the Creative Commons Attribution 4.0 License.
the Creative Commons Attribution 4.0 License.
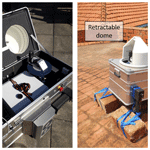
Greenhouse gas column observations from a portable spectrometer in Uganda
Hartmut Boesch
William Okello
Florian Dietrich
Mark F. Lunt
Liang Feng
Paul I. Palmer
Frank Hase
The extensive terrestrial ecosystems of tropical Africa are a significant store of carbon and play a key but uncertain role in the atmospheric budgets of carbon dioxide and methane. As ground-based observations in the tropics are scarce compared with other parts of the world, recent studies have instead made use of satellite observations assimilated into atmospheric chemistry and transport models to conclude that methane emissions from this geographical region have increased since 2010 as a result of increased wetland extent, accounting for up to a third of global methane growth, and that the tropical Africa region dominates net carbon emission across the tropics. These studies critically rely on the accuracy of satellite datasets, such as those from the Orbiting Carbon Observatory-2 (OCO-2), the Greenhouse gases Observing SATellite (GOSAT), and the Sentinel-5 Precursor TROPOspheric Monitoring Instrument (TROPOMI), along with results from atmospheric transport models, over a geographical region where there are little independent data to test the robustness of published results.
In this paper we present the first ground-based observations of greenhouse gas column concentrations over East Africa, obtained using a portable Bruker EM27/SUN Fourier transform infrared (FTIR) spectrometer during a deployment covering the first few months of 2020 in Jinja, Uganda. We operated the instrument near autonomously by way of an automated weatherproof enclosure and observed total atmospheric column concentrations of the greenhouse gases carbon dioxide and methane, as well as carbon monoxide, a useful proxy for emissions from incomplete combustion processes in the region. We discuss the performance of the combined enclosure and spectrometer system that we deployed in Jinja to obtain these data and show comparisons of our ground-based observations with satellite datasets from OCO-2 and Orbiting Carbon Observatory-3 (OCO-3) for carbon dioxide and TROPOMI for methane and carbon monoxide, whilst also comparing our results with concentration data from the GEOS-Chem and Copernicus Atmosphere Monitoring Service (CAMS) atmospheric inversions that provide a means of increasing spatial and temporal coverage where satellite data are not available. For our measurement period, we find mean differences in between OCO-2 and the EM27/SUN of −0.29 % and between OCO-3 and the EM27/SUN of −0.28 %. In the case of TROPOMI, the mean difference in that we find between TROPOMI and the EM27/SUN is −0.44 %, whilst for XCO the mean difference is −5.65 %. In each of these cases, the mean difference observed between the satellite and ground-based column concentrations is either close to or within the precision and accuracy requirements for the respective missions. With regard to the model and reanalysis comparisons with the EM27/SUN column concentrations, we see mean differences from the EM27/SUN of a global GEOS-Chem inversion for of −0.08 %, a regional high-resolution GEOS-Chem inversion for of −0.22 %, and the CAMS global reanalysis for XCO of −9.79 %. Our results demonstrate the value of ground-based observations of total column concentrations and show that the combined EM27/SUN and enclosure system employed would be suitable for acquisition of the longer-term observations needed to rigorously evaluate satellite observations and model and reanalysis calculations over tropical Africa.
- Article
(10413 KB) - Full-text XML
- BibTeX
- EndNote
Gaps in our understanding of the global carbon cycle add uncertainty to our predictions of future climate change, including how the future climate will respond to different carbon emissions scenarios (IPCC, 2021; Friedlingstein et al., 2022). One part of the carbon cycle which still requires further investigation is that of carbon fluxes from terrestrial tropical ecosystems, which store large quantities of carbon in vegetation and soil whilst being sensitive to changes in the climate (Pan et al., 2011; Crowther et al., 2015). Carbon dioxide is released into the atmosphere by these ecosystems through a combination of respiration and fire and is removed from the atmosphere by photosynthesis and subsequent conversion into plant biomass. The tropics are also home to extensive areas of wetlands, which are the most significant natural source of methane in the atmosphere via the decomposition of organic matter in anaerobic conditions (Kayranli et al., 2010; Mitsch et al., 2013). Further microbial sources of methane in tropical regions include agricultural practices, particularly the farming of ruminants, and waste disposal. Looking at Africa in particular, an additional factor having an increasingly significant impact on the tropical African carbon cycle is the recent increase in population in many African countries, resulting in increasing demand for energy (Ayompe et al., 2021), as reflected in the rapid projected growth of cities such as Kampala (Uganda), Nairobi (Kenya), and Kinshasa (Democratic Republic of the Congo). This combination of natural and anthropogenic fluxes that contribute to the atmospheric carbon budget in tropical Africa is challenging to accurately represent in climate and atmospheric chemistry models, so we need to make use of atmospheric composition measurements to evaluate our understanding.
However, compared with other parts of the world, ground-based measurements of atmospheric composition are scarce in tropical Africa, placing an upper limit on how well we can understand the carbon cycle in this region (López-Ballesteros et al., 2018; Nickless et al., 2020). This measurement gap is partially addressed by satellites such as the JAXA Greenhouse gases Observing SATellite (GOSAT) (Kuze et al., 2009), NASA Orbiting Carbon Observatory-2 (OCO-2) (Eldering et al., 2017) and Orbiting Carbon Observatory-3 (OCO-3) (Eldering et al., 2019), and Copernicus Sentinel-5 Precursor (Veefkind et al., 2012) missions, although the measurement technique employed usually requires cloud-free and low-aerosol conditions to retrieve molecular concentrations from the observed radiance spectra, resulting in relatively poor coverage over the tropics where conditions are often cloudy. However, the satellite data that are obtained can be used as an input for atmospheric chemistry models, which use prior estimates of surface fluxes and meteorological fields to calculate a most-likely state for the atmosphere constrained by the observations available (e.g. Basu et al., 2013; Deng et al., 2014; Feng et al., 2017; Chevallier et al., 2019; Crowell et al., 2019; Chen et al., 2022; Peiro et al., 2022). The atmospheric chemistry models can then be used in an inversion framework to produce a posteriori estimates of emissions where satellite data are not available, since increased greenhouse gas concentrations remain in the atmospheric column for some time downwind of where they are originally emitted. In addition, these models (such as GEOS-Chem; Turner et al., 2015; Feng et al., 2017; Lunt et al., 2019) are a useful means of estimating atmospheric concentrations where observations are not available and have underpinned a number of studies that address the tropical African carbon cycle (Palmer et al., 2019; Lunt et al., 2019, 2021; Pandey et al., 2021; Qu et al., 2022; Feng et al., 2022, 2023; Drinkwater et al., 2023). It is therefore important to validate model output with independent observations to confirm how well the models represent the atmosphere and add weight to the conclusions of the studies which use them.
In this study, we describe observations of the total column concentrations of greenhouse gases in Uganda in the first few months of 2020, obtained using a portable spectrometer with a built-in solar tracker. We used an automated enclosure to provide a weatherproof environment for the spectrometer and to allow us to operate the spectrometer remotely. This setup allowed us to produce for the first time a dataset of ground-based total column concentrations of carbon dioxide, methane, and carbon monoxide for a tropical East African location. In Sect. 2 we outline the measurement site and describe the instrument, enclosure, and retrieval algorithm used to obtain the dataset. Section 3 covers the satellite and model datasets that we then compare with our ground-based observations in Sect. 4. Finally, we conclude and consider the implications of this study in Sect. 5.
For this study, we established our measurement site at the headquarters of the Ugandan National Fisheries Resources Research Institute (NaFIRRI) in Jinja (0.4165° N, 33.2070° E; 1157 m above sea level). Jinja is located on the northern shore of Lake Victoria, approximately 70 km to the east of Kampala, Uganda's capital city with a population of approximately 3.5 million people across its wider urban area. The source of the White Nile is in Jinja, from where it flows northwards out of Lake Victoria, through lakes Kyoga and Albert, and onwards into South Sudan. The Nile feeds the neighbouring wetlands, which are amongst the main sources of methane emissions in East Africa through the anaerobic decomposition of organic matter. There is a strong link between CH4 emissions and water table depth in tropical regions such that anomalies in precipitation can lead to wetland CH4 emissions anomalies (Bloom et al., 2010). The hydrological flow from increased precipitation over Lake Victoria and higher water table depth in Ugandan and South Sudanese wetlands, as a result of increased volumes of water transported along the Nile, is covered in more detail by Lunt et al. (2019). Precipitation in Uganda is driven by the annual north–south movement of the inter-tropical convergence zone, resulting in two main wet seasons during the year: these are known as the “long rains”, which occur from March to May, and the “short rains”, occurring from October to December (Herrmann and Mohr, 2011). The amount of precipitation over East Africa during these wet seasons is in turn partly influenced by ocean temperatures in the Indian Ocean (Palmer et al., 2023), where an unusually high contrast in temperatures (greater than 0.4 °C) between the warmer western Indian Ocean and cooler eastern Indian Ocean (defined quantitatively as the Indian Ocean Dipole, Saji et al., 1999) in 2019 resulted in one of the wettest short-rain seasons on record (Wainwright et al., 2021).
In the wider region beyond Uganda, there are a number of environmental factors which can potentially affect the column of air that we measure at Jinja. To the north, the Sudd wetlands in South Sudan represent a significant natural source of CH4, as discussed and investigated by Lunt et al. (2019, 2021). To the west, atmospheric CO2 signals are dominated by the biospheric influence of the Congo rainforest (Palmer et al., 2019). This part of the world is also subject to a high frequency of biomass burning events, evidence of which can be seen in TROPOspheric Monitoring Instrument (TROPOMI) observations of carbon monoxide (a product of incomplete combustion; see Sect. 3.2).
To help us understand which sources influence the composition of the air columns we observe, we use a Lagrangian dispersion model to calculate the history of the air masses arriving over Jinja during the measurement period (Fleming et al., 2012; Panagi et al., 2020). We use the UK Met Office (UKMO) Numerical Atmospheric-dispersion Modelling Environment (NAME) to perform this task. NAME, along with other Lagrangian dispersion models, works by releasing a large number of inert particles from a specific location into the atmosphere and then tracking their pathways backwards in time using meteorological model data (which in the NAME modelling framework comes from the UKMO Unified Model). We then count the number of released particles that pass within 100 m of the surface over each spatial grid point to determine where and to what extent the back trajectories are influenced by surface emissions from that location within a certain period of time. To account for our measurements being sensitive to the whole atmospheric column, we perform the particle releases from heights throughout the vertical grid of the model domain and weight the contributions from each release height according to the pressure weighting function of the EM27/SUN observations. We perform the calculation for each day of the measurement period (the particle release is performed at 10:30 UTC each day, equivalent to 13:30 local time (LT) – the time at which TROPOMI and OCO-2 pass overhead – and tracked back in time for 1 and 5 d) to obtain a daily column footprint. Figure 1 shows the mean daily footprint for the whole measurement period (panels a and b show the results from back trajectories going back 1 and 5 d, respectively), giving us an estimate of where the surface has influenced the measured column. Although the highest contribution arrives via a region directly to the south, coinciding with Lake Victoria, the footprint of influence also covers regions to the north and east, reaching as far as South Sudan and Kenya, respectively, where emissions from wetlands and agriculture can potentially have an impact on the observed atmospheric column.
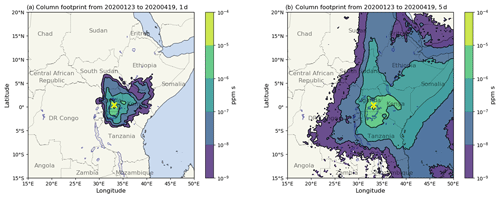
Figure 1Mean column footprint of 10:30 UTC (13:30 LT) column observations, calculated from 1 d (a) and 5 d (b) NAME back trajectories for each day within the measurement period (23 January to 19 April 2020). The yellow cross indicates the location of our EM27/SUN instrument in Jinja. The colour scale indicates the calculated contribution of the surface in that location to the observed atmospheric column, in parts per million, integrated over the duration of the back trajectory.
2.1 The EM27/SUN portable spectrometer
Ground-based remote sensing of the atmospheric column has proved to be an invaluable tool in the validation of atmospheric composition data from satellite observations. The global network of Bruker 125HR spectrometers that form the Total Carbon Column Observing Network (TCCON; Wunch et al., 2011), for example, is now routinely used in the validation of greenhouse gas (GHG) column observations from GOSAT, OCO-2, Sentinel-5P, and others, allowing those working on the retrieval algorithms to identify and correct for systematic biases in their data (Inoue et al., 2016; Wunch et al., 2017; Sha et al., 2021). The standard configuration for a TCCON site is, however, both expensive and logistically challenging to set up and maintain. As a result, there are certain regions around the world – South America, Africa, Central Asia, and South Asia – which do not currently have the resources and infrastructure in place to host TCCON sites, leaving significant gaps in the validation of GHG column data products, often in geographical areas of great scientific interest (e.g. the Amazon rainforest, sub-Saharan Africa).
The Bruker EM27/SUN Fourier transform infrared (FTIR) spectrometer concept (Gisi et al., 2012) was developed at the Karlsruhe Institute of Technology (KIT), in part to address this problem. It comprises a portable Fourier transform spectrometer with a built-in solar tracker, which trades off a reduced spectral resolution compared with the Bruker 125HR used at TCCON sites in favour of being less expensive and much easier to transport to and operate in different locations. A number of previous studies have demonstrated comparable stability and precision when operated side by side with the higher-resolution 125HR (Frey et al., 2015; Hedelius et al., 2016; Hase et al., 2016; Sha et al., 2020; Alberti et al., 2022). At the time of writing, over 150 EM27/SUNs had been purchased by research groups around the world and have been operating in a variety of locations. Prior to shipment, the instruments are first calibrated at KIT to obtain the instrument line shape parameters and are operated side by side with a reference instrument in Karlsruhe to derive instrument-specific scaling factors, which can be applied by the user to their retrieved GHG column data to maintain consistency between all EM27/SUN datasets, regardless of who operates the instrument and where. This work is done under the COllaborative Carbon Column Observing Network project (COCCON; Frey et al., 2019; Alberti et al., 2022), which also develops and maintains the PROFFAST retrieval software used to calculate atmospheric column concentrations from the measured interferograms. Information on the spectral ranges used in the retrievals, along with some example spectra and vertical column sensitivities, can be found in Appendix A.
As well as being used for validation studies in various locations (Jacobs et al., 2020; Tu et al., 2020; Frey et al., 2021), the portability and relatively low cost of the EM27/SUN have led to a variety of other scientific applications. In the city of Munich, Germany, a permanent network of five EM27/SUNs has been established to observe the city's carbon emissions using the differential column observation method (Chen et al., 2016; Dietrich et al., 2021) and provide a means of validating spatial gradients in OCO-2 target mode observations of X (Rißmann et al., 2022). Similar city-focused studies using EM27/SUNs have taken place in Berlin (Hase et al., 2015), St. Petersburg (Makarova et al., 2021), Beijing (Che et al., 2022; Zhou et al., 2022), and Indianapolis (Jones et al., 2021), amongst others. Further studies have taken advantage of the instrument's portability in another way, adapting the instrument with a specially designed solar tracker for operation on board a cargo ship to provide a unique opportunity for validation of satellite and model data over the ocean (Klappenbach et al., 2015; Knapp et al., 2021). Some of the studies listed here make use of various designs of weatherproof enclosures to operate the EM27/SUN more effectively; the enclosure we use here, developed at the Technical University (TU) of Munich, is described in Sect. 2.2.
