the Creative Commons Attribution 4.0 License.
the Creative Commons Attribution 4.0 License.
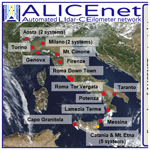
ALICENET – an Italian network of automated lidar ceilometers for four-dimensional aerosol monitoring: infrastructure, data processing, and applications
Annachiara Bellini
Henri Diémoz
Luca Di Liberto
Gian Paolo Gobbi
Alessandro Bracci
Ferdinando Pasqualini
Francesca Barnaba
Vertically resolved information on aerosol particles represents a key aspect in many atmospheric studies, including aerosol–climate interactions and aerosol impacts on air quality and human health. This information is primarily derived by lidar active remote sensing, in particular with extensive networks currently in operation worldwide. In Italy, the Institute of Atmospheric Sciences and Climate (ISAC) of the National Research Council (CNR) established the ALICENET network of automated lidar ceilometers (ALCs) in 2015. Since then, ALICENET has grown as a cooperative effort of Italian institutions dealing with atmospheric science and monitoring, and it currently includes instruments run by regional environmental protection agencies, universities, research centres, and private companies. In the current configuration, the network makes use of both single-channel ALCs and dual-channel, polarisation-sensitive-system ALCs (referred to as PLCs). The systems operate in very different environments (urban, coastal, mountainous, and volcanic areas) from northern to southern Italy, thus allowing the continuous monitoring of the aerosol vertical distribution across the country. ALICENET also contributes to the EUMETNET programme E-PROFILE, filling an Italian observational gap compared to other EU member states, which generally run extended ALC networks through national meteorological services. In this work, we present the ALICENET infrastructure and the specifically developed data processing centralised at CNR-ISAC, converting raw instrumental data into quantitative, quality-controlled information on aerosol properties ranging from attenuated backscatter to aerosol mass and vertical stratifications. This setup allows us to get insights into the 4D aerosol field over Italy with applications from near-real-time monitoring to long-term analyses, examples of which are reported in this work. Specific comparisons of the ALICENET products to independent measurements obtained with different techniques, such as particulate matter (PM) concentrations from in situ samplers and aerosol optical depth (AOD) from sun photometers, are also included here, revealing the good performances of the ALICENET algorithms. Overall, ALICENET represents a valuable resource to extend the current aerosol observational capabilities in Italy and in the Mediterranean area, and it contributes to bridging the gap between atmospheric science and its application to specific sectors, among which are air quality, solar energy, and aviation safety.
- Article
(10033 KB) - Full-text XML
-
Supplement
(5216 KB) - BibTeX
- EndNote
Aerosols influence the Earth system and human life in several ways. They affect the planetary radiation budget directly by extinction of solar radiation and indirectly by modification of cloud properties and lifetime, thus also influencing the hydrological cycle (Intergovernmental Panel on Climate Change, 2022). Atmospheric particles of both anthropogenic origin and natural origin contribute to deteriorating air quality (AQ), making them a main concern for human health (WHO, 2021). Furthermore, high aerosol loads reduce visibility and during major events, such as desert dust storms, volcanic eruptions, and wide forest fires, can damage aircraft engines, thus representing a threat to the aviation sector (e.g. Flentje et al., 2010; Papagiannopoulos et al., 2020; Brenot et al., 2021; Monteiro et al., 2022; Ryder et al., 2024). The vertical aerosol distribution is key to correctly quantifying aerosol effects on climate and human activities. In fact it impacts radiative transfer and atmospheric heating rates (e.g. Fasano et al., 2021; Fountoulakis et al., 2022), aerosol–cloud–precipitation interactions (e.g. Napoli et al., 2022), particle dispersion and transformation processes (e.g. Curci et al., 2015; Gobbi et al., 2019; Diémoz et al., 2019a, b), and the state of high-altitude pristine environments (e.g. Balestrini et al., 2024).
Active remote sensing through lidar sensors is a very efficient tool to provide range-resolved, accurate profiles of aerosol properties (e.g. Gobbi and Barnaba, 2002; Tesche et al., 2009; Ansmann et al., 2011). In the last decades, both ground-based and space-based lidar systems have been developed and widely used for scientific research purposes, and they are expected to play an increasingly important role in climate and public health studies (Remer et al., 2024). From space, the recently dismissed NASA–CNES CALIOP sensor aboard CALIPSO (Winker et al., 2010) provided one of the most valuable, vertically resolved, global aerosol datasets (2006–2023), which is expected to be extended by the just-launched ESA–JAXA mission EarthCARE (Earth Cloud, Aerosol and Radiation Explorer, Illingworth et al., 2015). From the ground, lidar remote sensing is often performed in the framework of globally distributed research networks. In Europe, a wide aerosol research lidar network (EARLINET, Pappalardo et al., 2014) has been developed in the last decade, and it is currently an important component of the European Strategy Forum on Research Infrastructures and Aerosol, Clouds, and Trace Gases Research Infrastructure (ESFRI–ACTRIS). Such a research-oriented network runs high-power, multi-wavelength Raman lidar systems, which were not designed for monitoring purposes. In fact, EARLINET lidar measurements are generally not performed continuously, and the spatial density of the measuring sites is still insufficient to capture the high spatio-temporal variability characterising aerosols.
In the last 2 decades, the use of automatic, low-energy, affordable, and robust single-channel elastic lidars, referred to as automated lidar ceilometers (ALCs), has increased. These systems emit single-wavelength laser pulses, mostly in the infrared range, and measure the time-dependent (and thus range-dependent) radiation that is elastically backscattered by atmospheric components (molecules, aerosols, cloud droplets and/or ice crystals). ALCs were originally conceived to only monitor the “cloud ceiling”, but recent technological improvements enabled ALCs to provide continuous information on aerosol profiles within the troposphere, including the boundary layer region, albeit with a lower signal-to-noise ratio (SNR) compared to high-power research lidars. This favoured the development of extended networks of automatic low-power lidars and ALCs worldwide, among which are the NASA Micro-Pulse Lidar Network (MPLnet; Welton et al., 2018), the US Environmental Protection Agency (EPA) network for Photochemical Assessment Monitoring Stations (PAMS; Caicedo et al., 2020), and the Asian dust and aerosol lidar observation network (ADnet; Shimizu et al., 2016). In Europe, several member states currently run dense ALC networks for monitoring purposes, mostly managed by national meteorological services, such as the DWD in Germany (Flentje et al., 2021) and the Met Office in the UK (Osborne et al., 2022). Recently, ACTRIS started considering automatic low-power lidars as useful tools within its aerosol remote sensing (ARS) component, although these systems have not yet been included in the relevant minimum or optimal setups recommended by ACTRIS ARS (https://www.actris.eu/topical-centre/cars/announcements-resources/documents, last access: 25 July 2024). Most ALC observations at the EU level are currently collected and further exploited in the framework of the E-PROFILE programme run by the European Meteorological Services Network (EUMETNET; http://www.eumetnet.eu/activities/observations-programme/current-activities/e-profile/, last access: 25 July 2024).
The development of such an extended ALC observational capacity was further accelerated after the eruption of the Icelandic volcano Eyjafjallajökull in 2010, which disrupted air transport due to the lack of readily accessible information on the horizontal and vertical displacement of the aerosol plume (Flentje et al., 2010; Mortier et al., 2013). Moreover, ALCs have been proven to be extremely useful in support of AQ evaluations, providing information on the vertical dilution of pollutants, transboundary transport of particles from medium-to-long-range distances (e.g. Rizza et al., 2017; Bucci et al., 2018; Diémoz et al., 2019a, b), secondary aerosol formation (e.g. Curci et al., 2015), or even particles reaching the boundary layer through evaporating rain (virgas, e.g. Karle et al., 2023). However, with few exceptions, standard air quality monitoring networks (AQMNs) in the EU currently lack such profiling capability. The feasibility of filling this gap is currently explored in the framework of the EC H2020 project RI-URBANS (https://riurbans.eu, last access: 25 July 2024), aiming at the development of service tools in support to AQ monitoring in European urban areas and pollution hotspots. In fact, the current ALC technology has been proven to be mature enough to allow a robust retrieval of the planetary boundary layer height (Kotthaus et al., 2023), a key parameter in AQ, and evaluations are currently ongoing at the EU level to assess the readiness of ALC-based retrievals for quantitative particulate matter (PM) monitoring (e.g. Shang et al., 2021; Osborne, 2024). The recently completed EC COST Action PROBE (PROfiling the atmospheric Boundary layer at European scale; Cimini et al., 2020; Kotthaus and Bravo Aranda, 2024) supported by the European Cooperation in Science and Technology (COST) was key to promoting and coordinating such activities, which are now being further explored within the E-PROFILE and ACTRIS communities.
In Italy, an effort to coordinate ALC activities at the national level and contribute to E-PROFILE was made by the National Research Council Institute of Atmospheric Sciences and Climate (CNR-ISAC), which set up the ALICENET network in 2015 (https://www.alice-net.eu/, last access: 25 July 2024), filling an observational gap over Italy.
The ALICENET measurements are particularly relevant for the Mediterranean area, which is a climatic hotspot (Intergovernmental Panel on Climate Change, 2022) affected by a complex mixture of atmospheric circulations (e.g. Lelieveld et al., 2002) and aerosol types (e.g. Barnaba and Gobbi, 2004; Di Iorio et al., 2009; Andrés Hernández et al., 2022). ALICENET is conceived as a cooperative, open consortium with contributions from several collaborating partners, among which are regional environmental protection agencies, universities, research institutions, and private companies.
The present work aims at presenting the ALICENET infrastructure and its data-processing chain, designed to derive quantitative and quality-checked vertically resolved information on aerosol properties and layering. The ALICENET data processing, centralised at CNR-ISAC, allows the homogeneous retrieval of aerosol properties from northern to southern Italy. It is based on specifically developed algorithms, benefiting from past and ongoing collaborations with the EU ALC community, particularly in the framework of the EC COST Actions TOPROF (2013–2016) and PROBE (2019–2024), the EU H2020 project RI-URBANS (2021–2015), and the E-PROFILE initiative (2019–2023, 2024–2028).
This work is organised as follows. Section 2 describes the ALICENET infrastructure. Section 3 introduces the main data-processing steps and includes different examples of the relevant ALICENET products and accuracy. To facilitate the reading, the detailed technical aspects of each processing step and quality control (QC) procedures are included in Sects. S1–S6 in the Supplement, targeted at readers interested in a deep understanding of the processing chain and possibly in reproducing it. Section 4 shows three examples of the near-real-time ALICENET monitoring capability, while Sect. 5 summarises the ALICENET achievements and some foreseen future developments within the network.
The ALICENET stations are geographically distributed from the north to the south of the Italian Peninsula as shown in Fig. 1. The network configuration allows the monitoring of aerosol vertical profiles over a wide range of environmental and atmospheric conditions (e.g. urban, coastal, and mountain) within the Mediterranean area. In fact, some stations are located in highly anthropised areas, such as those in the Po Valley and main urban/industrial sites in Italy (Milan, Genoa, Turin, Florence, Rome, Taranto); some operate in coastal sites (e.g. Genoa, Taranto, Lamezia Terme, Messina, Capo Granitola, Catania); and others operate at elevated (> 550 m a.s.l.) stations (Aosta, Mt Cimone, Potenza, Mt Etna). Most sites are frequently impacted by desert dust advections, which are particularly relevant in central and southern Italy (e.g. Barnaba et al., 2017; Gobbi et al., 2019; Barnaba et al., 2022), and by both short- and long-range transport of biomass burning plumes (e.g. Barnaba et al., 2011). Volcanic plumes are also registered in the southernmost ALICENET sites, mainly in the five stations located at the foothills of the Mt Etna volcano and in the Messina and Lamezia Terme stations, due to their proximity to the other active sicilian volcano of Stromboli.
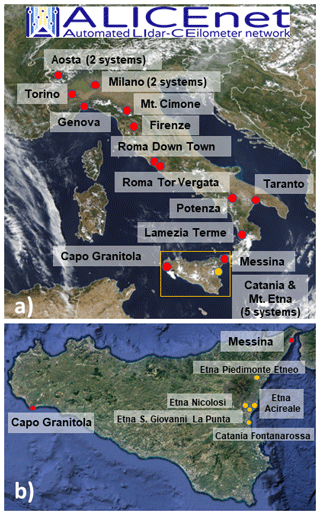
Figure 1Location and naming of the ALICENET stations (a, bullets). The yellow rectangle over Sicily in panel (a) is enlarged in panel (b) to show location of the five stations in the Mt Etna volcano area – from the northern to the southern foothills down to the city of Catania. Background map credits: (a) Suomi NPP VIIRS of NASA–NOAA and (b) © Google Maps.
For homogeneity of operations, since the beginning of the ALICENET activities (set as 1 January 2016), it has been agreed to operate standardised systems across the network, choosing the ones that allow us to accurately probe at least up to the middle troposphere, as well as for calibration purposes (e.g. Wiegner et al., 2014; see also Sect. 3.2). The single-channel, bistatic CHM15k instruments manufactured by Lufft (formerly Jenoptik ESW and now OTT HydroMet) were selected for this purpose. These are bistatic ALCs with a Nd:YAG solid-state laser emitting linearly polarised light at 1064 nm, with a 5–7 kHz repetition rate, a maximum vertical resolution of 5 m, and a maximum range of 15 km. The only exception in this instrumental setup was a modified-CHM15k prototype with polarisation-sensitive capabilities designed and developed in 2013 by Jenoptik ESW in collaboration with CNR-ISAC in the framework of the EC Life+ DIAPASON project (Gobbi et al., 2019). This first-ever polarisation-sensitive ALC (hereafter PLC) was conceived to explore the possibility of producing an affordable, robust system to be widely used in the identification and profiling of non-spherical (e.g. mineral dust) aerosol layers. The prototype PLC was tested in Rome (Italy), where it has been operating successfully since then (e.g. Gobbi et al., 2019; Andrés Hernández et al., 2022), but was never marketed by Lufft. More recently, PLC systems have been made available on the market by Vaisala (CL61 systems, operating at 910 nm) and, due to the important capability of such instruments to discriminate particle sphericity/non-sphericity, these are being progressively included in ALICENET.
