the Creative Commons Attribution 4.0 License.
the Creative Commons Attribution 4.0 License.
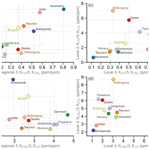
Local and regional enhancements of CH4, CO, and CO2 inferred from TCCON column measurements
Kavitha Mottungan
Chayan Roychoudhury
Vanessa Brocchi
Benjamin Gaubert
Wenfu Tang
Mohammad Amin Mirrezaei
John McKinnon
Yafang Guo
David W. T. Griffith
Dietrich G. Feist
Isamu Morino
Mahesh K. Sha
Manvendra K. Dubey
Martine De Mazière
Nicholas M. Deutscher
Paul O. Wennberg
Ralf Sussmann
Rigel Kivi
Tae-Young Goo
Voltaire A. Velazco
Wei Wang
Avelino F. Arellano Jr.
In this study, we demonstrate the utility of available correlative measurements of carbon species to identify regional and local air mass characteristics as well as their associated source types. In particular, we combine different regression techniques and enhancement ratio algorithms with carbon monoxide (CO), carbon dioxide (CO2), and methane (CH4) total column abundance from 11 sites of the Total Carbon Column Observing Network (TCCON) to infer relative contributions of regional and local sources to each of these sites. The enhancement ratios provide a viable alternative to univariate measures of relationships between the trace gases that are insufficient in capturing source-type and transport signatures. Regional enhancements are estimated from the difference between bivariate regressions across a specific time window of observed total abundance of these species (BERr for bulk enhancement regression ratio) and inferred anomalies (AERr for anomaly enhancement regression ratio) associated with a site-specific background. Since BERr and AERr represent the bulk and local species enhancement ratio, respectively, its difference simply represents the site-specific regional component of these ratios. We can then compare these enhancements for CO2 and CH4 with CO to differentiate between combustion and non-combustion air masses. Our results show that while the regional and local influences in enhancements vary across sites, dominant characteristics are found to be consistent with previous studies over these sites and with bottom-up anthropogenic and fire emission inventories. The site in Pasadena shows a dominant local influence (> 60 %) across all species enhancement ratios, which appear to come from a mixture of biospheric and combustion activities. In contrast, Anmyeondo shows more regionally influenced (> 60 %) air masses associated with high-temperature and/or biofuel combustion activities. Ascension Island appears to only show a large regional influence (> 80 %) on CO CO2 and CO CH4, which is indicative of transported and combustion-related CO from the nearby African region, consistent with a sharp rise in column CO (3.51 ± 0.43 % ppb yr−1) at this site. These methods have important applications to source analysis using spaceborne column retrievals of these species.
- Article
(7350 KB) - Full-text XML
-
Supplement
(2137 KB) - BibTeX
- EndNote
The rise in the abundance of greenhouse gases (e.g., CO2 (carbon dioxide), CH4 (methane)) in recent decades, because of anthropogenic activities and natural emissions associated with climate change, such as wetland and biomass burning emissions associated with El Niño (Zhang et al., 2018; Kumar et al., 2023; van Vuuren and Riahi, 2008; Arneth et al., 2017), has large implications for quantifying atmospheric chemistry–climate relationships. This rising trend increases the complexity of understanding the feedback mechanism between CH4, OH (hydroxyl), and CO (carbon monoxide); retrieval bias in less validated regions or unresolved uncertainty in tropical emissions (e.g., based on TROPOspheric Monitoring Instrument (TROPOMI) and Greenhouse Gases Observing Satellite (GOSAT)) (Lunt et al., 2019; Palmer et al., 2019); and emission estimates from fossil fuel use over growing megacities (Tang et al., 2020; Maasakkers et al., 2019). Understanding today's regional CO2 and CH4 sources and sinks is a key area in carbon cycle and atmospheric composition science, given the necessity for reliable projections of future atmospheric CO2 and CH4 concentrations. This is especially problematic in megacities with the fastest pace of urbanization and where the anthropogenic activities are most intense, accompanied by immense energy consumption mainly in the form of fossil fuel combustion (Kennedy et al., 2015; Grimm et al., 2008; Agudelo-Vera et al., 2012; Banerjee et al., 1999; Lamb et al., 2021). Emission estimates from fossil fuels remain uncertain due to the poor characterization of combustion activity, efficiency, fuel-use mixtures emerging from the lack of details on pollution control strategies, energy use, and combustion practices (Zhu et al., 2012; Creutzig et al., 2015; Kennedy et al., 2009; Baiocchi et al., 2015; Weisz and Steinberger, 2010; Bettencourt et al., 2007; Dodman, 2009; Bai et al., 2018). The high-efficiency combustion of fossil fuels leads to large CO2 emissions compared to CO, whereas low-efficiency combustion of residential combustion and biomass burning, among others, produces more CO (Andreae and Merlet, 2001; Silva and Arellano, 2017; Halliday et al., 2019; Tang et al., 2019; Wei et al., 2012; Andreae, 2019; Park et al., 2021). This uncertainty is further complicated by limited observations at the spatiotemporal scales necessary to resolve variations in combustion and fuel-use patterns (Streets et al., 2013; Nassar et al., 2013; Hutyra et al., 2014; Gately and Hutyra, 2017; Creutzig et al., 2019; Arioli et al., 2020). This leads to difficulties in teasing small anthropogenic signatures from the large natural sources and sinks dominating the carbon cycle and the uncertainties in modeling atmospheric transport (Peylin et al., 2013; Thompson et al., 2016; Erickson and Morgenstern, 2016; Oda et al., 2019; Duncan et al., 2020; Gaubert et al., 2019). This is especially true for flux estimations of CO2 and CH4 using top-down approaches, despite the increase in aircraft and satellite measurements of CO2 and CH4 abundance in recent years (Hutyra et al., 2014; Houweling et al., 2015, 2017; Chevallier et al., 2019; Crowell et al., 2019; Lu et al., 2021; Chandra et al., 2021). Studies have also highlighted the importance of fossil fuel emission uncertainties for their estimates, suggesting the need for temporally defined emission inventories (Gurney et al., 2005; Peylin et al., 2011; Thompson et al., 2016; Saeki and Patra, 2017; Gurney et al., 2020).
The abundance of a species at a particular location is mainly dependent on the variations of sources and sinks. Furthermore, both regional transport and local transport (long-range, vertical transport and dilution in the boundary layer) influence the abundance of the species (especially in the column) and confound measurement interpretations. The major sources of CO2 include anthropogenic emissions, especially fossil fuel combustion, cement production, and land-use change, while sinks include uptakes by ocean and land from the atmosphere (Friedlingstein et al., 2022). While CO is primarily produced through incomplete combustion of carbon-containing fuels, oxidation of CH4 and other volatile organic compounds by OH contributes to secondary production of CO (Bakwin et al., 1995; Gaubert et al., 2016; Hoesly et al., 2018). The main chemical sink of CO in the atmosphere is OH, followed by dry deposition through soil uptake (Levy, 1971; Bartholomew and Alexander, 1981; Khalil and Rasmussen, 1990; Cordero et al., 2019). This coupling of CH4–OH–CO has significant impacts on the growth rate and source–sink characterization of CH4 (Gaubert et al., 2017; Zhao et al., 2019, 2020; Guthrie, 1989; Prather, 1994; Lelieveld et al., 2002). Anthropogenic sources of CH4 include agricultural activities (rice and livestock), solid waste, fossil fuels, and biomass burning in addition to natural sources like anaerobic ecosystems and geological activities (Saunois et al., 2020; Stavert et al., 2022). CH4 and CO are thus coupled with common sources (combustion process, vehicular emission, etc.) and sinks (OH), and changes in one of these species will have a significant impact on the other (Sze, 1977; Gaubert et al., 2017). This covariation (co-emission) or the correlations of the species can be used to derive enhancement ratios or emission ratios which vary according to source regions and source type (Palmer et al., 2006; Wang et al., 2010; Tang et al., 2018). For example, a recent study by Lelandais et al. (2022) uses enhancement ratios and correlations to study the variability of ICOS-observed (Integrated Carbon Observation System, ICOS France) CO, CO2, and CH4 in a Mediterranean climate at different regional and temporal scales. Their results showed that 84 % of their data were representative of background concentrations that were dependent on both wind speed and direction, while 16 % were enhanced by anthropogenic plumes, emissions in the boundary layer, or short-term pollution events. Emission (enhancement) ratios are defined as ratios of excess abundance across two species, often in units of mass (molar) flux when the concentrations of the species are estimated near (away from) the emission source (Andreae, 2019; Lefer et al., 1994). These derived emission or enhancement ratios from multiple species are widely used to characterize emission sources and flux estimation for different parts of the world (Turnbull et al., 2011, 2015; Silva et al., 2013; Anderson et al., 2014; Ammoura et al., 2014; Popa et al., 2014; Parker et al., 2016; Silva and Arellano, 2017; Bukosa et al., 2019; Tang et al., 2019; Lee et al., 2020; Sim et al., 2022; Djuricin et al., 2010; Wunch et al., 2009; Miller et al., 2012; Wennberg et al., 2012; Bozhinova et al., 2014; Super et al., 2017; Hedelius et al., 2018; Plant et al., 2022; Bares et al., 2018). For example, a recent study by Plant et al. (2022) investigated the urban emissions of CH4 and CO using enhancement ratios derived from TROPOMI, while Halliday et al. (2019) categorized air masses during KORUS-AQ into regions of high- or low-efficiency combustion based on CO CO2 enhancement ratios derived from aircraft data. Bukosa et al. (2019) used shipborne measurements of CO, CO2, and CH4 to improve greenhouse gas (GHG) flux estimates by comparing them with GEOS-Chem simulations to identify missing/underestimated sources in the model.
The enhancement ratio between species X and Y is mainly calculated by two methods: the first is from a linearly regressed slope of X and Y (Andreae et al., 1988) and the second is by dividing the excess of X by the excess of Y (Andreae and Merlet, 2001) (see Methods 1 and 2 in Sect. 2.2). The first approach of enhancement ratio estimation using regression slopes is difficult to infer when emitted or locally produced species mix with different air masses (e.g., advection from the nearby sources or mixed air masses) downwind of the dominant source where measurements are made. This is especially the case for vertically integrated quantities like the column measurements (ground-, aircraft-, and satellite-based) (Cheng et al., 2017; Halliday et al., 2019; Tang et al., 2019) where vertical information of the species abundance is practically absent. If the emission or plume concentration is significantly higher than the background, the ratio from the regression slope approach does not change (Briggs et al., 2016). But, when emissions of the species mix with different backgrounds compared to a relatively uniform field, the abundances of X and Y change due to mixing and/or photochemical loss (Mauzerall et al., 1998; Yokelson et al., 2013; Guyon et al., 2005), thus making it difficult to track the locally emitted contribution to the observed abundance. The latter approach of using excess of the species requires a proper understanding of the background concentration to derive the excess abundance along with the instantaneous concentration of the species, which is not available in most cases. Vertical and horizontal transport also complicates the interpretation of abundance and the assessment of local and regional source influences at a particular location (Chatfield et al., 2020). A combination of these two approaches has also been used in previous studies (Hedelius et al., 2018) (see Method 3 in Sect. 2.2). Here, we utilize the column measurements of CO, CO2, and CH4 (denoted as XCO, , and , respectively) from the Total Carbon Column Observing Network (TCCON) (Wunch et al., 2011) to understand these variations in the column abundances.
The main objective of this study is to characterize the bulk characteristics of XCO, , and from ground-based TCCON measurements using a combination of enhancement ratio approaches. Specifically, we introduce a combination of established local and bulk regression algorithms for deriving enhancement ratios of the column abundances between these three species to understand their relationships, because these constituents are being mixed, dispersed, transported, and transformed in the atmosphere. More importantly, we present the utility of combining these techniques for quantifying the contributions of the regional and local influences to observed columns and the corresponding enhancements observed in the respective species. We then examine the regional and seasonal variations of these influences and make use of the variability in the relationship of the multi-species enhancement ratios to infer the dominant source type leading to these variations. While previous studies have used enhancement ratios to examine the source attribution of XCO, , and at a regional and/or local scale (Bukosa et al., 2019), the novelty of this study lies in investigating the bulk characteristics on a source-type basis using all three species and using a combination of different regression algorithms for globally distributed column-integrated measurements. This proof of concept has an important application for ongoing and planned satellite missions of these species, given that TCCON measurements serve as a basis for retrieval validation of these missions.
2.1 Data and location features
We make use of the column-averaged mixing ratios of CO, CO2, and CH4 (denoted as XCO, , ) from the ground-based TCCON from 2012 to 2019. TCCON retrieves the column abundance from the near-infrared solar absorption spectra using high-resolution Fourier transform spectrometers (Wunch et al., 2011). This network provides the column-averaged dry-air mole fractions by normalizing the column abundance of the species of interest to the retrieved oxygen column abundance. The precision of the column-averaged mole fraction of CO2 () is < 0.25 %, while for CH4 () it is < 0.3 % and for CO (XCO) it is < 1 % under clear or partly cloudy skies (Wunch et al., 2010). TCCON datasets are widely used in global carbon cycle studies to improve the carbon budget (source and sink information) and for validation of atmospheric trace gas estimates retrieved from the space-based instruments such as Orbiting Carbon Observatory (OCO-2), GOSAT, GOSAT-2, and TROPOMI (Miller et al., 2007; Morino et al., 2011; Frankenberg et al., 2015; Wunch et al., 2017; Qu et al., 2021; Wang et al., 2022; Kulawik et al., 2016; Yoshida et al., 2013; Noël et al., 2022; Liang et al., 2017; Kong et al., 2019; Sha et al., 2021). A total of 11 TCCON sites are selected for this analysis, which includes 6 sites in the Northern Hemisphere (NH) and 5 in the Southern Hemisphere (SH), and the locations are marked in Fig. 1. The average column abundance retrieved at each TCCON location is embedded in the monthly averaged spatial map of XCO from the Measurements Of Pollution In The Troposphere (MOPITT) instrument aboard Terra. A similar average column abundance is shown through the spatial maps of from OCO-2 averaged from 2015–2019 and of from GOSAT averaged from 2012–2019. XCO and from MOPITT (and GOSAT) show good agreement with a Pearson's correlation of 0.96 (and 0.97) and mean bias relative to TCCON of −12.81 ppb (−7.12 ppb). OCO-2 , on the other hand, has a higher bias and weaker correlation relative to TCCON (correlation of 0.6 and bias of 1.95 ppm), with the lowest (highest) bias in Pasadena (Manaus). The monthly mean variations in the three species across the sites shown in Fig. S3 in the Supplement highlight the hemispheric differences in XCO, , and among TCCON locations.
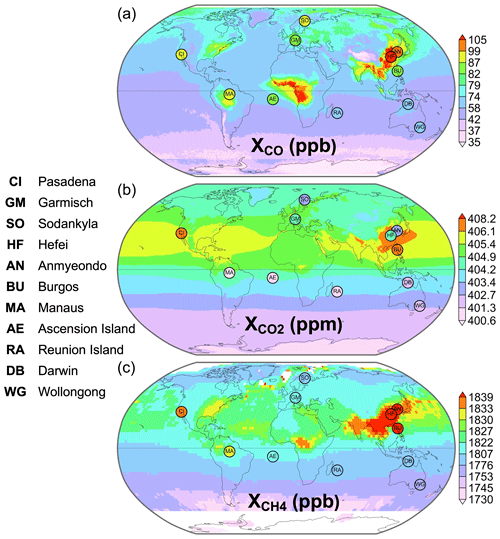
Figure 1Monthly average abundance of (a) CO from MOPITT between 2012–2019, (b) CO2 from OCO-2 between 2015–2019, and (c) CH4 from GOSAT-1 between 2012–2019. Locations of TCCON sites are superimposed as black circles with their respective TCCON ID (left legend).
