retrieves a higher isotropic BRDF than GRASP-CC at all wavelengths, with a bias ranging from 0.018 to 0.028. The RMSD ranges from 0.018 to 0.036. The largest differences (in absolute sense) occur over the Sahara and the Arabian Peninsula (not shown), where the largest difference in AE aerosol parameter is also observed. Only at 865 nm does RemoTAP retrieve a smaller isotropic BRDF parameter than GRASP-CC over equatorial Africa. Table 11 shows the RMSD and bias for the directional BRDF parameters and the BPDF. RemoTAP retrieves larger values for the directional BRDF parameters and substantially lower BPDF scaling parameter than GRASP-CC. Like for all surface parameters, the difference is largest over the Sahara and the Arabian Peninsula. To investigate the difference in the BPDF scaling parameter in more detail, Fig. 18 shows the difference in the BPDF scaling parameter as a function of the difference in the isotropic BRDF parameter (490 nm). There is a very clear dependence when the difference in the isotropic BRDF parameter is in the range 0–0.05. Here, the difference in BPDF scaling gets more negative with the increasing difference in the isotropic BRDF parameter. So, a larger isotropic BRDF is compensated for with a smaller BPDF scaling in RemoTAP. This can be explained by the fact that the Fresnel reflection matrix that is scaled in the BPDF model also contributes to the BRDF. This would imply that the surface polarization is either too small in RemoTAP or too large in GRASP-CC.
Table 11Statistical metrics for BRDF and BPDF comparison. BRDF2 refers to the coefficient of the Ross-thick kernel and BRDF3 to the coefficient of the Li-sparse kernel.
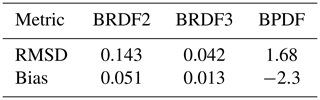
Figure 19 shows the difference in AOD between RemoTAP and GRASP-CC as a function of the difference in the retrieved isotropic BRDF parameter (at 490 nm) and the difference in the BPDF scaling parameter. It can be seen that for cases where RemoTAP retrieves a smaller isotropic BRDF parameter than GRASP-CC, it also retrieves a smaller AOD. The difference in AOD increases to −0.10 when the difference in the isotropic BRDF is also −0.10. For cases where RemoTAP retrieves a higher isotropic BRDF parameter, there is no clear dependence of AOD differences on the difference in the isotropic BRDF. There is also a clear dependence of the AOD difference on the difference in the BPDF scaling parameter. As noted above, RemoTAP retrieves much smaller values for the BPDF scaling than GRASP-CC. When the BPDF difference is −8, RemoTAP retrieves on average a higher AOD (0.10) than GRASP-CC. These cases correspond mostly to the Sahara and the Arabian Peninsula. On the other hand, when RemoTAP retrieves a higher BPDF than GRASP-CC (mostly over higher latitudes), it retrieves a smaller AOD, where the mean difference is −0.05. Figure 21 shows the difference in SSA between RemoTAP and GRASP-CC as a function of the difference in the retrieved isotropic BRDF parameter (at 490 nm) and the difference in the BPDF scaling parameter. For SSA, we see in particular a large effect on the isotropic BRDF parameter, where the SSA difference can be almost up to 0.10 when the difference in the isotropic BRDF parameter is −0.10. The dependence on BPDF is smaller and mostly apparent when RemoTAP retrieves a higher BPDF than GRASP-CC. Figure 20 shows the difference in AE between RemoTAP and GRASP-CC as a function of the difference in the retrieved isotropic BRDF parameter (at 490 nm) and the difference in the BPDF scaling parameter. Here we see a strong dependence on the BPDF scaling parameter. For large negative differences, i.e., when RemoTAP retrieves a smaller BPDF than GRASP-CC, there is a large positive difference in AE. These cases correspond mostly to the Sahara where indeed RemoTAP retrieves a higher AE than GRASP-CC. Here, the higher polarized reflectance retrieved by GRASP-CC will require bigger aerosol particles (smaller AE) and as a result a smaller degree of polarization over these areas to fit the top-of-atmosphere polarized reflectance. In contrast, smaller polarized surface reflectance provided by RemoTAP will result in smaller particles (bigger AE) and larger degree of polarization to insure the good fit of satellite polarization measurements. The AERONET comparison indicates a positive AE bias in RemoTAP over the Sahara, where a possible explanation is that RemoTAP retrieves a surface reflection matrix with polarization that is too small. Observed dependencies require additional studies to improve aerosol size characterization from polarimetric measurements. Having a “ground truth” for BRDF and BPDF retrievals, e.g., from airborne campaigns (Litvinov et al., 2011), would be useful for this purpose.
4.5 Comparison to MODIS
The MODIS Dark Target (MODIS-DT) (Levy et al., 2013) and Deep Blue (MODIS-DB) (Hsu et al., 2013) AOD products are the most widely used aerosol products. Here, we compare AOD and AE retrieved from PARASOL by both RemoTAP and GRASP-CC to the MODIS-DT and MODIS-DB products. Figure 22 shows the comparison of AOD over land and ocean. Over land, GRASP-CC has a smaller RMSD with MODIS than RemoTAP, but it should be noted that the RMSD between GRASP-CC and RemoTAP is smaller (see Fig. 9) than the agreement between both algorithms with MODIS. Over ocean, the agreement of both GRASP-CC and RemoTAP with MODIS is similar to the agreement between GRASP-CC and RemoTAP, although GRASP-CC has a smaller RMSD with MODIS than RemoTAP. The smaller differences between GRASP-CC and RemoTAP products over land than the differences between both algorithms and MODIS may suggest a more accurate AOD product over land for the PARASOL algorithms, especially because both PARASOL algorithms over land are closer to AERONET than MODIS (Chen et al., 2020). For the global distribution of differences (not shown), both GRASP-CC and RemoTAP show very similar patterns to MODIS, with a strong positive difference over most of Africa, India, and China; a weaker positive difference over Europe and the US; and some small spots of negative differences (e.g., over South America). Over the global ocean, RemoTAP shows a small (0.01–0.02) negative difference against MODIS, whereas GRASP-CC shows a small positive difference at midlatitudes and a small negative difference in most of the tropics.
Figure 23 shows the AE comparison of both PARASOL products with the MODIS-DT product over land and ocean. Over land, we can clearly see that the MODIS-DT AE tends to be centered around a number of discrete values. This is probably a result of the MODIS retrieval approach based on a discrete set of aerosol models. For both RemoTAP and GRASP-CC there is a large difference from the MODIS-DT AE, and the difference between RemoTAP and GRASP-CC, shown in Fig. 11, is much smaller than the agreement of both products with MODIS. This is expected because MODIS has limited information content on aerosol size over land which results in the poor comparison of MODIS-DT AE against AERONET (Chen et al., 2020; Reid et al., 2022). The same conclusions hold for the AE comparison with the MODIS-DB product over land (not shown). Over ocean, the difference between the PARASOL products and MODIS is smaller than over land but still substantially larger than the agreement between RemoTAP and GRASP-CC. This suggests that also over ocean both PARASOL algorithms provide a more accurate AE than MODIS, as expected.
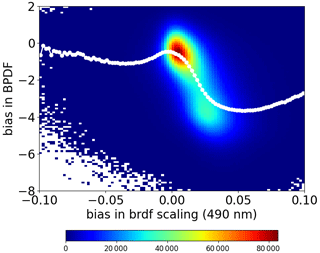
Figure 18Difference in BPDF scaling parameters as a function of the difference in the isotropic BRDF parameter (490 nm). Differences represent RemoTAP−GRASP. The x axis and y axis have been divided into 100 bins. The white circles indicate the median y value per x bin. The color indicates the number of data points per bin.
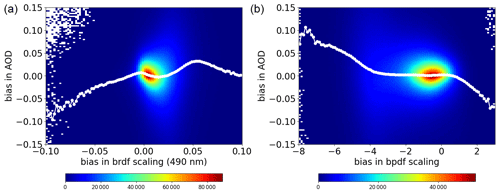
Figure 19Dependence of the AOD difference (RemoTAP−GRASP) on the difference in the isotropic BRDF parameter at 490 nm (a) and the BPDF scaling parameter (b). The x axis and y axis have been divided into 100 bins. The white circles indicate the median y value per x bin. The color indicates the number of data points per bin.
