the Creative Commons Attribution 4.0 License.
the Creative Commons Attribution 4.0 License.
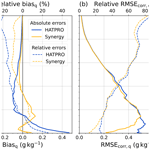
Combining low- and high-frequency microwave radiometer measurements from the MOSAiC expedition for enhanced water vapour products
Hannes J. Griesche
Mario Mech
Susanne Crewell
Kerstin Ebell
In the central Arctic, high-quality water vapour observations are sparse due to the low density of meteorological stations and uncertainties in satellite remote sensing. Different reanalyses also disagree on the amount of water vapour in the central Arctic. The Multidisciplinary drifting Observatory for the Study of the Arctic Climate (MOSAiC) expedition provides comprehensive observations that are suitable for evaluating satellite products and reanalyses. Radiosonde observations provide high-quality water vapour estimates with a high vertical but a low temporal resolution. Observations from the microwave radiometers (MWRs) on board the research vessel Polarstern complement these observations through high temporal resolution. In this study, we demonstrate the high accuracy of the combination of the two MWRs HATPRO (Humidity and Temperature Profiler) and MiRAC-P (Microwave Radiometer for Arctic Clouds – Passive). For this purpose, we developed new retrievals of integrated water vapour (IWV) and profiles of specific humidity and temperature using a neural network approach, including observations from both HATPRO and MiRAC-P to utilize their different water vapour sensitivity. The retrievals were trained with the European Centre for Medium-Range Weather Forecasts (ECMWF) reanalysis version 5 (ERA5) and synthetic MWR observations simulated with the Passive and Active Microwave radiative TRAnsfer tool (PAMTRA). We applied the retrievals to synthetic and real observations and evaluated them with ERA5 and radiosondes launched during MOSAiC, respectively. To assess the benefit of the combination of HATPRO and MiRAC-P compared to single MWR retrievals, we compared the errors with respect to MOSAiC radiosondes and computed the vertical information content of the specific humidity profiles. The root mean squared error (RMSE) of IWV was reduced by up to 15 %. Specific humidity biases and RMSE were reduced by up to 75 % and 50 %, respectively. The vertical information content of specific humidity could be increased from 1.7 to 2.4 degrees of freedom. We also computed relative humidity from the retrieved temperature and specific humidity profiles and found that RMSE was reduced from 45 % to 15 %. Finally, we show a case study demonstrating the enhanced humidity profiling capabilities compared to the standard HATPRO-based retrievals. The vertical resolution of the retrieved specific humidity profiles is still low compared to radiosondes, but the case study revealed the potential to resolve major humidity inversions. To what degree the MWR combination detects humidity inversions, also compared to satellites and reanalyses, will be part of future work.
- Article
(5032 KB) - Full-text XML
- BibTeX
- EndNote
The amplified warming of the Arctic, known as Arctic amplification, is a well-established phenomenon and has been discussed in several studies (e.g. Screen et al., 2012; Screen and Simmonds, 2010; Rantanen et al., 2022; Wendisch et al., 2023). Arctic amplification is caused by several positive climate feedback mechanisms, such as the ice albedo and the lapse rate feedback (Serreze and Barry, 2011; Wendisch et al., 2023). Following the Clausius–Clapeyron relation, a warmer atmosphere can contain more water vapour before condensation occurs. Higher water vapour loads enhance the greenhouse effect (stronger emission in the thermal infrared) and thus increase temperatures at the surface (Held and Soden, 2000; Graversen and Wang, 2009; Ghatak and Miller, 2013). This positive feedback loop is known as the water vapour feedback, and its role in Arctic amplification is still under investigation.
In the past decades, a moistening trend has been observed on a global scale (Chen and Liu, 2016; Allan et al., 2022) and also regionally in the Arctic (Ghatak and Miller, 2013; Maturilli and Kayser, 2017; Parracho et al., 2018; Rinke et al., 2019; Serreze et al., 2012). The relative increase in the vertically integrated water vapour (IWV) is strongest in the Arctic (Chen and Liu, 2016). However, IWV trends have a high spatial heterogeneity and depend on the season (Parracho et al., 2018; Rinke et al., 2019). Many studies have relied on atmospheric reanalyses, which assimilate measurements from synoptic stations, particularly radiosondes and satellites. However, ground-based observations are sparse and satellite observations have different challenges in the Arctic (Crewell et al., 2021): the derivation of water vapour products from visible and infrared observations is hindered by darkness or clouds, and satellite products from microwave observations are uncertain due to the high and variable sea ice emissivity (Mathew et al., 2008; Wang et al., 2017; Scarlat et al., 2017). The lack of ground-based observations and difficulties in satellite remote sensing in the Arctic lead to high uncertainties in water vapour products in reanalyses (Crewell et al., 2021; Parracho et al., 2018; Chen and Liu, 2016; Graham et al., 2019b). Therefore, it is not surprising to find a large spread of the IWV trend among reanalyses, often larger than the median trend itself for certain seasons and regions (Rinke et al., 2019).
A special feature of the Arctic is the high occurrence of humidity inversions, which are height layers where the water vapour concentration increases with height (Devasthale et al., 2011; Vihma et al., 2011; Nygård et al., 2014; Maturilli and Kayser, 2017; Naakka et al., 2018). Humidity inversions are strongly coupled with temperature inversions (Tjernström et al., 2004), which form due to radiative cooling in clear-sky conditions in winter or due to sea ice melt or advection of warm and moist air above the boundary layer in summer (Graversen et al., 2008; Devasthale et al., 2010; Tjernström et al., 2019). Humidity inversions are a moisture source for the formation and maintenance of clouds through entrainment at the cloud top (Nygård et al., 2014). It is therefore important to have humidity observations with a sufficiently high vertical resolution that allows capturing this characteristic feature of the Arctic humidity profile. Additionally, the vertical water vapour distribution affects the downward thermal infrared radiation. Tjernström et al. (2019) showed that in cases when humidity inversions were present, the downward thermal infrared radiation was higher, fostered by fog or low cloud formation.
Current reanalyses have difficulties in correctly representing the stable stratification of Arctic winter conditions (Wang et al., 2019; Yu et al., 2021; Graham et al., 2019a). For example, the widely used European Centre for Medium-Range Weather Forecasts (ECMWF) reanalysis version 5 (ERA5) (Hersbach et al., 2020), which is among the best-performing global reanalyses in the Arctic, still shows positive near-surface air temperature and humidity biases (Graham et al., 2019a; Avila-Diaz et al., 2021; Loeb et al., 2022; Yu et al., 2021). The biases are highest in cold stable conditions found over sea ice in winter and smaller in summer or over the open Arctic Ocean (e.g. Fram Strait; Wang et al., 2019; Graham et al., 2019b). Herrmannsdörfer et al. (2023) suggested that ERA5 does not sufficiently represent sea ice thickness and snow depth. Difficulties representing stable conditions and positive biases of temperature and humidity at the surface result in errors in the temperature and humidity profiles of ERA5 (and other reanalyses).
It follows that reanalyses and satellite products struggle with the representation of water vapour in the Arctic. To evaluate the accuracy of water vapour in current reanalyses and satellite products, we need reference measurements. However, reliable and high-quality water vapour measurements in the central Arctic are currently only available through field campaigns. The Multidisciplinary drifting Observatory for the Study of the Arctic Climate (MOSAiC; Shupe et al., 2022) expedition, where the research vessel (RV) Polarstern (Knust, 2017) was frozen into the ice to observe the Arctic climate for a full annual cycle, provides unique observations for this purpose. Radiosonde measurements (Maturilli et al., 2021) yield IWV and humidity profiles with a high vertical but low temporal resolution (3–6-hourly). Additionally, water vapour products have been derived from upward-looking microwave radiometers (MWRs) that were mounted on the OCEANET container (Macke et al., 2010; Engelmann et al., 2021) at the bow of RV Polarstern: Walbröl et al. (2022c) created retrievals of IWV and profiles of absolute humidity and temperature from the low-frequency Humidity and Temperature Profiler (HATPRO; Rose et al., 2005) and an IWV product specifically designed for dry conditions from the high-frequency Microwave Radiometer for Arctic Clouds – Passive (MiRAC-P; Mech et al., 2019a). The MWR products have a high temporal resolution (almost every second), but the humidity profile from HATPRO is coarse, with less than 2 degrees of freedom (Löhnert et al., 2009).
The high-frequency observations from MiRAC-P have a high sensitivity to atmospheric water vapour in dry conditions (IWV < 10 kg m−2) but get saturated in humid conditions (IWV ≥ 10 kg m−2; Cadeddu et al., 2007, 2022; Fionda et al., 2019). In contrast, the low-frequency observations from HATPRO have a high sensitivity in humid conditions but a weak signal in the dry conditions of the Arctic in winter. The complementary moisture sensitivity of HATPRO and MiRAC-P motivates the synergy of both instruments, as done for IWV in e.g. Cadeddu et al. (2009).
In this study, we develop retrievals of water vapour products by combining observations from HATPRO and MiRAC-P to improve the vertical resolution of specific humidity profiles and reduce errors compared to single MWR retrievals. We retrieved specific humidity instead of absolute humidity because it is a more commonly used humidity measure in atmospheric reanalyses and satellite products. Specifically, we answer the following questions.
-
How much are IWV and humidity profile errors reduced compared to single-instrument retrievals and what is the influence of using different retrieval setups?
-
What is the vertical information content benefit for humidity retrievals when combining two MWRs with different moisture sensitivity?
-
Is the vertical information content sensitive to cloud presence, temperature, or water vapour amount?
The paper is structured as follows: in Sect. 2, we start with a description of the data used for the retrieval development and the measurements from the MOSAiC expedition, which will be used for the application and evaluation of the retrieval. In Sect. 3, we elaborate on the preparation of the retrieval development data before giving details on the retrieval setup and vertical information content estimation. Afterwards, we evaluate the retrieval in Sect. 4 and estimate the information benefit in Sect. 5 before concluding the paper in Sect. 6 by answering the questions raised above.
2.1 Retrieval development data
Radiosondes are commonly used for the evaluation of temperature and humidity profile retrievals because of the high vertical resolution and accuracy (e.g. Cimini et al., 2010; Löhnert and Maier, 2012). Due to the lack of radiosonde stations and uncertain water vapour observations from satellites, we selected the ERA5 reanalysis (Hersbach et al., 2020) as a data source for the retrieval development. With a horizontal resolution of 31 km and 137 vertical levels, it has the highest horizontal and vertical resolution of all current global reanalyses. The high vertical resolution might be beneficial for developing humidity profile retrievals because a low vertical resolution could constrain the retrieval from reaching its true potential. ERA5 data are available for 1940–present with an hourly resolution. Despite having slightly higher biases in near-surface air temperatures and humidity in cold stable conditions over sea ice than other reanalyses, ERA5 overall performs best in the Arctic, especially concerning the representation of clouds and precipitation (Graham et al., 2019a). The better representation of clouds and precipitation is beneficial for the simulation of microwave radiances for the retrieval development (described in Sect. 3.1). Also, extreme precipitation and temperature events are better captured by ERA5 than other reanalyses (Avila-Diaz et al., 2021; Wang et al., 2019; Loeb et al., 2022).
2.2 MOSAiC observations for retrieval application and evaluation
RV Polarstern drifted with an ice floe from 4 October 2019 in the Laptev Sea across the central Arctic Ocean until it approached the marginal ice zone in the Fram Strait on 31 July 2020. Between mid-May and mid-June 2020, RV Polarstern had to leave the floe for logistical reasons. To capture the refreezing period of the ice, RV Polarstern drifted with a second ice floe close to the North Pole from 21 August to 20 September 2020. In early October 2020, RV Polarstern left the sea ice.
2.2.1 Radiosondes
Throughout MOSAiC, Vaisala RS41 radiosondes were launched from RV Polarstern at the standard synoptic times (00:00, 06:00, 12:00, and 18:00 UTC). The actual launch time is usually around 1 h before the respective synoptic time due to the relatively slow ascent rates of about 5 m s−1. During intense observation periods, additional radiosondes were launched at 03:00, 09:00, 15:00, and 21:00 UTC. Here, we use all radiosonde level 2 data from 1 October 2019 to 1 October 2020 (Maturilli et al., 2021). Radiosondes provide temperature, pressure, and relative humidity with accuracies of 0.2–0.4 K, 0.04–1.0 hPa, and 3 %–4 %, respectively. With a measurement frequency of 1 Hz, the vertical resolution is about 5 m. For comparison with the retrievals, the radiosonde data have been interpolated onto the retrieval height grid (see Sect. 3.1).
2.2.2 Cloudnet and surface meteorology measurements
To evaluate the presented retrievals in different atmospheric conditions, we included additional datasets from the MOSAiC expedition: to distinguish between freezing and non-freezing conditions at the surface (temperatures below and above 273.15 K), the 2 m temperature measurements from the tower at the Met City site (Cox et al., 2023) were used. The Met City site was located within the central observatory, only a few hundred metres away from RV Polarstern. Additionally, we identified cloudy scenes using the Cloudnet retrieval products (Griesche et al., 2024). Cloudnet uses a synergy of passive and active atmospheric remote sensing to provide profiles of cloud macro- and microphysical properties (liquid and ice water content, effective radii of liquid droplets and ice crystals) with a time and height resolution of 30 s and 30 m, respectively (Illingworth et al., 2007; Tukiainen et al., 2020).
Cloudnet delivers e.g. a classification of the atmospheric conditions, distinguishing between clear sky, different cloud types (ice, liquid, mixed-phase), and the presence of aerosols and insects for each time–height pixel. Because of technical limitations, the Cloudnet product starts at a height of 182 m and can therefore miss the presence of low-level stratus clouds, which are common in the Arctic (Gierens et al., 2020; Griesche et al., 2020). The additional low-level stratus detection developed by Griesche et al. (2020) was used to mask these cases.
