the Creative Commons Attribution 4.0 License.
the Creative Commons Attribution 4.0 License.
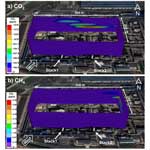
Development of a continuous UAV-mounted air sampler and application to the quantification of CO2 and CH4 emissions from a major coking plant
Tianran Han
Conghui Xie
Yayong Liu
Yanrong Yang
Yuheng Zhang
Yufei Huang
Xiangyu Gao
Xiaohua Zhang
Fangmin Bao
The development in uncrewed aerial vehicle (UAV) technologies over the past decade has led to a plethora of platforms that can potentially enable greenhouse gas emission quantification. Here, we report the development of a new air sampler, consisting of a pumped stainless coiled tube of 150 m in length with controlled time stamping, and its deployment from an industrial UAV to quantify CO2 and CH4 emissions from the main coking plant stacks of a major steel maker in eastern China. Laboratory tests show that the time series of CO2 and CH4 measured using the sampling system is smoothed when compared to online measurement by the cavity ring-down spectrometer (CRDS) analyzer. Further analyses show that the smoothing is akin to a convolution of the true time series signals with a heavy-tailed digital filter. For field testing, the air sampler was mounted on the UAV and flown in virtual boxes around two stacks in the coking plant of the Shagang Group (steel producer). Mixing ratios of CO2 and CH4 in air and meteorological parameters were measured from the UAV during the test flight. A mass-balance computational algorithm was used on the data to estimate the CO2 and CH4 emission rates from the stacks. Using this algorithm, the emission rates for the two stacks from the coking plant were calculated to be 0.12±0.014 t h−1 for CH4 and 110±18 t h−1 for CO2, the latter being in excellent agreement with material-balance-based estimates. A Gaussian plume inversion approach was also used to derive the emission rates, and the results were compared with those derived using the mass-balance algorithm, showing a good agreement between the two methods.
- Article
(4507 KB) - Full-text XML
-
Supplement
(609 KB) - BibTeX
- EndNote
Atmospheric carbon dioxide (CO2) and methane (CH4) are the two major anthropogenic greenhouse gases (GHGs). Both CO2 and CH4 in the atmosphere have been increasing since the industrial revolution, particularly rapidly over the past 10 years. Global networks consistently show that the globally averaged annual mean CO2 molar fraction in the atmosphere increased by 5.0 % from 2011 to 2019, reaching 409.9±0.4 ppm in 2019. Likewise, the globally averaged surface atmospheric molar fraction of CH4 in 2019 was 1866.3±3.3 ppb, 3.5 % higher than in 2011 (IPCC, 2021). CH4 is a stronger absorber of Earth's thermal infrared radiation than CO2, with its global warming potential (GWP) 32 times greater than that of CO2 over a 100-year horizon (Saunois et al., 2020). Although its molar fractions in the atmosphere are about 200 times lower than those of CO2, the total radiative forcing of ∼ 1.0 W m−2 for CH4 is about half of that of CO2 (∼ 2 W m−2) (IPCC, 2021), contributed by its direct radiative forcing of (0.6±0.1) W m−2 and indirect forcing of 0.4 W m−2 that results from chemical reactions, producing other GHGs including CO2, O3 and stratospheric water (Turner et al., 2019). Furthermore, although global anthropogenic CH4 emissions are estimated to be only 3 % of the global anthropogenic CO2 emissions in units of carbon mass flux, the increase in atmospheric CH4 is responsible for about 20 % of the warming induced by long-lived greenhouse gases since pre-industrial times (Etminan et al., 2016). Both CO2 and CH4 are produced and released into the atmosphere from a variety of natural and anthropogenic sources. Natural emission sources include vegetation, oceans, volcanoes and naturally occurring wildfires, but most of the increases in atmospheric CO2 and CH4 are considered to have resulted from anthropogenic emissions, from sources including fossil fuel production and uses, agricultural activities, land use, and industrial processes (IPCC, 2021).
Quantification of CO2 and CH4 emissions from sources requires continuous measurements of their mixing ratios as well as meteorological parameters using a variety of stationary and mobile platforms, including ground-based vehicles (Rella et al., 2015; Brantley et al., 2014), towers (Helfter et al., 2016; Takano and Ueyama, 2021), aircraft (Li et al., 2017; Liggio et al., 2019) and satellites (Miller et al., 2013; Turner et al., 2015). Small uncrewed aerial vehicles (UAVs) have become emerging platforms due to recent rapid technological developments. They are flexible, versatile and relatively inexpensive. Most importantly, a UAV platform fills the sampling space between the ground and altitudes of up to hundreds of meters above ground, in which other mobile platforms have been unable to operate (Shaw et al., 2021). Due to their relatively low flying speeds, UAV platforms offer a high spatiotemporal resolution for sampling and thus enable accurate plume mapping. On the other hand, UAVs have limited endurance, being constrained by battery capacities and payloads, making them more suitable for small-facility flux quantification.
UAV platforms have been used to quantify CH4 emissions in several studies, mainly focused on facility-scale emission sources including landfills (Allen et al., 2019; Bel Hadj Ali et al., 2020), coal mines (Andersen et al., 2021), dairy farms (Vinkovic et al., 2022), wastewater treatment plants (Gålfalk et al., 2021), and oil and gas facilities (Golston et al., 2018; Li et al., 2020; Nathan et al., 2015; Shah et al., 2020; Tuzson et al., 2021). UAV-based CH4 measurements are generally made with three different methods: collecting onboard samples for subsequent analysis, tethered sampling to a sensor on the ground and online measurements (Shaw et al., 2021). Gas samples could be stored on board a UAV for subsequent analyses on the ground after landing, using air bags (Brownlow et al., 2016) or sampling canisters (Chang et al., 2016). Andersen et al. (2018) developed a UAV-based active AirCore system, consisting of long coiled stainless-steel tubing, a small pinhole orifice and a pump that drags air through the tube, which allow for a higher spatiotemporal resolution in the measurements. Direct comparisons between a quantum cascade laser absorption spectrometer (QCLAS) and the active AirCore measurements show that the active AirCore measurements are smoothed by 20 s and have an average time lag of 7 s. The active AirCore measurements also stretch linearly with time at an average rate of 0.06 s for every second of QCLAS measurement (Morales et al., 2022). The advances in active AirCore sampling have made UAV measurements for CH4 emissions feasible, even if still with room for improvement. Studies of using UAVs for CO2 plume detection and mapping from anthropogenic sources have also been reported (Reuter el al., 2021; Liu et al., 2022; Leitner et al., 2023; Chiba et al., 2019). Reuter et al. (2021) presented the development of a UAV platform to quantify the CO2 emissions of anthropogenic point sources by deployment of an NDIR (non-dispersive infrared) detector and a 2-D ultrasonic acoustic resonance anemometer on the platform.
In this study, we developed a new active air sampling system for deployment from a UAV on a trajectory in three-dimensional space to measure CO2 and CH4. The complete sampler plus UAV system was deployed to quantify CO2 and CH4 emissions from the stacks of the main coking plant of the Shagang Group, the largest private steel maker in China. The top-down emission rate retrieval algorithm (TERRA) (Gordon et al., 2015) was applied to the UAV data to determine stack CH4 and CO2 emissions rates. The iron and steel industry is one of the largest contributing industries to global GHG emissions, accounting for around 7 % of global total GHG emissions (Hasanbeigi, 2022). Coke production is one major process of iron and steel making that generates emissions of CO2 and CH4. During coke production, coking coal is used to manufacture metallurgical coke that is subsequently used as the reducing agent in the production of iron and steel (U.S. Environmental Protection Agency, 2016). Coke oven gas is the main source of CO2 and CH4 emissions during coke production (Angeli et al., 2021; IPCC, 2006). China is the largest coke producer in the world, with coke production of 4.72×109 t in 2020. The GHG emissions from coke production in China are reported based on the Tier-1 methodology of the IPCC Guidelines, which multiplies generic default emission factors with the tonnage of coke produced (Ministry of Ecology and Environment of China, 2018). Tier-1 methodologies are the simplest and least complex, requiring fewer resources to collect the necessary data and produce GHG emission estimates. The present UAV-measurement-based emission results can be compared with material-balance-based emission estimates and the emissions based on the Tier-1 emission factors and coke production at the plant, and they can shed light on the uncertainties related to Tier-1 emission factors in the case of CH4 emissions.