In this paper, we have performed a systematic intercomparison of the GRASP-CC and RemoTAP algorithms for aerosol retrieval from MAP measurements. The study involved a comparison of forward models, synthetic retrievals, validation results with AERONET, and a global comparison of 1 year of data processed by both algorithms. The forward models of GRASP and RemoTAP agree within 2 % (1 % for most wavelengths and angles) for radiance and within 0.01 (0.005 for most wavelengths and angles) for degree of linear polarization (DoLP). The differences are within the range of PARASOL uncertainties but may become important for more accurate future polarimeters like SPEXone (Hasekamp et al., 2019a) and the CO2 Monitoring Mission (CO2M) (Sierk et al., 2019). Synthetic retrievals were performed on two sets of synthetic measurements: one created by the RemoTAP forward model and the other one by the GRASP forward model. Both sets of synthetic measurements were based on a more complex aerosol description than that assumed in the algorithms. Both algorithms perform well on both sets of synthetic measurements.
For the AERONET comparisons, we obtained very similar results for AOD retrieved by both RemoTAP and GRASP-CC over land: both algorithms show an RMSE of 0.10 (at 550 nm), and 54 % of the retrievals fall within the GCOS requirements. For SSA, both algorithms have a similar RMSE (0.04), but RemoTAP has a smaller bias (0.002) compared to GRASP-CC (0.021). For AE, GRASP-CC has a slightly smaller RMSE (0.367) than RemoTAP (0.387), which is related to a small overestimate of AE at low values (large particles) by RemoTAP. Over ocean, RemoTAP has an RMSE of 0.057 and GRASP-CC an RMSE of 0.047. For AE, the RMSEs of RemoTAP and GRASP-CC are 0.285 and 0.224, respectively. Based on the AERONET comparison, we conclude that both algorithms show very similar overall performance, where both algorithms have stronger and weaker points. It should be noted that for SSA the agreement with AERONET in terms of RMSE is already close to the SSA uncertainty of AERONET itself (0.03), so possible further improvements in the algorithms for SSA will be hard to evaluate with AERONET. On the other hand, the RMSE for AOD is much larger than the AERONET uncertainty (0.01–0.02), which means there is enough margin to evaluate further improvements.
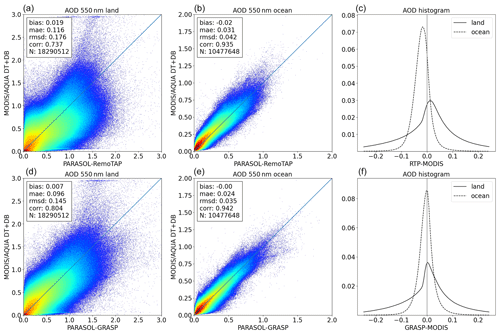
Figure 22AOD comparison with MODIS for RemoTAP (a, b, c) and GRASP-CC (d, e, f). Left panels show comparison over land, middle panels comparison over ocean, and right panels histograms of the differences. For MODIS the DT and DB aerosol products have been combined.
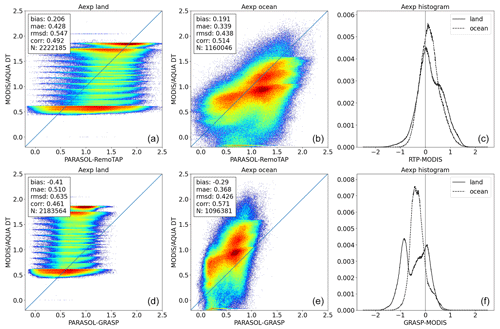
Figure 23AE comparison with MODIS-DT for RemoTAP (a, b, c) and GRASP-CC (d, e, f). Left panels show comparison over land, middle panels comparison over ocean, and right panels histograms of the differences.
Finally, global processing with both algorithms for PARASOL data of the year 2008 has been performed, and a comparison of gridded products (0.1 by 0.1°) has been performed. For the global comparison of AOD, the RMSD values are 0.12 and 0.037 over land and ocean, respectively. The largest differences occur over the biomass burning region in equatorial Africa. The global mean values are virtually unbiased with respect to each other. For AE the RMSD between RemoTAP and GRASP-CC is 0.33 over land and 0.23 over ocean when only retrievals with AOD > 0.2 are included. When taking retrievals with AOD > 0.1, the RMSD increases to 0.48 over land and 0.36 over ocean. Towards lower AOD, significant differences occur between the two data products because the AE calculation becomes very sensitive to even small errors in AOD at the different wavelengths when the AOD is small. Concerning fine- and coarse-mode AOD, over land, the RMSD is smaller for fine-mode AOD (RMSD = 0.063) than for coarse-mode AOD (RMSD = 0.11) and vice versa over ocean (RMSD = 0.049 for fine mode and 0.037 for coarse mode). Over ocean, the fine and coarse modes have opposite biases (0.02) that compensate for each other in the total AOD. For SSA, we find good agreement over land (RMSD = 0.030) for retrievals with AOD > 0.2. Over ocean the agreement is poor with a bias of 0.053 (where RemoTAP retrieves a higher SSA) and an RMSD of 0.074. As expected, the differences increase towards low AOD, over both land and ocean. For the AAOD, the agreement is reasonable over land and biased over ocean, where GRASP-CC retrieves higher AAOD than RemoTAP (as expected from the lower SSA). The surface BRDF products show reasonable agreement, where for the majority of cases RemoTAP retrieves a higher isotropic BRDF parameter than GRASP-CC. The differences in the BPDF are substantial, where RemoTAP retrieves a smaller BPDF scaling factor than GRASP-CC in most cases. The differences in AE are strongly related to differences in BPDF.
We also compared the GRASP-CC and RemoTAP AOD and AE products against MODIS. For AOD over land, the agreement of either GRASP-CC or RemoTAP with MODIS is worse than the agreement between the two PARASOL algorithms. Over ocean, the agreement is very similar among the three products for AOD. For AE, the difference between GRASP-CC and RemoTAP is much smaller than the difference between MODIS and both products, especially over land. This is expected because the PARASOL measurements have a larger information content on aerosol size than MODIS (e.g., Mishchenko and Travis, 1997a; Hasekamp and Landgraf, 2007).
To the best of our knowledge, the agreement between GRASP-CC and RemoTAP is unprecedented, which gives confidence in the quality of both RemoTAP and GRASP products. The agreement of the latest product versions with each other and with AERONET improved significantly compared to the previous version of the global products of GRASP (Chen et al., 2020) and RemoTAP (Lacagnina et al., 2017), and it surpasses that of widely used MODIS products (Chen et al., 2020). The results demonstrate that the dedicated effort in algorithm development for MAP aerosol retrievals still leads to substantial improvement in the resulting aerosol products, and this is still an ongoing process. In general, the comprehensive studies performed with GRASP-CC and RemoTAP on synthetic and real PARASOL measurements allowed the identification of weak and strong points of the algorithms, which are crucial for the further improvements in aerosol and surface characterization from space-borne remote sensing. There are still several aspects that need improvement. Away from the AERONET stations, differences between RemoTAP and GRASP-CC can be substantially larger, with systematic differences in a different direction than expected from the AERONET comparison. Most notably, over ocean GRASP-CC retrieves a smaller SSA than RemoTAP. Both GRASP-CC and RemoTAP show at some locations an unexpected sharp transition between land and ocean for SSA. For the Ångström exponent, both algorithms compare well for moderate and high AOD (>0.2). For low AOD, the retrieval of both AE and SSA becomes more challenging and needs improvement. For AOD, a relatively large bias between RemoTAP and GRASP-CC occurs over equatorial Africa. The issue of cloud screening has not been investigated in the present study. First of all, AERONET measurements have been cloud screened already, and hence a comparison between PARASOL and AERONET already involves an implicit cloud screening. Furthermore, because we have compared RemoTAP and GRASP-CC for common pixels, effectively the combination of both cloud-screening approaches has been used.
Possible points for improvements for MAP aerosol retrieval are improved cloud screening, quality filtering, and development of joint aerosol cloud retrievals (aerosol above cloud: Waquet et al., 2014; in between clouds: Hasekamp, 2010; Stap et al., 2016; and below cirrus: Lu et al., 2022). For future algorithm comparisons it will be useful to take into account formal retrieval uncertainties based on error propagation. Also, focused development is needed of higher-level data products, such as cloud condensation nuclei (CCN) (Hasekamp et al., 2019b), aerosol composition (van Diedenhoven et al., 2022; L. Li et al., 2022), PM2.5, and radiative fluxes. For validation, a better validation infrastructure is needed for “new” aerosol products like single scattering albedo (SSA), size distribution, and refractive index (chemical composition). AERONET validation of these products is restricted to high AOD cases (>0.4 at 440 nm), which will occur less in the coming decade because of expected reduced aerosol emissions. Also, more validation points for retrievals over ocean are needed. Finally, also for future MAP aerosol retrieval products, it is important to perform global comparisons between RemoTAP and GRASP-CC and preferably more algorithms.