In this study, clear-sky conditions were identified using Cloudnet target classification data (Engelmann et al., 2023) and the low-level stratus mask (Griesche et al., 2023) where quality flags indicated good quality (also including the Cloudnet-issued dataset; Griesche and Seifert, 2023). As we compare our retrievals with radiosonde measurements, we selected Cloudnet data at times of the radiosonde launch to 15 min after the launch. A radiosonde launch is considered clear-sky when no low-level stratus clouds were present and the Cloudnet target classification indicated either clear sky, aerosols, or insects.
2.2.3 Microwave radiometers
The two upward-looking microwave radiometers, HATPRO and MiRAC-P, measure radiation emitted from water vapour, oxygen, and hydrometeors. Measured radiances are typically expressed as brightness temperatures (TBs). HATPRO detects radiances in seven channels between 22.24 and 31.4 GHz (K-band) and in seven channels between 51.26 and 58 GHz (V-band). MiRAC-P has a double-sideband receiver that measures radiances at six frequencies from 183.31±0.6 to 183.31±7.5 GHz (G-band) and a two-channel receiver for 243 and 340 GHz. At MiRAC-P frequencies, the scattering of radiation by hydrometeors is relevant, and the contribution of the continuum water vapour absorption is stronger (Rosenkranz, 1998).
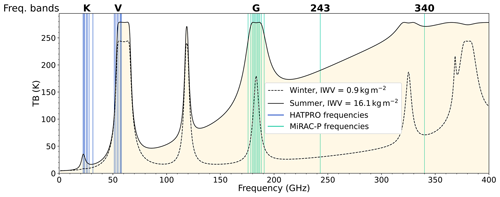
Figure 1Brightness temperatures (TBs) from 1 to 400 GHz simulated with PAMTRA for two radiosondes launched from RV Polarstern during MOSAiC (winter: 5 March 2020, 06:00 UTC; summer: 6 August 2020, 00:00 UTC). The dashed (solid) black line shows the TBs simulated with meteorological data from the winter (summer) radiosonde. The blue (cyan) lines indicate the frequencies at which HATPRO (MiRAC-P) measures. The labels K, V, G, 243, and 340 represent abbreviations for sets of frequency channels (bands) of HATPRO and MiRAC-P.
Figure 1 shows TBs simulated with the Passive and Active Microwave radiative TRAnsfer tool (PAMTRA; Mech et al., 2020) using two clear-sky radiosondes from MOSAiC (winter: 5 March 2020, 06:00 UTC; summer: 6 August 2020, 00:00 UTC). A higher atmospheric opacity generally results in higher TBs in the zenith. In the K-band channels of HATPRO and the G-band channels of MiRAC-P, which are located around resonant water vapour absorption lines, the different water vapour loads of winter and summer can be distinguished well by their large TB differences of up to 40 K in the K-band and more than 100 K in the G-band. Also in MiRAC-P's high-frequency channels at 243 and 340 GHz, TB differences are larger than at K-band frequencies (up to 200 K) due to continuum water vapour absorption. At the K-band frequencies, the relation between TBs and IWV is rather linear and becomes more nonlinear for the higher frequencies (G-band and above).
Observations along resonant water vapour absorption lines are well suited to derive IWV and humidity profiles (e.g. Crewell et al., 2001; Cadeddu et al., 2007; Cimini et al., 2010; Perro et al., 2016). Because of the high water vapour sensitivity, most of the G-band channels are saturated in the summer case, meaning they do not observe radiances from the entire atmospheric column. In contrast, the K-band channels show almost no water vapour signal in the extremely dry winter case (IWV of 0.9 kg m−2), while there is still a strong signal in the G-band.
Furthermore, higher TBs in summer compared to winter are caused by higher temperatures of the emitting gases and hydrometeors (Fig. 1). The V-band channels of HATPRO lie around the oxygen absorption complex and can be used for temperature profiling (Rose et al., 2005; Löhnert and Maier, 2012). As explained in Walbröl et al. (2022c), HATPRO also measured atmospheric radiances at different elevation angles every 30 min during MOSAiC, allowing for more detailed temperature profile retrievals in the lower troposphere (boundary layer temperature profiles).
In this study, we generally used TB measurements where flags indicate good quality (Walbröl et al., 2022c). We identified a few rain events between late May and late June 2020 that were not flagged by visual inspection. The quality flags have been updated. Additionally, we checked whether other flag values could be accepted and found that a receiver sanity flag was often set although the data looked reasonable. Therefore, we also included those data in our analysis. Times before the first successful calibration of both MWRs (22 October 2019, 05:40 UTC) have been excluded.
For the information benefit analysis, we compared the new synergistic retrievals to the single-instrument retrievals developed by Walbröl et al. (2022c), i.e. the two IWV products (HATPRO and MiRAC-P), and profiles of temperature and absolute humidity from HATPRO (Ebell et al., 2022; Walbröl et al., 2022b). We converted the retrieved absolute to specific humidity using the retrieved temperature profiles and air pressure from radiosondes. All retrieved quantities were averaged over 15 min, starting at the launch time of each radiosonde, for comparison with MOSAiC radiosondes. For boundary layer temperature profiles, we extended the averaging window to 30 min before and 30 min after each radiosonde launch due to the lower sampling rate.
The retrieval of an atmospheric state vector x (e.g. specific humidity profile) from an observation vector y (e.g. TBs at different frequencies) is an inverse problem. In its simplest form, the inverse problem can be formulated as , where F is the forward operator (e.g. radiative transfer model; here, PAMTRA). In atmospheric remote sensing, inverse problems are often ill-conditioned because small changes in observations can lead to large changes in the retrieved state vector and many different atmospheric states can lead to the same observations. Furthermore, the inverse problem is ill-posed because the radiative transfer equation cannot be inverted in a direct way.
The challenge is to find the most probable and realistic state of the atmosphere that fits the observations. In physical retrievals (e.g. optimal estimation; Rodgers, 2008; Ebell et al., 2017), the state vector x is adapted as long as the forward-simulated observations F(x) do not agree with the actual observations y within a given uncertainty range. Physical retrievals are computationally expensive but provide physically consistent state vectors and uncertainty estimation. However, at the high frequencies of MiRAC-P, the scattering of radiation by frozen hydrometeors cannot be neglected and may therefore introduce uncertainties in the radiative transfer calculations needed for the forward simulation F(x). The retrieval would require assumptions on hydrometeor properties (concentration, size, shape, orientation) or further hydrometeor observations, making it dependent on the availability of such observations.
Statistical retrievals are computationally cheap approaches that are also well established and provide similarly good results as physical retrievals (Solheim et al., 1998). In statistical retrievals, empirical relations are used to map observations to the state vector. The statistical relationship between observations and the state vector must be trained with large datasets covering the conditions of the area of interest. Regression or deep learning algorithms are examples of statistical retrievals. In this study, we use neural networks (NNs) because they can deal better with the nonlinear relationship between IWV and TB measurements in the G-band compared to regression. During the development of the MiRAC-P-only retrieval (Walbröl et al., 2022c), tests showed that the IWV retrieved with a multiple nonlinear regression had a significantly higher spread than when retrieved with NNs.
3.1 Retrieval preparation
For the NN retrievals of IWV, specific humidity, and temperature profiles during MOSAiC, a training dataset is needed that covers the variability of the environmental conditions in the central Arctic over an annual cycle. We selected ERA5 data for 2001–2018 with 6-hourly temporal resolution at 12 grid points, 9 of which are located in the central Arctic and 3 in the Fram Strait (see Fig. 2). The grid points in the Fram Strait cover more humid conditions, as this is a typical pathway for warm and moist air intrusions (Mewes and Jacobi, 2019).
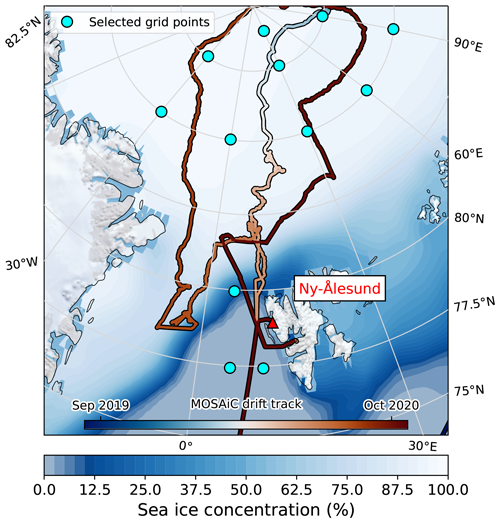
Figure 2Mean sea ice concentration in the Arctic over the years 2001–2018 based on daily ERA5 data at 12:00 UTC. Light blue circles mark the position of the 12 grid points selected for the retrieval development. The MOSAiC drift track is marked as a coloured line with a black outline.
Simulated HATPRO and MiRAC-P observations are needed in conjunction with the ERA5 data to train the NN. Meteorological data (temperature, relative humidity, geopotential height, pressure, 10 m wind) and vertical hydrometeor distributions from ERA5 (specific cloud liquid, ice, rain and snow content) have been used as input to simulate TBs with PAMTRA. The ERA5 skin temperature was used for the sea ice and sea surface temperatures. The TBs were simulated with PAMTRA's default gaseous absorption, hydrometeor absorption, and scattering models as described in Mech et al. (2020).
A total of 4 years of simulated TBs and ERA5 data (2001, 2006, 2011, and 2015) were held back from the retrieval development for the final evaluation (ERA5 evaluation dataset). With the remaining 14 years of data, we trained the NN and validated its performance (11 and 3 years for the training and validation datasets, respectively). The number of training (validation) samples is roughly 192 000 (52 000). To avoid training near-surface temperature and humidity biases from ERA5 into the retrieval, a small subset of about 5 % of level 2 MOSAiC radiosondes (Maturilli et al., 2021) was also included in the validation process. For the retrieval development and evaluation, atmospheric profiles have been interpolated onto the same height grid used in the standard HATPRO retrieval (Löhnert, 2023; Marke et al., 2024) and in Walbröl et al. (2022c), ranging from 0 to 10 000 m with the vertical spacing increasing from 50 m at the surface to 500 m at the top. The height grid was limited to 8000 m for temperature profiles to avoid the tropopause. Additionally, to imitate measurement uncertainties, random Gaussian noise with a mean of 0 and standard deviations of 0.5, 0.75, and 2.5 K has been added to the simulated TBs at K–V, G, and 243–340 GHz, respectively. We intentionally used a higher noise level for the higher frequencies to account for the higher PAMTRA simulation uncertainties due to scattering from hydrometeors and water vapour continuum absorption.
3.2 Retrieval setup
This study used multilayer perceptron NNs (fully connected layers) to retrieve IWV, specific humidity, and temperature profiles. To optimally use HATPRO's boundary layer observations, we retrieved temperature profiles from zenith and boundary layer observations separately. The challenge is to develop retrievals that are not overfitted and can therefore adapt well to new data. Overfitting occurs when the retrieval learns not only the relation between the observations and the atmospheric state but also the (synthetic) noise. Additionally, we wanted to ensure that the retrievals are robust by training an ensemble of 20 NNs with identical settings but with different random number seeds. The random number seeds affect the selection of years for the training and validation data, as well as the NN initialization (weight coefficients). The NNs are considered robust when the errors in the validation data show a small spread over the ensemble of 20 NNs. For example, the spread should be smaller than a given threshold (e.g. 0.2 kg m−2 for IWV) or smaller than the magnitude of the error.
To meet the retrieval performance requirements, we developed four NNs with different settings (see Appendix A), one for each retrieved quantity (IWV, specific humidity, and temperature profiles from zenith and boundary layer observations). The retrievals of profiles required deeper networks and stronger regularization measures (e.g. dropout layers, batch normalization; see Appendix A) to avoid overfitting and to achieve a good performance. Besides TBs at different frequencies, we also included seasonal information in the form of the cosine and sine of the day of the year as input to all NNs except for the boundary layer temperature profile (inspired by Billault-Roux and Berne, 2021). Additionally, adding the 2 m temperature and the retrieved IWV as input to the specific humidity profile retrieval slightly reduced errors during validation. Therefore, the specific humidity retrieval can only be performed after the IWV retrieval. For the boundary layer temperature profile, the input vector consists of V-band TBs at various elevation angles (90.0, 30.0, 19.2, 14.4, 11.4, 8.4, 6.6, and 5.4°), which are measured during HATPRO's boundary layer scan. TBs at other frequencies were not included because they were not measured at these elevation angles. Also, adding other parameters to the input vector did not improve errors. Therefore, the input vector is identical to the one used in the HATPRO regression retrieval described in Walbröl et al. (2022c). Further details of the NN retrieval principles and settings can be found in Appendix A.
3.3 Metrics for retrieval evaluation and vertical information content
The retrieved state vector x (e.g. specific humidity profile) is evaluated using the reference provided by ERA5 (ERA5 evaluation dataset) or MOSAiC radiosondes (MOSAiC evaluation dataset). For each component j of the state vector (i.e. jth height level), we calculate the bias, the root mean squared error (RMSE), and the bias-corrected RMSE.
Ns is the number of data samples of the respective evaluation dataset. For IWV, we also compute the Pearson product–moment correlation coefficient:
where () is the mean retrieved (reference) state vector.
