the Creative Commons Attribution 4.0 License.
the Creative Commons Attribution 4.0 License.
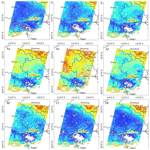
Multi-angle aerosol optical depth retrieval method based on improved surface reflectance
Lijuan Chen
Ren Wang
Ying Fei
Peng Fang
Yong Zha
Retrieval of atmospheric aerosol optical depth (AOD) has been a challenge for Earth satellite observations, mainly due to the difficulty of estimating surface reflectance with the combined influence of land–atmosphere coupling. Current major satellite AOD retrieval products have low spatial resolution under complex surface processes. In this study, we further improved the surface reflectance by modeling the error correction based on the previous AOD retrieval and obtained more accurate AOD retrieval results. A lookup table was constructed using the Second Simulation of Satellite Signal in the Solar Spectrum (6S) to enable high-precision AOD retrieval. The accuracy of the algorithm's retrieval was verified by observations of the Aerosol Robotic Network (AERONET). From the validation results, we find that among the nine Multi-angle Imaging SpectroRadiometer (MISR) angles, the retrieved AOD has the best retrieved results with the AOD observed at the An angle (Taihu: R = 0.81, relative mean bias (RMB) = 0.68; Xuzhou-CUMT: R = 0.73, RMB = 0.78). This study will help to further improve the retrieval accuracy of multi-angle AOD at large spatial scales and long time series. The retrieved AOD based on the improved method has the advantages of fewer missing pixels and finer spatial resolution compared to the MODIS AOD products and our previous estimates.
- Article
(7484 KB) - Full-text XML
-
Supplement
(827 KB) - BibTeX
- EndNote
Aerosols are liquid or solid particles suspended in the atmosphere, with particle diameters ranging from approximately 0.001 to 100 µm (Giles et al., 2019). Aerosol particles suspended in the atmosphere not only affect the radiative forcing of the Earth's air system through direct, indirect, or semi-direct action but also influence climate and cause environmental problems such as acid rain and haze (Hatzianastassiou et al., 2009; Dao et al., 2014; Daniel et al., 2014; Samset et al., 2018; Li et al., 2018; Huang et al., 2021). Therefore, the study of aerosol distribution and change trend has become a hot topic in the field of aerosol research (Holben et al., 2001; Li et al., 2020; Berhane et al., 2021; Sun et al., 2022). In addition, high concentrations of aerosols can pose a serious threat to human health (Lee et al., 2010; Morozova et al., 2015). The optical properties of aerosols include parameters such as aerosol optical depth (AOD), scattering phase function, single-scattering albedo, and absorbing optical depth. Among them, AOD is an important parameter defined as the integral of the aerosol extinction coefficient in the vertical direction. It describes the attenuation effect of aerosols on light and is an important indicator of the level of air pollution. Multi-channel spectrometers on board geostationary and polar-orbiting satellites have been used for AOD retrieval in the last 2 decades. The AOD products obtained through satellite retrievals are widely used in atmospheric environmental studies (Kaufman et al., 1997; Xie et al., 2019; Chen et al., 2021a, b). Although the accuracy of AOD retrieval has been improving, there is still much room for improvement in the AOD retrieval on land when more complex influences are taken into account.
Scholars have conducted research on AOD retrieval using satellite-based multi-angle sensors. Kokhanovsky et al. (2009) used the Along-Track Scanning Radiometer Dual View (ATSR-DV) algorithm to retrieve AOD over Germany and compared the retrieval results with the Medium Resolution Imaging Spectrometer (MERIS) and Multi-angle Imaging SpectroRadiometer (MISR) products. The results show that the ATSR-2 algorithm is also applicable to the Advanced Along-Track Scanning Radiometer (AATSR). Sundstrom et al. (2012) obtained an aerosol model of eastern China based on Aerosol Robotic Network (AERONET) observation data and used the ATSR-DV algorithm to retrieve the proportion of AOD and coarse to fine particles from AATSR data. Abdou et al. (2005) compared the MISR AOD and the Moderate-resolution Imaging Spectroradiometer (MODIS) AOD products carried by Terra using data from 62 AERONET observation sites. The results show that the MODIS AOD of 0.470 and 0.660 µm channels is 35 % and 10 % higher over land than that of MISR AOD values, respectively. In coastal and desert areas, MODIS retrieval error is large, while over the ocean, at 0.470 and 0.660 µm channels, MISR is 0.1 and 0.05 higher than the MODIS AOD value, mainly depending on the accuracy of radiometric calibration. Martonchik et al. (1997) proposed an algorithm for extracting aerosol optical parameters using MISR multi-angle observations. The results show that low-reflectance vegetation and multi-angle observation data are used to retrieve AOD in the case of dense land vegetation. As a new remote sensing tool, multi-angle remote sensing can provide aerosol optical depth, single-scattering albedo, phase function, and other features with sufficient accuracy, which makes it more suitable for playing its unique role in aerosol research than traditional single-angle optical remote sensing (Dubovik et al., 2019). Multi-angle remote sensing retrieval of aerosol optical properties can use the angle information contained in satellite signals to better separate the contributions of the surface and atmosphere, which is suitable for some bright surfaces. This provides a new method of AOD retrieval.