2.1 The air sampling system
To realize GHG emission quantification by UAV measurement, a new compact air sampling system was developed based on a variation in the active AirCore method. The AirCore system contains a 150 m long stainless-steel tube, open at one end and closed at the other, that relies on positive changes in ambient pressure for passive sampling of the atmosphere (Karion et al., 2010). Figure 1 shows an overview of the patented design for this sampler. It consists of 150 m long thin-walled in. outside-diameter stainless-steel tubing, a pump, a micro-orifice, a CO2 marker generator, two three-way solenoid valves and electric relays, with all electrical devices powered by a 12 V battery. The tubing is wound into a multilayer coil, in whose center the other components of the system are mounted. The system is housed in a highly compact patented carbon fiber assembly design of 280 mm diameter and 98 mm height that can be quickly mounted at and dismounted from the bottom of a UAV. The sampler weighs about 5.9 kg and allows for continuous sampling for up to 35 min.
The sampler air intake is mounted at 70 cm above the center of gravity of the UAV, placed near a sonic anemometer (below) for ensuring sampling of the same air mass as where wind speed is measured. The time stamp of the mixing-ratio observation was corrected for the short time lag of 4 s between sampling at the air intake and at the thin-walled stainless-steel tubing attributable to the length of the Teflon inlet tube. Shortly before every flight, the pump is remotely turned on to sample the CO2 marker for 5 s and then to collect air samples. The CO2 markers subsequently help in data extraction and analysis to identify the starting point and specific times during the UAV air sampling . During flight, the pump would alternatively sample the marker and the ambient air on a preset timing schedule. The sampling flow rate remains at 18 sccm during the entire flight, controlled with the micro-orifice which is placed between the pump and the coiled tubing. After landing, the pump is remotely turned off and the air sample in the sampling tubing is immediately analyzed with a cavity ring-down spectrometer (CRDS) (Picarro, Inc., CA, USA, model G2401) for CO2 and CH4 mixing ratios in the sampled air. Waiting longer would lead to unwanted mixing of the samples in the tubing. The air samples enter the tubing from the air inlet during sampling and leave the tubing from a different air outlet during later analysis. As a result, the samples at the beginning of the flight spend the same amount of time within the tubing as those at the end of the flight. Using the embedded CO2 marker data, the CO2 and CH4 data series can be mapped to the sampling times and GPS locations during flight.
2.2 The 3-D sonic anemometer
Previous studies that applied UAV platforms for GHG monitoring generally relied on wind data from nearby ground weather stations (Morales et al., 2022; Allen et al., 2019). However, Gålfalk et al. (2021) show that wind speeds were inconsistent between a ground weather station at a 1.5 m height and an anemometer mounted on their UAV, especially when altitude increases, showing the need to have an onboard weather station for accurate flux calculations. In the present study, in order to obtain meteorological data along the flight track, a 3-D sonic anemometer (Geotech Inc, Denver, USA, model TriSonica Mini) is attached on the top of the UAV via a 450 mm carbon fiber pole. The anemometer measures three-component wind speed (Ux, Uy, w) and temperature (T). The measured data were further transformed into actual wind speeds and wind directions after corrections for UAV attitude (pitch, yaw, roll) changes and accounting for its airspeed, as well as the perturbations caused by the UAV rotor propellers using a patented correction algorithm. The GPS information, airspeed and attitude data (pitch, yaw, and roll) were extracted from the UAV data transmitted to the ground control station. The anemometer measures wind speeds within the range of 0 to 50 m s−1, with an accuracy of ±0.1 m s−1 below the wind speed of 10 m s−1. The accuracy for wind direction measurement is ±1∘. For temperature measurement, the operating range for the anemometer is between −40 and 85 ∘C and the accuracy is ±2 ∘C.
For anemometers mounted on multi-rotor UAVs, how to correct for the effects of the translational and rotational movements of the UAVs as well as the flows induced by the rotors to obtain accurate wind data is an ongoing research topic (Gålfalk et al., 2021; Wolf et al., 2017; De Boisblanc et al., 2014; Palomaki et al., 2017; Zhou et al., 2018). During flight, rotary wing UAVs create thrust by drawing air from above the rotors and expelling it downwards at a higher velocity. Such flows may extend to the anemometer position in addition to true atmospheric airflows, masking the true wind signals in the data from the anemometer (Wolf et al., 2017). Previous studies have conducted laboratory testing (Wolf et al., 2017; De Boisblanc et al., 2014; Palomaki et al., 2017) or flow field simulation (Zhou et al., 2018) to determine the appropriate distance to place anemometers onto multi-rotor UAVs to minimize the impact from the rotor-induced airflows. The anemometer in this research is mounted at an upward distance of 70 cm from the center of gravity of the UAV. A full digital model of the UAV, the anemometer and its mounting frame, and the air sampler was created. Using this digital model, computational fluid dynamics (CFD) simulations were performed to quantify wind speed disturbances caused by the UAV's rotor propellers to the anemometer during flight under a vast array of different wind conditions. An overall correction algorithm was developed in which parameters for propeller disturbances determined based on the CFD simulations were included along with correction schemes for false signals resulting from translational motions and changes in UAV pitch, roll and yaw.
2.3 The UAV
The air sampler and the anemometer are mounted on a hexacopter UAV (KWT-X6L-15). The UAV has a maximum flight time of ∼ 30 min at a maximum payload of 15 kg or longer with a lighter payload. Such flight endurance and carrying capacity meet our needs for loading the air sampler and the anemometer onto the UAV to realize emission quantification. The UAV is capable of flying in wind speeds of up to 14.4 m s−1 to an altitude of about 4000 m and has a maximum horizontal flying speed of 18 m s−1, a maximum ascending speed of 4 m s−1 and a maximum descending speed of 3 m s−1. The horizontal hovering precision of the GPS on the UAV is ±2 m, and the vertical hovering precision is ±1.5 m.
2.4 Air sample analysis
After landing, the air sample collected in the tubing is immediately analyzed with the CRDS analyzer. The withdrawal flow rate of the air from the sample tubing during analysis is an important parameter in optimizing the results. High withdrawal rates lead to unwanted mixing in the cavity of the analyzer. However, direct withdrawal of air from the sample tubing by the analyzer at a flow rate as low as the sampling flow rate of 18 sccm results in smoothing of concentrations from the inner-wall surface drag and desorption inside the tubing. We optimized the flow rate of the air from the sample tubing into the CRDS analyzer at ∼ 54 sccm, 3 times the sampling flow rate, by diluting the air sample with zero air, with two mass flow controllers separately controlling the flow rate of zero air and the withdrawal rate of the air sample (Fig. 2b).
2.5 Mass-balance approaches for determining emission rates
The UAV-based measurements were coupled with the mass-balance approach TERRA to determine the emission rates of the measured pollutants using their measured mixing ratios and the meteorological data (three-component wind speed (Ux, Uy, w) and temperature (T)) collected on board the UAV during the flight. TERRA computes integrated mass fluxes through airborne virtual-box/screen measurements, including those made from aircraft and in this case UAVs. TERRA has been used successfully and extensively for emission rate determination of tens of volatile organic compounds (Li et al., 2017), CO2 (Liggio et al., 2019), CH4 (Baray et al., 2018), oxidized sulfur and nitrogen (Hayden et al., 2021), black carbon (Cheng et al., 2020), and secondary organic aerosol (Liggio et al., 2016) using aircraft measurements. To run TERRA based on a virtual-box flight, the first step is to map the CH4 and CO2 mixing-ratio data measured along the level flight tracks encircling a facility to the 2-D virtual walls of the virtual box, created from stacking the level flight tracks, that surrounds the facility. The 2-D virtual walls (or screens) are derived from the unwrapping of the virtual box to assist the presentation of the CH4 and CO2 plumes along the flight tracks, with the horizontal path length (i.e., the ground line projection of the fitted flight track) and altitude as the two dimensions. The start of the horizontal path is typically defined as the southeast corner of the virtual box, but the selection of this starting position has no effect on the emission rate computation, and the horizontal path distance increases in a counter-clockwise direction. This procedure results in a translation of each flight position point from a 3-D position of latitude (y), longitude (x) and altitude (z, meters above mean sea level) to a 2-D screen position of horizontal path distance . Subsequently, TERRA applies a simple kriging algorithm to interpolate the data and finally produces a mesh on the 2-D virtual box walls whose resolution can be set depending on applications. The kriging weights were obtained with an isotropic spherical semivariogram model. In TERRA, the nugget, sill and range can all be modified to fit the semivariogram model. The mixing ratios of both CH4 and CO2 are extrapolated from the lowest flight altitudes to the ground digital elevation using one of several methods or a combination thereof, namely (1) assuming a constant, (2) linear extrapolation between a constant and background, (3) a background value below flight altitudes, (4) a linear fit between the lowest flight altitude and zero at the ground, and (5) an exponential fit from the lower flight altitudes (Gordon et al., 2015). Concurrently measured wind speed from the UAV is decomposed into northerly and easterly components (UN(s,z), UE(s,z)) based on the wind direction and is similarly interpolated onto the 1 m × 2 m mesh. The decomposed wind speeds are further extrapolated to the ground digital elevation using a log profile fit (Gordon et al., 2015). Based on the interpolated/extrapolated CH4 and CO2 mixing ratio, temperature, pressure (calculated using the barometric height formula), and wind speeds, TERRA computes the fluxes of CH4 and CO2 through the virtual walls and finally their facility emission rates by integrating the fluxes.