The vertical information content of passive microwave observations was computed following the ideas of the physical retrievals of Rodgers (2008). Due to computation time, the information content was only computed for a randomly selected subset of 4 % of the ERA5 evaluation dataset (2803 samples). Firstly, we interpolated the vertical grid from the ERA5 model levels to the retrieval height grid and simulated new reference observation vectors y (here, TBs) with PAMTRA. For these simulations, the retrieval grid has been extended to 45 000 m to simulate emissions from gases (mainly oxygen) beyond the retrieval height grid. Secondly, each state vector component is perturbed step by step. We multiply the respective height level by 1.01 for specific humidity profiles, similar to Ebell et al. (2013). Thirdly, we simulate new TBs with PAMTRA for each perturbed state vector. Fourthly, the Jacobian matrix K is calculated with entries , where ∂yia is the ath component of the difference between the perturbation-based and reference observation vector of the ith data sample. ∂xij is the jth component (jth height level) of the difference between the perturbed and reference state vector. Fifthly, the averaging kernel matrix A is computed with , where Sa and Sε are the covariance matrices of the state and observation vectors, respectively. Sε contains the TB noise on the main diagonal, while the remaining entries are 0. Sa is calculated as a full covariance matrix from the ERA5 evaluation dataset. Finally, the degrees of freedom (DOF) are inferred from the trace of the averaging kernel A.
We applied the retrievals to both the ERA5 evaluation dataset and MOSAiC observations (MOSAiC evaluation dataset), for which the radiosondes serve as the reference dataset. The retrieval evaluation with respect to the ERA5 data allows us to assess the retrievals' theoretical best performance because it is an idealized world without measurement problems. Here, we compute errors for all 20 NNs to get an idea of the spread among the NNs. For the evaluation with the MOSAiC radiosondes, we selected the NN that has a low RMSE and bias in the validation dataset while also having the lowest RMSE in the 5 % MOSAiC radiosonde subset that we included in the validation process. Hereafter, this NN is referred to as the final NN.
4.1 IWV
The performance of the IWV retrieval applied to the ERA5 and MOSAiC evaluation datasets can be seen in Fig. 3. For the ERA5 data, we can evaluate the robustness of the NN through the spread of the errors among all 20 NNs. The RMSE of IWV varies little over the 20 NNs for IWV up to 24 kg m−2, indicated by the low spread (<0.3 kg m−2). Only for higher IWV does the spread increase significantly to 0.8 kg m−2. However, only 41 of 70 080 (< 0.1 %) of the synthetic dataset samples have an IWV above 24 kg m−2. Therefore, errors are computed over a very low fraction of the data and tend to vary more for different NNs. Most importantly, statistical retrievals such as NNs struggle to capture extreme conditions not well represented in the training dataset. This can also be seen in the bias, which is close to zero for IWV below 20 kg m−2, as expected for a well-trained NN, but deviates from zero for higher IWV. However, biases are still small for both the ERA5 and MOSAiC evaluation datasets, staying below 2 %.
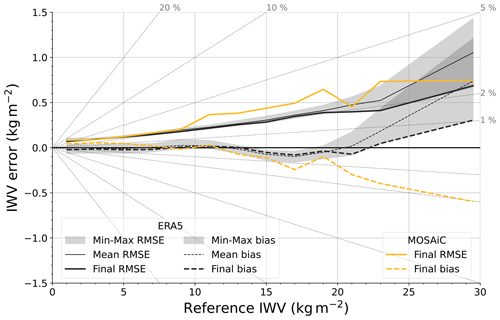
Figure 3IWV errors (RMSE and bias) for certain bins of reference IWV (0–2, 2–4, …, 22–24, 24–35 kg m−2). IWV errors based on the ERA5 (MOSAiC) evaluation dataset are displayed in black (yellow). The maximum and minimum spread of RMSE and bias over the 20 neural networks are indicated by grey shading. The RMSE (bias) of the mean over the 20 neural networks is displayed as a thin solid (dashed) black line. The RMSE (bias) of the final NN is shown as a thick solid (dashed) black line.
The RMSE of the final NN, which was selected based on errors in the validation dataset, is about 2 % of the IWV and therefore also at the lower end of the 20-NN ensemble for the ERA5 evaluation dataset. This shows that the retrieval is well trained because it performs similarly well on the evaluation dataset as on the validation dataset. For the comparison with MOSAiC observations, where we also use the final NN, the RMSE is slightly higher in most IWV regimes, reaching up to 3 %–4 %. In absolute terms, the RMSE increases from 0.1 to 0.7 kg m−2, with IWV increasing from 1 to 29 kg m−2. Here, the additional uncertainties in the radiosonde measurements and matching the MWR data must be considered.
4.2 Specific humidity profiles
We evaluate the retrieved specific humidity profiles (q) in terms of bias and RMSEcorr for the ERA5 and MOSAiC evaluation datasets (Fig. 4). The RMSE values are similar to RMSEcorr because of a small bias. For the MOSAiC data, the RMSEcorr increases from 0.25 g kg−1 at the surface to 0.5 g kg−1 at 1500 m, which is 15 % to 30 % of the mean specific humidity (Fig. 4b). At higher altitudes, the RMSEcorr is lower but the relative error increases because the mean specific humidity also decreases. While the RMSEcorr values are generally smaller for the ERA5 data, the shape is similar, with the highest RMSEcorr of about 0.25 g kg−1 (15 % of the mean q) at 1000 m and even lower values at the surface of 0.15 g kg−1 (8 %). The RMSEcorr spread across all 20 NNs is negligible, mostly ranging from 0.01 to 0.02 g kg−1.
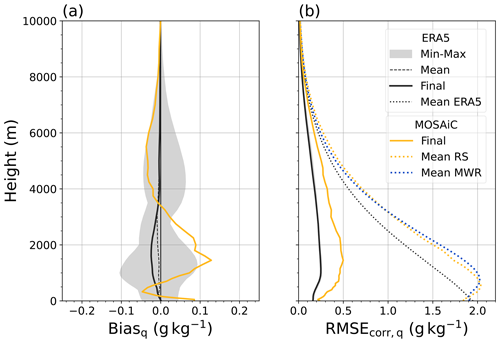
Figure 4Specific humidity q error profiles showing (a) the bias and (b) the bias-corrected RMSE with respect to the reference from the ERA5 and MOSAiC evaluation datasets. The dashed black line in each panel shows the mean over the 20 neural networks, while shading indicates the min–max spread. The prediction of the final neural network is indicated by the thick black (yellow) lines for the ERA5 (MOSAiC) evaluation dataset. The mean MOSAiC radiosonde (RS) profile and ERA5 profile are shown as dotted yellow and black lines, respectively, and serve as a reference for the absolute error values. The mean retrieved profile from MOSAiC microwave radiometer (MWR) observations is also included as a dotted blue line.
The mean MOSAiC radiosonde q profile shows the maximum value about 250 m lower than the mean retrieved q profile (Fig. 4). Because of the different heights of the humidity inversion, we find the highest RMSEcorr and bias slightly above the height level of the maximum q value (at 1500 m). At this height, the retrieved q profile overestimates the radiosonde measurement by up to 0.15 g kg−1 (see bias in Fig. 4a). Above 3500 m, the bias remains negative, with values up to −0.04 g kg−1 at 5500 m. For the ERA5 evaluation dataset, the final NN, which was also used to derive the q profile for MOSAiC, shows much smaller biases and is slightly negative for all heights (only up to −0.025 g kg−1). However, in the lowest 2000 m, the bias varies much more than the RMSEcorr, ranging from −0.1 to +0.1 g kg−1 depending on the chosen NN.
The smaller magnitude of the error profiles in the ERA5 evaluation dataset is likely due to the lower complexity of q profiles in ERA5 compared to radiosonde observations. Specific humidity profiles in reanalyses are typically much smoother and do not resolve small inversions (Chellini and Ebell, 2022). Passive microwave observations cannot resolve small inversions and average out strong vertical gradients. Therefore, errors of retrieved profiles are large when compared to radiosonde data in the presence of strong vertical gradients (i.e. humidity inversions), while the smoother profiles of reanalyses can be captured better. As the retrieval has been trained with reanalysis data, it is also expected to perform best when applied to the same reanalysis. Furthermore, the errors of the evaluation based on real observations can be higher due to measurement errors of radiosondes (noise, sonde drift, systematic errors due to sensor response time, etc.) and of the MWRs (noise, systematic errors).
4.3 Temperature profiles
For the evaluation of the retrieved temperature profiles, we also analyse the bias and RMSEcorr (Fig. 5) but distinguish between profiles retrieved from zenith observations (henceforth, zenith temperature profiles) and boundary layer scans (henceforth, BL temperature profiles). As for specific humidity, the spread over the 20 NNs is larger for the bias than for RMSEcorr but generally quite small (especially for BL temperature profiles).
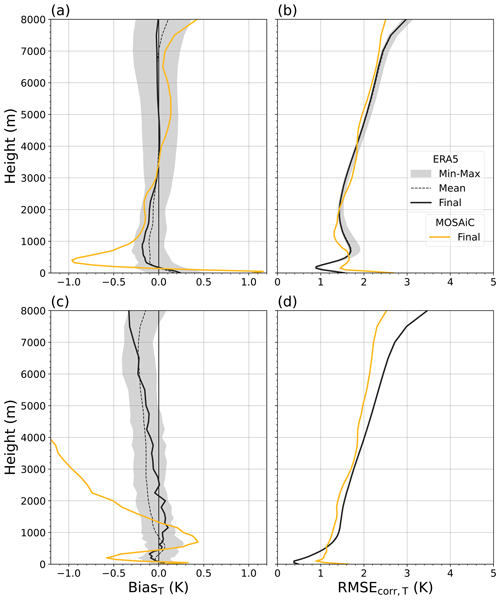
Figure 5Error profiles of (a, b) zenith and (b, d) boundary layer temperature T profiles. Panels (a) and (c) show the bias and panels (b) and (d) the bias-corrected RMSE with respect to the reference from the ERA5 and MOSAiC evaluation datasets. Shading and different line types are similar to Fig. 4.
Firstly, we evaluate the zenith temperature profiles: the biases and RMSEcorr of zenith temperature profiles are larger for MOSAiC compared to the ERA5 evaluation dataset below 1500 m but mostly similar at higher altitudes (see Fig. 5a and b). Within the lowest 150 m, the MOSAiC RMSEcorr decreases rapidly from 2.9 to 1.4 K. This large RMSEcorr is associated with near-surface temperature inversions that typically occur in the Arctic. In the ERA5 evaluation dataset, this steep error gradient is less pronounced because near-surface temperature inversions over sea ice are not well represented in ERA5. Between about 200 and 2000 m, the RMSEcorr is between 1.2 and 1.6 K for MOSAiC and between 0.8 and 1.6 K for the ERA5 evaluation dataset. At the top of the retrieval grid at 8000 m, the RMSEcorr increases to 2.5 K for MOSAiC and 3 K for ERA5.
In the lowest 500 m, the bias of the zenith temperature profiles lies between −1 and +1 K for MOSAiC and between −0.2 and +0.2 K for the ERA5 evaluation dataset (final NN; see Fig. 5a). Here, the strong surface temperature inversions, which are not well resolved by the retrieved profile, are also responsible for the large bias. Above 1500 m, the bias in both datasets is generally smaller than ±0.2 K. However, the MOSAiC observation bias varies over the seasons: in winter (22 October 2019–30 April 2020), the bias is mostly negative in the mid-troposphere, ranging from −0.4 to −0.8 K, while it is positive in summer (1 May–1 October 2020), ranging from +0.5 to +0.9 K (not shown).
As expected, biases and RMSEcorr are smaller for the BL temperature profiles in the lowest 1500 m compared to the zenith temperature profiles (see Fig. 5c and d). This result is consistent with the findings of Crewell and Löhnert (2007). For the MOSAiC data, the RMSEcorr is 2 K at the surface (0.9 K at 100 m) and smaller than 1.2 K up to 1 km height. The error is therefore 1 K (0.5–0.6 K) lower compared to the zenith temperature profile error. Based on the ERA5 evaluation dataset, the near-surface RMSEcorr values are only 0.4–0.5 K, which is lower than for the MOSAiC data because of the less complex temperature profile and the absence of measurement uncertainties. In the lowest 1500 m, the bias is also reduced, being nearly 0 K in the ERA5 evaluation dataset (with the final NN) and between −0.6 and +0.4 K in the MOSAiC data. Also, the seasonal variation of the MOSAiC BL temperature profile bias is smaller than that of the zenith temperature profiles. Above 2000 m, the RMSEcorr is similar for both the zenith and BL temperature profiles, but the bias above 2000 m is stronger (more negative) in BL temperature profiles, especially for the MOSAiC data (up to −2 K).
We conclude that if the 30 min temporal resolution is sufficient for the user, a combination of BL profiles and zenith profiles provides optimal performance. We recommend that BL temperature profiles be used in the lowest 1500 m, followed by a linear transition to the zenith temperature profile between 1500 and 2000 m, and only the zenith temperature profile above 2000 m.
After introducing the combined HATPRO and MiRAC-P retrieval, it still has to be demonstrated that the synergy is beneficial compared to single-instrument retrievals. The benefit is quantified through error reduction and gain in vertical information content. We compare the errors of the synergy with the single-instrument retrievals by Walbröl et al. (2022c) for MOSAiC observations to present the improvements for actual observations. As the retrieval methods also differ, we also analysed the influence of different retrieval architectures (i.e. NN instead of regression) and training datasets (ERA5 instead of Ny-Ålesund radiosondes) on the error reduction compared to HATPRO-only retrievals. This helps to isolate the pure benefit of the combination of low- and high-frequency microwave observations from potential effects due to different retrieval methods. In Sect. 5.1 and 5.2, the error estimates for the synergy correspond to the ones shown with respect to MOSAiC radiosondes in Sect. 4.1 and 4.2.
5.1 IWV
Figure 6 shows the RMSE and bias of IWV obtained from single-instrument observations (HATPRO-only, MiRAC-P-only) and from the synergy of both instruments, with radiosonde IWV as a reference. As found in Walbröl et al. (2022c), the HATPRO-only IWV retrieval shows high relative errors and a positive bias (> 20 %) for IWV below 5 kg m−2, while having lower relative errors (2 %–4 %) for IWV greater than 10 kg m−2. For MiRAC-P, the error behaviour is reversed: small biases and RMSE are found for extremely dry conditions and errors become much larger than the HATPRO-only retrieval for IWV greater than 10 kg m−2.