Surface reflectance measures the ability of land to absorb and reflect solar radiation. On land, surface reflectance is relatively complex and is detected by satellite sensors after atmospheric scattering and absorption. Satellite observations capture the combination of there two components, making it challenging to directly separate surface reflectance from atmospheric scattering. Therefore, simultaneous acquisition of atmospheric aerosols and surface reflectance is the main objective of quantitative satellite remote sensing (Deuzé et al., 2001). In optical remote sensing, the blue wavelength is shorter, and the surface reflectance is lower, resulting in more atmospheric reflection and scattering. The blue band is commonly used for AOD retrieval. In the process of AOD retrieval, overestimation of surface reflectance leads to underestimation of AOD, while underestimation of surface reflectance leads to overestimation of AOD. In general, aerosol signals are weaker than surface signals (Dong et al., 2023). Previous studies have shown that when the surface reflectance intercept error is 0.01, the error of satellite remote sensing retrieval AOD is about 0.1 (Zhang et al., 2021). Therefore, accurate estimation of surface reflectance is very important for the accurate retrieval of aerosol optical depth.
In order to improve the accuracy and resolution of MISR AOD retrieval, we analyzed the MISR AOD retrieval errors of nine camera angles in previous studies. Secondly, by establishing an error correction model, we modify the surface reflectance of MISR to improve the accuracy of surface reflectance estimation. Finally, the improved AOD is verified with the ground observation site AOD data, and the retrieval results are compared with the previous retrieval results and MODIS AOD products.
2.1 MISR data
In this study, we employed MISR Level 1B2 terrain data (MI1B2T) and used HEGTool (HDF-EOS To GeoTIFF Conversion Tool) software to extract radiation data. Blocks 64 and 65 covering the Yangtze River Delta region were extracted by selecting corresponding blocks, output data types, and projections based on the regional locations of 180 blocks (Fig. S1 in the Supplement). In addition, we extracted the solar zenith angle, solar azimuth angle, satellite zenith angle, and satellite azimuth angle data of nine cameras from the angle dataset (MI1B2GEOP) and selected the corresponding blocks, output data types, and projection information. To reduce the influence of cloud, we carried out cloud detection and cloud pixel removal on satellite remote sensing images with cloud pixel coverage less than 50 % and used the blue band threshold to remove cloud pixels. However, through repeated experiments, we found that the fixed threshold was not effective in removing cloud pixels at nine angles of the MISR sensor (Figs. S2 and S3 in the Supplement). Therefore, we use dynamic threshold method to remove cloud pixels from MISR data. Details of the data used in this study are shown in Table S2 in the Supplement.
2.2 MODIS data
In this study, we used MODIS L1B data, including radiance data (MOD02/MYD02) and geolocation data (MOD03/MYD03). Data preprocessing applied the MODIS Conversion Toolkit (MCTK) tool, including radiation calibration, butterfly processing, geometric correction, reprojection, and band extraction. MODIS bidirectional reflectance distribution function (BRDF)/Albedo is a standard Level 3 product that represents the surface characteristics of MODIS instruments on the Terra and Aqua satellite platforms. The product retrieval cycle is 16 d, and the observations on day 9 of each 16 d cycle are weighted to generate daily data, called the global daily surface albedo product (Hsu et al., 2004). The core dataset for the MODIS BRDF product is MCD43A1.
2.3 AERONET data
AERONET uses the CE-318 instrument, a solar radiation photometer produced in France, which obtains direct solar spectral radiation measurements at 340, 380, 440, 500, 670, 870, 936, 1020, and 1640 nm channels at 1.5° field angles every 3 min. The total water vapor content of the atmosphere can be obtained in the 936 nm channel. The AOD value can be retrieved by the measured value of other channels, and the retrieval error is about 0.01–0.02. Therefore, it can provide aerosol characteristic parameters with high accuracy and verify aerosol parameters retrieved by satellite (Lu et al., 2019). In this study, AERONET AOD values are used as truth values to verify the accuracy of satellite remote sensing AOD retrieval. AERONET AOD data are classified into three levels: Level 1.0 (unfiltered), Level 1.5 (cloud screening and quality control), and Level 2.0 (quality assurance). The study area mainly covers the Yangtze River Delta, and there are several ground observation sites in the area. However, only two observation sites, Taihu and Xuzhou-CUMT, have continuous observation data, while the duration of other sites is relatively limited. Therefore, AERONET Level 1.5 AOD data (NASA Goddard Space Flight Center, 2023), with a large number of observed values, were selected to verify the retrieval results of MISR AOD.
3.1 Problems in the previous surface reflectance estimation method
Accurate estimation of surface reflectance is a crucial and challenging aspect in the retrieval of AOD from satellite remote sensing data (Remer et al., 2009; Gupta et al., 2016). Previous research has identified the variation patterns of nine-angle MISR AOD (Chen et al., 2021a). However, the AOD retrieved from nine angles shows relatively large errors compared to AERONET AOD (Table S3 in the Supplement). Atmospheric correction can eliminate the effects of clouds and aerosols and obtain true surface reflectance. The reflectance of MISR is calculated by inputting geometric parameters, AOD, aerosol type, sensor radiation brightness data, and sensor height into the Second Simulation of Satellite Signal in the Solar Spectrum (6S) model. In this study, we input MISR geometric parameters and radiation data corresponding to Taihu and Xuzhou-CUMT sites, and AOD parameters are AERONET AOD values of these two sites. The reflectance of atmospheric correction for each pixel is calculated by the linear atmospheric correction formula. To explore the reasons for the high AOD value of the retrieval from nine MISR angles, the MISR atmospheric correction reflectance and MISR surface reflectance were compared at the corresponding locations of the two sites (Fig. 1) (the MISR surface reflectance calculation method is referenced in Chen et al., 2021a). The results show that the surface reflectance of MISR is lower than the atmospheric reflectance of MISR. The MISR AOD retrieved is higher compared to the AERONET AOD. Therefore, it is necessary to establish a correction model to improve the MISR surface reflectance and improve the retrieval accuracy of MISR AOD.
