the Creative Commons Attribution 4.0 License.
the Creative Commons Attribution 4.0 License.
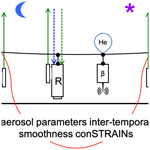
Comparison of diurnal aerosol products retrieved from combinations of micro-pulse lidar and sun photometer observations over the KAUST observation site
Anton Lopatin
Oleg Dubovik
Georgiy Stenchikov
Ellsworth J. Welton
Illia Shevchenko
David Fuertes
Marcos Herreras-Giralda
Tatsiana Lapyonok
Alexander Smirnov
This study focuses on the comparison of aerosol columnar aerosol optical depth (AOD) and lidar ratios together with vertical profiles of aerosol extinction and backscatter at 532 nm retrieved over the King Abdullah University of Science and Technology (KAUST) campus observation site for the period of 2019–2022 using the Generalized Retrieval of Atmosphere and Surface Properties (GRASP) and Micro-Pulse Lidar Network (MPLNET) approaches. An emphasis is placed on independent analysis of daylight and nighttime retrievals to estimate how strongly the differences in the assumptions of both methods made in the absence of nighttime AOD observations influence the retrieval results. Additionally, two aerosol products provided by GRASP excluding and including the volume depolarization observations at 532 nm provided by MPLNET are analyzed to estimate the potential benefits of usage of depolarization data in aerosol profile retrievals.
Overall, both columnar and vertical MPLNET and GRASP products demonstrated a better agreement for daytime retrievals for the GRASP product that excluded the depolarization information. At the same time, inclusion of the volume depolarization observations improved the agreement between MPLNET- and GRASP-estimated values at nighttime, both columnar and vertical.
In addition, estimated values of daytime extinction profiles at ground level were compared to assess the impact of the assumption of a constant aerosol vertical distribution in the cutoff zone of lidar observations implied in GRASP. The values estimated by GRASP demonstrated a good agreement with MPLNET, for retrievals both including and excluding volume depolarization information.
A seasonal variability in the diurnal cycle of aerosol properties estimated by GRASP over the KAUST site for the period 2019–2022 is presented, analyzed and discussed.
- Article
(7363 KB) - Full-text XML
- BibTeX
- EndNote
The vertical distribution of atmospheric aerosols plays an important role in effects that determine aerosol influence on the Earth's climate. This includes both the direct aerosol–radiation interaction that affects the Earth radiative budget and indirect effects through the modification of cloud formation and their life cycle (Twomey, 1974; Albrecht, 1989) as well as via a semi-direct effect that consists of modifying the cloud formation by change in atmospheric temperature due to the direct absorption of solar light by aerosols (Koch and Del Genio, 2010).
In addition, exposure to aerosol particles is also known to impact human health (Ault and Axson, 2017). High concentrations of fine particulates in air increase health risks associated with respiratory (Pope et al., 2002) and cardiopulmonary functions (Wellenius et al., 2012) as well as lung tumors (Raaschou-Nielsen et al., 2013). In this regard, knowledge about aerosol distribution near the surface becomes crucial to estimate long-term exposure to particulates and therefore the health risks to populations. Additionally, knowledge about aerosol vertical distribution is crucial for the verification and tuning of chemical transport and climate calculation models on both regional and global scales.
Remote sensing offers the most suitable observations for this purpose. In fact, remote sensing techniques are capable of characterizing the properties of ambient, non-perturbed aerosols and can provide continuous data at regional and even global scales. However, it is important to note that different types of remote sensing measurements have varying sensitivities and often yield complementary information about aerosols, which requires meticulous analysis and appropriate interpretation in order to maximize the benefits of the observations. Lidar (light detection and ranging) is one of the most common remote sensing techniques that allows us to observe aerosol vertical variation as well as its temporal evolution. Lidar detectors have the capability to measure the time delay between the emission of a light pulse, usually provided by a laser, and its return that is backscattered from the aerosol particles. This allows one to establish the location and, by measuring the magnitude of the returned signal, the particle concentration of the aerosol layer. Measured lidar data from backscatter lidars are inversely proportional to the range squared and depend on the emitted laser energy and other lidar-specific calibration factors (overlap, laser–detector crosstalk or afterpulse, and polarization quality) as well as the solar background at the laser wavelength. Lidar processing methods must calibrate and normalize the measured data to produce the so-called lidar signal (here referred to as the normalized relative backscatter, NRB) at the specified wavelength, described by the following equation:
where is extinction and is backscatter of the aerosol layer, containing both molecular and aerosol parts, respectively, and C is a so-called calibration constant that is a function of the receiver efficiency, aperture, and optical design. The other standard form of the lidar signal removes dependence on C, through either laboratory calibration or normalization against molecular background, and is referred to as attenuated backscatter. However, the determination of aerosol backscatter and extinction profiles using typical backscatter lidar retrievals (Fernald et al., 1972; Klett, 1981; Fernald, 1984) is independent of C, and thus either form of the lidar signal may be used. The Generalized Retrieval of Atmosphere and Surface Properties (GRASP) utilizes a different approach, with normalization of the NRB signal to exclude the influence of the calibration constant C (Lopatin et al., 2013, 2021):
The lidar equation (Eq. 1) depends on two, strictly speaking independent, profiles of extinction and backscatter, rendering retrieval of these properties from a single observation impossible. There are a variety of methods that allow for overcoming such a limitation by introducing additional, usually a priori, information in various forms about the relation between aerosol extinction and backscatter, making the solution of the lidar equation possible. In the simplest approach, this takes the form of a linear dependence parameter, known as the lidar ratio (LR):
where ωo is the single-scattering albedo and P11(180°) is the phase function at a 180° backscatter angle, providing the lidar ratio in units of steradians (sr).
One of the most straightforward estimations was proposed by Klett (1981) and consists in assuming the lidar ratio to be vertically constant and fixed to a selected value, which is usually equal to 50 sr or is chosen according to the properties of the expected aerosol type. For example, the Cloud-Aerosol Lidar with Orthogonal Polarization (CALIOP) aerosol typing algorithm (Kim et al., 2018) could be considered a further advancement of the Klett approach, allowing us to assign several pre-defined lidar ratios to different aerosol types based on climatological values. However, large errors in the retrieved aerosol backscatter and extinction profiles can occur if the assigned lidar ratio differs from the actual value. Another technique is utilized to reduce these errors, whereby independent measurements of the aerosol optical depth (AOD) are used to constrain a backward Fernald (1984) retrieval of the aerosol profiles (Welton et al., 2000), ensuring the retrieved extinction profile integrates with the measured AOD. With this technique, a column-averaged lidar ratio is calculated from the measurements, instead of being pre-assigned. Errors can still occur, since the lidar ratio is assumed to be constant through the atmospheric layer analyzed, but the results are expected be more accurate due to the AOD constraint.
Another option is improvement in observation techniques in order to perform measurements of extinction and backscatter separately by so-called Raman techniques (Wandinger, 2005). Such systems, together with the backscatter signal, can directly measure the attenuation of the atmosphere by triggering radiation emission by certain gases at different atmospheric layers, which provides direct sensitivity to the amount of aerosol below the level of the induced emission. As a further development of such techniques, high-spectral-resolution lidars (HSRLs; e.g., Hair et al., 2008) should be additionally mentioned. HSRL allows measurement of aerosol attenuation and backscatter separately at a much closer wavelength than traditional Raman techniques, which usually rely on assumptions of the aerosol Ångström exponent in order to correctly process Raman-shifted signals in combination with elastic channels.
Sophisticated lidar systems such as Raman and HSRL greatly enhance the information available from lidar observations of aerosol properties. However, even the most advanced lidars have limitations when it comes to capturing fine details of aerosol characteristics compared, for example, to passive multi-angular observations. This is partly because lidar systems typically utilize just a few spectral channels (between one and five) and can register intensity and the state of depolarization of reflected signals with the number of independent measurements summing to no more than 8, even for the most advanced setups. Furthermore, ground-based lidar observations have a blind zone close to the ground due to afterpulse and incomplete geometrical overlap between the laser beam and telescope field of view, which can extend from several hundred meters to several kilometers depending on the system's design and purpose. Additionally, the signals captured by lidar are typically weak and dim significantly with distance; therefore lidar measurements are subject to significant registration noise, particularly during daytime observations, which can limit the capabilities of Raman or HSRL observations in daylight. As such, it is always desirable to have ancillary data from co-located photometric measurements to aid the interpretation of lidar observations and to recognize the complementary nature of passive and active measurements, even with the use of advanced lidar systems.
Various algorithms have been proposed for the joint processing of coincident photometric and lidar ground-based observations to retrieve aerosol properties. Some of these methods focus on treating the data of available lidar systems combined into networks. In this study we utilize observations and aerosol data provided by the Micro-Pulse Lidar Network (MPLNET; Welton et al., 2001, 2018). MPLNET began operations in 2000 with the goal of providing co-located lidar profiling at key sites in the NASA Aerosol Robotic Network (AERONET) (Holben et al., 1998). MPLNET aerosol processing utilizes the constrained retrieval technique (Welton et al., 2000) with the AERONET AOD as the constraint, providing vertical distributions of aerosol optical properties, notably extinction and backscatter, and calculation of a column-averaged lidar ratio. The retrieval techniques and limitations from using a single wavelength backscatter lidar preclude the retrieval of microphysical parameters such as size and refractive index. However, MPLNET lidars have been polarized (Flynn et al., 2007; Welton et al., 2018) since 2014–2015, and thereafter the aerosol processing has included retrievals of the aerosol depolarization ratio, providing additional information on particle shape.
Other algorithmic techniques attempt to advance and derive vertical profiles of several aerosol components, as well as extra parameters of the column-integrated properties of aerosols. For example, the Lidar-Radiometer Inversion Code (LIRIC; Chaikovsky et al., 2016) and Generalized Aerosol Retrieval from Radiometer and Lidar Combined data (GaRRLiC)/GRASP (Lopatin et al., 2013, 2021) algorithms use joint data from multi-wavelength lidars and AERONET sun–sky-scanning radiometers. LIRIC, for example, uses microphysical columnar properties provided by AERONET as necessary a priori values in order to perform retrieval of aerosol vertical profiles. However, in such an approach the columnar property retrieval does not benefit from any extra sensitivity of lidar measurements and relies on several additional assumptions, e.g., the spectral interpolation of a complex refractive index (Chaikovsky et al., 2016).
Thus, this article focuses on the comparison of aerosol columnar and vertical optical products retrieved over the King Abdullah University of Science and Technology (KAUST) campus observation site using different methodologies, specifically using GRASP and MPLNET approaches. Both approaches have several similarities. For example, they use the same set of lidar signals from MPLNET standard processing and produce aerosol profiles of extinction and backscatter at 532 nm together with estimations of columnar AOD and the lidar ratio (LR) at the same wavelength. Also, both methods provide estimations performed during the daytime and nighttime. Unfortunately, the KAUST AERONET site does not provide lunar AOD observations, and therefore such a retrieval scheme will be out of the scope of this study. Indeed, the use of nighttime lunar observations could significantly improve the nocturnal retrievals, requiring fewer assumptions to be made regarding the aerosol temporal variability in the case of GRASP. A lack of AERONET lunar AOD also has a significant impact on the nighttime MPLNET aerosol processing, as described below. Instead, the study will focus on comparing the columnar and vertical values of nighttime retrievals in order to estimate how well the assumptions of different methodologies agree with each other and how strongly existing differences influence the retrieval results.
The KAUST campus is situated in Thuwal on the eastern coast of the Red Sea, on the western Arabian Peninsula (22.3° N, 39.1° E). The region experiences local dust storms that arise from the surrounding inland deserts (e.g., see Kalenderski and Stenchikov, 2016), as well as distant dust from northeastern Africa through the Tokar Gap (Parajuli et al., 2020). Consequently, there is a year-round presence of desert dust in the atmosphere over the site. KAUST is a unique lidar site on the Red Sea coast, and its co-location with the AERONET station allows for a more accurate retrieval of the vertical profile of aerosols (Welton et al., 2000; Parajuli et al., 2020; Lopatin et al., 2021). Additionally, KAUST has a meteorological station that performs measurements of air temperature, humidity, wind speed, and incoming shortwave and longwave radiative fluxes. Stations that measure various parameters of interest for dust-related research, such as the dust deposition rate, vertical profile, near-surface concentration and spectral optical depth, are particularly rare across the global dust belt. The collection of these co-located data provides a unique opportunity to obtain a more comprehensive understanding of dust emissions and transport in the region.