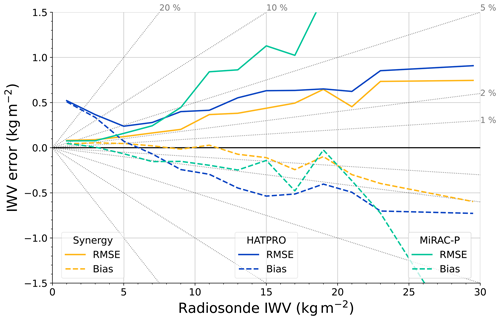
Figure 6RMSE (solid lines) and bias (dashed lines) of IWV retrieved from MOSAiC MWR observations for certain bins of radiosonde IWV (0–2, 2–4, …, 22–24, 24–35 kg m−2). Yellow lines indicate retrieved IWV from the synergy of HATPRO and MiRAC-P, dark blue lines show HATPRO-only retrievals, and cyan lines show MiRAC-P-only retrievals.
As expected, the synergy performs similarly well or even better than the single-instrument retrievals. For IWV below 5 kg m−2, the RMSE of the synergy is reduced by 75 % compared to HATPRO, while being similar to MiRAC-P. The RMSE of the synergy is also smaller by up to 0.2 kg m−2 compared to HATPRO only when IWV is above 5 kg m−2, corresponding to an RMSE reduction of 15 %–50 %. However, the improvement of RMSE for high IWV is mainly due to the bias reduction from more than −0.5 for HATPRO to −0.1 to −0.5 kg m−2 for the synergy. When considering the bias-corrected error (RMSEcorr), the synergy shows up to 20 % higher errors than the HATPRO regression retrieval for IWV above 10 kg m−2 (not shown). The error reduction compared to MiRAC-P is even higher in this IWV range.
To study the influence of the different retrieval methods and training datasets, we trained one NN with identical settings as used in the final synergy (see Appendix A, Table A1) but included only K-band TBs as the input vector. Therefore, the only differences between this NN and the HATPRO regression are the training data (ERA5 vs. Ny-Ålesund radiosondes) and the retrieval type (regression vs. NN). With this NN, we find that RMSE and biases of the retrieved IWV are similar to those of the HATPRO regression retrieval in almost the entire IWV range (see Appendix B, Fig. B1). Only in very dry conditions (IWV below 2 kg m−2) does the K-band-only NN show 0.1 kg m−2 smaller bias and RMSE. Thus, including the higher frequencies by MiRAC-P dominates the improvement of the error.
5.2 Specific humidity profiles
In Fig. 7, the bias and RMSEcorr for the specific humidity profiles of the HATPRO regression retrieval and the synergy NN retrieval are shown with respect to MOSAiC radiosondes. At altitudes below 1500 m altitude, the RMSEcorr is much smaller for the synergy compared to HATPRO. At the surface, the reduction of RMSEcorr is most prominent, decreasing from 0.5 g kg−1 to less than 0.25 g kg−1 in absolute terms and from 30 % to less than 15 % in relative terms (Fig. 7b). Above 1500 m, the RMSEcorr difference between HATPRO and the synergy is marginal and the relative RMSEcorr gradually increases from 25 % to 80 % until the top of the retrieval grid (10 000 m). Between the surface and 1000 m, the synergy also shows a much smaller bias (−0.05 to +0.1 g kg−1) than HATPRO (0.1 to 0.4 g kg−1). The strongest improvement was found near the surface, where the bias is reduced by up to 75 %. Above 1000 m, the bias reduction of the synergy compared to HATPRO is less pronounced: the bias of HATPRO (the synergy) lies between −0.1 and +0.1 g kg−1 (−0.05 and +0.15 g kg−1). Therefore, combining the two instruments is most beneficial in altitudes below 1500 m in the real-world application.
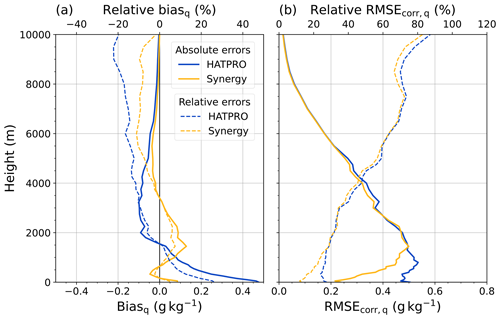
Figure 7Specific humidity q error profiles showing (a) the bias and (b) the bias-corrected RMSE in absolute (solid lines) and relative terms (dashed lines) with respect to MOSAiC radiosondes. Specific humidity errors of the synergy (HATPRO) retrieval are shown in yellow (blue).
Because of the different magnitude of specific humidity and the different performances of HATPRO and MiRAC-P over the seasons, we also investigated seasonal differences in error reduction (not shown): in winter (here, 22 October 2019–30 April 2020), the RMSEcorr is lower for both HATPRO and the synergy as the water vapour amount is also lower. However, the relative RMSEcorr of the synergy is similar to the error for the full MOSAiC year in the lowest 1000 m, while the relative error of the HATPRO retrieval is increased. Therefore, the benefit of the synergy in the lower troposphere is even more pronounced. The synergy also shows smaller errors than HATPRO in the middle and upper troposphere, which was not found for the entire MOSAiC year. The bias reduction of the synergy compared to HATPRO-only is also stronger in winter. In summer (here, 1 May–1 October 2020), the overall picture of the error profiles is similar to the full MOSAiC year, except that the RMSEcorr values (relative RMSEcorr) for both retrievals are shifted to slightly higher (lower) values. The bias reduction of the synergy compared to HATPRO is also a little less pronounced.
As in Sect. 5.1, to identify whether the error reduction is mainly due to the inclusion of the higher frequencies or due to the different training data and retrieval method, we trained one NN with the same setup as the final synergistic retrieval but used only K-band TBs as input. We applied this K-band-only NN retrieval and the HATPRO regression to the ERA5 and MOSAiC evaluation datasets as in Sect. 4.2 and found that the RMSEcorr was almost identical for both retrievals at all height levels (see Appendix B, Fig. B2b). Only the bias is closer to 0 for the K-band-only NN than for the regression (Fig. B2a). As the results for both retrieval architectures are mostly similar when using the same input vector (K-band TBs), it follows that the inclusion of the higher frequencies contributes most to the overall error reduction.
We also investigated the influence of the additional input parameters (2 m temperature, IWV, day of the year) on the retrieved specific humidity profile. In one experiment, we excluded the MiRAC-P TBs from the input vector of the NN but kept the HATPRO TBs, as well as the day of the year, the IWV, and the 2 m temperature. The resulting retrieved specific humidity also shows lower errors than the HATPRO-only regression at the surface (not shown). However, the vertical extent of the benefit is smaller, being mainly confined to the lowest 500 m, compared to the synergistic retrieval including the MiRAC-P TBs. Another experiment, where we used HATPRO and MiRAC-P TBs, as well as the IWV and day of the year, as input but excluded the 2 m temperature showed higher errors in the lowest 100 m. These experiments demonstrate that the MiRAC-P observations are needed to have a higher vertical extent of the error reduction and that the 2 m temperature effectively reduces errors at the surface.
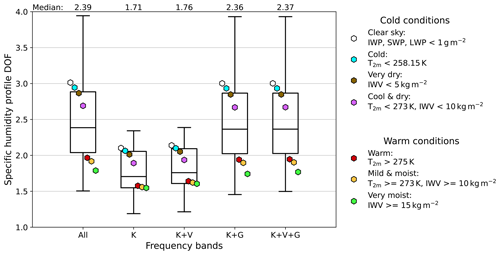
Figure 8Distribution of the degrees of freedom (DOF) over 2803 samples visualized as a box plot for different frequency combinations (all frequencies; K- and V-band; K- and G-band; K-, V-, and G-band). The box indicates the interquartile range (IQR; 1st–3rd quartile) of the distribution, and the horizontal line within the box shows the median. The whiskers extend from below the 1st quartile and above the 3rd quartile by 1.5×IQR, respectively. Additionally, the median DOF of different atmospheric conditions has been highlighted.
To quantify the synergy benefit, it is interesting to analyse not only the error of the retrieved profiles but also their vertical information content. This also offers the opportunity to investigate the impact of the different frequency bands. Thus, we computed the degrees of freedom (DOF) as a measure of the vertical information content for various frequency combinations as described in Sect. 3.3. In Fig. 8, the statistics of the DOF over a 4 % subset of the ERA5 evaluation dataset are visualized. When using only K-band frequencies, the specific humidity profile has about 1.7 DOF. Adding the V-band TBs only has a small effect as these frequencies are hardly sensitive to the water vapour amount. The largest increase in the DOF (from 1.7 to 2.4) is caused by the addition of G-band frequencies to the K-band frequencies. This increase is even more pronounced in cold, dry, and clear-sky conditions, where the DOF is increased from 1.9–2.1 to 2.7–3.0 (Fig. 8). In contrast, the DOF hardly improved from 1.6 to 1.8–2.0 in warm and humid conditions. Clear-sky scenes are typically associated with cold and dry conditions during the Arctic winter. The DOF is larger during cold and dry conditions than during warm and humid conditions because the G-band TBs are partly saturated. This means they no longer observe the entire tropospheric column and cannot add as much information. Adding the V-band or the 243 and 340 GHz frequencies to K- and G-band TBs only has a minor impact on the DOF distribution.
Ebell et al. (2013) and Löhnert et al. (2009) analysed the vertical information content of absolute humidity profiles from ground-based MWRs using K-band TBs at different mid-latitude sites and found 2.4 and 1.6 DOF, respectively. Additionally, Löhnert et al. (2009) obtained 2.7 DOF for a tropical site with a much higher mean IWV. Thus, the DOF depends strongly on the frequencies used to derive the humidity profile and the atmospheric conditions. In the Arctic, humidity profiling is more challenging with K-band frequencies due to the lower sensitivity, which is why the higher-frequency observations are needed to obtain similar DOF (see also Fig. 1).
Based on the averaging kernel and the vertical height grid spacing, we can also estimate the theoretical vertical resolution of the specific humidity profiles (e.g. , where dz is the height grid spacing and Ajj the diagonal entries of the averaging kernel at height level j). In Fig. 9, the estimated vertical resolution (effective resolution) is shown for the K-band only and for all frequencies. The other frequency combinations are not discussed as their averaging kernel values lie between those of the K-band and all frequencies. The effective resolution at a certain height level indicates to what vertical resolution the specific humidity profile is smoothed by the microwave observations. Generally, larger values of the effective resolution are found at higher altitudes, consistent with the decreased sensitivity of ground-based microwave observations at these altitudes. The jump of the effective resolution at 5000 m height is due to a strong change in height grid spacing. At the surface, using all frequencies instead of just the K-band improves the effective resolution by a factor of 2 (from 1200 m for K-band to 600 m for all frequencies). At higher altitudes, the relative improvement is smaller, but the absolute resolution improvement is still mostly between 1000 and 2000 m.
5.3 Relative humidity profiles
Relative humidity is an important parameter, particularly for cloud processes, and a desired variable for the modelling community. We computed relative humidity from the retrieved temperature, specific humidity profiles, and surface air pressure measured by the weather station attached to HATPRO using the hypsometric equation. For HATPRO, the conversion from absolute humidity to relative humidity profiles was straightforward. Due to the bias reduction that we achieved with the new NN retrievals in the retrieved temperature and specific humidity profiles, we also expect to see lower biases in relative humidity. In the following, we compare the relative humidity bias and RMSEcorr of HATPRO and the synergy with respect to the MOSAiC radiosondes, which are shown in Fig. 10.
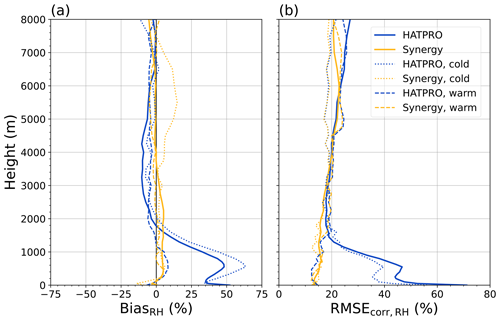
Figure 10Relative humidity error profiles showing (a) the bias and (b) the bias-corrected RMSE with respect to MOSAiC radiosondes. Relative humidity errors of the synergy (HATPRO) retrieval are shown in yellow (blue). Errors are also displayed for different atmospheric conditions: cold and clear-sky conditions (integrated water vapour (IWV) < 10 kg m−2, 2 m temperature (T2 m) < 273.15 K) as dotted lines and warm conditions (IWV ≥ 10 kg m−2, T2 m ≥ 273.15 K) as dashed lines.
The bias of the synergy (5 %) is much smaller compared to HATPRO (40 %) in the lowest 1000 m (Fig. 10a). Similarly strong improvements can be found in the lowest 1000 m of the RMSEcorr profile (Fig. 10b), where errors are reduced from more than 60 % to 15 % at the surface and from 35 %–45 % to 15 % at higher altitudes. Above 2000 m, the RMSEcorr of HATPRO and the synergy are similar (about 20 %), but the bias is closer to 0 %, while HATPRO shows a negative bias up to −10 %.
In cold and clear-sky conditions, where IWV and 2 m temperatures were below 10 kg m−2 and 273.15 K, respectively, and no clouds were detected by Cloudnet as described in Sect. 2.2.2, the bias reduction is even stronger below 1500 m (Fig. 10a). In warm conditions (IWV ≥ 10 kg m−2, 2 m temperature ≥ 273.15 K), both retrievals perform similarly well, suggesting no benefit of the synergy compared to the HATPRO-only retrieval. If low-level stratus clouds were not respected in the clear-sky detection, the RMSEcorr values of the HATPRO retrieval would be up to 10 percentage points higher in the lowest 1000 m, while the errors of the synergy would only slightly increase (not shown). In general, the relative humidity errors of the synergy are much less sensitive over these two types of atmospheric conditions (or over the seasons; not shown).