2.2 An automated enclosure for the EM27/SUN
The EM27/SUN, whilst very useful for greenhouse gas column observations, is not suitable for unattended operations “out of the box”. Firstly, the instrument itself is not weatherproof, so the user has to keep a close eye on the weather forecast when deciding whether to set up for a day of measurements and be in close attendance to move it indoors in the case of rain. In addition, the user has to manually start the solar tracker and then the spectrometer at the beginning of each day, before powering down and moving it indoors once the day's observations are complete. This labour-intensive mode of operations works well for short-term measurement campaigns but is less suitable if the goal is to obtain long-term observations in a single location.
To make the EM27/SUN suitable for use on longer-term deployments, the Environmental Sensing and Modelling Group at TU Munich have developed an automated enclosure (Heinle and Chen, 2018; Dietrich et al., 2021) that provides weatherproofing, environmental control, and automation of the observations. The main components of the TU Munich enclosure are labelled in Fig. 2 and are described in detail by Dietrich et al. (2021).
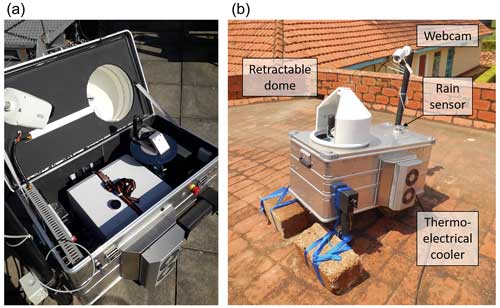
Figure 2(a) Internal view of the EM27/SUN spectrometer and solar tracker housed within the TU Munich enclosure system; (b) the enclosure system in operation at NaFIRRI, with two car jacks used to tilt the system to enable tracking of the sun at very high solar zenith angles (see text in Sect. 2.2). The bricks attached to the car jacks anchor the enclosure down in case of strong winds.
A modified Zarges K470 aluminium box is used for the main body of the enclosure system. On top of the housing, a rotating cover closes to protect the contents of the enclosure system when rain is detected by an optical rain sensor and overnight when no measurements are taking place. When conditions are dry during the daytime, the cover rotates to track the azimuth angle of the solar tracker and allow sunlight into the system.
The user controls and monitors the enclosure system by remotely accessing the enclosure computer, which also controls the EM27/SUN spectrometer and solar tracker and stores the measured interferograms. The automated features of the system are controlled by a programmable logic controller (PLC), ensuring that critical safety features protecting the system (detection of rain or power failure, control of the cover motor, temperature control) are not dependent on the enclosure computer. An additional challenge posed by the location of these measurements very close to the Equator is that of very high solar zenith angles, which at times are beyond the normal operating range of both the solar tracker and the protective cover. A pair of car jacks attached to the side of the enclosure (see Fig. 2) allow the entire enclosure system to be tilted such that the sun can be tracked throughout the middle of the day.
Control and automation of the enclosure system are achieved by two software programmes, both developed in-house at TU Munich (Dietrich et al., 2021). The first of these (Enclosure Control, or ECon; see Heinle and Chen, 2018) controls the enclosure itself – moving the rotating cover into the correct position, maintaining internal temperature using the thermo-electrical coolers, monitoring the rain sensor data and the uninterruptible power supply (UPS), and powering the spectrometer. ECon also checks that the Ethernet connections linking the different components of the enclosure system are working correctly and performs automatic restarts of specific components if a malfunction is detected. Alongside ECon, control of the spectrometer and solar tracker is automated using a Python programme called Pyra. Pyra effectively acts as a wrapper for the software provided by Bruker that controls the spectrometer and the solar tracker (OPUS and CamTracker, respectively), providing the means to start, stop, and control the software automatically. For these measurements we used Pyra in a semi-automated mode, which started and stopped the observations when the solar zenith angle passed a minimum threshold; a more detailed description of Pyra can be found in Appendix A of Dietrich et al. (2021), whilst the latest version is fully detailed by Aigner et al. (2023).
2.3 Total column concentrations over Jinja from the EM27/SUN and automated enclosure system
The data processing method we use, taking us from the raw interferograms measured by the EM27/SUN spectrometer to the column-averaged greenhouse gas abundances over Jinja presented in this paper, is described in more detail in Sect. 2.2 of Frey et al. (2021). The method comprises two parts, both written in FORTRAN: PREPROCESS, which performs fast Fourier transforms on the interferograms (that are first corrected for intensity fluctuations and apodised) to obtain solar absorption spectra, and PROFFAST, which then retrieves the column-averaged greenhouse gas abundances from the solar absorption spectra. Several quality filters, summarised in Table 1 of Frey et al. (2021), are applied to each interferogram by the PREPROCESS routine. The a priori profiles we use for trace gas concentrations, pressure, and temperature are those generated for use in the TCCON GGG2014 data version (Wunch et al., 2015). The profiles of pressure, temperature, geopotential height, and water vapour come from the National Centers for Environmental Prediction/National Center for Atmospheric Research (NCEP/NCAR; Kalnay et al., 1996) reanalysis and are used to generate the trace gas profiles using a set of empirical functions optimised to fit a range of in situ profile measurements, as described in Wunch et al. (2011).
We then apply the PROFFAST retrieval algorithm to spectra whose interferograms have passed the quality filters applied during the PREPROCESS stage. PROFFAST is a non-linear least squares algorithm which scales a priori trace gas profiles to fit forward-modelled atmospheric spectra to the measured spectra and then calculates the retrieved total column abundances from the scaled profiles. These are finally converted into column-averaged dry-air mole fractions Xgas, given by
where VCgas is the retrieved total column abundance for that gas. Taking the ratio of the total column abundances has the benefit of at least partially cancelling out any spectroscopic errors which affect both VCs in a similar way (Wunch et al., 2010) whilst also reducing the dependence on the measured ground pressure (Frey et al., 2021).
To monitor the stability of the spectrometer, we use the column-averaged amount of dry air (Xair). This is the ratio of the total column abundance of dry air calculated from the retrieved total column abundance of oxygen, , to the total column abundance of dry air calculated from the measured surface pressure, PS, and is given by
where the molecular masses of dry air and water vapour are given by and , respectively; g is the column-averaged gravitational acceleration; and VC is the retrieved water vapour total column (this correction is required to account for the measured surface pressure, which includes the whole-air column, whereas we retrieve the dry-air column using the oxygen absorption band). As long as the spectrometer is working nominally, Xair should remain close to 1.0 and stable over time. We therefore use Xair as a final quality filter on the retrieved column data by removing any data points where the difference from the daily median value of Xair is greater than 0.002 and then removing any further data points which deviate from the rolling hourly mean Xair by more than 0.0005.
A final step in the data processing is to apply calibration factors to the retrieved column concentrations, which bring the results in line with the rest of the EM27/SUNs involved in COCCON (as discussed earlier in this section, a full list of calibration factors is given in Table 6 of Frey et al., 2019). For the spectrometer used here (serial number 059), the calibration factors with respect to the reference EM27/SUN operated by the Karlsruhe Institute of Technology (serial number 037) are 0.9998, 0.9991, and 1.0019 for X, X, and O2, respectively. The column concentrations retrieved by following the procedure described above are shown in Fig. 3, along with the number of quality-controlled soundings obtained on each measurement date. The daily count of measurements leading to a valid retrieval is determined by a combination of weather conditions (cloudy vs. cloud-free) and the availability of mains power on the NaFIRRI site during times of the day when the sun is at least 20° above the horizon.
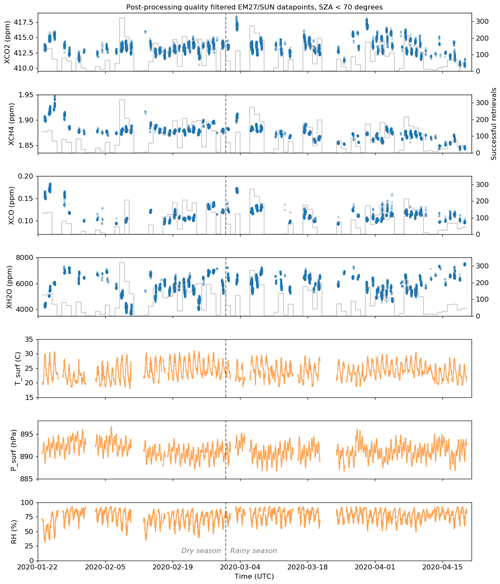
Figure 3From top to bottom: column concentrations of CO2, CH4, CO, and H2O retrieved from the EM27/SUN measurements using the PROFFAST algorithm, as described in Sect. 2.3, as well as surface air temperature, surface pressure, and relative humidity. The solid line in the upper four panels indicates the number of successful retrievals in each day, for each gas. The vertical dashed line marks the onset of the long rains as described in Sect. 2.
In this section, we introduce the satellite and model datasets that we compare with our Jinja EM27/SUN column concentration data.
3.1 OCO-2 and OCO-3 X retrievals
The Orbiting Carbon Observatory-2 (OCO-2) was launched in 2014 and was specifically designed by NASA to have the precision required to detect the changes in that correspond to surface emissions and uptake of CO2, on a regional scale with global coverage (Eldering et al., 2017). The sole OCO-2 payload comprises a three-band grating spectrometer, which measures the radiance spectra of sunlight reflected back into space by the Earth's surface. Of the three spectral bands, two coincide with carbon dioxide absorption features (the so-called “weak” and “strong” CO2 bands, centred at wavelengths of 1.6 and 2.0 µm, respectively), whilst the third band at 0.76 µm is used to measure absorption by molecular oxygen. The instrument samples eight spatial footprints across track, which are each nominally 1.25 km in width at the surface. Along track, each footprint is around 2.4 km in length owing to the distance travelled by the satellite during the instrument's 0.33 s integration time. The orbit track and the narrow swath width (approximately 10 km wide) mean that the same ground location is resampled once every 16 d. A full-physics retrieval algorithm based on an optimal estimation technique is used to retrieve from the OCO-2-measured spectra (O'Dell et al., 2012, 2018), taking into account multiple-scattering and polarisation effects. The retrieved column concentrations are validated against the TCCON ground-based network of Bruker 125HR spectrometers (Wunch et al., 2017). For this study, we use version 10r of the OCO-2 data (Taylor et al., 2023) – the spatially gridded mean CO2 column concentrations from this dataset observed over East Africa during our measurement period are shown in Fig. 4a.
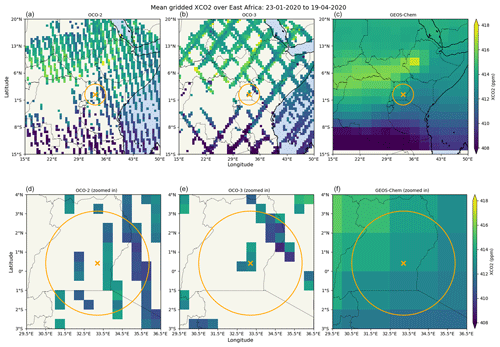
Figure 4X over East Africa retrieved from OCO-2 (a) and OCO-3 (b) observations, averaged over the EM27/SUN measurement period (23 January to 19 April 2020) and spatially binned into a 0.5° × 0.5° grid. The orange cross shows the location of the measurement site in Jinja, and the circle indicates the co-location criteria (300 km radius) used in the comparisons described in Sect. 4.1. Panel (c) shows the X output from the global GEOS-Chem inversion described in Sect. 3.3. Panels (d), (e), and (f) show the same data as panels (a), (b), and (c), respectively, zoomed in on the co-location region.
In 2019 NASA also integrated the flight spare of OCO-2, under the name Orbiting Carbon Observatory-3 (OCO-3; Eldering et al., 2019; Taylor et al., 2020), onto the International Space Station (ISS). The low-inclination orbit occupied by the ISS introduces significant differences to the sampling pattern, with the main implication being that overpasses of a particular location do not take place at the same local time each day. Instead, the overpass time shifts about 20 min earlier from 1 d to the next such that all times of the day are eventually observed. In contrast to the observations obtained from the sun-synchronous orbit followed by OCO-2, this means that OCO-3 can provide information on how varies with the time of the day. In addition, the pointing mirror assembly (PMA) allows for pointing towards the ocean glint spot to maximise the observed signal over water (for OCO-2 the whole spacecraft is rotated to achieve a similar goal) or towards stationary ground targets, such as validation sites. Uniquely to OCO-3, snapshot area maps (SAMs) can also be acquired. These involve sweeping the PMA fore-optics back and forth across an area approximately 85 km × 85 km in size, effectively producing spatially resolved two-dimensional images of over areas of interest. The same full-physics retrieval algorithm used on OCO-3 measurements is applied to OCO-2 to obtain the column concentrations of CO2. Here we use version 10.4r of the OCO-3 data (Taylor et al., 2023). The gridded mean over East Africa for our whole measurement period is shown in Fig. 4b, which clearly illustrates the different spatial sampling pattern employed by OCO-3 compared with OCO-2 (Fig. 4a). Note that Jinja is included on the list of targets for the SAM mode such that the OCO-3 soundings taken as the ISS passes over Uganda tend to be concentrated within a short distance of our measurement site.
3.2 TROPOMI X and XCO retrievals
The Copernicus Sentinel-5 Precursor (S5P) mission was launched in October 2017 to measure atmospheric composition, specifically air quality and climate change indicators, with daily global coverage and moderately high (up to 5.5 km × 3.5 km at nadir) spatial resolution. The sole payload of the S5P mission is the TROPOspheric Monitoring Instrument (TROPOMI; Veefkind et al., 2012), a grating spectrometer with four spectral bands covering ultraviolet, visible, near-infrared (NIR), and shortwave infrared (SWIR) wavelength ranges. The S5P operational CH4 retrieval algorithm uses the NIR and SWIR spectral channels in a full-physics, optimal estimation method to derive the column-averaged dry mixing ratio of methane (X) from the TROPOMI measurements (Hu et al., 2016; Hasekamp et al., 2021). The data used in this study were processed using version 01.03.02 of the TROPOMI CH4 processor, which has been shown to perform well within the mission requirements through comparison with ground-based observations from 28 TCCON stations (Sha et al., 2021). This version includes a surface-albedo-dependent a posteriori bias correction, based on comparisons between co-located TROPOMI and GOSAT data (Hasekamp et al., 2021). We show the gridded mean from this dataset over East Africa, averaging over our whole measurement period, in Fig. 5a. More recent TROPOMI data (measurements from 1 July 2021 onwards) use version 2 of the processor, which incorporates a number of improvements, including updated CH4, CO, and H2O spectroscopic cross sections and an updated a posteriori bias correction that is independent of external reference data (Lorente et al., 2021).