A micro-pulse lidar (MPL) has been in operation at the KAUST site since 2014, being a part of the Micro-Pulse Lidar Network (Welton et al., 2001, 2018). MPLNET Version 3 (V3) data products are automatically processed, providing near-real-time (NRT) data generated with NRT calibrations. MPLNET utilizes the same product level convention as AERONET. Level 1 and 1.5 data are NRT but with the latter including quality assurance screening. Final Level 2 products are generated after Level 2 AERONET data are available and using final calibrations. Meteorological data from the NASA GEOS-5 model are used to calculate molecular quantities and diagnostic parameters. The MPLNET product suite includes the signal product (NRB), which comprises the lidar signals, volume depolarization ratio and diagnostics (Campbell et al., 2002; Welton and Campbell, 2002; Welton et al., 2018). The MPLNET cloud product (CLD) includes multiple cloud layer heights and tops, cloud phase, and estimates of thin cloud optical depth (Lewis et al., 2016, 2020). The MPLNET aerosol product (AER) includes aerosol layer height; profiles of the extinction, backscatter and aerosol depolarization ratio; the columnar lidar ratio; and calculation of the lidar constant (C) (Welton et al., 2000, 2002, 2018). The aerosol variables are retrieved continuously using a running 20 min cloud-screened signal average (where cloud screening is only applied to clouds below the aerosol top height) and re-gridded to a 1 min temporal grid in the product. The MPLNET planetary boundary layer product (PBL) contains mixed-layer heights and estimates of the mixed-layer AOD (Lewis et al., 2013). All data products are publicly accessible on the MPLNET website (https://mplnet.gsfc.nasa.gov, last access: 18 July 2024) and are stored in netCDF4, CF-compliant formats. All variables in each product contain uncertainties derived from the error propagation of raw data and calibrations. More detailed information about MPLNET data is available on the abovementioned website. Here, comparisons are made between MPLNET V3 standard aerosol product retrievals (L1.5 AER) and those produced from GRASP using corresponding MPLNET L1.5 NRB signal data as described below.
We have processed almost 3 consecutive years of data starting from March 2019 and going to December 2022, collected over the KAUST observation site and including vertical profiles of volume depolarization provided by MPLNET lidar in combination with co-located AERONET observations, notably the L1.5 total optical thickness and raw almucantars available at https://aeronet.gsfc.nasa.gov/new_web/webtool_aod_v3.html (last access: 18 July 2024) and https://aeronet.gsfc.nasa.gov/new_web/webtool_inv_v3.html (last access: 18 July 2024), respectively, using Version 1.1.1 of the GRASP software. All available AERONET and MPLNET V3 Level 1.5 NRB signal data were processed at once, taking into account the non-simultaneity of data acquisition by lidar and sun photometer. More specifically, a so-called multi-pixel (Dubovik et al., 2011, 2014, 2021; Lopatin et al., 2021) approach was used. In this methodology additional a priori limitations on time variability in aerosol parameters were applied.
Additionally, a recently established concept of aerosol modeling allowing us to infer some information about aerosol composition from remote sensing observation (Li et al., 2019) was used. Specifically, the so-called GRASP/Components approach, where the spectrally dependent complex refractive indices for both fine and coarse modes of aerosol are modeled using an internal mixture of different chemical components with known spectral dependencies of the complex refractive index, was used. These components include black and brown carbon; fine and coarse insoluble dust material; coarse absorbing insoluble components, mainly represented by iron oxides that are commonly present in the desert dust and determine its absorbing properties; fine and coarse non-absorbing solubles that represent anthropogenic and natural salts, notably sulfur and ammonia; and aerosol water content. A Maxwell–Garnett effective medium approximation (e.g., Schuster et al., 2016a, b, 2009, 2005) and direct volume mixture can be used to estimate the effective refractive index by combining insoluble components into a host medium that contains soluble components dissolved in water (e.g., see Fig. 1).
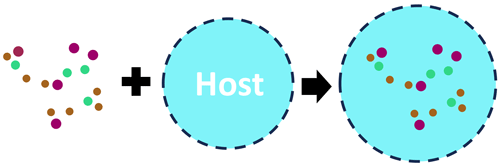
Figure 1The illustration of the general concept of modeling of the effective refractive index using the Maxwell–Garnett effective medium approximation (adapted from Li et al., 2019).
The approach has been applied to AERONET data, which has allowed the derivation of aerosol optical properties, such as AOD (aerosol optical depth), AE (Ångström exponent), size distribution and SSA (single-scattering albedo), that are fully consistent with the conventional AERONET retrieval (Li et al., 2019; Zhang et al., 2022). At the same time, this approach provides some insight about the aerosol type (e.g., desert dust, biomass burning, urban polluted or clean aerosol, sea salt). Moreover, the approach allows for identifying some variability within each type of aerosol (e.g., level of absorption, spectral dependence). It should be noted that, despite the Maxwell–Garnett approximation being exploited in the above application, the linear volume mixing approach is also realized in GRASP and demonstrates valuable features (Li et al., 2019).
In addition, the present study has also considered the possibility of using a priori knowledge on the temporal continuity of aerosol property evolution to be used as additional constraints in similar ways to those described by Lopatin et al. (2021). Specifically, a priori knowledge about the temporal continuity of aerosol property evolution was used as an additional constraint on temporal variability in the aerosol chemical composition, sphericity fraction and size distribution. All available AERONET measurements (see Table 1) collected on the preceding day (starting at noon) and following day (before noon) were used in combination with available day and night MPLNET lidar measurements of the normalized relative backscatter signal and volume depolarization. This allows for achieving improvements in the daytime AERONET and lidar retrievals for the observations close to noon, when sun-photometer observations provide rather limited constraints for the retrieval due to observation geometry with reduced coverage of scattering angles (that are usually more pronounced at low-latitude sites such as KAUST), resulting in a higher number of data observations processed. At night only lidar measurements were conducted. The measurements taken at 17:00, 20:00, 23:00 and 02:00 UTC were used.
Table 1Summary of the data and their combinations used by the GRASP multi-temporal retrieval scheme.
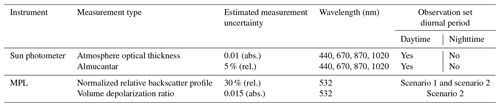
For GRASP processing, lidar signals were cropped and treated within a 270–5670 m altitude range, containing 73 vertical strobes at the MPLNET 75 m vertical resolution (https://mplnet.gsfc.nasa.gov/product-info/product_pages.cgi?p=NRB, last access: 18 July 2024). An accumulation of 15 min prior to and after the abovementioned times (including the times of evening and morning AERONET observations) was applied to the MPLNET profiles. If AERONET observations (both AOD and almucantar) were not available due to cloud contamination or any other reason, the NRB profiles around these observation times were also discarded. The newest available MPLNET V3 aerosol data of Level 1.5 were used for the comparisons. The details on data screening and quality assurance provided by the MPLNET team can be found at https://mplnet.gsfc.nasa.gov/versions.htm (last access: 18 July 2024) and https://mplnet.gsfc.nasa.gov/product-info/ (last access: 18 July 2024). Since nighttime observations cannot rely on AERONET cloud screening like daytime observations, cloud screening based on the V3 L1.0 CLD/cloud_base product was used for nighttime observations, thus effectively discarding the profiles that contained cloud base values within the altitude crop area. Observations containing unphysical values of volume depolarization (negative values of higher than 100 %) within the altitudes of interest were also discarded. The combined AERONET–MPLNET data were treated following two scenarios: excluding and including volume depolarization profiles at 532 nm, referenced below as scenario 1 and scenario 2, respectively. Accounting for volume depolarization (Welton et al., 2018) allows GRASP to use an extended aerosol microphysical model that distinguishes between some of the properties of fine and coarse aerosol particles (see Table 2 for details).
Table 2List of aerosol properties retrieved during GRASP AERONET + MPLNET inversion; parameters marked in bold are selected for the comparison.
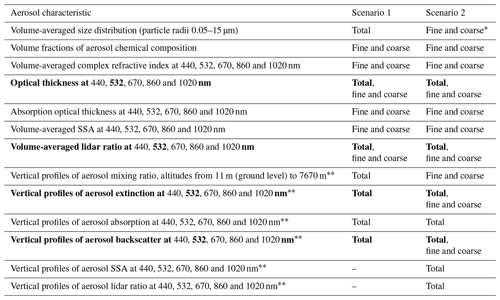
∗ The maximum radius of the fine mode is 0.57 µm; the minimal radius of the coarse mode is 0.33 µm.
The value of any aerosol property between the ground level and minimal reliable altitude of lidar measurements (270 m a.s.l.) is considered to be constant.
Table 1 summarizes instrument configurations of measurement times used for combined MPLNET–AERONET retrievals using GRASP. The details of MPLNET data preparation and combined retrievals can be found in Lopatin et al. (2021). It should be noted that values provided in the column “Estimated measurement uncertainty” do not represent exactly the uncertainty in the real observations but are used in order to weight the observations in GRASP retrieval to properly account for the uncertainty differences and information content of various types of observations. For example, sky radiances provided by AERONET observations are crucial for estimating aerosol microphysical properties that support the inversion applied to lidar profiles, while the AOD measurements give good constraints on aerosol quantity. Both of these are crucial to the correct inversion of the lidar equation (Lopatin et al., 2013, 2021); therefore AERONET-provided observations have lower estimated uncertainty in order to guarantee the convergence of the combined lidar–photometric data. All four observation uncertainties can be estimated on both a relative and an absolute scale, with observations having a substantial dynamic range (sky radiance and NRB) being estimated on a relative scale and AOD and volume depolarization (in percentage) being estimated on an absolute scale. At the same time, lidar profile uncertainty increases with range due to the lower signal-to-noise ratio, and therefore it cannot be estimated with a single value; considering this, values provided in Table 1 for NRB and volume depolarization signals represent an altitude average residual that is desired to be achieved (or surpassed) during the inversion process. It should be noted that the GRASP-weighted uncertainty values used for MPLNET lidars are significantly higher than the actual measurement uncertainties derived from signal calibrations and measurement conditions for both the signal and the volume depolarization. The actual measurement uncertainties for the data are provided by the MPLNET standard aerosol processing.
Overall, 6450 profiles for scenario 1 and 4380 profiles for scenario 2 were estimated from successfully processed combined MPLNET and sun-photometer data, covering the period of 23 March 2019–31 December 2022. The difference in the number of observations is explained by additional screening that was applied to the volume depolarization profiles, whereby the whole measurement combination was omitted for GRASP processing if no full volume depolarization profile within the altitude crop range was available after the accumulation within the ±15 min window.
Indeed, inclusion of additional observations into the retrieval can bring additional benefits only in the cases when there is sufficient information on aerosol properties. In this regard, both high and low volume depolarization ratios could be useful in providing information on properties of either coarse non-spherical particles or spherical particles (both fine and coarse). However, lidar observations with a low volume depolarization ratio can suffer from a significantly lower signal-to-noise ratio for the values that are close to 0. Therefore, quality assurance on volume depolarization provided by MPLNET L1.5 NRB data allows exclusion of such cases from the retrieval, assuring high-quality extended retrievals even in cases without significant dust loads.
Table 2 summarizes the estimations of aerosol properties provided by GRASP synergetic retrievals of the total atmospheric optical depth and sky radiance measurements in almucantar geometry from the sun photometer at four (440, 670, 870 and 1020 nm) wavelengths in combination with the normalized relative backscatter (NRB) and volume depolarization ratio at 532 nm using the approach described above.
While GRASP and MPLNET methodologies have several similar features, as was mentioned above, they have a significant number of differences as well. For example, several major differences in the data treatment between GRASP and MPLNET should be outlined. First of all, GRASP performs the inversion of both datasets simultaneously, allowing the lidar signal to influence the photometric retrievals and vice versa, while MPLNET retrieval relies on AOD to constrain the solution of the Fernald equation, which has to be interpolated into the operating wavelength of the lidar (532 nm), rendering the cross-influence of two observation types impossible. Secondly, GRASP, despite initially emerging from AERONET retrieval, features a number of changes that have accumulated over years of development, including radiative transfer optimizations and the inclusion of a chemical-component retrieval option that replaces the direct retrieval of complex refractive index values, like in a “classic” AERONET retrieval approach, with retrieval of the fraction of the components with known spectral dependencies of the refractive index, as well as the possibility of realizing a multi-pixel approach.
Nonetheless, the usage of exactly the same lidar signal datasets as an input for both approaches and the provision of directly comparable products in the form of vertical distributions of aerosol extinction and backscatter, as well as of vertically averaged columnar optical thickness and lidar ratio at 532 nm, open up a unique opportunity for inter-comparison of these intrinsically different methodologies. Also, both algorithms rely on the temporal limitation of key aerosol properties in order to be able to treat nighttime data, for which no sun-photometric observations were available for this site. In addition, the MPLNET aerosol data are provided continuously on a 1 min grid as described above, utilizing an alternative method to estimate AOD and LR between available AERONET observations. Indeed, AOD in a standard AERONET configuration is provided approximately every 15 min, while the almucantar inversions which provide estimations of microphysical properties (size distribution, complex refractive index and sphericity fraction) that are required to estimate the columnar lidar ratio are performed only 8 to 10 times a day. It should be noted that MPLNET retrieval allows for using lunar AOD during the nighttime; however these observations are not always available due to the changes in the lunar phase (Barreto et al., 2016) or due to the instrumentation limitations. As mentioned above, the AERONET station at the KAUST site was not equipped with a robotic photometer capable of performing lunar AOD observations; therefore a standard procedure (https://mplnet.gsfc.nasa.gov/product-info/product_pages.cgi?p=AER, last access: 18 July 2024) was used to estimate diurnal variations in AOD and LR with a 1 min time resolution.