A1 RemoTAP
The RemoTAP algorithm is based on the iterative fitting of a forward model to measurements of radiance and/or polarization of reflected light. The forward model consists of the LINTRAN2 radiative transfer model (Hasekamp and Landgraf, 2005; Hasekamp and Butz, 2008) and a model to compute optical aerosol properties from micro-physical properties using the Mie- and T-matrix-improved geometrical optics database by Dubovik et al. (2006) along with their proposed spheroid aspect ratio distribution for computing optical properties for a mixture of spheroids and spheres. For retrievals over land RemoTAP uses a surface reflection matrix (Litvinov et al., 2011) that accounts for the directional and polarization properties of the surface, based on the Ross–Li model (Wanner et al., 1995) total reflectance and the model of Maignan et al. (2009) for polarized reflectance. For a full description of the surface reflection model, which is the same for RemoTAP and GRASP, see Sect. B1. RemoTAP describes reflection by the ocean using the contribution of the Fresnel reflection matrix on the rough ocean (Cox and Munk, 1954; Zhang and Wang, 2010) and scattering and absorption inside the ocean body using a neural network trained with radiative transfer calculations in the ocean body (Fan et al., 2019). Additionally, a wavelength-dependent correction term is fitted. For more details see Appendix B2.
While earlier aerosol retrieval studies with RemoTAP used a bi-modal aerosol description (Hasekamp et al., 2011; Wu et al., 2015; Di Noia et al., 2017) or a five-mode aerosol description (Fu and Hasekamp, 2018; Fu et al., 2020), in this study we follow the approach of Lu et al. (2022) and describe the aerosol size distribution in RemoTAP by three log-normal modes, with one fine mode and two coarse modes (soluble and insoluble). The spectrally dependent refractive index m(λ) per mode is parameterized by
where mk(λ) is prescribed functions of wavelength, for which we use standard refractive index spectra for different aerosol components, i.e., dust (DU) (Torres et al., 2007), inorganic and sulfate (INORG) and black carbon (BC) (d'Almeida et al., 1991), and organic carbon (OC) (Kirchstetter et al., 2004).
For the fine mode the state vector includes reff; veff; Naer; fsph; and the refractive index coefficients αk that correspond to the standard refractive index spectra INORG, BC, and OC. The coarse insoluble mode consists of non-spherical dust. For this mode the state vector includes reff, Naer, and a coefficient for the imaginary part of the DU refractive index. The fixed parameters are fsph, veff, and αk for the DU real part of the refractive index. One value for zaer is included, which is assumed to be the same for modes 1 and 2. The width w0 of the altitude distribution is fixed. The third mode is a coarse soluble mode. For this mode the state vector includes reff, Naer, and coefficient αk of the INORG refractive index spectrum. The fixed parameters are fsph, veff, and zaer. An overview of the fit parameters is given in Table A1.
Table A1State vector elements, prior values, and parameter range for the parametric three-mode aerosol description. The superscripts ci and cs denote “coarse insoluble” and “coarse soluble”, respectively. The “fit” column indicates whether a parameter is fitted (fit = 1) or fixed (fit = 0) to the prior value. n/a: not applicable.
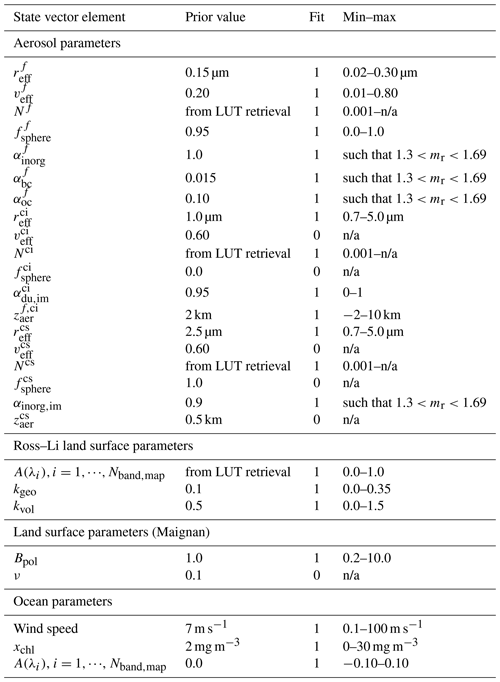
A2 GRASP
The Generalized Retrieval of Aerosol and Surface Properties is a new-generation algorithm developed for deriving extensive aerosol properties from diverse space-borne and ground-based instruments. The bigger the information content is in the remote sensing instrument, the higher the performance will be that the GRASP algorithm demonstrates. The overall concept of the algorithm is described by Dubovik et al. (2011) and Dubovik et al. (2021). The algorithm is based on a highly advanced statistically optimized fitting implemented as multi-term least-square minimization that had earlier been successfully implemented (Dubovik et al., 2002, 2006) for aerosol retrievals from ground-based AERONET radiometers. The GRASP aerosol model used for global processing in these studies is based on the chemical component approach (Li et al., 2019; J. Li et al., 2022) where aerosol is represented as external mixture of two different aerosol modes. Each aerosol mode itself is considered an internal mixture of different chemical species whose spectral complex refractive index is calculated through Maxwell Garnett (MG) effective approximation. The first mode is dominated mainly by small particles whose size is represented by three precalculated log-normal size distribution bins, and the complex refractive index is calculated from the mixture of predefined aerosol species: black and brown carbons, quartz, and ammonium nitrates. The second mode represents coarse aerosol particles with two precalculated log-normal size distribution bins and internal mixture of iron oxides, quartz, and ammonium nitrate species.
The retrieved parameters of the GRASP algorithm are presented in Table A2.
B1 Land surface reflection
Both RemoTAP and GRASP use the same model to describe the surface reflection matrix over land:
where D is the null matrix except D11=1. For the bidirectional reflection distribution function (BRDF) , the Ross–Li model modified for hot spot effect is used (Maignan et al., 2009). Moreover, following the studies performed in Litvinov et al. (2011), r11 is renormalized on the isotropic parameter to separate spectral and angular-dependent terms:
where fgeo and fvol are, respectively, the geometric (Li sparse) and volumetric (Ross thick, modified for hot spot effect) kernels (Wanner et al., 1995, and references therein). They are given by
Numerically, the absolute value of the right-hand side of Eq. (B6) can exceed 1. In this case we set cos t=1. The volumetric kernel represents the scattering within a dense vegetation canopy and is based on a radiative transfer approximation of single scattering due to small, uniformly distributed, and non-absorbing leaves. The angular behavior of this kernel is to have a minimum near the backscatter direction and bright limbs (Knobelspiesse et al., 2008). The geometric kernel represents surfaces with larger gaps between objects and thus accounts for self-shadowing. The angular behavior of this kernel is therefore to have a maximum at backscattering where there are no shadows. fgeo is based on the work of Wanner et al. (1995) and Li and Strahler (1992) but is used in the reciprocal form given in Lucht et al. (2000) for the case when the ratio of the height of the tree at the center of the crown to the vertical crown radius is and when the ratio of the vertical crown radius to the horizontal crown radius is (spherical or compact crowns) .
In Eq. (B1) Rpol is given by Maignan et al. (2009).
Here, Bpol is a scaling parameter (band-independent). Fp(m,Θ) is the Fresnel scattering matrix with refractive index m=1.5. We use ν= 0.1 (Litvinov et al., 2011).