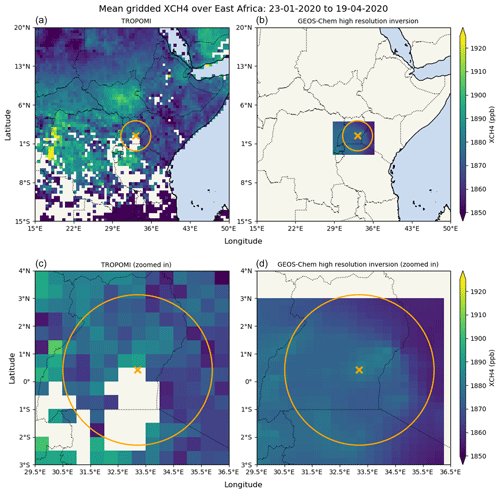
Figure 5X over East Africa retrieved from TROPOMI observations (a; see Sect. 3.2) and calculated from a high-resolution GEOS-Chem inversion (b; see Sect. 3.3 for details), averaged over the EM27/SUN measurement period (23 January to 19 April 2020). The TROPOMI data are spatially binned into a 0.5° × 0.5° grid. The orange cross shows the location of the measurement site in Jinja, and the circles indicate the co-location criteria (300 km radius for X) used in the comparisons described in Sect. 4.2. Panels (c) and (d) show the same data as panels (a) and (b), respectively, zoomed in on the co-location region.
The TROPOMI SWIR band is also used to retrieve the total column abundances of carbon monoxide, XCO (Landgraf et al., 2016). The retrieval uses a two-step process: first, a non-scattering retrieval of the total amount of CH4 is performed and then compared with modelled methane abundances to act as a cloud filter (if the retrieved CH4 assuming no scattering differs significantly from the model value, this indicates that the impact of scattering from high or optically thick clouds is too great to perform a useful carbon monoxide retrieval). In the second step, the CO column is retrieved using a profile scaling approach, along with the H2O abundance and effective cloud parameters using the a priori knowledge of methane acquired during the first step. Validation of the operational TROPOMI XCO against ground-based TCCON observations has demonstrated that the requirements for systematic and random uncertainties in the data are being met (Sha et al., 2021). Figure 6a shows the gridded mean XCO from the operational TROPOMI product for our measurement period, over the East Africa region. The less strict requirements with respect to accuracy and precision for XCO compared with those for allow retrievals to be made over land and ocean scenes, under both clear-sky and (with the exception of high or optically thick clouds) cloudy conditions. This is reflected in the comprehensive spatial coverage shown in Fig. 6a that is achieved by the XCO retrieval compared with that of (as seen in Fig. 5a), which requires cloud-free conditions and minimal scattering for a successful retrieval.
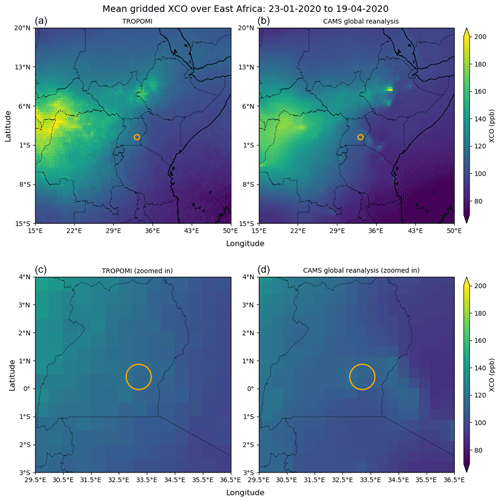
Figure 6XCO over East Africa retrieved from TROPOMI observations (a; see Sect. 3.2) and calculated from a global CAMS reanalysis (b; see Sect. 3.3 for details), averaged over the EM27/SUN measurement period (23 January to 19 April 2020). The TROPOMI data are spatially binned into a 0.5° × 0.5° grid. The circles indicate the co-location criteria (50 km radius for XCO, centred on the measurement site in Jinja) used in the comparisons described in Sect. 4.3. Panels (c) and (d) show the same data as panels (a) and (b), respectively, zoomed in on the co-location region.
3.3 GEOS-Chem and Copernicus Atmosphere Monitoring Service (CAMS) concentration data
GEOS-Chem is an atmospheric chemistry transport model that is used here to simulate the emissions, sinks, chemistry, and transport of carbon dioxide and methane (Turner et al., 2015; Feng et al., 2017; Lunt et al., 2019, 2021) and produce three-dimensional fields of their concentrations. This can provide a useful extension of satellite data in spatial regions and at times of the day when the satellite data are not available. For a more detailed description of the GEOS-Chem model and the ensemble Kalman filter inverse method used, the reader is referred to the papers cited below.
For carbon dioxide, we use a global GEOS-Chem model run on a 2.0° × 2.5° latitude–longitude grid with 47 vertical levels. We use emissions inventories for our a priori flux estimates, taking into account CO2 emissions from biomass burning (van der Werf et al., 2010), fossil fuels (Oda et al., 2018), ocean fluxes (Takahashi et al., 2009), and biosphere fluxes (Olsen and Randerson, 2004). An ensemble Kalman filter approach is then used to estimate the CO2 fluxes, with either in situ or satellite measurements of atmospheric CO2 used as prior information on concentration (Feng et al., 2009; Palmer et al., 2019). The mean X values for the measurement period calculated from the output of this global inversion are shown in Fig. 4c.
In the case of methane, we run GEOS-Chem in a nested configuration at high spatial resolution (0.25° × 0.3125°) over a latitude–longitude box covering sub-Saharan Africa (−36.0 to +20.0° N, −20.0 to 55.0° E), using the setup described in detail by Lunt et al. (2021). The inversion analysis we show here is an extension of the inversion presented in Lunt et al. (2021), from the end of 2019 to the first 4 months of 2020. For the a priori methane emissions inside the nested domain, we use the EDGAR v4.3.2 database for anthropogenic emissions (Janssens-Maenhout et al., 2019), the WetCHARTs dataset for emissions from wetlands (Bloom et al., 2017), and the Global Fire Assimilation System database for daily biomass burning emissions (GFAS; Kaiser et al., 2012). The boundary conditions for the nested domain come from a global GEOS-Chem model run at lower spatial resolution (2.0° × 2.5°). An ensemble Kalman filter system (Hunt et al., 2007) is then used to perform the inversion, taking into account column CH4 concentrations from TROPOMI (Lunt et al., 2021), which gives us estimates of the methane emissions within the nested domain along with the model-derived atmospheric concentrations. A subset of the mean X values for the measurement period, calculated from the output of this high-resolution regional inversion, covering the region surrounding the Jinja site (−3.0 to +3.0° N, +28.0 to +36.0° E), is shown in Fig. 5b.
The non-satellite dataset we use in this study for carbon monoxide is the Copernicus Atmosphere Monitoring Service (CAMS) global reanalysis dataset (Inness et al., 2019a), which covers the period from January 2003 to December 2021 with a spatial resolution of approximately 80 km (interpolated onto a regular 0.75° × 0.75° grid) and 60 vertical levels. A 4D-Var assimilation framework (Rabier et al., 2000; Hollingsworth et al., 2008) is used to produce the reanalysis, which is described in detail for carbon monoxide by Flemming et al. (2017). Total column carbon monoxide data retrieved from the Measurements Of Pollution In The Troposphere (MOPITT) instrument on board the NASA Terra satellite are used as input for the reanalysis (Deeter et al., 2014). Figure 6b shows the mean column concentration of carbon monoxide over East Africa for the measurement period, as calculated from the CAMS reanalysis output.
In this section we show how the total column concentrations observed using the EM27/SUN in Jinja compare with both satellite and model datasets, considering each species in turn. Before comparing the EM27/SUN and satellite data, we need to take into account that each retrieval algorithm used provides an estimate of the total column concentration that is based on different a priori information. Following the theory underpinning the optimal estimation retrieval method as described by Rodgers (2000), we correct for the different a priori profiles used in PROFFAST (see Sect. 2.3) and the respective satellite algorithms following Eq. (3) in Dils et al. (2014), which assumes the ground-based a priori profile to be the common a priori profile when validating satellite GOSAT data and ground-based TCCON total column concentrations of carbon dioxide and methane:
Here, xcor and x are the a-priori-corrected and a-priori-uncorrected dry-air total column concentrations and i is the vertical layer index, with the corresponding mass of dry air mi contained within the layer (derived from , where Δpi is the dry-air pressure difference over layer i, and gi is the acceleration due to gravity at that height). m0 is the total dry-air mass of the atmospheric column, obtained by taking the sum of mi over all layers. Ai is the column-averaging kernel used by the satellite retrieval algorithm, and, finally, api,sat and api,EM27 are the a priori dry-air concentrations in layer i assumed by the satellite and EM27/SUN retrieval algorithms, respectively.
For these comparisons, we use co-location criteria, which represent a trade-off between ensuring there are enough measurement days to be able to make meaningful conclusions from the observed EM27/SUN vs. satellite/model differences and ensuring that we are spatially comparing like with like. In the case of carbon dioxide retrievals from OCO-2 and OCO-3 and methane retrievals from TROPOMI, we employ a wider co-location radius (300 km) than that used in the validation study of Sha et al. (2021), as the cloudy conditions commonly encountered in the tropics limit the number of successful satellite retrievals. The close proximity of Lake Victoria to the south of the measurement site also has an impact here, as the low albedo of the lake surface at shortwave infrared wavelengths reduces the intensity of the observed signal below the level where a successful or retrieval is possible. We also split the time period in two to check whether there is a notable difference in the comparisons as a result of the onset of the long rains in March (see Sect. 2). In the following sections the two subsets are labelled “dry”, corresponding to January and February, and “rainy”, corresponding to the long-rain period from March onwards.
4.1 Comparison of EM27/SUN X with OCO-2, OCO-3, and GEOS-Chem
Here, we compare our EM27/SUN values with those retrieved from OCO-2 and OCO-3 observations (a priori corrected as described above) and those obtained from a global GEOS-Chem CO2 inversion which assimilates OCO-2 v10r data (see Sect. 3.3 for details). For the comparison we take OCO-2 and OCO-3 soundings (see Sect. 3.1) and GEOS-Chem grid points within a 300 km radius of the EM27/SUN location and calculate the median for each day (see Table B1 for a summary of how the choice of co-location radius affects the comparison). For we use all EM27/SUN data points, regardless of the time of day, in order to maximise the number of days of coincident OCO-2 and OCO-3 observations and to take into account the varying OCO-3 overpass time. We also limit the OCO-2 and OCO-3 vs. EM27/SUN comparison to days where there are at least 10 soundings of sufficient quality that meet the co-location criteria described here. Figure 7 shows time series of these data, along with scatterplots directly comparing the EM27/SUN daily with the satellite and model datasets. The statistics of the comparisons are summarised in Table 1. The mean (standard deviation) a priori profile corrections given by Eq. (3) are −0.238 (0.013) and −0.373 (0.082) ppm for OCO-2 and OCO-3, respectively.
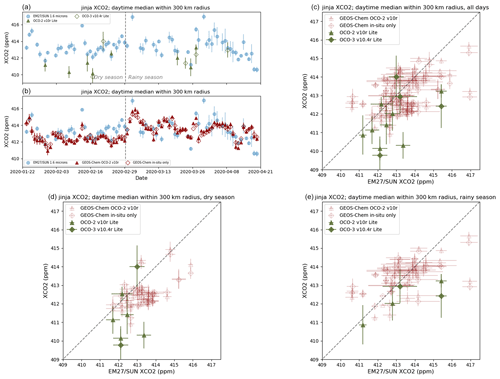
Figure 7(a) Daily median values of retrieved from EM27/SUN (blue circles), OCO-2 (filled olive-coloured triangles), and OCO-3 (open olive-coloured diamonds) measurements; the error bars show the interquartile range. (b) The same as panel (a) but with global GEOS-Chem model data (the filled red triangles are with OCO-2 data assimilated; the open red diamonds use in situ observations only). (c) Scatterplot showing EM27/SUN daily median observations vs. OCO-2 (filled olive-coloured triangles), OCO-3 (filled olive-coloured diamonds), and global GEOS-Chem (the open red triangles are with OCO-2 assimilated; the open red diamonds are with in situ data only); the error bars show the interquartile range. We use a co-location radius of 300 km. (d) The same as panel (c) but including data from the dry season only (January and February 2020). (e) The same as panel (c) but including data from the rainy season only (March and April 2020).
Table 1Mean and standard deviation of the differences ( in ppm) between daily median EM27/SUN and satellite/model . The dry subset includes data from January and February 2020, whilst the rainy subset covers data from March and April 2020.
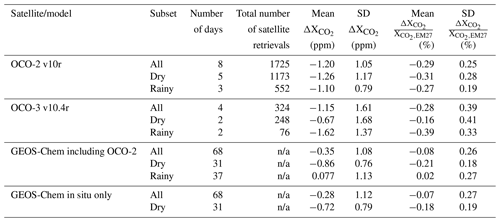
n/a: not applicable.
Whilst acknowledging that there are only a few days during the measurement period where OCO-2 and OCO-3 data can be compared with our EM27/SUN measurements, the data that we have available suggest that during this period the from OCO-2 is biased low with respect to that from the EM27/SUN by 1.20 ppm (standard deviation is 1.05 ppm). The data from OCO-3 are also lower on average than what we observed with the EM27/SUN; however there are insufficient days of coincident observations during the measurement period to conclude that there is a statistically significant difference between the two. In both cases, the percentage difference from the EM27/SUN measurements (−0.29 % for OCO-2, −0.28 % for OCO-3) falls just outside the OCO mission precision requirement of 0.25 %.
The GEOS-Chem model columns are also biased low on average with respect to the EM27/SUN data, and for our measurement period the inversion is insensitive to whether both satellite and in situ or only in situ observational data are assimilated. We see in both cases that these biases are primarily observed during the dry period, where there are statistically significant differences from the EM27/SUN columns of −0.86 and −0.72 ppm, respectively (standard deviations are 0.76 and 0.79 ppm). The differences are most clear during the last week of February 2020 (see Fig. 7b) and suggest the possibility of local sources not captured by the relatively coarse (2.0° × 2.5° latitude–longitude grid) global configuration of GEOS-Chem used here.
4.2 Comparison of EM27/SUN X with TROPOMI and GEOS-Chem
For we compare the EM27/SUN column concentrations with a-priori-corrected data from TROPOMI observations (see Sect. 3.2) and column concentrations calculated using a priori and a posteriori emissions from the high-resolution GEOS-Chem inversion (see Sect. 3.3 for details of the model run). A 300 km co-location radius is used for both satellite and model data, and we only use EM27/SUN data and GEOS-Chem time steps within ±2 h of the TROPOMI overpass time to calculate the median for each day (see Table B2 for a summary of how the choice of co-location radius affects the comparison). We also restrict the TROPOMI vs. EM27/SUN comparison to days where there are at least 10 soundings of sufficient quality meeting the spatial and temporal co-location criteria described here. Figure 8 shows time series of these data, along with scatterplots directly comparing the EM27/SUN daily with the satellite and model datasets. The statistics of the comparisons are summarised in Table 2. The mean (standard deviation) a priori profile correction applied to the TROPOMI data, given by Eq. (3), is +1.56 (0.15) ppb.