To summarize, in TERRA the mass balance in computing the emissions within a control box for a given inert pollutant such as CH4 or CO2 is presented by
where EC is the emission rate, EC,H is the horizontal advective transfer rate through the box walls, EC,V is the advective transfer rate through the box top and EC,M is the increase in mass within the volume due to a change in air density. Other terms listed in the Gordon et al. (2015) computation algorithm that were used to solve for the total emission rate were often neglected as they contribute little to the total emission rates. Each term from Eq. (1) is estimated as
where MR is the ratio of the compound molar mass to the molar mass of air, XC(s,z) is the mixing ratio of the compound in question, ρair(s,z) is the air density, w is the vertical wind velocity at the box top, XC,Top is the mixing ratio at the top of the box, and is the horizontal wind vector normal to the flight track calculated from the northerly and easterly components (UE(s,z), UN(s,z)):
The vertical transfer rate term EC,V is estimated by computing the air mass vertical transfer rate, determined from air mass balance within the box, and multiplying it with the CO2 or CH4 mixing ratios at the box top. This term is normally negligible in other top-down emission estimate approaches since it is typically minuscule compared to horizontal fluxes, but it can affect the computed emission rates when vertical air movement becomes more significant, such as under unstable atmospheric conditions. EC,M is often ignored in other mass-balance approaches; in TERRA it is estimated by taking the time derivative of the ideal gas law in temperature and pressure during the flight time, and typically it does not change significantly over the duration of 30 min or so for the UAV flight.
To suit the UAV measurements, the following modifications to the TERRA algorithm were made: (1) a much higher interpolation resolution for the kriging mesh was implemented for application to the UAV measurements in this study, with the interpolation mesh size adjusted to 1 m (vertical) by 2 m (horizontal), as UAVs fly significantly shorter distances compared to applications to piloted aircraft for which the interpolation resolution was 20 m (vertical) by 40 m (horizontal); (2) the modified TERRA now applies an embedded routine to automatically fit flight tracks using least squares, while this procedure was previously conducted manually offline through an geographic information system when using TERRA; and (3) the modified version of TERRA has added an algorithm for correcting negative weights during kriging interpolation following Deutsch (1995). TERRA was updated at Peking University, was recoded using the Python language, and runs under a browser–server environment with a new graphical user interface (GUI) and new interactive data flow.
3.1 Validation of the air sampler
Prior to flights in the field, we validated the air sampler in laboratory experiments by first sampling artificial air while making simultaneous online measurements of the artificial air with the CRDS analyzer, then analyzing the sampled artificial air with the same CRDS analyzer and finally comparing the results from the air sampler to the online measurements. An experimental apparatus was constructed for the simultaneous sampling of the same artificial air with the air sampler and the CRDS analyzer through a tee junction (Fig. 2a) and for subsequent air sample analysis using the same CRDS analyzer (Fig. 2b). In the artificial air, CH4 and CO2 standards were control-released into the lab air from an 8 L gas cylinder filled with a gas mixture of 5 ppm CH4, 2 ppm CO and 600 ppm CO2 to generate the artificial air source. The outlet of the standard gas cylinder was held at varying distances to the tee junction over time to yield a time series of different CH4 and CO2 mixing ratios, which were designed to mimic plumes expected in the real atmosphere. During analysis, the flow rate through the zero air (Mass Flow Controller 1) is adjusted to make sure that the flow rate through the air sampler (Mass Flow Controller 2) is stable and consistent at 54 sccm (Sect. 2.4).
Figure 4a illustrates the mixing ratios of CO2 and CH4 time series obtained from the air sampler and online measurements by the CRDS analyzer. It can be seen that the measured results from the air sampler and the online CRDS analyzer measurements are in good agreement throughout the tests, and the correlation coefficient is estimated to be 0.89 and 0.73 for CH4 and CO2 (Fig. 4c and f). For the measurements with the air sampler, short-term variations and noise in the CH4 and CO2 mixing ratios, which were fully captured by the CRDS analyzer during the online measurements, were smoothed out, while the main features and tendencies were preserved. In fact, the air sampler measurement results should be a smoothed version of the CRDS analyzer online measurements due to mixing in the analyzer cavity, molecular diffusion during sample storage in the sampler, inner-wall surface drag and desorption during the sample's withdrawal from the tubing during analysis, as well as Taylor dispersion during sampling and analysis (Karion et al., 2010). Dilution with zero air during later CRDS analysis also contributes to the smoothing.
3.2 Data deconvolution to achieve high time resolution
While it is impractical to delineate the individual smoothing effects when the air sample passes through the coupled system of the sampler plus the analysis setup as described above, the measured concentration y(t) can be treated as a result of the convolution of the air concentration before sampling x(t) and of a smoothing kernel g(i) consisting of a series of weights, which are inherently determined by factors including the sampler properties (tubing length, inner diameter, temperature, absorptive properties, flow rates), storage time, dilution and mixing in the cavity of the instrument. The smoothing can be described as
or expressed as a convolution of the form
where y(t) is the measured concentration at time t; x(t) the air concentration; and n(t) the unknown noise, assumed to be independent of x(t). The kernel g(i) contains non-zero kernel weight terms (). When all four terms in Eq. (7a) undergo Fourier transform, Eq. (7a) can be expressed in the frequency domain as follows:
In order to characterize the kernel weights g(i), a second lab experiment was conducted during which the sampler first sampled zero air for some time and then sampled the CO2 and CH4 standards for 1 s, before returning to sampling zero air again, creating an original concentration pulse signal in the x(t):
where j is the jth second when the sampler collected the standard of a known concentration C. This air sample was then analyzed with the CRDS as described above. After sampling, storing and analyzing, smoothing of the original concentration pulse leads to the concentration signal output Y(t) as follows:
where y(t) is the measured concentrations from the air sampler after sampling the concentration pulse and is non-zero when , with the index i taking the values from r to s. The noise n(t) term is zero for and can be assumed to have similar behavior for . Therefore,
The second lab experiment showed that y(t), and therefore the kernel g(t), consists of 70 non-zero values. To remove the noise n(t), g(t) is further smoothed using a box-car running mean of five terms:
It could be seen from Fig. 3 that has an asymmetrical distribution with a right-trailing tail and a half-height width of approximately 20 s for CO2 and 21 s for CH4, indicating that the smoothing had significantly reduced the sampling/analysis method time resolution to about 20 s from the 1 s resolution of the original pulse in the air concentration. The kernel shows that the influence that the neighboring points have on a given point decreases with increases in the gap between the two points.
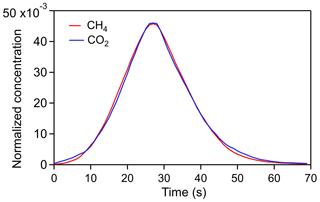
Figure 3The output of the 1 s signal after sampling, storing and analyzing using the air sampler for CO2 and CH4, normalized by their respective concentrations in the standard. As shown in the text, these curves are the actual kernel weights of .
To test whether the kernel weights can smooth the online measured concentrations from the first lab experiment (top line in Fig. 4a and b), the weights were used to convolute with the data from the online measurements (i.e., x(t)), resulting in an estimated (Fig. 4a and b, third line) that is in excellent agreement with the measurements from the air sampler, with the correlation coefficients increased to 0.99 and 0.98 for CH4 and CO2 (Fig. 4d and g).