B2 RemoTAP ocean reflection
For retrievals over ocean, RemoTAP describes the ocean reflection matrix as
where Rfrn is the contribution of the ocean surface, which is described by the Fresnel reflection matrix on a rough ocean surface, depending on the wind speed and direction to provide a Gaussian distribution of surface slopes (Cox and Munk, 1954). Rul is the ocean body (underlight) contribution. For the ocean body, we need a bio-optical model to compute optical properties of the ocean from bio-physical ocean parameters. We used the bio-optical model of Chowdhary et al. (2012) for case-1 waters (open ocean), which has the chlorophyll-a concentration xchl as the only bio-physical ocean parameter to compute the ocean optical properties (single scattering albedo, phase matrix). Using the hydrosol model of Chowdhary et al. (2012), the ocean is described as a mixture of seawater and a particulate component. The scattering and absorption coefficients of seawater are taken from Smith and Baker (1981), while the optical properties of the particulate components were calculated using detritus–plankton (D–P) mixtures. The particulates were assumed to be spherical, so the scattering phase matrix could be obtained using Mie calculations. The relative contribution of detritus and plankton are parameterized by Xchl. Here it should be noted that the underlight contribution is insensitive to the optical depth of ocean when the ocean optical thickness is larger than 10. In this study, we set the ocean optical depth to 20 and assumed a black ocean bottom surface. This ocean surface and body system was solved by a vector radiative transfer model (Hasekamp and Landgraf, 2002; Schepers et al., 2014). However, this model is computationally expensive because of the large ocean optical thickness. As an alternative, a neural network (NN) has been designed to simulate (Fourier coefficients of) the ocean body contribution to the reflection matrix just above the ocean surface, with the oceanic chlorophyll-a concentration as input. Finally, A(λ) in Eq. (B13) is a wavelength-dependent Lambertian albedo term that accounts for oceanic foam but may also correct for errors in Rul. Ws, Xchl, and A(λ) for the different spectral bands are included in the state vector.
B3 GRASP ocean reflection
The reflection matrix over water surfaces (Rs) in the GRASP algorithm used in these studies is represented as follows:
where Rfrn is the Fresnel reflection matrix from water surface facets with refractive index m, fshad is the shadowing function for the Gaussian random rough surface (Tsang et al., 1985; Mishchenko and Travis, 1997b), σ2 is the mean square facet slope related to wind speed (Cox and Munk, 1954), δfrn is fraction of water surface providing the Fresnel reflection matrix, and r0(λ) is the isotropic spectrally dependent water leaving reflectance. In the presented studies no a priori information about wind speed was used, and three parameters – σ2, δfrn, and r0(λ) – were retrieved with application of proper spatial and temporal constraints Table A2.
GRASP products are available at https://www.grasp-open.com/products/ (GRASP, 2023). RemoTAP products are available at https://public.spider.surfsara.nl/project/spexone/POLDER/GRIDDED/ (RemoTAP, 2023). The PARASOL level-1 data can be downloaded from https://www.icare.univ-lille.fr/data-access (ICARE, 2015). The AERONET data can be downloaded from https://aeronet.gsfc.nasa.gov/ (NASA and LOA-PHOTONS, 1993). MODIS products can be downloaded from https://www.icare.univ-lille.fr/data-access (ICARE, 2020).
OH, PL, and OD designed the research. GF, CC, OH, and PL performed the research. OH wrote the first draft, and all authors contributed to the final version through comments and writing of subsections.
At least one of the (co-)authors is a member of the editorial board of Atmospheric Measurement Techniques. The peer-review process was guided by an independent editor, and the authors also have no other competing interests to declare.
Publisher’s note: Copernicus Publications remains neutral with regard to jurisdictional claims made in the text, published maps, institutional affiliations, or any other geographical representation in this paper. While Copernicus Publications makes every effort to include appropriate place names, the final responsibility lies with the authors.
The research of this paper has been performed in the framework of the project HARPOL (Harmonizing and advancing retrieval approaches for present and future polarimetric space-borne atmospheric missions), funded through the ESA program EO Science for Society. We acknowledge Christian Retscher (ESA) for his work as technical officer for HARPOL. We also acknowledge Daniele Gasbarra (ESA) and Alexandru Dandocsi (ESA) for important feedback during the course of the HARPOL project.
This research has been supported by the European Space Agency (ESA contract no. 4000134035/21/I-DT-lr).
This paper was edited by Alexander Kokhanovsky and reviewed by six anonymous referees.
Andreae, M., Jones, C., and Cox, P.: Strong present-day aerosol cooling implies a hot future, Nature, 435, 1187, 2005. a
Bellouin, N., Quaas, J., Gryspeerdt, E., Kinne, S., Stier, P., Watson-Parris, D., Boucher, O., Carslaw, K. S., Christensen, M., Daniau, A.-L., Dufresne, J.-L., Feingold, G., Fiedler, S., Forster, P., Gettelman, A., Haywood, J. M., Lohmann, U., Malavelle, F., Mauritsen, T., McCoy, D. T., Myhre, G., Mülmenstädt, J., Neubauer, D., Possner, A., Rugenstein, M., Sato, Y., Schulz, M., Schwartz, S. E., Sourdeval, O., Storelvmo, T., Toll, V., Winker, D., and Stevens, B.: Bounding global aerosol radiative forcing of climate change, Rev. Geophys., 58, e2019RG000660, https://doi.org/10.1029/2019RG000660, 2020. a
Butz, A., Hasekamp, O. P., Frankenberg, C., and Aben, I.: Retrievals of atmospheric CO_2 from simulated space-borne measurements of backscattered near-infrared sunlight: accounting for aerosol effects, Appl. Opt., 48, 3322, https://doi.org/10.1364/AO.48.003322, 2009. a
Butz, A., Guerlet, S., Hasekamp, O., Schepers, D., Galli, A., Aben, I., Frankenberg, C., Hartmann, J.-M., Tran, H., Kuze, A., Keppel-Aleks, G., Toon, G., Wunch, D., Wennberg, P., Deutscher, N., Griffith, D., Macatangay, R., Messerschmidt, J., Notholt, J., and Warneke, T.: Toward accurate CO2 and CH4 observations from GOSAT, Geophys. Res. Lett., 38, L14812, https://doi.org/10.1029/2011GL047888, 2011. a
Chen, C., Dubovik, O., Henze, D. K., Lapyonak, T., Chin, M., Ducos, F., Litvinov, P., Huang, X., and Li, L.: Retrieval of desert dust and carbonaceous aerosol emissions over Africa from POLDER/PARASOL products generated by the GRASP algorithm, Atmos. Chem. Phys., 18, 12551–12580, https://doi.org/10.5194/acp-18-12551-2018, 2018. a
Chen, C., Dubovik, O., Henze, D. K., Chin, M., Lapyonok, T., Schuster, G. L., Ducos, F., Fuertes, D., Litvinov, P., Li, L., Lopatin, A., Hu, Q., and Torres, B.: Constraining global aerosol emissions using POLDER/PARASOL satellite remote sensing observations, Atmos. Chem. Phys., 19, 14585–14606, https://doi.org/10.5194/acp-19-14585-2019, 2019. a
