the Creative Commons Attribution 4.0 License.
the Creative Commons Attribution 4.0 License.
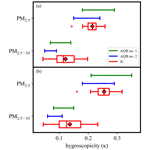
Deriving the hygroscopicity of ambient particles using low-cost optical particle counters
Wei-Chieh Huang
Ching-Wei Chu
Wei-Chun Hwang
Shih-Chun Candice Lung
This study investigates the chemical composition and physical properties of aerosols, which play a crucial role in influencing human health, cloud physics, and local climate. Our focus centers on the hygroscopicity of ambient aerosols, a key property reflecting the ability to take up moisture from the atmosphere and serve as cloud condensation nuclei. Employing home-built air quality box (AQB) systems equipped with low-cost sensors, we assess the ambient variability of particulate matter (PM) concentrations to determine PM hygroscopicity. The AQB systems effectively captured meteorological parameters and most pollutant concentrations, showing high correlations with data from the Taiwan Environmental Protection Administration (TW-EPA). With the application of κ-Köhler equation and certain assumptions, AQB-monitored PM concentrations are converted to dry particle mass concentration, providing optical particle counter sensitivity correction and resulting in improved correlation with TW-EPA data. The derived single hygroscopicity parameters (κ) range from 0.15 to 0.29 for integrated fine particles (PM2.5) and 0.05 to 0.13 for coarse particles (PM2.5−10), consistent with results of ionic chromatography analysis from a previous winter campaign nearby. Moreover, the analysis of PM10 division into PM2.5 and PM2.5−10, considering composition heterogeneity, provided improved dry PM10 concentration as the sensitivity coefficients for PM2.5−10 were notably higher than for PM2.5. Our methodology provides a comprehensive approach to assess ambient aerosol hygroscopicity, with significant implications for atmospheric modeling, particularly in evaluating aerosol efficiency as cloud condensation nuclei and in radiative transfer calculations. Overall, the AQB systems proved to be effective in monitoring air quality and deriving key aerosol properties, contributing valuable insights into atmospheric science.
- Article
(2627 KB) - Full-text XML
-
Supplement
(1511 KB) - BibTeX
- EndNote
In an era of increased industrialization, individuals face growing exposure to poor air quality, elevating the risks of cardiovascular and respiratory diseases (Chen et al., 2017; Brook et al., 2010; Heus et al., 2010). Within the realm of air pollutants, atmospheric aerosols emerge as critical components, playing a vital role in Earth's climate system. They influence radiative balance, cloud formation, and precipitation patterns, while significantly impacting human health, visibility, and ecosystems (Pöschl et al., 2010; Wu et al., 2010; Brook et al., 2010; Hamanaka and Mutlu, 2018). Their ability to scatter and absorb solar radiation, coupled with their role as cloud condensation nuclei (CCNs), emphasizes their significance in shaping both climate dynamics and air quality (Andreae and Rosenfeld, 2008; Rosenfeld et al., 2014; Lohmann and Feichter, 2005). However, understanding the complex interplay between aerosols and these processes requires the physical and chemical properties of aerosols, including hygroscopicity. The hygroscopic growth of aerosol particles, indicating their ability to take up moisture from the ambient air, alters their size distribution, mass, optical properties, and CCN activity, thereby impacting climate dynamics and air quality (Petters and Kreidenweis, 2007). The traditional methods such as hygroscopic tandem differential mobility analyzers and cloud condensation nuclei counters (Chan and Chan, 2005; Hung et al., 2016; Bian et al., 2014) have provided valuable insights into the hygroscopic properties of various aerosol types. However, their complexity and cost often limit their applicability for extensive, long-term measurements.
Over the past decade, the rise in popularity of low-cost optical particle counters (OPCs) can be attributed to their simplicity, portability, and affordability (Sá et al., 2022; Crilley et al., 2018; Samad et al., 2021). OPCs provide real-time data on particle size distributions and mass concentrations with high temporal resolution for monitoring ambient particles. However, challenges arise in ensuring the accuracy of OPCs, necessitating additional constraints or calibrations for optimal performance. The measurement principle of OPCs relies on the dependence of Mie scattering on particle size, yet this dependence is non-monotonic across all sizes. Additionally, particle composition influences light scattering, leading to varying scattering efficiencies (Kaliszewski et al., 2020; Formenti et al., 2021). Variations in particle density directly affect the mass concentration derived from the monitored number size concentration (Hagan and Kroll, 2020; Dacunto et al., 2015). A particularly challenging issue involves the removal of liquid water from ambient particles. Several studies have attempted to derive the dry mass concentration of ambient particles using OPC, employing calibration methods linked to the hygroscopic growth factor (HGF) under controlled relative humidity (RH) conditions. Notably, Crilley et al. (2018) improved OPC mass concentration correction by applying the derived hygroscopicity (κ) values of 0.38–0.41 and 0.48–0.51 for PM2.5 and PM10, respectively, achieving a 33 % improvement. Similarly, Di Antonio et al. (2018) and Venkatraman Jagatha et al. (2021) elevated calibration from a moderate to a high correlation by assuming a constant κ of 0.40. Furthermore, the chemical composition and physical properties of aerosols exhibit high temporal–spatial variation, making the analysis and correction of observational data from a physical perspective crucial. The widespread adoption of low-cost sensors, attributed to their affordability, enables more extensive use as users find them more accessible (Castell et al., 2017). This increased utilization enhances spatial resolution in environmental monitoring, deepening our understanding of pollution evolution. However, it is essential to emphasize that regular maintenance and calibration are necessary for accurate results (Concas et al., 2021; Sá et al., 2022).
In this study, we evaluate the performance of our home-built monitoring system, air quality box (AQB), through a comprehensive analysis and calibration by co-locating the two AQB systems with the Taiwan Environmental Protection Administration (TW-EPA) station. Our primary focus is on OPCs, for which we employed a physical model to elucidate the hygroscopic characteristics of ambient particles during the determination of dry particle mass concentrations for integrated fine particles (PM2.5, Dp≤2.5 µm) and coarse particles (PM2.5−10, 2.5 µm <Dp ≤ 10 µm). Additionally, we discuss various factors contributing to errors in hygroscopicity estimates, aiming to gain valuable insights into using low-cost sensors for extensive and prolonged monitoring applications.