3.2 Improved surface reflectance estimation method
To construct an improved surface reflectivity correction model, the specific steps are shown as follows:
(1) Calculation of MODIS atmospheric-corrected reflectance
The MODIS L1B data are corrected by the 6S model, and the MODIS atmospheric correction reflectance is obtained. When using the 6S model to calculate MODIS atmospheric correction reflectivity, it is necessary to provide geometric parameters, AOD, atmospheric mode, aerosol type, sensor radiance data, sensor height, spectral parameters, and other parameters. In this study, MODIS geometric parameters and radiance data corresponding to Taihu and Xuzhou-CUMT sites are used. The AOD parameters were obtained from AERONET AOD data at both sites. Atmospheric models select mid-latitude winter and mid-latitude summer to account for seasonal variations in atmospheric transport. The aerosol type selected in this study is continental aerosol. The sensor height is set to the observation height of the satellite. The spectral parameters are defined according to the band of the MODIS sensor. By providing these parameters, we can calculate the atmospheric correction reflectance of the MODIS sensor using the 6S model.
(2) Surface bidirectional reflectance calculation
MODIS BRDF/Albedo product MCD43A1 data and the Ross–Li model were used to simulate surface bidirectional reflectance under MODIS and MISR observation geometry. The linear-kernel-driven BRDF model includes three basic parameters: the reflectance of the lowest point view and the weighting coefficient of the two kernel functions. The model can be calculated using Eqs. (1)–(9).
In the above equations, is the surface bidirectional reflectance, while θs, θv, and φ are the solar zenith angle, the satellite zenith angle, and relative azimuth angle, respectively. Λ is bandwidth, while and are the volumetric scattering kernel and geometric optical scattering kernel, respectively, which are functions of the angle of incidence and the angle of observation. fiso, fvol, and fgeo are the weights assigned to isotropic scattering, volumetric scattering, and geometric optical scattering, respectively. ξ is the scattering angle, while b, h, and r represent the vertical radius of the sphere, horizontal radius of the sphere, and height of the center of the sphere, respectively. These three parameters can be defined as fixed values. In the production process of the MODIS BRDF model, the relationship between h, b, and r parameters is and (Schaaf et al., 1999). According to the above equation, the surface bidirectional reflection under any incident direction of the sun and observation direction of the satellite can be obtained by extrapolating the nucleus.
(3) Improved MISR surface reflectance calculation
The MODIS atmospheric correction reflectance is brought into Eqs. (10) and (11), and a new MISR surface reflectance estimate based on MODIS atmospheric correction is calculated. Regression fitting of MISR surface reflectance with the newly estimated MISR surface reflectance was performed (60 % of the total sample data was randomly selected), and the surface reflectance error correction model was finally established, as shown in the following equation:
In Eq. (10), and are BRDFs obtained under MISR and MODIS angles, respectively. θs is the solar zenith angle, θv is the satellite zenith angle, and ϕ is the relative azimuth angle. is the surface reflectance of MODIS at the geometric observation angle of MISR, and is the MODIS atmospheric-corrected reflectance.
In this study, spectral data containing the typical characteristics of 28 different types of vegetation, soil, and water bodies were selected from five standard spectral libraries included with the ENVI software. The surface reflectance of MODIS and MISR sensors in the blue band is calculated by the formula (Chen et al., 2021c).
The new MODIS surface reflectance ( at the MISR angle obtained from Eq. (10) is converted to the MISR surface reflectance by Eq. (11).
The MISR surface reflectance estimated by Eq. (11) was transformed using an error correction model to obtain an improved MISR surface reflectance. The improved MISR surface reflectance will be used for AOD retrieval. The MISR surface reflectance previously estimated based on MODIS V5.2 algorithm was fitted with the MISR surface reflectance estimated based on MODIS atmospheric correction (60 % of the data were randomly selected) by linear regression, and a MISR correction model was established, as shown in Eq. (12). The previously estimated nine-angle MISR surface reflectance is corrected by Eq. (12), and the improved surface reflectance of the MISR sensor at nine angles is obtained and finally used for MISR AOD retrieval at nine angles.
where is the improved MISR surface reflectance in Eq. (12).
3.3 Flow of improved multi-angle AOD retrieval
The flow of the improved surface reflectance algorithm in this study is shown in Fig. 2. Firstly, the atmospheric correction of MODIS L1B data is performed using the 6S model. Then, the MISR surface reflectance estimated by a previous study was combined with the new MISR surface reflectance estimated by Eq. (11) to build a MISR error correction model for obtaining the improved MISR surface reflectance (Chen et al., 2021a). The study retrieved the MISR AOD for nine camera angles using improved MISR surface reflectance. We validated the improved MISR AOD with AERONET AOD. We compared the improved AOD with the previously retrieved AOD and analyzed the accuracy and spatial distribution trends of the improved AOD. The AOD retrieval method used in this study is based on Chen et al. (2021a). In our study, we used continental aerosols for AOD retrieval and the 6S model for atmospheric correction. Choosing the right aerosol type is crucial for obtaining accurate aerosol optical depth. Previous studies have shown that continental aerosols can be used to estimate aerosol optical depth in the Yangtze River Delta (He et al., 2014). In this study, the same aerosol type was used for AOD retrieval and atmospheric correction, and the error transfer caused by aerosol type and atmospheric correction was not considered.