The MPLNET aerosol retrievals are generated using two methods specified by the nature of the AOD. Observation times with available co-located AERONET AOD (daytime or lunar) utilize the retrieval approach described above. The lidar calibration constant, C, is also calculated during the retrieval using the independently measured AOD (Welton et al., 2002). This produces a discrete number of C values per day from available daytime and lunar observations. A continuous 1 min gridded C variable is constructed by linearly interpolating between each discrete C value. For observations between AERONET measurements, the C value and the aerosol top height are used to calculate an effective column AOD from the lidar data and the molecular background. This requires a 1 km cloud-free layer 500 m above the top of the aerosol (the calibration zone). The AOD is then used as input to the same retrieval algorithm as that used for the co-located AERONET retrievals. This process produces three types of aerosol data in the MPLNET product: retrievals constrained by daytime AERONET AOD, lunar AERONET AOD or interpolated AOD. These are combined together in the 1 min re-gridded data variables, with quality flags available to discriminate the AOD utilized. Confidence flags are also provided, with the interpolated data having the lowest confidence and daytime AERONET data the highest. More details on the approach are available at https://mplnet.gsfc.nasa.gov/product-info/product_pages.cgi?p=AER (last access: 18 July 2024). For this study with no lunar AOD available, the standard MPLNET aerosol data from the nighttime are only those of the lowest confidence quality.
The GRASP approach on the other hand prioritizes photometric retrievals by selecting and accumulating lidar profiles in the vicinity of the available almucantar observations; however, without access to lunar AOD observations, it relies on limiting the time variation in the retrieved columnar aerosol properties, such as the sphericity fraction, size distribution and chemical composition, to be able to treat lidar profiles during the nighttime, when no coincident sun-photometric observations are available. In addition, such a limitation has to be fruitful in photometric retrievals close to noon, providing an additional constraint for the observations that usually lack some information due to the narrower range of scattering angles.
The MPLNET data (profiles of extinction and backscatter and columnar AOD and LR at 532 nm) were selected for the same times as GRASP retrievals, which during the daytime are driven by almucantar measurement times and at night are performed at 17:00, 20:00, 23:00 and 02:00 UTC. It should be noted that MPLNET processing uses the 20 min cloud-screened averages to provide a profile for each minute, which is comparable with 30 min profile averaging performed for GRASP retrievals, mentioned in Sect. 2. The selected profiles are compared in a bin-to-bin manner, guaranteeing comparison of the values provided for the same altitudes. Profiles that had fewer than 35 vertical bins in the altitude range of interest in the MPLNET V3 L1.5 AER product were omitted completely from the comparison. Additional quality assurance on GRASP-provided products could be applied in order to exclude values that corresponded to the retrievals that did not achieve expected levels of measurement observation accuracies (see Table 1).
Overall, 1904 out of 6450 profiles for scenario 1 and 972 out of 4380 profiles for scenario 2 are quality-assured from successfully processed combined MPLNET and sun-photometer data, corresponding to the filtering rates of 30 % and 22 %.
The comparison of aerosol properties provided by GRASP and MPLNET products is organized in the following way: a separate analysis is performed for daytime and nighttime property estimations; within each diurnal group, both columnar properties and their vertical distributions will be analyzed. It should be additionally noted that the MPLNET product has several levels of confidence, and using the same daytime data as GRASP that correspond to the time of combined AOD plus almucantar observations, we select the data with no AOD time interpolation, and therefore we operate with the best-quality data that are sun-photometer constrained.
At the same time, since no lunar AOD measurements were available at the KAUST AERONET site, the MPLNET data selected for comparison during the nighttime rely on “long-term” interpolation of AOD and therefore are the least assured. Meanwhile, GRASP nighttime retrievals rely on smoothness restrictions of time variation in columnar microphysical properties of aerosol, which gives a rather comparable yet different approach to constraining nighttime lidar retrievals.
4.1 Comparison of columnar properties
For adequate comparison of GRASP and MPLNET aerosol products, it is reasonable to start with the comparison of columnar properties, notably AOD and LR at 532 nm. Both of these values are not included into the state vector describing the aerosol properties that is optimized during GRASP retrievals (Lopatin et al., 2013, 2021), and both AOD and LR values are estimated on the basis of physical modeling including retrieved size distribution, sphericity and chemical composition. The estimations of columnar values of AOD and LR are crucial in GRASP estimations of profiles of vertical distributions of aerosol extinction and backscatter, which are performed in the following manner:
where N denotes the number of aerosol modes; τi(λ) denotes aerosol optical depth of the corresponding mode; Si denotes the lidar ratio of the corresponding aerosol mode at 532 nm, defined by Eq. (3); and vi(h) denotes the normalized aerosol vertical distribution profile of the corresponding mode. Since normalized vertical distribution profiles vi(h) are mostly influenced by lidar observations (Lopatin et al., 2013) and overall will be formed by the NRB profile, having the same columnar values for AOD and LR is crucial for GRASP to provide profiles of extinction and backscatter that are similar to those of MPLNET.
It should be noted that Eqs. (4) and (5) can be used in a situation when aerosol model is represented by several modes, e.g., fine and coarse, like in scenario 2 retrievals analyzed in this study. Figure 2 shows the results of the comparison of daytime AOD estimations provided by GRASP and MPLNET for two types of GRASP retrievals – excluding and including the volume depolarization data provided by MPLNET. The comparison is exceptionally good due to the fact that during the daytime, MPLNET retrieval utilizes AOD observations as constraints. Since GRASP inverts combined AOD and almucantar data, the time windows selected for the comparison should contain the same AOD observations as those performed by sun photometer. The observable differences are caused by the differences in the estimation of AOD at 532 nm, which is not directly observed by the sun photometer. In the case of MPLNET, it is done by second-order polynomial fitting of spectral AOD observed in the case of the standard AERONET sun photometer at 440, 675, 870 and 1020 nm, while GRASP relies on the common physical model, including aerosol chemical composition that satisfies all types of observations included in the retrieval (see Table 1 for details). Despite these differences, the statistical properties of the comparisons are exceptionally good, with correlation coefficients of 0.990 and 0.972 for scenarios 1 and 2, respectively; RMSE of 0.022 and 0.038; slope close to 1; and no biases at different ranges of AOD values.
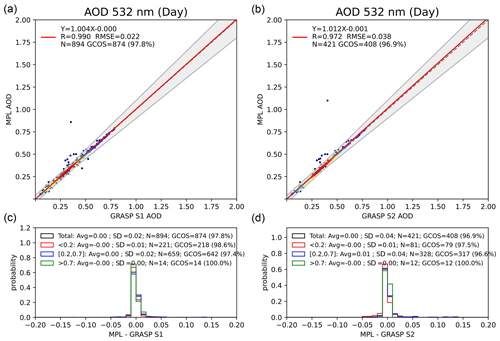
Figure 2Comparison of daytime columnar aerosol optical depth at 532 nm retrieved by GRASP and MPLNET over the KAUST observation site for the period 2019–2022, for scenario 1 (a, c) and scenario 2 (b, d) GRASP retrievals.
The slight differences between scenarios 1 and 2 are most likely related to the differences in the dataset used for the comparison, as additional requirements to the volume depolarization profiles exclude some of the data from processing using scenario 2 which nonetheless could be present in scenario 1, giving 4380 and 6450 profiles, respectively. Such additional filtering may solely be responsible for the improvement in comparison statistics by excluding the low-quality data that could still be present in NRB profiles, making the retrievals more accurate.
Another possibility is the use of the aerosol model with higher flexibility in scenario 2, which distinguishes the properties of fine and coarse aerosol particles and has double the number of parameters that can be used to reproduce observations compared to scenario 1. Specifically, in scenario 2 the properties of aerosol at each layer depend on the concentrations of fine and coarse particles, while LR is fixed for each fraction for the entire column; at the same time, LRs for fine and coarse particles are different. In scenario 1, the lidar signal is fit by the model that retrieves LR and values of total aerosol at each layer. Therefore, scenario 2 operates with a more flexible aerosol model and allows us to fit lidar observations more accurately under the same total AOD constraints.
Overall, 91 % and 90 % of GRASP quality-assured daytime AODs lie within the error intervals provided in the aerosol MPLNET L1.5 V3 product.
Figure 3 compares the columnar lidar ratio at 532 nm between four available products: GRASP scenario 1 and scenario 2, MPLNET LR, and LR from standard AERONET processing. Similarly to AOD comparison in Fig. 2, the LR values are selected for the moments when AOD measurements were provided, thereby guaranteeing the best quality for MPLNET LR estimations. Nonetheless, the results of the comparison demonstrate lower statistical results (summarized in Table 3) than those for AOD due to the different approaches used by MPLNET and GRASP to estimate LR. While MPLNET uses a modified Fernald (Welton et al., 2002; Marenco et al., 1997; Fernald, 1984) algorithm with AOD-provided calibration to effectively estimate columnar AOD and LR from the NRB lidar signal, GRASP relies on both angular dependencies of aerosol properties provided by almucantar observations and normalized attenuated backscatter as well as, in the case of scenario 2, volume depolarization profiles provided by MPLNET to estimate columnar microphysical properties of aerosol that are then used to estimate LR (Dubovik and King, 2000; Dubovik et al., 2006). It should be additionally noted that in the case of scenario 2, GRASP operates with two columnar LRs estimated for fine and coarse aerosol modes, respectively, with an effective total LR estimated following Eq. (3). The denominator in Eq. (3) in the case of several aerosol modes can be estimated as follows (Dubovik et al., 2011):
All four products provide close LR values with average bias not exceeding 10 sr.
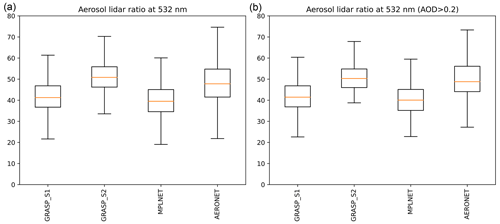
Figure 3Comparison of the daytime columnar lidar ratio at 532 nm retrieved by GRASP (scenarios 1 and 2) and MPLNET and estimated from AERONET retrievals over the KAUST observation site for the period of 2019–2022, including (a) and omitting (b) low AODs>0.2.
As seen in Fig. 3, both MPLNET and GRASP scenario 1 (S1) estimate LR at 532 nm as ∼40 ± 5 sr, which is slightly lower than the typical ranges for desert dust (e.g., Welton et al., 2002; Muller et al., 2007; Schuster et al., 2012; Papayannis et al., 2008; Li et al., 2022). It should be noted that variability in retrieved LR is quite low, due to the dominance of desert dust usually present over the KAUST site. Certainly, this limited variability range decreases the correlation and slope values.
Regarding scenario 1 (see Fig. 3), both the MPLNET and the GRASP approaches are closer due to their similarity in aerosol assumptions; notably both methods operate with only one columnar value of LR and use the same AERONET spheroidal model for the description of properties of non-spherical particles.
In the case of scenario 2 (S2), a more pronounced discrepancy could be observed, and MPLNET data have a notable bias of −14.2 sr as compared to GRASP S2 estimations. In scenario 2, GRASP estimations of LR are slightly higher, at ∼50 ± 5 sr. These observed differences are most likely present due to the possibility of columnar LR variations due to the presence of the second mode. At the same time GRASP-S2-estimated and AERONET-estimated LR show the closest values, with a small difference of ∼6 sr between the GRASP product and AERONET estimations. Overall AERONET-estimated values are ∼48 ± 7 sr.
Both GRASP S1 and S2 demonstrate quite low RMSEs of around 11 and 19 sr, respectively, as compared to MPLNET values. Overall, 28 % and 5 % of GRASP quality-assured daytime LRs for scenarios 1 and 2, respectively, lie within the error intervals provided in the aerosol MPLNET L1.5 V3 product.
The right panel of Fig. 3 compares the retrievals of columnar LR for scenarios 1 and 2 but with additional filtering that omits the retrieval cases with low AODs, below 0.2. Indeed, such filtering has proven to be very useful for comparing derived aerosol products such as the Ångström exponent or single-scattering albedo (SSA) (e.g., Wagner and Silva, 2008; Chen et al., 2020), allowing us to effectively exclude cases with weak aerosol contribution to the atmospheric observation and therefore with lower quality of retrievals. The filtered results show similar mean values of LRs, with noticeably lowered variation in GRASP values in scenario 2. Scenario 2 also demonstrates lower RMSE after filtering, being 17.14 instead of 19 sr, while scenario 1 remains almost the same (∼11 sr). At the same time, scenario 1 and MPLNET data in relation to AERONET-estimated values have comparable performance, with overestimation of ∼7 and ∼9 sr, respectively. Generally, both GRASP S2 and AERONET LR estimations provide reasonable values given that the expected aerosol type over the KAUST site is dust. In this regard, usage of volume depolarization in scenario 2 in contrast with scenario 1 should have provided additional sensitivity to dust properties, leading to a more stable retrieval.