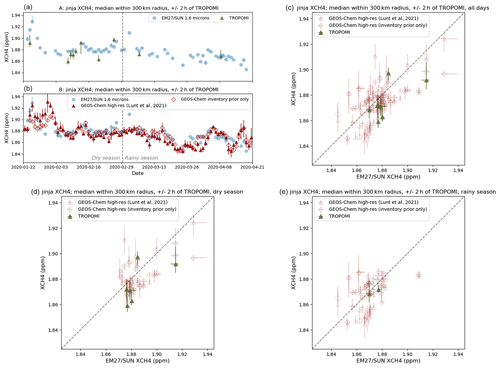
Figure 8(a) Daily median values of retrieved from EM27/SUN (blue) and TROPOMI (dark green) measurements; the bars show the interquartile range. (b) The same as panel (a) but with high-resolution regional GEOS-Chem model data (the darker shade of red is with TROPOMI data assimilated; the lighter shade uses inventory emissions only). (c) Scatterplot showing EM27/SUN observations vs. TROPOMI (filled dark green circles) and high-resolution regional GEOS-Chem (the open red circles are with TROPOMI assimilated; the blue diamonds are with inventory emissions only); the error bars show the interquartile range. We use a co-location radius of 300 km and only consider data and model output within ±2 h of the TROPOMI overpass at 10:30 UTC. (d) The same as panel (c) but including data from the dry period only (January and February 2020). (e) The same as panel (c) but including data from the rainy period only (March and April 2020).
Table 2Mean and standard deviation of the differences ( in ppb) between daily median EM27/SUN and satellite/model . The dry subset includes data from January and February 2020, whilst the rainy subset covers data from March and April 2020.
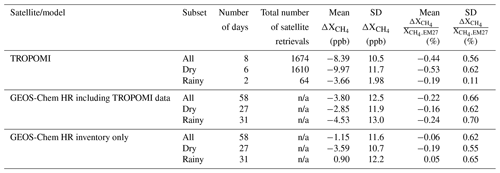
n/a: not applicable.
The short measurement period limits the number of days where comparisons can be made between the ground-based and satellite retrievals of . In the data we have, the TROPOMI retrievals are lower than the EM27/SUN columns by a mean of 8.33 ppb, albeit within the standard deviation (10.5 ppb) in the data. The mean percentage difference from the EM27/SUN measurements of −0.44 % falls within the 1.5 % bias requirement on the TROPOMI data product.
The data from the GEOS-Chem high-resolution inversions show better agreement with the EM27/SUN data in terms of the mean differences. The difference is slightly greater (−3.80 ppb compared with −1.15 ppb) when the a posteriori emissions incorporating TROPOMI are used, though the difference between the two is well within their respective standard deviations. The only comparison where there is a statistically significant difference from the EM27/SUN columns is that with the GEOS-Chem simulation using a posteriori emissions, though there is not sufficient data to attribute this to either the dry or the rainy periods considered here.
There are a couple of possible explanations for the differences seen between the EM27/SUN and GEOS-Chem data. Firstly, the posterior scale factors, which are applied to the prior emission fields, have an exponential correlation length scale of 50 km, meaning that smaller-scale variations in the emissions that influence the EM27/SUN measurements may not be reflected in the differences between the posterior and prior inversions. It is also worth noting that the a posteriori inversion minimises the residual to all TROPOMI data within the larger inversion domain rather than this specific grid box centred on Jinja. We can see from the EM27/SUN vs. TROPOMI comparison in Fig. 8 that there are only a limited number of TROPOMI data available to constrain emissions during the measurement period such that local emissions at the site are unlikely to be well represented in the inversion.
4.3 Comparison of EM27/SUN XCO with TROPOMI and CAMS
In the final part of this section we compare XCO retrieved from the EM27/SUN ground-based observations with XCO from TROPOMI data (see Sect. 3.2) and from the output of the global CAMS reanalysis (see Sect. 3.3 for details). The greater number of soundings with successful retrievals of XCO allows us to apply a narrower 50 km co-location radius to the satellite and reanalysis data (see Table B3 for a summary of how the choice of co-location radius affects the comparison). As for , we only use EM27/SUN data and CAMS time steps within ±2 h of the Sentinel-5P overpass time to calculate the median XCO value for each day. In addition we further restrict the TROPOMI vs. EM27/SUN comparison to days where there are at least 10 XCO soundings of sufficient quality meeting these spatial and temporal co-location criteria. Figure 9 shows time series of these data, along with scatterplots directly comparing the EM27/SUN daily XCO with the satellite and reanalysis datasets. The statistics of the XCO comparisons are summarised in Table 3. The mean (standard deviation) a priori profile correction applied to the TROPOMI data, given by Eq. (3), is −2.84 (3.27) ppb.
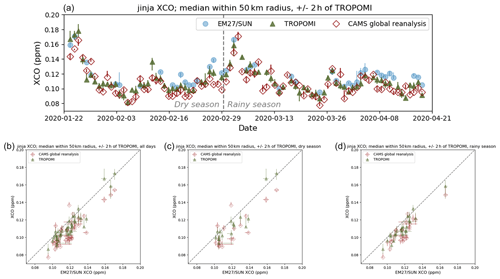
Figure 9(a) Daily median values of XCO retrieved from EM27/SUN (filled blue circles) and TROPOMI (filled dark green triangles) measurements and obtained from CAMS global reanalysis output (open red diamonds); the bars show the interquartile range. (b) Scatterplot showing EM27/SUN observations vs. TROPOMI (filled dark green triangles) and global CAMS reanalysis data (open red diamonds); the error bars show the interquartile range. We use a co-location radius of 50 km and only consider data and model output within 2 h of the TROPOMI overpass at 10:30 UTC. (c) The same as panel (b) but including data from the dry period only (January and February 2020). (d) The same as panel (b) but including data from the rainy period only (March and April 2020).
Table 3Mean and standard deviation of the differences (ΔXCO in ppb) between daily median EM27/SUN and satellite/reanalysis XCO. The dry subset includes data from January and February 2020, whilst the rainy subset covers data from March and April 2020.
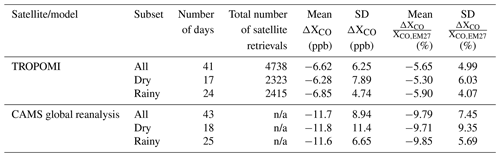
n/a: not applicable.
As discussed in Sect. 3.2, the availability of XCO data from TROPOMI in partially cloudy conditions means that we have a greater number of measurement days suitable for comparison compared with and . We find a statistically significant difference between our ground-based observations and the TROPOMI satellite data, with the XCO from TROPOMI being biased lower than that from the EM27/SUN by a mean value of 6.62 ppb, which falls just outside the standard deviation (6.25 ppb) of the bias. These values are still well within the mission data requirements for the TROPOMI carbon monoxide data product, which stipulate that the magnitude of the bias (−5.65 % for the whole measurement period, with a standard deviation of 4.99 %) should be less than 15 % and the random error less than 10 % (Landgraf et al., 2016).
The XCO values from the CAMS global reanalysis are also significantly low with respect to the ground-based EM27/SUN data by a mean value of 11.7 ppb. This mean bias is greater than the standard deviation (8.94 ppb) both throughout our measurement period and when separating the measurement period into dry and rainy periods, suggesting that the CAMS global model may not be taking into account all local sources of carbon monoxide. In addition, recent work by Inness et al. (2022) has shown that assimilating TROPOMI carbon monoxide data into the CAMS system (in addition to the satellite data from MOPITT and IASI that are already assimilated in this version) increases the CAMS carbon monoxide columns by 8 % on average, which would bring the CAMS reanalysis output into closer agreement with our EM27/SUN observations.
In this paper, we describe the first ground-based remote sensing observations of total column greenhouse gas concentrations to have been performed in the tropical East Africa region. We set up a Bruker EM27/SUN spectrometer at the headquarters of the National Fisheries Resources Research Institute in Jinja, Uganda, in January 2020. An automated enclosure for the instrument, designed and built by the Environmental Sensing and Modelling Group at the Technical University of Munich, allowed us to operate the instrument remotely and autonomously for a period of 3 months, providing a temporal density of greenhouse gas column data over this period that would have been challenging to achieve manually. The combined performance of the instrument and enclosure shown in this paper demonstrates the possibility to deploy EM27/SUN instruments as validation sites for satellite greenhouse gas retrievals, in parts of the world where it would be logistically difficult to establish new sites to extend established ground-based validation networks such as TCCON.
The ground-based measurements of carbon dioxide, methane, and carbon monoxide column concentrations that we acquired using the EM27/SUN and automated enclosure allow us, for the first time, to see how well satellite and model datasets performed in observing or calculating these concentrations over Uganda during our measurement period. For carbon dioxide, we find OCO-2 to be lower than our EM27/SUN measurements by a mean of 1.20 ppm, with a standard deviation 1.05 ppm. Given the lack of days of coincident observations during the measurement period, we do not observe a statistically significant difference between EM27/SUN and OCO-3 for this dataset (for the days we do have coincident observations, OCO-3 was lower by a mean of 1.15 (1.61) ppm). In both cases, the percentage difference from the EM27/SUN measurements (−0.29 % for OCO-2, −0.28 % for OCO-3) falls just outside the OCO mission precision requirement of 0.25 %. The global GEOS-Chem inversion we use for this study – irrespective of whether OCO-2 data have been assimilated – is also generally biased low with respect to the EM27/SUN measurements. We find GEOS-Chem to be lower by a mean of 0.35(1.08) ppm with OCO-2 included and 0.28 (1.12) ppm without OCO-2 included in the inversion.
In the case of , we find that TROPOMI is lower than our EM27/SUN by a mean of 8.33 (10.5) ppb, albeit for a limited number of days where we had coincident observations. However, the mean percentage difference from the EM27/SUN measurements of −0.44 % does fall within the 1.5 % bias requirement on the TROPOMI data product. The high-resolution GEOS-Chem inversion we use in this study, which incorporates TROPOMI data, is also biased low with respect to the EM27/SUN-observed (GEOS-Chem is lower by a mean of 3.80 (12.5) ppb). This may be a result of the a posteriori inversion being set up to minimise the residual to all TROPOMI data within the larger inversion domain rather than just data within the specific grid box centred on Jinja where TROPOMI methane soundings are relatively scarce. This means that local emissions at the measurement site are unlikely to be well represented in the inversion.
When we consider carbon monoxide from TROPOMI, the quality flagging of the column concentration retrievals is much less sensitive to cloud cover such that there were many more days with coincident observations that we could compare with our EM27/SUN measurements. Even over a 3-month period were there sufficient data to be able to conclude that the carbon monoxide columns from TROPOMI were biased low with respect to the EM27/SUN data by a mean value of 3.68 ppb (standard deviation of 7.00 ppb). This is still well within the mission data requirements for the TROPOMI carbon monoxide data product, which stipulate that the bias (−5.65 % for the whole measurement period, with a standard deviation of 4.99 %) should be less than 15 % and the random error less than 10 % (Landgraf et al., 2016). We also see a statistically significant difference between our EM27/SUN measurements and the CAMS global reanalysis (CAMS XCO lower by a mean of 11.7 ppb, with a standard deviation of 8.94 ppb), suggesting that the CAMS global reanalysis may not be taking into account all local sources of carbon monoxide. Recent work by Inness et al. (2022) has, however, shown that assimilating TROPOMI carbon monoxide data into the CAMS system (in addition to the satellite data from MOPITT and IASI that are already assimilated in this version) would increase the CAMS carbon monoxide columns by 8 % on average, which, if applicable to tropical East Africa, would bring the CAMS reanalysis output into closer agreement with our EM27/SUN observations.
An important aspect of this work is the comparison with atmospheric chemistry and transport model output. Models and reanalyses such as GEOS-Chem and CAMS provide a means of studying atmospheric processes where observations are not available. This is of particular relevance in tropical Africa (e.g. Lunt et al., 2019, 2021; Palmer et al., 2019; Feng et al., 2022), where ground-based observations of greenhouse gases are scarce, and the data coverage provided by satellites is often limited by cloud cover. Ground-based column concentration observations such as those presented in this study provide data that can be used to evaluate these models and reanalyses which, unlike in situ measurements, are not overly sensitive to emission sources on a local scale. Our results show that only 3 months of measurements can be sufficient to demonstrate the effectiveness of these models and reanalyses at this time of the year whilst also highlighting short periods where there are discrepancies that need to be investigated further. A comprehensive validation of the models would require at least a whole calendar year of observations. Figure 10 shows radial histograms of the wind direction for each month of the year 2020 from the ERA5 reanalysis dataset (Hersbach et al., 2023). These demonstrate how the typical wind direction at 800 hPa, in the lower troposphere where the retrieved column concentrations are generally most sensitive, varies throughout the year. During the measurement period for this study, as summarised by the radial histogram in Fig. 10a, the wind most frequently blew from the north (particularly in January and February) and from the southeast (from late February to April). A full year of measurements would be more representative of the variety of atmospheric conditions we would expect to observe from satellites or estimate from models and would give us greater confidence in the performances of the retrieval algorithms and the model and reanalysis calculations, respectively. The period from October to December would be particularly interesting to focus on in the future, as the typically northerly winds we see at that time of the year (Fig. 10b) coincide with the short rains. Lunt et al. (2021) have linked the intensity of these rains to changes in methane emissions from the Sudd wetlands, located in South Sudan, north of the measurement site.
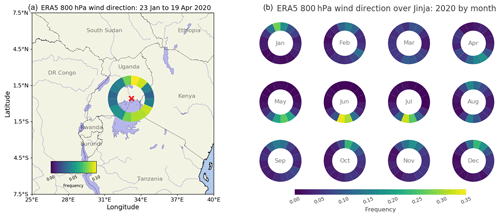
Figure 10(a) Radial histogram showing frequency of wind direction in the ERA5 reanalysis at 800 hPa above Jinja during the measurement period for this study. (b) Radial histograms showing the frequency of wind direction at 800 hPa in ERA5 over Jinja for each month in 2020. The angles of the histogram segments correspond to the direction that the wind is coming from. Note that we use different colour scales in panels (a) and (b).
In summary, this study demonstrates the feasibility of a longer-term, autonomous deployment of the EM27/SUN instrument in a tropical environment, through the use of an automated weatherproof enclosure. This EM27/SUN instrument with an enclosure system allows us to meet the goal of seasonal observations in support of studies focusing on the tropical carbon cycle and the validation of greenhouse gas column concentration data from satellite retrievals and from model and reanalysis calculations in the tropical East Africa region.