For both CHM15k ALCs and CL61 PLCs, the signal is characterised by high temporal and vertical resolution, with some variability depending on the system type and configuration (e.g. in ALICENET the CHM15k standard configuration implies a vertical and temporal resolution of 15 m and 15 s, respectively). A summary table with details on the ALICENET sites and instrumentation operating therein is provided in Table 1. It includes the beginning of operations in each site of the ALICENET network (joining date) or the operating period for those systems no longer active. Some systems joined the network very recently and are thus indicated as “ready to go” as instrumental setup and data transfer to the ALICENET database is currently in progress.
The ALICENET data-processing chain is summarised in Fig. 2, with the main inputs and outputs. It starts with generation of standardised and harmonised data files from instrumental raw data (using the raw2l1 tool, https://gitlab.in2p3.fr/ipsl/sirta/raw2l1, last access: 25 July 2024) and then proceeds with pre-processing and calibration procedures, the inversion of the ALC signal into aerosol properties, and the detection of aerosol layers. It is convenient to first introduce the main equations and variables used in the description of the different steps.
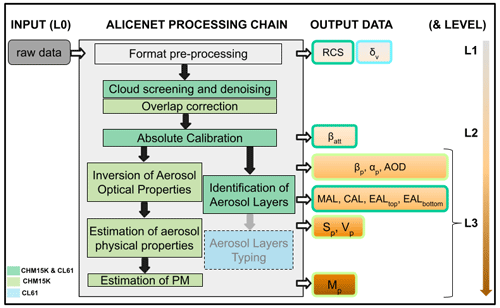
Figure 2Scheme of the ALICENET processing chain from the raw (L0) data to the aerosol products (L1–L3). The different colours in the processing box are used to indicate inversion steps valid for CHM15k (light green), CL61 (cyan), or both (dark green) systems. This same colour code (bounding box) is used for relevant output data products, which are further coloured from light to dark orange, indicating processing level from the more basic L1 quantities (range-corrected signal, RCS, and depolarisation, δv, profiles) to the L2 total attenuated backscatter (βatt) and the L3 aerosol optical (particle backscatter, βp, and extinction, αp) and physical (particle surface area, Sp; volume, Vp; and mass concentrations, Mp or PM) properties plus vertical layering (mixed, continuous, and elevated aerosol layers – MAL, CAL, and EALs, respectively).
As in any elastic backscatter lidar, the raw signal P(r,t) recorded by the ALC is a function of the distance from the emitter (range, r) and of the observation time t and can be described through the lidar equation:
Equation (1) includes the particle (p) and molecule (m) backscatter (β) and extinction (α) coefficients at the laser wavelength, as well as some instrumental factors, embedded into the instrument-specific calibration coefficient CL. Furthermore, particularly for bistatic systems (i.e. the CHM15k), measurements in the near range (generally < 500–700 m) are affected by signal losses due to the incomplete superposition (overlap) of the laser beam and the receiver field of view. The term Ovl in Eq. (1) therefore indicates the instrument-specific overlap function used to correct the signal loss in the near range. Equation (1) allows us to simply derive the total (i.e. aerosol + molecules) attenuated backscatter, βatt, as follows:
The complete ALICENET data-processing chain (Fig. 2) includes pre-processing procedures (namely cloud screening, denoising, and overlap correction; Sect. 3.1), the absolute calibration (to determine CL and, in turn, βatt; Sect. 3.2), the quantitative retrieval of aerosol optical (βp and αp) and physical (surface area, Sp; volume, Vp; and mass concentrations, Mp or PM) properties (Sect. 3.3) using an ALICENET original approach, and the detection of aerosol layers (mixed, continuous, and elevated aerosol layers – MAL, CAL, and EALs, respectively) through the ALICENET automatic aerosol layer detection algorithm (ALADIN; Sect. 3.4). The full processing chain is currently applied to CHM15k since, as mentioned above, these systems were the ones first implemented in the network. A similar scheme is under development for CL61 systems, for which data processing is currently limited to the cloud screening and denoising, the absolute calibration, and the detection of aerosol layers.
The ALICENET processing chain is completely automatic and allows continuous monitoring of the aerosol field over Italy, with L1/L2 data visualisation accessible in near real time through a dedicated website (https://www.alice-net.eu/, last access: 25 July 2024). Selected examples of this monitoring capability are provided in Sect. 4. The more advanced, quantitative retrieval of aerosol properties and layering (L3 products) is currently performed in post-processing and is planned to be released in the future through the ALICENET website.
3.1 Pre-processing
After the input data format harmonisation, the first pre-processing steps are aimed at avoiding cloud, precipitation, and noise contamination in aerosol retrievals (Sect. 3.1.1). Then data need to be corrected for overlap artefacts (Sect. 3.1.2) before proceeding with the determination of the instrument-specific calibration coefficient (Sect. 3.2). The way these preliminary steps are performed within ALICENET is described hereafter.
3.1.1 Cloud screening and denoising
At the ALC laser wavelengths, clouds generally produce complete extinction of the laser beam above the cloud base. Only in the event of optically thin clouds is the laser beam partially transmitted above the cloud base, although in most cases the return signal has an SNR that is too low to be employed for aerosol retrievals. The cloud screening applied to the ALICENET data exploits the cloud base height identified by the ALC firmware, with additional requirements to avoid the presence of cloud droplets frequently observed below the cloud base. Technical details of this procedure are reported in Sect. S1.
Cloud-screened profiles are then downscaled and denoised to improve the accuracy of the aerosol retrievals. Indeed, as mentioned above, the ALC signal is generally collected with high temporal and vertical resolution and features a decrease in the SNR along the profile. Denoising is performed by computing the signal mean and standard deviation over specific time and range windows and by filtering those data where the SNR (defined as the ratio between the mean and the standard deviation) is below a given threshold. A minimum SNR of 20 % is generally set for aerosol retrievals within ALICENET. The temporal resolution of the downscaled data is tuned depending on the timescales of the processes to be investigated. It may range from 1 min for the investigation of boundary layer dynamics up to 3 h for the identification of aerosol-loaded/aerosol-free regions in the upper troposphere, such as within the absolute calibration procedure.
3.1.2 Overlap correction
For bistatic systems such as CHM15k, an overlap correction of the signal in the near range is required (see Eq. 1). This is particularly important when ALC data are used for surface AQ applications and especially in those conditions in which particulate matter is confined to the lowermost atmospheric levels. An instrument-specific overlap function accounting for signal losses is generally provided by the manufacturer (Ovlman(r)). However, it has been demonstrated that changes in the instrument sensitivity require the use of an instrument-specific, temperature-dependent overlap correction. Within ALICENET, the derivation of such an overlap correction is largely based on the procedure developed by Hervo et al. (2016). Full details on its implementation in ALICENET, including additional quality control and quality assurance criteria (QC QA.OVL) added to the Hervo et al. (2016) procedure, are described in Sect. S2. The result is an instrument-, range- and temperature-dependent overlap model Ovlmodel(r,T) to be used in Eq. (1).
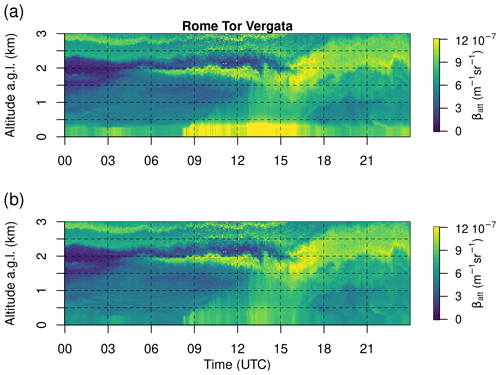
Figure 3Overlap-corrected ALC profiles using (a) the manufacturer overlap function and (b) the ALICENET overlap correction. Data refer to the ALICENET Rome Tor Vergata site on 12 August 2019.
Figure 3 shows an example of the application of the overlap model on ALC data collected in Rome Tor Vergata on 12 August 2019. This date was selected because of the high diurnal variation (15 K) of the instrument internal temperature. In Fig. 3, the continuous (24 h) βatt profiles derived using both the manufacturer overlap function (panel a) and the ALICENET overlap model (panel b) are shown. It is evident that the temperature-dependent overlap model is effective in correcting the false gradient and the aerosol overestimation in the lowermost 500 m coming from the manufacturer function.
A further attempt to evaluate the ability of this overlap-correction procedure to provide reliable results was conducted in the ALICENET mountain site of Aosta by exploiting the clean, nearly molecular conditions often registered at this Alpine station. In fact, due to its location, Aosta is frequently characterised by relatively low aerosol concentrations in the lowermost levels, in particular during föhn events (e.g. Mira-Salama et al., 2008). This makes it possible to compare the overlap-corrected βatt profiles with a theoretical molecular profile down to the lowermost levels. To perform this exercise, föhn-related, aerosol-free conditions of 3 to 6 h were identified by exploiting multi-sensor aerosol datasets (namely surface PM10 concentrations measured by an optical particle counter, OPC, and sun-photometer-derived aerosol optical depth, AOD) and meteorological parameters (wind, pressure, relative humidity) from the AQMN of ARPA Valle d'Aosta (Diémoz et al., 2021). For each of these selected cases, the mean βatt profiles retrieved using both the manufacturer correction and the ALICENET overlap correction were compared with a theoretical molecular profile. Figure 4 shows results for two cases (referring to 25 May and 6 October 2021) characterised by different values of the instrument internal temperature (308 and 292 K, respectively) and very low aerosol loads both at the surface (PM10 < 6 and 5 µg m−3, respectively) and along the atmospheric column (AOD at 1020 nm < 0.04 and 0.03, respectively).
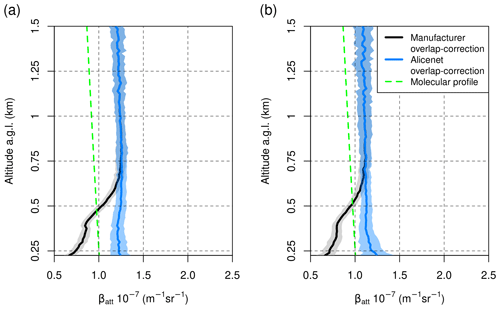
Figure 4βatt profiles at 1064 nm derived using the manufacturer overlap function (black line) and the ALICENET overlap correction (blue line) in two nearly molecular conditions registered in Aosta on (a) 25 May 2021 (05:00–08:00 UTC) and (b) 6 October 2021 (09:00–12:00 UTC). The shaded areas represent the βatt standard deviations within the selected time windows. A reference, molecular-only βatt profile is also reported (green line).
Overall, the results show that while the manufacturer overlap function is unable to properly account for signal losses and leads to unphysical values lower than the molecular profile in the firsts 750 m, the βatt profiles retrieved using the ALICENET overlap correction reasonably approach the nearly homogeneous, nearly molecular theoretical profiles expected in the selected episodes down to the ground.
3.2 Absolute calibration
The aim of the absolute calibration is the derivation of the calibration coefficient CL (see Eq. 1), which is required to convert the ALC signal into quantitative aerosol information. The ALICENET calibration procedure is based on the comparison of the pre-processed ALC signal with a theoretical molecular profile in aerosol-free atmospheric regions (Rayleigh calibration; Klett, 1985), typically in the middle troposphere. The procedure, which is fully automatic, is made in two steps: (a) the search for the best-suitable molecular window and (b) the computation of the calibration coefficient. It was built on the E-PROFILE algorithm, although some specificities and quality controls (QC.CAL) were introduced in both steps. A full description of the technical implementation of these steps is given in Sect. S3.
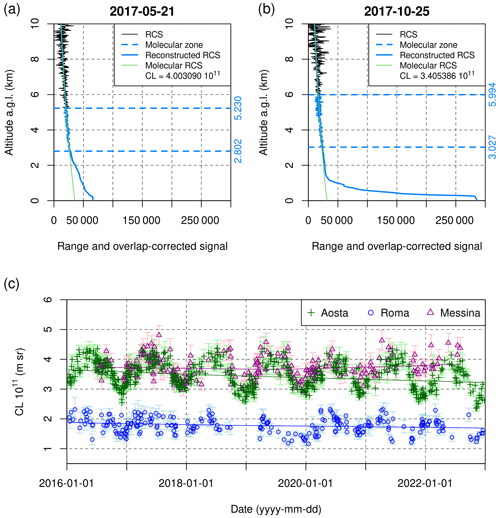
Figure 5(a, b) Examples of the application of the ALICENET calibration procedure, referring to the nighttime range-corrected signals from the Aosta CHM15k on 21 May and 25 October 2017, with the selected molecular windows and derived calibration coefficients (CL). (c) Multi-annual (2016–2022) time series of CL derived for the CHM15k systems operating in Aosta, Rome, and Messina, as well as the associated Loess fits (lines) used to derive the CL values used in the operational, year-round data inversions.
In Fig. 5, we show two examples of successful calibrations (Fig. 5a, b) and the multi-annual record of CL (Fig. 5c) derived from three ALICENET systems in northern, central, and southern Italy (Aosta, Rome, and Messina, respectively). Figure 5a and b refer to the ALICENET calibrations of the CHM15k in Aosta on 21 May and 25 October 2017, selected as these spring and autumn nighttime calibrations correspond to CL close to the maximum and minimum values over the year 2017 (see Fig. 5c). Figure 5c gives a more general overview of the long-term results of the calibration procedure, further revealing that the three CL time series feature a similar seasonal cycle, as also observed in other European ALC networks (e.g. Buxmann, 2024). The reasons for such a yearly cycle are currently under investigation within the European ALC community, also taking advantage of recent activities conducted within the EC COST Action PROBE (e.g. van Hove and Diémoz, 2024).
The CL values used within ALICENET inversions are currently obtained by filtering out the seasonal cycle and keeping only the long-term trends related to slow instrument changes (details are given in Sect. S3). Once the main driver of the CL seasonality is better identified, it will be taken into account in the calibration procedure. For now, we prefer to use the described approach and estimate the uncertainty associated with this CL variability (see Sect. 3.3.3).
3.3 Retrieval of aerosol properties
This section describes the ALICENET inversion of the aerosol optical (Sect. 3.3.1) and physical (Sect. 3.3.2) properties. Specific examples of the aerosol products at different ALICENET sites are also given and compared to a series of independent datasets in order to evaluate the relevant retrieval procedure performances.