In this study, we demonstrate the benefit of combining low- (22–58 GHz, HATPRO) and high-frequency (175–340 GHz, MiRAC-P) microwave radiometer (MWR) observations for humidity profiling and integrated water vapour (IWV) estimates in Arctic conditions. The newly developed neural network (NN) retrievals for IWV and for specific humidity and temperature profiles have been applied to synthetic measurements based on ERA5 and real observations from the MOSAiC expedition. Subsequently, they have been evaluated with ERA5 data and MOSAiC radiosondes, respectively, and compared to the retrievals by Walbröl et al. (2022c). Retrieved temperature and specific humidity profiles were used to compute relative humidity together with the surface air pressure from the weather station attached to HATPRO.
We illustrate the sensitivity of the NN to random perturbations with an ensemble of 20 NNs. The spread of errors over the 20 NNs is generally small, except for specific humidity biases. We selected one NN, whose errors were on the lower end of the spread during the retrieval development, as the final NN. Also in the final evaluation, the final NN showed one of the smallest errors of all 20 NNs. In the following paragraphs, we only summarize retrieval errors with respect to MOSAiC radiosondes as these errors are typically larger than the theoretical ones based on the ERA5 evaluation dataset: for IWV, the RMSE is about 3 %–4 % and biases are smaller than 2 % over a wide range of IWV conditions. Specific humidity is overestimated by up to +0.15 g kg−1 at 1500 m relative to radiosondes. At other height levels, the biases are smaller. The bias-corrected RMSE (RMSEcorr) is also highest at 1500 m with 0.5 g kg−1 (about 30 %). Temperature profile RMSEcorr values (biases) from zenith MWR observations lie between 1.4 and 2.9 K (−1 and +1 K) in the lowest 1500 m. Temperature profiles retrieved from boundary layer MWR observations showed much smaller errors in that height range, which is consistent with the findings of Crewell and Löhnert (2007).
In the next step, we compared the errors of the new synergistic NN retrievals to the single MWR retrievals of Walbröl et al. (2022c) to estimate the information benefit. Additionally, we computed the vertical information content of specific humidity profiles as degrees of freedom (DOF). The information benefit is only shown for MOSAiC observations to obtain the benefit for the real measurements. IWV errors of the synergy are generally smaller than or similar to those of the single MWR retrievals. In cases when IWV is greater than 10 kg m−2, the RMSE of the synergy is at least 15 % smaller than the HATPRO-only retrieval, which is mainly due to the lower biases of the synergy.
For specific humidity profiles, the largest information benefit was found. The combination of HATPRO and MiRAC-P increased the DOF from 1.7 to 2.4 and reduced the RMSEcorr by up to 50 %. Through the synergy, strong positive biases below 1000 m could also be reduced by up to 75 %. The benefit is most distinct in the lowest 1500 m because this is where the error reduction is the strongest. At these heights, the synergy enhanced the effective vertical resolution of the specific humidity profile by a factor of up to 2 compared to the HATPRO-only retrieval (from 1200 to 600 m). In cold and dry conditions, the DOF increase and the error reduction were even more pronounced.
We also analysed the influence of additional NN input parameters (2 m temperature, day of the year, and IWV) on the specific humidity profile errors and found that including the 2 m temperature is important to minimize errors at the surface. Because of the improvements in specific humidity (and temperature) profiles, the synergy also results in lower relative humidity errors compared to the HATPRO-only retrieval, which is particularly evident in the lowest 1500 m. Additionally, the errors of the relative humidity profiles from the synergy vary much less over different atmospheric conditions than those from the HATPRO-only retrieval.
Coming back to the research questions listed in Sect. 1, we can conclude the following.
-
For specific humidity profiles, the bias-corrected RMSE could be reduced by up to 50 %. Bias reductions are partly even higher. The information benefit is mainly attributed to the combination of HATPRO and MiRAC-P. The different retrieval training data and methods only had a small influence.
-
The vertical information content in the specific humidity profile was increased by 40 %.
-
The combination of HATPRO and MiRAC-P frequencies increased the vertical information content the most during cold and dry conditions and the least during moist and warm conditions.
HATPROs are used at different sites worldwide (polar, mid-latitude, and subtropical regions). In dry regions (high-altitude or polar sites), the observation network would clearly benefit from an instrument that includes the G-band frequencies for IWV and humidity profiling (relative and specific humidity) as these frequencies increased the DOF the most. It is planned to install MiRAC-P at Ny-Ålesund again in 2025 to enhance the continuous atmospheric observations at the German–French research station AWIPEV. We are confident that adding MiRAC-P to the already installed HATPRO will improve humidity profiling similarly as demonstrated for the MOSAiC expedition. The low specific humidity profile errors give us confidence that the synergy is suitable for gaining insights into the general structure of Arctic humidity profiles (i.e. inversions). However, a detailed analysis of the ability of the synergy to identify humidity inversions is still missing.
In the next step, the enhanced water vapour products from the synergy of HATPRO and MiRAC-P, as well as the radiosonde measurements from MOSAiC, will be used to quantify IWV and specific humidity errors of satellite products and reanalyses. As reanalyses assimilated the MOSAiC radiosonde observations, this comparison likely does not reflect the true performance of the reanalyses in the central Arctic. With the considerable specific humidity profile improvements of the synergy compared to HATPRO, the question arises of how well humidity inversions, which are important for cloud formation and maintenance, are captured. This question will be answered with a statistical analysis for the entire MOSAiC period. We will then evaluate the representation of humidity inversions in satellite products and reanalyses compared to observations from the MWRs and radiosondes. Radiative transfer simulations allow us to assess how biases in humidity inversion characteristics affect the downwelling thermal infrared radiation.
As noted in Sect. 3.2, all NNs in this paper are multilayer perceptrons (fully connected layers), but some include dropout layers and batch normalization (see Table A1), and have been created with Python's Keras module (contained in Tensorflow; Abadi et al., 2015). The forward propagation of a simple, fully connected NN starts with an input layer whose number of nodes equals the number of components of the input vector. The mathematical operations to propagate to the next layer of the network are similar to multiple linear regression: each node is multiplied by a randomly initialized weight before being summed up and a bias coefficient is added. Afterwards, the result is used as input to a so-called activation function (e.g. exponential or rectified linear unit, also known as ReLU). The output of the activation function is then forwarded to each node of the next layer where the process is repeated until the output layer is reached. We always use a linear activation function between the last hidden layer and the output layer. The output layer represents the prediction of the NN and is compared to the truth of the training and validation datasets using a certain loss function (here, mean squared error).
To minimize the loss function, an optimization algorithm (e.g. gradient descent) adapts the weights of each node in a backpropagation process. In this study, we used the Adam optimization algorithm (Kingma and Ba, 2017). The learning rate can be adjusted to reduce or enhance the magnitude of the gradient during backpropagation, leading to slower and smoother or faster and more erratic learning. The NN typically processes a specific number of training data samples, determined by the chosen batch size, before updating the weights. The epoch number determines the maximum number of times the training dataset is cycled through. In our retrievals, we activated the EarlyStopping function implemented in Keras that monitors the loss of the validation dataset over the epoch numbers. The training was terminated if the validation loss did not improve by more than the minimum delta value for a certain number of epochs (callback patience).
Dropout and batch normalization layers are tools to regularize the NN to make it less prone to overfitting. If batch normalization is set to true for a retrieval (see Table A1), we include a batch normalization layer after each hidden layer. It normalizes the output of the preceding hidden layer so that its mean (standard deviation) is close to 0 (1). The dropout chance noted in Table A1 indicates the chance that the value of a node is set to 0 during training. If the dropout chance is >0.0, we add a dropout layer after each hidden layer or, if applicable, after each batch normalization layer.
Figure B1 shows the IWV error with respect to MOSAiC radiosondes for the old single-instrument retrievals (HATPRO regression, MiRAC-P-only NN) and the new NN retrieval. However, in this case, the input vector of the NN consists of K-band TBs only. This demonstrates that the different retrieval method and training data compared to the HATPRO regression are not responsible for the error reduction in dry conditions seen in Fig. 6 and discussed in Sect. 5.1.
Similarly, the specific humidity error profiles for the HATPRO regression and the NN using only K-band TBs are shown in Fig. B2. The RMSEcorr of both retrievals is comparable for all height levels, but the lower-tropospheric bias of the NN, labelled as synergy, is smaller. Therefore, the strong RMSEcorr reduction is solely caused by including the higher frequencies in the retrieval. However, the different method and training dataset seem to contribute a little to the bias reduction.
For a fair comparison of the retrieved and radiosonde specific humidity profiles, the latter can be smoothed to the retrieval height resolution when the averaging kernel (AK) is available. Following Löhnert and Maier (2012), we compute the smoothed specific humidity profiles as
where qret and qrs are the retrieved and radiosonde specific humidity profiles, respectively, and A is the AK. In Fig. C1, the specific humidity errors with respect to the smoothed radiosonde profiles are shown. The displayed errors are therefore resolution-corrected. For HATPRO, the smoothing-based errors are much smaller compared to the true errors shown in Fig. 7 because the smoothing filtered out the humidity inversions. At the resolution of the retrieved HATPRO profile, the HATPRO-only retrieval can extract more information than the synergistic retrieval at the resolution of the synergy profile because the errors are slightly smaller (solid yellow and blue lines in Fig. C1a, b). However, when comparing the specific humidity from HATPRO with the radiosonde profile smoothed with the synergy (thus, slightly higher resolution), the errors are again similar to Fig. 7. Thus, the radiosonde profile smoothed with the synergy seems to represent the average true radiosonde profile relatively well. At the surface, the resolution-corrected RMSEcorr (Fig. C1b) of the synergy is similar to the true RMSEcorr (Fig. 7b). At heights where the resolution-corrected errors are lower than the true errors, e.g. around 1500 m, the low vertical resolution of the retrieval is a significant limitation.
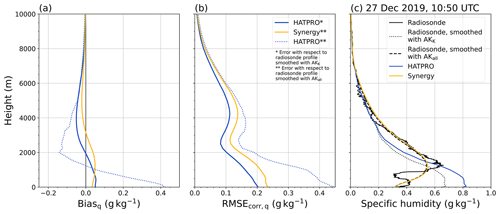
Figure C1Specific humidity q error profiles showing (a) the bias, (b) the bias-corrected RMSE of q retrieved from HATPRO (blue) and the synergy (yellow), and (c) an example specific humidity profile from 27 December 2019 at 10:50 UTC. In panels (a) and (b), the errors are computed with respect to radiosonde profiles that have been smoothed with the K-band-based averaging kernel (AKK) and the all-frequency averaging kernel (AKall), respectively. In panel (c), the original radiosonde profile is shown as a solid black line, while the profile smoothed with AKK (AKall) is visualized as a dotted (dashed) black line. The retrieved profiles from HATPRO (synergy) are displayed as solid blue (yellow) lines.
In the specific humidity profile example (Fig. C1c), the effect of the different smoothing strengths can be seen. The synergistic retrieval and the radiosonde profile at the resolution of the synergy can both identify the inversion observed by the radiosonde well. However, the specific humidity retrieved by HATPRO does not sense a strong humidity inversion near the surface. The strong overestimation of the HATPRO specific humidity compared to the HATPRO-smoothed radiosonde profile near the surface suggests that the resolution could only partly explain this deviation from the true radiosonde profile.
The retrieved synergistic profiles of temperature, specific humidity, and relative humidity, as well as integrated water vapour, are available on PANGAEA (https://doi.org/10.1594/PANGAEA.968760, Walbröl et al., 2024b; https://doi.org/10.1594/PANGAEA.968778, Walbröl et al., 2024a). The retrievals are based on brightness temperature observations from HATPRO (https://doi.org/10.1594/PANGAEA.941356, Engelmann et al., 2022) and MiRAC-P (https://doi.org/10.1594/PANGAEA.941407, Walbröl et al., 2022a). We used the single-instrument retrievals of temperature, absolute humidity, and IWV from HATPRO (https://doi.org/10.1594/PANGAEA.941389, Ebell et al., 2022) and IWV from MiRAC-P (https://doi.org/10.1594/PANGAEA.941470, Walbröl et al., 2022b) for the benefit estimation. Radiosonde measurements from MOSAiC (https://doi.org/10.1594/PANGAEA.928656, Maturilli et al., 2021) and the Polarstern track data (https://doi.org/10.1594/PANGAEA.924668, Rex, 2020; https://doi.org/10.1594/PANGAEA.924674, Haas, 2020; https://doi.org/10.1594/PANGAEA.924681, Kanzow, 2020; https://doi.org/10.1594/PANGAEA.926829, Rex, 2021a; https://doi.org/10.1594/PANGAEA.926910, Rex, 2021b) are also available on PANGAEA. Cloudnet target classifications, as well as the low-level stratus mask and the additional quality flag data, are available and can be accessed via https://doi.org/10.60656/60EA0DD0A99746BA (Engelmann et al., 2023), https://doi.org/10.1594/PANGAEA.961789 (Griesche et al., 2023), and https://doi.org/10.5281/ZENODO.7310858 (Griesche and Seifert, 2023), respectively. Met City observations have been downloaded from https://doi.org/10.18739/A2PV6B83F (Cox et al., 2023). ERA5 data on single and model levels used for the retrieval development and evaluation are accessible via https://doi.org/10.24381/cds.adbb2d47 (Hersbach et al., 2018) and https://doi.org/10.24381/cds.143582cf (Hersbach et al., 2017), respectively. On Zenodo, we published the retrieval training, test, and evaluation data (https://doi.org/10.5281/zenodo.10997365, Walbröl and Mech, 2024); the information content estimation output (https://doi.org/10.5281/zenodo.10997692, Walbröl, 2024b); and the ERA5 evaluation data predictions and reference (https://doi.org/10.5281/zenodo.10998146, Walbröl, 2024a). A snapshot of the GitHub repository containing the scripts is also archived (https://doi.org/10.5281/zenodo.13750797, Walbröl, 2024d). The PAMTRA code can be accessed via https://doi.org/10.5281/zenodo.3582992 (Mech et al., 2019b). The simulated brightness temperatures of the two radiosoundings shown in Fig. 1 can be found at https://doi.org/10.5281/zenodo.11092210 (Walbröl, 2024c).