Here, we provide further details on the EM27/SUN instrument and PROFFAST retrieval outputs for the reader's information. The spectral windows used by the PROFFAST retrieval code described in Sect. 2.3 are summarised in Table A1, and in Fig. A1 we show an example of spectra and spectral residuals, which can be used to check how well the retrieval has performed. Finally, Fig. A2 shows example column sensitivities for carbon dioxide, methane, and carbon monoxide, presented as a function of pressure and solar zenith angle.
Table A1Summary of the spectral windows used in the PROFFAST retrieval algorithm. The primary detector window near 1.6 µm is used for the results shown in this study.
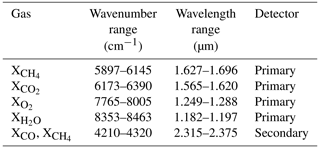
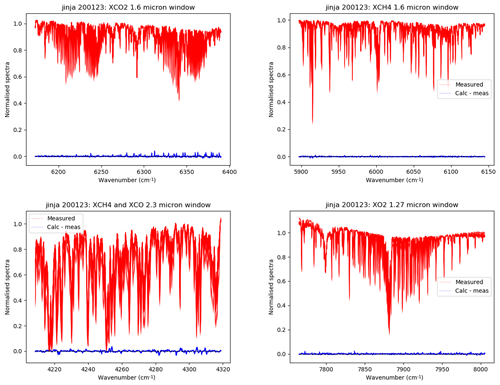
Figure A1Example of EM27/SUN spectra (shown in red) and spectral residual (shown in blue) output by PROFFAST for measurements taken on 23 January 2020.
In these tables, we show how the mean and standard deviation of the differences between the EM27/SUN and satellite Xgas vary with an increasing co-location radius. In each case, when choosing the co-location radius, we compromise between expanding the radius enough to include sufficient data for a meaningful comparison across the measurement period whilst also keeping the radius narrow enough so that we minimise the potential influence of geospatial biases.
Table B1Mean and standard deviation of the differences ( in ppm) between daily median EM27/SUN and satellite , assuming a range of different spatial co-location criteria. The co-location radius chosen for this study is highlighted in bold. The 100 and 200 km rows are identical for OCO-3 because of the sampling pattern used, which targets the measurement site whilst the ISS passes overhead (see Fig. 4).
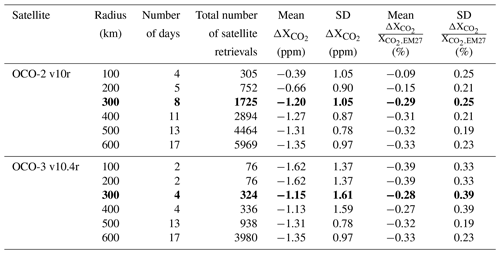
Table B2Mean and standard deviation of the differences ( in ppb) between daily median EM27/SUN and satellite , assuming a range of different spatial co-location criteria. The co-location radius chosen for this study is highlighted in bold.
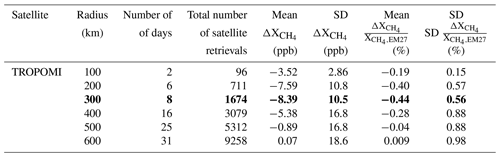
The EM27/SUN column data (https://doi.org/10.5285/7a8d0936ba1e4e1a8689c9e9010b43b2, Humpage et al., 2024) and GEOS-Chem data (https://doi.org/10.5285/925816bd869644ad9fe9b877d8f42d30, Feng and Palmer, 2024; https://doi.org/10.5285/7ecc607cb09747a59da6f46a0635f469, Lunt and Palmer, 2024) are available from the Centre for Environmental Data Analysis archive. The latest version of the Pyra software used to control the automated weatherproof enclosure is available at https://doi.org/10.21105/joss.05131 (Aigner et al., 2023). The latest version of the PROFFAST interferogram processing and analysis code is available from https://doi.org/10.21105/joss.06481 (Feld et al., 2024). The L2 column carbon dioxide data from OCO-2 and OCO-3 are available from the Goddard Earth Sciences Data and Information Services Center (https://doi.org/10.5067/E4E140XDMPO2, OCO-2 Science Team, 2020; https://doi.org/10.5067/970BCC4DHH24, OCO-2/OCO-3 Science Team, 2022). The TROPOMI column methane and carbon monoxide data are available from the Copernicus Data Space Ecosystem https://doi.org/10.5270/S5P-3lcdqiv (Copernicus Sentinel-5P, 2021a) and Copernicus Sentinel-5P2021b (Copernicus Sentinel-5P, 2021b). The CAMS global reanalysis carbon monoxide concentration data are available from the CAMS Atmosphere Data Store (see https://www.ecmwf.int/en/research/climate-reanalysis/cams-reanalysis, Inness et al., 2019b). The ERA5 reanalysis wind data are available from the Copernicus Climate Data Store (https://doi.org/10.24381/cds.bd0915c6, Hersbach et al., 2023).
NH set up the measurements, remotely monitored the instrument performance during the study period, performed the EM27/SUN data processing and analysis, prepared the figures, and wrote the paper. HB conceived the study, advised on interpretation of the results, and reviewed the paper. WO provided and prepared the measurement site, helped set up the measurements, oversaw the site once measurements were up and running, and reviewed the paper. JC (who also reviewed the paper) and FD developed and built the automated weatherproof enclosure for the EM27/SUN used in this study. MFL performed the GEOS-Chem methane model runs, advised on interpretation of the results, and reviewed the paper. LF performed the GEOS-Chem carbon dioxide model runs and advised on their interpretation. PIP advised on the wider context and interpretation of the results and reviewed the paper. FH devised the EM27/SUN instrument concept, developed the PROFFAST retrieval code used in this study, and reviewed the paper.
At least one of the (co-)authors is a member of the editorial board of Atmospheric Measurement Techniques. The peer-review process was guided by an independent editor, and the authors also have no other competing interests to declare.
Publisher’s note: Copernicus Publications remains neutral with regard to jurisdictional claims made in the text, published maps, institutional affiliations, or any other geographical representation in this paper. While Copernicus Publications makes every effort to include appropriate place names, the final responsibility lies with the authors.
The authors would like to acknowledge the staff at the National Fisheries Resources Research Institute in Jinja, Uganda, for their support of the measurements that underpin this work. Neil Humpage, Hartmut Boesch, Mark F. Lunt, Liang Feng, and Paul I. Palmer acknowledge the funding provided by the Natural Environment Research Council for this work through The Global Methane Budget (award ref. NE/N015681/1). Neil Humpage, Hartmut Boesch, Liang Feng, and Paul I. Palmer also acknowledge funding from the Natural Environment Research Council through the National Centre for Earth Observation (grant nos. NE/R016518/1 and NE/R000115/1). Jia Chen and Florian Dietrich acknowledge funding by the Deutsche Forschungsgemeinschaft (DGF; German Research Foundation; grant nos. CH 1792/2-1 and INST 95/1544). Frank Hase acknowledges the continuing support by the European Space Agency for development and operation of the COCCON central facility, specifically the COCCON-PROCEEDS projects (contract 4000121212/17/I-EF) and COCCON-OPERA (contract 4000140431/23/I-DT-Ir). This research used the ALICE High Performance Computing Facility at the University of Leicester, along with JASMIN (http://jasmin.ac.uk, last access: 2 July 2024), the UK collaborative data analysis facility. The OCO-2 and OCO-3 data were produced by the OCO-2 project at the Jet Propulsion Laboratory, California Institute of Technology, and obtained from the OCO-2 data archive maintained at the NASA Goddard Earth Sciences Data and Information Services Center.
This research has been supported by the Natural Environment Research Council, the National Centre for Earth Observation (grant nos. NE/N015681/1, NE/R016518/1, and NE/R000115/1), the Deutsche Forschungsgemeinschaft (grant nos. CH 1792/2-1 and INST 95/1544), and the European Space Agency (grant nos. 4000121212/17/I-EF and 4000140431/23/I-DT-Ir).
This paper was edited by Helen Worden and reviewed by Shima Bahramvash Shams and one anonymous referee.
Aigner, P., Makowski, M., Luther, A., Dietrich, F., and Chen, J.: Pyra: Automated EM27/SUN Greenhouse Gas Measurement Software, Journal of Open Source Software, 8, 5131, https://doi.org/10.21105/joss.05131, 2023. a, b
Alberti, C., Hase, F., Frey, M., Dubravica, D., Blumenstock, T., Dehn, A., Castracane, P., Surawicz, G., Harig, R., Baier, B. C., Bès, C., Bi, J., Boesch, H., Butz, A., Cai, Z., Chen, J., Crowell, S. M., Deutscher, N. M., Ene, D., Franklin, J. E., García, O., Griffith, D., Grouiez, B., Grutter, M., Hamdouni, A., Houweling, S., Humpage, N., Jacobs, N., Jeong, S., Joly, L., Jones, N. B., Jouglet, D., Kivi, R., Kleinschek, R., Lopez, M., Medeiros, D. J., Morino, I., Mostafavipak, N., Müller, A., Ohyama, H., Palmer, P. I., Pathakoti, M., Pollard, D. F., Raffalski, U., Ramonet, M., Ramsay, R., Sha, M. K., Shiomi, K., Simpson, W., Stremme, W., Sun, Y., Tanimoto, H., Té, Y., Tsidu, G. M., Velazco, V. A., Vogel, F., Watanabe, M., Wei, C., Wunch, D., Yamasoe, M., Zhang, L., and Orphal, J.: Improved calibration procedures for the EM27/SUN spectrometers of the COllaborative Carbon Column Observing Network (COCCON), Atmos. Meas. Tech., 15, 2433–2463, https://doi.org/10.5194/amt-15-2433-2022, 2022. a, b
Ayompe, L., Davis, S., and Egoh, B.: Trends and drivers of African fossil fuel CO2 emissions 1990–2017, Environ. Res. Lett., 15, 124039, https://doi.org/10.1088/1748-9326/abc64f, 2021. a
Basu, S., Guerlet, S., Butz, A., Houweling, S., Hasekamp, O., Aben, I., Krummel, P., Steele, P., Langenfelds, R., Torn, M., Biraud, S., Stephens, B., Andrews, A., and Worthy, D.: Global CO2 fluxes estimated from GOSAT retrievals of total column CO2, Atmos. Chem. Phys., 13, 8695–8717, https://doi.org/10.5194/acp-13-8695-2013, 2013. a
Bloom, A. A., Palmer, P. I., Fraser, A., Reay, D. S., and Frankenberg, C.: Large-Scale Controls of Methanogenesis Inferred from Methane and Gravity Spaceborne Data, Science, 327, 322–325, https://doi.org/10.1126/science.1175176, 2010. a
Bloom, A. A., Bowman, K. W., Lee, M., Turner, A. J., Schroeder, R., Worden, J. R., Weidner, R., McDonald, K. C., and Jacob, D. J.: A global wetland methane emissions and uncertainty dataset for atmospheric chemical transport models (WetCHARTs version 1.0), Geosci. Model Dev., 10, 2141–2156, https://doi.org/10.5194/gmd-10-2141-2017, 2017. a
Che, K., Cai, Z., Liu, Y., Wu, L., Yang, D., Chen, Y., Meng, X., Zhou, M., Wang, J., Yao, L., and Wang, P.: Lagrangian inversion of anthropogenic CO2 emissions from Beijing using differential column measurements, Environ. Res. Lett., 17, 075001, https://doi.org/10.1088/1748-9326/ac7477, 2022. a
Chen, J., Viatte, C., Hedelius, J. K., Jones, T., Franklin, J. E., Parker, H., Gottlieb, E. W., Wennberg, P. O., Dubey, M. K., and Wofsy, S. C.: Differential column measurements using compact solar-tracking spectrometers, Atmos. Chem. Phys., 16, 8479–8498, https://doi.org/10.5194/acp-16-8479-2016, 2016. a
Chen, Z., Jacob, D. J., Nesser, H., Sulprizio, M. P., Lorente, A., Varon, D. J., Lu, X., Shen, L., Qu, Z., Penn, E., and Yu, X.: Methane emissions from China: a high-resolution inversion of TROPOMI satellite observations, Atmos. Chem. Phys., 22, 10809–10826, https://doi.org/10.5194/acp-22-10809-2022, 2022. a
Chevallier, F., Remaud, M., O'Dell, C. W., Baker, D., Peylin, P., and Cozic, A.: Objective evaluation of surface- and satellite-driven carbon dioxide atmospheric inversions, Atmos. Chem. Phys., 19, 14233–14251, https://doi.org/10.5194/acp-19-14233-2019, 2019. a
Copernicus Sentinel-5P (processed by ESA): TROPOMI Level 2 Methane Total Column products, Version 02, European Space Agency [data set], https://doi.org/10.5270/S5P-3lcdqiv, 2021a. a
Copernicus Sentinel-5P (processed by ESA): TROPOMI Level 2 Carbon Monoxide total column products, Version 02, European Space Agency [data set], https://doi.org/10.5270/S5P-bj3nry0, 2021b. a
Crowell, S., Baker, D., Schuh, A., Basu, S., Jacobson, A. R., Chevallier, F., Liu, J., Deng, F., Feng, L., McKain, K., Chatterjee, A., Miller, J. B., Stephens, B. B., Eldering, A., Crisp, D., Schimel, D., Nassar, R., O'Dell, C. W., Oda, T., Sweeney, C., Palmer, P. I., and Jones, D. B. A.: The 2015–2016 carbon cycle as seen from OCO-2 and the global in situ network, Atmos. Chem. Phys., 19, 9797–9831, https://doi.org/10.5194/acp-19-9797-2019, 2019. a
Crowther, T. W., Glick, H. B., Covey, K. R., Bettigole, C., Maynard, D. S., Thomas, S. M., Smith, J. R., Hintler, G., Duguid, M. C., Amatulli, G., Tuanmu, M.-N., Jetz, W., Salas, C., Stam, C., Piotto, D., Tavani, R., Green, S., Bruce, G., Williams, S. J., Wiser, S. K., Huber, M. O., Hengeveld, G. M., Nabuurs, G.-J., Tikhonova, E., Borchardt, P., Li, C.-F., Powrie, L. W., Fischer, M., Hemp, A., Homeier, J., Cho, P., Vibrans, A. C., Umunay, P. M., Piao, S. L., Rowe, C. W., Ashton, M. S., Crane, P. R., and Bradford, M. A.: Mapping tree density at a global scale, Nature, 525, 201–205, https://doi.org/10.1038/nature14967, 2015. a