Chen, C., Dubovik, O., Fuertes, D., Litvinov, P., Lapyonok, T., Lopatin, A., Ducos, F., Derimian, Y., Herman, M., Tanré, D., Remer, L. A., Lyapustin, A., Sayer, A. M., Levy, R. C., Hsu, N. C., Descloitres, J., Li, L., Torres, B., Karol, Y., Herrera, M., Herreras, M., Aspetsberger, M., Wanzenboeck, M., Bindreiter, L., Marth, D., Hangler, A., and Federspiel, C.: Validation of GRASP algorithm product from POLDER/PARASOL data and assessment of multi-angular polarimetry potential for aerosol monitoring, Earth Syst. Sci. Data, 12, 3573–3620, https://doi.org/10.5194/essd-12-3573-2020, 2020. a, b, c, d, e, f, g, h, i, j, k, l, m, n
Chen, C., Dubovik, O., Litvinov, P., Fuertes, D., Lopatin, A., Lapyonok, T., Matar, C., Karol, Y., Fischer, J., Preusker, R., Hangler, A., Aspetsberger, M., Bindreiter, L., Marth, D., Chimot, J., Fougnie, B., Marbach, T., and Bojkov, B.: Properties of aerosol and surface derived from OLCI/Sentinel-3A using GRASP approach: Retrieval development and preliminary validation, Remote Sens. Environ., 280, 113142, https://doi.org/10.1016/j.rse.2022.113142, 2022a. a
Chen, C., Dubovik, O., Schuster, G. L., Chin, M., Henze, D. K., Lapyonok, T., Li, Z., Derimian, Y., and Zhang, Y.: Multi-angular polarimetric remote sensing to pinpoint global aerosol absorption and direct radiative forcing, Nat. Commun., 13, 7459, https://doi.org/10.1038/s41467-022-35147-y, 2022b. a
Chowdhary, J., Cairns, B., Waquet, F., Knobelspiesse, K., Ottaviani, M., Redemann, J., Travis, L., and Mishchenko, M.: Sensitivity of multiangle, multispectral polarimetric remote sensing over open oceans to water-leaving radiance: Analyses of RSP data acquired during the MILAGRO campaign, Remote Sens. Environ., 118, 284–308, https://doi.org/10.1016/j.rse.2011.11.003, 2012. a, b
Cox, C. and Munk, W.: Statistics of the sea surface derived from sun glitter, J. Mar. Res., 13, 198–227, 1954. a, b, c
d'Almeida, G., Koepke, P., and Shettle, E.: Atmospheric Aerosols: Global Climatology and Radiative Characteristics, A. Deepak Publishing, Hampton, VA, ISA, 1991. a
Deuzé, J. L., Bréon, F. M., Devaux, C., Goloub, P., Herman, M., Lafrance, B., Maignan, F., Marchand, A., Nadal, F., Perry, G., and Tanré, D.: Remote sensing of aerosols over land surfaces from POLDER-ADEOS-1 polarized measurements, J. Geophys. Res., 106, 4913–4926, 2001. a
Di Noia, A., Hasekamp, O. P., Wu, L., van Diedenhoven, B., Cairns, B., and Yorks, J. E.: Combined neural network/Phillips–Tikhonov approach to aerosol retrievals over land from the NASA Research Scanning Polarimeter, Atmos. Meas. Tech., 10, 4235–4252, https://doi.org/10.5194/amt-10-4235-2017, 2017. a
Dubovik, O., Smirnov, A., Holben, B. N., King, M. D., Kaufman, Y. J., Eck, T. F., and Slutsker, I.: Accuracy assessments of aerosol optical properties retrieved from Aerosol Robotic Network (AERONET) Sun and sky radiance measurements, J. Geophys. Res.-Atmos., 105, 9791–9806, https://doi.org/10.1029/2000JD900040, 2000. a
Dubovik, O., Holben, B., Eck, T. F., Smirnov, A., Kaufman, Y. J., King, M. D., Tanré, D., and Slutsker, I.: Variability of Absorption and Optical Properties of Key Aerosol Types Observed in Worldwide Locations, J. Atmos. Sci., 59, 590–608, https://doi.org/10.1175/1520-0469(2002)059<0590:VOAAOP>2.0.CO;2, 2002. a
Dubovik, O., Sinyuk, A., Lapyonok, T., Holben, B. N., Mishchenko, M., Yang, P., Eck, T. F., Volten, H., Muñoz, O., Veihelmann, B., van der Zande, W. J., Leon, J., Sorokin, M., and Slutsker, I.: Application of spheroid models to account for aerosol particle nonsphericity in remote sensing of desert dust, J. Geophys. Res.-Atmos., 111, D11208, https://doi.org/10.1029/2005JD006619, 2006. a, b, c
Dubovik, O., Herman, M., Holdak, A., Lapyonok, T., Tanré, D., Deuzé, J. L., Ducos, F., Sinyuk, A., and Lopatin, A.: Statistically optimized inversion algorithm for enhanced retrieval of aerosol properties from spectral multi-angle polarimetric satellite observations, Atmos. Meas. Tech., 4, 975–1018, https://doi.org/10.5194/amt-4-975-2011, 2011. a, b, c, d
Dubovik, O., Lapyonok, T., Litvinov, P., Herman, M., Fuertes, D., Ducos, F., Lopatin, A., Chaikovsky, A., Torres, B., Derimian, Y., Huang, X., Aspetsberger, M., and Federspiel, C.: GRASP: a versatile algorithm for characterizing the atmosphere, SPIE Newsroom, https://doi.org/10.1117/2.1201408.005558, 2014. a, b, c
Dubovik, O., Li, Z., Mishchenko, M. I., Tanré, D., Karol, Y., Bojkov, B., Cairns, B., Diner, D. J., Espinosa, W. R., Goloub, P., Gu, X., Hasekamp, O., Hong, J., Hou, W., Knobelspiesse, K. D., Landgraf, J., Li, L., Litvinov, P., Liu, Y., Lopatin, A., Marbach, T., Maring, H., Martins, V., Meijer, Y., Milinevsky, G., Mukai, S., Parol, F., Qiao, Y., Remer, L., Rietjens, J., Sano, I., Stammes, P., Stamnes, S., Sun, X., Tabary, P., Travis, L. D., Waquet, F., Xu, F., Yan, C., and Yin, D.: Polarimetric remote sensing of atmospheric aerosols: instruments, methodologies, results, and perspectives, J. Quant. Spectrosc. Ra., 224, 474–511, https://doi.org/10.1016/j.jqsrt.2018.11.024, 2019. a, b
Dubovik, O., Fuertes, D., Litvinov, P., Lopatin, A., Lapyonok, T., Doubovik, I., Xu, F., Ducos, F., Chen, C., Torres, B., Derimian, Y., Li, L., Herreras-Giralda, M., Herrera, M., Karol, Y., Matar, C., Schuster, G. L., Espinosa, R., Puthukkudy, A., Li, Z., Fischer, J., Preusker, R., Cuesta, J., Kreuter, A., Cede, A., Aspetsberger, M., Marth, D., Bindreiter, L., Hangler, A., Lanzinger, V., Holter, C., and Federspiel, C.: A Comprehensive Description of Multi-Term LSM for Applying Multiple a Priori Constraints in Problems of Atmospheric Remote Sensing: GRASP Algorithm, Concept, and Applications, Front. Remote Sens., 2, 706851, https://doi.org/10.3389/frsen.2021.706851, 2021. a, b, c, d, e, f
Dusek, U., Frank, G. P., Hildebrandt, L., Curtius, J., Schneider, J., Walter, S., Chand, D., Drewnick, F., Hings, S., Jung, D., Borrmann, S., and Andreae, M. O.: Size Matters More Than Chemistry for Cloud-Nucleating Ability of Aerosol Particles, Science, 312, 1375–1378, https://doi.org/10.1126/science.1125261, 2006. a
Fan, C., Fu, G., Di Noia, A., Smit, M., H. H. Rietjens, J., A. Ferrare, R., Burton, S., Li, Z., and P. Hasekamp, O.: Use of A Neural Network-Based Ocean Body Radiative Transfer Model for Aerosol Retrievals from Multi-Angle Polarimetric Measurements, Remote Sens., 11, 2877, https://doi.org/10.3390/rs11232877, 2019. a, b, c
Forster, P., Storelvmo, T., Armour, K., Collins, W., Dufresne, J. -L., Frame, D., Lunt, D., Mauritsen, T., Palmer, M., Watanabe, M., Wild, M., and Zhang, H.: The Earth’s Energy Budget, Climate Feedbacks, and Climate Sensitivity, 923–1054, Cambridge University Press, Cambridge, United Kingdom and New York, NY, USA, https://doi.org/10.1017/9781009157896.009, 2021. a, b
Fougnie, B., Bracco, G., Lafrance, B., Ruffel, C., Hagolle, O., and Tinel, C.: PARASOL in-flight calibration and performance, Appl. Opt., 46, 5435–5451, https://doi.org/10.1364/AO.46.005435, 2007. a, b