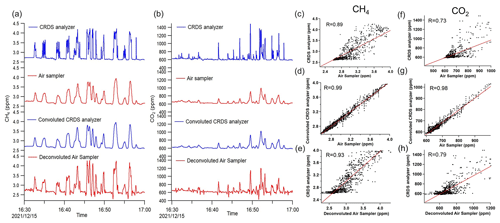
Figure 4(a, b) Mixing ratios of CO2 and CH4 measurements by online measurements with CRDS (the first line) and the air sampler (the second line) in laboratory tests. The third line represents the smoothed CRDS data after convolution with the kernel , and the fourth line represents the deconvoluted series after Wiener deconvolution. The signals of the same color represent the original signals and the corresponding signals after convolution or deconvolution. The date format is year/month/day, and the time is local time. (c–e) Correlation plots of CH4. (f–h) Correlation plots of CO2.
The ultimate goal of determining in Fig. 3 is to deconvolve y(t) from the air sampler to obtain the original concentration series x(t) using a number of deconvolution techniques. In the present study, we used the deconvolution method based on the Wiener theorem (Lin and Jin, 2013). The theorem provides the Wiener convolution filter h(t) so that x(t) can be estimated as follows:
where y(t) is the measured concentration, and an estimate of x(t). In the frequency domain, Eq. (12) may be rewritten as a product of two scalars:
where , H(f) and Y(f) are the Fourier transforms of , h(t) and y(t), respectively. The Wiener convolution filter h(t) is derived from the minimization of the mean square error:
with E denoting the expectation. When Eqs. (7b) and (13) are substituted into Eq. (14) and the quadratic is expanded, the mean square error ϵ(f) can be differentiated with respect to H(f) and the derivative is set to zero to achieve the minimization; under the assumption that the noise N(f) is independent of X(f), H(f) is derived as
where G(f) is the Fourier transform of derived from the second lab experiment described above S(f)=E|X(f)|2 and N(f)=E|N(f)|2 are the mean power spectral densities of the original concentration series x(t) and the noise n(t), respectively. Equation (15) could be rewritten as
where is the signal-to-noise ratio.
Substituting Eq. (16) into Eq. (13), , the Fourier transform of , is derived. The deconvolution is completed with the inverse Fourier transform of to give , the estimated air concentrations. The deconvolved series of CH4 and CO2 restored with the Wiener convolution filter are shown in Fig. 4a and b, and the correlation coefficients between the deconvoluted results and the online measurements with the CRDS analyzer are 0.93 and 0.79 for CH4 and CO2 (Fig. 4e and h), higher than those between the original air sampler measurement and the CRDS analyzer. These results indicate the effectiveness of the Wiener theorem in deconvolving a smoothed series to a much higher time resolution while accounting for noise. The restored series is improved in terms of time resolution, from about 20 s mentioned above to about 3–4 s after the deconvolution. The lab test data from the online measurements contain strong high-frequency components, artificially manipulated to provide an extreme case for testing the deconvolution algorithm. Such high frequencies lead to some residual noise in the deconvolved results, primarily as a result of choosing the cutoff frequencies for the mean power spectral densities S(f) and N(f). Nevertheless, such a situation will be improved for sampling in the real atmosphere where sub-second high-frequency variations are not common.
To apply the UAV-based measurement system described above to atmospheric measurements of CO2 and CH4, flights were made at the Shagang Group located in Jiangsu, China, on 28 December 2021. The Shagang Group is a major iron and steel company on the south shore of the Yangtze River (31.9704∘ N, 120.6443∘ E). The company produces over 40×106 t of steel each year, making it one of China's top five steel producers. On-site coke making for iron production is located in the western part of the Shagang steel complex. The coke-making process is to dry distill coal in a coking oven at ∼ 1000 ∘C temperature to boil off volatile components to form coke (metallic coal). During coke production, combustion of coking oven gas, blast furnace gas from steel making and coal tar plus light oil for heating the coking oven are the main CO2 and CH4 emission sources.
Two coking plant stacks were chosen as the target emission source for the field UAV flight. During flight, the UAV was flown in a rectangular pattern (200 m × 500 m) that encloses the two stacks, with repeated flight tracks at nine altitude levels that, when stacked, created a virtual box and intercepted the emitted CO2 and CH4 plumes on the downwind side of the box. The UAV ascended from the ground to 135 m a.g.l. and started the box flight at this altitude, ascending 15 m every level and reaching a maximum altitude of 255 m a.g.l. before landing. The UAV maintained a constant horizontal speed of 8 m s−1 during flight. The flight lasted for approximately 30 min. It is assumed that the plume remains steady during the time of measurement. After landing, the air sample collected in the sampler was immediately analyzed with the CRDS analyzer as per the procedure depicted above in Fig. 2b.
5.1 CH4 and CO2 mixing-ratio enhancement from the coking plant
Figure 5a shows the time series of CH4 and CO2 mixing ratios measured with the air sampler at the coking plant during the flight (red line). The air sampler sampled for a total of 30 min during the flight. After landing, the air sample was analyzed for 10 min, as the analysis flow rate triples the sampling flow rate (54.0 sccm vs. 18.0 sccm). The timescales of instrument readings were then stretched three times to restore the original timescales. The CH4 and CO2 time series were then deconvolved using the convolution kernel obtained from laboratory test (Sect. 3.2) to restore the mixing-ratio time series in air (black line). The meteorological parameters during the time of flight were measured by the 3-D anemometer, showing consistent southwesterly winds (Fig. 5b). The average wind speed is 4.7±4.9 m s−1, and the average wind direction is 216.4±38.4∘ during the time of flight. Consistency of wind measurements can be seen from the two wind rose plots for the northern wall and the southern wall, respectively. During the flight, the maximum mixing ratio measured was 5.6 ppm for CH4 and 1356 ppm for CO2. During the 30 min flight, a total of five CO2 makers were generated during the 30 min of sampling (Fig. 5a), and the decreases in the marker concentrations are corrected with a Gaussian form function.
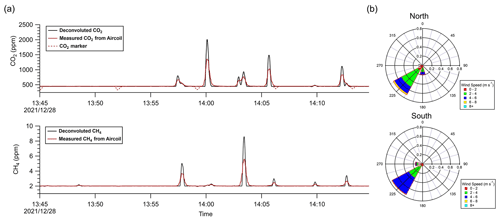
Figure 5(a) The red line represents CH4 and CO2 mixing ratios measured from the air samples collected with the air sampler during the flight at the coking plant. The black line represents the deconvolved CH4 and CO2 time series, and the dashed-red-line sections represent the original marker CO2 concentrations every 7 min. (b) Wind rose plots for the northern and southern wall based on the onboard meteorological measurements during the flight.
5.2 Emission estimation
The CO2 and CH4 emission rates for the stacks from the coking plant were estimated by applying a version of the computation algorithm TERRA specifically modified to suit UAV measurements. The deconvolved mixing-ratio time series of CO2 and CH4 were used in the TERRA algorithm. The algorithm first maps the mixing ratios to the walls of the virtual box, then applies a kriging scheme to interpolate the data and produces a 2 m (vertical) by 1 m (horizontal) mesh on the virtual box walls (200 m × 500 m) (Fig. 6). The semivariogram of the flight points was fitted with a spherical model (range = 300, sill = 3, nugget = 0). Wind speed and wind direction are first decomposed into northerly and easterly components and then further converted to vectors that are normal to and parallel to the walls of the virtual box before kriging. Background CH4 and CO2 were determined using upwind measurements. The background between upwind data was linearly interpolated and box-car-smoothed within a 3–4 min moving window to derive a variable baseline CH4 and CO2 for the entire 30 min flight. As shown in Fig. 6, the CH4 and CO2 plumes can be seen at different locations on the downwind side of the box wall, which indicates that the CH4 plume and the CO2 plume probably came from different sources within the box. Using the modified version of TERRA, the emission rates for the two stacks in the coking plant were calculated to be 0.12±0.01 t h−1 for CH4 and 110±20 t h−1 for CO2. The uncertainties for the estimates were derived from detailed analyses of each uncertainty source including measurement error in the mixing ratio and wind speed, the near-surface wind extrapolation, the near-surface mixing-ratio extrapolation, the box-top mixing ratio, the box-top height, and deconvolution.