2.1 Air quality box (AQB) system
Two home-built AQB systems (AQB no. 1 and AQB no. 2) consist of multiple sensors that monitor meteorological parameters such as temperature (T), relative humidity (RH), and pressure (P), as well as gaseous species and particulate matter (PM) with a temporal resolution of seconds as shown in Fig. 1 with sensor information summarized in Table S1. The gas sensors include five Alphasense amperometric B4 series sensors that measure CO, NO, NO2, Ox (O3+ NO2), and SO2; a photo-ionization detector (PID-AH2, Alphasense) monitoring volatile organic compounds; and a non-dispersive infrared CO2 sensor from Amphenol Advanced Sensors (T6713-5K). The PID sensor, equipped with a krypton lamp providing a photon energy of about 10.6 eV, cannot detect methane, which has a higher ionization potential of ∼ 13.7 eV (Glockler, 1926). Therefore, the data of non-methane hydrocarbons (NMHC) from TW-EPA are more comparable to PID data in our analysis. The PM sensor (OPC-N2, Alphasense), an optical particle counter, monitors the number size distribution between 0.38 and 17 µm, divided into 16 bins based on Mie scattering, with a sampling flow rate of ∼ 4 mL s−1 and a refractive index of 1.5+0 i. In addition, the mass concentration of PM1, PM2.5, and PM10 could be calculated from the number size distribution, assuming a particle density of 1.65 g cm−3. These sensors were controlled by a small single-board computer, Raspberry Pi Zero W, at a time resolution of 3 s with data stored in a microSD card and uploaded to cloud storage via 4G LTE. The entire system is housed in a remodeled enclosure with a dimension of 25 cm × 16 cm × 8 cm (L × D × H) and has well-ventilated openings for sampling and exhaust. The sampling flow rate is primarily controlled by an installed fan at ∼ 5.6 L min−1, corresponding to a residence time of approximately 34 s in the box. This configuration allows the system to effectively monitor ambient air quality independently without the need of additional inlets.
2.2 Calibration campaign and reference data
The calibration of AQB sensors was carried out by co-locating them with the TW-EPA Nanzi station (Fig. S1) in Kaohsiung, Taiwan (22°44′12′′ N, 120°19′42′′ E) from 4 to 19 February 2021. Nanzi station is situated on the roof of a 15 m high building in a well-ventilated environment. The primary gaseous components, dry PM2.5 and PM10 concentrations, and basic meteorological parameters are continuously monitored using standard instruments, as summarized in Table S1. For electrochemical sensors in AQB, the performance can be influenced by environmental parameters such as temperature, relative humidity, and other chemical species that have high cross sensitivity (Concas et al., 2021; Karagulian et al., 2019; Mead et al., 2013). Therefore, in this study, a linear regression with a multivariate function of voltage and the environmental temperature was applied to retrieve concentrations for gas species. For PM, the reported values by beta attenuation mass monitor (BAM) in the TW-EPA station reflect the dry-state PM concentration by controlling the measurement at RH less than 50 % (i.e., a heating device applied to reduce the sampling flow to 35 % water saturation when the ambient RH is > 50 %). On the contrary, the optical particle counter (OPC) in AQB directly monitors ambient PM concentration. The difference between BAM and OPC data reflects the amount of liquid water content in ambient conditions. A simple linear regression between them might not completely reveal the influence of hygroscopicity. Therefore, the κ-Köhler equation (Petters and Kreidenweis, 2007) was applied to derive the κ as discussed in the following section.
2.3 Sensitivity coefficients of OPCs and particle hygroscopicity
To bridge the PM concentration gap between BAM and OPC, the sensitivity correction of OPC and the conversion of ambient particles to dry particles are required. The sensitivity coefficient (α) was evaluated as the mass concentration ratio of BAM and OPC data at low RH (≤50 %) having limited water content, as follows:
where MBAM andMOPC are PM mass concentrations (µg m−3) measured by BAM and OPC, respectively. RH ≤ 50 % was applied as the threshold criteria for data selection to determine α, as the mass concentration of ambient particles might have significant water uptake at higher RH. The statistical distribution of MBAM to MOPC ratios at RH ≤ 50 % was analyzed to assign α as the mean value ± 0.5σ (σ: standard deviation) to prevent high-concentration data points from dominating the statistical result.
The particle size growth with the water saturation ratio (S) for a given κ can be evaluated using κ-Köhler equation as follows (Petters and Kreidenweis, 2007):
where Damb and Dd are the diameters (m) of the ambient and dry particulate matter, respectively; is the surface tension of the particle (J m−2); Mw is the molecular weight of water (g mol−1); R is the gas constant (J mol−1 K−1); T is the temperature; and ρw is the density of liquid water (1.0 g cm−3). The first term is the solute effect, while the second is the Kelvin effect. As the mass is dominated by the larger particles, the Kelvin effect in Eq. (2) is assumed to be negligible for simplification. The derived dry mass concentration (Md,derived) from the measured ambient particles from OPC data can be expressed as follows (Pope et al., 2010; Crilley et al., 2018):
where ρd is the density of dry aerosol particles and is assumed to be 1.20 g cm−3 in this study. With the determined α values (Eq. 1), κ can be derived from the data points of aqueous particles at RH above 70 %, and the deliquescence RH (DRH) can be verified using ion chromatography (IC) analyzed composition with the Extended Aerosol Inorganics Model (E-AIM) model. The mean absolute percentage error (MAPE) parameter between Md,derived and MBAM was used to assess the appropriate κ value as follows:
where n is the total number of data points. With the restricted range of α, κ can be derived under the minimum MAPE. The detailed process description for κ derivation is provided in the Supplement. Due to the heterogeneity between particles, PM10 was divided into integrated fine particles (Dp≤2.5 µm) and coarse particles (2.5 µm <Dp≤10 µm) to evaluate the individual sensitivity coefficient and hygroscopicity.
2.4 Composition analysis
Hygroscopicity can also be determined using the volume fraction of the major components. Based on an earlier field campaign, the ion chromatography (IC) method was applied to quantify water-soluble components for samples (both PM2.5 and PM10) collected at Fooyin University (22°36′09.8′′ N, 120°23′23.1′′ E) in Kaohsiung from 15 to 28 January 2013. Ambient aerosol samples were collected using a pair of dichotomous aerosol samplers (model RP-2025, R&P Co., Inc., Albany, New York) to collect integrated fine and coarse particles on Teflon filters with sampling flow rates of 15.0 and 16.7 L min−1, respectively. The samples were categorized into daytime and nighttime. Daytime samples were collected from 08:00 to 20:00 LT, and nighttime samples were collected from 20:00 to 08:00 LT the next day. The samplers were equipped with Teflon filters deployed for the measurement of water-soluble ions (Na+, Mg2+, K+, Ca2+, NH, Cl−, SO, and NO) via ion chromatography (model ICS-1000, Dionex). More information on the chemical analysis method can be found in Salvador and Chou (2014).