4.1 Improved MISR surface reflectance variation characteristics
The variation characteristics of MISR surface reflectance, MISR atmospheric correction reflectance, and improved MISR surface reflectance are shown in Fig. 3. These values are the average values of all sample data collected at the corresponding locations of the two sites in Taihu and Xuzhou-CUMT during the period of validity from 2016–2018. At Taihu and Xuzhou-CUMT sites, the improved MISR surface reflectance of nine camera angles is generally higher than that of MISR surface reflectance and lower than that of MISR atmospheric correction reflectance. The MISR surface reflectance of nine camera angles is between 0.02 and 0.04. On average, the improved surface reflectance is higher than the previously estimated MISR surface reflectance.
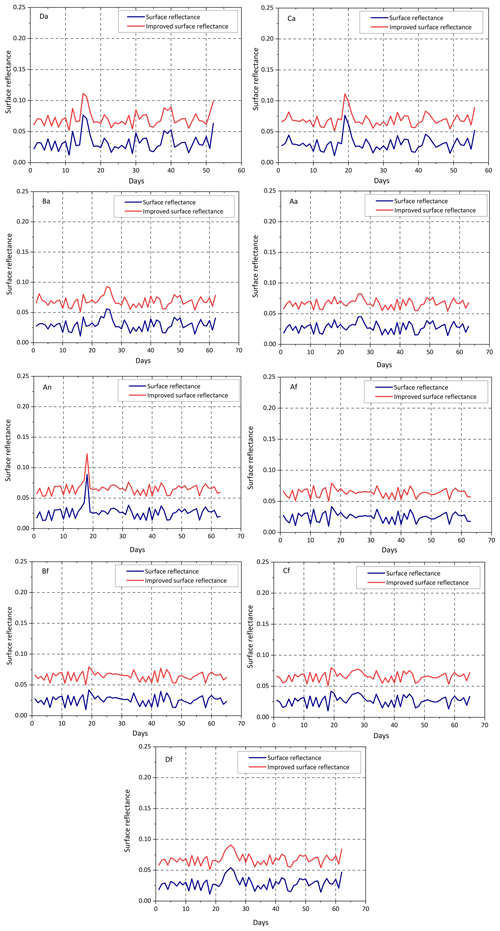
Figure 4Time series of surface reflectance in the blue band of the MISR sensor for nine observation angles (Table S2 lists the time series of the nine angles from front to back).
To clarify the trend of the improved surface reflectance, the study conducted a time-series analysis of the MISR surface reflectance and the improved surface reflectance. The improved MISR surface reflectance is always higher than the previously estimated MISR surface reflectance (Fig. 4). The MISR surface reflectance is generally between 0 and 0.05, while the improved surface reflectance values is about 0.05 to 0.1. Overall, the improved surface reflectance was increased.
4.2 Improved MISR AOD retrieval results
MISR AOD results spanning 3 years were obtained using improved surface reflectance retrieval. This study gives the results of the retrieval of the MISR sensor on 12 June 2018 for nine camera observation angles (Fig. 5). From the spatial distribution of AOD, it can be seen that the retrieval results in the study area do not exceed 1. The overall spatial distribution trend is generally consistent with the pre-improvement results (Chen et al., 2021a), but there are differences in the magnitude of the values. The values in the northeast and south range from 0.5 to 1, indicating poor air quality in these areas. The AOD values retrieved from five camera observation angles, Ba, Aa, An, Af, and Bf, are in the approximate range of 0.25–0.5. In the central region, most of the AOD values from the four camera observation angles, Da, Ca, Cf, and Df, are in the range of 0–0.25. The data show that the air quality in the central region is generally good, and the air pollution level is light in some areas. The higher AOD in southern Shandong and northern Jiangsu may be related to the increase in local aerosol emissions caused by human activities.
The improved MISR AOD spatial distribution results were then validated by comparing them with the MODIS AOD product. The MODIS AOD product has a resolution of 3 km. The trends in the spatial distribution of MODIS AOD products are consistent with the improved MISR AOD (Fig. 6). However, the MODIS AOD product has more missing data, and the AOD obtained using the improved algorithm for retrieval can avoid missing data. In addition, the AOD retrieval with the improved algorithm has a higher resolution compared to the image quality of the MISR AOD product.
4.3 Verification of improved MISR AOD
There are many AERONET sites in the Yangtze River Delta region, but currently only two sites, Taihu and Xuzhou-CUMT, are able to provide continuous data, and the other sites acquire data on limited timescales. Therefore, we selected the Taihu and Xuzhou-CUMT sites with more data for verification. To validate the retrieved MISR AODs, we selected valid AOD records in the 550 nm band within a 30 min interval between the AERONET ground observation site and the Terra satellite. The MISR sensor nine camera views take about 7 min to be able to observe the same geographic location at relatively short intervals. Therefore, we used the AERONET AOD averages as approximate truth values and compared them with the retrieved MISR AODs to validate and minimize errors due to time lag. Spatially, we selected the image elements observed by the MISR sensor from nine angles and compared them with the corresponding positional AERONET observations. Since the sun photometer did not have a wavelength of 550 nm corresponding to the retrieval results, the AOD at 550 nm was calculated by applying Ångström (Eq. 13).