Fougnie, B., Marbach, T., Lacan, A., Lang, R., Schlüssel, P., Poli, G., Munro, R., and Couto, A. B.: The multi-viewing multi-channel multi-polarisation imager – Overview of the 3MI polarimetric mission for aerosol and cloud characterization, J. Quant. Spectrosc. Ra., 219, 23–32, https://doi.org/10.1016/j.jqsrt.2018.07.008, 2018. a
Fu, G. and Hasekamp, O.: Retrieval of aerosol microphysical and optical properties over land using a multimode approach, Atmos. Meas. Tech., 11, 6627–6650, https://doi.org/10.5194/amt-11-6627-2018, 2018. a, b, c
Fu, G., Hasekamp, O., Rietjens, J., Smit, M., Di Noia, A., Cairns, B., Wasilewski, A., Diner, D., Seidel, F., Xu, F., Knobelspiesse, K., Gao, M., da Silva, A., Burton, S., Hostetler, C., Hair, J., and Ferrare, R.: Aerosol retrievals from different polarimeters during the ACEPOL campaign using a common retrieval algorithm, Atmos. Meas. Tech., 13, 553–573, https://doi.org/10.5194/amt-13-553-2020, 2020. a, b, c
Gao, M., Franz, B. A., Knobelspiesse, K., Zhai, P.-W., Martins, V., Burton, S., Cairns, B., Ferrare, R., Gales, J., Hasekamp, O., Hu, Y., Ibrahim, A., McBride, B., Puthukkudy, A., Werdell, P. J., and Xu, X.: Efficient multi-angle polarimetric inversion of aerosols and ocean color powered by a deep neural network forward model, Atmos. Meas. Tech., 14, 4083–4110, https://doi.org/10.5194/amt-14-4083-2021, 2021a. a
Gao, M., Knobelspiesse, K., Franz, B. A., Zhai, P.-W., Martins, V., Burton, S. P., Cairns, B., Ferrare, R., Fenn, M. A., Hasekamp, O., Hu, Y., Ibrahim, A., Sayer, A. M., Werdell, P. J., and Xu, X.: Adaptive Data Screening for Multi-Angle Polarimetric Aerosol and Ocean Color Remote Sensing Accelerated by Deep Learning, Front. Remote Sens., 2, ISSN 2673-6187, https://doi.org/10.3389/frsen.2021.757832, 2021b. a
Gao, M., Knobelspiesse, K., Franz, B. A., Zhai, P.-W., Sayer, A. M., Ibrahim, A., Cairns, B., Hasekamp, O., Hu, Y., Martins, V., Werdell, P. J., and Xu, X.: Effective uncertainty quantification for multi-angle polarimetric aerosol remote sensing over ocean, Atmos. Meas. Tech., 15, 4859–4879, https://doi.org/10.5194/amt-15-4859-2022, 2022. a
Gao, M., Franz, B. A., Zhai, P.-W., Knobelspiesse, K., Sayer, A., Xu, X., Martins, V., Cairns, B., Castellanos, P., Fu, G., Hannadige, N., Hasekamp, O., Hu, Y., Ibrahim, A., Patt, F., Puthukkudy, A., and Werdell, P. J.: Simultaneous retrieval of aerosol and ocean properties from PACE HARP2 with uncertainty assessment using cascading neural network radiative transfer models, EGUsphere [preprint], https://doi.org/10.5194/egusphere-2023-1843, 2023. a
GRASP: GRASP Chemical Component product, GRASP [data set], https://www.grasp-open.com/products/ (last access: 26 February 2024), 2023. a
Gryspeerdt, E., Mülmenstädt, J., Gettelman, A., Malavelle, F. F., Morrison, H., Neubauer, D., Partridge, D. G., Stier, P., Takemura, T., Wang, H., Wang, M., and Zhang, K.: Surprising similarities in model and observational aerosol radiative forcing estimates, Atmos. Chem. Phys., 20, 613–623, https://doi.org/10.5194/acp-20-613-2020, 2020. a
Hasekamp, O. P.: Capability of multi-viewing-angle photo-polarimetric measurements for the simultaneous retrieval of aerosol and cloud properties, Atmos. Meas. Tech., 3, 839–851, https://doi.org/10.5194/amt-3-839-2010, 2010. a
Hasekamp, O. P. and Butz, A.: Efficient calculation of intensity and polarization spectra in vertically inhomogeneous scattering and absorbing atmospheres, J. Geophys. Res.-Atmos., 113, D20309, https://doi.org/10.1029/2008JD010379, 2008. a, b
Hasekamp, O. and Landgraf, J.: A linearized vector radiative transfer model for atmospheric trace gas retrieval, J. Quant. Spectrosc. Ra., 75, 221–238, 2002. a, b
Hasekamp, O. P. and Landgraf, J.: Linearization of vector radiative transfer with respect to aerosol properties and its use in satellite remote sensing, J. Geophys. Res.-Atmos., 110, D04203, 2005. a, b
Hasekamp, O. P. and Landgraf, J.: Retrieval of aerosol properties over land surfaces: capabilities of multiple-viewing-angle intensity and polarization measurements, Appl. Opt., 46, 3332–3344, https://doi.org/10.1364/AO.46.003332, 2007. a
Hasekamp, O. P., Litvinov, P., and Butz, A.: Aerosol properties over the ocean from PARASOL multiangle photopolarimetric measurements, J. Geophys. Res., 116, D14204, https://doi.org/10.1029/2010jd015469, 2011. a, b, c
Hasekamp, O. P., Fu, G., Rusli, S. P., Wu, L., Noia, A. D., aan de Brugh, J., Landgraf, J., Smit, J. M., Rietjens, J., and van Amerongen, A.: Aerosol measurements by SPEXone on the NASA PACE mission: expected retrieval capabilities, J. Quant. Spectrosc. Ra., 227, 170–184, https://doi.org/10.1016/j.jqsrt.2019.02.006, 2019a. a, b, c
Hasekamp, O. P., Gryspeerdt, E., and Quaas, J.: Analysis of polarimetric satellite measurements suggests stronger cooling due to aerosol-cloud interactions, Nat. Commun., 10, 1–11, https://doi.org/10.1038/s41467-019-13372-2, 2019b. a, b
Herman, M., Deuzé, J. L., Devaux, C., Goloub, P., Bréon, F. M., and Tanré, D.: Remote sensing of aerosols over land surfaces, including polarisation measurements: Application to POLDER Measurements, J. Geophys. Res., 102, 17039–17049, 1997. a
Herreras-Giralda, M., Litvinov, P., Dubovik, O., Derimian, Y., Lapyonok, T., Fuertes, D., Sourdeval, O., Preusker, R., and Fischer, J.: Thermal emission in the successive orders of scattering (SOS) radiative transfer approach, J. Quant. Spectrosc. Ra., 291, 108327, https://doi.org/10.1016/j.jqsrt.2022.108327, 2022. a
Hsu, N., Jeong, M.-J., Bettenhausen, C., Sayer, A., Hansell, R., Seftor, C., Huang, J., and Tsay, S.-C.: Enhanced Deep Blue aerosol retrieval algorithm: The second generation, J. Geophys. Res.-Atmos., 118, 9296–9315, https://doi.org/10.1002/jgrd.50712, 2013. a, b
ICARE: PARASOL L1_B.C3 Product, ICAR [data set], https://www.icare.univ-lille.fr/asd-content/archive/?dir=PARASOL/L1_B.C3/ (last access: 26 February 2024), 2015. a
ICARE: MODIS MYD04_L2 product, ICAR [data set], https://www.icare.univ-lille.fr/asd-content/archive/?dir=MODIS/MYD04_L2/ (last access: 26 February 2024), 2020. a
Kirchstetter, T. W., Novakov, T., and Hobbs, P. V.: Evidence that the spectral dependence of light absorption by aerosols is affected by organic carbon, J. Geophys. Res.-Atmos., 109, D21208, https://doi.org/10.1029/2004JD004999, 2004. a
Knobelspiesse, K. D., Cairns, B., Schmid, B., Román, M. O., and Schaaf, C. B.: Surface BRDF estimation from an aircraft compared to MODIS and ground estimates at the Southern Great Plains site, J. Geophys. Res.-Atmos., 113, D20105, https://doi.org/10.1029/2008JD010062, 2008. a
Lacagnina, C., Hasekamp, O. P., Bian, H., Curci, G., Myhre, G., van Noije, T., Schulz, M., Skeie, R. B., Takemura, T., and Zhang, K.: Aerosol single-scattering albedo over the global oceans: Comparing PARASOL retrievals with AERONET, OMI, and AeroCom models estimates, J. Geophys. Res.-Atmos., 120, 9814–9836, https://doi.org/10.1002/2015jd023501, 2016. a