To derive the hygroscopicity from samplings, the ions from IC analysis were converted to chemical components via the following sequence: ammonium sulfate, ammonium bisulfate, ammonium nitrate (when there is residual ammonium), sodium nitrate, and sodium chloride. With the assumption of the hygroscopicity of insoluble components as zero and negligible residual ion contribution (less than 5 % of total mass), the overall hygroscopicity can be derived by the volume fraction (εi) weighted hygroscopicity from individual soluble component (i species) as follows:
where κi is the hygroscopicity of i species, vtotal is the volume of particles, and vi is the volume of i species. The conversion of particle mass to volume is based on a density of 1.20 g cm−3. The applied hygroscopicity, molecular weight, and density for the related chemical species are summarized in Table S2. With the assumption that these ions dissolve completely in the aqueous phase, which represents the maximum estimation, the hygroscopicity contributed by the residual ions were found to be approximately up to 1.8 % and 6.4 % of the overall κ value for integrated fine particles (PM2.5) and coarse particles (PM2.5−10), respectively. Given their limited impact on the hygroscopic behavior of the particles, the contribution of the residual ions was not taken into account in the calculation. Additionally, other PM2.5 IC data for samples collected at the National Kaohsiung University of Science and Technology (22°46′22.4′′ N, 120°24′03.4′′ E) in Kaohsiung for the period of 8–18 December 2021 samples were also applied for further comparison (no PM10 collection for that campaign). We opted for the 2013 dataset for more discussion due to its comprehensive analysis encompassing both PM2.5 and PM2.5−10. Furthermore, the composition data obtained from IC analysis was applied to E-AIM Model III (for systems containing H+, NH, Na+, SO, NO, Cl−, and H2O) to evaluate the characteristics of volume variation as a function of RH in the range of 30 % to 90 % (Clegg et al., 1998). The partitioning of selected trace gases (HNO3, HCl, NH3, and H2SO4) into the vapor phase was disabled to keep a consistent quantity of applied chemical species in the particle phase. The growth factor, , above DRH, was applied to retrieve the κ value using Eq. (2) but without the Kelvin effect term (Luo et al., 2020). Both the individual sample concentrations and the overall averaged composition conditions were analyzed to evaluate the hygroscopic behavior of the particles.
3.1 Performance of AQB systems
Figure 2 shows the time series of the meteorological parameters and pollutant concentrations between calibrated AQB and TW-EPA data from 14 to 17 February 2021. T, RH, CO, and Ox showed a good correlation with r>0.9, while NO, NO2, PM2.5, and PM10 had a moderate correlation (r≥0.48). The high correlation (r=0.976) for CO (with a lifetime of ∼ 2 months) indicates a similar air parcel sampled by both AQB and the instrumentation in TW-EPA. The PID sensor had consistent peaks with high NMHC concentrations and could not reveal temporal variation at low concentrations, resulting in a low correlation. Overall, the AQB system performs well in capturing the ambient variability of pollutants stated above. The low correlation of SO2 was due to the cross sensitivity of this SO2 sensor, which was highly sensitive to O3 and NO2 (about −120 % reported in the technical specifications of Alphasense). O3 and NO2 generally have higher concentrations than SO2 and cause a significant contribution to the response of the SO2 sensor. However, if high-SO2-concentration events occur, the SO2 sensor might reflect the variation in SO2 concentration. The PM concentration shown in Fig. 2 was calibrated using a simple linear regression, which roughly reflects the trend of mass concentration but shows more significant deviations at higher RH due to more water uptake in particles, as discussed in Sect. 3.2. Most gas species showed a high correlation (r≥0.95) between different AQB systems except for NMHC (r=0.675) as summarized in Table S3. Further results and discussions focus on the PM analysis using AQB no. 1, which has a more consistent sampling rate during the observation period, unless stated otherwise.
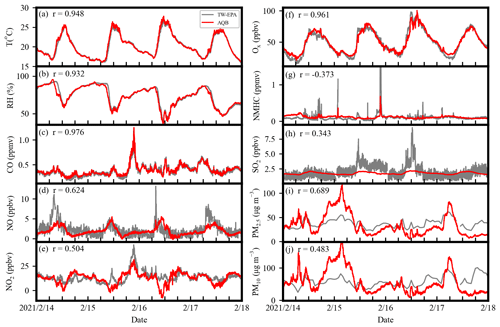
Figure 2The temporal profiles of calibrated AQB data (red lines) and the TW-EPA measurement (gray lines) for (a) temperature, (b) relative humidity, (c) CO, (d) NO, (e) NO2, (f) Ox (≡ NO2+ O3), (g) non-methane hydrocarbon, (h) SO2, (i) PM2.5, and (j) PM10 during the period of 14–17 February 2021 (4 of 16 d in period). All the species were calibrated using linear regression.
3.2 Comparison between OPC and BAM data
3.2.1 Sensitivity coefficient of OPC
Figure 3a and c show the scatter distribution of the mass concentrations between OPC (with no calibration) and BAM data for PM2.5 and PM10, respectively. Overall, the PM mass concentrations measured by OPC have a similar trend to those measured by BAM but exhibit some variability. The results reveal an apparent influence of ambient RH, indicating the contribution of water content. The red-shaded area represents a regression line with a slope corresponding to the inverse of the α derived from data points at ambient RH ≤ 50 % (17 out of 356 points, 5 %). The notable deviation of the red-shaded area from the 1:1 line towards the right side indicates the requirement of α>1 corrections, contributed by the different measurement principles and calibration techniques, which may result from the assumed particle density and refractive index (RI) (dust, density: 1.65 g cm−3; RI: 1.5+0 i). The estimated α values, as summarized in Table 1, are higher for PM10 than for PM2.5, i.e., 2.02 ± 0.34 vs. 1.26 ± 0.16, and are reasonably conclusive as tested with more data points selected at higher RH thresholds (Fig. S2). The α difference between PM2.5 and PM10 might be attributed to the complex composition of ambient particles, which differs from the samples used for instrument calibration, as well as possible sensitivity variations in OPC over time. With sensitivity calibration, the performance at ambient RH ≤ 50 % exhibits a strong correlation with MAPE at 12.8 % and 18.5 % as well as a root mean squared error (RMSE) at 3.7 µg m−3 and 10.3 µg m−3 for PM2.5 and PM10, respectively, as summarized in Table 2 excluding the two significant outliers (shown as hollow circles in Fig. 3). The results confirm the effectiveness of OPCs in capturing PM concentrations after proper calibration, consistent with other real-time outdoor field studies, reporting R2 ranging from 0.34 to 0.97, RMSE ranging from 0.52 to 12.3 µg m−3, and MAPE about 22 % (Gillooly et al., 2019; Demanega et al., 2021; Sá et al., 2022; Crilley et al., 2018). Additionally, the OPC sampling flow rate has an impact on measurement performance. AQB no. 1 maintained a steady rate at 3.6 ± 0.2 L min−1, whereas AQB no. 2 exhibits two distinct periods with sampling flow rates of 3.6–4.2 L min−1 for the first period and 3.2–3.6 L min−1 for the second period. The distinctive sampling flow rates result in a non-linear change in α, suggesting the need to separate the data into two parts to estimate the individual α (Fig. S3).
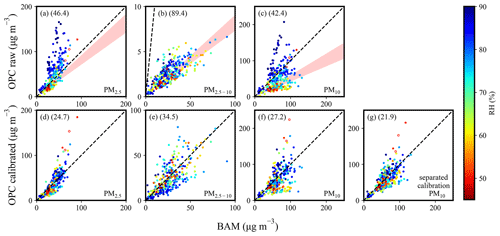
Figure 3The correlation of mass concentration between BAM and OPC in AQB no. 1 (raw data or calibrated data): (a, d) PM2.5, (b, e) PM2.5−10, (c, f) PM10, and (f) separated calibration PM10. Panels (a)–(c) are the raw data, while panels (d)–(g) are the calibrated data. The marker color corresponds to relative humidity. The hollow points are the two significant outliers under conditions of RH ≤ 50 %. The shaded region represents the data associated with the sensitivity coefficient (α). The value in parentheses is the MAPE in percentage.