The site for Ascension Island (Ascension hereafter) is on a small island with virtually no influence from local sources, but it captures the long-range transport of emissions from Africa (Geibel et al., 2010; Feist et al., 2017; Swap et al., 1996). A significant positive trend in XCO is observed over Ascension (3.51 ± 0.43 % ppb yr−1) with negative XCO trends in the other sites (Table 2). This can be attributed to an increase in burned area and transport from southern Africa reported in previous studies (Buchholz et al., 2021; Andela et al., 2017; Borsdorff et al., 2018). This in combination with the low trend observed in over Ascension may be attributed to a decrease in sources (reduced respiration, increase in lower-quality fossil fuels) or an increase in sinks (enhanced photosynthesis) over the African region. Hickman et al. (2021) reported an increasing trend in CO over northern equatorial Africa due to a decline in biomass burning emissions from a woodier biome. Among the selected sites of study, Ascension and Réunion are representative of remote island sites located in the South Atlantic and the Indian Ocean, respectively. The humidity in the eastern part of Réunion island is higher than its western counterpart. There is also a regularly occurring outflow of biomass burning emissions from southern Africa, Madagascar, and South America to Réunion island (Vigouroux et al., 2012; De Mazière et al., 2017; Zhou et al., 2018). The sites in Manaus, Darwin, Garmisch, and Sodankylä are reported to be mostly influenced by sources related to local biogenic emissions and regional anthropogenic emissions. Manaus is in the center of the Amazon, the world's largest rainforest, and is the seventh largest city in Brazil (Dubey et al., 2014). The measurement site in Garmisch is situated in the Alps mountain range in southern Germany (Sussmann and Rettinger, 2018), while the site in Sodankylä is in northern Finland, mainly surrounded by Scots pine forest within the Fennoscandia region. Wintertime measurements at this location are not possible due to the absence of sunlight (Kivi et al., 2014). Finally, Darwin is the largest city in the sparsely populated Northern Territory of Australia and is situated on the Timor Sea. The site is 9 km from the city of Darwin and adjacent to the airport (Griffith et al., 2014a).
It has been previously reported that local emissions and nearby sources are significant at Pasadena, Anmyeondo, and Wollongong (Griffith et al., 2014b; Wennberg et al., 2015; Goo et al., 2014). The measurement site in Pasadena is situated at the northern limit of the South Coast Air Basin, which is bounded by mountains on three sides and the Pacific Ocean on the other side. The northern and eastern regions of the basin are sparsely populated deserts and receive polluted air under normal meteorological conditions, occasionally with cleaner air (Wunch et al., 2009; Wennberg et al., 2012). In the SH, the measurement site of Wollongong is representative of an urban location. The urban sources are local and are mainly from Sydney's motorway flanks, coal mining, and steelmaking facilities (Buchholz et al., 2016). Biogenic emissions and bushfires also impact the air at this site, along with agricultural activities on the southwest side of the urban extent (Griffith et al., 2014b; Buchholz et al., 2016). Anmyeondo island is located on the west coast of the Korean Peninsula, 180 km southeast of Seoul. Although the surrounding area mainly consists of agricultural lands, there is vegetation in and around the site, consisting of pine trees, natural forest, and urban developments. This site is regularly influenced by Asian pollution outflows, especially during spring (Goo et al., 2014; Oh et al., 2018). From Table 2, increased and trends are observed at all locations. The trend in is highest over Anmyeondo (0.81 ± 0.10 % ppm yr−1) and lowest over Ascension (0.60 ± 0.01 % ppm yr−1). Similarly, shows a high trend over Sodankylä (0.48 ± 0.02 % ppm yr−1) and a low trend over Anmyeondo (0.21 ± 0.15 % ppm yr−1). This is possibly due to differences in the distribution of sources and/or sinks across these sites as described in the previous paragraphs.
The air masses in the Burgos and Hefei sites are mainly dominated by regionally transported emissions (Morino et al., 2018; Liu et al., 2018). The average XCO is high in these regions (also Anmyeondo) compared to other sites (Table 2), which is consistent with previous literature that reports higher emissions from fossil fuels, coal, agricultural activities, and wetlands (Tang et al., 2019; Zhang et al., 2020). Hefei is an inland city in the eastern part of China, and it is a rapidly developing city with a population of 8 million. The site is adjacent to a lake in flat terrain and is in the northwestern rural area of Hefei city. A large anthropogenic influence in Hefei mainly comes from heavily polluted areas in northern China and cities in the Yangtze River Delta, while natural emissions come from cultivated lands or wetlands surrounding the site (Tian et al., 2018; Wang et al., 2017). The site in Burgos is in a town in Ilocos Norte Province in the Philippines. This region is a coal-free province and encounters relatively clean marine air from the western Pacific but also polluted air from long-range transport during monsoon transitions (Velazco et al., 2017). The data period and a summary of the characteristics of these selected TCCON sites are listed in Table 1. The sites at Pasadena, Garmisch, Réunion, Ascension, Sodankylä, Darwin, and Wollongong have longer records (> 7 years of data) as opposed to Anmyeondo, Hefei, Manaus, and Burgos (∼ 2 years with more gaps in between).
2.2 Estimating regional and local enhancement ratios
The observed column abundance (C) of any species (spc) retrieved at any location of TCCON measurement sites (s) and at a particular time (t) is generally represented as
where Ctrue is the true species concentration being measured, and ϵmeas is the measurement error. Letting , CY=CCO, and , the true concentration can be broken down into specific contributions following Levin et al. (2003) and Turnbull et al. (2009) as
The subscripts in the above equations represent the associated sources and sinks: background (bg); anthropogenic processes such as fossil fuel (ff), biomass burning (bb), cement (c), and livestock (live); biospheric processes such as ecosystem respiration (r) and photosynthesis uptake (p); natural processes such as ocean (o), soil uptake (su), and wetland (wet); chemical processes such as oxidation from hydrocarbons (ox), chemical loss by OH (l), and chemical loss by OH and Cl (cl); and other sources (oth). The background component (bg) accounts for initial abundance, dilution, and transport processes. Direct biogenic CO emissions and oxidation of CH4 (Zcl) as a source of CO are included in Yox. We also consider the oxidation of Y to X as a source X to be negligible in this analysis.
In this study, we adopt the following three main methods to derive enhancement ratios.
-
Method 1: regression of the abundances (i.e., associated linear slope from the scatterplots between CX and CY, CX and CZ, or CY and CZ). This method is denoted as bulk enhancement regression ratio (BERr) (Andreae et al., 1988; Lefer et al., 1994; Silva et al., 2013; Tang et al., 2019) – see Eqs. (5), (6), and (7).
-
Method 2: ratio of Cspc anomalies (anomaly enhancement ratio or AERa) (Andreae and Merlet, 2001; Silva and Arellano, 2017; Le Canut et al., 1996) – see Eqs. (8), (9), and (10).
-
Method 3: regression of Cspc anomalies (anomaly enhancement regression ratio or AERr) (Mauzerall et al., 1998; Yokelson et al., 2013; Hobbs et al., 2003; Wunch et al., 2009; Hedelius et al., 2018; Sim et al., 2022) – see Eqs. (11) and (12).
The regressions and anomaly of abundances are calculated using daily average data points across a monthly time window. The number of daily column abundance data points available in each month at the selected TCCON location sites is provided in Fig. S1. This information is used further in the analysis for selecting the data range for comparison purposes and interpreting the results.
Method 1. The enhancement ratio based on the regression of the daily average abundances of the species is considered the bulk or global enhancement ratio (BERr), which is interpreted to represent the sum of all the associated source and sink contributions. The BERr or regression slope of daily average abundances of species X and Y, for example, is calculated simply as the ratio of the covariance of CX and CY to the variance of CX from a least-squares linear fit of the data. That is,
where sources of X = ff, bb, c, and r and sinks of X = p, o, and su, while subscript 1 denotes Method 1.
Note that for different linear regression approaches there is a significant difference in the slope estimation when the representation of the error (ϵmeas,spc) associated with the data is included (Wu and Yu, 2018). To account for the differences in the estimates due to the choice of algorithm, we use three regression methods (ordinary least squares, geometric mean, and York) (York et al., 2004) for calculating the enhancement ratios derived based on regression approaches in Methods 1 and 3. The enhancement ratios of BERr and AERr reported in the study are the mean of these estimates weighted by the associated error (Verhulst et al., 2017).
where BERr is the bulk enhancement ratio (or the weighted average of the slopes calculated from three regression algorithms). The weights are based on the associated errors (σ) from each regression algorithm.
Method 2. Local enhancement ratios are derived based on Methods 2 and 3, where the background influences/transport components are removed from the total abundances used in Method 1 using two ways to estimate anomalies (Eq. 8). That is, (1) we remove the dilution/boundary layer influence from the total abundance (broadly denoted as Cbg,spc) by taking the difference between average morning values and the average afternoon values (Wunch et al., 2009; Yokelson et al., 2013), and (2) we remove the background by calculating the difference between the background value Cbg,spc (assumed here as the 5th percentile of the daily data) and the individual daily average values. Using the difference between morning and afternoon values of the abundance minimizes (1) the influence of high concentration of the species within the boundary layer in the morning (Yokelson et al., 2013) and (2) the spectroscopic errors (Wunch et al., 2009). The anomaly of Cspc after removing these influences from the total abundance is expressed as
with AERa between species X and Y for Method 2, for example, given by
The average AERa is the weighted average of AERa calculated using the AERa from (1) boundary layer influence and (2) the 5th percentile methods. The weights are based on the errors (standard deviations) of based on ways (1) and (2).
Method 3. Accordingly, the regression slope (AERr) between species X and Y for Method 3, for example, can be calculated using the combination of Eqs. (5) and (8):
The regression slopes are calculated using three algorithms as in Method 1 on the anomalies calculated from Method 2. AERr is the weighted average of the regression slopes and their associated errors similar to Eq. (7). Similar expressions can be applied to BERr, AERa, and AERr for species X and Z, as well as for Y and Z.
We also derive the enhancement of each species due to these regional and local enhancements. The regional enhancement ratio is calculated by subtracting the enhancement ratios, derived based on the regression slope of total abundances in Method 1 (BERr), from that of the ratio derived from the anomalies in Method 3 (AERr) (Cheng et al., 2017; Briggs et al., 2016; Le Canut et al., 1996). The local enhancement ratio is given by AERr (from Method 3). Thus, the mean enhancement ()i of a species, Y for example, can be calculated as the product of and , where i is either R = BERr − AERr or L = AERr, representing the regional (R) and local (L) enhancement ratios, respectively, and is the anomaly of species X calculated using Method 2 (AERa). That is, the regional (R) enhancement of CO for this example can be derived from the enhancement ratio in CO CO2 as
and similarly from the enhancement ratio in CO CH4 as
We then take the mean of two enhancements ( and ) for species Y to account for species variations. Similar calculations are carried out for local (L) enhancements. The relative contributions of the regional and local enhancement ratio are calculated as and , respectively.
This section describes the spatial and temporal variation and covariation of Cspc along with their corresponding local and regional enhancement ratios. We also present in this section several qualitative inferences on the dominant processes leading to these covariations.
3.1 Covariation of CO, CO2, and CH4
From Fig. S2, a clear seasonal cycle in XCO over all the locations is observed. This can indicate the presence of a non-steady-state source/sink at the locations including potential regional transport into and out of the site. The seasonal cycle of and is evident for Pasadena, Garmisch, and Sodankylä based on the proximity of these sites to emission sources and sinks (e.g., carbon uptake by biosphere during summer) as described in Sect. 2.1. The low seasonal cycles of CO2 and CH4 at other sites can be mainly due to their remote location with relatively mixed air masses and smaller influences of local emissions (e.g., Ciais et al., 2019).
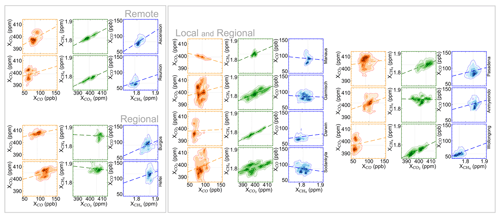
Figure 2Joint probability distributions between XCO and (orange), and (green), and and XCO (blue) using daily values across 11 TCCON sites chosen for this study. The sites are grouped according to the site type and source influence on the species in these regions. XCO is shown in parts per billion (ppb), whereas and have units in parts per million (ppm). The straight lines denote the best-fit line from linear regression.
To elucidate the dependence of similar variations and/or similar sources of origin, we show in Fig. 2 the joint probability density distribution (PDF) between XCO and , XCO and , and and . We provide estimates of the associated dependencies (linear vs. non-linear) among these species for the period listed in Table 2. The linear relationship is quantified using the Pearson's correlation, while the non-linear dependency is estimated using mutual information (Kraskov et al., 2004). Consistent correlations across all three species suggest a similar source of origin, seen in the strong linear correlation across the species over Ascension and strong non-linear correlation across the species in Anmyeondo and Hefei. Strong dependencies are observed among and in most locations, where the correlations are higher than the ones between XCO and and XCO and . This is also seen in the joint distributions where the relationship between and is more apparent compared to others and points towards a shared signature from biospheric/natural and anthropogenic activities, leading to a strong relationship between and . The differences observed between the non-linear and linear dependencies highlight the complexity of the relationship between the species and can be associated with the presence of daily variation in the sources and sinks, seasonality, differences in the lifetime of the species, and changes in the background present in the entire analysis period. We further investigate the variations in corresponding enhancement ratios in the next section to understand these differences.
3.2 Enhancement ratios of XCO, , and
Figure 3 shows the mean variation of these enhancement ratios in XCO , , and XCO . Note that these ratios are calculated monthly across the daily data based on the methods explained in Sect. 2.2. The bulk enhancement ratio (BERr), which accounts for the total emission sources, sinks, and other contributions to observed abundances, is higher for all species at all measurement sites in comparison to the local enhancement ratios (AERa and AERr). Regionally, BERr in is at a maximum over the Southeast Asian region (Anmyeondo, Burgos, and Hefei) followed by the sites in the SH (Darwin, Wollongong, Réunion, and Ascension) when compared to other NH sites. This higher value of BERr in the Southeast Asian region follows the regional maximum of XCO and described in Sect. 3.1 and shown in Fig. S3. The case is similar for the regional site variation of BERr in XCO . The value of from BERr is highest over Burgos and Wollongong followed by Garmisch, Sodankylä, Anmyeondo, and Pasadena. Relative differences can be observed between the correlations across the species and BERr, suggesting more complex mixtures of the sources and sinks of these species at these sites.
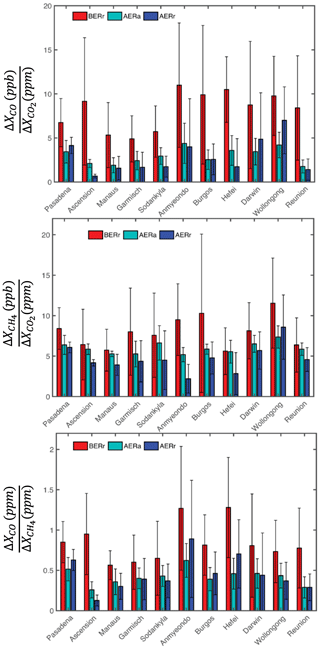
Figure 3Mean variation of enhancement ratios calculated as bulk enhancement ratio (BERr), anomaly enhancement ratio (AERa), and anomaly enhancement regression ratio (AERr) of XCO , , and XCO during 2012–2019 over the 11 TCCON sites.
We note that the enhancement ratios derived in this work are within the range of ratio estimates reported in the literature (Wunch et al., 2009; Wennberg et al., 2012; Silva et al., 2013; Buchholz et al., 2016; Hedelius et al., 2018; Bukosa et al., 2019). In Pasadena, Silva et al. (2013) reported an enhancement ratio in CO CO2 of about 9.3–13.5 ppb ppm−1 based on MOPITTv5 and ACOS2.9/GOSAT CO2 data, while Wunch et al. (2009) and Wennberg et al. (2012) reported 11 and 8.4 ppb ppm−1, respectively, along with the more recent study by Hedelius et al. (2018) which reported 7.1 to 7.5 ppb ppm−1. Buchholz et al. (2016) and Bukosa et al. (2019) reported a range of ratios of about 1.3–37.4 ppb ppm−1 in CO CO2, 9.8–61 ppb ppm−1 in CH4 CO2, and 0.3–13 ppb ppb−1 in CH4 CO over Australia. While generally consistent, our estimates also show that the range of ratios reported in these studies can vary (as can be expected), depending on the dominant processes (natural and/or anthropogenic) driving species abundance.