It should be noted that it is expected that all methods will demonstrate better agreement in daytime extinction profiles. Indeed, all methods are constrained by AOD observations for both GRASP and MPLNET. At the same time, backscatter profile comparison results rely on how close the estimations of columnar lidar ratios provided by these methods are. The differences in LR estimations observed in Fig. 3 could have different origins. For example, GRASP S2 used VLDR data that provide additional information that is expected to improve the retrievals. However, the accurate interpretation of VLDR requires a reliable model of non-spherical aerosol scattering properties. The approach of randomly oriented spheroids developed by Dubovik et al. (2006) has been used in all four methods (GRASP S1 and S2, MPLNET, and AERONET). While spheroid mixture is evidently an idealistic modeling approach, it has been shown to be efficient in many applications for the quantitative characterization of intensity and polarization scattering properties of non-spherical particles in wide angular and spectral ranges. Indeed, spheroids have been successfully employed in passive AERONET ground-based (Dubovik et al., 2006) and spaceborne multi-angular polarimeters (Dubovik et al., 2011, 2021; Hasekamp et al., 2024); in extensive complex datasets of observations including in situ ones (Espinosa et al., 2017, 2019; Bazo et al., 2024), as well as in active measurements (e.g., Lopatin et al., 2021); and in various combinations of active and passive remote sensing observations, both ground-based (e.g., Lopatin et al., 2013, 2021) and spaceborne (Xu et al., 2021). At the same time, MPLNET products used in this study do not rely on volume depolarization observations, and hence they provide extinction and backscatter profiles together with columnar lidar ratio without any relation to a spheroid model.
Taking this into account, it will be of particular interest to analyze in detail the GRASP–MPLNET diurnal comparisons, e.g., during a time period of 1 or 2 d in order to assess the possible impact of modeling assumptions on columnar lidar ratio estimations and hence the extinction and backscatter profiles. It should be emphasized though that analysis of a 1 d period cannot be very profound and might represent a particular case that is not typical of the majority of observations. Figure 4 shows an example of the time sequence of AODs and lidar ratios provided by GRASP scenarios 1 and 2 and MPLNET retrievals for the period of 21 September 2022. The nighttime period is shaded in blue; MPLNET estimations are presented in black; GRASP estimations are plotted in green and red for scenarios 1 and 2, respectively; and AERONET-provided estimations of AOD at 532 nm (interpolated from the 440/670 nm Ångström exponent) and LR calculated on the basis of retrieved microphysical properties (including the size distribution, complex refractive index and spherical particle fraction) are shown in blue. It should be noted that MPLNET provides the data for each minute (with a 20 min sliding window), while GRASP uses 15 min lidar data accumulation (totaling a 30 min accumulation) around available combined AOD–almucantar measurements performed by AERONET during the daytime and around 02:00, 20:00 and 23:00 UTC at nighttime (shown in rounds). As can be clearly seen, similarly to the right part of Fig. 2, the AOD daytime comparison is exceptionally good between all four products. Such an outcome is expected, since GRASP directly uses AOD values to fit with sky radiance and lidar data; at the same time MPLNET uses AOD provided by AERONET to constrain its retrievals. Similarly to Figs. 3 and 4, the behavior of time evolution could also be observed for the lidar ratio estimations. The estimations performed during the daytime are closer than during the nighttime, though a significant difference could be observed between scenario 1 and scenario 2, covering a range of almost 30 sr. Overall MPLNET and AERONET LR estimations demonstrate higher variability, with both AERONET and MPLNET values being overall closer to scenario 1 during daytime observations.
4.2 Comparison of vertical profiles
Figure 5 shows the results of a layer-to-layer comparison of daytime estimations of vertical extinction profiles provided by GRASP and MPLNET for two types of GRASP retrievals – excluding and including the volume depolarization data provided by MPLNET. Both methods show very good agreement, with scenario 1 having slightly better agreement due to the bigger similarities between the GRASP and MPLNET approaches, notably the use of only one aerosol mode distributed within a single vertical profile. The correlation coefficients are 0.980 and 0.975 for scenario 1 and scenario 2, respectively. RMSEs are very low, not exceeding 16 Mm−1, and linear regression slopes are exceptionally good, being 0.85 and 0.84, respectively. Overall, both methods do not have significant biases against each other, with these parameters no lower than −5.94 and −5.43 Mm−1 for scenarios 1 and 2, respectively, and the majority of differences located within the range of −50 to 25 Mm−1. Overall, 86 % and 85 % of GRASP quality-assured vertical extinction profile values are within the error margin provided for this parameter in the aerosol MPLNET L1.5 V3 product for scenarios 1 and 2, respectively.
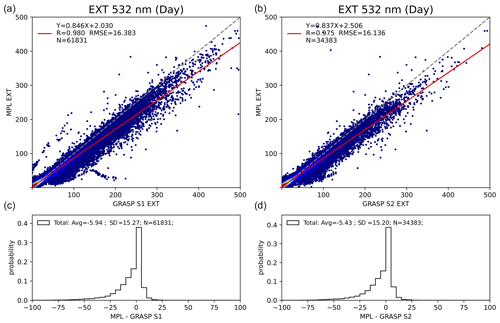
Figure 5Layer-to-layer comparison of daytime aerosol vertical extinction profiles at 532 nm retrieved by GRASP and estimated by MPLNET over the KAUST observation site for the period of 2019–2022, for scenario 1 (a, c) and scenario 2 (b, d) GRASP retrievals.
It is worth mentioning that according to the diagram in Fig. 6, GRASP generally provides lower values for the extinction profiles than the ones provided by MPLNET. The reason for this discrepancy may lie in the differences in the aerosol profile treatment implied by both methods. Indeed, both methods provide a vertical profile of extinction whose integration provides almost identical columnar AOD values (see Fig. 2). The main difference is the integration ranges and the extra assumptions made to perform it. MPLNET lidar signals are provided from 250 m to 30 km above the ground in the NRB products for all instruments at 532 nm (the lower limit was higher for older instruments and fixed at 527 m). MPLNET aerosol processing first determines the aerosol top height as described above, and the bottom of the calibration zone serves as the upper range limit for aerosol retrievals. The bottom limit is the surface, and lidar signals below 250 m are filled in as a constant using the signal value just above 250 m. At the same time, GRASP extrapolates the aerosol profile outside the range of 270–5670 m by assuming aerosol to be constant from the lower limit to the ground level and linearly decreasing up to the altitudes of 40 km and starting from the upper limit (Lopatin et al., 2013). Thus, a comparison within the limited altitude range leaves some parts of a wider bottom-to-top profile behind, effectively lowering this part of the profile, since in GRASP retrievals the omitted parts still contribute to the columnar AODs, which may not be fully accounted for by MPLNET.
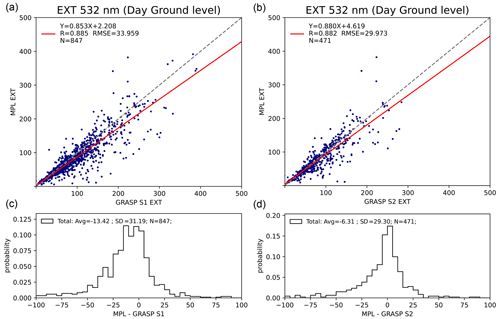
Figure 6Comparison of daytime aerosol extinction at 532 nm at ground level estimated by GRASP and MPLNET over the KAUST observation site for the period of 2019–2022, for scenario 1 (a, c) and scenario 2 (b, d) GRASP retrievals.
In order to clarify the above aspect, an additional comparison of the aerosol extinction estimated at the ground layer was performed in order to investigate how well an assumption of a constant aerosol distribution or lidar signal at lower layers, notably in the lidar cutoff zone, affects the estimation of this value. Such a comparison is reasonable to perform for daytime observations, where both columnar AOD and vertical extinction profiles demonstrate outstanding agreement (see Figs. 2 and 6), thus allowing us to limit the influence of other factors that affect the estimates of the ground level extinction to the differences between approaches used to estimate extinction in both products.
Figure 6 illustrates the comparison of aerosol daytime extinction at 532 nm in the lowest-altitude layer provided by GRASP and MPLNET products, located approximately at 50 m above sea level. It should be noted that these values are not supported by lidar observations but rather are estimated using the constraining of total columnar AOD and assumptions described above. Following the general logic of the comparison, GRASP products excluding and including information on volume depolarization provided by MPLNET are presented. As can be seen in Fig. 6a, the rather simple assumption about aerosol distribution made in GRASP still allows us to estimate ground level extinctions rather accurately, with RMSEs not exceeding 38.3 and 43.5 Mm−1, correlation coefficients of 0.89 and 0.88, impressive linear regression slopes of 0.85 and 0.88, and average biases not exceeding −13.4 and −6.3 Mm−1 for scenarios 1 and 2, respectively. It should be noted that scenario 2 uses two vertical distribution profiles separated between fine and coarse modes, which provides it with an additional flexibility in describing the total aerosol extinction at the ground level, which in turn may explain why the values of slope and bias are better in the case of comparison with scenario 2.
Figure 7 shows results of the layer-to-layer comparison of daytime estimations of vertical backscatter profiles provided by GRASP and MPLNET for two types of GRASP retrievals – excluding and including the volume depolarization data provided by MPLNET. Both methods show very good agreement, with scenario 1 having slightly better agreement due to the bigger similarities between GRASP and MPLNET approaches, notably the use of only one aerosol mode distributed within a single vertical profile. The correlation coefficients are 0.96 and 0.95 for scenario 1 and scenario 2, respectively; RMSEs are very low, not exceeding 0.53 and 0.8 , respectively; and linear regression slopes are 0.8 for scenario 1 and slightly lower (0.65) for scenario 2. This most likely is related to the differences in columnar LR estimations discussed above; additionally, the presence of the second vertical profile, providing a more detailed distribution of GRASP scenario 2 LR vertically as compared to MPLNET retrievals may impact the comparison. Scenario 1 has very low negative bias (−0.18 ), following the trends of extinction profile and columnar LR estimations (see Eq. 5). A low (−0.41 ), but observable, bias is present in scenario 2, similarly to scenario 1 propagating into the backscattering estimations from vertical extinction profiles and columnar LR comparison differences.
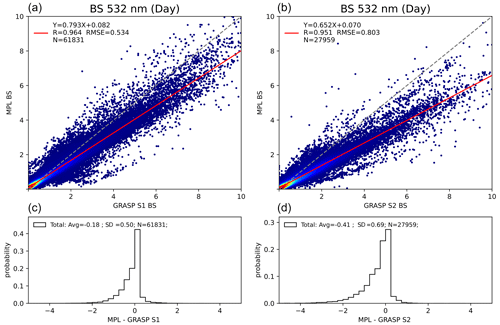
Figure 7Layer-to-layer comparison of daytime aerosol vertical backscatter profiles at 532 nm retrieved by GRASP and estimated by MPLNET over the KAUST observation site for the period of 2019–2022, for scenario 1 (a, c) and scenario 2 (b, d) GRASP retrievals.
Overall, 81 % and 69 % of GRASP quality-assured daytime vertical backscatter profile values are within the error margin provided for this parameter in the aerosol MPLNET L1.5 V3 product for scenarios 1 and 2, respectively.
Similar to Figs. 5 and 7, the daytime comparison of profiles of aerosol extinction and backscatter provided for GRASP scenarios 1 and 2 and MPLNET at 532 nm for 21 September 2022 shown in Fig. 8 are very encouraging. Small biases that could be observed in backscatter profiles are due to the differences in lidar ratio estimations (see the right panel of Fig. 4) used in different scenarios of GRASP and MPLNET L1.5 retrievals. Significant differences could sometimes be observed in the lower part of the profiles, which are located in the cutoff zone of the MPL. This, however, does not have large significance for the overall comparison for the ground-based concentration levels shown in Fig. 6. It should be noted that for this particular case, the signal top cutoff in GRASP and MPLNET treatment is slightly different, with MPLNET reaching 6000 m altitudes. This creates some discrepancy in the estimation of extinction at the highest altitude (∼5700 m) between both GRASP products and MPLNET profiles. Since the value of the top layer is extrapolated to the top of the atmosphere (TOA), this may cause some observable bias between different products, with AOD values nonetheless being exactly the same (see, e.g., Fig. 4). Indeed, MPLNET, unlike GRASP, allows lidar signal top cutoff to vary with time, and a similar approach will be applied to GRASP processing of MPLNET data to avoid such discrepancies in the future.
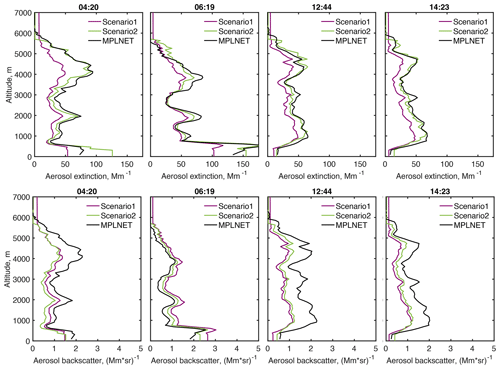
Figure 8Comparison of profiles of aerosol extinction (top) and backscatter (bottom) at 532 nm retrieved by GRASP for scenario 1 (purple) and scenario 2 (green) with the MPLNET-provided aerosol product (black) for the daytime period of 21 September 2022.
Additionally, it should be emphasized that, unlike the majority of the comparison cases presented in Fig. 5, where very little difference could be observed between estimations provided by both scenarios, scenario 2 for the case on 21 September demonstrates better accordance with MPLNET-provided extinction profiles, and backscatter comparisons differences directly propagate from biases of columnar LR estimations for GRASP S1 and S2 and MPLNET.