Table 1The sensitivity coefficients and the hygroscopicity for PM2.5, PM10, and PM2.5−10.
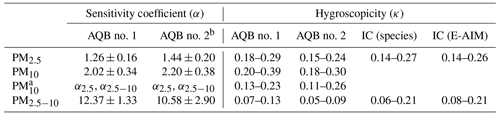
a The hygroscopicity derived using different sensitivity coefficients for different size ranges. α2.5 and α2.5−10 are sensitivity coefficients for PM2.5 and PM2.5−10, respectively. More details are provided in the description of the Supplement. b The sensitivity of AQB no. 2 presents the value in the period of sampling flow rates at 3.6–4.2 L min−1.
Table 2Performance metrics of different calibration methods for PM2.5, PM2.5−10, and PM10.

a Only for data points at RH ≤ 50 %. The value in parentheses is the performance result without two significant outliers shown in Fig. 3. b Coefficient of determination (R2) is calculated as the proportion of variation in the calibrated dry mass concentration. c The combination of calibrated data from PM2.5 all data (κ=0.29) and PM2.5−10 all data (κ=0.09).
3.2.2 Hygroscopicity derivation
With the derived α, the hygroscopicities were retrieved using Eq. (3), resulting in κ ranging from 0.18 to 0.29 for PM2.5 and 0.20 to 0.39 for PM10 during the studied period, as summarized in Table 1. Figure 3d and f show the scatter distribution of the derived dry concentration vs. BAM concentration for PM2.5 and PM10, respectively. The results from the two OPCs exhibit slight differences but are consistent overall. Considering both the sensitivity coefficient and hygroscopicity, the performance of OPC in deriving dry PM concentration is significantly improved with lower MAPE, RMSE, and higher R2 than the results obtained using only the sensitivity coefficient, as summarized in Table 2. With a similar methodology, Crilley et al. (2018) applied the κ-Köhler equation to compare measured data between OPC-N2 and tapered element oscillating microbalance (TEOM) and derived the κ ranging from 0.38 to 0.41 and 0.48 to 0.51 for PM2.5 and PM10, respectively, which is within the range for Europe (i.e., 0.36 ± 0.16) (Pringle et al., 2010).
Due to the heterogeneity of composition among different sizes, PM10 can be divided into PM2.5 and PM2.5−10 for further analysis. The estimated α value for PM2.5−10, as summarized in Table 1, is approximately 1 order of magnitude higher than that for PM2.5. The lower κ for PM2.5−10 might suggest a significant contribution from dust or other less hygroscopic species, consistent with the IC analyses in Table 3 and discussed further in Sect. 3.3. With the retrieved α and κ for PM2.5 and PM2.5−10, Fig. 3e shows the scatter distribution between the derived dry PM2.5−10 from OPC and BAM data, exhibiting a MAPE of 31.8 %, which is more significant than the 24.8 % for PM2.5. The higher MAPE might result from the low particle number concentration in the coarse mode, with only about 0.01 to 0.1 particles per bin per cubic centimeter in the size range of 3.0 to 10.0 µm. The results are consistent with the findings of Kaliszewski et al. (2020), which showed that the correlation between OPC-N3 (a newer version of OPC-N2) and AeroTrak 8220 (TSI Inc., Shoreview, MN, USA) measurement data decreases with particle size, from 0.3–0.5 µm (r=0.99) to 5–10 µm (r=0.74). The dry PM10 derived from OPC through the divided PM2.5 and PM2.5−10 analysis demonstrates better consistency with the reported BAM data than the direct calibration method. This is evidenced by a lower MAPE in Fig. 3g (18.2 %) compared to Fig. 3f (29.2 %) and a significant improvement than the simple linear regression method, which has a higher MAPE at 62.5 % (Table 2). Moreover, the derived κ for PM10 with the size-dependent sensitivity coefficient correction ranges from 0.13 to 0.23 (Table 1). This value falls between those for PM2.5 and PM2.5−10 and is more reasonable compared to κ derived with a fixed sensitivity coefficient (κ=0.20–0.39, higher than those for PM2.5 and PM2.5−10). The results substantiate the importance of considering composition heterogeneity among particle sizes for accurate dry PM derivation.
3.3 Hygroscopicity derivation using IC data
3.3.1 Composition analysis
The major soluble composition and concentrations obtained from the IC analysis are summarized in Table 3. The mean concentrations of PM2.5 and PM2.5−10 are 67 ± 19 and 36 ± 7 µg m−3, respectively. The determined soluble composition of PM2.5 constitutes approximately 53 % of the mass fraction and is predominantly composed of NH, SO, and NO, which are formed through chemical reactions involving industrial and agricultural emissions. In contrast, ∼ 30 % of PM2.5−10 is soluble components, including NO, SO, Na+, Cl−, NH, and some alkaline earth metal ions (Ca2+ and Mg2+), with a more significant proportion being insoluble components (∼ 70 %), likely attributed to dust, metallic elements, and unanalyzed organic components. The higher portion of sea salt (Na+ and Cl−) in PM2.5−10 than in PM2.5 is likely transported by the sea breeze during the daytime, while the increased fractions of Ca2+ and Mg2+ might correspond to sand or dust particles (Li et al., 2022).
The temporal variation of derived κ, based on the IC soluble composition analysis, ranges from 0.14 to 0.26 for PM2.5 and 0.06 to 0.21 for PM2.5−10, as shown in Fig. S4a and summarized in Table 1. A similar analysis for the winter of 2021 showed a similar κ range for PM2.5, as illustrated in Fig. S5. This consistency across distinct study periods indicates typical winter hygroscopic characteristics for ambient PM2.5 in Kaohsiung, applicable for further discussion with the OPC data. For PM2.5−10, the more significant variability in κ compared to PM2.5 can be attributed to substantial fluctuations of soluble composition in coarse particles, primarily driven by significant quantities of thenardite (Na2SO4) and halite (NaCl) (Tang et al., 2019). Due to the dominance of the northeast monsoon wind during the filter sampling period, the influence of the sea–land breeze was too relatively weak to cause apparent diurnal variation in κ.
The κ values derived from IC analysis reflect the temporal variation, while those from OPC analysis indicate the overall physical properties of ambient aerosols for the studied period. Factors such as spatial and temporal variations in aerosols, different campaign years and locations (∼ 20 km apart, as shown in Fig. S1), and technique uncertainties, such as ammonia and nitrate sampling evaporation during filter sampling (Hering and Cass, 1999; Chen et al., 2021), as well as OPC detection uncertainties and assumption required for calculation, can influence comparisons. However, the results (i.e., ∼ 0.22 (OPC) vs. 0.14–0.27 (IC) for PM2.5 and ∼ 0.09 (OPC) vs. 0.06–0.21 (IC) for PM2.5−10) summarized in Table 1 and Fig. 4a suggest that the derived κ values from OPC data likely reflect the mean hygroscopicity for the integrated fine and coarse particles during winter in Kaohsiung.