In the formula, τ(λ) is the AOD at wavelength λ, β is the concentration of aerosols throughout the atmosphere, and α is the wavelength index of Ångström.
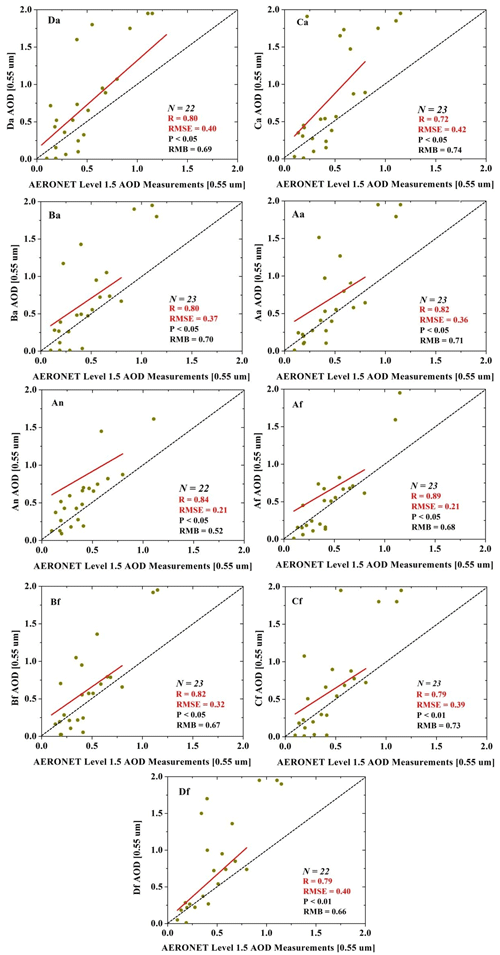
Figure 7Comparison between improved MISR AOD and AERONET AOD at the Taihu site (N is the number of verification points, and the red line represents a linear fitting line).
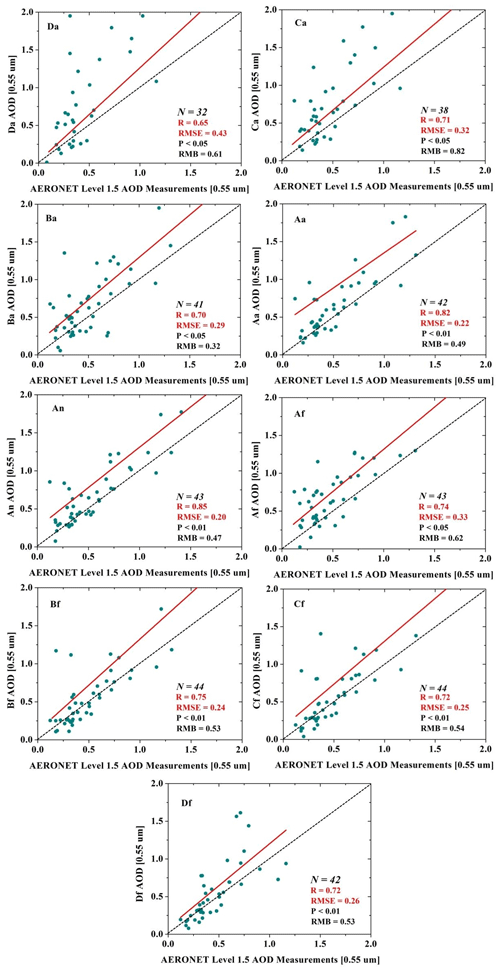
Figure 8Comparison between improved MISR AOD and AERONET AOD at the Xuzhou-CUMT site (N is the number of verification points, and the red line represents a linear fitting line).
In this study, four parameters, correlation coefficient (R), root mean square error (RMSE), p value, and relative mean bias (RMB), will be used to assess the accuracy of the remotely sensed AOD dataset. The specific calculation principles for the three parameters R, RMSE, and RMB are shown in Eqs. (14)–(16). The validation results of AOD dataset of Taihu and Xuzhou-CUMT sites during 2016–2018 after improvement in this study are shown in Figs. 7 and 8.
Generally, the scatter plots are distributed above and below the 1:1 line. R is a parameter characterizing the correlation between the remote sensing retrieval results and the ground retrieval results. R reached 0.89 for the Taihu site and 0.85 for the Xuzhou-CUMT site. RMSE is a parameter characterizing the absolute error of the remote sensing retrieval results, and the minimum RMSE is 0.21 for the Taihu site. The minimum RMSE is 0.20 for the Xuzhou-CUMT site. RMB is a parameter used to describe the relative error of remote sensing retrieval results, and the minimum RMB is 0.52 for the Taihu site and 0.32 for the Xuzhou-CUMT site. Overall, the retrieval results at the An angle are optimal after algorithm improvements (Taihu: R = 0.84, RMB = 0.52; Xuzhou-CUMT: R = 0.85, RMB = 0.47). By comparing the results with the pre-improvement AOD validation, the improved AOD retrieval accuracy has been significantly improved (Table 1). In Table 1, R is the correlation between the AOD retrieval results and AERONET AOD before improvement. RMB is the relative deviation between the AOD retrieval by the pre-improvement algorithm and AERONET AOD. Improved R is the correlation between the improved AOD retrieval results and AERONET AOD. Improved RMB is the relative deviation between the AOD retrieved with the improved algorithm and AERONET AOD.
where Ai is the retrieved MISR AOD; is the corresponding AERONET AOD; and and are the mean values of the retrieve MISR AOD and AERONET AOD, respectively. N is the number of valid matching results for AERONET AOD and MISR AOD.