5.3 Uncertainty analysis
To determine the overall uncertainty in the emission rates, each source of uncertainty contributing to the overall uncertainty needs to be identified and quantified. For the emission rate quantification from UAV measurement, the sources of uncertainties include the following: measurement uncertainties in the mixing ratios and wind speeds (δM), the near-surface wind extrapolation (δWind), the near-surface mixing-ratio extrapolation (δEx), the box-top mixing ratio (δTop), box-top height (δBH), and uncertainties due to data deconvolution as shown in the main text (δDeconv). Each uncertainty is treated as an independent estimate, and all uncertainties are propagated in quadrature to determine the overall uncertainty in the estimated emission rate:
The accuracy of the mixing-ratio measurements from the Picarro CRDS analyzer is 50 and 1 ppb for CO2 and CH4, respectively. By adding variations in the measured mixing ratios based on the measurement accuracies and re-applying TERRA, the derived emission rates varied within 1 % for both CO2 and CH4. Thus, the uncertainties in the emission rates due to mixing-ratio measurements (δM) were estimated at 1 % for both CH4 and CO2.
The anemometer measures wind speeds with an accuracy of ±0.1 m s−1 at wind speeds < 10 m s−1 and wind directions with an accuracy of ±1∘. The uncertainty in the wind measurements (δWind) was estimated using error propagation in the normal wind , as it is calculated from the northerly and easterly wind components and thus from wind speed (WS) and wind direction (WD):
Using this calculation, the uncertainty in the normal wind was derived at each location. The uncertainty that contributed to the total emission rates was examined by setting the normal wind to its upper and lower bounds defined by its uncertainty range and followed by computing the emission rates using TERRA. The derived CH4 and CO2 emission rates varied by 1.5 % and 1.9 %, respectively. Hence the uncertainties from wind speed measurements (δWind) were conservatively estimated to be 2 % for both CH4 and CO2.
Due to a lack of near-surface measurements along the box walls, extrapolation of CH4 and mixing ratios from the lowest flight path (∼ 150 m above ground level) to the ground level has been shown to be a source of potentially large uncertainty within TERRA. The magnitude of the uncertainty depends on the nature of the emissions; for example, surface emissions which may not be fully captured by the flight altitude range have higher uncertainties at ≈ 20 %, whereas elevated stack emissions which are fully captured by the flight altitude range lead to much smaller uncertainties of < 4 % in the emission estimates (Gordon et al., 2015). In the present study, to estimate uncertainties due to extrapolating mixing ratios from the lowest flight track to the ground (δEx), results from all extrapolation techniques (i.e., linear to the ground, constant value to the ground, linear to background value, or some combination of methods) were derived and compared with the result using a background value below flight altitudes (Table 1). Therefore, this term of uncertainty was evaluated at 2 % and 6 % for CH4 and CO2, respectively.
Additional components contributing to uncertainties in the computed emission rates specific to the box approach include the box-top mixing ratio (δTop) and box-top height (δBH). The TERRA box approach assumes a constant mixing ratio at the box top (XC,Top) by averaging the measured value at the top level. The term δTop is determined from the 95 % confidence interval () of the interpolated measurements. The calculated confidence interval of the mixing ratio at the box top is 0.01±0.13 ppm for CH4 and 70.1±89.1 ppm for CO2. Top average mixing ratios of 0.14 ppm for CH4 and 159.2 ppm for CO2 are set as input parameters to derive resulting uncertainties in the emissions rates. Thus, 106.6 kg h−1 for CH4 and 93 760 kg h−1 for CO2 were derived. Then, this uncertainty term is conservatively taken as 8 % and 16 % for CH4 and CO2.
The uncertainty due to the choice of box height, δBH, within TERRA is estimated by recomputing the emission rate with a reduced box height (z) of 100 m. The recalculated emission rate after reducing the box height of 100 m is 106.4 kg h−1 for CH4 and 113 500 kg h−1 for CO2; thus δBH is estimated as 8 % for CH4 and 3 % for CO2.
For cases that use the air sampling system instead of online measuring instruments, as the CH4 and CO2 time series measured from the air sampler were deconvoluted to restore the unsmoothed time series before being input into the TERRA algorithm, it is necessary to account for the uncertainty that comes from such deconvolution as outlined in the main text. Time series before and after deconvolution were applied to the TERRA algorithm to obtain the total emission rates. The computations show that emission rates before and after deconvolution vary within 1 %, which was taken as the uncertainty δDeconv. The assessment of uncertainties for the TERRA-computed emission rates from the coking plant is given in Table 2.
Table 2Assessment of percent uncertainties for CH4 and CO2 emission rate estimations. The sources of uncertainties include the following: measurement uncertainties in the mixing ratios and wind speeds (δM), the near-surface wind extrapolation (δWind), the near-surface mixing-ratio extrapolation (δEx), the box-top mixing ratio (δTop), box-top height (δBH), and uncertainties due to data deconvolution as shown in the main text (δDeconv).
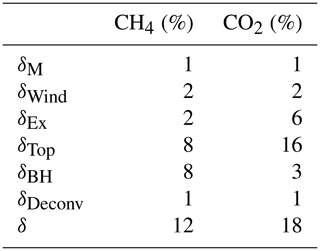
5.4 Comparison with Gaussian inversion approach
The TERRA computation results can be further evaluated. Of the multiple CH4 plumes that were captured on the north and east walls of the virtual box, the largest CH4 one resembles a nearly perfect Gaussian plume distribution and is clearly associated with the east stack of the two, for which the emission rate may be recalculated using the Gaussian plume model. The Gaussian plume model makes basic assumptions that the plume is emitted from a point source and that the atmospheric turbulence is constant in space and time (Visscher, 2014). In this study, the captured plume was completely elevated and thus not constrained by boundaries. In the absence of boundaries, the equation for pollutant mixing ratios in Gaussian plumes is as follows:
where c is the concentration at a given position x, y and z (g m−3); Q is the emission rate (g s−1); is the mean wind speed (m s−1); h is the effective source height (m); and σy and σz are dispersion parameters in the horizontal (lateral) and vertical directions, respectively (m).
The dispersion parameters σy and σz were obtained by fitting the spatial distribution of CH4 mixing ratios on the measurement screen into a Gaussian function. As the wall intercepting the plume is not perpendicular to the wind direction, the plume was projected to a different virtual wall perpendicular to the wind direction before fitting the Gaussian function. By calculating the standard deviations of the Gaussian distributions in the y and z directions, σz is estimated to be 6.3±0.3 m and σy is 15.7±0.4 m. The downwind measurement plane is examined to find the point with the highest CH4 mixing ratio of 6.575 ppm and its location (s=160 m, z=217 m). For the separate CH4 plume, the Gaussian plume model gives an emission rate of 40±6.8 kg h−1. The uncertainty is quantified by considering the accuracy of the mixing-ratio measurement, the variation in wind speed and the confidence interval for the dispersion parameters given by Gaussian function fitting. CH4 measurement uncertainties from the instrument comprise < 1 %. The uncertainty contributed by the mean wind speed estimation was examined by varying the average wind speed by the standard deviation of the wind data around the plume (3.8±0.6 m s−1), followed by input into Gaussian plume model. This mean wind speed sensitivity analysis resulted in CH4 emission rates that varied by 16 %. The same sensitivity analysis was done with σy (15.7±0.4 m) and σz (6.3±0.3 m), which resulted in CH4 emission rates that varied by 4 % and 3 %, respectively. Thus, the total uncertainty is added in quadrature to be 17 %. The TERRA algorithm is able to obtain the emission rate for a selected section through a certain area of the screen. For this isolated CH4 plume, the TERRA algorithm computed an emission rate of 65±8 kg h−1, which is comparable to the emission rate estimation from the Gaussian plume model.
5.5 Validation of UAV-based emissions and comparison with IPCC-based emissions
The coking process is one of the most energy-consuming operations during iron and steel production and tends to emit large amounts of CO2 and CH4. According to the Chinese national GHG inventory report, CO2 and CH4 emissions from coke production in iron and steel production processes were calculated using the Tier-1 method in the IPCC Guidelines (Ministry of Ecology and Environment of China, 2018). In the Tier-1 method, default emission factors for coke production are used to estimate the CO2 and CH4 emissions without considering local variations:
respectively, where and represent the CO2 and CH4 emission rates from coke production; Pcoke represents coke production; and and are the IPCC default emission factors for CO2 and CH4, which are 0.56 t CO2 t−1 of coke and 0.1 g CH4 t−1 of coke, respectively. The measured Shagang coking plant consists of two coke oven batteries, each with its own stack. Each battery produced 127.8 t coke h−1, thus totaling 255.6 t coke h−1 (Pcoke) between the two batteries during the UAV measurement period with a coke yield of 78.5 %. A material-balance analysis revealed that CO2 emitted from the stacks during the full coking process was 103±32 t CO2 h−1 (see the Supplement). In comparison, the UAV measurement-based emission rate obtained in this study is 110±18 t CO2 h−1, which is consistent with the CO2 emissions based on the material-balance analysis. For comparison, multiplying the IPCC default emission factor with the coke production at the Shagang coking plant yields an emission rate from coking of 143 t CO2 h−1, higher than both the material-balance-based result by about 39 % and the UAV-based result by 30 %. This suggests that the IPCC default emission factor is too high for this particular coking plant.