3.3.1 Aerosol optical properties
The aerosol backscatter and extinction profiles are calculated from the total attenuated backscatter (βatt) profile based on the forward Klett inversion (Wiegner and Geiß, 2012; Wiegner et al., 2014) of Eq. (1). Since both βp and αp are unknown in Eq. (1), an assumption on the relationship linking the two variables is necessary to solve the Klett inversion. Within ALICENET, we do not fix an a priori, vertically constant extinction-to-backscatter ratio (also referred to as the lidar ratio, LR), as often done in elastic lidar retrievals. Instead, the aerosol extinction is linked to backscatter through a specific functional relationship (αp=αp(βp)) already presented and discussed in Dionisi et al. (2018). This was obtained at the CHM15k operating wavelength (1064 nm) based on a large set of simulated optical properties from a continental-type aerosol model. Details on the implementation of the functional relationship within the forward Klett inversion are given in Sect. S4.1.
It is important to note that, with this procedure, no ancillary data (e.g. co-located sun-photometer AOD) and no a priori assumptions (e.g. selection of the LR constant value to be used) are needed in the retrieval. Therefore, a posteriori comparison to co-located sun-photometer AOD provides a way to check the performance of the ALICENET optical property retrievals. These comparisons were performed using both short- and long-term datasets thanks to some co-located or nearby AERONET (https://aeronet.gsfc.nasa.gov/, last access: 25 July 2024) or SKYNET (https://www.skynet-isdc.org/, last access: 25 July 2024) sun photometers. Specific examples are shown in Figs. 6 and 7, respectively.
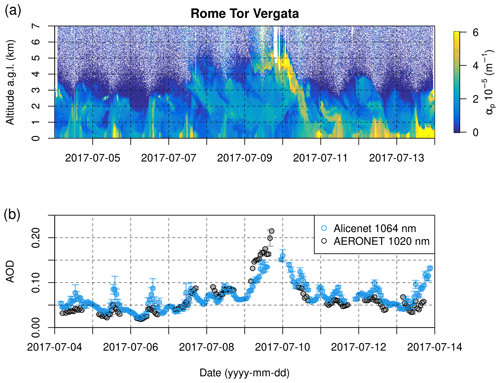
Figure 6(a) Aerosol extinction profiles in Rome Tor Vergata retrieved by the ALICENET inversion during the EMERGE campaign in July 2017 and (b) comparison between the ALICENET-derived AOD and the co-located AERONET L2 data. Both ALICENET and AERONET AODs are hourly averaged (error bars are the AOD standard deviations within the averaging interval).
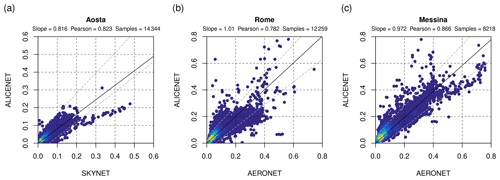
Figure 7Long-term (2016–2022) comparison between the AOD derived by ALICENET (at 1064 nm) and AERONET/SKYNET sun photometers (at 1020 nm) in (a) Aosta, (b) Rome Tor Vergata, and (c) Messina. Colours refer to the data density. The black line is the linear fit. The fit slope and Pearson correlation coefficients are reported in each panel together with the total number of data pairs (samples). Dashed grey lines delimit deviations of ±0.01 ± 0.15 × AODsun photometer from the 1:1 line.
Figure 6a shows the aerosol extinction profiles derived from the Rome Tor Vergata ALC during the EMERGE-EU field campaign in July 2017 (Andrés Hernandez et al., 2022), while in Fig. 6b the corresponding ALC-derived AOD (blue) is compared with the one measured by the co-located AERONET sun photometer (grey).
Figure 6 shows that the time series of the two independent datasets, both averaged at an hourly resolution, mostly agree within the expected AERONET (Giles et al., 2019) and ALICENET (Sect. 3.3.3) uncertainties. Exceptions are found during days strongly impacted by transport of Saharan dust (e.g. 9 July 2017). This is expected because, as mentioned, the functional relationship employed in the inversion was optimised for a continental-type aerosol and does not properly describe the different backscatter-to-extinction relation in the presence of non-spherical particles (e.g. Barnaba and Gobbi, 2001). Also note that, despite using L2 AERONET data, the maximum sun-photometer AOD value on 9 July corresponds to a cloud-screened time window in the ALC record, with this indicating some cloud contamination on the sun-photometer data. The extension of the ALICENET retrieval approach to other aerosol types and relevant testing is planned for the future, also taking advantage of the depolarisation measurement capabilities of PLCs operating within the network.
Figure 7 shows a multi-annual (2016–2022), multi-site (Aosta, Rome, Messina) comparison between ALC AOD and sun-photometer AOD. In this case AERONET L2 data were used in Rome Tor Vergata and Messina, while SKYNET AOD data in Aosta were derived by taking into account the temperature correction of the POM-02 photometer as described in Uchiyama et al. (2018). We included in the figures only ALC and sun-photometer measurements that are recorded in temporal coincidence (within 5 min). The overall number of pairs considered in each site is reported in Fig. 7. This comparison shows that the ALICENET retrieval is able to quantify the actual aerosol load in a variety of conditions. In fact, the number of data pairs lying within ±0.01 ± 0.15 × AODsun photometer (dashed lines) from the 1:1 line is 84 % in Aosta, 73 % in Rome, and 70 % in Messina. Some ALC overestimations are mainly due to instrumental noise at higher altitudes, while underestimations are mainly related to the presence of non-continental aerosol types, such as dust and marine particles in Messina or shallow aerosol layers in the blind region (i.e. below 225 m a.g.l.), as is the case in Aosta during winter and further discussed below. The effects of non-continental aerosol types is better illustrated in Sect. S4.1 (Fig. S4), where the same data are shown together with their associated Ångstrøm exponents.
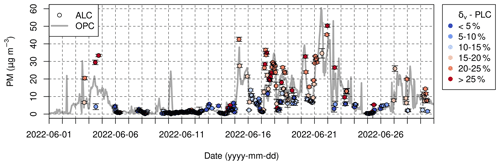
Figure 8Aerosol mass concentrations derived in the month of June 2022 from the Aosta ALC (bullets) and from in situ instrumentation (grey line). In particular, ALC values refer to the vertical layer 3500 ± 200 m a.s.l., and the colour code indicates depolarisation values from the co-located PLC (δv). In situ PM10 concentrations were derived from an OPC at the mountain (3500 m a.s.l.) observatory Testa Grigia (Plateau Rosa, 35 km from Aosta; data courtesy of Stefania Gilardoni, CNR-ISP).
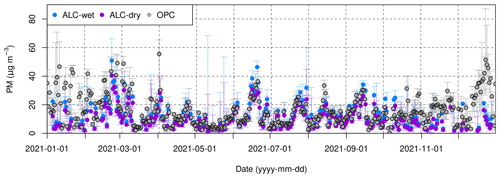
Figure 9The 1-year (2021) dataset of surface aerosol mass concentrations as derived by the ALICENET ALC inversion and by OPC measurements in Aosta. Data refer to daily median values (points) and relevant 25th–75th percentiles (vertical bars). ALC-based data are those derived from the ALICENET retrieval (wet) and corresponding ones further corrected to dry values (see Sect. S4).
3.3.2 Aerosol physical properties
Aerosol physical properties such as particle surface area and volume (Sp and Vp) are also derived based on functional relationships linking these to aerosol backscatter and provided in Dionisi et al. (2018). Being of particular interest for AQ applications, aerosol mass concentrations (Mp) can then be derived from the estimated aerosol volume as Mp = ρpVp, using an a priori aerosol density ρp. It is worth highlighting that remote sensing aerosol retrievals provide aerosol properties in unperturbed atmospheric conditions, i.e. including hygroscopic effects. Conversely, most in situ instrumentation (such as that operating in AQMN in compliance with the EU AQ Directive) generally provides dry particulate matter mass values. Therefore, a RH adjustment is necessary when comparing the ALC-based aerosol properties (including mass) to dry in situ data (e.g. Barnaba et al., 2010). Details on the hygroscopic correction used within ALICENET are reported in Sect. S4.2. In the following, we show a short-term (Fig. 8) and long-term (Fig. 9) comparison between the Mp retrieved by ALICENET using ALC data collected in Aosta and in situ reference measurements.
In Fig. 8, the Mp values at 3500 m a.s.l. extracted from ALC aerosol profiles are compared with the aerosol mass concentrations measured in situ by an OPC operating at the same altitude in the Testa Grigia–Plateau Rosa observatory (western Alps, 35 km east of Aosta; see Fig. S5 in the Supplement for details on relative site locations) in June 2022. This period was selected because in summer secondary hygroscopic particles from the Po basin are regularly transported to the western Alps, reaching altitudes > 3 km a.g.l. (Diémoz et al., 2019a, b). In fact, June 2022 registered both medium-range transport of Po Valley pollution and long-range transport of desert dust to Plateau Rosa. Figure 8 shows the 30 d temporal evolution of the ALC-based Mp (bullets) in the ALC vertical bin 3500 ± 200 m a.s.l. over Aosta and the corresponding values from the Testa Grigia OPC (grey line). The aerosol density used to derive ALC and OPC aerosol mass concentrations was 1.2 (1.6) g cm−3 in the presence of non-dust (dust-dominated) aerosol mixtures (Diémoz et al., 2019b). Moreover, assuming desert dust to be mainly hydrophobic, the hygroscopic correction as described in Sect. S4.2 was only applied to ALC data in non-dust conditions. This discrimination was done using the linear volume depolarisation ratio (δv) profiles of a co-located PLC and assuming that aerosol mixtures associated with 15 % are dominated by secondary (dust) particles. Overall, Fig. 8 shows that the two mass concentration series exhibit similar time evolution, with good agreement both in low-aerosol conditions (e.g. 6–15 June 2022) and during transport events increasing the local aerosol load. In the considered period, main transport events were associated with desert dust intrusions (e.g. 3–5, 18–22, and 27–28 June 2022) and Po Valley pollution advections (e.g. 13–14 and 25–26 June 2022). This result is very promising considering that the horizontal distance between the ALC-probed/PLC-probed column and the Plateau Rosa station is > 30 km and that the in situ OPC measurements may also be influenced by local dynamics and surface emissions.
A longer comparison for the ALICENET aerosol mass product is reported in Fig. 9. It shows the 1-year (2021) record of ALC-derived Mp at ground level and the corresponding in situ, surface PM10 concentrations derived by OPC measurements in downtown Aosta, 4 km away from the Aosta ALC (Diémoz et al., 2021). Data are shown in terms of daily median values and corresponding 25th–75th percentiles. To convert the volume into mass the aerosol density was set to 1.5 g cm−3, while to convert the ALC-derived wet aerosol mass (blue) into dry aerosol mass (purple) we applied the hygroscopic correction for a continental aerosol type as described in Sect. S4.2 using surface RH measurements from the AQMN of ARPA Valle d'Aosta. As can be observed, the ALICENET-retrieved Mp is able to reproduce the variability observed in the in situ-measured PM10, with some underestimations in the winter months. We investigated these underestimations further and found these are mainly attributable to (a) the shallow (i.e. few tens of metres), frequent temperature inversions occurring during winter in the Alpine valleys and capping aerosols in the lowermost levels (e.g. Giovannini et al., 2020) and (b) the higher wintertime local emissions in the urban site of downtown Aosta with respect to the semi-rural site where the ALC is operating (Diémoz et al., 2019b).
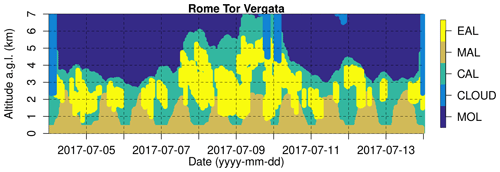
Figure 10Aerosol layering mask derived from the ALADIN processing on the CHM15k operating in Rome Tor Vergata in the same period presented in Fig. 6. The mask discriminates the following layers: the continuous aerosol layer (CAL), the mixed aerosol layer (MAL), and elevated aerosol layers (EALs). Aerosol-free (i.e. molecular, MOL) and cloud-screened (CLOUD) regions as identified in the overall ALICENET processing are also shown.
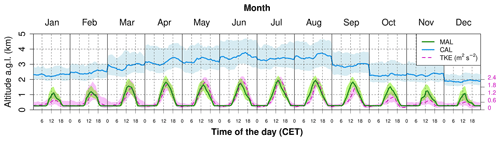
Figure 11Monthly and daily resolved statistics (median and 25th–75th percentiles as shaded dashed areas) of the MAL and CAL heights (left y axis) derived from the ALADIN tool application over the multi-annual (2016–2022) dataset of the CHM15k in Rome Tor Vergata. Similar statistics of the turbulent kinetic energy (TKE) derived from a co-located ultrasonic anemometer (violet) are also plotted (right y axis) as a proxy for convection intensity and timing.
3.3.3 Estimated uncertainty of aerosol property retrievals
The previous sections describe the ALICENET efforts to exploit the great potential of ALC in providing quantitative aerosol-related geophysical parameters and demonstrate the good performances of the current algorithms. Nonetheless, due to several factors also discussed above, the expected uncertainties associated with the output products range from 20 % for the attenuated backscatter (product L2 in Fig. 1) to 50 % for the aerosol mass (L3 in Fig. 1). The main factors contributing to these uncertainties are listed hereafter.
- 1.
The instrumental noise of the signal. This factor depends on the instrument status and mainly impacts the retrievals in the middle to upper troposphere.
- 2.
The overlap correction applied to the signal. As discussed, this factor is critical in the lowermost levels, and accurate instrument-specific, overlap-correction models are necessary to derive quantitative information in the first 800 m. Accuracy of the retrievals in this lowermost vertical region depends on the statistical and physical representativeness of the ensemble of overlap functions from which the overlap model is derived (Sect. S2).
- 3.
The variability in the instrument calibration coefficient. This third factor (see Sect. 3.2) directly impacts the accuracy of βatt. For example, it is found by error propagation that changes of 30 % in the instrument calibration coefficient (which are quite usual in some ALICENET and E-PROFILE stations) translate into a variability in βatt of up to 20 %.
- 4.
The accuracy of the functional relationships used in ALICENET to link the aerosol backscatter to the other aerosol properties, impacting the estimation of αp, Sp, Vp, Mp, and to a lesser extent βp. This factor strongly depends on the actual aerosol conditions: the currently used functional relationships can give a good estimate of the aerosol properties in the presence of continental aerosols, while in the presence of non-continental particles they are less accurate (a relative error of 30 %–40 % was derived by Dionisi et al., 2018). As mentioned, the extension of the ALICENET approach to include other aerosol types is foreseen for the near future. In particular, exploitation of the PLC depolarisation profiles for aerosol typing will drive the selection of aerosol-type-specific functional relationships and is expected to be particularly useful in the presence of desert dust (e.g. Gobbi et al., 2002).