3.3 Regional and local contributions
Additionally, the differences in the enhancement ratio from BERr, AERa, and AERr in Fig. 3 can be indicative of different regional and local influences. As described in Sect. 2.2, the enhancement ratio calculated from the regression slope of the anomalies (AERr) represents a local enhancement ratio, where the associated regional enhancement ratio can then be derived by subtracting AERr from BERr (i.e., regional = bulk − local). Figure 4 shows the average seasonal variation of the regional (BERr − AERr) and local enhancement ratios (AERr) for each species. This reveals how the contribution and influence of regional and local enhancement ratios in the bulk ratio vary seasonally. The seasonal variations calculated for DJF should read as winter in the NH and summer in the SH, MAM months should read as spring in the NH and fall in the SH, JJA months should read as summer in the NH and winter in the SH, and SON months should read as fall in the NH and spring in the SH. The corresponding number of months available to generate the average seasonal variation of regional and local enhancement ratios is provided in the Supplement (Tables S1 and S2). Note that for sites like Sodankylä, there are only four data points for seasonal averaging during winter months due to limited measurements during this period.
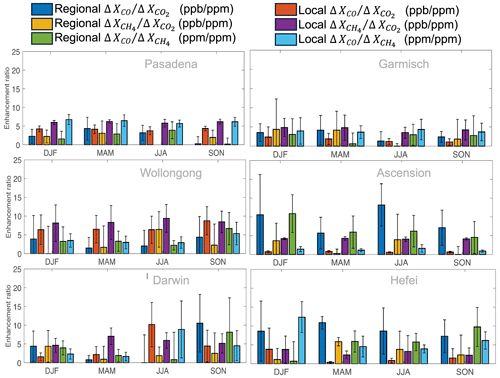
Figure 4Average seasonal variation of regional and local enhancement ratio for CO CO2, CH4 CO2, and CO CH4 during 2012–2019 over Pasadena, Garmisch, Wollongong, Ascension, Darwin, and Hefei.
We see in Fig. 4 that the seasonal variation of regional and local enhancement ratios at different measurement sites reveals the presence of seasonally varying driving factors in the bulk enhancement ratios. The local enhancement ratio appears to dominate over the regional ratios for Pasadena across all seasons. The local enhancement ratios in XCO and XCO compared to the regional ratios are more significant during fall season (SON). This may be due to the poor dependency between transported CH4, CO2 coming from biospheric sources, or any non-combustion sources of CO. This is evident in Fig. S3, which shows a significant peak in the abundance of during fall months over Pasadena but not in XCO. Furthermore, the low value of regional enhancement ratio in during summer over Pasadena may be associated with the poor correlation among the species from independent sources or from biospheric sinks of CO2 (see Tables S1 and S2). A similar seasonal variation is observed at Wollongong, which shows a dominant influence of local enhancements of species ratios for most of the seasons. Relative to the regional ratio, the magnitude of local enhancement ratio in is more significant during the months of DJF, which is the summer season in the SH. The seasonal variation of XCO follows a different pattern in Wollongong with the regional influence dominating for all seasons except JJA (winter in the SH). This can be attributed to a similar chemical loss of CO and CH4 through OH, especially in spring and summer in the SH (Lelieveld et al., 2016; Fisher et al., 2015). The seasonal variation of species enhancement ratio in and XCO at Darwin follows similar variations as those in Wollongong, although there are differences in absolute magnitude due to seasonal bushfire occurrences and fire emissions across south and north Australia (Dowdy et al., 2018). The regional enhancement ratio in XCO dominates during DJF (summer) and SON (spring) at Darwin, whereas the local enhancement ratio dominates in other seasons. A large difference of about 10 ppb ppm−1 is also observed between local and regional enhancement ratios in XCO during JJA (winter) months.
Furthermore, over Ascension, the influence of regional enhancement ratios in XCO and XCO is high during all seasons, whereas the seasonal variation in shows a different pattern. Except in spring (SON) and fall (MAM), the seasonal influence of the regional and local enhancement ratio in is comparable. The low values of regional enhancement in during spring and fall may be associated with the poor correlation among the species from independent sources or from biospheric sources of CO2. The seasonal variation of enhancement ratio at Manaus and Réunion follows this characteristic as well (shown in Fig. S4). The relative importance of regional and local enhancement ratios varies among species in Garmisch and Sodankylä (in Fig. S3). The regional enhancement ratio in XCO and local enhancement ratio in XCO dominate for all seasons at Garmisch (Fig. 4) and Sodankylä (Fig. S4), while the local enhancement ratio in dominates during JJA (winter) and SON (spring) months compared to other seasons over these sites. Finally, irrespective of the season, regional enhancements in XCO dominate at Hefei and Burgos (Fig. S4), while the same is true in at Anmyeondo (Fig. S4). The local enhancement ratio in and XCO dominates only during DJF (winter) at Hefei, while the local enhancement ratio in XCO dominates for all seasons at Anmyeondo except fall (SON). The local enhancement ratio in XCO also dominates regardless of season at Burgos.
Table 3Percent contribution of regional and local enhancements and their associated errors to the ratios of XCO , , and XCO during 2012–2019 over the 11 TCCON sites.
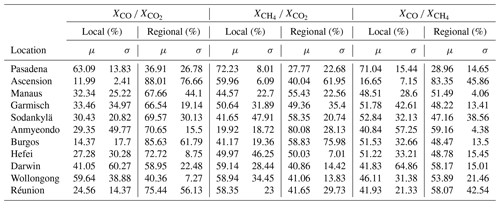
The average relative contribution of local and regional enhancement ratios towards the bulk enhancement ratio at each measurement site is provided in Table 3. A clear difference is observed in the contribution of the local and regional enhancement ratios across each measurement site and among species. Locations like Pasadena and Wollongong show the dominant local influence for XCO , whereas the rest of the locations report significant regional influences. This regional contribution of XCO to the bulk enhancement ratio is highest over Ascension followed by Burgos (> 80 %). This can be attributed to the fact that Ascension is a remote location, and the sharp rise in the column abundance of CO at Ascension can be associated with a rise in transported CO from the nearby African region. Previous studies over Burgos and the vicinity also reported enhanced CO and CH4 due to transport of emissions from East Asia (Velazco et al., 2017; Hilario et al., 2021). This inference is in support of the location features provided in Sect. 2.1 and source information as reported in previous studies. The contribution of regional enhancement ratios dominates over Manaus, Anmyeondo, Sodankylä, Hefei, and Burgos to the bulk enhancement ratio in , while the remaining sites report dominance of its local enhancement ratio. Except for Ascension, Manaus, Darwin, Anmyeondo, and Réunion, the contribution of local enhancement ratio for XCO is higher than the regional one at all other measurement sites.
With the difference in the contributions of regional and local enhancement ratios, we also derive the enhancement of each species due to these regional and local enhancements as outlined in Sect. 2.2. The average variation of the regional and local enhancements of XCO, , and is provided in Fig. S5. A large difference (10–28 ppb) is observed in the relative increase in XCO between regional and local enhancements over Burgos, Ascension, and Réunion. The relative increase in at Sodankylä, Anmyeondo, and Burgos shows the dominance of local enhancements, while the remaining locations show higher importance of regional processes. Except at Ascension and Anmyeondo, all other measurement sites show that the relative rise in comes from regional processes. The difference in the relative increase in and between regional and local enhancements is less in most of the locations compared to the corresponding relative increase in XCO. This smaller difference in the relative increase can be attributed to the long lifetime, uniform mixing characteristic, and large background value of and compared to that of XCO in the atmosphere. The different processes and source types leading to this regional variation and seasonality in the local and regional enhancement ratios are further analyzed using the scatterplots of multi-species ratios in the next section.
3.4 Inferring the dominant process contribution from multi-species enhancement ratios
Figure 5 shows the scatterplot of the ratio in XCO vs. XCO and vs. XCO for regional and local enhancements. We use the relationship of the multi-species ratios (XCO vs. XCO and vs. XCO) to qualitatively infer the processes influencing the regional and local enhancement ratios at each measurement site. For example, high-temperature or more efficient combustion processes lead to the emission of more CO2 compared to CO, and low-temperature combustion produces more CO (Silva and Arellano, 2017). Similarly, activities associated with the extraction of coal and distribution of natural gas, wetlands, rice cultivation, landfill, and livestock result in higher emission of CH4 compared to emissions of CO and CO2. Lower (higher) ratio values of both XCO vs. XCO in the scatterplots can be related to processes emitting lower (higher) CO. A similar approach is applied for ratio variations in vs. XCO. We confirm the classification of these low to high values using K-means clustering. A summary of these categories for both regional and local enhancements is listed in Tables 4 and 5.
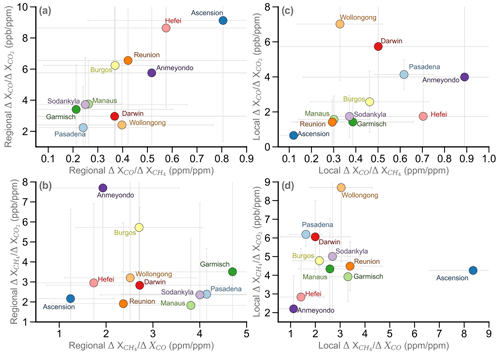
Figure 5Scatterplot of average regional (a, b) and local (c, d) enhancement ratios in CO CO2 vs. CO CH4 (a, c) and CH4 CO2 and CH4 CO (b, d) during 2012–2019 with their associated errors.
Table 4Regional process inference based on the ratios of XCO , XCO , , and XCO over the 11 TCCON sites.
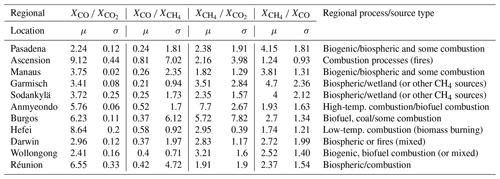
Table 5Local process inference based on the ratios of XCO , XCO , , and XCO over the 11 TCCON sites.
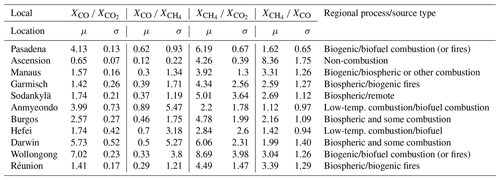
The scatterplot of regional enhancement ratio of species at Pasadena, Manaus, Garmisch, Sodankylä, Darwin, and Wollongong shows relatively low values of XCO vs. XCO and medium/high values of vs. XCO. The regional enhancement ratio showed a value between 2.24 and 3.75 ppb ppm−1 for XCO , between 1.83 and 3.51 ppb ppm−1 for , and between 3.81 and 4.69 ppm ppm−1 for XCO over these regions. This pattern can suggest a dominant process (or a combination) that is characterized by low CO and high CH4, CO2 emissions from natural and biospheric sources, and/or anthropogenic sources with high activity and efficiency. These values fall within the range of previously reported ratios for a mixture of natural and anthropogenic emissions (2–6 ppb ppb−1 for and 3.3–8.0 ppb ppm−1 for XCO ; Bukosa et al., 2019). The location features of these measurement sites provided in Sect. 2.1 also support this result.
A relatively high/medium value of XCO (6.55–9.12 ppb ppm−1) vs. XCO and relatively low values of (1.91–2.95 ppb ppm−1) vs. XCO (1.24–2.37 ppb ppm−1) can be seen over Réunion, Ascension, and Hefei. This variation appears to suggest the presence of low-temperature combustion processes (i.e., biomass burning, especially smoldering fires) emitting more CO. A study by Bremer et al. (2004) attributed the enhancement in MOPITT-based CO column abundance at Ascension to sub-Saharan biomass burning emissions, while Zhou et al. (2018) reported that the seasonality of CO at two sites, Saint-Denis and Maïdo (in Réunion), is primarily driven by biomass burning emissions in Africa and South America. Wang et al., 2017 also reported an enhancement ratio of 5.6 ppb ppm−1 for XCO at Hefei during October 2014 and recognized incomplete combustion of fossil fuels as the main source of CO in this area. The relatively medium value of XCO (5.76 and 6.23 ppb ppm−1) vs. XCO and high (7.69 and 5.72 ppb ppm−1) vs. XCO (1.93 and 2.71 ppm ppm−1) suggest the presence of fossil fuel emissions, coal/biofuel processes, agriculture, or wetland emissions over Anmyeondo and Burgos. The ratio is close to the range of ratios of 3.3–8 ppb ppm−1 for XCO and 1.6–4.2 ppb ppb−1 for XCO reported in emissions of mixed anthropogenic sources from rural and urban areas (Bukosa et al., 2019). Initial analysis of TCCON data in Burgos by Velazco et al. (2017) suggested that the enhancement in CO over the northern part of the Philippines is mostly from fossil fuel emissions, which is dominated by transported emissions from East Asia, and has little influence from biomass burning, which can be large over the southern part of the region (Edwards et al., 2022).
The scatterplot of local enhancement ratio over Wollongong conveys a relatively high/medium ratio in XCO (7.02 ppb ppm−1) vs. XCO and relatively high/medium ratio in (8.69 ppb ppm−1) vs. XCO (3.04 ppm ppm−1). This appears to suggest active low-temperature combustion (biomass burning or fires) producing CO and biofuel combustion or coal activities leading to the production of more CH4. This value is within the range of values reported for mixed anthropogenic emissions in Wollongong (Buchholz et al., 2016). Our estimated value is less than the ratio of 13–61 ppb ppm−1 in reported in Wollongong for coal mining. This may be due to the impact of mixing (dilution) of other sources. The ratios between 4.13 and 5.73 ppb ppm−1 in XCO , between 6.18 and 6.06 ppb ppm−1 in , and between 1.62 and 1.99 ppm ppm−1 in XCO (a lower ratio) appear to suggest the presence of mixed emissions from anthropogenic or combustion activities in Pasadena and Darwin. This coincides with reports by Hedelius et al. (2018) of a canyon gas leak and wildfire activities based on a ratio of 7.3 ppb ppm−1 in CH4 CO2 and 7.1 ppb ppm−1 in XCO in Pasadena. The local enhancement ratio at remaining locations shows a relatively low ratio in XCO vs. XCO and relatively medium/high ratio in vs. XCO, which can indicate dominance of biogenic or non-combustion processes influencing these ratios at these locations. The scatterplots of these enhancement ratios between species across seasons (Figs. S6 to S9) reveal similar results as those shown in Fig. 5, but slight seasonal variations are observed at Hefei, Réunion, Darwin, and Wollongong.
3.5 Comparison of column abundance with emission estimates
We show in Fig. 6 the average contribution (in %) to the emissions of CO, CO2, and CH4 over these measurement sites from the anthropogenic sector, as reported in the Copernicus Atmosphere Monitoring Service emission inventory (CAMS v4.1; Granier et al., 2019), and the biomass burning sector, as reported in the Global Fire Emission Database (GFED4; Giglio et al., 2013). These emission inventories are utilized for qualitative comparison of local emission sources or processes inferred from the scatterplot relationships of multi-species enhancement ratios (see Tables 4 and 5). It has to be noted that most of the emissions from the anthropogenic sector of CAMS have emissions with less temporal variability compared to seasonal variability, including inter-annual variability of biomass burning emissions from GFED. The average total emissions around the grid location of the TCCON measurement site are also provided in Fig. 6.
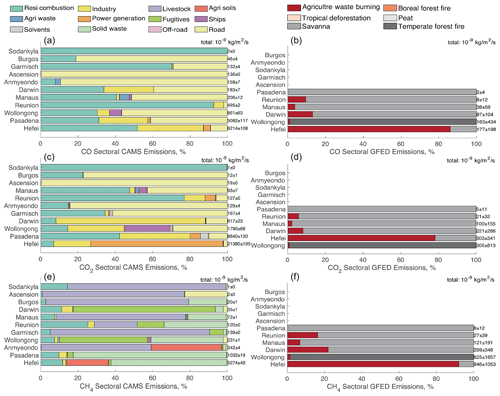
Figure 6Sectoral emission distribution (%) of CO, CO2, and CH4 from CAMS anthropogenic emissions (a, c, e) and GFED fire (b, d, f) at TCCON measurement sites during 2012–2019. Corresponding total emissions are indicated on the secondary (right) y axis.