Deeter, M. N., Martínez-Alonso, S., Edwards, D. P., Emmons, L. K., Gille, J. C., Worden, H. M., Sweeney, C., Pittman, J. V., Daube, B. C., and Wofsy, S. C.: The MOPITT Version 6 product: algorithm enhancements and validation, Atmos. Meas. Tech., 7, 3623–3632, https://doi.org/10.5194/amt-7-3623-2014, 2014. a
Deng, F., Jones, D. B. A., Henze, D. K., Bousserez, N., Bowman, K. W., Fisher, J. B., Nassar, R., O'Dell, C., Wunch, D., Wennberg, P. O., Kort, E. A., Wofsy, S. C., Blumenstock, T., Deutscher, N. M., Griffith, D. W. T., Hase, F., Heikkinen, P., Sherlock, V., Strong, K., Sussmann, R., and Warneke, T.: Inferring regional sources and sinks of atmospheric CO2 from GOSAT XCO2 data, Atmos. Chem. Phys., 14, 3703–3727, https://doi.org/10.5194/acp-14-3703-2014, 2014. a
Dietrich, F., Chen, J., Voggenreiter, B., Aigner, P., Nachtigall, N., and Reger, B.: MUCCnet: Munich Urban Carbon Column network, Atmos. Meas. Tech., 14, 1111–1126, https://doi.org/10.5194/amt-14-1111-2021, 2021. a, b, c, d, e
Dils, B., Buchwitz, M., Reuter, M., Schneising, O., Boesch, H., Parker, R., Guerlet, S., Aben, I., Blumenstock, T., Burrows, J. P., Butz, A., Deutscher, N. M., Frankenberg, C., Hase, F., Hasekamp, O. P., Heymann, J., De Mazière, M., Notholt, J., Sussmann, R., Warneke, T., Griffith, D., Sherlock, V., and Wunch, D.: The Greenhouse Gas Climate Change Initiative (GHG-CCI): comparative validation of GHG-CCI SCIAMACHY/ENVISAT and TANSO-FTS/GOSAT CO2 and CH4 retrieval algorithm products with measurements from the TCCON, Atmos. Meas. Tech., 7, 1723–1744, https://doi.org/10.5194/amt-7-1723-2014, 2014. a
Drinkwater, A., Palmer, P. I., Feng, L., Arnold, T., Lan, X., Michel, S. E., Parker, R., and Boesch, H.: Atmospheric data support a multi-decadal shift in the global methane budget towards natural tropical emissions, Atmos. Chem. Phys., 23, 8429–8452, https://doi.org/10.5194/acp-23-8429-2023, 2023. a
Eldering, A., O'Dell, C. W., Wennberg, P. O., Crisp, D., Gunson, M. R., Viatte, C., Avis, C., Braverman, A., Castano, R., Chang, A., Chapsky, L., Cheng, C., Connor, B., Dang, L., Doran, G., Fisher, B., Frankenberg, C., Fu, D., Granat, R., Hobbs, J., Lee, R. A. M., Mandrake, L., McDuffie, J., Miller, C. E., Myers, V., Natraj, V., O'Brien, D., Osterman, G. B., Oyafuso, F., Payne, V. H., Pollock, H. R., Polonsky, I., Roehl, C. M., Rosenberg, R., Schwandner, F., Smyth, M., Tang, V., Taylor, T. E., To, C., Wunch, D., and Yoshimizu, J.: The Orbiting Carbon Observatory-2: first 18 months of science data products, Atmos. Meas. Tech., 10, 549–563, https://doi.org/10.5194/amt-10-549-2017, 2017. a, b
Eldering, A., Taylor, T. E., O'Dell, C. W., and Pavlick, R.: The OCO-3 mission: measurement objectives and expected performance based on 1 year of simulated data, Atmos. Meas. Tech., 12, 2341–2370, https://doi.org/10.5194/amt-12-2341-2019, 2019. a, b
Feld, L., Herkommer, B., Vestner, J., Dubravica, D., Alberti, C., and Hase F.: PROFFASTpylot: Running PROFFAST with Python, J. Open Source Soft., 9, 6481, https://doi.org/10.21105/joss.06481, 2024. a
Feng, L. and Palmer, P.: CO2 column concentrations from GEOS-Chem, covering East Africa from January to April 2020, NERC EDS Centre for Environmental Data Analysis, 11 September 2024, CEDA Archive [data set], https://doi.org/10.5285/925816bd869644ad9fe9b877d8f42d30, 2024. a
Feng, L., Palmer, P. I., Bösch, H., and Dance, S.: Estimating surface CO2 fluxes from space-borne CO2 dry air mole fraction observations using an ensemble Kalman Filter, Atmos. Chem. Phys., 9, 2619–2633, https://doi.org/10.5194/acp-9-2619-2009, 2009. a
Feng, L., Palmer, P. I., Bösch, H., Parker, R. J., Webb, A. J., Correia, C. S. C., Deutscher, N. M., Domingues, L. G., Feist, D. G., Gatti, L. V., Gloor, E., Hase, F., Kivi, R., Liu, Y., Miller, J. B., Morino, I., Sussmann, R., Strong, K., Uchino, O., Wang, J., and Zahn, A.: Consistent regional fluxes of CH4 and CO2 inferred from GOSAT proxy XCH4 : XCO2 retrievals, 2010–2014, Atmos. Chem. Phys., 17, 4781–4797, https://doi.org/10.5194/acp-17-4781-2017, 2017. a, b, c
Feng, L., Palmer, P. I., Zhu, S., Parker, R. J., and Liu, Y.: Tropical methane emissions explain large fraction of recent changes in global atmospheric methane growth rate, Nat. Commun., 13, 1378, https://doi.org/10.1038/s41467-022-28989-z, 2022. a, b
Feng, L., Palmer, P. I., Parker, R. J., Lunt, M. F., and Bösch, H.: Methane emissions are predominantly responsible for record-breaking atmospheric methane growth rates in 2020 and 2021, Atmos. Chem. Phys., 23, 4863–4880, https://doi.org/10.5194/acp-23-4863-2023, 2023. a
Fleming, Z. L., Monks, P. S., and Manning, A. J.: Review: Untangling the influence of air-mass history in interpreting observed atmospheric composition, Atmos. Res., 104–105, 1–39, https://doi.org/10.1016/j.atmosres.2011.09.009, 2012. a
Flemming, J., Benedetti, A., Inness, A., Engelen, R. J., Jones, L., Huijnen, V., Remy, S., Parrington, M., Suttie, M., Bozzo, A., Peuch, V.-H., Akritidis, D., and Katragkou, E.: The CAMS interim Reanalysis of Carbon Monoxide, Ozone and Aerosol for 2003–2015, Atmos. Chem. Phys., 17, 1945–1983, https://doi.org/10.5194/acp-17-1945-2017, 2017. a
Frey, M., Hase, F., Blumenstock, T., Groß, J., Kiel, M., Mengistu Tsidu, G., Schäfer, K., Sha, M. K., and Orphal, J.: Calibration and instrumental line shape characterization of a set of portable FTIR spectrometers for detecting greenhouse gas emissions, Atmos. Meas. Tech., 8, 3047–3057, https://doi.org/10.5194/amt-8-3047-2015, 2015. a
Frey, M., Sha, M. K., Hase, F., Kiel, M., Blumenstock, T., Harig, R., Surawicz, G., Deutscher, N. M., Shiomi, K., Franklin, J. E., Bösch, H., Chen, J., Grutter, M., Ohyama, H., Sun, Y., Butz, A., Mengistu Tsidu, G., Ene, D., Wunch, D., Cao, Z., Garcia, O., Ramonet, M., Vogel, F., and Orphal, J.: Building the COllaborative Carbon Column Observing Network (COCCON): long-term stability and ensemble performance of the EM27/SUN Fourier transform spectrometer, Atmos. Meas. Tech., 12, 1513–1530, https://doi.org/10.5194/amt-12-1513-2019, 2019. a, b
Frey, M. M., Hase, F., Blumenstock, T., Dubravica, D., Groß, J., Göttsche, F., Handjaba, M., Amadhila, P., Mushi, R., Morino, I., Shiomi, K., Sha, M. K., de Mazière, M., and Pollard, D. F.: Long-term column-averaged greenhouse gas observations using a COCCON spectrometer at the high-surface-albedo site in Gobabeb, Namibia, Atmos. Meas. Tech., 14, 5887–5911, https://doi.org/10.5194/amt-14-5887-2021, 2021. a, b, c, d
Friedlingstein, P., Jones, M. W., O'Sullivan, M., Andrew, R. M., Bakker, D. C. E., Hauck, J., Le Quéré, C., Peters, G. P., Peters, W., Pongratz, J., Sitch, S., Canadell, J. G., Ciais, P., Jackson, R. B., Alin, S. R., Anthoni, P., Bates, N. R., Becker, M., Bellouin, N., Bopp, L., Chau, T. T. T., Chevallier, F., Chini, L. P., Cronin, M., Currie, K. I., Decharme, B., Djeutchouang, L. M., Dou, X., Evans, W., Feely, R. A., Feng, L., Gasser, T., Gilfillan, D., Gkritzalis, T., Grassi, G., Gregor, L., Gruber, N., Gürses, Ö., Harris, I., Houghton, R. A., Hurtt, G. C., Iida, Y., Ilyina, T., Luijkx, I. T., Jain, A., Jones, S. D., Kato, E., Kennedy, D., Klein Goldewijk, K., Knauer, J., Korsbakken, J. I., Körtzinger, A., Landschützer, P., Lauvset, S. K., Lefèvre, N., Lienert, S., Liu, J., Marland, G., McGuire, P. C., Melton, J. R., Munro, D. R., Nabel, J. E. M. S., Nakaoka, S.-I., Niwa, Y., Ono, T., Pierrot, D., Poulter, B., Rehder, G., Resplandy, L., Robertson, E., Rödenbeck, C., Rosan, T. M., Schwinger, J., Schwingshackl, C., Séférian, R., Sutton, A. J., Sweeney, C., Tanhua, T., Tans, P. P., Tian, H., Tilbrook, B., Tubiello, F., van der Werf, G. R., Vuichard, N., Wada, C., Wanninkhof, R., Watson, A. J., Willis, D., Wiltshire, A. J., Yuan, W., Yue, C., Yue, X., Zaehle, S., and Zeng, J.: Global Carbon Budget 2021, Earth Syst. Sci. Data, 14, 1917–2005, https://doi.org/10.5194/essd-14-1917-2022, 2022. a
Gisi, M., Hase, F., Dohe, S., Blumenstock, T., Simon, A., and Keens, A.: XCO2-measurements with a tabletop FTS using solar absorption spectroscopy, Atmos. Meas. Tech., 5, 2969–2980, https://doi.org/10.5194/amt-5-2969-2012, 2012. a
Hase, F., Frey, M., Blumenstock, T., Groß, J., Kiel, M., Kohlhepp, R., Mengistu Tsidu, G., Schäfer, K., Sha, M. K., and Orphal, J.: Application of portable FTIR spectrometers for detecting greenhouse gas emissions of the major city Berlin, Atmos. Meas. Tech., 8, 3059–3068, https://doi.org/10.5194/amt-8-3059-2015, 2015. a
Hase, F., Frey, M., Kiel, M., Blumenstock, T., Harig, R., Keens, A., and Orphal, J.: Addition of a channel for XCO observations to a portable FTIR spectrometer for greenhouse gas measurements, Atmos. Meas. Tech., 9, 2303–2313, https://doi.org/10.5194/amt-9-2303-2016, 2016. a
Hasekamp, O., Lorente, A., Hu, H., Butz, A., aan de Brugh, J., and Landgraf, J.: Algorithm Theoretical Baseline Document for Sentinel-5 Precursor Methane Retrieval, Tech. Rep. SRON-S5P-LEV2-RP-001, SRON Netherlands Institute for Space Research, https://sentiwiki.copernicus.eu/web/document-library (last access: 3 July 2024), 2021. a, b
Hedelius, J. K., Viatte, C., Wunch, D., Roehl, C. M., Toon, G. C., Chen, J., Jones, T., Wofsy, S. C., Franklin, J. E., Parker, H., Dubey, M. K., and Wennberg, P. O.: Assessment of errors and biases in retrievals of X, X, XCO, and X from a 0.5 cm−1 resolution solar-viewing spectrometer, Atmos. Meas. Tech., 9, 3527–3546, https://doi.org/10.5194/amt-9-3527-2016, 2016. a
Heinle, L. and Chen, J.: Automated enclosure and protection system for compact solar-tracking spectrometers, Atmos. Meas. Tech., 11, 2173–2185, https://doi.org/10.5194/amt-11-2173-2018, 2018. a, b
Herrmann, S. M. and Mohr, K. I.: A Continental-Scale Classification of Rainfall Seasonality Regimes in Africa Based on Gridded Precipitation and Land Surface Temperature Products, J. Appl. Meteorol. Clim., 50, 2504–2513, https://doi.org/10.1175/JAMC-D-11-024.1, 2011. a
Hersbach, H., Bell, B., Berrisford, P., Biavati, G., Horányi, A., Muñoz Sabater, J., Nicolas, J., Peubey, C., Radu, R., Rozum, I., Schepers, D., Simmons, A., Soci, C., Dee, D., and Thépaut, J.-N.: ERA5 hourly data on pressure levels from 1940 to present, Copernicus Climate Change Service (C3S) Climate Data Store (CDS), https://doi.org/10.24381/cds.bd0915c6, 2023. a, b
Hollingsworth, A., Engelen, R. J., Textor, C., Benedetti, A., Boucher, O., Chevallier, F., Dethof, A., Elbern, H., Eskes, H., Flemming, J., Granier, C., Kaiser, J. W., Morcrette, J.-J., Rayner, P., Peuch, V.-H., Rouil, L., Schultz, M. G., and Simmons, A. J.: TOWARD A MONITORING AND FORECASTING SYSTEM FOR ATMOSPHERIC COMPOSITION: The GEMS Project, B. Am. Meteorol. Soc., 89, 1147–1164, https://doi.org/10.1175/2008BAMS2355.1, 2008. a
Hu, H., Hasekamp, O., Butz, A., Galli, A., Landgraf, J., Aan de Brugh, J., Borsdorff, T., Scheepmaker, R., and Aben, I.: The operational methane retrieval algorithm for TROPOMI, Atmos. Meas. Tech., 9, 5423–5440, https://doi.org/10.5194/amt-9-5423-2016, 2016. a
Humpage, N., Okello, W., and Boesch, H.: Ground-based greenhouse gas column concentrations from Jinja, Uganda, January to April 2020, NERC EDS Centre for Environmental Data Analysis, 11 September 2024, CEDA Archive [data set], https://doi.org/10.5285/7a8d0936ba1e4e1a8689c9e9010b43b2, 2024. a
Hunt, B. R., Kostelich, E. J., and Szunyogh, I.: Efficient data assimilation for spatiotemporal chaos: A local ensemble transform Kalman filter, Physica D, 230, 112–126, https://doi.org/10.1016/j.physd.2006.11.008, 2007. a