Comparing Figs. 8 and 4, representing the estimations of the total columnar lidar ratio by four different methods (AERONET, MPLNET, GRASP S1 and S2), it is clearly seen that once the extinction is constrained by AOD, the main difference in profiles originates from the total columnar LR estimation difference and propagates into the backscatter profiles. As discussed in Sect. 4.1, there are several differences in the modeling approach between the four methods. Three of them (AERONET, GRASP S1 and S2) utilize the same spheroidal particle assumption to model non-spherical scattering, which provides a rather broad range of total columnar lidar ratios to reproduce diverse observations (Dubovik et al., 2006; Lopatin et al., 2021). For example, in the same way as in similar studies by Lopatin et al. (2021), the spheroid model demonstrated the ability to provide adequate fits for all available observation data on 21 September 2022 within the expected accuracy of each observation (see Table 1). Those included spectral AOD (daily average residual 0.0018 and 0.0035 for S1 and S2, respectively), sky radiances in almucantars (daily average residual 4.44 % and 4.46 % for S1 and S2, respectively), attenuated backscatter (daily average residual 1.32 % and 3.42 % for S1 and S2, respectively) and volume depolarization at 532 nm (daily average residual 0.9 % for S2), and derived LR 532 nm values closer to literature-based expectations for desert dust as compared to the LR used in MPLNET retrievals. In addition, it should be noted that the impact of utilizing the spheroidal aerosol model could not be isolated from other factors that significantly influence the retrievals. Namely, differently from the MPLNET approach, GRASP accounts for aerosol in the total atmospheric column and not only in the part observed by lidar (e.g., see inconsistencies in profile estimations above 6000 m in Fig. 8), and in GRASP scenario 2, aerosol is represented by two aerosol modes. In addition, the temporal restrictions on variability in aerosol columnar properties do not allow sharp temporal variations in LR in both GRASP retrievals that, in contrast, could be observed in MPLNET retrievals (see, e.g., ∼ 06:00 and ∼ 12:00 UTC values in the right panel of Fig. 4). Such analysis remains out of the scope of this study and could be performed in the future should additional data (e.g., coincident LR retrievals from Raman lidars) become available.
This section presents comparisons of retrieved columnar and vertical properties of aerosol from MPLNET and GRASP during the nighttime. It should be additionally noted that during the nighttime, both methods do not rely on they any photometric observations due to the lack of lunar AOD at the KAUST site, and use completely different methods to estimate the values of aerosol properties. Without lunar AOD from AERONET, nighttime MPLNET estimations are performed from lidar observations only and do not rely on any spectral interpolation as compared to daytime retrievals, being the least assured data in the MPLNET V3 L1.5 dataset. GRASP on the other hand estimates columnar aerosol properties due to a combination of consecutive lidar observations combined with sun-photometric measurements performed during the daytime under an assumption of limited change in aerosol columnar properties over time (see Lopatin et al., 2021, for details). As a matter of fact, the KAUST observation site, which is dominated by one aerosol type and provides quite a stable temporal aerosol load (Parajuli et al., 2020), is more than suitable for the retrievals under such assumptions. However, the multi-temporal approach used in GRASP is not limited only to stable aerosol situations, as was demonstrated by Lopatin et al. (2021).
5.1 Comparison of columnar properties
Figure 9a and b show the results of the comparison of nighttime AOD estimations provided by GRASP and MPLNET for GRASP retrievals according to scenarios 1 and 2, respectively. The comparison is less convincing as compared to the daytime retrievals (see Fig. 2). At the same time, taking into account that during nighttime both methods do not rely on any AOD observations as compared to the daytime and, overall, use completely different methods to estimate the AOD values, this comparison is more than encouraging.
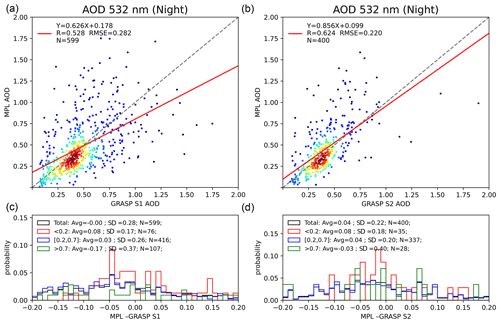
Figure 9Comparison of nighttime columnar aerosol optical depth at 532 nm retrieved by GRASP and MPLNET over KAUST observation site for the period 2019–2022 over the KAUST observation site for the period of 2019–2022, for scenario 1 (a, c) and scenario 2 (b, d) GRASP retrievals.
Despite these differences the statistical properties of the comparisons are inspiring, with correlation coefficients of 0.53 and 0.62, RMSE of 0.282 and 0.22, and slope values of 0.62 and 0.85 for scenarios 1 and 2, respectively. Total biases are low, 0 and 0.05, respectively, with the same biases at low AOD (<0.2) of 0.08. The slight differences between scenarios 1 and 2 are most likely related to the differences in the dataset used for the comparison, as additional requirements of the volume depolarization profiles exclude some of the data from processing when using scenario 2 which nonetheless could be present in scenario 1. Such additional filtering may be responsible for the improvement in comparison statistics: excluding low-quality data that could still be present in NRB profiles makes retrievals of scenario 1 less accurate. Another possibility is higher flexibility of the aerosol model used in scenario 2, which distinguishes between properties of fine and coarse aerosol particles and therefore operates with a more flexible set of retrieval parameters, allowing more accurate retrievals. Overall, 5 % and 6 % of GRASP quality-assured nighttime AODs for scenario 1 and scenario 2, respectively, lie within the error intervals provided in the aerosol MPLNET L1.5 V3 product.
Figure 10 shows the results of the comparison of nighttime LR estimations provided by GRASP and MPLNET for two types of GRASP retrievals – excluding and including the volume depolarization data provided by MPLNET. Similarly to the daytime, both MPLNET and GRASP S2 estimate LR at 532 nm of around ∼52 ± 6 sr, which is within the typical ranges for desert dust, with GRASP S1 providing slightly lower values of ∼40 ± 7 sr. As already noted above, the variability in retrieved LR is quite low due to the dominance of desert dust, which leads to the lower correlation and less stable slope values, rendering linear fit metrics to be less helpful than in the AOD cases presented.
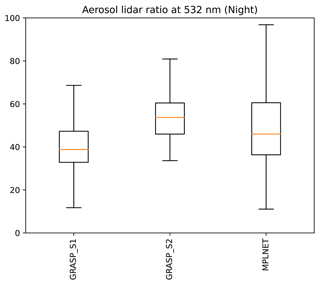
Figure 10Comparison of nighttime columnar lidar ratio at 532 nm retrieved by GRASP (scenarios 1 and 2) and MPLNET over the KAUST observation site for the period 2019–2022.
In general, the nighttime statistics of columnar LR comparison at 532 nm is very similar to those of the daytime (see Fig. 3). For scenario 1, both MPLNET and GRASP approaches are closer due to the similarity in aerosol assumptions. Both scenarios demonstrate a wider spread as compared to daytime retrievals. Consecutively, both scenarios demonstrate higher RMSEs as compared to the daytime of around 22.8 and 12.3 sr, respectively. Both GRASP retrievals demonstrate similar spreads as compared to MPLNET, notably due to the use of physical model to estimate LR with the parameters additionally limited in temporal variation. Also, a bigger discrepancy could be observed, with both GRASP S1 and S2 and MPLNET values showing a notable bias of −7 and 9 sr, respectively, as compared to GRASP estimations from both scenarios. Similarly to the daytime, in scenario 2 GRASP estimations of LR are slightly higher, being ∼52 ± 5 sr. These observed differences are most likely present due to the possibility of columnar LR variations due to the presence of the second mode. Overall, 8 % and 7 % of GRASP quality-assured nighttime LRs for scenarios 1 and 2, respectively, lie within the error intervals provided in the aerosol MPLNET L1.5 V3 product.
A more detailed analysis, performed for the nighttime period of 21 September 2021, can be seen in the blue-shaded areas of Fig. 5. The AOD comparisons shown in Fig. 10, similarly to the overall ones, are quite encouraging. While this is expected for the daytime data, since the AOD measurements are included in the GRASP retrievals, it is not the case for the nighttime, where no AOD data were used. At the same time, an observable bias (up to ∼0.1) in nighttime AOD estimations can be seen between scenario 1 and scenario 2, which becomes higher in the middle of the night. Its presence is explained by the additional restrictions on columnar AOD caused by the necessity to fit volume depolarization profiles, which may make the smoothness restrictions applied to aerosol concentration less important for scenario 2. It could also be observed that GRASP AOD estimations for both scenarios, being restricted by time variability, are quite smooth, while the data provided by MPLNET (derived from lidar observations, as indicated) undergo significant variations, most likely due to the time interpolation methods that are used to provide lidar calibration in between the available AOD observations provided by sun photometer. Since no lunar AOD is available to stabilize the temporal interpolation, these assumptions are likely to accumulate significant errors overnight. Similar behavior of time evolution is also observed for the lidar ratio estimations. While estimations performed during the daytime are close, some significant differences may be observed during the night.
5.2 Comparison of vertical profiles
Figure 11 shows the results of the layer-to-layer comparison of nighttime estimations of vertical extinction profiles provided by GRASP and MPLNET for two types of GRASP retrievals – excluding and including the volume depolarization data provided by MPLNET. Both methods show good agreement, with scenario 1 having slightly better agreement due to the bigger similarities between GRASP and MPLNET approaches, notably the use of only one aerosol mode distributed within a single vertical profile. The correlation coefficients are 0.774 and 0.784 for scenario 1 and scenario 2, respectively, with similar RMSEs not exceeding 43 Mm−1, and linear regression slopes are quite good: 0.65 for scenario 1 and slightly higher (0.70) for scenario 2. This most likely can be explained by the presence of the second vertical profile, which provides a more detailed distribution of aerosol vertically as compared to MPLNET retrievals. Scenario 1 has a negative bias (−7.65 Mm−1), following the trends of extinction profile and columnar LR estimations (see Eq. 5). Even lower (−5.37 Mm−1) bias is present in scenario 2 as compared to scenario 1. As compared to daytime retrievals (Fig. 3), vertical profiles of extinction show less agreement, which most likely results from nighttime AOD retrieval uncertainties through Eq. (4). Overall, 44 % and 43 % of GRASP quality-assured nighttime vertical extinction profile values are within the error margin provided for this parameter in the aerosol MPLNET L1.5 V3 product for scenarios 1 and 2, respectively.
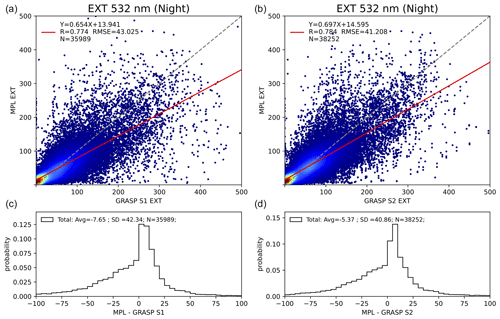
Figure 11Layer-to-layer comparison of nighttime aerosol vertical extinction profiles at 532 nm retrieved by GRASP and estimated by MPLNET for scenario 1 (a, c) and scenario 2 (b, d) GRASP retrievals.
Figure 12 shows results of the layer-to-layer comparison of nighttime estimations of vertical backscatter profiles provided by GRASP and MPLNET for two types of GRASP retrievals – excluding and including the volume depolarization data provided by MPLNET. Both methods show good agreement, with scenario 2 having a slightly better one. The correlation coefficients are 0.67 and 0.79 for scenario 1 and scenario 2, respectively; RMSEs are very low, not exceeding 1.2 and 0.8 , respectively; and linear regression slopes are quite good at 0.84 for scenario 1 and slightly lower (0.64) for scenario 2. Most likely the presence of the second vertical profile, providing a more detailed distribution of LR vertically (following Eqs. 4 and 5) as compared to MPLNET retrievals, explains the observable differences between the two scenarios. Scenario 1 has very low negative bias (−0.12 ), following the trends of extinction profile and columnar LR estimations (see Eq. 5). A low (−0.30 ), but observable, bias is present in scenario 2, similarly to daytime retrievals propagating into the backscattering estimations from vertical extinction profiles and columnar LR estimation differences (see Fig. 11), following Eq. (5).
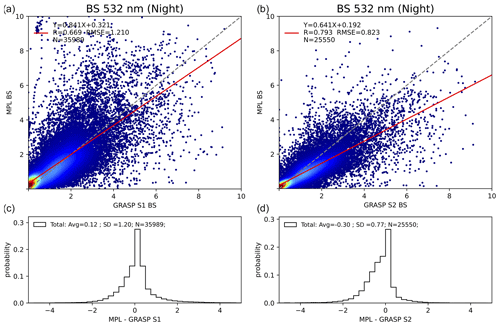
Figure 12Layer-to-layer comparison of nighttime aerosol vertical backscatter profiles at 532 nm retrieved by GRASP and estimated by MPLNET over the KAUST observation site for the period of 2019–2022, for scenario 1 (a, c) and scenario 2 (b, d) GRASP retrievals.