Regionally, the anthropogenic and fire emission sectors dominate over Hefei, Wollongong, and Darwin compared to other sites (Fig. 6). The anthropogenic emission sectors for CO, CO2, and CH4 are also significant over Hefei, Pasadena, Wollongong, and Anmyeondo. Residential combustion, industries, power generation, and road transport influence local CO at Hefei. Similarly, residential combustion, industries, and road transport influence local CO in Pasadena, whereas in Wollongong CO emissions only come from residential combustion and road transport sectors. A large portion of CO2 emission in Hefei comes from the power generation sector, followed by industries and residential combustion. The major CO2 emission sectors in Pasadena include industry and residential combustion. Wollongong has CO2 emissions from the following sectors: industry, residential combustion, and ships. Note that Hefei, Pasadena, Anmyeondo, and Wollongong have significant emissions of CH4 from anthropogenic sectors. Solid waste and agricultural soils are the significant emission sectors for CH4 at Hefei. The main sectors for CH4 emissions at Anmyeondo include livestock and agricultural soils. Emissions from fugitives, solid waste, and water are significant emitters of CH4 at Wollongong. These mixtures of emission sectors at these sites support the dominant processes identified in the previous section using the correlation of enhancement ratios of these species from TCCON (Fig. 5 and Tables 4 and 5).
The emission from biomass burning is one of the main factors influencing the seasonality and inter-annual variability in the abundance of species. The strong monthly variability of CO and CO2 at Darwin, Wollongong, Réunion, and Pasadena can be attributed to the seasonality of biomass burning emissions (Figs. S2 and 5). Agricultural waste burning is the main emission sector for CO, CO2, and CH4 at Hefei. The seasonality of CO, CO2, and CH4 at Wollongong is due to emissions from temperate forest fires (Fig. 5), while the biomass burning activity at Darwin, Réunion, and Manaus appears to be dominated by savanna fires followed by agricultural waste burning (Fig. 5). Sodankylä, Ascension, and Burgos sites are remote locations, and surrounding (local) emissions are therefore lower than those of the other sites. Even though Réunion is a relatively small and isolated island, the contribution from local biomass burning activity and other anthropogenic sources is found to be considerable.
Despite the growing global burden in CO2 and CH4, current measurements of total column CO2 () and CH4 () provide a limited verifiable capability in identifying and quantifying specific types of their corresponding sources and sinks. In addition to the lack of vertical information from these column measurements, the diffusive nature of the atmosphere (mixing air masses influenced by spatially and temporally heterogenous sources and sinks) makes it very challenging to track source-type contributions to these observed column abundances. In this work, we combine simultaneous ground-based measurements of total column abundances of CO2 and CH4 with CO (XCO) to further characterize the associated enhancements in the column abundance of the respective species by taking advantage of their temporal covariations. A total of 11 sites from the Total Carbon Column Observing Network (TCCON), including 6 stations in the NH and 5 in the SH, are selected to investigate associated multi-species patterns during the 2012 to 2019 period. We also introduce a combination of established regression and anomaly approaches to derive mean local and bulk enhancement ratios between XCO , XCO . and across each month of daily data. We first derive bulk enhancement ratios (BERr) using three regression algorithms (ordinary least squares, geometric regression, and York regression), where we report the BERr as the mean across these algorithms weighted by the associated errors. We also employ a local anomaly approach, where observed column abundances are pre-subtracted by assumed background values. These background values are derived as the mean of (a) daily anomalies calculated by subtracting the morning from afternoon columns and (b) the 5th percentile of daily data. The enhancement ratios based on anomalies are derived either from monthly mean ratios (AERa) or regressed slope (AERr) between these anomalies. This combination of approaches allows us to not only account for the variability in our estimates of enhancement ratios due in fact from the algorithms and assumptions of background values, but also to separate the regional and local influences on these ratios by subtracting BERr (bulk or global) from AERr or AERr (local) estimates.
Our results show that (a) estimates of enhancement ratios are within the range of ratio estimates reported in the literature, (b) regional and local influences on these ratios can be disentangled with resulting values that appear to be physically reasonable relative to the current understanding of process drivers at these site locations, and (c) multi-species analysis of these enhancement ratios can augment current techniques aimed at characterizing dominant types of sources and sinks influencing observed abundances. We find that Pasadena (Wollongong, Manaus) shows a dominant (moderate) local influence (> 60 % in Pasadena, > 50 % in Wollongong and Manaus) across CO, CH4, and CO2 which appears to come from a mixture of biospheric and combustion activities. In contrast, Anmyeondo shows a dominant regional influence (≳ 60 %) across all species, which appears to come from high-temperature and/or biofuel combustion activities. A comparable influence of regional and local enhancement is observed in Darwin (biospheric and/or low-temperature combustion) for all species. Interestingly, Sodankylä and Garmisch (mostly biospheric and wetlands), Hefei (low-temperature combustion), and Burgos (biofuel combustion) are characterized by a larger regional influence (∼ 67 for Garmisch, ∼ 70 % for Sodankylä, ∼ 73 % for Hefei, and 86 % for Burgos) in XCO and relatively comparable regional and local influences in and XCO . On the other hand, Ascension shows a large regional influence (> 80 %) for both XCO and XCO , indicative of fire activities (high CO). While Ascension is characterized as relatively remote with little local influence in column CO, it appears to show the impact of long-range-transported emissions (most likely fires). Note that total column CO (XCO) can capture this fire signature as opposed to several reports over Ascension which have indicated that fire plumes from southern Africa cannot be observed from ground-based sites on the island. A similar finding is observed for Réunion (albeit not as large a regional influence, ∼ 75 % in XCO and ∼ 58 % in XCO ). As with Ascension, Réunion is an isolated island and characterized as remote but with a large presence of combustion (fire) influence as it receives higher quantities of smoke outflows from African fires on its west side, sometimes even with South American fires. These results are qualitatively consistent with corresponding estimates from CAMS and GFED emission inventories. We do, however, want to note that additional work is needed for a more robust estimation of the BERr and the regional enhancement, considering the high variability observed across the species in BERr (as in Fig. 3), which consequently leads to higher variability in the regional enhancement estimates.
This work is envisioned to serve as one of the bases for interpreting enhancement ratios derived from current spaceborne co-located column measurements of CO, CO2, and/or CH4 (e.g., TROPOMI, GOSAT-2, OCO-2, and OCO-3). The method presented here can also be applied to future geostationary satellites with high-temporal-resolution measurements. Our method provides a preliminary framework towards the evaluation of the enhancement ratios (i.e., species sensitivities) along with the abundances derived from these satellite missions to reduce the discrepancies between the top-down and bottom-up inversions and emission-based studies, as well as to provide more robust source-type attribution of these abundances that otherwise is difficult to obtain by single-species analysis alone. The use of enhancement ratios and their separation into regional and local influence allows us to effectively disentangle the source-type and transport signatures of these species over the sites, unlike the correlation estimates in Table 2 which do not provide a complete picture considering the diffused (non-linear) behavior of their sources and sinks. Separating the contributions of megacity emissions from fire and biogenic sources is a future application of this study. Use of data-driven machine learning regression algorithms can also assist in inferring the contribution from different emission sources. Including additional sites and a longer time period from the newer software version of the TCCON data (GGG2020) will also aid in constraining the uncertainty of the regional versus local enhancements, as well as the source/transport signatures inferred in this study.
The TCCON data were obtained from the TCCON Data Archive, hosted by CaltechDATA at https://tccondata.org/2014 (last access: 6 June 2023). The DOI for the entire 2014 dataset is https://doi.org/10.14291/TCCON.GGG2014 (Total Carbon Column Observing Network (TCCON) Team, 2017). The DOIs for each site are provided in Table 1. Supporting datasets were obtained from the following: Emissions of atmospheric Compounds and Compilation of the Ancillary Data (ECCAD, 2023, https://eccad.sedoo.fr/) for CAMS v4.1 and GFED4, MOPITT from NASA through the Earthdata portal at https://doi.org/10.5067/TERRA/MOPITT/MOP03JM_L3.008 (NASA/LARC/SD/ASDC, 2000), GOSAT-1 from NIES at https://data2.gosat.nies.go.jp (NIES, 2023), and OCO-2 from NASA through the Goddard Earth Science Data and Information Services Center for registered users.
The supplement related to this article is available online at: https://doi.org/10.5194/amt-17-5861-2024-supplement.
Conceptualization: AFA Jr., WT; investigation: KM, VB, CR, and AFA Jr.; methodology: KM, VB, AFA Jr., CR; formal analysis: KM, VB, AFA Jr., CR; data curation: KM, CR, DWTG, DGF, IM, MKS, MKD, MDM, NMD, POW, RS, RK, TYG, VAV, WW; validation: KM, CR; visualization: KM, CR; supervision: AFA Jr.; writing (original draft preparation): KM, AFA Jr.; writing (review and editing): BG, WT, CR, MAM, JM, YG, MKS, VAV, and AFA Jr.
At least one of the (co-)authors is a member of the editorial board of Atmospheric Measurement Techniques. The peer-review process was guided by an independent editor, and the authors also have no other competing interests to declare.
Publisher's note: Copernicus Publications remains neutral with regard to jurisdictional claims made in the text, published maps, institutional affiliations, or any other geographical representation in this paper. While Copernicus Publications makes every effort to include appropriate place names, the final responsibility lies with the authors.
This research work is supported by NASA ACMAP (grant no. 80NSSC19K0947). Wenfu Tang is supported by the National Center for Atmospheric Research (NCAR) Advanced Study Program Postdoctoral Fellowship. The TCCON data for total column measurements of CO, CO2, and CH4 at Pasadena, Ascension, Manaus, Garmisch, Sodankylä, Anmyeondo, Burgos, Hefei, Darwin, Wollongong, and Réunion were obtained from the TCCON Data Archive (GGG 2014), hosted by CaltechDATA at https://tccondata.org. The Ascension Island TCCON station has been supported by the European Space Agency (ESA) under grant no. 4000120088/17/I-EF and by the German Bundesministerium für Wirtschaft und Energie (BMWi) under grant nos. 50EE1711C and 50EE1711E. We thank the ESA Ariane Tracking Station at North East Bay, Ascension Island, for hosting and local support. The TCCON site at Réunion has been operated by the Royal Belgian Institute for Space Aeronomy with financial support since 2014 by the EU project ICOS-Inwire (grant no. ID 313169), the ministerial decree for ICOS (FR/35/IC1 to FR/35/C6), ESFRI-FED ICOS-BE project (EF/211/ICOS-BE), and local activities supported by LACy/UMR8105 and by OSU-R/UMS3365 – Université de La Réunion. The Burgos TCCON site is supported in part by the GOSAT series project, with local support from the Energy Development Corp., Philippines. We also acknowledge the Emission of Atmospheric Compounds and Compilation of the Ancillary Data (ECCAD, https://eccad.sedoo.fr) for anthropogenic and biomass burning emission data of CO, CO2, and CH4 from the inventories of Copernicus Atmosphere Monitoring Service (CAMS v4.1) and Global Fire Emission Database (GFED4) during the 2012–2019 period. This material is partly based upon work supported by the US NSF NCAR, which is a major facility sponsored by the National Science Foundation under cooperative agreement no. 1852977.
This research has been supported by the National Aeronautics and Space Administration (grant no. 80NSSC19K0947).
This paper was edited by Abhishek Chatterjee and reviewed by two anonymous referees.
Agudelo-Vera, C. M., Leduc, W. R. W. A., Mels, A. R., and Rijnaarts, H. H. M.: Harvesting Urban Resources towards More Resilient Cities, Resour. Conserv. Recycl., 64, 3–12, https://doi.org/10.1016/j.resconrec.2012.01.014, 2012.
Ammoura, L., Xueref-Remy, I., Gros, V., Baudic, A., Bonsang, B., Petit, J.-E., Perrussel, O., Bonnaire, N., Sciare, J., and Chevallier, F.: Atmospheric measurements of ratios between CO2 and co-emitted species from traffic: a tunnel study in the Paris megacity, Atmos. Chem. Phys., 14, 12871–12882, https://doi.org/10.5194/acp-14-12871-2014, 2014.
Andela, N., Morton, D. C., Giglio, L., Chen, Y., van der Werf, G. R., Kasibhatla, P. S., DeFries, R. S., Collatz, G. J., Hantson, S., Kloster, S., Bachelet, D., Forrest, M., Lasslop, G., Li, F., Mangeon, S., Melton, J. R., Yue, C., and Randerson, J. T.: A human-driven decline in global burned area, Science, 356, 1356–1362, https://doi.org/10.1126/science.aal4108, 2017.
Anderson, D. C., Loughner, C. P., Diskin, G., Weinheimer, A., Canty, T. P., Salawitch, R. J., Worden, H. M., Fried, A., Mikoviny T., Wisthaler A., and Dickerson R. R.: Measured and modeled CO and NOy in DISCOVER-AQ: An evaluation of emissions and chemistry over the eastern US, Atmos. Environ., 96, 78–87, https://doi.org/10.1016/j.atmosenv.2014.07.004, 2014.
Andreae, M. O.: Emission of trace gases and aerosols from biomass burning – an updated assessment, Atmos. Chem. Phys., 19, 8523–8546, https://doi.org/10.5194/acp-19-8523-2019, 2019.
Andreae, M. O. and Merlet, P.: Emission of trace gases and aerosols from biomass burning, Global Biogeochem. Cy., 15, 955–966, https://doi.org/10.1029/2000GB001382, 2001.
Andreae, M. O., Browell, E. V., Garstang, M., Gregory, G. L., Harriss, R. C., Hill, G. F., Jacob, D. J., Pereira, M. C., Sachse, G. W., Setzer, A. W., Silva Dias, P. L., Talbot, R. W., Torres, A. L., and Wofsy, S. C.: Biomass-burning emissions and associated haze layers over Amazonia, J. Geophys. Res.-Atmos., 93, 1509–1527, https://doi.org/10.1029/JD093iD02p01509, 1988.
Arioli, M. S., D'Agosto, M. A., Amaral, F. G., and Cybis, H. B. B.: The evolution of city-scale GHG emissions inventory methods: A systematic review, Environ. Impact Assess. Rev., 80, 106316, https://doi.org/10.1016/j.eiar.2019.106316, 2020.
Arneth, A., Sitch, S., Pongratz, J., Stocker, B. D., Ciais, P., Poulter, B., Bayer, A. D., Bondeau, A., Calle, L., Chini, L. P., Gasser, T., Fader, M., Friedlingstein, P., Kato, E., Li, W., Lindeskog, M., Nabel, J. E. M. S., Pugh, T. A. M., Robertson, E., Viovy, N., Yue, C., and Zaehle, S.: Historical carbon dioxide emissions caused by land-use changes are possibly larger than assumed, Nat. Geosci., 10, 79–84, https://doi.org/10.1038/ngeo2882, 2017.
Bai, X., Dawson, R. J., Ürge-Vorsatz, D., Delgado, G. C., Barau, A. S., Dhakal, S., Dodman, D., Leonardsen, L., Masson-Delmotte, V., Roberts, D. C., and Schultz, S.: Six research priorities for cities and climate change, Nature, 555, 23–25, https://doi.org/10.1038/d41586-018-02409-z, 2018.
Baiocchi, G., Creutzig, F., Minx, J., and Pichler, P.-P.: A spatial typology of human settlements and their CO2 emissions in England, Glob. Environ. Change, 34, 13–21, https://doi.org/10.1016/j.gloenvcha.2015.06.001, 2015.
Bakwin, P. S., Tans, P. P., Zhao, C., Ussler III, W., and Quesnell, E.: Measurements of carbon dioxide on a very tall tower, Tellus B, 47, 535–549, https://doi.org/10.1034/j.1600-0889.47.issue5.2.x, 1995.
Banerjee, R., Inamdar, A. B., Phulluke, S., and Pateriya, B.: Decision support system for energy planning in a district: Residential module, Econ. Polit. Wkly., 34, 3545–3552, 1999.