AW and MM were involved in the retrieval preparation. AW performed retrieval development, evaluation, and information benefit analysis. All visualizations have been created by AW. AW, KE, HJG, and SC conceptualized this study and discussed the results. All authors reviewed this manuscript.
The contact author has declared that none of the authors has any competing interests.
Neither the European Commission nor ECMWF is responsible for any use that may be made of the Copernicus information or data it contains.
Publisher's note: Copernicus Publications remains neutral with regard to jurisdictional claims made in the text, published maps, institutional affiliations, or any other geographical representation in this paper. While Copernicus Publications makes every effort to include appropriate place names, the final responsibility lies with the authors.
We gratefully acknowledge the funding by the Deutsche Forschungsgemeinschaft (DFG, German Research Foundation) for ArctiC amplification: Climate Relevant Atmospheric and SurfaCe Processes, and Feedback Mechanisms (AC)3 (project number 268020496 – TRR 172 within the Transregional Collaborative Research Center). Data used in this manuscript were produced as part of the international Multidisciplinary drifting Observatory for the Study of the Arctic Climate (MOSAiC) with the tag MOSAiC20192020 and the Polarstern expedition AWI_PS122_00. We thank all those who contributed to MOSAiC and made this endeavour possible (Nixdorf et al., 2021). The microwave radiometer HATPRO was funded by the Federal Ministry of Education and Research (BMBF) under FKZ 01LKL1603A. We acknowledge the support from the OCEANET-Atmosphere project, funded by the German Federal Ministry for Education and Research (BMBF) via the SCiAMO project (MOSAIC-FKZ 03F0915A), within the framework of which the two microwave radiometers were operated. Radiosonde data were obtained through a partnership between the leading Alfred Wegener Institute (AWI), the Atmospheric Radiation Measurement (ARM) user facility (a US Department of Energy facility managed by the Biological and Environmental Research Program), and the German Weather Service (DWD). ERA5 data (Hersbach et al., 2017, 2018) were downloaded from the Copernicus Climate Change Service (C3S) Climate Data Store. The results contain modified Copernicus Climate Change Service information 2022. This work used resources of the Deutsches Klimarechenzentrum (DKRZ) granted by its Scientific Steering Committee (WLA) under project ID bb1320. Finally, the authors appreciate the discussions within the working group and among the co-authors.
This research has been supported by the Deutsche Forschungsgemeinschaft (grant no. 268020496) and the Bundesministerium für Bildung und Forschung (grant nos. 01LKL1603A and 03F0915A).
This open-access publication was funded by Universität zu Köln.
This paper was edited by Laura Bianco and reviewed by two anonymous referees.
Abadi, M., Agarwal, A., Barham, P., Brevdo, E., Chen, Z., Citro, C., Corrado, G. S., Davis, A., Dean, J., Devin, M., Ghemawat, S., Goodfellow, I., Harp, A., Irving, G., Isard, M., Jia, Y., Jozefowicz, R., Kaiser, L., Kudlur, M., Levenberg, J., Mané, D., Monga, R., Moore, S., Murray, D., Olah, C., Schuster, M., Shlens, J., Steiner, B., Sutskever, I., Talwar, K., Tucker, P., Vanhoucke, V., Vasudevan, V., Viégas, F., Vinyals, O., Warden, P., Wattenberg, M., Wicke, M., Yu, Y., and Zheng, X.: TensorFlow: Large-Scale Machine Learning on Heterogeneous Systems [software], https://www.tensorflow.org/ (last access: 23 October 2024), 2015. a
Allan, R. P., Willett, K. M., John, V. O., and Trent, T.: Global Changes in Water Vapor 1979–2020, J. Geophys. Res.-Atmos., 127, e2022JD036728, https://doi.org/10.1029/2022JD036728, 2022. a
Avila-Diaz, A., Bromwich, D. H., Wilson, A. B., Justino, F., and Wang, S.-H.: Climate Extremes across the North American Arctic in Modern Reanalyses, J. Climate, 34, 2385–2410, https://doi.org/10.1175/JCLI-D-20-0093.1, 2021. a, b
Billault-Roux, A.-C. and Berne, A.: Integrated water vapor and liquid water path retrieval using a single-channel radiometer, Atmos. Meas. Tech., 14, 2749–2769, https://doi.org/10.5194/amt-14-2749-2021, 2021. a
Cadeddu, M., Turner, D., and Liljegren, J.: A Neural Network for Real-Time Retrievals of PWV and LWP From Arctic Millimeter-Wave Ground-Based Observations, IEEE T. Geosci. Remote, 47, 1887–1900, https://doi.org/10.1109/TGRS.2009.2013205, 2009. a
Cadeddu, M. P., Liljegren, J. C., and Pazmany, A. L.: Measurements and Retrievals From a New 183-GHz Water-Vapor Radiometer in the Arctic, IEEE T. Geosci. Remote, 45, 2207–2215, https://doi.org/10.1109/TGRS.2006.888970, 2007. a, b
Cadeddu, M. P., Cimini, D., Ghate, V., Lubin, D., Vogelmann, A. M., and Silber, I.: Examination of Humidity and Ice Supersaturation Profiles Over West Antarctica Using Ground-Based G-Band Radiometer Retrievals, IEEE T. Geosci. Remote, 60, 1–16, https://doi.org/10.1109/TGRS.2021.3077088, 2022. a
Chellini, G. and Ebell, K.: Can state-of-the-art infrared satellite sounders and reanalyses detect moisture inversions in the Arctic?, Atmos. Meas. Tech. Discuss. [preprint], https://doi.org/10.5194/amt-2022-22, 2022. a
Chen, B. and Liu, Z.: Global water vapor variability and trend from the latest 36 year (1979 to 2014) data of ECMWF and NCEP reanalyses, radiosonde, GPS, and microwave satellite, J. Geophys. Res.-Atmos., 121, 11442–11462, https://doi.org/10.1002/2016JD024917, 2016. a, b, c
Cimini, D., Westwater, E. R., and Gasiewski, A. J.: Temperature and Humidity Profiling in the Arctic Using Ground-Based Millimeter-Wave Radiometry and 1DVAR, IEEE T. Geosci. Remote, 48, 1381–1388, https://doi.org/10.1109/TGRS.2009.2030500, 2010. a, b
Cox, C., Gallagher, M., Shupe, M., Persson, O., Blomquist, B., Grachev, A., Riihimaki, L., Kutchenreiter, M., Morris, V., Solomon, A., Brooks, I., Costa, D., Gottas, D., Hutchings, J., Osborn, J., Morris, S., Preusser, A., and Uttal, T.: Met City meteorological and surface flux measurements (Level 3 Final), Multidisciplinary Drifting Observatory for the Study of Arctic Climate (MOSAiC), central Arctic, October 2019–September 2020, Arctic Data Center [data set], https://doi.org/10.18739/A2PV6B83F, 2023. a, b
Crewell, S. and Löhnert, U.: Accuracy of Boundary Layer Temperature Profiles Retrieved With Multifrequency Multiangle Microwave Radiometry, IEEE T. Geosci. Remote, 45, 2195–2201, https://doi.org/10.1109/TGRS.2006.888434, 2007. a, b
Crewell, S., Czekala, H., Löhnert, U., Simmer, C., Rose, T., Zimmermann, R., and Zimmermann, R.: Microwave Radiometer for Cloud Carthography: A 22-channel ground-based microwave radiometer for atmospheric research, Radio Sci., 36, 621–638, https://doi.org/10.1029/2000RS002396, 2001. a
Crewell, S., Ebell, K., Konjari, P., Mech, M., Nomokonova, T., Radovan, A., Strack, D., Triana-Gómez, A. M., Noël, S., Scarlat, R., Spreen, G., Maturilli, M., Rinke, A., Gorodetskaya, I., Viceto, C., August, T., and Schröder, M.: A systematic assessment of water vapor products in the Arctic: from instantaneous measurements to monthly means, Atmos. Meas. Tech., 14, 4829–4856, https://doi.org/10.5194/amt-14-4829-2021, 2021. a, b
Devasthale, A., Willén, U., Karlsson, K.-G., and Jones, C. G.: Quantifying the clear-sky temperature inversion frequency and strength over the Arctic Ocean during summer and winter seasons from AIRS profiles, Atmos. Chem. Phys., 10, 5565–5572, https://doi.org/10.5194/acp-10-5565-2010, 2010. a
Devasthale, A., Sedlar, J., and Tjernström, M.: Characteristics of water-vapour inversions observed over the Arctic by Atmospheric Infrared Sounder (AIRS) and radiosondes, Atmos. Chem. Phys., 11, 9813–9823, https://doi.org/10.5194/acp-11-9813-2011, 2011. a
Ebell, K., Orlandi, E., Hünerbein, A., Löhnert, U., and Crewell, S.: Combining ground-based with satellite-based measurements in the atmospheric state retrieval: Assessment of the information content, J. Geophys. Res.-Atmos., 118, 6940–6956, https://doi.org/10.1002/jgrd.50548, 2013. a, b
Ebell, K., Löhnert, U., Päschke, E., Orlandi, E., Schween, J. H., and Crewell, S.: A 1‐D variational retrieval of temperature, humidity, and liquid cloud properties: Performance under idealized and real conditions, J. Geophys. Res.-Atmos., 122, 1746–1766, https://doi.org/10.1002/2016JD025945, 2017. a
Ebell, K., Walbröl, A., Engelmann, R., Griesche, H., Radenz, M., Hofer, J., and Althausen, D.: Temperature and humidity profiles, integrated water vapour and liquid water path derived from the HATPRO microwave radiometer onboard the Polarstern during the MOSAiC expedition, PANGAEA [data set], https://doi.org/10.1594/PANGAEA.941389, 2022. a, b
Engelmann, R., Ansmann, A., Ohneiser, K., Griesche, H., Radenz, M., Hofer, J., Althausen, D., Dahlke, S., Maturilli, M., Veselovskii, I., Jimenez, C., Wiesen, R., Baars, H., Bühl, J., Gebauer, H., Haarig, M., Seifert, P., Wandinger, U., and Macke, A.: Wildfire smoke, Arctic haze, and aerosol effects on mixed-phase and cirrus clouds over the North Pole region during MOSAiC: an introduction, Atmos. Chem. Phys., 21, 13397–13423, https://doi.org/10.5194/acp-21-13397-2021, 2021. a
Engelmann, R., Griesche, H., Radenz, M., Hofer, J., Althausen, D., Walbröl, A., and Ebell, K.: Brightness temperatures of the HATPRO microwave radiometer onboard the Polarstern during the MOSAiC expedition, PANGAEA [data set], https://doi.org/10.1594/PANGAEA.941356, 2022. a
Engelmann, R., Althausen, D., Baars, H., Griesche, H., Hofer, J., Radenz, M., and Seifert, P.: Custom collection of classification data from RV Polarstern between 11 Oct 2019 and 30 Sep 2020, ACTRIS Cloud remote sensing data centre unit (CLU) [data set], https://doi.org/10.60656/60EA0DD0A99746BA, 2023. a, b
Fionda, E., Cadeddu, M., Mattioli, V., and Pacione, R.: Intercomparison of Integrated Water Vapor Measurements at High Latitudes from Co-Located and Near-Located Instruments, Remote Sens., 11, 2130, https://doi.org/10.3390/rs11182130, 2019. a
Ghatak, D. and Miller, J.: Implications for Arctic amplification of changes in the strength of the water vapor feedback: Water vapor feedback in Arctic, J. Geophys. Res.-Atmos., 118, 7569–7578, https://doi.org/10.1002/jgrd.50578, 2013. a, b
Gierens, R., Kneifel, S., Shupe, M. D., Ebell, K., Maturilli, M., and Löhnert, U.: Low-level mixed-phase clouds in a complex Arctic environment, Atmos. Chem. Phys., 20, 3459–3481, https://doi.org/10.5194/acp-20-3459-2020, 2020. a
Graham, R. M., Cohen, L., Ritzhaupt, N., Segger, B., Graversen, R. G., Rinke, A., Walden, V. P., Granskog, M. A., and Hudson, S. R.: Evaluation of Six Atmospheric Reanalyses over Arctic Sea Ice from Winter to Early Summer, J. Climate, 32, 4121–4143, https://doi.org/10.1175/JCLI-D-18-0643.1, 2019a. a, b, c
Graham, R. M., Hudson, S. R., and Maturilli, M.: Improved Performance of ERA5 in Arctic Gateway Relative to Four Global Atmospheric Reanalyses, Geophys. Res. Lett., 46, 6138–6147, https://doi.org/10.1029/2019GL082781, 2019b. a, b
Graversen, R. G. and Wang, M.: Polar amplification in a coupled climate model with locked albedo, Clim. Dynam., 33, 629–643, https://doi.org/10.1007/s00382-009-0535-6, 2009. a