Overall, 44 % and 47 % of GRASP quality-assured nighttime vertical backscatter profile values are within the error margin provided for this parameter in the aerosol MPLNET L1.5 V3 product for scenarios 1 and 2, respectively.
Detailed analysis of nighttime profiles for 21 September 2022, presented in Fig. 13 and similar to the overall comparison presented in Figs. 11 and 12, shows high similarities between both scenarios' GRASP and MPLNET products and an almost exact correspondence (e.g., 17:00, 20:00 and 23:00) to slightly different profiles' magnitudes with similar shapes (e.g., 02:00). Such discrepancies evidently propagate from the differences in the estimation of AOD and lidar ratios at 532 nm that are provided by different scenarios of GRASP and MPLNET products. As can be seen in Fig. 4, even when nighttime AOD values from all three products demonstrate very close values, LR undergoes some significant shifts for all of the observations on 21 September 2022. Overall, both AOD and LR biases between scenarios 1 and 2 of GRASP products directly propagate in the backscatter profiles, taking into account that the analyzed case was dominated by coarse particles, which means that the influence of a separated fine mode in scenario 2 had limited significance. Nonetheless, for this particular period, nighttime retrievals demonstrate agreement in extinction and backscatter similar to and better than, respectively, the daytime comparison shown in Fig. 8. However, it should be noted that such behavior is not typical, as demonstrated by Figs. 5 and 7 and, correspondingly, Figs. 11 and 12.
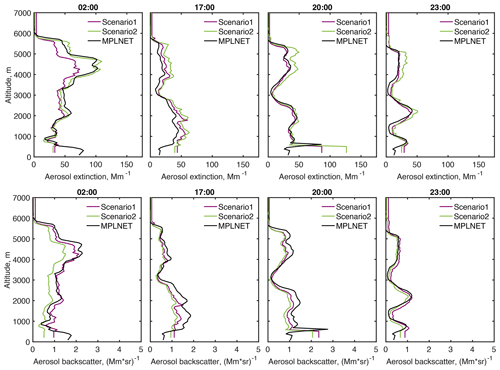
Figure 13Comparison of profiles of aerosol extinction (top) and backscatter (bottom) at 532 nm retrieved by GRASP for scenario 1 (purple) and scenario 2 (green) with MPLNET-provided aerosol product (black) for the nighttime period of 21 September 2022.
Table 3 summarizes the comparison results for GRASP- and MPLNET-retrieved parameters for columnar AOD, LR, and vertical extinction and backscatter at 532 nm retrieved during the day and night, for GRASP retrievals excluding and including the volume depolarization data.
This section focuses on analyzing the differences in aerosol properties that are retrieved during the daytime and nighttime over the KAUST site during the observation period used in this study (March 2019–December 2022). Scenario 2 data were used for the analysis, since they provide the most complex aerosol modeling and allow us to separate aerosol vertical profiles into fine and coarse modes (see Table 2 for details). Due to the inclusion of vertical profiles of volume depolarizations provided by MPLNET in the retrieval, scenario 2 is expected to provide the most detailed description of aerosol properties available (as compared to scenario 1).
Figure 14 presents comparison of the diurnal median aerosol fine, coarse spherical and non-spherical fractions together with the lidar ratio at 532 nm, estimated for four seasons (winter, 1 December–28 February; spring, 1 March–21 May; summer, 1 June–31 August; autumn, 1 September–30 November). These components can generally be associated with anthropogenic activities (fine), maritime aerosols (coarse spherical) and desert dust (coarse non-spherical). It should be noted that, generally, for each particular retrieval, these fractions represent 100 % of the aerosol by volume; however median values may not add up to 100 % for each of the seasons.
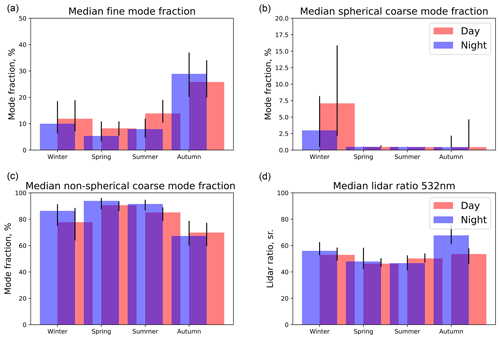
Figure 14Median aerosol fine (a), coarse spherical (b) and coarse non-spherical (c) component fractions and corresponding total lidar ratios at 532 nm (d) for daytime (red) and nighttime (blue) retrievals for the winter, spring, summer and autumn seasons. The corresponding 25th–75th percentile variations are shown in black.
Figure 14 clearly shows significant variation in aerosol composition from season to season; e.g., the winter season demonstrates a much higher coarse spherical contribution, while autumn has a more pronounced fine mode. It should be noted that all seasons apart from winter are dominated by coarse non-spherical particles that may be associated with a more significant presence of desert dust. Additionally, in winter a more significant diurnal variation in both spherical and non-spherical components could be observed; at the same time, the fine mode shows a much lower difference between day and night. Such behavior is most likely related to local aerosol transport events, notably the prevailing winds, that bring more maritime particles during the day. Generally, the LR at 532 nm follows the trends of aerosol composition change for each season, showing higher lidar ratios of ∼60 sr in winter, a value that has been reported for clean marine aerosol by several studies (Masonis et al., 2003; Li et al., 2022), and a significant increase in lidar ratios and their diurnal variations in autumn.
The spring and summer seasons could be described as a “dusty” period, indicating a higher non-spherical particle concentration (>90 % by volume) as compared to autumn and winter. During this period a more significant variation in the fine mode could be observed, especially in summer, with higher fine-particle load during the day, indicating most probably a contribution from human activity. LR 532 nm values support these trends, generally showing values typical of desert dust with observable increase in daytime values for summer.
Figure 15 showing the diurnal median complex refractive index at 532 nm for four seasons additionally supports the conclusions described above. For example, the real part of the complex refractive index of the coarse aerosol component (spherical and non-spherical components are not distinguished by the refractive index and have the same values) indicates lower indices in winter (both real and imaginary), which is reasonable for mixtures of maritime and dust aerosols. It should be noted that in autumn the real part of the refractive index has similar values to those in the winter season; however the imaginary part suggests absorption that is stronger than in winter and similar to spring–summer, while the fraction of the coarse spherical mode remains very low (see Fig. 15), most likely indicating changes in the microphysical properties of desert dust.
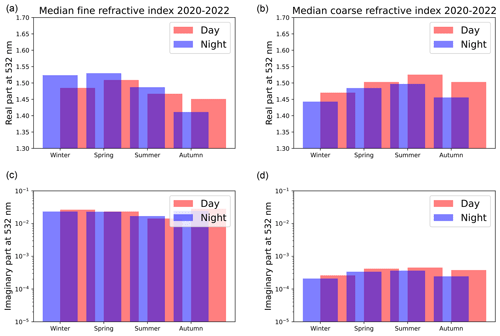
Figure 15Median complex refractive index at 532 nm for fine (a, c) and combined coarse spherical and non-spherical (b, d) aerosol components for daytime (red) and nighttime (blue) retrievals for the winter, spring, summer and autumn seasons; the real part is presented at the top (a, b) and the imaginary part at the bottom (c, d).
Overall, the fine-mode refractive index in the left panels of Fig. 16 shows stronger absorption than the coarse component, indicating particles of different chemical composition that are most likely related to human activities. At the same time, it should be emphasized that discrimination between fine- and coarse-mode refractive indices in a generally coarse-dominated (see Fig. 15) environment remains a challenging task.
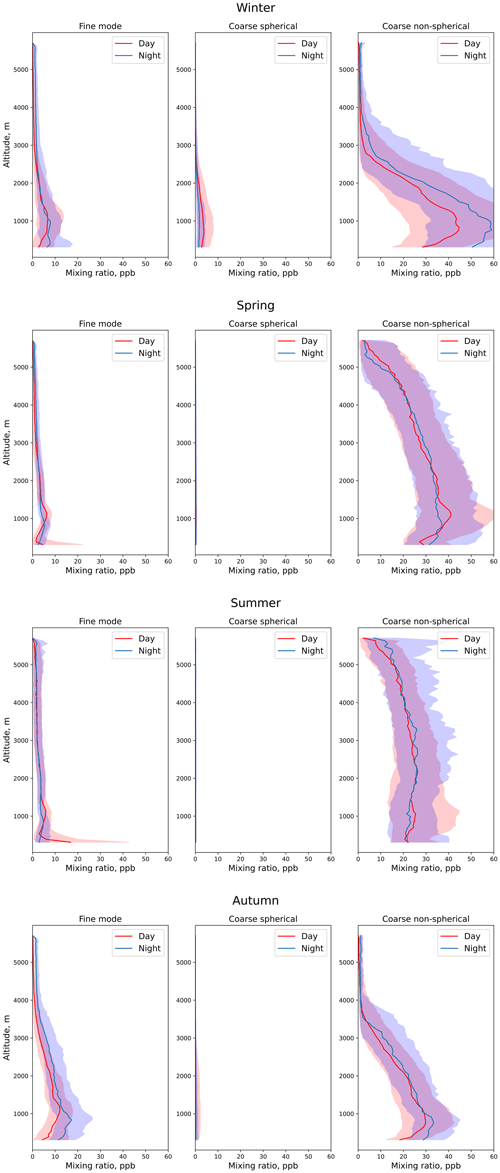
Figure 16Median vertical profiles of fine (left), coarse spherical (middle) and coarse non-spherical (right) components for daytime (red) and nighttime (blue) retrievals for (top to bottom) the winter, spring, summer and autumn seasons. The corresponding 25th–75th percentile variations are shown in the shading of corresponding colors.
Figure 16 demonstrates median vertical profiles of fine, coarse spherical and non-spherical components for the daytime and nighttime for four seasons. Generally, all component profiles follow the same trends as the columnar compositions, e.g., showing little to no spherical particles in spring–autumn. At the same time, it could be observed that in winter and autumn, all aerosol components are generally located lower (usually below 3.5 km) than in summer and spring, while all components appear to be well mixed.
Similarly to the columnar properties in Fig. 14a, a diurnal cycle of notable increase in coarse spherical particle concentration with a corresponding decrease in the non-spherical one during the daytime in winter could be observed (top middle panel of Fig. 16); additionally, it should be noted that the biggest change appears in the lower part of the atmosphere, below 2 km.
During the spring and summer, a significant diurnal variation in the fine component (left panels of Fig. 16) could also be observed, notably at altitude layers close to the ground (below 500 m), while in winter–autumn these layers appear to be more elevated (∼1 km) with an overall increase at nighttime, most likely indicating seasonal diurnal shift in anthropogenic activities.
Coarse non-spherical component profiles (right panels in Fig. 16) have a noticeable maximum at around 1 km during both the daytime and the nighttime in all seasons except summer, when the layers appear to be well mixed up to the maximum observation altitudes. This peak has a slight decrease in winter and autumn during the nighttime. A significant diurnal cycle of the coarse non-spherical component in the layers above 3 km could be observed in autumn, which could be associated with the change in the prevailing winds at these altitudes, introducing more maritime particles.
Data spanning almost 3 consecutive years starting from March 2019 and going to December 2022 and collected over the KAUST observation site, including vertical profiles of volume depolarization provided by MPLNET lidar, were processed using the GRASP software under the assumption of limited time variability in columnar properties such as the size distribution, chemical composition and sphericity fraction. As the result of the processing, columnar optical properties such as AOD and lidar ratio together with vertical profiles of extinction and backscatter at 532 nm were estimated for the retrievals excluding and including volume depolarization data provided by MPLNET.
The resulting properties were co-located with the MPLNET V3 L1.5 aerosol product and compared. Additional emphasis was placed on separating daytime and nighttime retrievals as well as on the potential benefits of utilizing volume depolarization profiles in the retrievals.
Overall, both columnar and vertical MPLNET and GRASP products demonstrated a better agreement for daytime retrievals excluding the depolarization information. Such an outcome is rather expected, as in scenario 1 GRASP and MPLNET share more assumptions as compared to scenario 2. It should be additionally noted that both products demonstrate lower columnar LRs than would be expected of a dust-dominated site such as KAUST as well as compared to AERONET estimations.
Overall, the following results for daytime retrievals without accounting for polarization profiles were achieved:
-
for columnar AOD, a correlation coefficient of 0.99, RMSE of 0.022, total bias of 0.0 including bias at low (<0.2) AOD and linear regression slope of 1;
-
a columnar LR correlation coefficient of 0.282, RMSE of 10.76 sr, bias of −1.3 sr and linear regression slope of 0.41;
-
vertical profiles of the extinction correlation coefficient of 0.98, RMSE of 16.38 Mm−1, total bias of −5.94 Mm−1 and linear regression slope of 0.85;
-
vertical profiles of the backscatter correlation coefficient of 0.964, RMSE of 0.53 , total bias of −0.18 and linear regression slope of 0.8.
Inclusion of volume depolarization profiles in the GRASP retrievals allows us to distinguish between columnar properties and the vertical distribution of fine and coarse aerosol modes, thus providing a more complex model and diverging further from the assumptions implied in MPLNET retrievals (e.g., the same lidar ratio for all observed atmospheric layers). At the same time, in dust-dominated cases, these differences are not expected to have a strong impact on the retrievals. Meanwhile the presence of the volume depolarization profiles causes a significant difference in columnar LR estimations, thus limiting the agreement between MPLNET and GRASP products. At the same time, the scenario 2 product demonstrates LRs that would be expected at a dust-dominated site such as KAUST; additionally they are closer to AERONET estimations than scenario 1.