Bares, R., Lin, J. C., Hoch, S. W., Baasandorj, M., Mendoza, D. L., Fasoli, B., Mitchell, L., Catharine, D., and Stephens, B. B.: The wintertime covariation of CO2 and criteria pollutants in an urban valley of the western United States, J. Geophys. Res.-Atmos., 123, 2684–2703, https://doi.org/10.1002/2017JD027917, 2018.
Bartholomew, G. W. and Alexander, M.: Soil as a sink for atmospheric carbon monoxide, Science, 212, 1389–1391, https://doi.org/10.1126/science.212.4501.1389, 1981.
Bettencourt, L. M. A., Lobo, J., Helbing, D., Kühnert, C., and West, G. B.: Growth, innovation, scaling, and the pace of life in cities, P. Natl. Acad. Sci. USA, 104, 7301–7306, https://doi.org/10.1073/pnas.0610172104, 2007.
Borsdorff, T., aan de Brugh, J., Hu, H., Hasekamp, O., Sussmann, R., Rettinger, M., Hase, F., Gross, J., Schneider, M., Garcia, O., Stremme, W., Grutter, M., Feist, D. G., Arnold, S. G., De Mazière, M., Kumar Sha, M., Pollard, D. F., Kiel, M., Roehl, C., Wennberg, P. O., Toon, G. C., and Landgraf, J.: Mapping carbon monoxide pollution from space down to city scales with daily global coverage, Atmos. Meas. Tech., 11, 5507–5518, https://doi.org/10.5194/amt-11-5507-2018, 2018.
Bozhinova, D., van der Molen, M. K., van der Velde, I. R., Krol, M. C., van der Laan, S., Meijer, H. A. J., and Peters, W.: Simulating the integrated summertime Δ14CO2 signature from anthropogenic emissions over Western Europe, Atmos. Chem. Phys., 14, 7273–7290, https://doi.org/10.5194/acp-14-7273-2014, 2014.
Bremer, H., Kar, J., Drummond, J. R., Nichitu, F., Zou, J., Liu, J., Gille, J. C., Deeter, M. N., Francis, G., Ziskin, D., and Warner, J.: Carbon monoxide from biomass burning in the tropics and its impact on the tropospheric ozone, J. Geophys. Res.-Atmos., 109, D12304, https://doi.org/10.1029/2003JD004234, 2004.
Briggs, N. L., Jaffe, D. A., Gao, H., Hee, J. R., Baylon, P. M., Zhang, Q., Zhou, S., Collier, S. C., Sampson, P. D., and Cary, R. A.: Particulate matter, ozone, and nitrogen species in aged wildfire plumes observed at the Mount Bachelor Observatory, Aerosol Air Qual. Res., 16, 3075–3087, https://doi.org/10.4209/aaqr.2016.03.0120, 2016.
Buchholz, R. R., Paton-Walsh, C., Griffith, D. W. T., Kubistin, D., Caldow, C., Fisher, J. A., Deutscher, N. M., Kettlewell, G., Riggenbach, M., Macatangay, R., Krummel, P. B., and Langenfelds, R. L.: Source and meteorological influences on air quality (CO, CH4 & CO2) at a southern hemisphere urban site, Atmos. Environ., 126, 274–289, https://doi.org/10.1016/j.atmosenv.2015.11.041, 2016.
Buchholz, R. R., Worden, H. M., Park, M., Francis, G., Deeter, M. N., Edwards, D. P., Emmons, L. K., Gaubert, B., Gille, J., Martínez-Alonso, S., Tang, W., Kumar, R., Drummond, J. R., Clerbaux, C., George, M., Coheur, P.-F., Hurtmans, D., Bowman, K. W., Luo, M., Payne, V. H., Worden, J. R., Chin, M., Levy, R. C., Warner, J., Wei, Z., and Kulawik, S. S.: Air pollution trends measured from Terra: CO and AOD over industrial, fire-prone, and background regions, Remote Sens. Environ., 256, 112275, https://doi.org/10.1016/j.rse.2020.112275, 2021.
Bukosa, B., Deutscher, N. M., Fisher, J. A., Kubistin, D., Paton-Walsh, C., and Griffith, D. W. T.: Simultaneous shipborne measurements of CO2, CH4 and CO and their application to improving greenhouse-gas flux estimates in Australia, Atmos. Chem. Phys., 19, 7055–7072, https://doi.org/10.5194/acp-19-7055-2019, 2019.
Chandra, N., Patra, P. K., Bisht, J. S. H., Ito, A., Umezawa, T., Saigusa, N., Morimoto, S., Aoki, S., Janssens-Maenhout, G., Fujita, R., Takigawa, M., Watanabe, S., Saitoh, N., and Canadell, J. G.: Emissions from the oil and gas sectors, coal mining and ruminant farming drive methane growth over the past three decades, J. Meteorol. Soc. Jpn. Ser. II, 99, 309–337, https://doi.org/10.2151/jmsj.2021-015, 2021.
Chatfield, R. B., Andreae, M. O., ARCTAS Science Team, and SEAC4RS Science Team: Emissions relationships in western forest fire plumes – Part 1: Reducing the effect of mixing errors on emission factors, Atmos. Meas. Tech., 13, 7069–7096, https://doi.org/10.5194/amt-13-7069-2020, 2020.
Cheng, Y., Wang, Y., Zhang, Y., Chen, G., Crawford, J. H., Kleb, M. M., Diskin, G. S., and Weinheimer, A. J.: Large biogenic contribution to boundary layer O3-CO regression slope in summer, Geophys. Res. Lett., 44, 7061–7068, https://doi.org/10.1002/2017GL074405, 2017.
Chevallier, F., Remaud, M., O'Dell, C. W., Baker, D., Peylin, P., and Cozic, A.: Objective evaluation of surface- and satellite-driven carbon dioxide atmospheric inversions, Atmos. Chem. Phys., 19, 14233–14251, https://doi.org/10.5194/acp-19-14233-2019, 2019.
Ciais, P., Tan, J., Wang, X., Roedenbeck, C., Chevallier, F., Piao, S.-L., Moriarty, R., Broquet, G., Le Quéré, C., Canadell, J. G., Peng, S., Poulter, B., Liu, Z., and Tans, P.: Five decades of northern land carbon uptake revealed by the interhemispheric CO2 gradient, Nature, 568, 221–225, https://doi.org/10.1038/s41586-019-1078-6, 2019.
Cordero, P. R. F., Bayly, K., Leung, P. M., Huang, C., Islam, Z. F., Schittenhelm, R. B., King, G. M., and Greening, C.: Atmospheric carbon monoxide oxidation is a widespread mechanism supporting microbial survival, ISME J., 13, 2868–2881, https://doi.org/10.1038/s41396-019-0479-8, 2019.
Creutzig, F., Baiocchi, G., Bierkandt, R., Pichler, P.-P., and Seto, K. C.: Global typology of urban energy use and potentials for an urbanization mitigation wedge, P. Natl. Acad. Sci. USA, 112, 6283–6288, https://doi.org/10.1073/pnas.1315545112, 2015.
Creutzig, F., Lohrey, S., Bai, X., Baklanov, A., Dawson, R., Dhakal, S., Lamb, W. F., McPhearson, T., Minx, J., Munoz, E., and Walsh, B.: Upscaling urban data science for global climate solutions, Global Sustain., 2, e2, https://doi.org/10.1017/sus.2018.16, 2019.
Crowell, S., Baker, D., Schuh, A., Basu, S., Jacobson, A. R., Chevallier, F., Liu, J., Deng, F., Feng, L., McKain, K., Chatterjee, A., Miller, J. B., Stephens, B. B., Eldering, A., Crisp, D., Schimel, D., Nassar, R., O'Dell, C. W., Oda, T., Sweeney, C., Palmer, P. I., and Jones, D. B. A.: The 2015–2016 carbon cycle as seen from OCO-2 and the global in situ network, Atmos. Chem. Phys., 19, 9797–9831, https://doi.org/10.5194/acp-19-9797-2019, 2019.
De Mazière, M., Sha, M. K., Desmet, F., Hermans, C., Scolas, F., Kumps, N., Metzger, J.-M., Duflot, V., and Cammas, J.-P.: TCCON data from Réunion Island (RE), Release GGG2014.R1 (Version R1), CaltechDATA [data set], https://doi.org/10.14291/TCCON.GGG2014.REUNION01.R1, 2017.
Deutscher, N. M., Griffith, D. W. T., Bryant, G. W., Wennberg, P. O., Toon, G. C., Washenfelder, R. A., Keppel-Aleks, G., Wunch, D., Yavin, Y., Allen, N. T., Blavier, J.-F., Jiménez, R., Daube, B. C., Bright, A. V., Matross, D. M., Wofsy, S. C., and Park, S.: Total column CO2 measurements at Darwin, Australia – site description and calibration against in situ aircraft profiles, Atmos. Meas. Tech., 3, 947–958, https://doi.org/10.5194/amt-3-947-2010, 2010.
Djuricin, S., Pataki, D. E., and Xu, X.: A comparison of tracer methods for quantifying CO2 sources in an urban region, J. Geophys. Res.-Atmos., 115, D11303, https://doi.org/10.1029/2009JD012236, 2010.
Dodman, D.: Blaming cities for climate change? An analysis of urban greenhouse gas emissions inventories, Environ. Urban., 21, 185–201, https://doi.org/10.1177/0956247809103016, 2009.
Dowdy, A. J.: Climatological Variability of Fire Weather in Australia, J. Appl. Meteorol. Clim., 57, 221–234, https://doi.org/10.1175/JAMC-D-17-0167.1, 2018.
Dubey, M. K., Henderson, B. G., Green, D., Butterfield, Z. T., Keppel-Aleks, G., Allen, N. T., Blavier, J.-F., Roehl, C. M., Wunch, D., and Lindenmaier, R.: TCCON data from Manaus (BR), release GGG2014.R0, CaltechDATA [data set], https://doi.org/10.14291/TCCON.GGG2014.MANAUS01.R0/1149274, 2014.
ECCAD: Emissions of atmospheric Compounds and Compilation of Ancillary Data, https://eccad.sedoo.fr/, last access: 6 June 2023.
Edwards, E.-L., Reid, J. S., Xian, P., Burton, S. P., Cook, A. L., Crosbie, E. C., Fenn, M. A., Ferrare, R. A., Freeman, S. W., Hair, J. W., Harper, D. B., Hostetler, C. A., Robinson, C. E., Scarino, A. J., Shook, M. A., Sokolowsky, G. A., van den Heever, S. C., Winstead, E. L., Woods, S., Ziemba, L. D., and Sorooshian, A.: Assessment of NAAPS-RA performance in Maritime Southeast Asia during CAMP2Ex, Atmos. Chem. Phys., 22, 12961–12983, https://doi.org/10.5194/acp-22-12961-2022, 2022.
Erickson, P. and Morgenstern, T.: Fixing greenhouse gas accounting at the city scale, Carbon Manag., 7, 313–316, https://doi.org/10.1080/17583004.2016.1238743, 2016.
Feist, D. G., Arnold, S. G., John, N., and Geibel, M. C.: TCCON data from Ascension Island (SH), Release GGG2014.R0 (GGG2014.R0), CaltechDATA [data set], https://doi.org/10.14291/TCCON.GGG2014.ASCENSION01.R0/1149285, 2017.
Fisher, J. A., Wilson, S. R., Zeng, G., Williams, J. E., Emmons, L. K., Langenfelds, R. L., Krummel, P. B., and Steele, L. P.: Seasonal changes in the tropospheric carbon monoxide profile over the remote Southern Hemisphere evaluated using multi-model simulations and aircraft observations, Atmos. Chem. Phys., 15, 3217–3239, https://doi.org/10.5194/acp-15-3217-2015, 2015.
Frankenberg, C., Pollock, R., Lee, R. A. M., Rosenberg, R., Blavier, J.-F., Crisp, D., O'Dell, C. W., Osterman, G. B., Roehl, C., Wennberg, P. O., and Wunch, D.: The Orbiting Carbon Observatory (OCO-2): spectrometer performance evaluation using pre-launch direct sun measurements, Atmos. Meas. Tech., 8, 301–313, https://doi.org/10.5194/amt-8-301-2015, 2015.
Friedlingstein, P., Jones, M. W., O'Sullivan, M., Andrew, R. M., Bakker, D. C. E., Hauck, J., Le Quéré, C., Peters, G. P., Peters, W., Pongratz, J., Sitch, S., Canadell, J. G., Ciais, P., Jackson, R. B., Alin, S. R., Anthoni, P., Bates, N. R., Becker, M., Bellouin, N., Bopp, L., Chau, T. T. T., Chevallier, F., Chini, L. P., Cronin, M., Currie, K. I., Decharme, B., Djeutchouang, L. M., Dou, X., Evans, W., Feely, R. A., Feng, L., Gasser, T., Gilfillan, D., Gkritzalis, T., Grassi, G., Gregor, L., Gruber, N., Gürses, Ö., Harris, I., Houghton, R. A., Hurtt, G. C., Iida, Y., Ilyina, T., Luijkx, I. T., Jain, A., Jones, S. D., Kato, E., Kennedy, D., Klein Goldewijk, K., Knauer, J., Korsbakken, J. I., Körtzinger, A., Landschützer, P., Lauvset, S. K., Lefèvre, N., Lienert, S., Liu, J., Marland, G., McGuire, P. C., Melton, J. R., Munro, D. R., Nabel, J. E. M. S., Nakaoka, S.-I., Niwa, Y., Ono, T., Pierrot, D., Poulter, B., Rehder, G., Resplandy, L., Robertson, E., Rödenbeck, C., Rosan, T. M., Schwinger, J., Schwingshackl, C., Séférian, R., Sutton, A. J., Sweeney, C., Tanhua, T., Tans, P. P., Tian, H., Tilbrook, B., Tubiello, F., van der Werf, G. R., Vuichard, N., Wada, C., Wanninkhof, R., Watson, A. J., Willis, D., Wiltshire, A. J., Yuan, W., Yue, C., Yue, X., Zaehle, S., and Zeng, J.: Global Carbon Budget 2021, Earth Syst. Sci. Data, 14, 1917–2005, https://doi.org/10.5194/essd-14-1917-2022, 2022.
Gately, C. K. and Hutyra, L. R.: Large Uncertainties in Urban-Scale Carbon Emissions, J. Geophys. Res.-Atmos., 122, 11242–11260, https://doi.org/10.1002/2017JD027359, 2017.
Gaubert, B., Arellano, A. F. Jr., Barré, J., Worden, H. M., Emmons, L. K., Tilmes, S., Buchholz, R. R., Vitt, F., Raeder, K., Collins, N., Anderson, J. L., Wiedinmyer, C., Martinez Alonso, S., Edwards, D. P., Andreae, M. O., Hannigan, J. W., Petri, C., Strong, K., and Jones, N.: Toward a Chemical Reanalysis in a Coupled Chemistry-Climate Model: An Evaluation of MOPITT CO Assimilation and Its Impact on Tropospheric Composition, J. Geophys. Res.-Atmos., 121, 7310–7343, https://doi.org/10.1002/2016JD024863, 2016.
Gaubert, B., Worden, H. M., Arellano, A. F. J., Emmons, L. K., Tilmes, S., Barré, J., Martinez Alonso, S., Vitt, F., Anderson, J. L., Alkemade, F., Houweling, S., and Edwards, D. P.: Chemical Feedback From Decreasing Carbon Monoxide Emissions, Geophys. Res. Lett., 44, 9985–9995, https://doi.org/10.1002/2017GL074987, 2017.
Gaubert, B., Stephens, B. B., Basu, S., Chevallier, F., Deng, F., Kort, E. A., Patra, P. K., Peters, W., Rödenbeck, C., Saeki, T., Schimel, D., Van der Laan-Luijkx, I., Wofsy, S., and Yin, Y.: Global atmospheric CO2 inverse models converging on neutral tropical land exchange, but disagreeing on fossil fuel and atmospheric growth rate, Biogeosciences, 16, 117–134, https://doi.org/10.5194/bg-16-117-2019, 2019.
Geibel, M. C., Gerbig, C., and Feist, D. G.: A new fully automated FTIR system for total column measurements of greenhouse gases, Atmos. Meas. Tech., 3, 1363–1375, https://doi.org/10.5194/amt-3-1363-2010, 2010.