Lacagnina, C., Hasekamp, O. P., and Torres, O.: Direct radiative effect of aerosols based on PARASOL and OMI satellite observations, J. Geophys. Res.-Atmos., 122, 2366–2388, https://doi.org/10.1002/2016jd025706, 2017. a, b, c, d, e, f, g
Landgraf, J., Hasekamp, O., and Trautmann, T.: Linearization of radiative transfer with respect to surface properties, J. Quant. Spectrosc. Ra., 72, 327–339, 2002. a
Lenoble, J., Herman, M., Deuze, J.-L., Lafrance, B., Santer, R., and Tanre, D.: A successive order of scattering code for solving the vector equation of transfer in the earth's atmosphere with aerosols, J. Quant. Spectrosc. Ra., 107, 479–507, 2007. a
Levy, R. C., Mattoo, S., Munchak, L. A., Remer, L. A., Sayer, A. M., Patadia, F., and Hsu, N. C.: The Collection 6 MODIS aerosol products over land and ocean, Atmos. Meas. Tech., 6, 2989–3034, https://doi.org/10.5194/amt-6-2989-2013, 2013. a, b
Li, J., Carlson, B. E., Yung, Y. L., Lv, D., Hansen, J., Penner, J. E., Liao, H., Ramaswamy, V., Kahn, R. A., Zhang, P., Dubovik, O., Ding, A., Lacis, A. A., Zhang, L., and Dong, Y.: Scattering and absorbing aerosols in the climate system, Nat. Rev. Earth Environ., https://doi.org/10.1038/s43017-022-00296-7, early Online, 2022. a, b
Li, L., Dubovik, O., Derimian, Y., Schuster, G. L., Lapyonok, T., Litvinov, P., Ducos, F., Fuertes, D., Chen, C., Li, Z., Lopatin, A., Torres, B., and Che, H.: Retrieval of aerosol components directly from satellite and ground-based measurements, Atmos. Chem. Phys., 19, 13409–13443, https://doi.org/10.5194/acp-19-13409-2019, 2019. a, b, c
Li, L., Derimian, Y., Chen, C., Zhang, X., Che, H., Schuster, G. L., Fuertes, D., Litvinov, P., Lapyonok, T., Lopatin, A., Matar, C., Ducos, F., Karol, Y., Torres, B., Gui, K., Zheng, Y., Liang, Y., Lei, Y., Zhu, J., Zhang, L., Zhong, J., Zhang, X., and Dubovik, O.: Climatology of aerosol component concentrations derived from multi-angular polarimetric POLDER-3 observations using GRASP algorithm, Earth Syst. Sci. Data, 14, 3439–3469, https://doi.org/10.5194/essd-14-3439-2022, 2022. a, b
Li, X. and Strahler, A. H.: Geometric-optical bidirectional reflectance modeling of the discrete crown vegetation canopy: effect of crown shape and mutual shadowing, IEEE Trans. Geosci. Remote Sens., 30, 276–292, 1992. a
Litvinov, P., Hasekamp, O., and Cairns, B.: Models for surface reflection of radiance and polarized radiance: Comparison with airborne multi-angle photopolarimetric measurements and implications for modeling top-of-atmosphere measurements, Remote Sens. Environ., 115, 781–792, https://doi.org/10.1016/j.rse.2010.11.005, 2011. a, b, c, d, e
Lopatin, A., Dubovik, O., Chaikovsky, A., Goloub, P., Lapyonok, T., Tanré, D., and Litvinov, P.: Enhancement of aerosol characterization using synergy of lidar and sun-photometer coincident observations: the GARRLiC algorithm, Atmos. Meas. Tech., 6, 2065–2088, https://doi.org/10.5194/amt-6-2065-2013, 2013. a
Lopatin, A., Dubovik, O., Fuertes, D., Stenchikov, G., Lapyonok, T., Veselovskii, I., Wienhold, F. G., Shevchenko, I., Hu, Q., and Parajuli, S.: Synergy processing of diverse ground-based remote sensing and in situ data using the GRASP algorithm: applications to radiometer, lidar and radiosonde observations, Atmos. Meas. Tech., 14, 2575–2614, https://doi.org/10.5194/amt-14-2575-2021, 2021. a
Lu, S., Landgraf, J., Fu, G., van Diedenhoven, B., Wu, L., Rusli, S. P., and Hasekamp, O. P.: Simultaneous Retrieval of Trace Gases, Aerosols, and Cirrus Using RemoTAP – The Global Orbit Ensemble Study for the CO2M Mission, Front. Remote Sens., 3, ISSN 2673-6187, https://doi.org/10.3389/frsen.2022.914378, 2022. a, b, c, d, e, f
Lucht, W., Hyman, A., Strahler, A., Barnsley, M., Hobson, P., and Muller, J.-P.: A Comparison of Satellite-Derived Spectral Albedos to Ground-Based Broadband Albedo Measurements Modeled to Satellite Spatial Scale for a Semidesert Landscape, Remote Sens. Environ., 74, 85–98, https://doi.org/10.1016/S0034-4257(00)00125-5, 2000. a
Maignan, F., Bréon, F.-M., Fédèle, E., and Bouvier, M.: Polarized reflectances of natural surfaces: Spaceborne measurements and analytical modeling, Remote Sens. Environ., 113, 2642–2650, https://doi.org/10.1016/j.rse.2009.07.022, 2009. a, b, c
Mishchenko, M. I. and Travis, L. D.: Satellite retrieval of aerosol properties over the ocean using polarization as well as intensity of reflected sunlight, J. Geophys. Res., 102, 16989–17013, 1997a. a
Mishchenko, M. I. and Travis, L. D.: Satellite retrieval of aerosol properties over the ocean using measurements of reflected sunlight, J. Geophys. Res., 102, 13543–13553, 1997b. a
NASA and LOA-PHOTONS: AERONET, NASA [data set], https://aeronet.gsfc.nasa.gov/ (last access: 24 February 2024), 1993. a
Popp, T., De Leeuw, G., Bingen, C., Brühl, C., Capelle, V., Chedin, A., Clarisse, L., Dubovik, O., Grainger, R., Griesfeller, J., Heckel, A., Kinne, S., Klüser, L., Kosmale, M., Kolmonen, P., Lelli, L., Litvinov, P., Mei, L., North, P., Pinnock, S., Povey, A., Robert, C., Schulz, M., Sogacheva, L., Stebel, K., Stein Zweers, D., Thomas, G., Tilstra, L. G., Vandenbussche, S., Veefkind, P., Vountas, M., and Xue, Y.: Development, Production and Evaluation of Aerosol Climate Data Records from European Satellite Observations (Aerosol cci), Remote Sens., 8, 421, https://doi.org/10.3390/rs8050421, 2016. a, b
Quaas, J., Arola, A., Cairns, B., Christensen, M., Deneke, H., Ekman, A. M. L., Feingold, G., Fridlind, A., Gryspeerdt, E., Hasekamp, O., Li, Z., Lipponen, A., Ma, P.-L., Mülmenstädt, J., Nenes, A., Penner, J. E., Rosenfeld, D., Schrödner, R., Sinclair, K., Sourdeval, O., Stier, P., Tesche, M., van Diedenhoven, B., and Wendisch, M.: Constraining the Twomey effect from satellite observations: issues and perspectives, Atmos. Chem. Phys., 20, 15079–15099, https://doi.org/10.5194/acp-20-15079-2020, 2020. a
Reid, J. S., Gumber, A., Zhang, J., Holz, R. E., Rubin, J. I., Xian, P., Smirnov, A., Eck, T. F., O’Neill, N. T., Levy, R. C., Reid, E. A., Colarco, P. R., Benedetti, A., and Tanaka, T.: A Coupled Evaluation of Operational MODIS and Model Aerosol Products for Maritime Environments Using Sun Photometry: Evaluation of the Fine and Coarse Mode, Remote Sens., 14, 2978, https://doi.org/10.3390/rs14132978, 2022. a
RemoTAP: RemoTAP PARASOL product, surfsara [data set], https://public.spider.surfsara.nl/project/spexone/POLDER/GRIDDED/ (last access: 24 February 2024), 2023. a
Schepers, D., aan de Brugh, J., Hahne, P., Butz, A., Hasekamp, O., and Landgraf, J.: LINTRAN v2.0: A linearised vector radiative transfer model for efficient simulation of satellite-born nadir-viewing reflection measurements of cloudy atmospheres, J. Quant. Spectrosc. Ra., 149, 347–359, https://doi.org/10.1016/j.jqsrt.2014.08.019, 2014. a, b
Schutgens, N., Dubovik, O., Hasekamp, O., Torres, O., Jethva, H., Leonard, P. J. T., Litvinov, P., Redemann, J., Shinozuka, Y., de Leeuw, G., Kinne, S., Popp, T., Schulz, M., and Stier, P.: AEROCOM and AEROSAT AAOD and SSA study – Part 1: Evaluation and intercomparison of satellite measurements, Atmos. Chem. Phys., 21, 6895–6917, https://doi.org/10.5194/acp-21-6895-2021, 2021. a