On the other hand, the UAV-measurement-based emission of 0.12±0.014 t h−1 for CH4 is 4 orders of magnitude higher than t h−1 emissions for CH4 estimated using the IPCC Tier-1 emission factor EF. The IPCC emission factor for coke production is derived by averaging plant-specific CH4 emissions data for 11 European coke plants reported in the IPPC I&S BAT document (European IPPC Bureau, 2001), but information about the data collection method such as sampling methods, analysis methods, time intervals, computation methods and reference conditions is not available according to the report. It is important to note that the present UAV measurement represents a one-time measurement where there was only one flight conducted in this campaign. The result clearly serves the purpose for validating the overall methodology from air sampling and analysis and computing the emission rates to estimating the associated errors. The fundamental assumption in the mass-balance approach is that plumes and emissions remain constant throughout the measurement period. Given the short duration of the flight and the good comparison between the present emission result and the material-balance emission estimate, such an assumption appears to be valid. However, a hypothesis of a constant emission rate over time remains to be tested. Conducting multiple flights over time, computing emission rates and assessing their uncertainties will allow for statistical sampling of the probability distribution of the emission rates and hence deriving the mathematical expectation of the emission rate. Only then can the derived emission factors be used for inventory preparation and/or comparison with existing ones with statistical confidence. Given the limited circumstance of having only one flight in this study, it becomes clear such a purpose cannot be achieved. Consequently, the emission values of CH4 derived from measurements in this section are only suitable for qualitative comparisons with published emission factors. The comparison results indicate that real-world emission factors may significantly differ from the default emission factors, but more work is needed. The additional CH4 may come from the leakage of the coke oven gas when it is recycled as fuel in firing the coke oven (see the Supplement). Both reasons point to a need for further emission measurements to determine the local emission factors and a further validation of the CH4 emission factors of coke production.
In this paper, we present the development of a UAV measurement system for quantifying GHG emissions at facility levels. The key element of this system is a newly designed air sampler, consisting of a 150 m long thin-walled stainless-steel tube with remote-controlled time stamping. Through laboratory testing, we found that the air sampler generated smoothed time series data compared to online measurement by the CRDS analyzer. To address the smoothing effect, we developed a deconvolution algorithm to restore the resolution of the time series obtained by the air sampler. For field validation, the new UAV measurement system was deployed to obtain CO2 and CH4 emissions from the main coking plant of the Shagang Group. Mixing ratios of CO2 and CH4 together with meteorological parameters were measured during the test flight. The mass-balance algorithm TERRA was used to estimate the coking plant CO2 and CH4 emission rates based on the UAV-measured data. For further analysis, we compared these emission results with those derived using Gaussian plume inversion approach and carbon material-balance methods, demonstrating good consistency among different approaches. In addition, when compared the top-down UAV-based measurement results to those derived from the bottom-up emission inventory method, the present findings indicate that the IPCC emission factors can be significantly different from the actual emission factors.
Data are available upon request to the corresponding author Shao-Meng Li (shaomeng.li@pku.edu.cn).
The supplement related to this article is available online at: https://doi.org/10.5194/amt-17-677-2024-supplement.
TH, CX, YL and SML conducted the fieldwork with support from XG, XZ and FB. TH and CX conducted laboratory experiments with guidance by SML. TH performed the primary data analysis and wrote the initial draft of the manuscript. YH provided expertise in model analysis. Algorithm programming was provided by YL. YY and YZ performed the wind data correction. SML reviewed and edited the manuscript and ensured the accuracy and integrity of the study.
The contact author has declared that none of the authors has any competing interests.
Publisher’s note: Copernicus Publications remains neutral with regard to jurisdictional claims made in the text, published maps, institutional affiliations, or any other geographical representation in this paper. While Copernicus Publications makes every effort to include appropriate place names, the final responsibility lies with the authors.
The authors acknowledge financial support of the National Natural Science Foundation of China Creative Research Group Fund.
This research has been supported by the National Natural Science Foundation of China Creative Research Group Fund (grant no. 22221004).
This paper was edited by Christian Brümmer and reviewed by two anonymous referees.
Allen, G., Hollingsworth, P., Kabbabe, K., Pitt, J. R., Mead, M. I., Illingworth, S., Roberts, G., Bourn, M., Shallcross, D. E., and Percival, C. J.: The development and trial of an unmanned aerial system for the measurement of methane flux from landfill and greenhouse gas emission hotspots, Waste Management, 87, 883–892, https://doi.org/10.1016/j.wasman.2017.12.024, 2019.
Andersen, T., Scheeren, B., Peters, W., and Chen, H.: A UAV-based active AirCore system for measurements of greenhouse gases, Atmos. Meas. Tech., 11, 2683–2699, https://doi.org/10.5194/amt-11-2683-2018, 2018.
Andersen, T., Vinkovic, K., de Vries, M., Kers, B., Necki, J., Swolkien, J., Roiger, A., Peters, W., and Chen, H.: Quantifying methane emissions from coal mining ventilation shafts using an unmanned aerial vehicle (UAV)-based active AirCore system, Atmospheric Environment: X, 12, 100135, https://doi.org/10.1016/j.aeaoa.2021.100135, 2021.
Angeli, S. D., Gossler, S., Lichtenberg, S., Kass, G., Agrawal, A. K., Valerius, M., Kinzel, K. P., and Deutschmann, O.: Reduction of CO2 emission from off-gases of steel industry by dry reforming of methane, Angew. Chem. Int. Edit., 60, 11852–11857, https://doi.org/10.1002/anie.202100577, 2021.
Baray, S., Darlington, A., Gordon, M., Hayden, K. L., Leithead, A., Li, S.-M., Liu, P. S. K., Mittermeier, R. L., Moussa, S. G., O'Brien, J., Staebler, R., Wolde, M., Worthy, D., and McLaren, R.: Quantification of methane sources in the Athabasca Oil Sands Region of Alberta by aircraft mass balance, Atmos. Chem. Phys., 18, 7361–7378, https://doi.org/10.5194/acp-18-7361-2018, 2018.
Bel Hadj Ali, N., Abichou, T., and Green, R.: Comparing estimates of fugitive landfill methane emissions using inverse plume modeling obtained with Surface Emission Monitoring (SEM), Drone Emission Monitoring (DEM), and Downwind Plume Emission Monitoring (DWPEM), J. Air Waste Manage., 70, 410–424, https://doi.org/10.1080/10962247.2020.1728423, 2020.
Brantley, H. L., Thoma, E. D., Squier, W. C., Guven, B. B., and Lyon, D.: Assessment of methane emissions from oil and gas production pads using mobile measurements, Environ. Sci. Technol., 48, 14508–14515, https://doi.org/10.1021/es503070q, 2014.
Brownlow, R., Lowry, D., Thomas, R. M., Fisher, R. E., France, J. L., Cain, M., Richardson, T. S., Greatwood, C., Freer, J., Pyle, J. A., MacKenzie, A. R., and Nisbet, E. G.: Methane mole fraction and ä13C above and below the trade wind inversion at Ascension Island in air sampled by aerial robotics, Geophys. Res. Lett., 43, 11893–11902, https://doi.org/10.1002/2016gl071155, 2016.
Chang, C. C., Wang, J. L., Chang, C. Y., Liang, M. C., and Lin, M. R.: Development of a multicopter-carried whole air sampling apparatus and its applications in environmental studies, Chemosphere, 144, 484–492, https://doi.org/10.1016/j.chemosphere.2015.08.028, 2016.
Cheng, Y., Li, S. M., Liggio, J., Gordon, M., Darlington, A., Zheng, Q., Moran, M., Liu, P., and Wolde, M.: Top-Down Determination of Black Carbon Emissions from Oil Sand Facilities in Alberta, Canada Using Aircraft Measurements, Environ. Sci. Technol., 54, 412–418, https://doi.org/10.1021/acs.est.9b05522, 2020.