Comparing the validation results of the MODIS AOD product with those of the observation sites (Taihu: R = 0.59, RMSE = 0.19, P < 0.05, RMB = 0.52; Xuzhou-CUMT: R = 0.71, RMSE = 0.25, P < 0.05, RMB = 0.44) (Chen et al., 2021a), we found that the Taihu and Xuzhou-CUMT sites have high correlation between the improved MISR AOD and MODIS AOD products. The smaller the observation angle of the improved MISR AOD, the closer the error is to the MODIS AOD product. The An observation angle of MISR is the same as that of MODIS. Therefore, we selected two sites for validation at the An observation angle for the MODIS AOD product (Fig. 9). The results show that the An AOD retrieval by the improved algorithm correlates well with the MODIS AOD product. The position errors of the two image elements are close to each other. The RMSE of the Xuzhou-CUMT site is slightly higher than that of the Taihu site, and the RMB of the Taihu site is slightly higher than that of the Xuzhou-CUMT site.
In this study, we first explored the problems of estimating surface reflectance in our previous study. We obtained an error correction model for surface reflectance using a linear fit of the MISR surface reflectance and a new estimate of the MISR surface reflectance. The improved MISR surface reflectance was obtained through the error correction model. We then retrieved a new AOD product using the improved surface reflectance and a lookup table constructed from the 6S model. Two AERONET ground observation sites with longer time series were used to validate the AOD obtained by satellites.
Overall, the improved AOD and its spatial distribution trends are consistent with our previous results. The AOD estimated by improved method exhibited higher accuracy and a high degree of agreement with the AERONET ground-based observational AOD. Overall, the retrieval results at the An angle are optimal after algorithm improvements (Taihu: R = 0.84, RMB = 0.52; Xuzhou-CUMT: R = 0.85, RMB = 0.47). The improvements at the Xuzhou-CUMT site are more pronounced compared to the Taihu site.
More importantly, the optimal AOD obtained in this study has fewer missing pixels and finer spatial resolution than the MODIS AOD product. Through the validation with MODIS AOD products, the AOD of the optimal An angle obtained by the improved algorithm is highly correlated with MODIS AOD products.
In future, more aerosol models that conform to the actual situation in the study area can be constructed using the AERONET ground observation data and introduced into the MISR AOD retrieval algorithm to further improve the accuracy of the AOD retrieval results. In this study, the AERONET AOD was used as the true value and as an input for the AOD parameter in the 6S model for atmospheric correction of MISR and MODIS images. We then obtained a surface reflectivity error correction model to retrieve AOD for the entire region. It should be emphasized that the more AERONET sites used to train the corrected model, the more accurate AOD retrieved may be obtained by this method. However, the data from the AERONET ground observation sites were limited. In future, the study area can be expanded on a large scale and for a longer time series.
The 6S model and codes are available from the authors upon request (lijuan@nuist.edu.cn).
The Earthdata Atmospheric Science Data Center provided the MI1B2T (https://doi.org/10.5067/Terra/MISR/MI1B2T_L1.003; NASA, 2007) and MI1B2GEOP (https://doi.org/10.5067/Terra/MISR/MIB2GEOP_L1.002; NASA, 2008) datasets. The MODIS L1B and MODIS BRDF datasets are available from the LAADS DAAC (https://ladsweb.modaps.eosdis.nasa.gov/search/, NASA LAADS DAAC, 2023). The AERONET product datasets are available for download from the AErosol RObotic NETwork website (https://aeronet.gsfc.nasa.gov/new_web/index.html; AERONET, 2023).
The supplement related to this article is available online at: https://doi.org/10.5194/amt-17-4411-2024-supplement.
LC and YZ developed the idea with respect to improving the retrieval of AOD; LC wrote the manuscript; RW reviewed the manuscript; YF and PF contributed to algorithm development and data analysis; and HC reviewed the manuscript. All the co-authors reviewed and edited the manuscript.
The contact author has declared that none of the authors has any competing interests.
Publisher's note: Copernicus Publications remains neutral with regard to jurisdictional claims made in the text, published maps, institutional affiliations, or any other geographical representation in this paper. While Copernicus Publications makes every effort to include appropriate place names, the final responsibility lies with the authors.
The authors are grateful to the Jet Propulsion Laboratory for providing the MISR data. We also wish to thank the editor (Jian Xu) and the anonymous reviewers for their valuable comments.
This study was supported by the National Natural Science Foundation of China (grant no. 42021004), the Natural Science Foundation of Jiangsu Province (grant no. BK20220455), the National Natural Science Foundation of China (grant no. 42201028), and the Jiangsu Funding Program for Excellent Postdoctoral Talent (grant no. 2023ZB482).
This paper was edited by Jian Xu and reviewed by three anonymous referees.