Graversen, R. G., Mauritsen, T., Tjernström, M., Källén, E., and Svensson, G.: Vertical structure of recent Arctic warming, Nature, 451, 53–56, https://doi.org/10.1038/nature06502, 2008. a
Griesche, H., Seifert, P., Engelmann, R., Radenz, M., Hofer, J., and Althausen, D.: Low-level stratus mask from Polarstern during MOSAiC, PANGAEA [data set], https://doi.org/10.1594/PANGAEA.961789, 2023. a, b
Griesche, H. J. and Seifert, P.: MOSAiC Cloudnet issue data set, Zenodo [data set], https://doi.org/10.5281/ZENODO.7310858, 2023. a, b
Griesche, H. J., Seifert, P., Ansmann, A., Baars, H., Barrientos Velasco, C., Bühl, J., Engelmann, R., Radenz, M., Zhenping, Y., and Macke, A.: Application of the shipborne remote sensing supersite OCEANET for profiling of Arctic aerosols and clouds during Polarstern cruise PS106, Atmos. Meas. Tech., 13, 5335–5358, https://doi.org/10.5194/amt-13-5335-2020, 2020. a, b
Griesche, H. J., Seifert, P., Engelmann, R., Radenz, M., Hofer, J., Althausen, D., Walbröl, A., Barrientos-Velasco, C., Baars, H., Dahlke, S., Tukiainen, S., and Macke, A.: Cloud micro- and macrophysical properties from ground-based remote sensing during the MOSAiC drift experiment, Scientific Data, 11, 505, https://doi.org/10.1038/s41597-024-03325-w, 2024. a
Haas, C.: Links to master tracks in different resolutions of POLARSTERN cruise PS122/2, Arctic Ocean – Arctic Ocean, 2019-12-13 – 2020-02-24 (Version 2), Alfred Wegener Institute, Helmholtz Centre for Polar and Marine Research, Bremerhaven, PANGAEA [data set], https://doi.org/10.1594/PANGAEA.924674, 2020. a
Held, I. M. and Soden, B. J.: Water Vapor Feedback and Global Warming, Annu. Rev. Energ. Env., 25, 441–475, https://doi.org/10.1146/annurev.energy.25.1.441, 2000. a
Herrmannsdörfer, L., Müller, M., Shupe, M. D., and Rostosky, P.: Surface temperature comparison of the Arctic winter MOSAiC observations, ERA5 reanalysis, and MODIS satellite retrieval, Elem. Sci. Anth., 11, 00085, https://doi.org/10.1525/elementa.2022.00085, 2023. a
Hersbach, H., Bell, B., Berrisford, P., Hirahara, S., Horányi, A., Muñoz-Sabater, J., Nicolas, J., Peubey, C., Radu, R., Schepers, D., Simmons, A., Soci, C., Abdalla, S., Abellan, X., Balsamo, G., Bechtold, P., Biavati, G., Bidlot, J., Bonavita, M., De Chiara, G., Dahlgren, P., Dee, D., Diamantakis, M., Dragani, R., Flemming, J., Forbes, R., Fuentes, M., Geer, A., Haimberger, L., Healy, S., Hogan, R., Hólm, E., Janisková, M., Keeley, S., Laloyaux, P., Lopez, P., Lupu, C., Radnoti, G., de Rosnay, P., Rozum, I., Vamborg, F., Villaume, S., and Thépaut, J.-N.: Complete ERA5 from 1940: Fifth generation of ECMWF atmospheric reanalyses of the global climate, Copernicus Climate Change Service (C3S) Data Store (CDS) [data set], https://doi.org/10.24381/cds.143582cf, 2017. a, b
Hersbach, H., Bell, B., Berrisford, P., Biavati, G., Horányi, A., Muñoz Sabater, J., Nicolas, J., Peubey, C., Radu, R., Rozum, I., Schepers, D., Simmons, A., Soci, C., Dee, D., and Thépaut, J.-N.: ERA5 hourly data on single levels from 1959 to present, Copernicus Climate Change Service (C3S) Climate Data Store (CDS) [data set], https://doi.org/10.24381/cds.adbb2d47, 2018. a, b
Hersbach, H., Bell, B., Berrisford, P., Hirahara, S., Horányi, A., Muñoz‐Sabater, J., Nicolas, J., Peubey, C., Radu, R., Schepers, D., Simmons, A., Soci, C., Abdalla, S., Abellan, X., Balsamo, G., Bechtold, P., Biavati, G., Bidlot, J., Bonavita, M., Chiara, G., Dahlgren, P., Dee, D., Diamantakis, M., Dragani, R., Flemming, J., Forbes, R., Fuentes, M., Geer, A., Haimberger, L., Healy, S., Hogan, R. J., Hólm, E., Janisková, M., Keeley, S., Laloyaux, P., Lopez, P., Lupu, C., Radnoti, G., Rosnay, P., Rozum, I., Vamborg, F., Villaume, S., and Thépaut, J.: The ERA5 global reanalysis, Q. J. Roy. Meteor. Soc., 146, 1999–2049, https://doi.org/10.1002/qj.3803, 2020. a, b
Illingworth, A. J., Hogan, R. J., O'Connor, E., Bouniol, D., Brooks, M. E., Delanoé, J., Donovan, D. P., Eastment, J. D., Gaussiat, N., Goddard, J. W. F., Haeffelin, M., Baltink, H. K., Krasnov, O. A., Pelon, J., Piriou, J.-M., Protat, A., Russchenberg, H. W. J., Seifert, A., Tompkins, A. M., van Zadelhoff, G.-J., Vinit, F., Willén, U., Wilson, D. R., and Wrench, C. L.: Cloudnet, B. Am. Meteorol. Soc., 88, 883–898, https://doi.org/10.1175/BAMS-88-6-883, 2007. a
Kanzow, T.: Links to master tracks in different resolutions of POLARSTERN cruise PS122/3, Arctic Ocean – Longyearbyen, 2020-02-24 – 2020-06-04 (Version 2), Alfred Wegener Institute, Helmholtz Centre for Polar and Marine Research, Bremerhaven, PANGAEA [data set], https://doi.org/10.1594/PANGAEA.924681, 2020. a
Kingma, D. P. and Ba, J.: Adam: A Method for Stochastic Optimization, arXiv [preprint], https://doi.org/10.48550/arXiv.1412.6980, 30 January 2017. a
Knust, R.: Polar Research and Supply Vessel POLARSTERN operated by the Alfred-Wegener-Institute, Journal of Large-Scale Research Facilities JLSRF, 3, A119, https://doi.org/10.17815/jlsrf-3-163, 2017. a
Loeb, N. A., Crawford, A., Stroeve, J. C., and Hanesiak, J.: Extreme Precipitation in the Eastern Canadian Arctic and Greenland: An Evaluation of Atmospheric Reanalyses, Front. Environ. Sci., 10, 866929, https://doi.org/10.3389/fenvs.2022.866929, 2022. a, b
Löhnert, U.: Ground-based microwave radiometer reprocessing mwr_pro, Zenodo [code], https://doi.org/10.5281/zenodo.7973553, 2023. a
Löhnert, U. and Maier, O.: Operational profiling of temperature using ground-based microwave radiometry at Payerne: prospects and challenges, Atmos. Meas. Tech., 5, 1121–1134, https://doi.org/10.5194/amt-5-1121-2012, 2012. a, b, c
Löhnert, U., Turner, D. D., and Crewell, S.: Ground-Based Temperature and Humidity Profiling Using Spectral Infrared and Microwave Observations. Part I: Simulated Retrieval Performance in Clear-Sky Conditions, J. Appl. Meteorol. Clim., 48, 1017–1032, https://doi.org/10.1175/2008JAMC2060.1, 2009. a, b, c
Macke, A., Kalisch, J., Zoll, Y., and Bumke, K.: Radiative effects of the cloudy atmosphere from ground and satellite based observations, EPJ Web Conf., 9, 83–94, https://doi.org/10.1051/epjconf/201009006, 2010. a
Marke, T., Löhnert, U., Tukiainen, S., Siipola, T., and Pospichal, B.: MWRpy: A Python package for processing microwave radiometer data, Journal of Open Source Software, 9, 6733, https://doi.org/10.21105/joss.06733, 2024. a
Mathew, N., Heygster, G., Melsheimer, C., and Kaleschke, L.: Surface Emissivity of Arctic Sea Ice at AMSU Window Frequencies, IEEE T. Geosci. Remote, 46, 2298–2306, https://doi.org/10.1109/TGRS.2008.916630, 2008. a
Maturilli, M. and Kayser, M.: Arctic warming, moisture increase and circulation changes observed in the Ny-Ålesund homogenized radiosonde record, Theor. Appl. Climatol., 130, 1–17, https://doi.org/10.1007/s00704-016-1864-0, 2017. a, b
Maturilli, M., Holdridge, D. J., Dahlke, S., Graeser, J., Sommerfeld, A., Jaiser, R., Deckelmann, H., and Schulz, A.: Initial radiosonde data from 2019-10 to 2020-09 during project MOSAiC, Alfred Wegener Institute, Helmholtz Centre for Polar and Marine Research, Bremerhaven, PANGAEA [data set], https://doi.org/10.1594/PANGAEA.928656, 2021. a, b, c, d
Mech, M., Kliesch, L.-L., Anhäuser, A., Rose, T., Kollias, P., and Crewell, S.: Microwave Radar/radiometer for Arctic Clouds (MiRAC): first insights from the ACLOUD campaign, Atmos. Meas. Tech., 12, 5019–5037, https://doi.org/10.5194/amt-12-5019-2019, 2019a. a
Mech, M., Maahn, M., Ori, D., and Orlandi, E.: PAMTRA: Passive and Active Microwave TRAnsfer tool v1.0, Zenodo [code], https://doi.org/10.5281/zenodo.3582992, 2019b. a
Mech, M., Maahn, M., Kneifel, S., Ori, D., Orlandi, E., Kollias, P., Schemann, V., and Crewell, S.: PAMTRA 1.0: the Passive and Active Microwave radiative TRAnsfer tool for simulating radiometer and radar measurements of the cloudy atmosphere, Geosci. Model Dev., 13, 4229–4251, https://doi.org/10.5194/gmd-13-4229-2020, 2020. a, b
Mewes, D. and Jacobi, C.: Heat transport pathways into the Arctic and their connections to surface air temperatures, Atmos. Chem. Phys., 19, 3927–3937, https://doi.org/10.5194/acp-19-3927-2019, 2019. a
Naakka, T., Nygård, T., and Vihma, T.: Arctic Humidity Inversions: Climatology and Processes, J. Climate, 31, 3765–3787, https://doi.org/10.1175/JCLI-D-17-0497.1, 2018. a
Nixdorf, U., Dethloff, K., Rex, M., Shupe, M., Sommerfeld, A., Perovich, D. K., Nicolaus, M., Heuzé, C., Rabe, B., Loose, B., Damm, E., Gradinger, R., Fong, A., Maslowski, W., Rinke, A., Kwok, R., Spreen, G., Wendisch, M., Herber, A., Hirsekorn, M., Mohaupt, V., Frickenhaus, S., Immerz, A., Weiss-Tuider, K., König, B., Mengedoht, D., Regnery, J., Gerchow, P., Ransby, D., Krumpen, T., Morgenstern, A., Haas, C., Kanzow, T., Rack, F. R., Saitzev, V., Sokolov, V., Makarov, A., Schwarze, S., Wunderlich, T., Wurr, K., and Boetius, A.: MOSAiC Extended Acknowledgement, Zenodo, https://doi.org/10.5281/zenodo.5541624, 2021. a
Nygård, T., Valkonen, T., and Vihma, T.: Characteristics of Arctic low-tropospheric humidity inversions based on radio soundings, Atmos. Chem. Phys., 14, 1959–1971, https://doi.org/10.5194/acp-14-1959-2014, 2014. a, b
Parracho, A. C., Bock, O., and Bastin, S.: Global IWV trends and variability in atmospheric reanalyses and GPS observations, Atmos. Chem. Phys., 18, 16213–16237, https://doi.org/10.5194/acp-18-16213-2018, 2018. a, b, c
Perro, C., Lesins, G., Duck, T. J., and Cadeddu, M.: A microwave satellite water vapour column retrieval for polar winter conditions, Atmos. Meas. Tech., 9, 2241–2252, https://doi.org/10.5194/amt-9-2241-2016, 2016. a
Rantanen, M., Karpechko, A. Y., Lipponen, A., Nordling, K., Hyvärinen, O., Ruosteenoja, K., Vihma, T., and Laaksonen, A.: The Arctic has warmed nearly four times faster than the globe since 1979, Communications Earth & Environment, 3, 168, https://doi.org/10.1038/s43247-022-00498-3, 2022. a
Rex, M.: Links to master tracks in different resolutions of POLARSTERN cruise PS122/1, Tromsø – Arctic Ocean, 2019-09-20 – 2019-12-13 (Version 2), Alfred Wegener Institute, Helmholtz Centre for Polar and Marine Research, Bremerhaven, PANGAEA [data set], https://doi.org/10.1594/PANGAEA.924668, 2020. a
Rex, M.: Master tracks in different resolutions of POLARSTERN cruise PS122/4, Longyearbyen – Arctic Ocean, 2020-06-04 – 2020-08-12, Alfred Wegener Institute, Helmholtz Centre for Polar and Marine Research, Bremerhaven, PANGAEA [data set], https://doi.org/10.1594/PANGAEA.926829, 2021a. a
Rex, M.: Master tracks in different resolutions of POLARSTERN cruise PS122/5, Arctic Ocean – Bremerhaven, 2020-08-12 – 2020-10-12, Alfred Wegener Institute, Helmholtz Centre for Polar and Marine Research, Bremerhaven, PANGAEA [data set], https://doi.org/10.1594/PANGAEA.926910, 2021b. a
Rinke, A., Segger, B., Crewell, S., Maturilli, M., Naakka, T., Nygård, T., Vihma, T., Alshawaf, F., Dick, G., Wickert, J., and Keller, J.: Trends of Vertically Integrated Water Vapor over the Arctic during 1979–2016: Consistent Moistening All Over?, J. Climate, 32, 6097–6116, https://doi.org/10.1175/JCLI-D-19-0092.1, 2019. a, b, c
Rodgers, C. D.: Inverse methods for atmospheric sounding: theory and practice, no. 2 in Series on atmospheric, oceanic and planetary physics, World Scientific, Singapore, repr edn., ISBN 978-981-02-2740-1, 2008. a, b