Overall, the following results for daytime retrievals accounting for polarization profiles were achieved:
-
for columnar AOD, a correlation coefficient of 0.972, RMSE of 0.038, total bias including bias at low (<0.2) AOD of 0.0 and linear regression slope of 1.01;
-
a columnar LR correlation coefficient of 0.185, RMSE of 19.27 sr, total bias of −14.9 sr and linear regression slope of 0.23;
-
vertical profiles of the extinction correlation coefficient of 0.975, RMSE of 16.14 Mm−1, total bias of −5.43 Mm−1 and linear regression slope of 0.84;
-
vertical profiles of the backscatter correlation coefficient of 0.951, RMSE of 0.80 , total bias of −0.41 and linear regression slope of 0.65.
Additional comparison for estimated values of daytime extinction profiles at a ground level was made in order to assess the impact of assumptions of constant aerosol vertical distribution in the cutoff zone of lidar observations implied in GRASP. Estimations provided by GRASP for retrievals including the volume depolarization profiles demonstrated a slightly better linear regression slope and bias with comparable correlation coefficients and RMSE, most notably due to higher flexibility allowing us to describe the total ground level extinction as a sum of the values of fine and coarse aerosol modes.
The comparison of properties retrieved during the nighttime is, as expected, worse compared to the daytime retrievals. During the nighttime, neither method relied on any photometric observations due to the lack of lunar AOD at this site, and they use completely different approaches to estimate the values of aerosol properties. Nighttime MPLNET estimations were made from lidar observations only and do not rely on any spectral interpolation as compared to daytime retrievals; GRASP on the other hand estimates columnar aerosol properties due to a combination of consecutive lidar observations combined with sun-photometric measurements performed during the daytime under an assumption of limited change in aerosol columnar properties over time.
Despite these differences, the statistical properties of the comparisons are encouraging, and the following results for nighttime retrievals without accounting for polarization profiles were achieved:
-
for columnar AOD, a correlation coefficient of 0.528, RMSE of 0.282, total bias of 0.0 including bias at low (<0.2) AOD of 0.09 and linear regression slope of 0.63;
-
a columnar LR correlation coefficient of 0.027, RMSE of 22.81 sr, total bias of 6.85 sr and linear regression slope of 0.04;
-
vertical profiles of the extinction correlation coefficient of 0.774, RMSE of 43.03 Mm−1, total bias of −7.65 Mm−1 and linear regression slope of 0.654;
-
vertical profiles of the backscatter correlation coefficient of 0.669, RMSE of 1.21 , total bias of 0.12 and linear regression slope of 0.84.
Concerning nighttime retrievals accounting for polarization profiles, the following results were achieved overall:
-
for columnar AOD, a correlation coefficient of 0.624, RMSE of 0.220, total bias of 0.04 including bias at low (<0.2) AOD of 0.08 and linear regression slope of 0.86;
-
a columnar LR correlation coefficient of 0.003, RMSE of 21.80 sr, total bias of −6.85 sr and linear regression slope of 0.04;
-
vertical profiles of the extinction correlation coefficient of 0.784, RMSE of 41.21 Mm−1, total bias of −5.37 Mm−1 and linear regression slope of 0.697;
-
vertical profiles of the backscatter correlation coefficient of 0.793, RMSE of 0.82 , total bias of −0.30 and linear regression slope of 0.64.
Inclusion of the volume depolarization observations had an observable influence on the agreement between MPLNET- and GRASP-estimated values, both columnar and vertical for both nighttime and daytime values. The strongest difference was observed in columnar LR estimations, with retrievals of scenario 2 having a noticeable positive bias against GRASP-S1-estimated, MPLNET-estimated and AERONET-estimated values. Biased values belong to a range that is expected for desert dust particles, a primary aerosol component over the KAUST observation site. However, a decisive conclusion on the improvements in accounting for depolarization data based on nighttime retrievals would require additional studies. Those should include independent nighttime observations of aerosol columnar and vertical properties, e.g., lunar photometry and/or Raman lidars that allow us to precisely evaluate estimations of nighttime aerosol properties provided by both methods and thus accurately estimate the impact of polarization data inclusion on GRASP products.
Analysis of the statistical distribution of columnar and vertical aerosol properties distinguishing between fine, coarse spherical and coarse non-spherical aerosol components suggests noticeable changes in aerosol diurnal and seasonal variability.
Data are available upon request by email (anton.lopatin@grasp-earth.com).
AL: project administration, investigation, methodology, validation, data curation, writing (original draft); OD: conceptualization, supervision, writing (review and editing); GS: conceptualization, supervision, project administration, funding acquisition, resources, writing (review and editing); EJW: methodology, investigation, data curation, writing (review and editing); IS: data curation; DF: development, software, data curation; MHG: development, methodology; TL: development, software; AS: data curation, writing (review and editing).
At least one of the (co-)authors is a member of the editorial board of Atmospheric Measurement Techniques. The peer-review process was guided by an independent editor, and the authors also have no other competing interests to declare.
Publisher's note: Copernicus Publications remains neutral with regard to jurisdictional claims made in the text, published maps, institutional affiliations, or any other geographical representation in this paper. While Copernicus Publications makes every effort to include appropriate place names, the final responsibility lies with the authors.
Authors thank the KAUST Supercomputing Laboratory for providing computer resources.
The research reported in this publication was partially supported by funding from the King Abdullah University of Science and Technology (KAUST) via the KAUST Base Research Fund (grant no. BAS/1/1309-01-01).
This paper was edited by Daniel Perez-Ramirez and reviewed by Gregory L. Schuster and one anonymous referee.
Albrecht, B. A.: Aerosols, cloud microphysics, and fractional cloudiness, Science, 245, 1227–1230, 1989.
Ault, A. P. and Axson, J. L.: Atmospheric aerosol chemistry: Spectroscopic and microscopic advances, Anal. Chem., 89, 430–452, 2017.
Barreto, Á., Cuevas, E., Granados-Muñoz, M.-J., Alados-Arboledas, L., Romero, P. M., Gröbner, J., Kouremeti, N., Almansa, A. F., Stone, T., Toledano, C., Román, R., Sorokin, M., Holben, B., Canini, M., and Yela, M.: The new sun-sky-lunar Cimel CE318-T multiband photometer – a comprehensive performance evaluation, Atmos. Meas. Tech., 9, 631–654, https://doi.org/10.5194/amt-9-631-2016, 2016.
Bazo, E., Martins, J. V., Perez-Ramirez, D., Valenzuela, A., Titos, G., Cazorla, A., Fuertes, D., Weiss, M., Turpie, A., Li, C., García-Izquierdo, F. J., Foyo-Moreno, I., Alados-Arboledas, L., and Olmo, F. J.: Optimization of the Polarized Imaging Nephelometer (PI-Neph) for continuous monitoring of multiwavelength aerosol phase functions in support of space polarimetry missions, Atmos. Environ., 316, 120181, https://doi.org/10.1016/j.atmosenv.2023.120181, 2024.
Campbell, J. R., Hlavka, D. L., Welton, E. J., Flynn, C. J., Turner, D. D., Spinhirne, J. D., Scott III, V. S., and Hwang, I. H.: Full-time, eye-safe cloud and aerosol lidar observation at atmospheric radiation measurement program sites: Instruments and data processing, J. Atmos. Ocean. Tech., 19, 431–442, 2002.
Chaikovsky, A., Dubovik, O., Holben, B., Bril, A., Goloub, P., Tanré, D., Pappalardo, G., Wandinger, U., Chaikovskaya, L., Denisov, S., Grudo, J., Lopatin, A., Karol, Y., Lapyonok, T., Amiridis, V., Ansmann, A., Apituley, A., Allados-Arboledas, L., Binietoglou, I., Boselli, A., D'Amico, G., Freudenthaler, V., Giles, D., Granados-Muñoz, M. J., Kokkalis, P., Nicolae, D., Oshchepkov, S., Papayannis, A., Perrone, M. R., Pietruczuk, A., Rocadenbosch, F., Sicard, M., Slutsker, I., Talianu, C., De Tomasi, F., Tsekeri, A., Wagner, J., and Wang, X.: Lidar-Radiometer Inversion Code (LIRIC) for the retrieval of vertical aerosol properties from combined lidar/radiometer data: development and distribution in EARLINET, Atmos. Meas. Tech., 9, 1181–1205, https://doi.org/10.5194/amt-9-1181-2016, 2016.
Chen, C., Dubovik, O., Fuertes, D., Litvinov, P., Lapyonok, T., Lopatin, A., Ducos, F., Derimian, Y., Herman, M., Tanré, D., Remer, L. A., Lyapustin, A., Sayer, A. M., Levy, R. C., Hsu, N. C., Descloitres, J., Li, L., Torres, B., Karol, Y., Herrera, M., Herreras, M., Aspetsberger, M., Wanzenboeck, M., Bindreiter, L., Marth, D., Hangler, A., and Federspiel, C.: Validation of GRASP algorithm product from POLDER/PARASOL data and assessment of multi-angular polarimetry potential for aerosol monitoring, Earth Syst. Sci. Data, 12, 3573–3620, https://doi.org/10.5194/essd-12-3573-2020, 2020.
Dubovik, O. and King, M. D.: A flexible inversion algorithm for retrieval of aerosol optical properties from Sun and sky radiance measurements, J. Geophys. Res.-Atmos., 105, 20673–20696, 2000.
Dubovik, O., Smirnov, A., Holben, B. N., King, M. D., Kaufman, Y. J., Eck, T. F., and Slutsker, I.: Accuracy assessments of aerosol optical properties retrieved from Aerosol Robotic Network (AERONET) Sun and sky radiance measurements, J. Geophys. Res.-Atmos., 105, 9791–9806, 2000.
Dubovik, O., Sinyuk, A., Lapyonok, T., Holben, B. N., Mishchenko, M., Yang, P., Eck, T. F., Volten, H., Munoz, O., Veihelmann, B., and Van der Zande, W. J.: Application of spheroid models to account for aerosol particle nonsphericity in remote sensing of desert dust, J. Geophys. Res.-Atmos., 111, D11208, https://doi.org/10.1029/2005JD006619, 2006.
Dubovik, O., Herman, M., Holdak, A., Lapyonok, T., Tanré, D., Deuzé, J. L., Ducos, F., Sinyuk, A., and Lopatin, A.: Statistically optimized inversion algorithm for enhanced retrieval of aerosol properties from spectral multi-angle polarimetric satellite observations, Atmos. Meas. Tech., 4, 975–1018, https://doi.org/10.5194/amt-4-975-2011, 2011.
Dubovik, O., Lapyonok, T., Litvinov P., Herman, M., Fuertes, D., Ducos, F., Lopatin, A., Chaikovsky, A., Torres, B., Derimian, Y., Huang, X., Aspetsberger, M., and Federspiel, C.: GRASP: a versatile algorithm for characterizing the atmosphere, SPIE: Newsroom, published Online: 19 September 2014, https://doi.org/10.1117/2.1201408.005558, 2014.
Dubovik, O., Fuertes, D., Litvinov, P., Lopatin, A., Lapyonok, T., Doubovik, I., Xu, F., Ducos, F., Chen, C., Torres B., Derimian, Y., Li, L., Herreras-Giralda, M., Herrera, M., Karol, Y., Matar, C., Schuster, G. L., Espinosa, R., Puthukkudy, A., Li, Z., Fischer, J., Preusker, R., Cuesta, J., Kreuter, A., Cede, A., Aspetsberger, M., Marth, D., Bindreiter, L., Hangler, A., Lanzinger, V., Holter, C., and Federspiel, C.: A Comprehensive Description of Multi-Term LSM for Applying Multiple a Priori Constraints in Problems of Atmospheric Remote Sensing: GRASP Algorithm, Concept, and Applications, Frontiers in Remote Sensing, 2, 23, https://doi.org/10.3389/frsen.2021.706851, 2021.
Espinosa, W. R., Remer, L. A., Dubovik, O., Ziemba, L., Beyersdorf, A., Orozco, D., Schuster, G., Lapyonok, T., Fuertes, D., and Martins, J. V.: Retrievals of aerosol optical and microphysical properties from Imaging Polar Nephelometer scattering measurements, Atmos. Meas. Tech., 10, 811–824, https://doi.org/10.5194/amt-10-811-2017, 2017.
Espinosa, W. R., Vanderlei Martins, J., Remer, L. A., Dubovik, O., Lapyonok, T., Fuertes, D., Puthukkudy, A., Orozco, D., Ziemba, L., Thornhill, K. L., and Levy, R.: Retrievals of aerosol size distribution, spherical fraction, and complex refractive index from airborne in situ angular light scattering and absorption measurements, J. Geophys. Res.-Atmos., 124, 7997–8024, https://doi.org/10.1029/2018JD030009, 2019.
Fernald, F. G., Herman, B. M., and Reagan, J. A.: Determination of aerosol height distributions by lidar, J. Appl. Meteorol. Clim., 11, 482–489, 1972.