Abdou, W. A., Diner, D. J., Martonchik, J. V., Bruegge, C. J., Kahn, R. A., Gaitley, B. J., Crean, K. A., Remer, L. A., and Holben, B.: Comparison of coincident multiangle imaging spectroradiometer and moderate resolution imaging spectroradiometer aerosol optical depths over land and ocean scenes containing aerosol robotic network sites, J. Geophys. Res., 110, D10S07, https://doi.org/10.1029/2004JD004693, 2005.
AERONET (Aerosol Robotic Network): https://aeronet.gsfc.nasa.gov/new_web/index.html, last access: 10 August 2023.
Berhane, S. A. and Bu, L.: Aerosol-Cloud Interaction with Summer Precipitation over Major Cities in Eritrea, Remote Sens.-Basel, 13, 21, https://doi.org/10.3390/rs13040677, 2021.
Chen, L., Wang, R., and Han, J.: Influence of observation angle change on satellite retrieval of aerosol optical depth, Tellus B, 73, 1–14, https://doi.org/10.1080/16000889.2021.1940758, 2021a.
Chen, L., Fei, Y., and Wang, R.: Retrieval of high temporal resolution aerosol optical depth using the GOCI remote sensing data, Remote Sens.-Basel, 13, 2376, https://doi.org/10.3390/rs13122376, 2021b.
Chen, L., Wang, R., and Wei, G.: A surface reflectance correction model to improve the retrieval of MISR aerosol optical depth supported by MODIS data, Adv. Space. Res., 67, 858–867, https://doi.org/10.1016/j.asr.2020.10.033, 2021c.
Daniel, R., Steven, S., Robert, W., and Leo, D.: Climate Effects of Aerosol-Cloud Interactions, Science, 343, 379–380, https://doi.org/10.1126/science.1247490, 2014.
Dao, Y. and Gong, W.: Observed holiday aerosol reduction and temperature cooling over East Asia, J. Geophys. Res.-Atmos., 11, 6306–6324, https://doi.org/10.1002/2014JD021464, 2014.
Deuzé, J. L., Bréon, F. M., Devaux, C., Goloub, P., Herman, M., Lafrance, B., Maignan, F., Marchand, A., Nadal, F., Perry, G., and Tanré, D.: Remote sensing of aerosols over land surfaces from POLDER-ADEOS-1 polarized measurements, J. Geophys. Res.-Atmos., 106, 4913–4926, https://doi.org/10.1029/2000JD900364, 2001.
Dong, W., Tao, M., Xu, X., Wang, J., Wang, Y., Wang, L., Song, Y., Fan, M., and Chen, L.: Satellite Aerosol Retrieval From Multiangle Polarimetric Measurements: Information Content and Uncertainty Analysis, IEEE T. Geosci. Remote, 61, 1–13, https://doi.org/10.1109/TGRS.2023.3264554, 2023,.
Dubovik, O., Li, Z., and Mishchenko, M. I.: Polarimetric remote sensing of atmospheric aerosols: Instruments, methodologies, results, and perspectives, Pergamon, 224, 474–511, https://doi.org/10.1016/j.jqsrt.2018.11.024, 2019.
Giles, D. M., Sinyuk, A., Sorokin, M. G., Schafer, J. S., Smirnov, A., Slutsker, I., Eck, T. F., Holben, B. N., Lewis, J. R., Campbell, J. R., Welton, E. J., Korkin, S. V., and Lyapustin, A. I.: Advancements in the Aerosol Robotic Network (AERONET) Version 3 database – automated near-real-time quality control algorithm with improved cloud screening for Sun photometer aerosol optical depth (AOD) measurements, Atmos. Meas. Tech., 12, 169–209, https://doi.org/10.5194/amt-12-169-2019, 2019.
Gupta, P., Levy, R. C., Mattoo, S., Remer, L. A., and Munchak, L. A.: A surface reflectance scheme for retrieving aerosol optical depth over urban surfaces in MODIS Dark Target retrieval algorithm, Atmos. Meas. Tech., 9, 3293–3308, https://doi.org/10.5194/amt-9-3293-2016, 2016.
Hatzianastassiou, N.: The direct effect of aerosols on the radiation budget and climate of the Earth-atmosphere system: its variability in space and time, EGU General Assembly Conference Abstracts EGU General Assembly Conference Abstracts, 11, EGU2009-10109, 2009.
He, J., Zha, Y., Zhang, J., and Gao, J.: Aerosol Indices Derived from MODIS Data for Indicating Aerosol-Induced Air Pollution, Remote Sens., 6, 1587–1604, https://doi.org/10.3390/rs6021587, 2014.
Holben, B. N., Tanré, D., and Smirnov, A.: An emerging ground-based aerosol climatology: Aerosol optical depth from AERONET, J. Geophys. Res., 106, 12067–12097, https://doi.org/10.1029/2001JD900014, 2001.
Hsu, N. C., Tsay, S. C., and King, M. D.: Aerosol properties over bright-reflecting source regions, IEEE T. Geosci. Remote, 42, 557–569, https://doi.org/10.1109/TGRS.2004.824067, 2004.
Huang, X. and Ding, A.: Aerosol as a critical factor causing forecast biases of air temperature in global numerical weather prediction models, Sci. Bull., 18, 1917–1924, https://doi.org/10.1016/j.scib.2021.05.009, 2021.