Concerning the retrieval of aerosol mass concentrations, the assumed particle densities are a major source of uncertainty, and the accuracy of the retrieval depends on the possibility to better constrain the aerosol density profiles, e.g. through ancillary data, including depolarisation information.
Overall, the above factors result in instrument-, time-, and range-dependent uncertainties of the ALC-based aerosol optical and physical properties. The expected uncertainty with an optimal SNR up to at least 7 km a.g.l., with an overlap error < 10 % in the lowermost levels, and in the presence of continental aerosol types is 20 % for βatt, is 30 %–40 % for AOD, and reaches 50 % for aerosol mass.
3.4 The ALICENET automatic aerosol layer detection algorithm (ALADIN)
As previously mentioned, a main advantage of ALCs is their ability to operate continuously, which allows detecting and tracking the variability in the aerosol vertical stratifications at multiple timescales using aerosols as passive tracers. Besides atmospheric research (e.g. Jozef et al., 2024), this information can be beneficial for several sectors, among which are AQ and meteorology (e.g. Moreira et al., 2020; Ravnik et al., 2024; Körmöndi et al., 2024) as well as aviation (e.g. Osborne et al., 2019; Salgueiro et al., 2023).
Commonly identified atmospheric stratifications based on ALC data analysis include the atmospheric boundary layer and the mixed layer (ABL and ML, respectively, e.g. Poltera et al., 2017; Kotthaus et al., 2020; Caicedo et al., 2020), as well as lofted aerosol layers in the free troposphere (e.g. Adam et al., 2022). The ABL is a thermodynamic layer connected to the Earth's surface and capped by a temperature inversion, while the ML is an ABL sublayer mixed by turbulent fluxes (Stull, 1988; Kotthaus et al., 2023).
However, it should be noticed that aerosols are “delayed” tracers of atmospheric dispersion processes and may not always consistently represent the thermodynamic state of the atmosphere (Haeffelin et al., 2012). The tracking of thermodynamic layers through aerosol lidars can be also complicated by superimposing phenomena such as medium-to-large-scale advections, natural and anthropogenic emissions, and particle physicochemical transformations. These processes may remove or transport particles in specific atmospheric ranges (e.g. Collaud Coen et al., 2018; Diémoz et al., 2019a), modulate the daily cycle of aerosol profiles (e.g. Diémoz et al., 2021), and form aerosol layers within and above the ABL (e.g. Curci et al., 2015; Sandrini et al., 2016), thus decoupling the aerosol-related and thermodynamic stratifications. This decoupling is expected to be further enhanced over complex terrain (e.g. Serafin et al., 2018) and/or over regions affected by multiple natural and anthropogenic sources, as is the case in the Italian territory.
For all these reasons, the ALICENET approach to aerosol layer detection and naming emphasises a clear connection to observable aerosol structures, rather than relying on traditional thermodynamics-based terminology. In particular, we developed a novel aerosol layer detection algorithm (ALADIN) tool to automatically derive aerosol layering information from ALCs and/or PLCs across the network. ALADIN targets the following aerosol layers:
- 1.
the continuous aerosol layer (CAL), which is the layer extending from the ground level and characterised by the continuous presence of aerosols;
- 2.
the mixed aerosol layer (MAL), which is a CAL sublayer within which aerosols are mixed by surface-driven turbulent fluxes; and
- 3.
elevated aerosol layers (EALs), which are lofted aerosol layers that lie above the MAL and within or above the CAL.
Within ALADIN, each layer type (CAL, MAL, and EALs) is detected from ALC and/or PLC L2 data using a specific methodology. The CAL is determined by comparing the aerosol and the molecular βatt profiles. The identification of the MAL is based on dynamic time warping (DTW, Giorgino, 2009) and variance analyses of the ALC profiles. The detection of EALs is performed with continuous wavelet transform (CWT, Du et al., 2006) and iterative techniques. Full details on the ALADIN procedures are reported in Sect. S5, which also contains a schematic description of the ALADIN processing flow (Fig. S7).
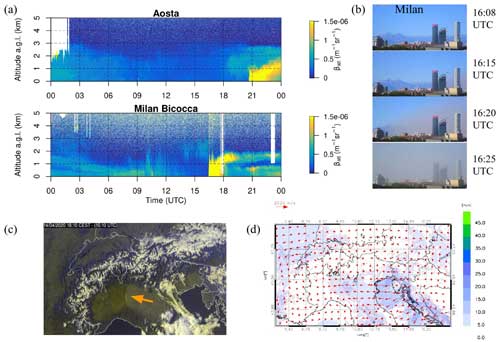
Figure 12(a) Total attenuated backscatter profiles at the Aosta and Milan Bicocca sites on 14 April 2020, (b) central Milan webcam (source: Arzaga Meteorological Observatory, https://www.osservatorioarzaga.it/, last access: 25 July 2024) showing the rapid decrease in visibility on 14 April 2020 (from top to bottom: 16:08, 16:15, 16:20, and 16:25 UTC), (c) Po Valley satellite true colour image (14 April 2020 at 18:10 UTC; credits: EUMETSAT) with the regional dust front (orange arrow), and (d) 10 m wind speed and direction simulated by WRF over northern Italy (14 April 2020 at 17:00 UTC; data courtesy of Stefano Federico, CNR-ISAC) illustrating the extension of the gust and wind fronts. The arrival of the regional dust front in Milan at 16:20 UTC and in Aosta at 20:40 UTC is clearly visible from ALC profiles in panel (a).
Figure 10 shows the layering mask corresponding to the same ALC data shown in Fig. 6. It includes the ALADIN output discriminating the CAL, MAL, and EALs, plus the aerosol-free (i.e. molecular, MOL) and cloud-screened (CLOUD) regions as inferred from the overall ALICENET processing. In this episode, the EALs above 3 km a.g.l. are mostly due to minor (7–8 and 10–11 July) and major (9 July) Saharan dust intrusions, while the ones between 1 and 3 km a.g.l. are due to fire plumes (e.g. 11 July; Andrés Hernandez et al., 2022) and/or to aerosol formation and growth within the residual layer during nighttime (e.g. 5–6 July).
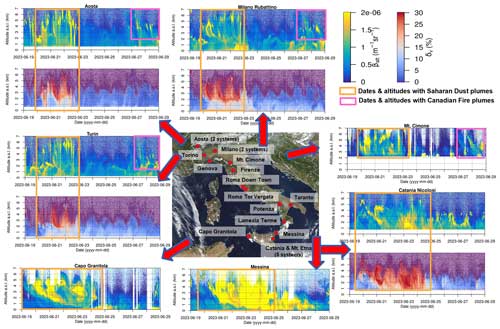
Figure 13Vertical profiles of total attenuated backscatter βatt (for both ALCs and PLCs) and volume depolarisation δv (for PLCs) as recorded at selected northern to southern ALICENET sites in the period 19–28 June 2023, affected by Saharan dust and Canadian fire plumes (orange and magenta boxes, respectively). Background map credits: Suomi NPP VIIRS of NASA–NOAA.
Further discrimination of aerosol layers in terms of aerosol type could be derived by exploiting PLC-based δv profiles (see Fig. 2). In fact, the inclusion of the PLC depolarisation information within the ALICENET processing is in progress as a first step to automate the aerosol-typing capacity within the network (thus complementing the aerosol-layer-typing capacity from more complex lidar systems, e.g. Nicolae et al., 2018; Córdoba-Jabonero et al., 2018).
Routine application of the automated ALADIN tool on a daily basis also allows us to get statistics of vertical aerosol stratifications in the atmosphere. An example of this long-term application is presented in Fig. 11, which shows the monthly and daily resolved cycle of MAL and CAL heights over Rome Tor Vergata derived from the 2016–2022 ALC dataset (continuous lines are median values, while shaded areas represent 25th–75th percentiles). Figure 11 clearly shows the marked yearly cycle of the CAL height (minimum in winter and maximum in summer), due to the increased convection and photochemistry in the warmest months (e.g. Barnaba et al., 2010). As expected, all over the year the MAL shows a marked daily cycle, with maximum heights in summer (about 2 km thick in July–August) doubling those in winter (about 1 km in December–January). A similar statistics of turbulent kinetic energy (TKE) from a co-located ultrasonic anemometer (magenta lines) is also reported as a proxy for convection, which is the main driving factor of the MAL temporal evolution. Note that in this figure the time axis is reported as central European time (CET) to better highlight the diurnal variability of the addressed quantities.
A follow-up work is currently in preparation within ALICENET to present a more detailed multi-annual analysis of the ALC-based aerosol properties and layering in selected ALICENET sites from northern to southern Italy, in synergy with in situ aerosol measurements and model (ERA5, CAMS) products.
A main advantage of lidar-ceilometer networks is their continuous, near-real-time monitoring capability. In fact, ALICENET has already been exploited in past events to follow the evolution and characterise specific aerosol transport features and/or to quantify the impact of aerosol dynamics on local aerosol concentrations, mostly in synergy with other tools and measuring techniques such as in situ aerosol observations, ground-based passive remote sensors, satellites, or models (Gobbi et al., 2019; Diémoz et al., 2019a, b; Di Bernardino et al., 2021; Ferrero et al., 2019; Rizza et al., 2017, 2022; Tositti et al., 2022; Andrés Hernández et al., 2022). This section describes, through some recently recorded examples, the potential of this near-real-time 4D ALICENET monitoring capability at the national scale, which is particularly useful for nowcasting, warnings, and alerts in the case of noteworthy events.
4.1 Po Valley local dust front (14 April 2020)
In previous studies (Diemoz et al., 2019a, b), the operational use of ALICENET provided observation-based evidence of the export of pollutants from the northern Italy Po Valley to surrounding areas. The phenomenon, previously observed by lidar profiling performed at the EC JRC in Ispra (about 60 km northwest of Milan, Barnaba et al., 2010), was further analysed and quantified thanks to the ALICENET combination of sites (Milan and Aosta, i.e. within and at the border of the Po Valley). That study demonstrated that such pollution-rich advections markedly affect PM-related AQ even in the pristine mountain environments mainly transporting hygroscopic particles of secondary origin. However, transport of particles of primary origin (particularly from soil-related sources) across the Po Valley has also been observed, particularly during dry periods. Figure 12 shows an example of such events (14 April 2020), largely impacting regional AQ and visibility.
This episode was due to an extended (about 100 km) gust front originating from the cold and intense Bora winds from the east, as well as to anomalous dry conditions affecting Europe in April 2020. Resuspended, soil-originated particles from the cultivated fields were transported across the whole Po Valley, as it is also visible from space (Fig. 12c). ALC profiles (Fig. 12a) well capture the timing of the plume's arrival in Milan (as also seen from central Milan webcams, Fig. 12b) and show the vertical extent of the particle-rich layer associated with the episode. As also revealed by satellite measurements (Fig. 12c) and model simulations (Fig. 12d), after impacting the Milan area, the plume continued to travel westward and was detected by the ALC in Aosta 4 h later, indicating a wind speed > 12 m s−1.
4.2 Advection of Saharan dust and Canadian fire plumes over Italy (19–28 June 2023)
The Mediterranean area is frequently affected by the transport of desert dust from North Africa and the Middle East (e.g. Barnaba and Gobbi, 2004; Querol et al., 2009; Basart et al., 2012a; Gama et al., 2020). In Italy, these events are estimated to reach the ground on 10 % (northern regions) to over 30 % (southern regions) of the days in a year and to impact on surface daily mean PM10 concentrations with 10–15 µg m−3 (Barnaba et al., 2022). Transport of fire plumes from global-to-medium distances is also an important contributor to aerosol loads in Europe. A significant contribution is given by forest fires regularly developing during boreal summers in Canada (e.g. Ceamanos et al., 2023; Shang et al., 2024), and a major contribution from agricultural fires in eastern Europe and Russia has also been detected over the continent, particularly in spring and summer (Barnaba et al., 2011). Summer 2023 was particularly impacted by multiple episodes of severe wildfires in central Canada. Almost 480 Mt of carbon was emitted, resulting in a major impact on AQ across Canada and the northern US. The plumes have also been observed to be regularly transported towards Europe (https://atmosphere.copernicus.eu/copernicus-canada- produced-23-global-wildfire-carbon-emissions-2023, last access: 25 July 2024). Figure 13 shows a composite of measurements collected at multiple ALICENET sites across the country during a 10 d period (19–28 June 2023) affected by both desert dust (time–altitude windows identified by orange boxes) and forest fire plumes (time–altitude windows identified by magenta boxes). More specifically, this period was characterised by the intrusion of Saharan dust from southern to northern Italy (19–24 June 2023), followed by the transport of Canadian fire plumes over central and northern Italy (27–28 June 2023). The ALC profiles (βatt and δv) at the seven selected ALICENET sites (central panel in Fig. 13) allow us to follow the spatio-temporal evolution of the different aerosol layers and identify the relevant aerosol type. The Saharan dust layers were first observed over southwestern Italy (Capo Granitola, 19 June in the morning) and then moving westward to Messina and Catania (19 June, afternoon) and northward to Turin, Aosta, Milan, and Mt Cimone, where the dust plume is detected in the evening. All over Italy, the dust plume affects atmospheric layers up to 7 km altitude, reaching down to the surface on 20 June. In fact, the PLC systems clearly indicate the presence of irregularly shaped mineral particles aloft (depolarisation values δv > 30 %) and mixing with local (mainly spherical) particles, with δv ∼ 10 %–20 % when reaching the lowermost levels.