Fernald, F. G.: Analysis of atmospheric lidar observations: some comments, Appl. Optics, 23, 652–653, 1984.
Flynn, C. J., Mendoza, A., Zheng, Y., and Mathur, S.: Novel polarization-sensitive micropulse lidar measurement technique, Opt. Express, 15, 2785–2790, https://doi.org/10.1364/OE.15.002785, 2007.
Hair, J. W., Hostetler, C. A., Cook, A. L., Harper, D. B., Ferrare, R. A., Mack, T. L., Welch, W., Izquierdo, L. R., and Hovis, F. E.: Airborne high spectral resolution lidar for profiling aerosol optical properties, Appl. Optics, 47, 6734–6752, 2008.
Hasekamp, O., Litvinov, P., Fu, G., Chen, C., and Dubovik, O.: Algorithm evaluation for polarimetric remote sensing of atmospheric aerosols, Atmos. Meas. Tech., 17, 1497–1525, https://doi.org/10.5194/amt-17-1497-2024, 2024.
Holben, B. N., Eck, T. F., Slutsker, I. A., Tanré, D., Buis, J. P., Setzer, A., Vermote, E., Reagan, J. A., Kaufman, Y. J., Nakajima, T., and Lavenu, F.: AERONET – A federated instrument network and data archive for aerosol characterization, Remote Sens. Environ., 66, 1–16, 1998.
Kalenderski, S. and Stenchikov, G.: High-resolution regional modeling of summertime transport and impact of African dust over the Red Sea and Arabian Peninsula, J. Geophys. Res.-Atmos., 121, 6435–6458, 2016.
Kim, M.-H., Omar, A. H., Tackett, J. L., Vaughan, M. A., Winker, D. M., Trepte, C. R., Hu, Y., Liu, Z., Poole, L. R., Pitts, M. C., Kar, J., and Magill, B. E.: The CALIPSO version 4 automated aerosol classification and lidar ratio selection algorithm, Atmos. Meas. Tech., 11, 6107–6135, https://doi.org/10.5194/amt-11-6107-2018, 2018.
Klett, J. D.: Stable analytical inversion solution for processing lidar returns, Appl. Optics, 20, 211–220, 1981.
Koch, D. and Del Genio, A. D.: Black carbon semi-direct effects on cloud cover: review and synthesis, Atmos. Chem. Phys., 10, 7685–7696, https://doi.org/10.5194/acp-10-7685-2010, 2010.
Lewis, J. R., Welton, E. J., Molod, A. M., and Joseph, E.: Improved boundary layer depth retrievals from MPLNET, J. Geophys. Res.-Atmos., 118, 9870–9879, 2013.
Lewis, J. R., Campbell, J. R., Welton, E. J., Stewart, S. A., and Haftings, P. C.: Overview of MPLNET Version 3 Cloud Detection, J. Atmos. Ocean. Tech., 33, 2113–2134, https://doi.org/10.1175/JTECH-D-15-0190.1, 2016.
Lewis, J. R., Campbell, J. R., Stewart, S. A., Tan, I., Welton, E. J., and Lolli, S.: Determining cloud thermodynamic phase from the polarized Micro Pulse Lidar, Atmos. Meas. Tech., 13, 6901–6913, https://doi.org/10.5194/amt-13-6901-2020, 2020.
Li, L., Dubovik, O., Derimian, Y., Schuster, G. L., Lapyonok, T., Litvinov, P., Ducos, F., Fuertes, D., Chen, C., Li, Z., Lopatin, A., Torres, B., and Che, H.: Retrieval of aerosol components directly from satellite and ground-based measurements, Atmos. Chem. Phys., 19, 13409–13443, https://doi.org/10.5194/acp-19-13409-2019, 2019.
Li, Z., Painemal, D., Schuster, G., Clayton, M., Ferrare, R., Vaughan, M., Josset, D., Kar, J., and Trepte, C.: Assessment of tropospheric CALIPSO Version 4.2 aerosol types over the ocean using independent CALIPSO–SODA lidar ratios, Atmos. Meas. Tech., 15, 2745–2766, https://doi.org/10.5194/amt-15-2745-2022, 2022.
Lopatin, A., Dubovik, O., Chaikovsky, A., Goloub, P., Lapyonok, T., Tanré, D., and Litvinov, P.: Enhancement of aerosol characterization using synergy of lidar and sun-photometer coincident observations: the GARRLiC algorithm, Atmos. Meas. Tech., 6, 2065–2088, https://doi.org/10.5194/amt-6-2065-2013, 2013.
Lopatin, A., Dubovik, O., Fuertes, D., Stenchikov, G., Lapyonok, T., Veselovskii, I., Wienhold, F. G., Shevchenko, I., Hu, Q., and Parajuli, S.: Synergy processing of diverse ground-based remote sensing and in situ data using the GRASP algorithm: applications to radiometer, lidar and radiosonde observations, Atmos. Meas. Tech., 14, 2575–2614, https://doi.org/10.5194/amt-14-2575-2021, 2021.
Marenco, F., Santacesaria, V., Bais, A. F., Balis, D., di Sarra, A., Papayannis, A., and Zerefos, C.: Optical properties of tropospheric aerosols determined by lidar and spectrophotometric measurements (Photochemical Activity and Solar Ultraviolet Radiation campaign), Appl. Optics, 36, 6875–6886, 1997.
Masonis, S. J., Anderson, T. L., Covert, D. S., Kapustin, V., Clarke, A. D., Howell, S., and Moore, K.: A Study of the Extinction-to-Backscatter Ratio of Marine Aerosol during the Shoreline Environment Aerosol Study, J. Atmos. Ocean. Tech., 20, 1388–1402, 2003.
Müller, D., Ansmann, A., Mattis, I., Tesche, M., Wandinger, U., Althausen, D., and Pisani, G.: Aerosol-type-dependent lidar ratios observed with Raman lidar, J. Geophys. Res.-Atmos., 112, D16202, https://doi.org/10.1029/2006JD008292, 2007.
Papayannis, A., Amiridis, V., Mona, L., Tsaknakis, G., Balis, D., Bösenberg, J., Chaikovski, A., De Tomasi, F., Grigorov, I., Mattis, I., and Mitev, V.: Systematic lidar observations of Saharan dust over Europe in the frame of EARLINET (2000–2002), J. Geophys. Res.-Atmos., 113, D10204, https://doi.org/10.1029/2007JD009028, 2008.
Parajuli, S. P., Stenchikov, G. L., Ukhov, A., Shevchenko, I., Dubovik, O., and Lopatin, A.: Aerosol vertical distribution and interactions with land/sea breezes over the eastern coast of the Red Sea from lidar data and high-resolution WRF-Chem simulations, Atmos. Chem. Phys., 20, 16089–16116, https://doi.org/10.5194/acp-20-16089-2020, 2020.
Pope III, C. A., Burnett, R. T., Thun, M. J., Calle, E. E., Krewski, D., Ito, K., and Thurston, G. D.: Lung cancer, cardiopulmonary mortality, and long-term exposure to fine particulate air pollution, J. Amer. Med. Assoc. (JAMA), 287, 1132–1141, 2002.
Raaschou-Nielsen, O., Andersen, Z. J., Beelen, R., Samoli, E., Stafoggia, M., Weinmayr, G., Hoffmann, B., Fischer, P., Nieuwenhuijsen, M. J., Brunekreef, B., and Xun, W. W.: Air pollution and lung cancer incidence in 17 European cohorts: prospective analyses from the European Study of Cohorts for Air Pollution Effects (ESCAPE), Lancet Oncol., 14, 813–822, 2013.
Schuster, G. L., Dubovik, O., Holben, B. N., and Clothiaux, E. E.: Inferring black carbon content and specific absorption from Aerosol Robotic Network (AERONET) aerosol retrievals, J. Geophys. Res., 110, D10S17, https://doi.org/10.1029/2004JD004548, 2005.
Schuster, G. L., Lin, B., and Dubovik, O.: Remote sensing of aerosol water uptake, Geophys. Res. Lett., 36, L03814, https://doi.org/10.1029/2008GL036576, 2009.
Schuster, G. L., Vaughan, M., MacDonnell, D., Su, W., Winker, D., Dubovik, O., Lapyonok, T., and Trepte, C.: Comparison of CALIPSO aerosol optical depth retrievals to AERONET measurements, and a climatology for the lidar ratio of dust, Atmos. Chem. Phys., 12, 7431–7452, https://doi.org/10.5194/acp-12-7431-2012, 2012.
Schuster, G. L., Dubovik, O., Arola, A., Eck, T. F., and Holben, B. N.: Remote sensing of soot carbon – Part 2: Understanding the absorption Ångström exponent, Atmos. Chem. Phys., 16, 1587–1602, https://doi.org/10.5194/acp-16-1587-2016, 2016a.
Schuster, G. L., Dubovik, O., and Arola, A.: Remote sensing of soot carbon – Part 1: Distinguishing different absorbing aerosol species, Atmos. Chem. Phys., 16, 1565–1585, https://doi.org/10.5194/acp-16-1565-2016, 2016b.
Twomey, S.: Pollution and the planetary albedo, Atmos. Environ. (1967), 8, 1251–1256, https://doi.org/10.1016/0004-6981(74)90004-3, 1974.
Wagner, F. and Silva, A. M.: Some considerations about Ångström exponent distributions, Atmos. Chem. Phys., 8, 481–489, https://doi.org/10.5194/acp-8-481-2008, 2008.
Wandinger, U.: Raman Lidar, in: Lidar, edited by: Weitkamp, C., Springer Series in Optical Sciences, Springer, New York, NY, 102, https://doi.org/10.1007/0-387-25101-4_9, 2005.
Wellenius, G. A., Burger, M. R., Coull, B. A., Schwartz, J., Suh, H. H., Koutrakis, P., Schlaug, G., Gold, D. R., and Mittleman, M. A.: Ambient air pollution and the risk of acute ischemic stroke, Arch. Intern. Med., 172, 229–234, 2012.
Welton, E. J. and Campbell, J.R.: Micropulse lidar signals: Uncertainty analysis, J. Atmos. Ocean. Tech., 19, 2089–2094, 2002.
Welton, E. J., Voss, K. J., Gordon, H. R., Maring, H., Smirnov, A., Holben, B., Schmid, B., Livingston, J. M., Russell, P. B., Durkee, P. A., Formenti, P., and Andreae, M. O.: Ground-based Lidar Measurements of Aerosols During ACE-2: Instrument Description, Results, and Comparisons with other Ground-based and Airborne Measurements, Tellus B, 52, 635–650, 2000.
Welton, E. J., Campbell, J. R., Spinhirne, J. D., and Scott, V. S.: Global monitoring of clouds and aerosols using a network of micro-pulse lidar systems, Proc. SPIE, 4153, 151–158, 2001.
Welton, E. J., Voss, K. J., Quinn, P. K., Flatau, P. J., Markowicz, K., Campbell, J. R., Spinhirne, J. D., Gordon, H. R., and Johnson, J. E.: Measurements of aerosol vertical profiles and optical properties during INDOEX 1999 using micro-pulse lidars, J. Geophys. Res., 107, 8019, https://doi.org/10.1029/2000JD000038, 2002.
Welton, E. J., Stewart, S. A., Lewis, J. R., Belcher, L. R., Campbell, J. R., and Lolli, S.: Status of the NASA Micro Pulse Lidar Network (MPLNET): Overview of the network and future plans, new Version 3 data products, and the polarized MPL, EPJ Web Conf., 176, 09003, https://doi.org/10.1051/epjconf/201817609003, 2018.
Xu, F., Gao, L., Redemann, J., Flynn, C. J., Espinosa, W. R., da Silva, A. M., Stamnes, S., Burton, Sh. P., Liu, X., Ferrare, R., Cairns, B., and Dubovik, O.: A Combined Lidar-Polarimeter Inversion Approach for Aerosol Remote Sensing Over Ocean, Frontiers in Remote Sensing, 2, 620871, https://doi.org/10.3389/frsen.2021.620871, 2021.
Zhang, X., Li, L., Chen, C., Zheng, Y., Dubovik, O., Derimian, Y., Lopatin, A., Gui, K., Wang, Y., Zhao, H., Liang, Y., Holben, B. N., Che, H., and Zhang, X.: Extensive characterization of aerosol optical properties and chemical component concentrations: Application of the GRASP/Component approach to long-term AERONET measurements, Sci. Total Environ., 812, 152553, https://doi.org/10.1016/j.scitotenv.2021.152553, 2022.
- Abstract
- Introduction
- Dataset description and methodology
- Comparison strategy
- Daylight property comparison
- Nighttime property comparison
- Seasonal diurnal analysis of aerosol properties over the KAUST site in 2020–2022
- Conclusions
- Data availability
- Author contributions
- Competing interests
- Disclaimer
- Acknowledgements
- Financial support
- Review statement
- References
- Abstract
- Introduction
- Dataset description and methodology
- Comparison strategy
- Daylight property comparison
- Nighttime property comparison
- Seasonal diurnal analysis of aerosol properties over the KAUST site in 2020–2022
- Conclusions
- Data availability
- Author contributions
- Competing interests
- Disclaimer
- Acknowledgements
- Financial support
- Review statement
- References