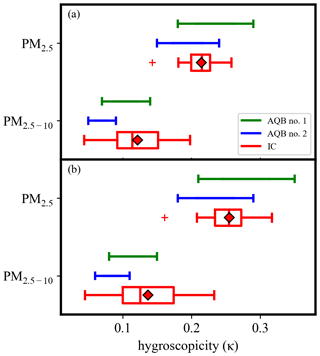
Figure 4The hygroscopicities of PM2.5 and PM2.5−10 derived based on data from OPCs and ion chromatography with the assumption particle density of (a) 1.2 g cm−3 and (b) 1.42 ± 0.03 and 1.34 ± 0.07 g cm−3 for PM2.5 and PM2.5−10, respectively, based on analyzed composition. The average value is shown as a red diamond.
3.3.2 E-AIM analysis
With the measured composition, the hygroscopicity can be derived from the growth pattern. The particle growth might follow the κ-Köhler equation (Eq. 2) when all soluble species are fully dissolved, typically occurring above the DRH. Using the averaged soluble composition determined from the IC analysis, HGF as a function of RH calculated using E-AIM is shown in Fig. 5. For PM2.5, partial deliquescence initiates at 60 % RH with some residual solid components such as ((NH4)2SO4 and 2NH4NO3 ⋅ (NH4)2SO4). Complete dissolution occurs at RH ∼ 72 %. In the case of PM2.5−10, water uptake begins at 42 % RH, leaving a residual solid composed of 3NH4NO3(NH4)2SO4, NH4Cl, and NaNO3 ⋅ Na2SO4 ⋅ H2O until reaching 68 % RH. The daily DRH happens at 71.3 ± 4.9 % and 67.1 ± 3.4 % for PM2.5 and PM2.5−10, respectively, as shown in Fig. S4b and c. In the OPC data analysis, an RH threshold of ≤ 50 % was applied to determine the sensitivity. At this threshold, PM2.5 particles have not yet deliquesced, and PM2.5−10 shows minimal volume growth, indicating the applicability of the selected RH threshold for sensitivity calculation. A DRH threshold of 70 % was applied to ensure sufficient data points for κ calculation but slightly lower than the DRH of PM2.5.
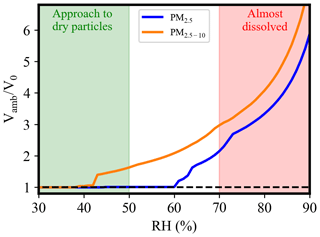
Figure 5The volume ratio of a given soluble composition as a function of RH under thermodynamic equilibrium calculated using E-AIM at 298.15 K (composition is the averaged IC data with a molarity ratio of Na+ : NH : Cl− : SO:NO as for PM2.5 and for PM2.5−10).
To assess the potential bias associated with the selected DRH threshold, Fig. S6 shows the HGF of mean soluble composition as a function of RH estimated using E-AIM. With Eq. (2) (without the Kelvin effect term) and the assumption of volume additivity between the particle and the water taken up, κ values derived using 70 % and 75 % thresholds show less than 1 % difference for PM2.5 and PM2.5−10 compositions but 13 % and 6 % less than that estimated from the composition calculation (Eq. 5) for PM2.5 and PM2.5−10, respectively. As the selected DRH threshold decreases, the derived κ decreases slightly due to the interference of adding data points with incompletely dissociated composition to the fitting analysis. However, the temporal composition variation in the applied OPC dataset (∼ 16 d of observation) might lead to a higher variation (Fig. S7), making the κ deviation due to the applied DRH threshold appear negligible in this study. Furthermore, the lower derived κ for E-AIM compared to the composition estimation is likely due to the applied individual κ values in composition estimation being based on CCN activation measurement, which was reported to be 10 % to 17 % higher than that derived from the growth factor analysis (Petters and Kreidenweis, 2007). During the particle growth process, the partial dissociation leads to the RH-dependent van't Hoff factor, as noted by Petters and Kreidenweis (2007). Similar findings were reported by Kreidenweis et al. (2008) regarding the differences in derived water contents between the κ-Köhler equation and E-AIM to be within ± 20 % under the condition of approximately 85 % RH, which might cause differences for κ evaluation. Overall, the differences in derived κ among methods are primarily due to the given hygroscopicity of chemical species, likely due to the fixed van't Hoff factor and the assumptions of volume additivity in the κ-Köhler equation.
3.4 Sensitivity of assumed parameters on derived hygroscopicity
For simplicity, κ was derived from OPC and BAM data without considering the Kelvin effect and under an assumed particle density. Neglecting the Kelvin effect may result in minor differences for particles larger than 100 nm under sub-saturated conditions (Pope et al., 2010; Topping et al., 2005; Crilley et al., 2018). To confirm the appropriateness, we assessed biases for particles at 0.1 and 1 µm without considering the Kelvin effect, as shown in Fig. S8. For particles with a κ value of 0.3 under RH ranging from 70 % to 95 %, the deviation of κ due to neglecting the Kelvin effect is −10 % for 0.1 µm particles and −1 % for 1 µm particles, decreasing with particle diameter. The particle diameter is overestimated under the same RH conditions because the positive Kelvin effect is ignored. To compensate for the deficiency in saturation, the balanced particle diameter needs to be larger with a more significant solute effect. However, the average mass-weighted mean diameter for PM2.5 is about 1.3 µm. Therefore, neglecting the Kelvin effect in our analysis has limited influence on the derived κ.
Furthermore, the derived κ from OPC data (using Eq. 3) and IC data (using Eq. 5) are notably influenced by the assumed particle density. Assuming that the undetermined composition mainly consists of secondary organic species with a density of 1.2 g cm−3, within the reported densities ranging from 0.9 to 1.6 g cm−3 depending on the formation process (Malloy et al., 2009; Kostenidou et al., 2007; Zelenyuk et al., 2008), along with the properties of analyzed soluble chemical species summarized in Table S2, the calculated densities are 1.42 ± 0.03 and 1.34 ± 0.05 g cm−3 for PM2.5 and PM2.5−10, respectively (Fig. S9). These calculated densities are about 15 % and 10 % higher than the assumed fixed density (1.2 g cm−3) for PM2.5 and PM2.5−10, respectively. Consequently, the derived κ from OPC data increases by approximately 17 % for PM2.5 and 9 % for PM2.5−10, while the derived κ from IC data is proportional to density (i.e., 15 % and 10 % for PM2.5 and PM2.5−10, respectively) as shown in Fig. 4b. This influence is particularly noticeable for components with a high portion of higher-density species, such as black carbon (a non-hygroscopic species with κ∼ 0) having a high density of about 1.8 g cm−3 (Park et al., 2004; Shiraiwa et al., 2008). Despite the potential uncertainties associated with particle density, the derived κ exhibits consistency between the OPC and IC analyses.