Inness, A., Ades, M., Agustí-Panareda, A., Barré, J., Benedictow, A., Blechschmidt, A.-M., Dominguez, J. J., Engelen, R., Eskes, H., Flemming, J., Huijnen, V., Jones, L., Kipling, Z., Massart, S., Parrington, M., Peuch, V.-H., Razinger, M., Remy, S., Schulz, M., and Suttie, M.: The CAMS reanalysis of atmospheric composition, Atmos. Chem. Phys., 19, 3515–3556, https://doi.org/10.5194/acp-19-3515-2019, 2019a. a
Inness, A., Ades, M., Agustí-Panareda, A., Barré, J., Benedictow, A., Blechschmidt, A., Dominguez, J., Engelen, R., Eskes, H., Flemming, J., Huijnen, V., Jones, L., Kipling, Z., Massart, S., Parrington, M., Peuch, V.-H., Razinger, M., Remy, S., Schulz, M., and Suttie, M.: CAMS global reanalysis (EAC4), Copernicus Atmosphere Monitoring Service (CAMS) Atmosphere Data Store (ADS), https://ads.atmosphere.copernicus.eu/cdsapp#!/dataset/cams-global-reanalysis-eac4?tab=overview (last access: 3 July 2024), 2019b. a
Inness, A., Aben, I., Ades, M., Borsdorff, T., Flemming, J., Jones, L., Landgraf, J., Langerock, B., Nedelec, P., Parrington, M., and Ribas, R.: Assimilation of S5P/TROPOMI carbon monoxide data with the global CAMS near-real-time system, Atmos. Chem. Phys., 22, 14355–14376, https://doi.org/10.5194/acp-22-14355-2022, 2022. a, b
Inoue, M., Morino, I., Uchino, O., Nakatsuru, T., Yoshida, Y., Yokota, T., Wunch, D., Wennberg, P. O., Roehl, C. M., Griffith, D. W. T., Velazco, V. A., Deutscher, N. M., Warneke, T., Notholt, J., Robinson, J., Sherlock, V., Hase, F., Blumenstock, T., Rettinger, M., Sussmann, R., Kyrö, E., Kivi, R., Shiomi, K., Kawakami, S., De Mazière, M., Arnold, S. G., Feist, D. G., Barrow, E. A., Barney, J., Dubey, M., Schneider, M., Iraci, L. T., Podolske, J. R., Hillyard, P. W., Machida, T., Sawa, Y., Tsuboi, K., Matsueda, H., Sweeney, C., Tans, P. P., Andrews, A. E., Biraud, S. C., Fukuyama, Y., Pittman, J. V., Kort, E. A., and Tanaka, T.: Bias corrections of GOSAT SWIR XCO2 and XCH4 with TCCON data and their evaluation using aircraft measurement data, Atmos. Meas. Tech., 9, 3491–3512, https://doi.org/10.5194/amt-9-3491-2016, 2016. a
IPCC: Climate Change 2021: The Physical Science Basis. Contribution of Working Group I to the Sixth Assessment Report of the Intergovernmental Panel on Climate Change, vol. In Press, Cambridge University Press, Cambridge, United Kingdom and New York, NY, USA, https://doi.org/10.1017/9781009157896, 2021. a
Jacobs, N., Simpson, W. R., Wunch, D., O'Dell, C. W., Osterman, G. B., Hase, F., Blumenstock, T., Tu, Q., Frey, M., Dubey, M. K., Parker, H. A., Kivi, R., and Heikkinen, P.: Quality controls, bias, and seasonality of CO2 columns in the boreal forest with Orbiting Carbon Observatory-2, Total Carbon Column Observing Network, and EM27/SUN measurements, Atmos. Meas. Tech., 13, 5033–5063, https://doi.org/10.5194/amt-13-5033-2020, 2020. a
Janssens-Maenhout, G., Crippa, M., Guizzardi, D., Muntean, M., Schaaf, E., Dentener, F., Bergamaschi, P., Pagliari, V., Olivier, J. G. J., Peters, J. A. H. W., van Aardenne, J. A., Monni, S., Doering, U., Petrescu, A. M. R., Solazzo, E., and Oreggioni, G. D.: EDGAR v4.3.2 Global Atlas of the three major greenhouse gas emissions for the period 1970–2012, Earth Syst. Sci. Data, 11, 959–1002, https://doi.org/10.5194/essd-11-959-2019, 2019. a
Jones, T. S., Franklin, J. E., Chen, J., Dietrich, F., Hajny, K. D., Paetzold, J. C., Wenzel, A., Gately, C., Gottlieb, E., Parker, H., Dubey, M., Hase, F., Shepson, P. B., Mielke, L. H., and Wofsy, S. C.: Assessing urban methane emissions using column-observing portable Fourier transform infrared (FTIR) spectrometers and a novel Bayesian inversion framework, Atmos. Chem. Phys., 21, 13131–13147, https://doi.org/10.5194/acp-21-13131-2021, 2021. a
Kaiser, J. W., Heil, A., Andreae, M. O., Benedetti, A., Chubarova, N., Jones, L., Morcrette, J.-J., Razinger, M., Schultz, M. G., Suttie, M., and van der Werf, G. R.: Biomass burning emissions estimated with a global fire assimilation system based on observed fire radiative power, Biogeosciences, 9, 527–554, https://doi.org/10.5194/bg-9-527-2012, 2012. a
Kalnay, E., Kanamitsu, M., Kistler, R., Collins, W., Deaven, D., Gandin, L., Iredell, M., Saha, S., White, G., Woollen, J., Zhu, Y., Chelliah, M., Ebisuzaki, W., Higgins, W., Janowiak, J., Mo, K. C., Ropelewski, C., Wang, J., Leetmaa, A., Reynolds, R., Jenne, R., and Joseph, D.: The NCEP/NCAR 40-Year Reanalysis Project, B. Am. Meteorol. Soc., 77, 437–472, https://doi.org/10.1175/1520-0477(1996)077<0437:TNYRP>2.0.CO;2, 1996. a
Kayranli, B., Scholz, M., Mustafa, A., and Hedmark, r.: Carbon Storage and Fluxes within Freshwater Wetlands: a Critical Review, Wetlands, 30, 111–124, https://doi.org/10.1007/s13157-009-0003-4, 2010. a
Klappenbach, F., Bertleff, M., Kostinek, J., Hase, F., Blumenstock, T., Agusti-Panareda, A., Razinger, M., and Butz, A.: Accurate mobile remote sensing of XCO2 and XCH4 latitudinal transects from aboard a research vessel, Atmos. Meas. Tech., 8, 5023–5038, https://doi.org/10.5194/amt-8-5023-2015, 2015. a
Knapp, M., Kleinschek, R., Hase, F., Agustí-Panareda, A., Inness, A., Barré, J., Landgraf, J., Borsdorff, T., Kinne, S., and Butz, A.: Shipborne measurements of XCO2, XCH4, and XCO above the Pacific Ocean and comparison to CAMS atmospheric analyses and S5P/TROPOMI, Earth Syst. Sci. Data, 13, 199–211, https://doi.org/10.5194/essd-13-199-2021, 2021. a
Kuze, A., Suto, H., Nakajima, M., and Hamazaki, T.: Thermal and near infrared sensor for carbon observation Fourier-transform spectrometer on the Greenhouse Gases Observing Satellite for greenhouse gases monitoring, Appl. Optics, 48, 6716–6733, https://doi.org/10.1364/AO.48.006716, 2009. a
Landgraf, J., aan de Brugh, J., Scheepmaker, R., Borsdorff, T., Hu, H., Houweling, S., Butz, A., Aben, I., and Hasekamp, O.: Carbon monoxide total column retrievals from TROPOMI shortwave infrared measurements, Atmos. Meas. Tech., 9, 4955–4975, https://doi.org/10.5194/amt-9-4955-2016, 2016. a, b, c
Lorente, A., Borsdorff, T., Butz, A., Hasekamp, O., aan de Brugh, J., Schneider, A., Wu, L., Hase, F., Kivi, R., Wunch, D., Pollard, D. F., Shiomi, K., Deutscher, N. M., Velazco, V. A., Roehl, C. M., Wennberg, P. O., Warneke, T., and Landgraf, J.: Methane retrieved from TROPOMI: improvement of the data product and validation of the first 2 years of measurements, Atmos. Meas. Tech., 14, 665–684, https://doi.org/10.5194/amt-14-665-2021, 2021. a
Lunt, M. and Palmer, P.: CH4 column concentrations calculated from a high-res GEOS-Chem model run for Uganda, January to April 2020, NERC EDS Centre for Environmental Data Analysis, 11 September 2024, CEDA Archive [data set], https://doi.org/10.5285/7ecc607cb09747a59da6f46a0635f469, 2024. a
Lunt, M. F., Palmer, P. I., Feng, L., Taylor, C. M., Boesch, H., and Parker, R. J.: An increase in methane emissions from tropical Africa between 2010 and 2016 inferred from satellite data, Atmos. Chem. Phys., 19, 14721–14740, https://doi.org/10.5194/acp-19-14721-2019, 2019. a, b, c, d, e, f
Lunt, M. F., Palmer, P. I., Lorente, A., Borsdorff, T., Landgraf, J., Parker, R. J., and Bösch, H.: Rain-fed pulses of methane from East Africa during 2018–2019 contributed to atmospheric growth rate, Environ. Res. Lett., 16, 024021, https://doi.org/10.1088/1748-9326/abd8fa, 2021. a, b, c, d, e, f, g, h
López-Ballesteros, A., Beck, J., Bombelli, A., Grieco, E., Lorencová, E. K., Merbold, L., Brümmer, C., Hugo, W., Scholes, R., Vačkář, D., Vermeulen, A., Acosta, M., Butterbach-Bahl, K., Helmschrot, J., Kim, D.-G., Jones, M., Jorch, V., Pavelka, M., Skjelvan, I., and Saunders, M.: Towards a feasible and representative pan-African research infrastructure network for GHG observations, Environ. Res. Lett., 13, 085003, https://doi.org/10.1088/1748-9326/aad66c, 2018. a
Makarova, M. V., Alberti, C., Ionov, D. V., Hase, F., Foka, S. C., Blumenstock, T., Warneke, T., Virolainen, Y. A., Kostsov, V. S., Frey, M., Poberovskii, A. V., Timofeyev, Y. M., Paramonova, N. N., Volkova, K. A., Zaitsev, N. A., Biryukov, E. Y., Osipov, S. I., Makarov, B. K., Polyakov, A. V., Ivakhov, V. M., Imhasin, H. Kh., and Mikhailov, E. F.: Emission Monitoring Mobile Experiment (EMME): an overview and first results of the St. Petersburg megacity campaign 2019, Atmos. Meas. Tech., 14, 1047–1073, https://doi.org/10.5194/amt-14-1047-2021, 2021. a
Mitsch, W. J., Bernal, B., Nahlik, A. M., Mander, U., Zhang, L., Anderson, C. J., Jørgensen, S. E., and Brix, H.: Wetlands, carbon, and climate change, Landscape Ecol., 28, 583–597, https://doi.org/10.1007/s10980-012-9758-8, 2013. a
Nickless, A., Scholes, R. J., Vermeulen, A., Beck, J., López-Ballesteros, A., Ardö, J., Karstens, U., Rigby, M., Kasurinen, V., Pantazatou, K., Jorch, V., and Kutsch, W.: Greenhouse gas observation network design for Africa, Tellus B, 72, 1–30, https://doi.org/10.1080/16000889.2020.1824486, 2020. a
OCO-2 Science Team (Gunson, M. and Eldering, A.): OCO-2 Level 2 bias-corrected XCO2 and other select fields from the full-physics retrieval aggregated as daily files, Retrospective processing V10r, Greenbelt, MD, USA, Goddard Earth Sciences Data and Information Services Center (GES DISC), Earth Data [data set], https://doi.org/10.5067/E4E140XDMPO2, 2020. a
OCO-2/OCO-3 Science Team (Chatterjee, A. and Payne, V.): OCO-3 Level 2 bias-corrected XCO2 and other select fields from the full-physics retrieval aggregated as daily files, Retrospective processing v10.4r, Greenbelt, MD, USA, Goddard Earth Sciences Data and Information Services Center (GES DISC), Earth Data [data set], https://doi.org/10.5067/970BCC4DHH24, 2022. a
Oda, T., Maksyutov, S., and Andres, R. J.: The Open-source Data Inventory for Anthropogenic CO2, version 2016 (ODIAC2016): a global monthly fossil fuel CO2 gridded emissions data product for tracer transport simulations and surface flux inversions, Earth Syst. Sci. Data, 10, 87–107, https://doi.org/10.5194/essd-10-87-2018, 2018. a
O'Dell, C. W., Connor, B., Bösch, H., O'Brien, D., Frankenberg, C., Castano, R., Christi, M., Eldering, D., Fisher, B., Gunson, M., McDuffie, J., Miller, C. E., Natraj, V., Oyafuso, F., Polonsky, I., Smyth, M., Taylor, T., Toon, G. C., Wennberg, P. O., and Wunch, D.: The ACOS CO2 retrieval algorithm – Part 1: Description and validation against synthetic observations, Atmos. Meas. Tech., 5, 99–121, https://doi.org/10.5194/amt-5-99-2012, 2012. a
O'Dell, C. W., Eldering, A., Wennberg, P. O., Crisp, D., Gunson, M. R., Fisher, B., Frankenberg, C., Kiel, M., Lindqvist, H., Mandrake, L., Merrelli, A., Natraj, V., Nelson, R. R., Osterman, G. B., Payne, V. H., Taylor, T. E., Wunch, D., Drouin, B. J., Oyafuso, F., Chang, A., McDuffie, J., Smyth, M., Baker, D. F., Basu, S., Chevallier, F., Crowell, S. M. R., Feng, L., Palmer, P. I., Dubey, M., García, O. E., Griffith, D. W. T., Hase, F., Iraci, L. T., Kivi, R., Morino, I., Notholt, J., Ohyama, H., Petri, C., Roehl, C. M., Sha, M. K., Strong, K., Sussmann, R., Te, Y., Uchino, O., and Velazco, V. A.: Improved retrievals of carbon dioxide from Orbiting Carbon Observatory-2 with the version 8 ACOS algorithm, Atmos. Meas. Tech., 11, 6539–6576, https://doi.org/10.5194/amt-11-6539-2018, 2018. a
Olsen, S. C. and Randerson, J. T.: Differences between surface and column atmospheric CO2 and implications for carbon cycle research, J. Geophys. Res.-Atmos., 109, D02301, https://doi.org/10.1029/2003JD003968, 2004. a
Palmer, P. I., Feng, L., Baker, D., Chevallier, F., Bösch, H., and Somkuti, P.: Net carbon emissions from African biosphere dominate pan-tropical atmospheric CO2 signal, Nat. Commun., 10, 3344, https://doi.org/10.1038/s41467-019-11097-w, 2019. a, b, c, d
Palmer, P. I., Wainwright, C. M., Dong, B., Maidment, R. I., Wheeler, K. G., Gedney, N., Hickman, J. E., Madani, N., Folwell, S. S., Abdo, G., Allan, R. P., Black, E. C. L., Feng, L., Gudoshava, M., Haines, K., Huntingford, C., Kilavi, M., Lunt, M. F., Shaaban, A., and Turner, A. G.: Drivers and impacts of Eastern African rainfall variability, Nature Reviews Earth & Environment, 4, 254–270, https://doi.org/10.1038/s43017-023-00397-x, 2023. a