Giglio, L., Randerson, J. T., and van der Werf, G. R.: Analysis of Daily, Monthly, and Annual Burned Area Using the Fourth-Generation Global Fire Emissions Database (GFED4), J. Geophys. Res.-Biogeo., 118, 317–328, https://doi.org/10.1002/jgrg.20042, 2013.
Goo, T.-Y., Oh, Y.-S., and Velazco, V. A.: TCCON Data from Anmeyondo (KR), Release GGG2014.R0, CaltechDATA [data set], https://doi.org/10.14291/TCCON.GGG2014.ANMEYONDO01.R0/1149284, 2014.
Granier, C., Darras, S., Denier van der Gon, H., Doubalova, J., Elguindi, N., Galle, B., Gauss, M., Guevara, M., Jalkanen, J.-P., Kuenen, J., Liousse, C., Quack, B., Simpson, D., and Sindelarova, K.: The Copernicus Atmosphere Monitoring Service Global and Regional Emissions (April 2019 Version), https://doi.org/10.24380/D0BN-KX16, 2019.
Griffith, D. W. T., Deutscher, N. M., Velazco, V. A., Wennberg, P. O., Yavin, Y., Keppel-Aleks, G., Washenfelder, R. A., Toon, G. C., Blavier, J.-F., Paton-Walsh, C., Jones, N. B., Kettlewell, G. C., Connor, B. J., Macatangay, R. C., Roehl, C., Ryczek, M., Glowacki, J., Culgan, T., and Bryant, G. W.: TCCON Data from Darwin (AU), Release GGG2014.R0, CaltechDATA [data set], https://doi.org/10.14291/TCCON.GGG2014.DARWIN01.R0/1149290, 2014a.
Griffith, D. W. T., Velazco, V. A., Deutscher, N. M., Paton-Walsh, C., Jones, N. B., Wilson, S. R., Macatangay, R. C., Kettlewell, G. C., Buchholz, R. R., and Riggenbach, M. O.: TCCON Data from Wollongong (AU), Release GGG2014.R0, CaltechDATA [data set], https://doi.org/10.14291/TCCON.GGG2014.WOLLONGONG01.R0/1149291, 2014b.
Grimm, N. B., Faeth, S. H., Golubiewski, N. E., Redman, C. L., Wu, J., Bai, X., and Briggs, J. M.: Global Change and the Ecology of Cities, Science, 319, 756–760, https://doi.org/10.1126/science.1150195, 2008.
Gurney, K. R., Chen, Y.-H., Maki, T., Kawa, S. R., Andrews, A., and Zhu, Z.: Sensitivity of Atmospheric CO2 Inversions to Seasonal and Interannual Variations in Fossil Fuel Emissions, J. Geophys. Res.-Atmos., 110, D10308, https://doi.org/10.1029/2004JD005373, 2005.
Gurney, K. R., Song, Y., Liang, J., and Roest, G.: Toward Accurate, Policy-Relevant Fossil Fuel CO2 Emission Landscapes, Environ. Sci. Technol., 54, 9896–9907, https://doi.org/10.1021/acs.est.0c01175, 2020.
Guthrie, P. D.: The CH4 – CO – OH Conundrum: A Simple Analytic Approach, Global Biogeochem. Cy., 3, 287–298, https://doi.org/10.1029/GB003i004p00287, 1989.
Guyon, P., Frank, G. P., Welling, M., Chand, D., Artaxo, P., Rizzo, L., Nishioka, G., Kolle, O., Fritsch, H., Silva Dias, M. A. F., Gatti, L. V., Cordova, A. M., and Andreae, M. O.: Airborne measurements of trace gas and aerosol particle emissions from biomass burning in Amazonia, Atmos. Chem. Phys., 5, 2989–3002, https://doi.org/10.5194/acp-5-2989-2005, 2005.
Halliday, H. S., DiGangi, J. P., Choi, Y., Diskin, G. S., Pusede, S. E., Rana, M., Nowak, J. B., Knote, C., Ren, X., He, H., Dickerson, R. R., and Li, Z.: Using Short-Term CO CO2 Ratios to Assess Air Mass Differences Over the Korean Peninsula During KORUS-AQ, J. Geophys. Res.-Atmos., 124, 10951–10972, https://doi.org/10.1029/2018JD029697, 2019.
Hedelius, J. K., Liu, J., Oda, T., Maksyutov, S., Roehl, C. M., Iraci, L. T., Podolske, J. R., Hillyard, P. W., Liang, J., Gurney, K. R., Wunch, D., and Wennberg, P. O.: Southern California megacity CO2, CH4, and CO flux estimates using ground- and space-based remote sensing and a Lagrangian model, Atmos. Chem. Phys., 18, 16271–16291, https://doi.org/10.5194/acp-18-16271-2018, 2018.
Hickman, J. E., Andela, N., Tsigaridis, K., Galy-Lacaux, C., Ossohou, M., and Bauer, S. E.: Reductions in NO2 Burden over North Equatorial Africa from Decline in Biomass Burning in Spite of Growing Fossil Fuel Use, 2005 to 2017, P. Natl. Acad. Sci. USA, 118, e2002579118, https://doi.org/10.1073/pnas.2002579118, 2021.
Hilario, M. R. A., Crosbie, E., Shook, M., Reid, J. S., Cambaliza, M. O. L., Simpas, J. B. B., Ziemba, L., DiGangi, J. P., Diskin, G. S., Nguyen, P., Turk, F. J., Winstead, E., Robinson, C. E., Wang, J., Zhang, J., Wang, Y., Yoon, S., Flynn, J., Alvarez, S. L., Behrangi, A., and Sorooshian, A.: Measurement report: Long-range transport patterns into the tropical northwest Pacific during the CAMP2Ex aircraft campaign: chemical composition, size distributions, and the impact of convection, Atmos. Chem. Phys., 21, 3777–3802, https://doi.org/10.5194/acp-21-3777-2021, 2021.
Hobbs, P. V., Sinha, P., Yokelson, R. J., Christian, T. J., Blake, D. R., Gao, S., Kirchstetter, T. W., Novakov, T., and Pilewskie, P.: Evolution of Gases and Particles from a Savanna Fire in South Africa, J. Geophys. Res.-Atmos., 108, 8485, https://doi.org/10.1029/2002JD002352, 2003.
Hoesly, R. M., Smith, S. J., Feng, L., Klimont, Z., Janssens-Maenhout, G., Pitkanen, T., Seibert, J. J., Vu, L., Andres, R. J., Bolt, R. M., Bond, T. C., Dawidowski, L., Kholod, N., Kurokawa, J.-I., Li, M., Liu, L., Lu, Z., Moura, M. C. P., O'Rourke, P. R., and Zhang, Q.: Historical (1750–2014) anthropogenic emissions of reactive gases and aerosols from the Community Emissions Data System (CEDS), Geosci. Model Dev., 11, 369–408, https://doi.org/10.5194/gmd-11-369-2018, 2018.
Houweling, S., Baker, D., Basu, S., Boesch, H., Butz, A., Chevallier, F., Deng, F., Dlugokencky, E. J., Feng, L., Ganshin, A., Hasekamp, O., Jones, D., Maksyutov, S., Marshall, J., Oda, T., O'Dell, C. W., Oshchepkov, S., Palmer, P. I., Peylin, P., Poussi, Z., Reum, F., Takagi, H., Yoshida, Y., and Zhuravlev, R.: An Intercomparison of Inverse Models for Estimating Sources and Sinks of CO2 Using GOSAT Measurements, J. Geophys. Res.-Atmos., 120, 5253–5266, https://doi.org/10.1002/2014JD022962, 2015.
Houweling, S., Bergamaschi, P., Chevallier, F., Heimann, M., Kaminski, T., Krol, M., Michalak, A. M., and Patra, P.: Global inverse modeling of CH4 sources and sinks: an overview of methods, Atmos. Chem. Phys., 17, 235–256, https://doi.org/10.5194/acp-17-235-2017, 2017.
Hutyra, L. R., Duren, R., Gurney, K. R., Grimm, N., Kort, E. A., Larson, E., and Shrestha, G.: Urbanization and the Carbon Cycle: Current Capabilities and Research Outlook from the Natural Sciences Perspective, Earths Future, 2, 473–495, https://doi.org/10.1002/2014EF000255, 2014.
Kennedy, C., Steinberger, J., Gasson, B., Hansen, Y., Hillman, T., Havránek, M., Pataki, D., Phdungsilp, A., Ramaswami, A., and Villalba Mendez, G.: Greenhouse Gas Emissions from Global Cities, Environ. Sci. Technol., 43, 7297–7302, https://doi.org/10.1021/es900213p, 2009.
Kennedy, C. A., Stewart, I., Facchini, A., Cersosimo, I., Mele, R., Chen, B., Uda, M., Kansal, A., Chiu, A., Kim, K.-G., Dubeux, C., Lebre La Rovere, E., Cunha, B., Pincetl, S., Keirstead, J., Barles, S., Pusaka, S., Gunawan, J., Adegbile, M., Nazariha, M., Hoque, S., Marcotullio, P. J., González Otharán, F., Genena, T., Ibrahim, N., Farooqui, R., Cervantes, G., and Sahin, A. D.: Energy and Material Flows of Megacities, P. Natl. Acad. Sci. USA, 112, 5985–5990, https://doi.org/10.1073/pnas.1504315112, 2015.
Khalil, M. A. K. and Rasmussen, R. A.: The Global Cycle of Carbon Monoxide: Trends and Mass Balance, Chemosphere, 20, 227–242, https://doi.org/10.1016/0045-6535(90)90098-E, 1990.
Kivi, R., Heikkinen, P., and Kyro, E.: TCCON data from Sodankylä (FI), Release GGG2014.R0 (GGG2014.R0), CaltechDATA [data set], https://doi.org/10.14291/TCCON.GGG2014.SODANKYLA01.R0/1149280, 2014.
Kong, Y., Chen, B., and Measho, S.: Spatio-Temporal Consistency Evaluation of XCO2 Retrievals from GOSAT and OCO-2 Based on TCCON and Model Data for Joint Utilization in Carbon Cycle Research, Atmosphere, 10, 354, https://doi.org/10.3390/atmos10070354, 2019.
Kraskov, A., Stögbauer, H., and Grassberger, P.: Estimating Mutual Information, Phys. Rev. E, 69, 066138, https://doi.org/10.1103/PhysRevE.69.066138, 2004.
Kulawik, S., Wunch, D., O'Dell, C., Frankenberg, C., Reuter, M., Oda, T., Chevallier, F., Sherlock, V., Buchwitz, M., Osterman, G., Miller, C. E., Wennberg, P. O., Griffith, D., Morino, I., Dubey, M. K., Deutscher, N. M., Notholt, J., Hase, F., Warneke, T., Sussmann, R., Robinson, J., Strong, K., Schneider, M., De Mazière, M., Shiomi, K., Feist, D. G., Iraci, L. T., and Wolf, J.: Consistent evaluation of ACOS-GOSAT, BESD-SCIAMACHY, CarbonTracker, and MACC through comparisons to TCCON, Atmos. Meas. Tech., 9, 683–709, https://doi.org/10.5194/amt-9-683-2016, 2016.
Kumar, A., Mishra, S., Bakshi, S., Upadhyay, P., and Thakur, T. K.: Response of Eutrophication and Water Quality Drivers on Greenhouse Gas Emissions in Lakes of China: A Critical Analysis, Ecohydrology, 16, e2483, https://doi.org/10.1002/eco.2483, 2023.
Lamb, W. F., Wiedmann, T., Pongratz, J., Andrew, R., Crippa, M., Olivier, J. G. J., Wiedenhofer, D., Mattioli, G., Al Khourdajie, A., House, J., Pachauri, S., Figueroa, M., Saheb, Y., Slade, R., Hubacek, K., Sun, L., Ribeiro, S. K., Khennas, S., de la Rue du Can, S., Chapungu, L., Davis, S. J., Bashmakov, I., Dai, H., Dhakal, S., Tan, X., Geng, Y., Gu, B., and Minx, J.: A Review of Trends and Drivers of Greenhouse Gas Emissions by Sector from 1990 to 2018, Environ. Res. Lett., 16, 073005, https://doi.org/10.1088/1748-9326/abee4e, 2021.
Le Canut, P., Andreae, M. O., Harris, G. W., Wienhold, F. G., and Zenker, T.: Airborne Studies of Emissions from Savanna Fires in Southern Africa: 1. Aerosol Emissions Measured with a Laser Optical Particle Counter, J. Geophys. Res., 101, 23615–23630, https://doi.org/10.1029/95JD02610, 1996.
Lee, H., Dlugokencky, E. J., Turnbull, J. C., Lee, S., Lehman, S. J., Miller, J. B., Pétron, G., Lim, J.-S., Lee, G.-W., Lee, S.-S., and Park, Y.-S.: Observations of atmospheric 14CO2 at Anmyeondo GAW station, South Korea: implications for fossil fuel CO2 and emission ratios, Atmos. Chem. Phys., 20, 12033–12045, https://doi.org/10.5194/acp-20-12033-2020, 2020.
Lefer, B. L., Talbot, R. W., Harriss, R. H., Bradshaw, J. D., Sandholm, S. T., Olson, J. O., Sachse, G. W., Collins, J., Shipham, M. A., Blake, D. R., Klemm, K. I., Klemm, O., Gorzelska, K., and Barrick, J.: Enhancement of Acidic Gases in Biomass Burning Impacted Air Masses over Canada, J. Geophys. Res., 99, 1721–1737, https://doi.org/10.1029/93JD02091, 1994.
Lelandais, L., Xueref-Remy, I., Riandet, A., Blanc, P. E., Armengaud, A., Oppo, S., Yohia, C., Ramonet, M., and Delmotte, M.: Analysis of 5.5 years of atmospheric CO2, CH4, CO continuous observations (2014–2020) and their correlations, at the Observatoire de Haute Provence, a station of the ICOS-France national greenhouse gases observation network, Atmos. Environ., 277, 119020, https://doi.org/10.1016/j.atmosenv.2022.119020, 2022.
Lelieveld, J., Peters, W., Dentener, F. J., and Krol, M. C.: Stability of Tropospheric Hydroxyl Chemistry, J. Geophys. Res., 107, ACH 17-1–ACH 17-11, https://doi.org/10.1029/2002JD002272, 2002.
Lelieveld, J., Gromov, S., Pozzer, A., and Taraborrelli, D.: Global tropospheric hydroxyl distribution, budget and reactivity, Atmos. Chem. Phys., 16, 12477–12493, https://doi.org/10.5194/acp-16-12477-2016, 2016.
Levin, I., Kromer, B., Schmidt, M., and Sartorius, H.: A Novel Approach for Independent Budgeting of Fossil Fuel CO2 over Europe by 14CO2 Observations, Geophys. Res. Lett., 30, 2194, https://doi.org/10.1029/2003GL018477, 2003.
Levy, H.: Normal Atmosphere: Large Radical and Formaldehyde Concentrations Predicted, Science, 173, 141–143, https://doi.org/10.1126/science.173.3992.141, 1971.
Liang, A., Gong, W., Han, G., and Xiang, C.: Comparison of Satellite-Observed XCO2 from GOSAT, OCO-2, and Ground-Based TCCON, Remote Sens., 9, 1033, https://doi.org/10.3390/rs9101033, 2017.
Liu, C., Wang, W., and Sun, Y.: TCCON Data from Hefei (PRC), Release GGG2014.R0, Application/x-netcdf, CaltechDATA [data set], https://doi.org/10.14291/TCCON.GGG2014.HEFEI01.R0, 2018.
Lu, X., Jacob, D. J., Zhang, Y., Maasakkers, J. D., Sulprizio, M. P., Shen, L., Qu, Z., Scarpelli, T. R., Nesser, H., Yantosca, R. M., Sheng, J., Andrews, A., Parker, R. J., Boesch, H., Bloom, A. A., and Ma, S.: Global methane budget and trend, 2010–2017: complementarity of inverse analyses using in situ (GLOBALVIEWplus CH4 ObsPack) and satellite (GOSAT) observations, Atmos. Chem. Phys., 21, 4637–4657, https://doi.org/10.5194/acp-21-4637-2021, 2021.