In this study, we evaluated the performances of home-built air quality box (AQB) systems equipped with low-cost sensors and focused on the ambient variability of particulate matter (PM) concentrations to derive the hygroscopicity of PM and the conversion to dry particle concentrations. The AQB systems revealed their effectiveness in capturing meteorological parameters and most pollutant concentrations with high correlations (r≥0.96) for temperature, relative humidity, CO, and Ox (O3+ NO2) and moderate correlations (r≥0.48) for NOx and PM, as compared to TW-EPA data. In the PM analysis, PM10 was divided into PM2.5 and PM2.5−10 to account for compositional heterogeneity among different particle sizes. Comparing the OPC-monitored ambient PM data and the BAM data (for dry particles) at RH ≤ 50 %, the derived sensitivity coefficients (α) for PM2.5−10 (10.58–12.37) were higher than those for PM2.5 (1.26–1.44), likely due to the significant sensitivity variation in the OPC over time. By considering hygroscopicity with the κ-Köhler equation and assuming a constant composition density for sensitivity-corrected OPC data, the derived dry particle mass concentrations show improved consistency with BAM data compared to the simple linear regression approach. The derived κ values range from 0.15 to 0.29 for PM2.5 and 0.05 to 0.13 for PM2.5−10, consistent with those from IC soluble composition analysis (0.14 to 0.27 for PM2.5 and 0.06 to 0.21 for PM2.5−10) and primarily influenced by the proportion of soluble components, ∼ 53 % in PM2.5 and ∼ 30 % in PM2.5−10. The sensitivity analysis of various parameters showed that the effects of the chosen deliquescence relative humidity (DRH) thresholds and Kelvin effect have a minor impact on κ values (less than 1 %). Conversely, recalculating particle densities for PM2.5 (1.42 ± 0.03 g cm−3) and PM2.5−10 (1.34 ± 0.07 g cm−3) led to higher κ values by approximately 17 % and 9 %, respectively, compared to the results assuming a density of 1.2 g cm−3. Overall, the AQB systems are potentially helpful in understanding the temporal and spatial variability of air quality by effectively monitoring pollutant concentrations and providing the capability for hygroscopicity derivation. This study also emphasizes the need for careful consideration of uncertainties and calibration techniques to interpret low-cost sensor data accurately in atmospheric research.
AQB | air quality box |
α | sensitivity coefficient |
BAM | beta attenuation mass monitor |
CCN | cloud condensation nuclei |
Damb | diameters of the ambient particulate matter |
Dd | diameters of the dry particulate matter |
DRH | deliquescence relative humidity |
E-AIM | Extended Aerosol Inorganics Model |
εi | volume fraction of i species |
HGF | hygroscopic growth factor |
IC | ion chromatography |
κ | hygroscopicity (single hygroscopicity |
parameter) | |
κi | hygroscopicity of i species |
ρd | density of dry aerosol particles |
ρw | density of liquid water |
MBAM | particulate matter mass concentrations |
measured by BAM | |
Md,derived | derived dry mass concentration |
Mw | molecular weight of water |
MOPC | particulate matter mass concentrations |
measured by optical particle counter | |
MAPE | mean absolute percentage error |
NMHC | non-methane hydrocarbons |
OPC | optical particle counter |
P | pressure |
PM | particulate matter |
PM2.5 | integrated fine particles with |
a diameter ≤ 2.5 µm | |
PM2.5−10 | coarse particles with a diameter in a range |
of 2.5 to 10 µm | |
R | gas constant (8.314 J mol−1 K−1) |
RH | relative humidity |
RI | refractive index |
RMSE | root mean squared error |
S | water saturation ratio |
surface tension of the particle | |
T | temperature |
TW-EPA | Taiwan Environmental Protection |
Administration | |
vi | volume of i species |
vtotal | total volume of particles |
The code is not publicly accessible, but readers can contact Hui-Ming Hung (hmhung@ntu.edu.tw) for more information. The observation data for AQBs and TW-EPA, the E-AIM model output, and the hygroscopicity derivation result used in this study can be accessed online at https://doi.org/10.5281/zenodo.13896790 (Huang et al., 2024).
The supplement related to this article is available online at: https://doi.org/10.5194/amt-17-6073-2024-supplement.
WCH carried out the calibration campaign, analyzed the data, and prepared the manuscript draft. HMH supervised the project, which included data discussion and manuscript editing. CWC and WCH designed the home-built AQB system and performed the database generation. SCCL supervised the field study in 2013 and conducted the aerosol composition analysis in 2021.
The contact author has declared that none of the authors has any competing interests.
Publisher’s note: Copernicus Publications remains neutral with regard to jurisdictional claims made in the text, published maps, institutional affiliations, or any other geographical representation in this paper. While Copernicus Publications makes every effort to include appropriate place names, the final responsibility lies with the authors.
We appreciate the Taiwan Environmental Protection Administration for providing the minute-averaged data of meteorological parameters and chemical species for calibration and comparison as well as Shih-Chieh Hsu at the Research Center for Environmental Changes, Academia Sinica, Taipei, for composition data of PM2.5 and PM10 in Kaohsiung (2013).
This research has been supported by the National Science and Technology Council (grant nos. 111-2111-M-002-009 and 112-2111-M-002-014).
This paper was edited by Albert Presto and reviewed by three anonymous referees.
Andreae, M. O. and Rosenfeld, D.: Aerosol–cloud–precipitation interactions, Part 1. The nature and sources of cloud-active aerosols, Earth-Sci. Rev., 89, 13–41, https://doi.org/10.1016/j.earscirev.2008.03.001, 2008.
Bian, Y. X., Zhao, C. S., Ma, N., Chen, J., and Xu, W. Y.: A study of aerosol liquid water content based on hygroscopicity measurements at high relative humidity in the North China Plain, Atmos. Chem. Phys., 14, 6417–6426, https://doi.org/10.5194/acp-14-6417-2014, 2014.
Brook, R. D., Rajagopalan, S., Pope, C. A., Brook, J. R., Bhatnagar, A., Diez-Roux, A. V., Holguin, F., Hong, Y., Luepker, R. V., Mittleman, M. A., Peters, A., Siscovick, D., Smith, S. C., Whitsel, L., and Kaufman, J. D.: Particulate Matter Air Pollution and Cardiovascular Disease, Circulation, 121, 2331–2378, https://doi.org/10.1161/CIR.0b013e3181dbece1, 2010.
Castell, N., Dauge, F. R., Schneider, P., Vogt, M., Lerner, U., Fishbain, B., Broday, D., and Bartonova, A.: Can commercial low-cost sensor platforms contribute to air quality monitoring and exposure estimates?, Environ. Int., 99, 293–302, https://doi.org/10.1016/j.envint.2016.12.007, 2017.
Chan, M. N. and Chan, C. K.: Mass transfer effects in hygroscopic measurements of aerosol particles, Atmos. Chem. Phys., 5, 2703–2712, https://doi.org/10.5194/acp-5-2703-2005, 2005.