The Canadian fire plumes were first observed by ALICENET systems operating in northwestern Italy (Aosta, Turin) on 27 June 2023 in the range of 2–7 km a.s.l. They then travelled through the whole Po Valley and were clearly observed in Milan and Mt Cimone. Being mainly composed of processed particles, these long-range-transported fire plumes do not show increased depolarisation and appear as thinner aerosol layers with respect to the ones typically associated with dust layers. These vertically resolved measurements well complement the information that can be gathered from satellites. For instance, a comparison between ALICENET data and MSG and Metop retrievals was conducted with respect to the dust event (e.g. https://vuser.eumetsat.int/resources/case-studies/dust-transport-from-the-sahara-to-the-mediterranean, last access: 25 July 2024). At the same time, vertical aerosol profiling also provides an observational verification of the picture that can be obtained by modelling tools. In this respect, Fig. 14 shows the CAMS EU forecast maps (ensemble model) for two dates within the temporal window addressed, i.e. 22 June 2023 (dust intrusion, left panels) and 27 June 2023 (Canadian fires, bottom panels), at two altitude levels (100 and 3000 m a.g.l., top and bottom panels, respectively). The horizontal evolution of the aerosol advections qualitatively agrees with the ALICENET observations. It is more difficult to correctly model the aerosol vertical distribution, due to both their coarse vertical resolution and simplified parameterisations of the aerosol-related atmospheric processes (e.g. Koffi et al., 2016). Indeed, remote sensing observations by ALC and/or PLCs represent an added value for both AQ monitoring and AQ modelling. In fact, specific efforts are currently ongoing for the assimilation of ceilometer information into CAMS (e.g. the H2020 CAMS AERosol Advancement (CAMAERA) project, https://camaera-project.eu/, last access: 25 July 2024).
4.3 Aerosol particles from the Mt Etna eruption (13–14 August 2023)
A recent example of Mt Etna volcano eruptions is reported in Fig. 15 to highlight the important information that ALC and/or PLC observations can provide in volcanic areas to complement in situ, satellite-based and modelling data (e.g. Corradini et al., 2018; Scollo et al., 2019; Bedoya-Velásquez et al., 2022). During the night between 13 and 14 August 2023, Mt Etna, which is Europe's most active volcano, erupted. Its southeast crater emitted a volcanic cloud that the PLC in Nicolosi detected to reach up to 5 km altitude at 21:00 UTC (Fig. 15a). On 13 August at 20:41 UTC, a Volcano Observatory Notice for Aviation (VONA) was issued by INGV (https://www.ct.ingv.it/Dati/informative/vona/VONA_Etna_202308132041Z_2023005708E01.pdf, last access: 25 July 2024) with a red alert for aviation. VONAs are short, plain-English messages aimed at dispatchers, pilots, and air-traffic controllers to inform them of volcanic unrest and eruptive activity that could produce ash-cloud hazards. In fact, flights serving Catania were halted. The most intense phase of the eruption occurred between 01:40 and 02:30 UTC, when PLC depolarisation reached values > 40 %, indicating a predominance of irregular ash particles. The ash plume was then observed to rapidly reach the ground, while moving southward in the Mediterranean Sea (Fig. 15b). In fact, less than 5 h after the beginning of the eruption, the plume was detectable east of Malta. In agreement with the ALC record, the VONA issued by INGV at 05:54 UTC indicates that no ash plumes were produced and that the volcanic ash was confined to the summit areas of the volcano (corresponding to an orange aviation colour code, https://www.ct.ingv.it/Dati/informative/vona/VONA_Etna_202308140554Z_2023005808F01.pdf, last access: 25 July 2024).
In this work we present ALICENET, the Italian network of automated lidar ceilometers (ALCs) operating from north to south across the peninsula (Fig. 1). It is a cooperative network set up by CNR-ISAC in 2015 and currently running with active contributions from several regional EPAs, universities, research centres, and private companies. The network contributes to fill an Italian observational gap at the EU level, where most member states generally run extended ALC networks managed by national meteorological agencies (e.g. the German Weather Service, DWD, running over 100 instruments, https://www.dwd.de/EN/research/observing_atmosphere/composition_atmosphere/aerosol/cont_nav/aerosolprofiles.html, last access: 25 July 2024). Since its setup, the ALICENET network has kept expanding (Table 1), and it currently covers very different environments (urban, coastal, mountainous, and volcanic areas), thus providing information across a large spectrum of atmospheric conditions and aerosol regimes. ALICENET promoted a standardisation of instruments and a homogeneous data processing specifically developed within the network. It mainly runs single-channel ALCs (CHM15k systems by OTT HydroMet) but is progressively introducing polarisation-sensitive systems (PLCs) recently commercialised by Vaisala (CL61) to further exploit their ability to discriminate among aerosol types. Since the beginning of the ALICENET activities, particular care has been devoted to data retrievals and exploitation. These activities also took advantage of technical–scientific exchanges within European initiatives, such as the EC Cost Actions TOPROF (2013–2016) and PROBE (2019–2024), the ongoing EUMETNET programme E-PROFILE (2020–2028), and the EC H2020 project RI-URBANS (2021–2025). In this context, ALICENET developed a specific, centralised, and automated data-processing chain with associated data quality control (QC) procedures, as presented in detail in this work. The data-processing steps either were refined from previously published work (e.g. Hervo et al., 2016; Dionisi et al., 2018) or are completely new, such as the automatic aerosol layer detection algorithm (ALADIN). Overall, the processing chain includes signal correction and calibration procedures (Sect. 3.1, 3.2), aerosol property inversions (Sect. 3.3), and the identification of vertical stratifications (mixed, continuous, and elevated aerosol layers – MAL, CAL, and EALs, respectively, Sect. 3.4). Output products with different levels of complexity and associated uncertainties are thus provided (Fig. 2). These range from more basic L1 quantities (such as the range-corrected signal, RCS, and, where applicable, depolarisation, δv) to the L2 total attenuated backscatter βatt and the L3 aerosol optical (βp, αp, and thus AOD) and physical (Sp, Vp, and Mp) properties plus vertical layering (MAL, CAL, EALs).
Level 1 and/or Level 2 products are provided in near real time on a dedicated website (https://www.alice-net.eu/, last access: 25 July 2024), while L3 products are obtained offline and are currently only available upon request. Examples of product types are reported in Sects. 3 and 4. For L3 products, this work also includes direct comparisons with relevant, independent data (in situ or remote sensing, depending on the variable addressed), showing that the ALICENET data processing is able to provide robust and quantitative aerosol information within the discussed limits of the data accuracy (Sect. 3.3.3). In fact, long-term comparisons of aerosol mass retrievals with surface PM10 data show mean discrepancies of 35 %, while AOD comparisons to thousands of relevant data points from co-located sun photometers show correlation coefficients > 0.8 and fit slopes ranging between 0.8 and 1.0, depending on the site location.
Efforts to evaluate the ALICENET retrieval performances are constantly performed as well as comparisons to different inversion approaches and tools. For example, a preliminary algorithm intercomparison exercise was recently performed within PROBE to evaluate differences in the outcomes produced by different national networks in the EU (namely ALICENET, Italy; Met Office, UK; V-Profiles, Norway; and DWD, Germany; Osborne, 2024). An additional analysis of the ALICENET L3 products is currently in preparation based on multi-annual datasets of selected ALICENET systems located across Italy and relevant comparisons to independent data and models.
The next steps foreseen within the network are (a) a better characterisation of the instruments artefacts and calibration, (b) the extension of the ALICENET ALC retrieval methodology to different aerosol types, and (c) the development of a full retrieval for PLCs (CL61), further exploiting the depolarisation information to identify aerosol types. Since the CL61 operates at a different wavelength with respect to CHM15k, the evaluation of water vapour absorption corrections (e.g. Wiegner and Gasteiger, 2015) and the definition of new, wavelength-specific functional relationships (e.g. Dionisi et al., 2018) to be used within the data inversion process are also required and will be explored. The feasibility of a regular dissemination of ALICENET L3 products via the network website in addition to the near-real-time L1 and L2 ones is also under evaluation.
Overall, ALICENET represents a valuable resource to complement the aerosol observational capabilities in Italy with the unique capacity of continuous 4D monitoring. The maturity of both instrumental technologies and data-processing tools such as the ones described here suggests that ALC and/or PLCs could fruitfully contribute to aerosol measurements within European research infrastructures (e.g. ACTRIS) and/or EEA AQMNs.
At the national level, ALICENET also intends to bridge a gap between the research-oriented and the operational use of active aerosol remote sensing in several sectors, among which are (a) air quality, (b) radiative budget/solar energy, and (c) aviation safety, thus representing a good example of earth observation science applications for society. Its outputs have already been proven to be useful in the validation of models and satellite products.
Of particular interest for the AQ sector are the abilities of the ALC- and PLC-based ALICENET data to (i) automatically identify medium-to-long-range aerosol advections and estimate the relevant contribution to surface PM10 concentrations and (ii) provide continuous information on particulate matter layering, including the mixed aerosol layer (MAL), i.e. the atmospheric volume in which locally emitted particles are diluted (e.g. Kotthaus et al., 2023), and the elevated aerosol layers (EALs) reaching the surface. The effectiveness of using these ALC and PLC abilities in support of standard AQMNs is being currently explored within the ongoing EC H2020 project RI-URBANS, aimed at developing an air quality monitoring system that complements those currently available. In this framework, tests for upscaling the ALICENET tools to other urban sites in the EU are in progress (e.g. Barnaba et al., 2024). Concerning the other applications mentioned above, the continuous ALC-based information on the vertical distribution of aerosol properties and layering is useful for better estimation of the relevant radiative effects (beneficial, for example, within an operational short-term solar forecasting system, e.g. Papachristopoulou et al., 2024), for validation of/assimilation in models (e.g. Chan et al., 2018; Valmassoi et al., 2023), or for the provision of near-real-time alerts for aviation safety during specific extreme events such as desert dust storms and volcanic eruptions (e.g. Papagiannopoulos et al., 2020). Continuous aerosol monitoring capabilities of ALC and PLC systems and availability of relevant long-term records is also expected to be particularly important in the verification of satellite aerosol products including vertical layering (e.g. Jänicke et al., 2023), considering that aerosol vertical profiles and ABL characteristics are recognised as priority targeted observables for space-based Earth observation programmes (e.g. National Academies of Sciences, Engineering, and Medicine, 2018) and that the joint ESA–JAXA mission EarthCARE with a lidar instrument aboard was recently successfully launched (e.g. van Zadelhoff et al., 2023).
ABL | Atmospheric boundary layer |
ACTRIS | Aerosol, Clouds, and Trace Gases Research Infrastructure |
AERONET | Aerosol Robotic Network |
ALADIN | Aerosol layer detection algorithm |
ALC | automated lidar ceilometer |
ALICENET | Automated LIdar-CEilometer network |
AQ | Air quality |
AQMN | Air quality monitoring network |
AOD | Aerosol optical depth |
ARS | Aerosol remote sensing |
BG test | Breusch–Godfrey test |
CAL | Continuous aerosol layer |
CAMAERA | CAMS AERosol Advancement project |
CAMS | Copernicus Atmosphere Monitoring Service |
CHM15k | Lufft (now OTT HydroMet) automated lidar-ceilometer instrument |
CL61 | Vaisala polarisation-sensitive lidar-ceilometer instrument |
CWT | Continuous wavelet transform |
DTW | Dynamic time warping |
DWD | German Weather Service |
EAL | Elevated aerosol layer |
EARLINET | European Aerosol Research Lidar Network |
EarthCARE | Earth Cloud, Aerosol and Radiation Explorer |
EC | European Commission |
ECMWF | European Centre for Medium-Range Weather Forecasts |
EEA | European Environment Agency |
EPA | Environmental Protection Agency |
E-PROFILE | Programme for surface-based profile observations by EUMETNET |
ERA5 | ECMWF Reanalysis version 5 |
ESA | European Space Agency |
ESFRI | European Strategy Forum on Research Infrastructures |
EUMETNET | European Meteorological Services Network |
INGV | Istituto Nazionale di Geofisica e Vulcanologia |
JAXA | Japan Aerospace Exploration Agency |
LR | Lidar ratio |
MAL | Mixed aerosol layer |
ML | Mixed layer |
MPLnet | Micro-Pulse Lidar Network |
NASA | National Aeronautics and Space Administration |
OPC | Optical particle counter |
PLC | Polarisation-sensitive automated lidar ceilometer |
PM | Particulate matter |
PM10 | Particulate matter with diameter below 10 µm |
PROBE | PROfiling the atmospheric Boundary layer at European scale (past EC COST Action) |
QA | Quality assurance |
QC | Quality control |
RI-URBANS | Research Infrastructures Services Reinforcing Air Quality Monitoring Capacities in European Urban and Industrial Areas (EC H2020 project, GA no. 101036245) |
RH | Relative humidity |
SNR | Signal-to-noise ratio |
SKYNET | Ground-based observation network dedicated to aerosol–cloud–solar-radiation interactions |
TOPROF | Towards operational ground-based profiling with ceilometers, Doppler lidars and microwave radiometers (past EC COST Action) |
VONA | Volcano Observatory Notice for Aviation |
WHO | World Health Organization |
The ALICENET output products on aerosol optical and physical properties and vertical layering presented in this work are freely accessible at https://doi.org/10.5281/zenodo.13332405 (ALICENET, 2024).
The supplement related to this article is available online at: https://doi.org/10.5194/amt-17-6119-2024-supplement.
Conceptualisation, data curation, and investigation: AnB, FB, and HD. Formal analysis and software: AnB. Visualisation: AnB, FB, and HD. ALC instruments and database management: LDL, AlB, FP, HD, and GPG. Funding acquisition and supervision: FB. Writing (original draft preparation): AnB, FB, and HD. Writing (review and editing): AnB, FB, HD, AlB, and GPG.
The contact author has declared that none of the authors has any competing interests.
Publisher's note: Copernicus Publications remains neutral with regard to jurisdictional claims made in the text, published maps, institutional affiliations, or any other geographical representation in this paper. While Copernicus Publications makes every effort to include appropriate place names, the final responsibility lies with the authors.
This article is part of the special issue “Profiling the atmospheric boundary layer at a European scale (AMT/GMD inter-journal SI)”. It is not associated with a conference.
This research benefited from work done within the Action PROBE (CA18235), supported by COST (European Cooperation in Science and Technology).
Annachiara Bellini performed this work in the framework of her doctoral programme at the Sapienza University of Rome (DIET, Rome, Italy) under the scientific supervision of Francesca Barnaba and with the financial contribution of RI-URBANS.
Alessandro Bracci and Ferdinando Pasqualini were supported by the project IR0000032 – ITINERIS, Italian Integrated Environmental Research Infrastructures System (D.D. no. 130/2022 – CUP B53C22002150006) funded by the EU (Next Generation EU PNRR Mission 4 “Education and Research”, Component 2 “From research to business”, Investment 3.1 “Fund for the realisation of an integrated system of research and innovation infrastructures”).