Lunt, M. F., Palmer, P. I., Feng, L., Taylor, C. M., Boesch, H., and Parker, R. J.: An increase in methane emissions from tropical Africa between 2010 and 2016 inferred from satellite data, Atmos. Chem. Phys., 19, 14721–14740, https://doi.org/10.5194/acp-19-14721-2019, 2019.
Maasakkers, J. D., Jacob, D. J., Sulprizio, M. P., Scarpelli, T. R., Nesser, H., Sheng, J.-X., Zhang, Y., Hersher, M., Bloom, A. A., Bowman, K. W., Worden, J. R., Janssens-Maenhout, G., and Parker, R. J.: Global distribution of methane emissions, emission trends, and OH concentrations and trends inferred from an inversion of GOSAT satellite data for 2010–2015, Atmos. Chem. Phys., 19, 7859–7881, https://doi.org/10.5194/acp-19-7859-2019, 2019.
Mauzerall, D. L., Logan, J. A., Jacob, D. J., Anderson, B. E., Blake, D. R., Bradshaw, J. D., Heikes, B., Sachse, G. W., Singh, H., and Talbot, B.: Photochemistry in Biomass Burning Plumes and Implications for Tropospheric Ozone over the Tropical South Atlantic, J. Geophys. Res., 103, 8401–8423, https://doi.org/10.1029/97JD02612, 1998.
Miller, C. E., Crisp, D., DeCola, P. L., Olsen, S. C., Randerson, J. T., Michalak, A. M., Alkhaled, A., Rayner, P., Jacob, D. J., Suntharalingam, P., Jones, D. B. A., Denning, A. S., Nicholls, M. E., Doney, S. C., Pawson, S., Boesch, H., Connor, B. J., Fung, I. Y., O'Brien, D., Salawitch, R. J., Sander, S. P., Sen, B., Tans, P., Toon, G. C., Wennberg, P. O., Wofsy, S. C., Yung, Y. L., and Law, R. M.: Precision Requirements for Space-Based Data, J. Geophys. Res., 112, D10314, https://doi.org/10.1029/2006JD007659, 2007.
Miller, J. B., Lehman, S. J., Montzka, S. A., Sweeney, C., Miller, B. R., Karion, A., Wolak, C., Dlugokencky, E. J., Southon, J., Turnbull, J. C., and Tans, P. P.: Linking emissions of fossil fuel CO2 and other anthropogenic trace gases using atmospheric 14CO2, J. Geophys. Res. Atmos., 117, D8, https://doi.org/10.1029/2011JD017048, 2012.
Morino, I., Uchino, O., Inoue, M., Yoshida, Y., Yokota, T., Wennberg, P. O., Toon, G. C., Wunch, D., Roehl, C. M., Notholt, J., Warneke, T., Messerschmidt, J., Griffith, D. W. T., Deutscher, N. M., Sherlock, V., Connor, B., Robinson, J., Sussmann, R., and Rettinger, M.: Preliminary validation of column-averaged volume mixing ratios of carbon dioxide and methane retrieved from GOSAT short-wavelength infrared spectra, Atmos. Meas. Tech., 4, 1061–1076, https://doi.org/10.5194/amt-4-1061-2011, 2011.
Morino, I., Velazco, V. A., Hori, A., Uchino, O., and Griffith, D. W. T.: TCCON data from Burgos, Ilocos Norte (PH), Release GGG2014.R0 (GGG2014.R0), CaltechDATA [data set], https://doi.org/10.14291/TCCON.GGG2014.BURGOS01.R0/1368175, 2018.
NASA/LARC/SD/ASDC: MOPITT CO gridded monthly means (Near and Thermal Infrared Radiances) V008, NASA Langley Atmospheric Science Data Center DAAC [data set], https://doi.org/10.5067/TERRA/MOPITT/MOP03JM_L3.008, 2000.
Nassar, R., Napier-Linton, L., Gurney, K. R., Andres, R. J., Oda, T., Vogel, F. R., and Deng, F.: Improving the Temporal and Spatial Distribution of CO2 Emissions from Global Fossil Fuel Emission Data Sets, J. Geophys. Res., 118, 917–933, https://doi.org/10.1029/2012JD018196, 2013.
NIES (National Institute for Environmental Studies): GOSAT Data Archive Service (GDAS), https://data2.gosat.nies.go.jp, last access: 6 June 2023.
Noël, S., Reuter, M., Buchwitz, M., Borchardt, J., Hilker, M., Schneising, O., Bovensmann, H., Burrows, J. P., Di Noia, A., Parker, R. J., Suto, H., Yoshida, Y., Buschmann, M., Deutscher, N. M., Feist, D. G., Griffith, D. W. T., Hase, F., Kivi, R., Liu, C., Morino, I., Notholt, J., Oh, Y.-S., Ohyama, H., Petri, C., Pollard, D. F., Rettinger, M., Roehl, C., Rousogenous, C., Sha, M. K., Shiomi, K., Strong, K., Sussmann, R., Té, Y., Velazco, V. A., Vrekoussis, M., and Warneke, T.: Retrieval of greenhouse gases from GOSAT and GOSAT-2 using the FOCAL algorithm, Atmos. Meas. Tech., 15, 3401–3437, https://doi.org/10.5194/amt-15-3401-2022, 2022.
Oda, T., Bun, R., Kinakh, V., Topylko, P., Halushchak, M., Marland, G., Lauvaux, T., Jonas, M., Maksyutov, S., Nahorski, Z., Lesiv, M., Danylo, O., and Horabik-Pyzel, J.: Errors and Uncertainties in a Gridded Carbon Dioxide Emissions Inventory, Mitig. Adapt. Strateg. Glob. Change, 24, 1007–1050, https://doi.org/10.1007/s11027-019-09877-2, 2019.
Oh, Y.-S., Kenea, S. T., Goo, T.-Y., Chung, K.-S., Rhee, J.-S., Ou, M.-L., Byun, Y.-H., Wennberg, P. O., Kiel, M., DiGangi, J. P., Diskin, G. S., Velazco, V. A., and Griffith, D. W. T.: Characteristics of greenhouse gas concentrations derived from ground-based FTS spectra at Anmyeondo, South Korea, Atmos. Meas. Tech., 11, 2361–2374, https://doi.org/10.5194/amt-11-2361-2018, 2018.
Palmer, P. I., Suntharalingam, P., Jones, D. B. A., Jacob, D. J., Streets, D. G., Fu, Q., Vay, S. A., and Sachse, G. W.: Using CO2:CO Correlations to Improve Inverse Analyses of Carbon Fluxes, J. Geophys. Res., 111, D12318, https://doi.org/10.1029/2005JD006697, 2006.
Palmer, P. I., Feng, L., Baker, D., Chevallier, F., Bösch, H., and Somkuti, P.: Net Carbon Emissions from African Biosphere Dominate Pan-Tropical Atmospheric CO2 Signal, Nat. Commun., 10, 3344, https://doi.org/10.1038/s41467-019-11097-w, 2019.
Park, H., Jeong, S., Park, H., Labzovskii, L. D., and Bowman, K. W.: An assessment of emission characteristics of Northern Hemisphere cities using spaceborne observations of CO2, CO, and NO2, Remote Sens. Environ., 254, 112246, https://doi.org/10.1016/j.rse.2020.112246, 2021.
Parker, R. J., Boesch, H., Wooster, M. J., Moore, D. P., Webb, A. J., Gaveau, D., and Murdiyarso, D.: Atmospheric CH4 and CO2 enhancements and biomass burning emission ratios derived from satellite observations of the 2015 Indonesian fire plumes, Atmos. Chem. Phys., 16, 10111–10131, https://doi.org/10.5194/acp-16-10111-2016, 2016.
Peylin, P., Houweling, S., Krol, M. C., Karstens, U., Rödenbeck, C., Geels, C., Vermeulen, A., Badawy, B., Aulagnier, C., Pregger, T., Delage, F., Pieterse, G., Ciais, P., and Heimann, M.: Importance of fossil fuel emission uncertainties over Europe for CO2 modeling: model intercomparison, Atmos. Chem. Phys., 11, 6607–6622, https://doi.org/10.5194/acp-11-6607-2011, 2011.
Peylin, P., Law, R. M., Gurney, K. R., Chevallier, F., Jacobson, A. R., Maki, T., Niwa, Y., Patra, P. K., Peters, W., Rayner, P. J., Rödenbeck, C., van der Laan-Luijkx, I. T., and Zhang, X.: Global atmospheric carbon budget: results from an ensemble of atmospheric CO2 inversions, Biogeosciences, 10, 6699–6720, https://doi.org/10.5194/bg-10-6699-2013, 2013.
Plant, G., Kort, E. A., Murray, L. T., Maasakkers, J. D., and Aben, I.: Evaluating Urban Methane Emissions from Space Using TROPOMI Methane and Carbon Monoxide Observations, Remote Sens. Environ., 268, 112756, https://doi.org/10.1016/j.rse.2021.112756, 2022.
Popa, M. E., Vollmer, M. K., Jordan, A., Brand, W. A., Pathirana, S. L., Rothe, M., and Röckmann, T.: Vehicle emissions of greenhouse gases and related tracers from a tunnel study: CO : CO2, N2O : CO2, CH4 : CO2, O2 : CO2 ratios, and the stable isotopes 13C and 18O in CO2 and CO, Atmos. Chem. Phys., 14, 2105–2123, https://doi.org/10.5194/acp-14-2105-2014, 2014.
Prather, M. J.: Lifetimes and Eigenstates in Atmospheric Chemistry, Geophys. Res. Lett., 21, 801–804, https://doi.org/10.1029/94GL00840, 1994.
Qu, Z., Jacob, D. J., Shen, L., Lu, X., Zhang, Y., Scarpelli, T. R., Nesser, H., Sulprizio, M. P., Maasakkers, J. D., Bloom, A. A., Worden, J. R., Parker, R. J., and Delgado, A. L.: Global distribution of methane emissions: a comparative inverse analysis of observations from the TROPOMI and GOSAT satellite instruments, Atmos. Chem. Phys., 21, 14159–14175, https://doi.org/10.5194/acp-21-14159-2021, 2021.
Saeki, T., and Patra, P. K.: Implications of Overestimated Anthropogenic CO2 Emissions on East Asian and Global Land CO2 Flux Inversion, Geosci. Lett., 4, 9, https://doi.org/10.1186/s40562-017-0074-7, 2017.
Saunois, M., Stavert, A. R., Poulter, B., Bousquet, P., Canadell, J. G., Jackson, R. B., Raymond, P. A., Dlugokencky, E. J., Houweling, S., Patra, P. K., Ciais, P., Arora, V. K., Bastviken, D., Bergamaschi, P., Blake, D. R., Brailsford, G., Bruhwiler, L., Carlson, K. M., Carrol, M., Castaldi, S., Chandra, N., Crevoisier, C., Crill, P. M., Covey, K., Curry, C. L., Etiope, G., Frankenberg, C., Gedney, N., Hegglin, M. I., Höglund-Isaksson, L., Hugelius, G., Ishizawa, M., Ito, A., Janssens-Maenhout, G., Jensen, K. M., Joos, F., Kleinen, T., Krummel, P. B., Langenfelds, R. L., Laruelle, G. G., Liu, L., Machida, T., Maksyutov, S., McDonald, K. C., McNorton, J., Miller, P. A., Melton, J. R., Morino, I., Müller, J., Murguia-Flores, F., Naik, V., Niwa, Y., Noce, S., O'Doherty, S., Parker, R. J., Peng, C., Peng, S., Peters, G. P., Prigent, C., Prinn, R., Ramonet, M., Regnier, P., Riley, W. J., Rosentreter, J. A., Segers, A., Simpson, I. J., Shi, H., Smith, S. J., Steele, L. P., Thornton, B. F., Tian, H., Tohjima, Y., Tubiello, F. N., Tsuruta, A., Viovy, N., Voulgarakis, A., Weber, T. S., van Weele, M., van der Werf, G. R., Weiss, R. F., Worthy, D., Wunch, D., Yin, Y., Yoshida, Y., Zhang, W., Zhang, Z., Zhao, Y., Zheng, B., Zhu, Q., Zhu, Q., and Zhuang, Q.: The Global Methane Budget 2000–2017, Earth Syst. Sci. Data, 12, 1561–1623, https://doi.org/10.5194/essd-12-1561-2020, 2020.
Sha, M. K., Langerock, B., Blavier, J.-F. L., Blumenstock, T., Borsdorff, T., Buschmann, M., Dehn, A., De Mazière, M., Deutscher, N. M., Feist, D. G., García, O. E., Griffith, D. W. T., Grutter, M., Hannigan, J. W., Hase, F., Heikkinen, P., Hermans, C., Iraci, L. T., Jeseck, P., Jones, N., Kivi, R., Kumps, N., Landgraf, J., Lorente, A., Mahieu, E., Makarova, M. V., Mellqvist, J., Metzger, J.-M., Morino, I., Nagahama, T., Notholt, J., Ohyama, H., Ortega, I., Palm, M., Petri, C., Pollard, D. F., Rettinger, M., Robinson, J., Roche, S., Roehl, C. M., Röhling, A. N., Rousogenous, C., Schneider, M., Shiomi, K., Smale, D., Stremme, W., Strong, K., Sussmann, R., Té, Y., Uchino, O., Velazco, V. A., Vigouroux, C., Vrekoussis, M., Wang, P., Warneke, T., Wizenberg, T., Wunch, D., Yamanouchi, S., Yang, Y., and Zhou, M.: Validation of methane and carbon monoxide from Sentinel-5 Precursor using TCCON and NDACC-IRWG stations, Atmos. Meas. Tech., 14, 6249–6304, https://doi.org/10.5194/amt-14-6249-2021, 2021.
Silva, S. J. and Arellano, A. F.: Characterizing regional-scale combustion using satellite retrievals of CO, NO2, and CO2, Remote Sens., 9, 744, https://doi.org/10.3390/rs9070744, 2017.
Silva, S. J., Arellano, A. F., and Worden, H. M.: Toward anthropogenic combustion emission constraints from space-based analysis of urban CO2 CO sensitivity, Geophys. Res. Lett., 40, 4971–4976, https://doi.org/10.1002/grl.50954, 2013.
Sim, S., Lee, H., Oh, E., Kim, S., Ciais, P., Piao, S., Lin, J. C., Mallia, D. V., Lee, S., Kim, Y.-H., Park, H., Yun, J., and Jeong, S.: Short-term reduction of regional enhancement of atmospheric CO2 in China during the first COVID-19 pandemic period, Environ. Res. Lett., 17, 024036, https://doi.org/10.1088/1748-9326/ac507d, 2022.
Stavert, A. R., Saunois, M., Canadell, J. G., Poulter, B., Jackson, R. B., Regnier, P., Lauerwald, R., Raymond, P. A., Allen, G. H., Patra, P. K., Bergamaschi, P., Bousquet, P., Chandra, N., Ciais, P., Gustafson, A., Ishizawa, M., Ito, A., Kleinen, T., Maksyutov, S., McNorton, J., Melton, J. R., Müller, J., Niwa, Y., Peng, S., Riley, W. J., Segers, A., Tian, H., Tsuruta, A., Yin, Y., Zhang, Z., Zheng, B., and Zhuang, Q.: Regional trends and drivers of the global methane budget, Glob.l Change Biol., 28, 182–200, https://doi.org/10.1111/gcb.15901, 2022.
Streets, D. G., Canty, T., Carmichael, G. R., de Foy, B., Dickerson, R. R., Duncan, B. N., Edwards, D. P., Haynes, J. A., Henze, D. K., Houyoux, M. R., Jacob, D. J., Krotkov, N. A., Lamsal, L. N., Liu, Y., Lu, Z., Martin, R. V., Pfister, G. G., Pinder, R. W., Salawitch, R. J., and Wecht, K. J.: Emissions estimation from satellite retrievals: A review of current capability, Atmos. Environ., 77, 1011–1042, https://doi.org/10.1016/j.atmosenv.2013.05.051, 2013.