Chen, C. L., Chen, T. Y., Hung, H. M., Tsai, P. W., Chou, C. C. K., and Chen, W. N.: The influence of upslope fog on hygroscopicity and chemical composition of aerosols at a forest site in Taiwan, Atmos. Environ., 246, 118150, https://doi.org/10.1016/j.atmosenv.2020.118150, 2021.
Chen, S. Y., Chan, C. C., and Su, T. C.: Particulate and gaseous pollutants on inflammation, thrombosis, and autonomic imbalance in subjects at risk for cardiovascular disease, Environ. Pollut., 223, 403–408, https://doi.org/10.1016/j.envpol.2017.01.037, 2017.
Clegg, S. L., Brimblecombe, P., and Wexler, A. S.: Thermodynamic model of the system H+-NH-Na+-SO-NO-Cl−-H2O at 298.15 K, J. Phys. Chem. A, 102, 2155–2171, https://doi.org/10.1021/jp973043j, 1998.
Concas, F., Mineraud, J., Lagerspetz, E., Varjonen, S., Liu, X. L., Puolamaki, K., Nurmi, P., and Tarkoma, S.: Low-Cost Outdoor Air Quality Monitoring and Sensor Calibration: A Survey and Critical Analysis, ACM Trans. Sens. Netw., 17, 1–44, https://doi.org/10.1145/3446005, 2021.
Crilley, L. R., Shaw, M., Pound, R., Kramer, L. J., Price, R., Young, S., Lewis, A. C., and Pope, F. D.: Evaluation of a low-cost optical particle counter (Alphasense OPC-N2) for ambient air monitoring, Atmos. Meas. Tech., 11, 709–720, https://doi.org/10.5194/amt-11-709-2018, 2018.
Dacunto, P. J., Klepeis, N. E., Cheng, K.-C., Acevedo-Bolton, V., Jiang, R.-T., Repace, J. L., Ott, W. R., and Hildemann, L. M.: Determining PM2.5 calibration curves for a low-cost particle monitor: common indoor residential aerosols, Environ. Sci., 17, 1959–1966, https://doi.org/10.1039/c5em00365b, 2015.
Demanega, I., Mujan, I., Singer, B. C., Andelkovic, A. S., Babich, F., and Licina, D.: Performance assessment of low-cost environmental monitors and single sensors under variable indoor air quality and thermal conditions, Build. Environ., 187, 107415, https://doi.org/10.1016/j.buildenv.2020.107415, 2021.
Di Antonio, A., Popoola, O. A. M., Ouyang, B., Saffell, J., and Jones, R. L.: Developing a Relative Humidity Correction for Low-Cost Sensors Measuring Ambient Particulate Matter, Sensors, 18, 2790, https://doi.org/10.3390/s18092790, 2018.
Formenti, P., Di Biagio, C., Huang, Y., Kok, J., Mallet, M. D., Boulanger, D., and Cazaunau, M.: Look−up tables resolved by complex refractive index to correct particle sizes measured by common research−grade optical particle counters, Atmos. Meas. Tech. Discuss. [preprint], https://doi.org/10.5194/amt-2021-403, 2021.
Gillooly, S. E., Zhou, Y., Vallarino, J., Chu, M. T., Michanowicz, D. R., Levy, J. I., and Adamkiewicz, G.: Development of an in-home, real-time air pollutant sensor platform and implications for community use, Environ. Pollut., 244, 440–450, https://doi.org/10.1016/j.envpol.2018.10.064, 2019.
Glockler, G.: The ionization potential of methane, J. Am. Chem. Soc., 48, 2021–2026, https://doi.org/10.1021/ja01419a002, 1926.
Hagan, D. H. and Kroll, J. H.: Assessing the accuracy of low-cost optical particle sensors using a physics-based approach, Atmos. Meas. Tech., 13, 6343–6355, https://doi.org/10.5194/amt-13-6343-2020, 2020.
Hamanaka, R. B. and Mutlu, G. M.: Particulate Matter Air Pollution: Effects on the Cardiovascular System, Front. Endocrinol., 9, 680, https://doi.org/10.3389/fendo.2018.00680, 2018.
Hering, S. and Cass, G.: The Magnitude of Bias in the Measurement of PM25 Arising from Volatilization of Particulate Nitrate from Teflon Filters, J. Air Waste Manag. Assoc., 49, 725–733, https://doi.org/10.1080/10473289.1999.10463843, 1999.
Heus, T., van Heerwaarden, C. C., Jonker, H. J. J., Siebesma, A. P., Axelsen, S., van den Dries, K., Geoffroy, O., Moene, A. F., Pino, D., de Roode, S. R., and de Arellano, J. V. G.: Formulation of the Dutch Atmospheric Large-Eddy Simulation (DALES) and overview of its applications, Geosci. Model Dev., 3, 415–444, https://doi.org/10.5194/gmd-3-415-2010, 2010.
Huang, W.-C., Hung, H.-M., Chu, C.-W., Hwang, W.-C., and Lung, S.-C. C.: Deriving the hygroscopicity of ambient particles using low-cost optical particle counters, Zenodo [data set], https://doi.org/10.5281/zenodo.13896790, 2024.
Hung, H.-M., Hsu, C.-H., Lin, W.-T., and Chen, Y.-Q.: A case study of single hygroscopicity parameter and its link to the functional groups and phase transition for urban aerosols in Taipei City, Atmos. Environ., 132, 240–248, https://doi.org/10.1016/j.atmosenv.2016.03.008, 2016.
Kaliszewski, M., Włodarski, M., Młyńczak, J., and Kopczyński, K.: Comparison of Low-Cost Particulate Matter Sensors for Indoor Air Monitoring during COVID-19 Lockdown, Sensors, 20, 7290, https://doi.org/10.3390/s20247290, 2020.
Karagulian, F., Barbiere, M., Kotsev, A., Spinelle, L., Gerboles, M., Lagler, F., Redon, N., Crunaire, S., and Borowiak, A.: Review of the Performance of Low-Cost Sensors for Air Quality Monitoring, Atmosphere, 10, 506, https://doi.org/10.3390/atmos10090506, 2019.
Kostenidou, E., Pathak, R. K., and Pandis, S. N.: An algorithm for the calculation of secondary organic aerosol density combining AMS and SMPS data, Aerosol Sci. Technol., 41, 1002–1010, https://doi.org/10.1080/02786820701666270, 2007.
Kreidenweis, S. M., Petters, M. D., and DeMott, P. J.: Single-parameter estimates of aerosol water content, Environ. Res. Lett., 3, 035002, https://doi.org/10.1088/1748-9326/3/3/035002, 2008.
Li, J., Liu, W. Y., Castarede, D., Gu, W. J., Li, L. J., Ohigashi, T., Zhang, G. Q., Tang, M. J., Thomson, E. S., Hallquist, M., Wang, S., and Kong, X. R.: Hygroscopicity and Ice Nucleation Properties of Dust/Salt Mixtures Originating from the Source of East Asian Dust Storms, Front. Environ. Sci., 10, 897127, https://doi.org/10.3389/fenvs.2022.897127, 2022.