Pan, Y., Birdsey, R. A., Fang, J., Houghton, R., Kauppi, P. E., Kurz, W. A., Phillips, O. L., Shvidenko, A., Lewis, S. L., Canadell, J. G., Ciais, P., Jackson, R. B., Pacala, S. W., McGuire, A. D., Piao, S., Rautiainen, A., Sitch, S., and Hayes, D.: A Large and Persistent Carbon Sink in the World's Forests, Science, 333, 988–993, https://doi.org/10.1126/science.1201609, 2011. a
Panagi, M., Fleming, Z. L., Monks, P. S., Ashfold, M. J., Wild, O., Hollaway, M., Zhang, Q., Squires, F. A., and Vande Hey, J. D.: Investigating the regional contributions to air pollution in Beijing: a dispersion modelling study using CO as a tracer, Atmos. Chem. Phys., 20, 2825–2838, https://doi.org/10.5194/acp-20-2825-2020, 2020. a
Pandey, S., Houweling, S., Lorente, A., Borsdorff, T., Tsivlidou, M., Bloom, A. A., Poulter, B., Zhang, Z., and Aben, I.: Using satellite data to identify the methane emission controls of South Sudan's wetlands, Biogeosciences, 18, 557–572, https://doi.org/10.5194/bg-18-557-2021, 2021. a
Peiro, H., Crowell, S., Schuh, A., Baker, D. F., O'Dell, C., Jacobson, A. R., Chevallier, F., Liu, J., Eldering, A., Crisp, D., Deng, F., Weir, B., Basu, S., Johnson, M. S., Philip, S., and Baker, I.: Four years of global carbon cycle observed from the Orbiting Carbon Observatory 2 (OCO-2) version 9 and in situ data and comparison to OCO-2 version 7, Atmos. Chem. Phys., 22, 1097–1130, https://doi.org/10.5194/acp-22-1097-20222, 2022. a
Qu, Z., Jacob, D. J., Zhang, Y., Shen, L., Varon, D. J., Lu, X., Scarpelli, T., Bloom, A., Worden, J., and Parker, R. J.: Attribution of the 2020 surge in atmospheric methane by inverse analysis of GOSAT observations, Environ. Res. Lett., 17, 094003, https://doi.org/10.1088/1748-9326/ac8754, 2022. a
Rabier, F., Järvinen, H., Klinker, E., Mahfouf, J.-F., and Simmons, A.: The ECMWF operational implementation of four-dimensional variational assimilation. I: Experimental results with simplified physics, Q. J. Roy. Meteor. Soc., 126, 1143–1170, https://doi.org/10.1002/qj.49712656415, 2000. a
Rißmann, M., Chen, J., Osterman, G., Zhao, X., Dietrich, F., Makowski, M., Hase, F., and Kiel, M.: Comparison of OCO-2 target observations to MUCCnet – is it possible to capture urban gradients from space?, Atmos. Meas. Tech., 15, 6605–6623, https://doi.org/10.5194/amt-15-6605-2022, 2022. a
Rodgers, C. D.: Inverse Methods for Atmospheric Sounding: Theory and Practice, World Scientific Publishing, https://doi.org/10.1142/3171, 2000. a
Saji, N., Goswami, B., Vinayachandran, P., and Yamagata, T.: A dipole mode in the tropical Indian Ocean, Nature, 401, 360–363, https://doi.org/10.1038/43854, 1999. a
Sha, M. K., De Mazière, M., Notholt, J., Blumenstock, T., Chen, H., Dehn, A., Griffith, D. W. T., Hase, F., Heikkinen, P., Hermans, C., Hoffmann, A., Huebner, M., Jones, N., Kivi, R., Langerock, B., Petri, C., Scolas, F., Tu, Q., and Weidmann, D.: Intercomparison of low- and high-resolution infrared spectrometers for ground-based solar remote sensing measurements of total column concentrations of CO2, CH4, and CO, Atmos. Meas. Tech., 13, 4791–4839, https://doi.org/10.5194/amt-13-4791-2020, 2020. a
Sha, M. K., Langerock, B., Blavier, J.-F. L., Blumenstock, T., Borsdorff, T., Buschmann, M., Dehn, A., De Mazière, M., Deutscher, N. M., Feist, D. G., García, O. E., Griffith, D. W. T., Grutter, M., Hannigan, J. W., Hase, F., Heikkinen, P., Hermans, C., Iraci, L. T., Jeseck, P., Jones, N., Kivi, R., Kumps, N., Landgraf, J., Lorente, A., Mahieu, E., Makarova, M. V., Mellqvist, J., Metzger, J.-M., Morino, I., Nagahama, T., Notholt, J., Ohyama, H., Ortega, I., Palm, M., Petri, C., Pollard, D. F., Rettinger, M., Robinson, J., Roche, S., Roehl, C. M., Röhling, A. N., Rousogenous, C., Schneider, M., Shiomi, K., Smale, D., Stremme, W., Strong, K., Sussmann, R., Té, Y., Uchino, O., Velazco, V. A., Vigouroux, C., Vrekoussis, M., Wang, P., Warneke, T., Wizenberg, T., Wunch, D., Yamanouchi, S., Yang, Y., and Zhou, M.: Validation of methane and carbon monoxide from Sentinel-5 Precursor using TCCON and NDACC-IRWG stations, Atmos. Meas. Tech., 14, 6249–6304, https://doi.org/10.5194/amt-14-6249-2021, 2021. a, b, c, d
Takahashi, T., Sutherland, S. C., Wanninkhof, R., Sweeney, C., Feely, R. A., Chipman, D. W., Hales, B., Friederich, G., Chavez, F., Sabine, C., Watson, A., Bakker, D. C., Schuster, U., Metzl, N., Yoshikawa-Inoue, H., Ishii, M., Midorikawa, T., Nojiri, Y., Körtzinger, A., Steinhoff, T., Hoppema, M., Olafsson, J., Arnarson, T. S., Tilbrook, B., Johannessen, T., Olsen, A., Bellerby, R., Wong, C., Delille, B., Bates, N., and de Baar, H. J.: Climatological mean and decadal change in surface ocean pCO2, and net sea–air CO2 flux over the global oceans, Deep Sea Research Part II: Topical Studies in Oceanography, 56, 554–577, https://doi.org/10.1016/j.dsr2.2008.12.009, 2009. a
Taylor, T. E., Eldering, A., Merrelli, A., Kiel, M., Somkuti, P., Cheng, C., Rosenberg, R., Fisher, B., Crisp, D., Basilio, R., Bennett, M., Cervantes, D., Chang, A., Dang, L., Frankenberg, C., Haemmerle, V. R., Keller, G. R., Kurosu, T., Laughner, J. L., Lee, R., Marchetti, Y., Nelson, R. R., O'Dell, C. W., Osterman, G., Pavlick, R., Roehl, C., Schneider, R., Spiers, G., To, C., Wells, C., Wennberg, P. O., Yelamanchili, A., and Yu, S.: OCO-3 early mission operations and initial (vEarly) XCO2 and SIF retrievals, Remote Sens. Environ., 251, 112032, https://doi.org/10.1016/j.rse.2020.112032, 2020. a
Taylor, T. E., O'Dell, C. W., Baker, D., Bruegge, C., Chang, A., Chapsky, L., Chatterjee, A., Cheng, C., Chevallier, F., Crisp, D., Dang, L., Drouin, B., Eldering, A., Feng, L., Fisher, B., Fu, D., Gunson, M., Haemmerle, V., Keller, G. R., Kiel, M., Kuai, L., Kurosu, T., Lambert, A., Laughner, J., Lee, R., Liu, J., Mandrake, L., Marchetti, Y., McGarragh, G., Merrelli, A., Nelson, R. R., Osterman, G., Oyafuso, F., Palmer, P. I., Payne, V. H., Rosenberg, R., Somkuti, P., Spiers, G., To, C., Weir, B., Wennberg, P. O., Yu, S., and Zong, J.: Evaluating the consistency between OCO-2 and OCO-3 XCO2 estimates derived from the NASA ACOS version 10 retrieval algorithm, Atmos. Meas. Tech., 16, 3173–3209, https://doi.org/10.5194/amt-16-3173-2023, 2023. a, b
Tu, Q., Hase, F., Blumenstock, T., Kivi, R., Heikkinen, P., Sha, M. K., Raffalski, U., Landgraf, J., Lorente, A., Borsdorff, T., Chen, H., Dietrich, F., and Chen, J.: Intercomparison of atmospheric CO2 and CH4 abundances on regional scales in boreal areas using Copernicus Atmosphere Monitoring Service (CAMS) analysis, COllaborative Carbon Column Observing Network (COCCON) spectrometers, and Sentinel-5 Precursor satellite observations, Atmos. Meas. Tech., 13, 4751–4771, https://doi.org/10.5194/amt-13-4751-2020, 2020. a
Turner, A. J., Jacob, D. J., Wecht, K. J., Maasakkers, J. D., Lundgren, E., Andrews, A. E., Biraud, S. C., Boesch, H., Bowman, K. W., Deutscher, N. M., Dubey, M. K., Griffith, D. W. T., Hase, F., Kuze, A., Notholt, J., Ohyama, H., Parker, R., Payne, V. H., Sussmann, R., Sweeney, C., Velazco, V. A., Warneke, T., Wennberg, P. O., and Wunch, D.: Estimating global and North American methane emissions with high spatial resolution using GOSAT satellite data, Atmos. Chem. Phys., 15, 7049–7069, https://doi.org/10.5194/acp-15-7049-2015, 2015. a, b
van der Werf, G. R., Randerson, J. T., Giglio, L., Collatz, G. J., Mu, M., Kasibhatla, P. S., Morton, D. C., DeFries, R. S., Jin, Y., and van Leeuwen, T. T.: Global fire emissions and the contribution of deforestation, savanna, forest, agricultural, and peat fires (1997–2009), Atmos. Chem. Phys., 10, 11707–11735, https://doi.org/10.5194/acp-10-11707-2010, 2010. a
Veefkind, J., Aben, I., McMullan, K., Förster, H., de Vries, J., Otter, G., Claas, J., Eskes, H., de Haan, J., Kleipool, Q., van Weele, M., Hasekamp, O., Hoogeveen, R., Landgraf, J., Snel, R., Tol, P., Ingmann, P., Voors, R., Kruizinga, B., Vink, R., Visser, H., and Levelt, P.: TROPOMI on the ESA Sentinel-5 Precursor: A GMES mission for global observations of the atmospheric composition for climate, air quality and ozone layer applications, Remote Sens. Environ., 120, 70–83, https://doi.org/10.1016/j.rse.2011.09.027, 2012. a, b
Wainwright, C. M., Finney, D. L., Kilavi, M., Black, E., and Marsham, J. H.: Extreme rainfall in East Africa, October 2019–January 2020 and context under future climate change, Weather, 76, 26–31, https://doi.org/10.1002/wea.3824, 2021. a
Wunch, D., Toon, G. C., Wennberg, P. O., Wofsy, S. C., Stephens, B. B., Fischer, M. L., Uchino, O., Abshire, J. B., Bernath, P., Biraud, S. C., Blavier, J.-F. L., Boone, C., Bowman, K. P., Browell, E. V., Campos, T., Connor, B. J., Daube, B. C., Deutscher, N. M., Diao, M., Elkins, J. W., Gerbig, C., Gottlieb, E., Griffith, D. W. T., Hurst, D. F., Jiménez, R., Keppel-Aleks, G., Kort, E. A., Macatangay, R., Machida, T., Matsueda, H., Moore, F., Morino, I., Park, S., Robinson, J., Roehl, C. M., Sawa, Y., Sherlock, V., Sweeney, C., Tanaka, T., and Zondlo, M. A.: Calibration of the Total Carbon Column Observing Network using aircraft profile data, Atmos. Meas. Tech., 3, 1351–1362, https://doi.org/10.5194/amt-3-1351-2010, 2010. a
Wunch, D., Toon, G. C., Blavier, J.-F. L., Washenfelder, R. A., Notholt, J., Connor, B. J., Griffith, D. W. T., Sherlock, V., and Wennberg, P. O.: The Total Carbon Column Observing Network, Philos. T. Roy. Soc. A, 369, 2087–2112, https://doi.org/10.1098/rsta.2010.0240, 2011. a, b
Wunch, D., Toon, G. C., Sherlock, V., Deutscher, N. M., Liu, X., Feist, D. G., and Wennberg, P. O.: The Total Carbon Column Observing Network's GGG 2014 Data Version, CaltechDATA, https://doi.org/10.14291/tccon.ggg2014.documentation.R0/1221662, 2015. a
Wunch, D., Wennberg, P. O., Osterman, G., Fisher, B., Naylor, B., Roehl, C. M., O'Dell, C., Mandrake, L., Viatte, C., Kiel, M., Griffith, D. W. T., Deutscher, N. M., Velazco, V. A., Notholt, J., Warneke, T., Petri, C., De Maziere, M., Sha, M. K., Sussmann, R., Rettinger, M., Pollard, D., Robinson, J., Morino, I., Uchino, O., Hase, F., Blumenstock, T., Feist, D. G., Arnold, S. G., Strong, K., Mendonca, J., Kivi, R., Heikkinen, P., Iraci, L., Podolske, J., Hillyard, P. W., Kawakami, S., Dubey, M. K., Parker, H. A., Sepulveda, E., García, O. E., Te, Y., Jeseck, P., Gunson, M. R., Crisp, D., and Eldering, A.: Comparisons of the Orbiting Carbon Observatory-2 (OCO-2) measurements with TCCON, Atmos. Meas. Tech., 10, 2209–2238, https://doi.org/10.5194/amt-10-2209-2017, 2017. a, b
Zhou, M., Ni, Q., Cai, Z., Langerock, B., Nan, W., Yang, Y., Che, K., Yang, D., Wang, T., Liu, Y., and Wang, P.: CO2 in Beijing and Xianghe Observed by Ground-Based FTIR Column Measurements and Validation to OCO-2/3 Satellite Observations, Remote Sensing, 14, 3769, https://doi.org/10.3390/rs14153769, 2022. a
- Abstract
- Introduction
- The measurement site at NaFIRRI in Jinja, Uganda
- Satellite and model datasets used in this study
- Comparisons of the EM27/SUN total column data with satellite, model, and reanalysis datasets
- Conclusions and outlook
- Appendix A: Further characteristics of the EM27/SUN instrument
- Appendix B: Effect of spatial co-location criteria on EM27/SUN vs. satellite Xgas comparison
- Code and data availability
- Author contributions
- Competing interests
- Disclaimer
- Acknowledgements
- Financial support
- Review statement
- References
- Abstract
- Introduction
- The measurement site at NaFIRRI in Jinja, Uganda
- Satellite and model datasets used in this study
- Comparisons of the EM27/SUN total column data with satellite, model, and reanalysis datasets
- Conclusions and outlook
- Appendix A: Further characteristics of the EM27/SUN instrument
- Appendix B: Effect of spatial co-location criteria on EM27/SUN vs. satellite Xgas comparison
- Code and data availability
- Author contributions
- Competing interests
- Disclaimer
- Acknowledgements
- Financial support
- Review statement
- References