Kaufman, Y. J., Wald, A. E., Remer, L. A., Gao, B. C., Li, R., and Flynn, L.: The MODIS 2.1-um channel-correlation with visible reflectance for use in remote sensing of aerosol, IEEE T. Geosci. Remote, 35, 1286–1298, https://doi.org/10.1109/36.628795, 1997.
Kokhanovsky, A. A., Curier, R. L., Leeuw, G. D., and Grey, W. M. F.: The intercomparison of AATSR dual-view aerosol optical thickness retrievals with results from various algorithms and instruments, Int. J. Remote Sens., 30, 4525–4537, https://doi.org/10.1080/01431160802578012, 2009.
Lee, S. S., Donner, L. J., and Penner, J. E.: Thunderstorm and stratocumulus: how does their contrasting morphology affect their interactions with aerosols?, Atmos. Chem. Phys., 10, 6819–6837, https://doi.org/10.5194/acp-10-6819-2010, 2010.
Li, E., Zhang, Z., and Tan, Y.: A Novel Cloud Detection Algorithm Based on Simplified Radiative Transfer Model for Aerosol Retrievals: Preliminary Result on Himawari-8 Over Eastern China, IEEE T. Geosci. Remote, 59, 1–12, https://doi.org/10.1109/TGRS.2020.3004719, 2020.
Li, Y., Xue, Y., and Guang, J.: Ground-Level PM2.5 Concentration Estimation from Satellite Data in the Beijing Area Using a Specific Particle Swarm Extinction Mass Conversion Algorithm, Remote Sens.-Basel, 10, 1906, https://doi.org/10.3390/rs10121906, 2018.
Lu, S., Xue, Y., Yang, X. H., Leys, J., Guang, J., Che, Y. H., Fan, C., Xie, Y. Q., and Li, Y.: Joint Retrieval of Aerosol Optical Depth and Surface Reflflectance Over Land Using Geostationary Satellite Data, IEEE T. Geosci. Remote, 57, 1489–1501, https://doi.org/10.1109/TGRS.2018.2867000, 2019.
Martonchik, J. V.: Determination of aerosol optical depth and land surface directional reflectances using multiangle imagery, J. Geophys. Res., 102, 17015–17022, https://doi.org/10.1029/96JD02444, 1997.
Morozova, A. L. and Mironova, I. A.: Aerosols over continental Portugal (1978–1993): their sources and an impact on the regional climate, Atmos. Chem. Phys., 15, 6407–6418, https://doi.org/10.5194/acp-15-6407-2015, 2015.
NASA: MI1B2T, NASA Langley Atmospheric Science Data Center DAAC [data set], https://doi.org/10.5067/Terra/MISR/MI1B2T_L1.003, 2007.
NASA: MI1B2GEOP, NASA Langley Atmospheric Science Data Center DAAC [data set], https://doi.org/10.5067/Terra/MISR/MIB2GEOP_L1.002, 2008.
NASA Goddard Space Flight Center: AERONET data, available at: https://aeronet.gsfc.nasa.gov/new_web/aerosols.html, last access: 20 October 2023.
NASA LAADS DAAC: MODIS L1B data and MODIS BRDF data, available at: https://ladsweb.modaps.eosdis.nasa.gov/search/, last access: 10 August 2023.
Remer, L. A., Tanré, D., and Kaufman, Y. J.: Algorithm for remote sensing of tropospheric aerosol from MODIS: Collection 005, NASA's Earth Observing System, https://eospso.gsfc.nasa.gov/atbd/algorithm-remote-sensing-tropospheric-aerosol-modis (last access: 2 September 2023), 2009.
Samset, B. H., Sand, M., and Smith, C. J.: Climate Impacts From a Removal of Anthropogenic Aerosol Emissions, Geophys. Res. Lett., 45, 1020–1029, https://doi.org/10.1002/2017GL076079, 2018.
Schaaf, C. B., Strahler, A. H., and Gao, F.: MODIS BRDF Albedo Product ATBD V 5.0, Eospso.nasa.gov, https://modis.gsfc.nasa.gov/data/atbd/ (last access: 10 May 2024), 1999.
Sun, E., Fu, C., and Yu, W.: Variation and Driving Factor of Aerosol Optical Depth over the South China Sea from 1980 to 2020, Atmosphere, 13, 372, https://doi.org/10.3390/atmos13030372, 2022.
Sundstrom, A. M., Kolmonen, P., Sogacheva, L., and Leeuw, G. D.: Aerosol retrieval over China with the AATSR dual view algorithm, Remote Sens. Environ., 116, 189–198, https://doi.org/10.1016/j.rse.2011.04.041, 2012.
Xie, Y., Xue, Y., and Jie, G.: Deriving a Global and Hourly Data Set of Aerosol Optical Depth Over Land Using Data From Four Geostationary Satellites: GOES-16, MSG-1, MSG-4, and Himawari-8, IEEE T. Geosci. Remote, 99, 1–12, https://doi.org/10.1109/TGRS.2019.2944949, 2019.
Zhang, Y., Li, Z., Liu, Z., Wang, Y., Qie, L., Xie, Y., Hou, W., and Leng, L.: Retrieval of aerosol fine-mode fraction over China from satellite multiangle polarized observations: validation and comparison, Atmos. Meas. Tech., 14, 1655–1672, https://doi.org/10.5194/amt-14-1655-2021, 2021.