Super, I., Denier van der Gon, H. A. C., Visschedijk, A. J. H., Moerman, M. M., Chen, H., van der Molen, M. K., and Peters, W.: Interpreting continuous in-situ observations of carbon dioxide and carbon monoxide in the urban port area of Rotterdam, Atmos. Pollut. Res., 8, 174–187, https://doi.org/10.1016/j.apr.2016.08.008, 2017.
Sussmann, R. and Rettinger, M.: TCCON data from Garmisch (DE), Release GGG2014.R2, CaltechDATA [data set], https://doi.org/10.14291/TCCON.GGG2014.GARMISCH01.R2, 2018.
Swap, R., Garstang, M., Macko, S. A., Tyson, P. D., Maenhaut, W., Artaxo, P., Kållberg, P., and Talbot, R.: The long-range transport of Southern African aerosols to the tropical South Atlantic, J. Geophys. Res.-Atmos., 101, 23777–23791, https://doi.org/10.1029/95JD01049, 1996.
Sze, N. D.: Anthropogenic CO emissions: Implications for the atmospheric CO-OH-CH4 cycle, Science, 195, 673–675, https://doi.org/10.1126/science.195.4279.673, 1977.
Tang, W., Arellano, A. F., DiGangi, J. P., Choi, Y., Diskin, G. S., Agustí-Panareda, A., Parrington, M., Massart, S., Gaubert, B., Lee, Y., Kim, D., Jung, J., Hong, J., Hong, J.-W., Kanaya, Y., Lee, M., Stauffer, R. M., Thompson, A. M., Flynn, J. H., and Woo, J.-H.: Evaluating high-resolution forecasts of atmospheric CO and CO2 from a global prediction system during KORUS-AQ field campaign, Atmos. Chem. Phys., 18, 11007–11030, https://doi.org/10.5194/acp-18-11007-2018, 2018.
Tang, W., Arellano, A. F., Gaubert, B., Miyazaki, K., and Worden, H. M.: Satellite data reveal a common combustion emission pathway for major cities in China, Atmos. Chem. Phys., 19, 4269–4288, https://doi.org/10.5194/acp-19-4269-2019, 2019.
Tang, W., Gaubert, B., Emmons, L., Choi, Y., DiGangi, J. P., Diskin, G. S., Xu, X., He, C., Worden, H., Tilmes, S., Buchholz, R., Halliday, H. S., and Arellano, A. F.: On the relationship between tropospheric CO and CO2 during KORUS-AQ and its role in constraining anthropogenic CO2, Atmos. Chem. Phys. Discuss. [preprint], https://doi.org/10.5194/acp-2020-864, 2020.
Thompson, R. L., Patra, P. K., Chevallier, F., Maksyutov, S., Law, R. M., Ziehn, T., van der Laan-Luijkx, I. T., Peters, W., Ganshin, A., Zhuravlev, R., Maki, T., Nakamura, T., Shirai, T., Ishizawa, M., Saeki, T., Machida, T., Poulter, B., Canadell, J. G., and Ciais, P.: Top–down assessment of the Asian carbon budget since the mid 1990s, Nat. Commun., 7, 10724, https://doi.org/10.1038/ncomms10724, 2016.
Tian, Y., Sun, Y., Liu, C., Wang, W., Shan, C., Xu, X., and Hu, Q.: Characterisation of methane variability and trends from near-infrared solar spectra over Hefei, China, Atmos. Environ., 173, 198–209, https://doi.org/10.1016/j.atmosenv.2017.11.001, 2018.
Total Carbon Column Observing Network (TCCON) Team: 2014 TCCON Data Release, Version GGG2014, CaltechDATA [data set], https://doi.org/10.14291/TCCON.GGG2014, 2017.
Turnbull, J., Rayner, P., Miller, J., Naegler, T., Ciais, P., and Cozic, A.: On the use of 14CO2 as a tracer for fossil fuel CO2: Quantifying uncertainties using an atmospheric transport model, J. Geophys. Res.-Atmos., 114, D22302, https://doi.org/10.1029/2009JD012308, 2009.
Turnbull, J. C., Tans, P. P., Lehman, S. J., Baker, D., Conway, T. J., Chung, Y. S., Gregg, J., Miller, J. B., Southon, J. R., and Zhou, L.-X.: Atmospheric observations of carbon monoxide and fossil fuel CO2 emissions from East Asia, J. Geophys. Res.-Atmos., 116, D24306, https://doi.org/10.1029/2011JD016691, 2011.
Turnbull, J. C., Sweeney, C., Karion, A., Newberger, T., Lehman, S. J., Tans, P. P., Davis, K. J., Lauvaux, T., Miles, N. L., Richardson, S. J., Cambaliza, M. O., Shepson, P. B., Gurney, K., Patarasuk, R., and Razlivanov, I.: Toward quantification and source sector identification of fossil fuel CO2 emissions from an urban area: Results from the INFLUX experiment, J. Geophys. Res.-Atmos., 120, 292–312, https://doi.org/10.1002/2014JD022555, 2015.
van Vuuren, D. P., and Riahi, K.: Do recent emission trends imply higher emissions forever?, Clim. Change, 91, 237–248, https://doi.org/10.1007/s10584-008-9485-y, 2008.
Velazco, V. A., Morino, I., Uchino, O., Hori, A., Kiel, M., Bukosa, B., Deutscher, N. M., Sakai, T., Nagai, T., Bagtasa, G., Izumi, T., Yoshida, Y., and Griffith, D. W. T.: TCCON Philippines: First measurement results, satellite data and model comparisons in Southeast Asia, Remote Sens., 9, 1228, https://doi.org/10.3390/rs9121228, 2017.
Verhulst, K. R., Karion, A., Kim, J., Salameh, P. K., Keeling, R. F., Newman, S., Miller, J., Sloop, C., Pongetti, T., Rao, P., Wong, C., Hopkins, F. M., Yadav, V., Weiss, R. F., Duren, R. M., and Miller, C. E.: Carbon dioxide and methane measurements from the Los Angeles Megacity Carbon Project – Part 1: calibration, urban enhancements, and uncertainty estimates, Atmos. Chem. Phys., 17, 8313–8341, https://doi.org/10.5194/acp-17-8313-2017, 2017.
Vigouroux, C., Stavrakou, T., Whaley, C., Dils, B., Duflot, V., Hermans, C., Kumps, N., Metzger, J.-M., Scolas, F., Vanhaelewyn, G., Müller, J.-F., Jones, D. B. A., Li, Q., and De Mazière, M.: FTIR time-series of biomass burning products (HCN, C2H6, C2H2, CH3OH, and HCOOH) at Reunion Island (21° S, 55° E) and comparisons with model data, Atmos. Chem. Phys., 12, 10367–10385, https://doi.org/10.5194/acp-12-10367-2012, 2012.
Wang, W., Tian, Y., Liu, C., Sun, Y., Liu, W., Xie, P., Liu, J., Xu, J., Morino, I., Velazco, V. A., Griffith, D. W. T., Notholt, J., and Warneke, T.: Investigating the performance of a greenhouse gas observatory in Hefei, China, Atmos. Meas. Tech., 10, 2627–2643, https://doi.org/10.5194/amt-10-2627-2017, 2017.
Wang, Y., Munger, J. W., Xu, S., McElroy, M. B., Hao, J., Nielsen, C. P., and Ma, H.: CO2 and its correlation with CO at a rural site near Beijing: implications for combustion efficiency in China, Atmos. Chem. Phys., 10, 8881–8897, https://doi.org/10.5194/acp-10-8881-2010, 2010.
Wang, Y., Yuan, Q., Zhou, S., and Zhang, L.: Global spatiotemporal completion of daily high-resolution TCCO from TROPOMI over land using a swath-based local ensemble learning method, ISPRS J. Photogramm. Remote Sens., 194, 167–180, https://doi.org/10.1016/j.isprsjprs.2022.10.012, 2022.
Wei, W., Zhang, W., Hu, D., Ou, L., Tong, Y., Shen, G., Shen, H., and Wang, X.: Emissions of carbon monoxide and carbon dioxide from uncompressed and pelletized biomass fuel burning in typical household stoves in China, Atmos. Environ., 56, 136–142, https://doi.org/10.1016/j.atmosenv.2012.03.060, 2012.
Weisz, H. and Steinberger, J. K.: Reducing energy and material flows in cities, Curr. Opin. Environ. Sustain., 2, 185–192, https://doi.org/10.1016/j.cosust.2010.05.010, 2010.
Wennberg, P. O., Mui, W., Wunch, D., Kort, E. A., Blake, D. R., Atlas, E. L., Santoni, G. W., Wofsy, S. C., Diskin, G. S., Jeong, S., and Fischer, M. L.: On the sources of methane to the Los Angeles atmosphere, Environ. Sci. Technol., 46, 9282–9289, https://doi.org/10.1021/es301138y, 2012.
Wennberg, P. O., Wunch, D., Roehl, C. M., Blavier, J.-F., Toon, G. C., and Allen, N. T.: TCCON data from Caltech (US), Release GGG2014.R1, CaltechDATA [data set], https://doi.org/10.14291/TCCON.GGG2014.PASADENA01.R1/1182415, 2015.
Wu, C. and Yu, J. Z.: Evaluation of linear regression techniques for atmospheric applications: the importance of appropriate weighting, Atmos. Meas. Tech., 11, 1233–1250, https://doi.org/10.5194/amt-11-1233-2018, 2018.
Wunch, D., Wennberg, P. O., Toon, G. C., Keppel-Aleks, G., and Yavin, Y. G.: Emissions of greenhouse gases from a North American megacity, Geophys. Res. Lett, 36, L15810, https://doi.org/10.1029/2009GL039825, 2009.
Wunch, D., Toon, G. C., Wennberg, P. O., Wofsy, S. C., Stephens, B. B., Fischer, M. L., Uchino, O., Abshire, J. B., Bernath, P., Biraud, S. C., Blavier, J.-F. L., Boone, C., Bowman, K. P., Browell, E. V., Campos, T., Connor, B. J., Daube, B. C., Deutscher, N. M., Diao, M., Elkins, J. W., Gerbig, C., Gottlieb, E., Griffith, D. W. T., Hurst, D. F., Jiménez, R., Keppel-Aleks, G., Kort, E. A., Macatangay, R., Machida, T., Matsueda, H., Moore, F., Morino, I., Park, S., Robinson, J., Roehl, C. M., Sawa, Y., Sherlock, V., Sweeney, C., Tanaka, T., and Zondlo, M. A.: Calibration of the Total Carbon Column Observing Network using aircraft profile data, Atmos. Meas. Tech., 3, 1351–1362, https://doi.org/10.5194/amt-3-1351-2010, 2010.
Wunch, D., Toon, G. C., Blavier, J.-F. L., Washenfelder, R. A., Notholt, J., Connor, B. J., Griffith, D. W. T., Sherlock, V., and Wennberg, P. O.: The Total Carbon Column Observing Network, Philos. Trans. R. Soc. A, 369, 2087–2112, https://doi.org/10.1098/rsta.2010.0240, 2011.
Wunch, D., Wennberg, P. O., Osterman, G., Fisher, B., Naylor, B., Roehl, C. M., O'Dell, C., Mandrake, L., Viatte, C., Kiel, M., Griffith, D. W. T., Deutscher, N. M., Velazco, V. A., Notholt, J., Warneke, T., Petri, C., De Maziere, M., Sha, M. K., Sussmann, R., Rettinger, M., Pollard, D., Robinson, J., Morino, I., Uchino, O., Hase, F., Blumenstock, T., Feist, D. G., Arnold, S. G., Strong, K., Mendonca, J., Kivi, R., Heikkinen, P., Iraci, L., Podolske, J., Hillyard, P. W., Kawakami, S., Dubey, M. K., Parker, H. A., Sepulveda, E., García, O. E., Te, Y., Jeseck, P., Gunson, M. R., Crisp, D., and Eldering, A.: Comparisons of the Orbiting Carbon Observatory-2 (OCO-2) measurements with TCCON, Atmos. Meas. Tech., 10, 2209–2238, https://doi.org/10.5194/amt-10-2209-2017, 2017.
Yokelson, R. J., Andreae, M. O., and Akagi, S. K.: Pitfalls with the use of enhancement ratios or normalized excess mixing ratios measured in plumes to characterize pollution sources and aging, Atmos. Meas. Tech., 6, 2155–2158, https://doi.org/10.5194/amt-6-2155-2013, 2013.
York, D., Evensen, N. M., López Martıìnez, M., and De Basabe Delgado, J.: Unified equations for the slope, intercept, and standard errors of the best straight line, Am. J. Phys., 72, 367–375, https://doi.org/10.1119/1.1632486, 2004.
Yoshida, Y., Kikuchi, N., Morino, I., Uchino, O., Oshchepkov, S., Bril, A., Saeki, T., Schutgens, N., Toon, G. C., Wunch, D., Roehl, C. M., Wennberg, P. O., Griffith, D. W. T., Deutscher, N. M., Warneke, T., Notholt, J., Robinson, J., Sherlock, V., Connor, B., Rettinger, M., Sussmann, R., Ahonen, P., Heikkinen, P., Kyrö, E., Mendonca, J., Strong, K., Hase, F., Dohe, S., and Yokota, T.: Improvement of the retrieval algorithm for GOSAT SWIR XCO2 and XCH4 and their validation using TCCON data, Atmos. Meas. Tech., 6, 1533–1547, https://doi.org/10.5194/amt-6-1533-2013, 2013.
Zhang, X., Liu, J., Han, H., Zhang, Y., Jiang, Z., Wang, H., Meng, L., Li, Y. C., and Liu, Y.: Satellite-observed variations and trends in carbon monoxide over Asia and their sensitivities to biomass burning, Remote Sens., 12, 830, https://doi.org/10.3390/rs12050830, 2020.
Zhang, Z., Zimmermann, N. E., Calle, L., Hurtt, G., Chatterjee, A., and Poulter, B.: Enhanced response of global wetland methane emissions to the 2015–2016 El Niño-Southern Oscillation event, Environ. Res. Lett., 13, 074009, https://doi.org/10.1088/1748-9326/aac939, 2018.
Zhao, Y., Saunois, M., Bousquet, P., Lin, X., Berchet, A., Hegglin, M. I., Canadell, J. G., Jackson, R. B., Hauglustaine, D. A., Szopa, S., Stavert, A. R., Abraham, N. L., Archibald, A. T., Bekki, S., Deushi, M., Jöckel, P., Josse, B., Kinnison, D., Kirner, O., Marécal, V., O'Connor, F. M., Plummer, D. A., Revell, L. E., Rozanov, E., Stenke, A., Strode, S., Tilmes, S., Dlugokencky, E. J., and Zheng, B.: Inter-model comparison of global hydroxyl radical (OH) distributions and their impact on atmospheric methane over the 2000–2016 period, Atmos. Chem. Phys., 19, 13701–13723, https://doi.org/10.5194/acp-19-13701-2019, 2019.
Zhao, Y., Saunois, M., Bousquet, P., Lin, X., Berchet, A., Hegglin, M. I., Canadell, J. G., Jackson, R. B., Deushi, M., Jöckel, P., Kinnison, D., Kirner, O., Strode, S., Tilmes, S., Dlugokencky, E. J., and Zheng, B.: On the role of trend and variability in the hydroxyl radical (OH) in the global methane budget, Atmos. Chem. Phys., 20, 13011–13022, https://doi.org/10.5194/acp-20-13011-2020, 2020.
Zhou, M., Langerock, B., Vigouroux, C., Sha, M. K., Ramonet, M., Delmotte, M., Mahieu, E., Bader, W., Hermans, C., Kumps, N., Metzger, J.-M., Duflot, V., Wang, Z., Palm, M., and De Mazière, M.: Atmospheric CO and CH4 time series and seasonal variations on Reunion Island from ground-based in situ and FTIR (NDACC and TCCON) measurements, Atmos. Chem. Phys., 18, 13881–13901, https://doi.org/10.5194/acp-18-13881-2018, 2018.
Zhu, T., Melamed, M. L., Parrish, D., Gauss, M., Gallardo Klenner, L., Lawrence, M. G., Konare, A., and Liousse, C.: WMO/IGAC impacts of megacities on air pollution and climate, World Meteorological Organization, https://igacproject.org/sites/default/files/2016-07/GAW%20Report%20205.pdf (last access: 20 September 2021), 2012.