Rose, T., Crewell, S., Löhnert, U., and Simmer, C.: A network suitable microwave radiometer for operational monitoring of the cloudy atmosphere, Atmos. Res., 75, 183–200, https://doi.org/10.1016/j.atmosres.2004.12.005, 2005. a, b
Rosenkranz, P. W.: Water vapor microwave continuum absorption: A comparison of measurements and models, Radio Sci., 33, 919–928, https://doi.org/10.1029/98RS01182, 1998. a
Scarlat, R. C., Heygster, G., and Pedersen, L. T.: Experiences With an Optimal Estimation Algorithm for Surface and Atmospheric Parameter Retrieval From Passive Microwave Data in the Arctic, IEEE J. Sel. Top. Appl., 10, 3934–3947, https://doi.org/10.1109/JSTARS.2017.2739858, 2017. a
Screen, J. A. and Simmonds, I.: The central role of diminishing sea ice in recent Arctic temperature amplification, Nature, 464, 1334–1337, https://doi.org/10.1038/nature09051, 2010. a
Screen, J. A., Deser, C., and Simmonds, I.: Local and remote controls on observed Arctic warming: Controls on Arctic warming, Geophys. Res. Lett., 39, L10709, https://doi.org/10.1029/2012GL051598, 2012. a
Serreze, M. C. and Barry, R. G.: Processes and impacts of Arctic amplification: A research synthesis, Global Planet. Change, 77, 85–96, https://doi.org/10.1016/j.gloplacha.2011.03.004, 2011. a
Serreze, M. C., Barrett, A. P., and Stroeve, J.: Recent changes in tropospheric water vapor over the Arctic as assessed from radiosondes and atmospheric reanalyses: Changes in Arctic water vapor, J. Geophys. Res.-Atmos., 117, D10104, https://doi.org/10.1029/2011JD017421, 2012. a
Shupe, M. D., Rex, M., Blomquist, B., Persson, P. O. G., Schmale, J., Uttal, T., Althausen, D., Angot, H., Archer, S., Bariteau, L., Beck, I., Bilberry, J., Bucci, S., Buck, C., Boyer, M., Brasseur, Z., Brooks, I. M., Calmer, R., Cassano, J., Castro, V., Chu, D., Costa, D., Cox, C. J., Creamean, J., Crewell, S., Dahlke, S., Damm, E., de Boer, G., Deckelmann, H., Dethloff, K., Dütsch, M., Ebell, K., Ehrlich, A., Ellis, J., Engelmann, R., Fong, A. A., Frey, M. M., Gallagher, M. R., Ganzeveld, L., Gradinger, R., Graeser, J., Greenamyer, V., Griesche, H., Griffiths, S., Hamilton, J., Heinemann, G., Helmig, D., Herber, A., Heuzé, C., Hofer, J., Houchens, T., Howard, D., Inoue, J., Jacobi, H.-W., Jaiser, R., Jokinen, T., Jourdan, O., Jozef, G., King, W., Kirchgaessner, A., Klingebiel, M., Krassovski, M., Krumpen, T., Lampert, A., Landing, W., Laurila, T., Lawrence, D., Lonardi, M., Loose, B., Lüpkes, C., Maahn, M., Macke, A., Maslowski, W., Marsay, C., Maturilli, M., Mech, M., Morris, S., Moser, M., Nicolaus, M., Ortega, P., Osborn, J., Pätzold, F., Perovich, D. K., Petäjä, T., Pilz, C., Pirazzini, R., Posman, K., Powers, H., Pratt, K. A., Preußer, A., Quéléver, L., Radenz, M., Rabe, B., Rinke, A., Sachs, T., Schulz, A., Siebert, H., Silva, T., Solomon, A., Sommerfeld, A., Spreen, G., Stephens, M., Stohl, A., Svensson, G., Uin, J., Viegas, J., Voigt, C., von der Gathen, P., Wehner, B., Welker, J. M., Wendisch, M., Werner, M., Xie, Z., and Yue, F.: Overview of the MOSAiC expedition – Atmosphere, Elem. Sci. Anth., 10, 00060, https://doi.org/10.1525/elementa.2021.00060, 2022. a
Solheim, F., Godwin, J. R., Westwater, E. R., Han, Y., Keihm, S. J., Marsh, K., and Ware, R.: Radiometric profiling of temperature, water vapor and cloud liquid water using various inversion methods, Radio Sci., 33, 393–404, https://doi.org/10.1029/97RS03656, 1998. a
Tjernström, M., Leck, C., Persson, P. O. G., Jensen, M. L., Oncley, S. P., and Targino, A.: Experimental Equipment: A Supplement to The Summertime Arctic Atmosphere: Meteorological Measurements during the Arctic Ocean Experiment 2001, B. Am. Meteorol. Soc., 85, ES14–ES18, https://doi.org/10.1175/BAMS-85-9-Tjernstrom, 2004. a
Tjernström, M., Shupe, M. D., Brooks, I. M., Achtert, P., Prytherch, J., and Sedlar, J.: Arctic Summer Airmass Transformation, Surface Inversions, and the Surface Energy Budget, J. Climate, 32, 769–789, https://doi.org/10.1175/JCLI-D-18-0216.1, 2019. a, b
Tukiainen, S., O'Connor, E., and Korpinen, A.: CloudnetPy: A Python package for processing cloud remote sensing data, Journal of Open Source Software, 5, 2123, https://doi.org/10.21105/joss.02123, 2020. a
Vihma, T., Kilpeläinen, T., Manninen, M., Sjöblom, A., Jakobson, E., Palo, T., Jaagus, J., and Maturilli, M.: Characteristics of Temperature and Humidity Inversions and Low-Level Jets over Svalbard Fjords in Spring, Adv. Meteorol., 2011, 1–14, https://doi.org/10.1155/2011/486807, 2011. a
Walbröl, A.: Neural Network predictions and ERA5 reference of integrated water vapour, and temperature and specific humidity profiles based on simulated microwave radiometer observations, Zenodo [data set], https://doi.org/10.5281/zenodo.10998146, 2024a. a
Walbröl, A.: Information content estimation output for specific humidity profiles, Zenodo [data set], https://doi.org/10.5281/zenodo.10997692, 2024b. a
Walbröl, A.: Simulated microwave brightness temperatures based on two radiosoundings performed during the MOSAiC expedition, Zenodo [data set], https://doi.org/10.5281/zenodo.11092210, 2024c. a
Walbröl, A.: Codes for: Combining low and high frequency microwave radiometer measurements from the MOSAiC expedition for enhanced water vapour products, Zenodo [code], https://doi.org/10.5281/zenodo.13750797, 2024d. a
Walbröl, A. and Mech, M.: ERA5 based training, validation and evaluation data for retrievals combining 22–58 GHz with 175–340 GHz microwave radiometer measurements during MOSAiC, Zenodo [data set], https://doi.org/10.5281/zenodo.10997365, 2024. a
Walbröl, A., Ebell, K., Engelmann, R., Griesche, H., Radenz, M., Hofer, J., Althausen, D., and Crewell, S.: Brightness temperatures of the MiRAC-P microwave radiometer onboard the Polarstern during the MOSAiC expedition, PANGAEA [data set], https://doi.org/10.1594/PANGAEA.941407, 2022a. a
Walbröl, A., Orlandi, E., Crewell, S., and Ebell, K.: Integrated water vapour derived from the MiRAC-P microwave radiometer onboard the Polarstern during the MOSAiC expedition, PANGAEA [data set], https://doi.org/10.1594/PANGAEA.941470, 2022b. a, b
Walbröl, A., Crewell, S., Engelmann, R., Orlandi, E., Griesche, H., Radenz, M., Hofer, J., Althausen, D., Maturilli, M., and Ebell, K.: Atmospheric temperature, water vapour and liquid water path from two microwave radiometers during MOSAiC, Scientific Data, 9, 534, https://doi.org/10.1038/s41597-022-01504-1, 2022c. a, b, c, d, e, f, g, h, i, j, k
Walbröl, A., Engelmann, R., Griesche, H., Radenz, M., Hofer, J., Althausen, D., Ebell, K., Mech, M., and Crewell, S.: Improved integrated water vapour derived from the combination of the microwave radiometers HATPRO and MiRAC-P aboard the Polarstern during the MOSAiC expedition, PANGAEA [data set], https://doi.org/10.1594/PANGAEA.968778, 2024a. a
Walbröl, A., Engelmann, R., Griesche, H., Radenz, M., Hofer, J., Althausen, D., Ebell, K., Mech, M., and Crewell, S.: Improved temperature and humidity profiles derived from the combination of the microwave radiometers HATPRO and MiRAC-P aboard the Polarstern during the MOSAiC expedition, PANGAEA [data set], https://doi.org/10.1594/PANGAEA.968760, 2024b. a
Wang, C., Graham, R. M., Wang, K., Gerland, S., and Granskog, M. A.: Comparison of ERA5 and ERA-Interim near-surface air temperature, snowfall and precipitation over Arctic sea ice: effects on sea ice thermodynamics and evolution, The Cryosphere, 13, 1661–1679, https://doi.org/10.5194/tc-13-1661-2019, 2019. a, b, c
Wang, D., Prigent, C., Kilic, L., Fox, S., Harlow, C., Jimenez, C., Aires, F., Grassotti, C., and Karbou, F.: Surface Emissivity at Microwaves to Millimeter Waves over Polar Regions: Parameterization and Evaluation with Aircraft Experiments, J. Atmos. Ocean. Tech., 34, 1039–1059, https://doi.org/10.1175/JTECH-D-16-0188.1, 2017. a
Wendisch, M., Brückner, M., Crewell, S., Ehrlich, A., Notholt, J., Lüpkes, C., Macke, A., Burrows, J. P., Rinke, A., Quaas, J., Maturilli, M., Schemann, V., Shupe, M. D., Akansu, E. F., Barrientos-Velasco, C., Bärfuss, K., Blechschmidt, A.-M., Block, K., Bougoudis, I., Bozem, H., Böckmann, C., Bracher, A., Bresson, H., Bretschneider, L., Buschmann, M., Chechin, D. G., Chylik, J., Dahlke, S., Deneke, H., Dethloff, K., Donth, T., Dorn, W., Dupuy, R., Ebell, K., Egerer, U., Engelmann, R., Eppers, O., Gerdes, R., Gierens, R., Gorodetskaya, I. V., Gottschalk, M., Griesche, H., Gryanik, V. M., Handorf, D., Harm-Altstädter, B., Hartmann, J., Hartmann, M., Heinold, B., Herber, A., Herrmann, H., Heygster, G., Höschel, I., Hofmann, Z., Hölemann, J., Hünerbein, A., Jafariserajehlou, S., Jäkel, E., Jacobi, C., Janout, M., Jansen, F., Jourdan, O., Jurányi, Z., Kalesse-Los, H., Kanzow, T., Käthner, R., Kliesch, L. L., Klingebiel, M., Knudsen, E. M., Kovács, T., Körtke, W., Krampe, D., Kretzschmar, J., Kreyling, D., Kulla, B., Kunkel, D., Lampert, A., Lauer, M., Lelli, L., von Lerber, A., Linke, O., Löhnert, U., Lonardi, M., Losa, S. N., Losch, M., Maahn, M., Mech, M., Mei, L., Mertes, S., Metzner, E., Mewes, D., Michaelis, J., Mioche, G., Moser, M., Nakoudi, K., Neggers, R., Neuber, R., Nomokonova, T., Oelker, J., Papakonstantinou-Presvelou, I., Pätzold, F., Pefanis, V., Pohl, C., van Pinxteren, M., Radovan, A., Rhein, M., Rex, M., Richter, A., Risse, N., Ritter, C., Rostosky, P., Rozanov, V. V., Donoso, E. R., Saavedra Garfias, P., Salzmann, M., Schacht, J., Schäfer, M., Schneider, J., Schnierstein, N., Seifert, P., Seo, S., Siebert, H., Soppa, M. A., Spreen, G., Stachlewska, I. S., Stapf, J., Stratmann, F., Tegen, I., Viceto, C., Voigt, C., Vountas, M., Walbröl, A., Walter, M., Wehner, B., Wex, H., Willmes, S., Zanatta, M., and Zeppenfeld, S.: Atmospheric and Surface Processes, and Feedback Mechanisms Determining Arctic Amplification: A Review of First Results and Prospects of the (AC)3 Project, B. Am. Meteorol. Soc., 104, E208–E242, https://doi.org/10.1175/BAMS-D-21-0218.1, 2023. a, b
Yu, Y., Xiao, W., Zhang, Z., Cheng, X., Hui, F., and Zhao, J.: Evaluation of 2-m Air Temperature and Surface Temperature from ERA5 and ERA-I Using Buoy Observations in the Arctic during 2010–2020, Remote Sens., 13, 2813, https://doi.org/10.3390/rs13142813, 2021. a, b
- Abstract
- Introduction
- Datasets
- Methods
- Retrieval evaluation
- Information benefit analysis
- Conclusions
- Appendix A: Neural network retrieval details
- Appendix B: Information benefit: influence of different methods
- Appendix C: Comparison with smoothed radiosonde profiles
- Code and data availability
- Author contributions
- Competing interests
- Disclaimer
- Acknowledgements
- Financial support
- Review statement
- References
- Abstract
- Introduction
- Datasets
- Methods
- Retrieval evaluation
- Information benefit analysis
- Conclusions
- Appendix A: Neural network retrieval details
- Appendix B: Information benefit: influence of different methods
- Appendix C: Comparison with smoothed radiosonde profiles
- Code and data availability
- Author contributions
- Competing interests
- Disclaimer
- Acknowledgements
- Financial support
- Review statement
- References