We would like to thank Marina and Clerico Daniele Poggi (PLC Torino), Roberto Cresta and Andrea Bisignano (PLC Genova), Elena Collino and Daniele Perona (PLC Milano Rubattino), Paolo Cristofanelli (ALC Mt Cimone), Samantha Melani and Andrea Antonini (PLC Firenze), Marco Rosoldi (ALC Potenza), Simona Ottonelli (ALC Taranto), Claudia Calidonna (ALC Lamezia Terme), Mauro Coltelli and Roberto Gueli (Catania and Etna ALC and PLC systems) for their contribution to the ALICENET infrastructure, as well as Luca Ferrero, Alessandro Di Giosa, Michele Furnari and Giorgio Tranchida for support in the ALICENET sites of Milan Bicocca, Rome downtown, Messina, and Capo Granitola, respectively.
PLC data at Milano Rubattino were collected by RSE in the framework of the 3-year research plan (2022–2024) for the Italian electrical system (DM MITE no. 337, 15 September 2022), in compliance with the decree of 16 April 2018.
We acknowledge the Italian Air Force CAMM Mt Cimone for their support in the operation of the CMN-IT ceilometer, funded by the Ministry of University and Research (MUR) through the project “Potenziamento della Rete di Osservazione ICOS-Italia nel Mediterraneo” (PRO-ICOS_MED; PIR01_00019), and the WMO GAW regional station Rita Atria for hosting the ceilometer in Capo Granitola.
We also gratefully acknowledge Stefania Gilardoni, Paolo Bonasoni, and Antonello Provenzale for providing the OPC data collected at the Testa Grigia station at Plateau Rosa managed by the CNR Department of Earth System Science and Environmental Technologies, as well as ARPA Lazio for providing the Rome Tor Vergata TKE dataset.
We acknowledge the Copernicus Atmosphere Monitoring Service (CAMS) for the CAMS European air quality forecast ensemble data.
This research has been partially supported by the EC H2020 project RI-URBANS (grant no. 101036245).
This paper was edited by Stelios Kazadzis and reviewed by two anonymous referees.
Adam, M., Fragkos, K., Binietoglou, I., Wang, D., Stachlewska, I. S., Belegante, L., and Nicolae, V.: Towards Early Detection of Tropospheric Aerosol Layers Using Monitoring with Ceilometer, Photometer, and Air Mass Trajectories, Remote Sens.-Basel, 14, 1217, https://doi.org/10.3390/rs14051217, 2022.
ALICENET: Aerosol products presented in “ALICENET – an Italian network of automated lidar ceilometers for four-dimensional aerosol monitoring: infrastructure, data processing, and applications”, Zenodo [data set], https://doi.org/10.5281/zenodo.13332405, 2024.
Andrés Hernández, M. D., Hilboll, A., Ziereis, H., Förster, E., Krüger, O. O., Kaiser, K., Schneider, J., Barnaba, F., Vrekoussis, M., Schmidt, J., Huntrieser, H., Blechschmidt, A.-M., George, M., Nenakhov, V., Harlass, T., Holanda, B. A., Wolf, J., Eirenschmalz, L., Krebsbach, M., Pöhlker, M. L., Kalisz Hedegaard, A. B., Mei, L., Pfeilsticker, K., Liu, Y., Koppmann, R., Schlager, H., Bohn, B., Schumann, U., Richter, A., Schreiner, B., Sauer, D., Baumann, R., Mertens, M., Jöckel, P., Kilian, M., Stratmann, G., Pöhlker, C., Campanelli, M., Pandolfi, M., Sicard, M., Gómez-Amo, J. L., Pujadas, M., Bigge, K., Kluge, F., Schwarz, A., Daskalakis, N., Walter, D., Zahn, A., Pöschl, U., Bönisch, H., Borrmann, S., Platt, U., and Burrows, J. P.: Overview: On the transport and transformation of pollutants in the outflow of major population centres – observational data from the EMeRGe European intensive operational period in summer 2017, Atmos. Chem. Phys., 22, 5877–5924, https://doi.org/10.5194/acp-22-5877-2022, 2022.
Ansmann, A., Tesche, M., Seifert, P., Groß, S., Freudenthaler, V., Apituley, A., Wilson, K. M., Serikov, I., Linné, H., Heinold, B., Hiebsch, A., Schnell, F., Schmidt, J., Mattis, I., Wandinger, U., and Wiegner, M.: Ash and fine-mode particle mass profiles from EARLINET-AERONET observations over central Europe after the eruptions of the Eyjafjallajökull volcano in 2010, J. Geophys. Res., 116, D00U02, https://doi.org/10.1029/2010JD015567, 2011.
Balestrini, R., Diémoz, H., Freppaz, M., Delconte, C. A., Caschetto, M., and Matiatos, I.: Nitrogen atmospheric deposition in a high-altitude Alpine environment: A chemical and isotopic approach to investigate the influence from anthropized areas, Atmos. Environ., 328, 120513, https://doi.org/10.1016/j.atmosenv.2024.120513, 2024.
Barnaba, F. and Gobbi, G. P.: Lidar estimation of tropospheric aerosol extinction, surface area and volume: Maritime and desert-dust cases, J. Geophys. Res., 106, 3005–3018, https://doi.org/10.1029/2000JD900492, 2001.
Barnaba, F. and Gobbi, G. P.: Aerosol seasonal variability over the Mediterranean region and relative impact of maritime, continental and Saharan dust particles over the basin from MODIS data in the year 2001, Atmos. Chem. Phys., 4, 2367–2391, https://doi.org/10.5194/acp-4-2367-2004, 2004.
Barnaba, F., Putaud, J. P., Gruening, C., Dell'Acqua, A., and Dos Santos, S.: Annual cycle in co-located in situ, total-column, and height-resolved aerosol observations in the Po Valley (Italy): Implications for ground-level particulate matter mass concentration estimation from remote sensing, J. Geophys. Res.-Atmos., 115, D19209, https://doi.org/10.1029/2009JD013002, 2010.
Barnaba, F., Angelini, F., Curci, G., and Gobbi, G. P.: An important fingerprint of wildfires on the European aerosol load, Atmos. Chem. Phys., 11, 10487–10501, https://doi.org/10.5194/acp-11-10487-2011, 2011.
Barnaba, F., Bolignano, A., Di Liberto, L., Morelli, M., Lucarelli, F., Nava, S., Perrino, C., Canepari, S., Basart, S., Costabile, F., Dionisi, D., Ciampichetti, S., Sozzi, R., and Gobbi, G. P.: Desert dust contribution to PM10 loads in Italy: Methods and recommendations addressing the relevant European Commission Guidelines in support to the Air Quality Directive 2008/50, Atmos. Environ., 161, 288–305, https://doi.org/10.1016/j.atmosenv.2017.04.038, 2017.
Barnaba, F., Romero, N., Bolignano, A., Basart, S., Renzi, M., and Stafoggia, M.: Multiannual assessment of the desert dust impact on air quality in Italy combining PM10 data with physics-based and geostatistical models, Environ. Int., 163, 107204, https://doi.org/10.1016/j.envint.2022.107204, 2022.
Barnaba, F., Bellini, A., Diémoz, H., Bracci, A., Di Liberto, L., Pasqualini, F., Mona, L., Dupont, J.-C., Haeffelin, M., Delville, P., Kotthaus, S., Lapouge, F., and Pietras, C.: Operational aerosol monitoring through remote sensing in support of air quality networks, Actris Science Conference, Rennes, France, 13–16 May 2024.
Bedoya-Velásquez, A. E., Hoyos-Restrepo, M., Barreto, A., García, R. D., Romero-Campos, P. M., García, O., Ramos, R., Roininen, R., Toledano, C., and Sicard, M.: Estimation of the Mass Concentration of Volcanic Ash Using Ceilometers: Study of Fresh and Transported Plumes from La Palma Volcano, Remote Sens.-Basel, 14, 5680, https://doi.org/10.3390/rs14225680, 2022.
Brenot, H., Theys, N., Clarisse, L., van Gent, J., Hurtmans, D. R., Vandenbussche, S., Papagiannopoulos, N., Mona, L., Virtanen, T., Uppstu, A., Sofiev, M., Bugliaro, L., Vázquez-Navarro, M., Hedelt, P., Parks, M. M., Barsotti, S., Coltelli, M., Moreland, W., Scollo, S., Salerno, G., Arnold-Arias, D., Hirtl, M., Peltonen, T., Lahtinen, J., Sievers, K., Lipok, F., Rüfenacht, R., Haefele, A., Hervo, M., Wagenaar, S., Som de Cerff, W., de Laat, J., Apituley, A., Stammes, P., Laffineur, Q., Delcloo, A., Lennart, R., Rokitansky, C.-H., Vargas, A., Kerschbaum, M., Resch, C., Zopp, R., Plu, M., Peuch, V.-H., Van Roozendael, M., and Wotawa, G.: EUNADICS-AV early warning system dedicated to supporting aviation in the case of a crisis from natural airborne hazards and radionuclide clouds, Nat. Hazards Earth Syst. Sci., 21, 3367–3405, https://doi.org/10.5194/nhess-21-3367-2021, 2021.
Bucci, S., Cristofanelli, P., Decesari, S., Marinoni, A., Sandrini, S., Größ, J., Wiedensohler, A., Di Marco, C. F., Nemitz, E., Cairo, F., Di Liberto, L., and Fierli, F.: Vertical distribution of aerosol optical properties in the Po Valley during the 2012 summer campaigns, Atmos. Chem. Phys., 18, 5371–5389, https://doi.org/10.5194/acp-18-5371-2018, 2018.
Buxmann, J.: Investigating the seasonal fluctuations of the CHM15K Ceilometer calibration constant, Zenodo, https://doi.org/10.5281/zenodo.11108620, 2024.
Caicedo, V., Delgado, R., Sakai, R., Knepp, T., Williams, D., Cavender, K., Lefer, B., and Szykman, J.: An Automated Common Algorithm for Planetary Boundary Layer Retrievals Using Aerosol Lidars in Support of the U.S. EPA Photochemical Assessment Monitoring Stations Program, J. Atmos. Ocean. Tech., 37, 1847–1864, https://doi.org/10.1175/JTECH-D-20-0050.1, 2020.
Ceamanos, X., Coopman, Q., George, M., Riedi, J., Parrington, M., and Clerbaux, C.: Remote sensing and model analysis of biomass burning smoke transported across the Atlantic during the 2020 Western US wildfire season, Sci. Rep.-UK, 13, 16014, https://doi.org/10.1038/s41598-023-39312-1, 2023.
Chan, K. L., Wiegner, M., Flentje, H., Mattis, I., Wagner, F., Gasteiger, J., and Geiß, A.: Evaluation of ECMWF-IFS (version 41R1) operational model forecasts of aerosol transport by using ceilometer network measurements, Geosci. Model Dev., 11, 3807–3831, https://doi.org/10.5194/gmd-11-3807-2018, 2018.
Cimini, D., Haeffelin, M., Kotthaus, S., Löhnert, U., Martinet, P., O'Connor, E., Walden, C., Collaud Coen, M., and Preissler, J.: Towards the profiling of the atmospheric boundary layer at European scale–introducing the COST Action PROBE, Bulletin of Atmospheric Science and Technology, 1, 23–42, https://doi.org/10.1007/s42865-020-00003-8, 2020.
Collaud Coen, M., Andrews, E., Aliaga, D., Andrade, M., Angelov, H., Bukowiecki, N., Ealo, M., Fialho, P., Flentje, H., Hallar, A. G., Hooda, R., Kalapov, I., Krejci, R., Lin, N.-H., Marinoni, A., Ming, J., Nguyen, N. A., Pandolfi, M., Pont, V., Ries, L., Rodríguez, S., Schauer, G., Sellegri, K., Sharma, S., Sun, J., Tunved, P., Velasquez, P., and Ruffieux, D.: Identification of topographic features influencing aerosol observations at high altitude stations, Atmos. Chem. Phys., 18, 12289–12313, https://doi.org/10.5194/acp-18-12289-2018, 2018.
Córdoba-Jabonero, C., Sicard, M., Ansmann, A., del Águila, A., and Baars, H.: Separation of the optical and mass features of particle components in different aerosol mixtures by using POLIPHON retrievals in synergy with continuous polarized Micro-Pulse Lidar (P-MPL) measurements, Atmos. Meas. Tech., 11, 4775–4795, https://doi.org/10.5194/amt-11-4775-2018, 2018.
Corradini, S., Guerrieri, L., Lombardo, V., Merucci, L., Musacchio, M., Prestifilippo, M., Scollo, S., Silvestri, M., Spata, G., and Stelitano, D.: Proximal monitoring of the 2011-2015 Etna lava fountains using MSG-SEVIRI data, Geosciences, 8, 140, https://doi.org/10.3390/geosciences8040140, 2018.
Curci, G., Ferrero, L., Tuccella, P., Barnaba, F., Angelini, F., Bolzacchini, E., Carbone, C., Denier van der Gon, H. A. C., Facchini, M. C., Gobbi, G. P., Kuenen, J. P. P., Landi, T. C., Perrino, C., Perrone, M. G., Sangiorgi, G., and Stocchi, P.: How much is particulate matter near the ground influenced by upper-level processes within and above the PBL? A summertime case study in Milan (Italy) evidences the distinctive role of nitrate, Atmos. Chem. Phys., 15, 2629–2649, https://doi.org/10.5194/acp-15-2629-2015, 2015.
Di Bernardino, A., Iannarelli, A. M., Casadio, S., Perrino, C., Barnaba, F., Tofful, L., Campanelli, M., Di Liberto, L., Mevi, G., Siani, A. M, and Cacciani, M.: Impact of synoptic meteorological conditions on air quality in three different case studies in Rome, Italy, Atmos. Pollut. Res., 12, 76–88, https://doi.org/10.1016/j.apr.2021.02.019, 2021.
Diémoz, H., Barnaba, F., Magri, T., Pession, G., Dionisi, D., Pittavino, S., Tombolato, I. K. F., Campanelli, M., Della Ceca, L. S., Hervo, M., Di Liberto, L., Ferrero, L., and Gobbi, G. P.: Transport of Po Valley aerosol pollution to the northwestern Alps – Part 1: Phenomenology, Atmos. Chem. Phys., 19, 3065–3095, https://doi.org/10.5194/acp-19-3065-2019, 2019a.
Diémoz, H., Gobbi, G. P., Magri, T., Pession, G., Pittavino, S., Tombolato, I. K. F., Campanelli, M., and Barnaba, F.: Transport of Po Valley aerosol pollution to the northwestern Alps – Part 2: Long-term impact on air quality, Atmos. Chem. Phys., 19, 10129–10160, https://doi.org/10.5194/acp-19-10129-2019, 2019b.