Sierk, B., Bézy, J.-L., Löscher, A., and Meijer, Y.: The European CO2 Monitoring Mission: observing anthropogenic greenhouse gas emissions from space, in: International Conference on Space Optics, Event: International Conference on Space Optics – ICSO 2018, 2018, Chania, Greece, https://doi.org/10.1117/12.2535941, 2019. a
Smith, R. and Baker, K.: Optical properties of the clearest natural waters, Appl. Opt., 20, 177–184, 1981. a
Snik, F., Craven-Jones, J., Escuti, M., Fineschi, S., Harrington, D., Martino, A. D., Mawet, D., Riedi, J., and Tyo, J. S.: An overview of polarimetric sensing techniques and technology with applications to different research fields, Proc. SPIE, 9099, Event: SPIE Sensing Technology + Applications, 2014, Baltimore, MD, United States, https://doi.org/10.1117/12.2053245, 2014. a, b
Stamnes, S., Hostetler, C., Ferrare, R., Burton, S., Liu, X., Hair, J., Hu, Y., Wasilewski, A., Martin, W., van Diedenhoven, B., Chowdhary, J., Cetinic, I., Berg, L., Stamnes, K., and Cairns, B.: Simultaneous polarimeter retrievals of microphysical aerosol and ocean color parameters from the “MAPP” algorithm with comparison to high spectral resolution lidar aerosol and ocean products, Appl. Opt., 57, 2394–2413, https://doi.org/10.1364/AO.57.002394, 2018. a
Stap, F. A., Hasekamp, O. P., and Röckmann, T.: Sensitivity of PARASOL multi-angle photopolarimetric aerosol retrievals to cloud contamination, Atmos. Meas. Tech., 8, 1287–1301, https://doi.org/10.5194/amt-8-1287-2015, 2015. a
Stap, F. A., Hasekamp, O. P., Emde, C., and Röckmann, T.: Multiangle photopolarimetric aerosol retrievals in the vicinity of clouds: Synthetic study based on a large eddy simulation, J. Geophys. Res.-Atmos., 121, 12914–12935, https://doi.org/10.1002/2016JD024787, 2016. a
Torres, O., Tanskanen, A., Veihelman, B., Ahn, C., Braak, R., Bhartia, P. K., Veefkind, P., and Levelt, P.: Aerosols and surface UV products from OMI Observations: an overview, J. Geophys. Res., 112, D24S47, https://doi.org/10.1029/2007JD008809, 2007. a
Tsang, L., Kong, J. A., and Shin, R. T.: Theory of Microwave Remote Sensing, Wiley, New York, 1985. a
Tsikerdekis, A., Schutgens, N. A. J., and Hasekamp, O. P.: Assimilating aerosol optical properties related to size and absorption from POLDER/PARASOL with an ensemble data assimilation system, Atmos. Chem. Phys., 21, 2637–2674, https://doi.org/10.5194/acp-21-2637-2021, 2021. a
Tsikerdekis, A., Hasekamp, O. P., Schutgens, N. A. J., and Zhong, Q.: Assimilation of POLDER observations to estimate aerosol emissions, Atmos. Chem. Phys., 23, 9495–9524, https://doi.org/10.5194/acp-23-9495-2023, 2023. a, b
Twomey, S.: Pollution and the planetary albedo, Atmos. Environ. (1967), 8, 1251–1256, https://doi.org/10.1016/0004-6981(74)90004-3, 1974. a
van Diedenhoven, B., Hasekamp, O. P., Cairns, B., Schuster, G. L., Stamnes, S., Shook, M., and Ziemba, L.: Remote sensing of aerosol water fraction, dry size distribution and soluble fraction using multi-angle, multi-spectral polarimetry, Atmos. Meas. Tech., 15, 7411–7434, https://doi.org/10.5194/amt-15-7411-2022, 2022. a
Wanner, W., Li, X., and Strahler, A. H.: On the derivation of kernels for kernel-driven models of bidirectional reflectance, J. Geophys. Res.-Atmos., 100, 21077–21089, https://doi.org/10.1029/95JD02371, 1995. a, b, c
Waquet, F. and Herman, M.: The truncation problem, J. Quant. Spectrosc. Ra., 229, 80–91, 2019. a
Waquet, F., Peers, F., Ducos, F., Goloub, P., Platnick, S., Riedi, J., Tanré, D., and Thieuleux, F.: Global analysis of aerosol properties above clouds, Geophys. Res. Lett., 40, 5809–5814, https://doi.org/10.1002/2013GL057482, 2014. a
Werdell, P. J., Behrenfeld, M. J., Bontempi, P. S., Boss, E., Cairns, B., Davis, G. T., Franz, B. A., Gliese, U. B., Gorman, E. T., Hasekamp, O., Knobelspiesse, K. D., Mannino, A., Martins, J. V., McClain, C. R., Meister, G., and Remer, L. A.: The Plankton, Aerosol, Cloud, Ocean Ecosystem Mission: Status, Science, Advances, B. Am. Meteorol. Soc., 100, 1775–1794, https://doi.org/10.1175/BAMS-D-18-0056.1, 2019. a
Wu, L., Hasekamp, O., van Diedenhoven, B., and Cairns, B.: Aerosol retrieval from multiangle, multispectral photopolarimetric measurements: importance of spectral range and angular resolution, Atmos. Meas. Tech., 8, 2625–2638, https://doi.org/10.5194/amt-8-2625-2015, 2015. a, b
Wu, L., Hasekamp, O., van Diedenhoven, B., Cairns, B., Yorks, J. E., and Chowdhary, J.: Passive remote sensing of aerosol layer height using near-UV multi-angle polarization measurements, Geophys. Res. Lett., 43, 8783–8790, https://doi.org/10.1002/2016GL069848, 2016. a
Xu, F., van Harten, G., Diner, D. J., Kalashnikova, O. V., Seidel, F. C., Bruegge, C. J., and Dubovik, O.: Coupled retrieval of aerosol properties and land surface reflection using the Airborne Multiangle SpectroPolarimetric Imager, J. Geophys. Res.-Atmos., 122, 7004–7026, https://doi.org/10.1002/2017JD026776, 2017. a
Xu, F., Harten, G., Diner, D. J., Davis, A. B., Seidel, F. C., Rheingans, B., Tosca, M., Alexandrov, M. D., Cairns, B., Ferrare, R. A., Burton, S. P., Fenn, M. A., Hostetler, C. A., Wood, R., and Redemann, J.: Coupled Retrieval of Liquid Water Cloud and Above-Cloud Aerosol Properties Using the Airborne Multiangle SpectroPolarimetric Imager (AirMSPI), J. Geophys. Res.-Atmos., 123, 3175–3204, https://doi.org/10.1002/2017JD027926, 2018. a
Zeng, S., Parol, F., Riedi, J., Cornet, C., and Thieuleux, F.: Examination of POLDER/PARASOL and MODIS/Aqua cloud fractions and properties representativeness, B. Am. Meteorol. Soc., 24, 4435–4450, https://doi.org/10.1175/2011JCLI3857.1, 2011. a
Zhang, H. and Wang, M.: Evaluation of sun glint models using MODIS measurements, J. Quant. Spectrosc. Ra., 111, 492–506, https://doi.org/10.1016/j.jqsrt.2009.10.001, 2010. a
Zhang, X., Li, L., Chen, C., Chen, X., Dubovik, O., Derimian, Y., Gui, K., Zheng, Y., Zhao, H., Zhang, L., Guo, B., Wang, Y., Holben, B., Che, H., and Zhang, X.: Validation of the aerosol optical property products derived by the GRASP/Component approach from multi-angular polarimetric observations, Atmos. Res., 263, 105802, https://doi.org/10.1016/j.atmosres.2021.105802, 2021. a, b
- Abstract
- Introduction
- Methodology
- Comparison of simulated observations
- Comparison of real PARASOL observations
- Conclusion and outlook
- Appendix A: Algorithm descriptions
- Appendix B: Land and ocean reflection
- Data availability
- Author contributions
- Competing interests
- Disclaimer
- Acknowledgements
- Financial support
- Review statement
- References
- Abstract
- Introduction
- Methodology
- Comparison of simulated observations
- Comparison of real PARASOL observations
- Conclusion and outlook
- Appendix A: Algorithm descriptions
- Appendix B: Land and ocean reflection
- Data availability
- Author contributions
- Competing interests
- Disclaimer
- Acknowledgements
- Financial support
- Review statement
- References