Chiba, T., Haga, Y., Inoue, M., Kiguchi, O., Nagayoshi, T., Madokoro, H., and Morino, I.: Measuring Regional Atmospheric CO2 Concentrations in the Lower Troposphere with a Non-Dispersive Infrared Analyzer Mounted on a UAV, Ogata Village, Akita, Japan, Atmosphere, 10, 487, https://doi.org/10.3390/atmos10090487, 2019.
De Boisblanc, I., Dodbele, N., Kussmann, L., Mukherji, R., Chestnut, D., Phelps, S., Lewin, G. C., and de Wekker, S.: Designing a hexacopter for the collection of atmospheric flow data, 2014 Systems and Information Engineering Design Symposium (SIEDS), Charlottesville, VA, USA, 25 April 2014, 147–152, https://doi.org/10.1109/SIEDS.2014.6829915, 2014.
Deutsch, C. V.: Correcting for negative weights in ordinary kriging, Comput. Geosci., 22, 765–773, https://doi.org/10.1016/0098-3004(96)00005-2, 1996.
Etminan, M., Myhre, G., Highwood, E., and Shine, K.: Radiative forcing of carbon dioxide, methane, and nitrous oxide: A significant revision of the methane radiative forcing, Geophys. Res. Lett., 43, 12614–612623, https://doi.org/10.1002/2016GL071930, 2016.
European IPPC Bureau: Integrated Pollution Prevention and Control (IPPC) Best Available Techniques Reference Document on the Production of Iron and Steel, https://doi.org/10.2791/97469, 2001.
Gålfalk, M., Nilsson Paledal, S., and Bastviken, D.: Sensitive Drone Mapping of Methane Emissions without the Need for Supplementary Ground-Based Measurements, ACS Earth Space Chem., 5, 2668–2676, https://doi.org/10.1021/acsearthspacechem.1c00106, 2021.
Golston, L., Aubut, N., Frish, M., Yang, S., Talbot, R., Gretencord, C., McSpiritt, J., and Zondlo, M.: Natural Gas Fugitive Leak Detection Using an Unmanned Aerial Vehicle: Localization and Quantification of Emission Rate, Atmosphere, 9, 333, https://doi.org/10.3390/atmos9090333, 2018.
Gordon, M., Li, S.-M., Staebler, R., Darlington, A., Hayden, K., O'Brien, J., and Wolde, M.: Determining air pollutant emission rates based on mass balance using airborne measurement data over the Alberta oil sands operations, Atmos. Meas. Tech., 8, 3745–3765, https://doi.org/10.5194/amt-8-3745-2015, 2015.
Hasanbeigi, A.: Steel Climate Impact – An International Benchmarking of Energy and CO2 Intensities, Global Efficiency Intelligence, Florida, United States, https://www.globalefficiencyintel.com/steel-climate-impact- international-benchmarking-energy-co2-intensities (last access: 25 January 2024), 2022.
Hayden, K., Li, S.-M., Makar, P., Liggio, J., Moussa, S. G., Akingunola, A., McLaren, R., Staebler, R. M., Darlington, A., O'Brien, J., Zhang, J., Wolde, M., and Zhang, L.: New methodology shows short atmospheric lifetimes of oxidized sulfur and nitrogen due to dry deposition, Atmos. Chem. Phys., 21, 8377–8392, https://doi.org/10.5194/acp-21-8377-2021, 2021.
Helfter, C., Tremper, A. H., Halios, C. H., Kotthaus, S., Bjorkegren, A., Grimmond, C. S. B., Barlow, J. F., and Nemitz, E.: Spatial and temporal variability of urban fluxes of methane, carbon monoxide and carbon dioxide above London, UK, Atmos. Chem. Phys., 16, 10543–10557, https://doi.org/10.5194/acp-16-10543-2016, 2016.
IPCC: 2006 IPCC Guidelines for National Greenhouse Gas Inventories, Chapter 4: Metal Industry Emissions, edited by: Eggleston, H. S., Institute for Global Environmental Strategies, Hayama, Japan, ISBN 978-4-88788-032-0, 2006.
IPCC: Climate Change 2021: The Physical Science Basis. Contribution of Working Group I to the Sixth Assessment Report of the Intergovernmental Panel on Climate Change, edited by: Masson-Delmotte, V., Zhai, P., Pirani, A., Connors, S. L., Péan, C., Berger, S., Caud, N., Chen, Y., Goldfarb, L., Gomis, M. I., Huang, M., Leitzell, K., Lonnoy, E., Matthews, J. B. R., Maycock, T. K., Waterfield, T., Yelekçi, O., Yu, R., and Zhou, B., Cambridge University Press, Cambridge, United Kingdom and New York, NY, USA, 2391 pp., https://doi.org/10.1017/9781009157896, 2021.
Karion, A., Sweeney, C., Tans, P., and Newberger, T.: AirCore: An innovative atmospheric sampling system, J. Atmos. Ocean. Tech., 27, 1839–1853, https://doi.org/10.1175/2010JTECHA1448.1, 2010.
Leitner, S., Feichtinger, W., Mayer, S., Mayer, F., Krompetz, D., Hood-Nowotny, R., and Watzinger, A.: UAV-based sampling systems to analyse greenhouse gases and volatile organic compounds encompassing compound-specific stable isotope analysis, Atmos. Meas. Tech., 16, 513–527, https://doi.org/10.5194/amt-16-513-2023, 2023.
Li, H. Z., Mundia-Howe, M., Reeder, M. D., and Pekney, N. J.: Gathering Pipeline Methane Emissions in Utica Shale Using an Unmanned Aerial Vehicle and Ground-Based Mobile Sampling, Atmosphere, 11, 716, https://doi.org/10.3390/atmos11070716, 2020.
Li, S. M., Leithead, A., Moussa, S. G., Liggio, J., Moran, M. D., Wang, D., Hayden, K., Darlington, A., Gordon, M., Staebler, R., Makar, P. A., Stroud, C. A., McLaren, R., Liu, P. S. K., O'Brien, J., Mittermeier, R. L., Zhang, J., Marson, G., Cober, S. G., Wolde, M., and Wentzell, J. J. B.: Differences between measured and reported volatile organic compound emissions from oil sands facilities in Alberta, Canada, P. Natl. Acad. Sci. USA, 114, E3756–E3765, https://doi.org/10.1073/pnas.1617862114, 2017.
Liggio, J., Li, S. M., Hayden, K., Taha, Y. M., Stroud, C., Darlington, A., Drollette, B. D., Gordon, M., Lee, P., Liu, P., Leithead, A., Moussa, S. G., Wang, D., O'Brien, J., Mittermeier, R. L., Brook, J. R., Lu, G., Staebler, R. M., Han, Y., Tokarek, T. W., Osthoff, H. D., Makar, P. A., Zhang, J., Plata, D. L., and Gentner, D. R.: Oil sands operations as a large source of secondary organic aerosols, Nature, 534, 91–94, https://doi.org/10.1038/nature17646, 2016.
Liggio, J., Li, S. M., Staebler, R. M., Hayden, K., Darlington, A., Mittermeier, R. L., O'Brien, J., McLaren, R., Wolde, M., Worthy, D., and Vogel, F.: Measured Canadian oil sands CO2 emissions are higher than estimates made using internationally recommended methods, Nat. Commun., 10, 1863, https://doi.org/10.1038/s41467-019-09714-9, 2019.
Lin, F. and Jin, C.: An improved Wiener deconvolution filter for high-resolution electron microscopy images, Micron, 50, 1–6, https://doi.org/10.1016/j.micron.2013.03.005, 2013.
Liu, Y., Paris, J.-D., Vrekoussis, M., Antoniou, P., Constantinides, C., Desservettaz, M., Keleshis, C., Laurent, O., Leonidou, A., Philippon, C., Vouterakos, P., Quéhé, P.-Y., Bousquet, P., and Sciare, J.: Improvements of a low-cost CO2 commercial nondispersive near-infrared (NDIR) sensor for unmanned aerial vehicle (UAV) atmospheric mapping applications, Atmos. Meas. Tech., 15, 4431–4442, https://doi.org/10.5194/amt-15-4431-2022, 2022.