Diémoz, H., Magri, T., Pession, G., Tarricone, C., Tombolato, I. K. F., Fasano, G., and Zublena, M.: Air Quality in the Italian Northwestern Alps during Year 2020: Assessment of the COVID-19 “Lockdown Effect” from Multi-Technique Observations and Models, Atmosphere, 12, 1006, https://doi.org/10.3390/atmos12081006, 2021.
Di Iorio, T., di Sarra, A., Sferlazzo, D. M., Cacciani, M., Meloni, D., Monteleone, F., Fuà, D., and Fiocco, G.: Seasonal evolution of the tropospheric aerosol vertical profile in the central Mediterranean and role of desert dust, J. Geophys. Res., 114, D02201, https://doi.org/10.1029/2008jd010593, 2009.
Dionisi, D., Barnaba, F., Diémoz, H., Di Liberto, L., and Gobbi, G. P.: A multiwavelength numerical model in support of quantitative retrievals of aerosol properties from automated lidar ceilometers and test applications for AOT and PM10 estimation, Atmos. Meas. Tech., 11, 6013–6042, https://doi.org/10.5194/amt-11-6013-2018, 2018.
Du, P., Kibbe, W. A., and Lin, S. M.: Improved peak detection in mass spectrum by incorporating continuous wavelet transform-based pattern matching, Bioinformatics, 22, 2059–2065, https://doi.org/10.1093/bioinformatics/btl355, 2006.
Fasano, G., Diémoz, H., Fountoulakis, I., Cassardo, C., Kudo, R., Siani, A. M., and Ferrero, L.: Vertical profile of the clear-sky aerosol direct radiative effect in an Alpine valley, by the synergy of ground-based measurements and radiative transfer simulations, Bulletin of Atmospheric Science and Technology, 2, 11, https://doi.org/10.1007/s42865-021-00041-w, 2021.
Ferrero, L., Riccio, A., Ferrini, B. S., D'Angelo, L., Rovelli, G., Casati, M., Angelini, F., Barnaba, F., Gobbi, G. P., Cataldi, M., and Bolzacchini, E.: Satellite AOD conversion into ground PM10, PM2.5 and PM1 over the Po valley (Milan, Italy) exploiting information on aerosol vertical profiles, chemistry, hygroscopicity and meteorology, Atmos. Pollut. Res., 10, 1895–1912, https://doi.org/10.1016/j.apr.2019.08.003, 2019.
Flentje, H., Claude, H., Elste, T., Gilge, S., Köhler, U., Plass-Dülmer, C., Steinbrecht, W., Thomas, W., Werner, A., and Fricke, W.: The Eyjafjallajökull eruption in April 2010 – detection of volcanic plume using in-situ measurements, ozone sondes and lidar-ceilometer profiles, Atmos. Chem. Phys., 10, 10085–10092, https://doi.org/10.5194/acp-10-10085-2010, 2010.
Flentje, H., Mattis, I., Kipling, Z., Rémy, S., and Thomas, W.: Evaluation of ECMWF IFS-AER (CAMS) operational forecasts during cycle 41r1–46r1 with calibrated ceilometer profiles over Germany, Geosci. Model Dev., 14, 1721–1751, https://doi.org/10.5194/gmd-14-1721-2021, 2021.
Fountoulakis, I., Papachristopoulou, K., Proestakis, E., Amiridis, V., Kontoes, C., and Kazadzis, S.: Effect of Aerosol Vertical Distribution on the Modeling of Solar Radiation, Remote Sens.-Basel, 14, 1143, https://doi.org/10.3390/rs14051143, 2022.
Giles, D. M., Sinyuk, A., Sorokin, M. G., Schafer, J. S., Smirnov, A., Slutsker, I., Eck, T. F., Holben, B. N., Lewis, J. R., Campbell, J. R., Welton, E. J., Korkin, S. V., and Lyapustin, A. I.: Advancements in the Aerosol Robotic Network (AERONET) Version 3 database – automated near-real-time quality control algorithm with improved cloud screening for Sun photometer aerosol optical depth (AOD) measurements, Atmos. Meas. Tech., 12, 169–209, https://doi.org/10.5194/amt-12-169-2019, 2019.
Giorgino, T.: Computing and Visualizing Dynamic Time Warping Alignments in R: The dtw Package, J. Stat. Softw., 31, 1–24, https://doi.org/10.18637/jss.v031.i07, 2009.
Giovannini, L., Ferrero, E., Karl, T., Rotach, M. W., Staquet, C., Trini Castelli, S., and Zardi, D.: Atmospheric Pollutant Dispersion over Complex Terrain: Challenges and Needs for Improving Air Quality Measurements and Modeling, Atmosphere, 11, 646, https://doi.org/10.3390/atmos11060646, 2020.
Gobbi, G. P. and Barnaba, F.: Atmospheric Backscatter and Lidars, in: Remote Sensing of Atmosphere and Ocean from Space: Models, Instruments and Techniques, edited by: Marzano, F. S. and Visconti, G., Advances in Global Change Research, vol. 13, Springer, Dordrecht, https://doi.org/10.1007/0-306-48150-2_1, 2002.
Gobbi, G. P., Barnaba, F., Blumthaler, M., Labow, G., and Herman, J. R.: Observed effects of particles nonsphericity on the retrieval of marine and desert dust aerosol optical depth by lidar, Atmos. Res., 61, 1–14, https://doi.org/10.1016/S0169-8095(01)00104-1, 2002.
Gobbi, G. P., Barnaba, F., Di Liberto, L., Bolignano, A., Lucarelli, F., Nava, S., Perrino, C., Pietrodangelo, A., Basart, S., Costabile, F., Dionisi, D., Rizza, U., Canepari, S., Sozzi, R., Morelli, M., Manigrasso, M., Drewnick, F., Struckmeier, C., Poenitz, K., and Wille, H.: An inclusive view of Saharan dust advections to Italy and the Central Mediterranean, Atmos. Environ., 201, 242–256, https://doi.org/10.1016/j.atmosenv.2019.01.002, 2019.
Haeffelin, M., Angelini, F., Morille, Y., Martucci, G., Frey, S., Gobbi, G. P., Lolli, S., O'Dowd, C. D., Sauvage, L., Xueref-Rémy, I., Wastine, B., and Feist D. G.: Evaluation of Mixing-Height Retrievals from Automatic Profiling Lidars and Ceilometers in View of Future Integrated Networks in Europe, Bound.-Lay. Meteorol., 143, 49–75, https://doi.org/10.1007/s10546-011-9643-z, 2012.
Haeffelin, M., Laffineur, Q., Bravo-Aranda, J.-A., Drouin, M.-A., Casquero-Vera, J.-A., Dupont, J.-C., and De Backer, H.: Radiation fog formation alerts using attenuated backscatter power from automatic lidars and ceilometers, Atmos. Meas. Tech., 9, 5347–5365, https://doi.org/10.5194/amt-9-5347-2016, 2016.
Hervo, M., Poltera, Y., and Haefele, A.: An empirical method to correct for temperature-dependent variations in the overlap function of CHM15k ceilometers, Atmos. Meas. Tech., 9, 2947–2959, https://doi.org/10.5194/amt-9-2947-2016, 2016.
Illingworth, A. J., Barker, H. W., Beljaars, A., Ceccaldi, M., Chepfer, H., Clerbaux, N., Cole, J., Delanoë, J., Domenech, C., Donovan, D. P., Fukuda, S., Hirakata, M., Hogan, R. J., Huenerbein, A., Kollias, P., Kubota T., Nakajima, T., Nakajima, T. Y., Nishizawa, T., Ohno, Y., Okamoto, H., Oki, R., Sato, K., Satoh, M., Shephard, M. W., Velázquez-Blázquez, A., Wandinger, U., Wehr, T., and van Zadelhoff, G. J.: The EarthCARE satellite: The next step forward in global measurements of clouds, aerosols, precipitation, and radiation, B. Am. Meteorol. Soc., 96, 1311–1332, https://doi.org/10.1175/BAMS-D-12-00227.1, 2015.
Intergovernmental Panel on Climate Change (IPCC): Climate Change 2022 – impacts, adaptation and vulnerability, Cambridge University Press, https://doi.org/10.1017/9781009325844, 2023.
Jänicke, L. K., Preusker, R., Docter, N., and Fischer, J.: Estimation of Aerosol Layer Height from OLCI Measurements in the O2A-Absorption Band over Oceans, Remote Sens.-Basel, 15, 4080, https://doi.org/10.3390/rs15164080, 2023.
Jozef, G. C., Cassano, J. J., Dahlke, S., Dice, M., Cox, C. J., and de Boer, G.: An overview of the vertical structure of the atmospheric boundary layer in the central Arctic during MOSAiC, Atmos. Chem. Phys., 24, 1429–1450, https://doi.org/10.5194/acp-24-1429-2024, 2024.
Karle, N. N., Sakai, R. K., Fitzgerald, R. M., Ichoku, C., Mercado, F., and Stockwell, W. R.: Systematic analysis of virga and its impact on surface particulate matter observations, Atmos. Meas. Tech., 16, 1073–1085, https://doi.org/10.5194/amt-16-1073-2023, 2023.
Koffi, E. N., Bergamaschi, P., Karstens, U., Krol, M., Segers, A., Schmidt, M., Levin, I., Vermeulen, A. T., Fisher, R. E., Kazan, V., Klein Baltink, H., Lowry, D., Manca, G., Meijer, H. A. J., Moncrieff, J., Pal, S., Ramonet, M., Scheeren, H. A., and Williams, A. G.: Evaluation of the boundary layer dynamics of the TM5 model over Europe, Geosci. Model Dev., 9, 3137–3160, https://doi.org/10.5194/gmd-9-3137-2016, 2016.
Körmöndi, B., Szkordilisz, F., Kotthaus, S., Haeffelin, M., Céspedes, J., Martinet, P., Jurcakova, K., Bellini, A., Diémoz, H., and Barnaba, F.: Impact of atmospheric boundary layer profiling: Urban environments (D1.5), Zenodo, https://doi.org/10.5281/zenodo.11584507, 2024.
Kotthaus, S. and Bravo Aranda, J. A.: Deliverable 2.1 Advanced ABL profiling: ABL characterisation, Zenodo, https://doi.org/10.5281/zenodo.11636591, 2024.
Kotthaus, S., Haeffelin, M., Drouin, M.-A., Dupont, J.-C., Grimmond, S., Haefele, A., Hervo, M., Poltera, Y., and Wiegner, M.: Tailored Algorithms for the Detection of the Atmospheric Boundary Layer Height from Common Automatic Lidars and Ceilometers (ALC), Remote Sens.-Basel, 12, 3259, https://doi.org/10.3390/rs12193259, 2020.
Kotthaus, S., Bravo-Aranda, J. A., Collaud Coen, M., Guerrero-Rascado, J. L., Costa, M. J., Cimini, D., O'Connor, E. J., Hervo, M., Alados-Arboledas, L., Jiménez-Portaz, M., Mona, L., Ruffieux, D., Illingworth, A., and Haeffelin, M.: Atmospheric boundary layer height from ground-based remote sensing: a review of capabilities and limitations, Atmos. Meas. Tech., 16, 433–479, https://doi.org/10.5194/amt-16-433-2023, 2023.
Klett J. D.: Lidar inversion with variable backscatter/extinction ratios, Appl. Optics, 24, 1638–1643, https://doi.org/10.1364/AO.24.001638, 1985.
Lelieveld, J., Berresheim, H., Borrmann, S., Crutzen, P. J., Dentener, F. J., Fischer, H., Feichter, J., Flatau, P. J., Heland, J., Holzinger, R., Korrmann, R., Lawrence, M. G., Levin, Z., Markowicz, K. M., Mihalopoulos, N., Minikin, A., Ramanathan, V., De Reus, M., Roelofs, G. J., Scheeren, H. A., Sciare, J., Schlager, H., Schultz, M., Siegmund, P., Steil, B., Stephanou, E. G., Stier, P., Traub, M., Warneke, C., Williams, J., and Ziereis, H.: Global air pollution crossroads over the Mediterranean, Science, 298, 794–799, https://doi.org/10.1126/science.1075457, 2002.
Mira-Salama, D., Van Dingenen, R., Gruening, C., Putaud, J.-P., Cavalli, F., Cavalli, P., Erdmann, N., Dell'Acqua, A., Dos Santos, S., Hjorth, J., Raes, F., and Jensen, N. R.: Using Föhn conditions to characterize urban and regional sources of particles, Atmos. Res., 90, 159–169, 2008.
Monteiro, A., Basart, S., Kazadzis, S., Votsis, A., Gkikas, A., Vandenbussche, S., Tobias, A., Gama, C., Pérez García-Pando, C., Terradellas, E., Notas, G., Middleton, K., Kushta, J., Amiridis, V., Lagouvardos, K., Kosmopoulos, P., Kotroni, V., Kanakidou, M., Mihalopoulos, N., Kalivitis, N., Dagsson-Waldhauserová, P., El-Askary, H., Sievers, K., Giannaros, T., Mona, L., Hirtl, M., Skomorowski, P., Virtanen, T., Christoudias, T., Di Mauro, B., Trippetta, S., Kutuzov, S., Meinander, O., and Nickovic, S.: Multi-sectoral impact assessment of an extreme African dust episode in the Eastern Mediterranean in March 2018, Sci. Total Environ., 843, 156861, https://doi.org/10.1016/j.scitotenv.2022.156861, 2022.
Moreira, G. A., Guerrero-Rascado J. L., Bravo-Aranda, J. A., Foyo-Moreno, I., Cazorla, A., Alados, I., Lyamani, H., Landulfo, E., and Alados-Arboledas, L.: Study of the planetary boundary layer height in an urban environment using a combination of microwave radiometer and ceilometer, Atmos. Res., 240, 104932, https://doi.org/10.1016/j.atmosres.2020.104932, 2020.
Mortier, A., Goloub, P., Podvin, T., Deroo, C., Chaikovsky, A., Ajtai, N., Blarel, L., Tanre, D., and Derimian, Y.: Detection and characterization of volcanic ash plumes over Lille during the Eyjafjallajökull eruption, Atmos. Chem. Phys., 13, 3705–3720, https://doi.org/10.5194/acp-13-3705-2013, 2013.