Lohmann, U. and Feichter, J.: Global indirect aerosol effects: a review, Atmos. Chem. Phys., 5, 715–737, https://doi.org/10.5194/acp-5-715-2005, 2005.
Luo, Q., Hong, J., Xu, H., Han, S., Tan, H., Wang, Q., Tao, J., Ma, N., Cheng, Y., and Su, H.: Hygroscopicity of amino acids and their effect on the water uptake of ammonium sulfate in the mixed aerosol particles, Sci. Total Environ., 734, 139318, https://doi.org/10.1016/j.scitotenv.2020.139318, 2020.
Malloy, Q. G. J., Nakao, S., Qi, L., Austin, R., Stothers, C., Hagino, H., and Cocker, D. R.: Real-Time Aerosol Density Determination Utilizing a Modified Scanning Mobility Particle Sizer – Aerosol Particle Mass Analyzer System, Aerosol Sci. Technol., 43, 673–678, https://doi.org/10.1080/02786820902832960, 2009.
Mead, M. I., Popoola, O. A. M., Stewart, G. B., Landshoff, P., Calleja, M., Hayes, M., Baldovi, J. J., McLeod, M. W., Hodgson, T. F., Dicks, J., Lewis, A., Cohen, J., Baron, R., Saffell, J. R., and Jones, R. L.: The use of electrochemical sensors for monitoring urban air quality in low-cost, high-density networks, Atmos. Environ., 70, 186–203, https://doi.org/10.1016/j.atmosenv.2012.11.060, 2013.
Pöschl, U., Martin, S. T., Sinha, B., Chen, Q., Gunthe, S. S., Huffman, J. A., Borrmann, S., Farmer, D. K., Garland, R. M., Helas, G., Jimenez, J. L., King, S. M., Manzi, A., Mikhailov, E., Pauliquevis, T., Petters, M. D., Prenni, A. J., Roldin, P., Rose, D., Schneider, J., Su, H., Zorn, S. R., Artaxo, P., and Andreae, M. O.: Rainforest aerosols as biogenic nuclei of clouds and precipitation in the Amazon, Science, 329, 1513–1516, https://doi.org/10.1126/science.1191056, 2010.
Park, K., Kittelson, D. B., Zachariah, M. R., and McMurry, P. H.: Measurement of Inherent Material Density of Nanoparticle Agglomerates, J. Nanopart. Res., 6, 267–272, https://doi.org/10.1023/B:NANO.0000034657.71309.e6, 2004.
Petters, M. D. and Kreidenweis, S. M.: A single parameter representation of hygroscopic growth and cloud condensation nucleus activity, Atmos. Chem. Phys., 7, 1961–1971, https://doi.org/10.5194/acp-7-1961-2007, 2007.
Pope, F. D., Dennis-Smither, B. J., Griffiths, P. T., Clegg, S. L., and Cox, R. A.: Studies of Single Aerosol Particles Containing Malonic Acid, Glutaric Acid, and Their Mixtures with Sodium Chloride. I. Hygroscopic Growth, J. Phys. Chem. A, 114, 5335–5341, https://doi.org/10.1021/jp100059k, 2010.
Pringle, K. J., Tost, H., Pozzer, A., Pöschl, U., and Lelieveld, J.: Global distribution of the effective aerosol hygroscopicity parameter for CCN activation, Atmos. Chem. Phys., 10, 5241–5255, https://doi.org/10.5194/acp-10-5241-2010, 2010.
Rosenfeld, D., Andreae, M. O., Asmi, A., Chin, M., de Leeuw, G., Donovan, D. P., Kahn, R., Kinne, S., Kivekas, N., Kulmala, M., Lau, W., Schmidt, K. S., Suni, T., Wagner, T., Wild, M., and Quaas, J.: Global observations of aerosol-cloud-precipitation-climate interactions, Rev. Geophys., 52, 750–808, https://doi.org/10.1002/2013rg000441, 2014.
Salvador, C. M. and Chou, C. C. K.: Analysis of semi-volatile materials (SVM) in fine particulate matter, Atmos. Environ., 95, 288–295, https://doi.org/10.1016/j.atmosenv.2014.06.046, 2014.
Samad, A., Mimiaga, F. E. M., Laquai, B., and Vogt, U.: Investigating a Low-Cost Dryer Designed for Low-Cost PM Sensors Measuring Ambient Air Quality, Sensors, 21, 804, https://doi.org/10.3390/s21030804, 2021.
Shiraiwa, M., Kondo, Y., Moteki, N., Takegawa, N., Sahu, L. K., Takami, A., Hatakeyama, S., Yonemura, S., and Blake, D. R.: Radiative impact of mixing state of black carbon aerosol in Asian outflow, J. Geophys. Res.-Atmos., 113, D24210, https://doi.org/10.1029/2008JD010546, 2008.
Sá, J. P., Alvim-Ferraz, M. C. M., Martins, F. G., and Sousa, S. I. V.: Application of the low-cost sensing technology for indoor air quality monitoring: A review, Environ. Technol. Innov., 28, 102551, https://doi.org/10.1016/j.eti.2022.102551, 2022.
Tang, M. J., Zhang, H. H., Gu, W. J., Gao, J., Jian, X., Shi, G. L., Zhu, B. Q., Xie, L. H., Guo, L. Y., Gao, X. Y., Wang, Z., Zhang, G. H., and Wang, X. M.: Hygroscopic Properties of Saline Mineral Dust From Different Regions in China: Geographical Variations, Compositional Dependence, and Atmospheric Implications, J. Geophys. Res.-Atmos., 124, 10844–10857, https://doi.org/10.1029/2019jd031128, 2019.
Topping, D. O., McFiggans, G. B., and Coe, H.: A curved multi-component aerosol hygroscopicity model framework: Part 1 – Inorganic compounds, Atmos. Chem. Phys., 5, 1205–1222, https://doi.org/10.5194/acp-5-1205-2005, 2005.
Venkatraman Jagatha, J., Klausnitzer, A., Chacón-Mateos, M., Laquai, B., Nieuwkoop, E., van der Mark, P., Vogt, U., and Schneider, C.: Calibration Method for Particulate Matter Low-Cost Sensors Used in Ambient Air Quality Monitoring and Research, Sensors, 21, 3960, https://doi.org/10.3390/s21123960, 2021.
Wu, C. F., Kuo, I. C., Su, T. C., Li, Y. R., Lin, L. Y., Chan, C. C., and Hsu, S. C.: Effects of Personal Exposure to Particulate Matter and Ozone on Arterial Stiffness and Heart Rate Variability in Healthy Adults, Am. J. Epidemiol., 171, 1299–1309, https://doi.org/10.1093/aje/kwq060, 2010.
Zelenyuk, A., Yang, J., Song, C., Zaveri, R. A., and Imre, D.: A new real-time method for determining particles' sphericity and density: application to secondary organic aerosol formed by ozonolysis of alpha-pinene, Environ. Sci. Technol., 42, 8033–8038, https://doi.org/10.1021/es8013562, 2008.