Miller, S. M., Wofsy, S. C., Michalak, A. M., Kort, E. A., Andrews, A. E., Biraud, S. C., Dlugokencky, E. J., Eluszkiewicz, J., Fischer, M. L., Janssens-Maenhout, G., Miller, B. R., Miller, J. B., Montzka, S. A., Nehrkorn, T., and Sweeney, C.: Anthropogenic emissions of methane in the United States, P. Natl. Acad. Sci. USA, 110, 20018–20022, https://doi.org/10.1073/pnas.1314392110, 2013.
Ministry of Ecology and Environment of China: The People's Republic of China Second Biennial Update Report on Climate Change, Part II National Greenhouse Gas Inventory, Chapter 1.3 Industrial Process, https://unfccc.int/sites/default/files/resource/China%202BUR_English.pdf (last access: 25 January 2024), 2018.
Morales, R., Ravelid, J., Vinkovic, K., Korbeń, P., Tuzson, B., Emmenegger, L., Chen, H., Schmidt, M., Humbel, S., and Brunner, D.: Controlled-release experiment to investigate uncertainties in UAV-based emission quantification for methane point sources, Atmos. Meas. Tech., 15, 2177–2198, https://doi.org/10.5194/amt-15-2177-2022, 2022.
Nathan, B. J., Golston, L. M., O'Brien, A. S., Ross, K., Harrison, W. A., Tao, L., Lary, D. J., Johnson, D. R., Covington, A. N., Clark, N. N., and Zondlo, M. A.: Near-Field Characterization of Methane Emission Variability from a Compressor Station Using a Model Aircraft, Environ. Sci. Technol., 49, 7896–7903, https://doi.org/10.1021/acs.est.5b00705, 2015.
Palomaki, R. T., Rose, N. T., van den Bossche, M., Sherman, T. J., and De Wekker, S. F. J.: Wind Estimation in the Lower Atmosphere Using Multirotor Aircraft, J. Atmos. Ocean. Tech., 34, 1183–1191, https://doi.org/10.1175/jtech-d-16-0177.1, 2017.
Rella, C. W., Tsai, T. R., Botkin, C. G., Crosson, E. R., and Steele, D.: Measuring emissions from oil and natural gas well pads using the mobile flux plane technique, Environ. Sci. Technol., 49, 4742–4748, https://doi.org/10.1021/acs.est.5b00099, 2015.
Reuter, M., Bovensmann, H., Buchwitz, M., Borchardt, J., Krautwurst, S., Gerilowski, K., Lindauer, M., Kubistin, D., and Burrows, J. P.: Development of a small unmanned aircraft system to derive CO2 emissions of anthropogenic point sources, Atmos. Meas. Tech., 14, 153–172, https://doi.org/10.5194/amt-14-153-2021, 2021.
Saunois, M., Stavert, A. R., Poulter, B., Bousquet, P., Canadell, J. G., Jackson, R. B., Raymond, P. A., Dlugokencky, E. J., Houweling, S., Patra, P. K., Ciais, P., Arora, V. K., Bastviken, D., Bergamaschi, P., Blake, D. R., Brailsford, G., Bruhwiler, L., Carlson, K. M., Carrol, M., Castaldi, S., Chandra, N., Crevoisier, C., Crill, P. M., Covey, K., Curry, C. L., Etiope, G., Frankenberg, C., Gedney, N., Hegglin, M. I., Höglund-Isaksson, L., Hugelius, G., Ishizawa, M., Ito, A., Janssens-Maenhout, G., Jensen, K. M., Joos, F., Kleinen, T., Krummel, P. B., Langenfelds, R. L., Laruelle, G. G., Liu, L., Machida, T., Maksyutov, S., McDonald, K. C., McNorton, J., Miller, P. A., Melton, J. R., Morino, I., Müller, J., Murguia-Flores, F., Naik, V., Niwa, Y., Noce, S., O'Doherty, S., Parker, R. J., Peng, C., Peng, S., Peters, G. P., Prigent, C., Prinn, R., Ramonet, M., Regnier, P., Riley, W. J., Rosentreter, J. A., Segers, A., Simpson, I. J., Shi, H., Smith, S. J., Steele, L. P., Thornton, B. F., Tian, H., Tohjima, Y., Tubiello, F. N., Tsuruta, A., Viovy, N., Voulgarakis, A., Weber, T. S., van Weele, M., van der Werf, G. R., Weiss, R. F., Worthy, D., Wunch, D., Yin, Y., Yoshida, Y., Zhang, W., Zhang, Z., Zhao, Y., Zheng, B., Zhu, Q., Zhu, Q., and Zhuang, Q.: The Global Methane Budget 2000–2017, Earth Syst. Sci. Data, 12, 1561–1623, https://doi.org/10.5194/essd-12-1561-2020, 2020.
Shah, A., Ricketts, H., Pitt, J. R., Shaw, J. T., Kabbabe, K., Leen, J. B., and Allen, G.: Unmanned aerial vehicle observations of cold venting from exploratory hydraulic fracturing in the United Kingdom, Environmental Research Communications, 2, 021003, https://doi.org/10.1088/2515-7620/ab716d, 2020.
Shaw, J. T., Shah, A., Yong, H., and Allen, G.: Methods for quantifying methane emissions using unmanned aerial vehicles: a review, Philos. T. Roy. Soc. A, 379, 20200450, https://doi.org/10.1098/rsta.2020.0450, 2021.
Takano, T. and Ueyama, M.: Spatial variations in daytime methane and carbon dioxide emissions in two urban landscapes, Sakai, Japan, Urban Climate, 36, 100798, https://doi.org/10.1016/j.uclim.2021.100798, 2021.
Turner, A. J., Jacob, D. J., Wecht, K. J., Maasakkers, J. D., Lundgren, E., Andrews, A. E., Biraud, S. C., Boesch, H., Bowman, K. W., Deutscher, N. M., Dubey, M. K., Griffith, D. W. T., Hase, F., Kuze, A., Notholt, J., Ohyama, H., Parker, R., Payne, V. H., Sussmann, R., Sweeney, C., Velazco, V. A., Warneke, T., Wennberg, P. O., and Wunch, D.: Estimating global and North American methane emissions with high spatial resolution using GOSAT satellite data, Atmos. Chem. Phys., 15, 7049–7069, https://doi.org/10.5194/acp-15-7049-2015, 2015.
Turner, A. J., Frankenberg, C., and Kort, E. A.: Interpreting contemporary trends in atmospheric methane, P. Natl. Acad. Sci. USA, 116, 2805–2813, 10.1073/pnas.1814297116, 2019.
Tuzson, B., Morales, R., Graf, M., Scheidegger, P., Looser, H., Kupferschmid, A., and Emmenegger, L.: Bird's-eye View of Localized Methane Emission Sources: Highlights of Analytical Sciences in Switzerland, Chimia, 75, 802–802, https://doi.org/10.2533/chimia.2021.802, 2021.
USEPA: Inventory of U.S. Greenhouse Gas Emissions and Sinks: 1990–2014, US Environmental Protection Agency, Washington, DC, USA, https://www.epa.gov/sites/default/files/2017-04/documents/us-ghg-inventory-2016-main-text.pdf (last access: 25 January 2024), 2016.
Vinkovic, K., Andersen, T., de Vries, M., Kers, B., van Heuven, S., Peters, W., Hensen, A., van den Bulk, P., and Chen, H.: Evaluating the use of an Unmanned Aerial Vehicle (UAV)-based active AirCore system to quantify methane emissions from dairy cows, Sci. Total. Environ., 831, 154898, https://doi.org/10.1016/j.scitotenv.2022.154898, 2022.
Visscher, A. D.: Air Dispersion Modeling: Foundations and Applications, John Wiley & Sons, Inc., Hoboken, New Jersey, ISBN 978-1-118-07859-4, 2014.
Wolf, C. A., Hardis, R. P., Woodrum, S. D., Galan, R. S., Wichelt,H. S., Metzger, M. C., Bezzo, N., Lewin, G. C., and de Wekker, S. F.: Wind data collection techniques on a multi-rotor platform, 2017 Systems and Information Engineering Design Symposium (SIEDS), Charlottesville, VA, USA, 28 April 2017, 32–37, https://doi.org/10.1109/SIEDS.2017.7937739, 2017.
Zhou, S., Peng, S., Wang, M., Shen, A., and Liu, Z.: The Characteristics and Contributing Factors of Air Pollution in Nanjing: A Case Study Based on an Unmanned Aerial Vehicle Experiment and Multiple Datasets, Atmosphere, 9, 343, https://doi.org/10.3390/atmos9090343, 2018.