Napoli, A., Desbiolles, F., Parodi, A., and Pasquero, C.: Aerosol indirect effects in complex-orography areas: a numerical study over the Great Alpine Region, Atmos. Chem. Phys., 22, 3901–3909, https://doi.org/10.5194/acp-22-3901-2022, 2022.
National Academies of Sciences, Engineering, and Medicine: Thriving on Our Changing Planet: A Decadal Strategy for Earth Observation from Space, National Academies Press, https://doi.org/10.17226/24938, 2018.
Nicolae, D., Vasilescu, J., Talianu, C., Binietoglou, I., Nicolae, V., Andrei, S., and Antonescu, B.: A neural network aerosol-typing algorithm based on lidar data, Atmos. Chem. Phys., 18, 14511–14537, https://doi.org/10.5194/acp-18-14511-2018, 2018.
Osborne, M., Malavelle, F. F., Adam, M., Buxmann, J., Sugier, J., Marenco, F., and Haywood, J.: Saharan dust and biomass burning aerosols during ex-hurricane Ophelia: observations from the new UK lidar and sun-photometer network, Atmos. Chem. Phys., 19, 3557–3578, https://doi.org/10.5194/acp-19-3557-2019, 2019.
Osborne, M. J.: Comparison of CHM15k extinction and mass products from ALICEnet, A-Profiles and the UK Met Office, Zenodo, https://doi.org/10.5281/zenodo.11196654, 2024.
Osborne, M. J., de Leeuw, J., Witham, C., Schmidt, A., Beckett, F., Kristiansen, N., Buxmann, J., Saint, C., Welton, E. J., Fochesatto, J., Gomes, A. R., Bundke, U., Petzold, A., Marenco, F., and Haywood, J.: The 2019 Raikoke volcanic eruption – Part 2: Particle-phase dispersion and concurrent wildfire smoke emissions, Atmos. Chem. Phys., 22, 2975–2997, https://doi.org/10.5194/acp-22-2975-2022, 2022.
Papachristopoulou, K., Fountoulakis, I., Bais, A. F., Psiloglou, B. E., Papadimitriou, N., Raptis, I.-P., Kazantzidis, A., Kontoes, C., Hatzaki, M., and Kazadzis, S.: Effects of clouds and aerosols on downwelling surface solar irradiance nowcasting and short-term forecasting, Atmos. Meas. Tech., 17, 1851–1877, https://doi.org/10.5194/amt-17-1851-2024, 2024.
Papagiannopoulos, N., D'Amico, G., Gialitaki, A., Ajtai, N., Alados-Arboledas, L., Amodeo, A., Amiridis, V., Baars, H., Balis, D., Binietoglou, I., Comerón, A., Dionisi, D., Falconieri, A., Fréville, P., Kampouri, A., Mattis, I., Mijić, Z., Molero, F., Papayannis, A., Pappalardo, G., Rodríguez-Gómez, A., Solomos, S., and Mona, L.: An EARLINET early warning system for atmospheric aerosol aviation hazards, Atmos. Chem. Phys., 20, 10775–10789, https://doi.org/10.5194/acp-20-10775-2020, 2020.
Pappalardo, G., Amodeo, A., Apituley, A., Comeron, A., Freudenthaler, V., Linné, H., Ansmann, A., Bösenberg, J., D'Amico, G., Mattis, I., Mona, L., Wandinger, U., Amiridis, V., Alados-Arboledas, L., Nicolae, D., and Wiegner, M.: EARLINET: towards an advanced sustainable European aerosol lidar network, Atmos. Meas. Tech., 7, 2389–2409, https://doi.org/10.5194/amt-7-2389-2014, 2014.
Poltera, Y., Martucci, G., Collaud Coen, M., Hervo, M., Emmenegger, L., Henne, S., Brunner, D., and Haefele, A.: PathfinderTURB: an automatic boundary layer algorithm. Development, validation and application to study the impact on in situ measurements at the Jungfraujoch, Atmos. Chem. Phys., 17, 10051–10070, https://doi.org/10.5194/acp-17-10051-2017, 2017.
Querol, X., Pey, J., Pandolfi, M., Alastuey, A., Cusack, M., Pérez, N., Moreno, T., Viana, M., Mihalopoulos, N., Kallos, G., and Kleanthous, S.: African dust contributions to mean ambient PM10 mass-levels across the Mediterranean Basin, Atmos. Environ., 43, 4266–4277, https://doi.org/10.1016/j.atmosenv.2009.06.013, 2009.
Ravnik, L., Laffineur, Q., Ferrario, M. E., Diémoz, H., and Kotthaus, S.: Impact of atmospheric boundary layer profiling for Environmental agencies and air quality, Zenodo, https://doi.org/10.5281/zenodo.11176517, 2024.
Remer, L. A., Levy, R. C., and Martins, J. V.: Opinion: Aerosol remote sensing over the next 20 years, Atmos. Chem. Phys., 24, 2113–2127, https://doi.org/10.5194/acp-24-2113-2024, 2024.
Rizza, U., Barnaba, F., Miglietta, M. M., Mangia, C., Di Liberto, L., Dionisi, D., Costabile, F., Grasso, F., and Gobbi, G. P.: WRF-Chem model simulations of a dust outbreak over the central Mediterranean and comparison with multi-sensor desert dust observations, Atmos. Chem. Phys., 17, 93–115, https://doi.org/10.5194/acp-17-93-2017, 2017.
Rizza, U., Avolio, E., Morichetti, M., Di Liberto, L., Bellini, A., Barnaba, F., Virgili, S., Passerini, G., and Mancinelli, E.: On the Interplay between Desert Dust and Meteorology Based on WRF-Chem Simulations and Remote Sensing Observations in the Mediterranean Basin, Remote Sens.-Basel, 15, 435, https://doi.org/10.3390/rs15020435, 2023.
Ryder, C. L., Bézier, C., Dacre, H. F., Clarkson, R., Amiridis, V., Marinou, E., Proestakis, E., Kipling, Z., Benedetti, A., Parrington, M., Rémy, S., and Vaughan, M.: Aircraft engine dust ingestion at global airports, Nat. Hazards Earth Syst. Sci., 24, 2263–2284, https://doi.org/10.5194/nhess-24-2263-2024, 2024.
Salgueiro, V., Guerrero-Rascado, J. L., Costa, M.J., Román, R., Cazorla, A., Serrano, A., Molero, F., Sicard, M., Córdoba-Jabonero, C., and Bortoli, D.: Characterization of Tajogaite Volcanic Plumes Detected over the Iberian Peninsula from a Set of Satellite and Ground-Based Remote Sensing Instrumentation, Remote Sens. Environ., 295, 113684, https://doi.org/10.1016/j.rse.2023.113684, 2023.
Sandrini, S., van Pinxteren, D., Giulianelli, L., Herrmann, H., Poulain, L., Facchini, M. C., Gilardoni, S., Rinaldi, M., Paglione, M., Turpin, B. J., Pollini, F., Bucci, S., Zanca, N., and Decesari, S.: Size-resolved aerosol composition at an urban and a rural site in the Po Valley in summertime: implications for secondary aerosol formation, Atmos. Chem. Phys., 16, 10879–10897, https://doi.org/10.5194/acp-16-10879-2016, 2016.
Scollo, S., Prestifilippo, M., Bonadonna, C., Cioni, R., Corradini, S., Degruyter, W., Rossi, E., Silvestri, M., Biale, E., Carparelli, G., Cassisi, C., Merucci, L., Musacchio, M., and Pecora, E.: Near-Real-Time Tephra Fallout Assessment at Mt. Etna, Italy, Remote Sens.-Basel, 11, 2987, https://doi.org/10.3390/rs11242987, 2019.
Serafin, S., Adler, B., Cuxart, J., De Wekker, S. F. J., Gohm, A., Grisogono, B., Kalthoff, N., Kirshbaum, D. J., Rotach, M. W., Schmidli, J., Stiperski, I., Večenaj, Z., and Zardi, D.: Exchange Processes in the Atmospheric Boundary Layer Over Mountainous Terrain, Atmosphere, 9, 102, https://doi.org/10.3390/atmos9030102, 2018.
Shang, X., Mielonen, T., Lipponen, A., Giannakaki, E., Leskinen, A., Buchard, V., Darmenov, A. S., Kukkurainen, A., Arola, A., O'Connor, E., Hirsikko, A., and Komppula, M.: Mass concentration estimates of long-range-transported Canadian biomass burning aerosols from a multi-wavelength Raman polarization lidar and a ceilometer in Finland, Atmos. Meas. Tech., 14, 6159–6179, https://doi.org/10.5194/amt-14-6159-2021, 2021.
Shang, X., Lipponen, A., Filioglou, M., Sundström, A.-M., Parrington, M., Buchard, V., Darmenov, A. S., Welton, E. J., Marinou, E., Amiridis, V., Sicard, M., Rodríguez-Gómez, A., Komppula, M., and Mielonen, T.: Monitoring biomass burning aerosol transport using CALIOP observations and reanalysis models: a Canadian wildfire event in 2019, Atmos. Chem. Phys., 24, 1329–1344, https://doi.org/10.5194/acp-24-1329-2024, 2024.
Shimizu, A., Nishizawa, T., Jin, Y., Kim, S.-W., Wang, Z., Batdorj, D., and Sugimoto, N.: Evolution of a lidar network for tropospheric aerosol detection in East Asia, Opt. Eng., 56, 1–12, https://doi.org/10.1117/1.OE.56.3.031219, 2016.
Stull, R. B.: Boundary Conditions and Surface Forcings, in: An Introduction to Boundary Layer Meteorology, Springer Netherlands, Dordrecht, 251–294, https://doi.org/10.1007/978-94-009-3027-8_7, 1988.
Tesche, M., Ansmann, A., Müller, D., Althausen, D., Engelmann, R., Freudenthaler, V., and Groß, S.: Vertically resolved separation of dust and smoke over Cape Verde using multiwavelength Raman and polarisation lidars during Saharan Mineral Dust Experiment 2008, J. Geophys. Res., 114, D13202, https://doi.org/10.1029/2009JD011862, 2009.
Tositti, L., Brattich, E., Cassardo, C., Morozzi, P., Bracci, A., Marinoni, A., Di Sabatino, S., Porcù, F., and Zappi, A.: Development and evolution of an anomalous Asian dust event across Europe in March 2020, Atmos. Chem. Phys., 22, 4047–4073, https://doi.org/10.5194/acp-22-4047-2022, 2022.
Uchiyama, A., Matsunaga, T., and Yamazaki, A.: The instrument constant of sky radiometers (POM-02) – Part 2: Solid view angle, Atmos. Meas. Tech., 11, 5389–5402, https://doi.org/10.5194/amt-11-5389-2018, 2018.
Valmassoi, A., Keller, J. D., Kleist, D. T., English, S., Ahrens, B., Ďurán, I. B., Bauernschubert, E., Bosilovich, M. G., Fujiwara, M., Hersbach, H., Lei, L., Löhnert, U., Mamnun, N., Martin, C. R., Moore, A., Niermann, D., Ruiz, J. J., and Scheck, L.: Current Challenges and Future Directions in Data Assimilation and Reanalysis, B. Am. Meteorol. Soc., 104, E756–E767, https://doi.org/10.1175/bams-d-21-0331.1, 2023.
van Hove, M. and Diémoz, H.: Seasonal variation in the Rayleigh calibration factor of Automatic Lidar- Ceilometers: amplitude across Europe and possible explanations, Zenodo, https://doi.org/10.5281/zenodo.11074353, 2024.
van Zadelhoff, G.-J., Donovan, D. P., and Wang, P.: Detection of aerosol and cloud features for the EarthCARE atmospheric lidar (ATLID): the ATLID FeatureMask (A-FM) product, Atmos. Meas. Tech., 16, 3631–3651, https://doi.org/10.5194/amt-16-3631-2023, 2023.
Welton, E. J., Stewart, S. A., Lewis, J. R., Belcher, L. R., Campbell, J. R., and Lolli, S.: Status of the NASA Micro Pulse Lidar Network (MPLNET): overview of the network and future plans, new version 3 data products, and the polarised MPL, EPJ Web Conf., 176, 09003, https://doi.org/10.1051/EPJCONF/201817609003, 2018.
WHO: WHO global air quality guidelines: particulate matter (PM2.5 and PM10), ozone, nitrogen dioxide, sulphur dioxide and carbon monoxide, Licence: CC BY-NC-SA 3.0 IGO, https://iris.who.int/handle/10665/345329 (last access: 25 July 2024), 2021.
Wiegner, M. and Gasteiger, J.: Correction of water vapor absorption for aerosol remote sensing with ceilometers, Atmos. Meas. Tech., 8, 3971–3984, https://doi.org/10.5194/amt-8-3971-2015, 2015.
Wiegner, M. and Geiß, A.: Aerosol profiling with the Jenoptik ceilometer CHM15kx, Atmos. Meas. Tech., 5, 1953–1964, https://doi.org/10.5194/amt-5-1953-2012, 2012.
Wiegner, M., Madonna, F., Binietoglou, I., Forkel, R., Gasteiger, J., Geiß, A., Pappalardo, G., Schäfer, K., and Thomas, W.: What is the benefit of ceilometers for aerosol remote sensing? An answer from EARLINET, Atmos. Meas. Tech., 7, 1979–1997, https://doi.org/10.5194/amt-7-1979-2014, 2014.
Winker, D. M., Pelon, J., Coakley, J. A., Ackerman, S. A., Charlson, R. J., Colarco, P. R., Flamant, P., Fu, Q., Hoff, R. M., and Kittaka, C.: The CALIPSO mission: A global 3D view of aerosols and clouds, B. Am. Meteorol. Soc., 91, 1211–1230, https://doi.org/10.1175/2010BAMS3009.1, 2010.
- Abstract
- Introduction
- ALICENET sites and instruments
- ALICENET data processing and relevant products
- Potential of 4D near-real-time aerosol monitoring
- Conclusions and future perspectives
- Appendix A: List of abbreviations
- Data availability
- Author contributions
- Competing interests
- Disclaimer
- Special issue statement
- Acknowledgements
- Financial support
- Review statement
- References
- Supplement
- Abstract
- Introduction
- ALICENET sites and instruments
- ALICENET data processing and relevant products
- Potential of 4D near-real-time aerosol monitoring
- Conclusions and future perspectives
- Appendix A: List of abbreviations
- Data availability
- Author contributions
- Competing interests
- Disclaimer
- Special issue statement
- Acknowledgements
- Financial support
- Review statement
- References
- Supplement