the Creative Commons Attribution 4.0 License.
the Creative Commons Attribution 4.0 License.
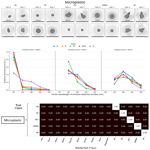
Merging holography, fluorescence, and machine learning for in situ continuous characterization and classification of airborne microplastics
Julia Burkart
Elias Graf
Yanick Zeder
Lea Ann Dailey
Bernadett Weinzierl
The continued increase in global plastic production and poor waste management ensures that plastic pollution will be a serious environmental concern for years to come. Because of their size, shape, and relatively low density, plastic particles between 1 and 1000 µm in size (known as microplastics or MPs) emitted directly into the environment (“primary”) or created due to degradation (“secondary”) may be transported through the atmosphere, similarly to other coarse-mode particles such as mineral dust. MPs can thus be advected over great distances, reaching even the most pristine and remote areas of Earth, and may have significant negative consequences for humans and the environment. The detection and analysis of MPs once airborne, however, remains a challenge because most observational methods are offline and resource-intensive and, therefore, not capable of providing continuous quantitative information.
In this study, we present results using an online in situ airflow cytometer (SwisensPoleno Jupiter; Swisens AG; Emmen, Switzerland) – coupled with machine learning – to detect, analyze, and classify airborne single-particle MPs in near real time. The performance of the instrument in differentiating between single-particle MPs of five common polymer types (including polypropylene, polyethylene, polyamide, poly(methyl methacrylate), and polyethylene terephthalate) was investigated under laboratory conditions using combined information about their size and shape (determined using holographic imaging) and fluorescence measured using three excitation wavelengths and five emission detection windows. The classification capability using these methods was determined alongside other coarse-mode aerosol particles with similar morphology or fluorescence characteristics, such as a mineral dust and several pollen taxa.
The tested MPs exhibit a measurable fluorescence signal that not only allows them to be distinguished from other fluorescent particles, such as pollen, but also differentiated from each other, with high (> 90 %) classification accuracy based on their multispectral fluorescence signatures. The classification accuracies of machine learning models using only holographic images of particles, only the fluorescence response, and combined information from holography and fluorescence to predict particle types are presented and compared. The last model, using both the holographic images and fluorescence information for each particle, was the most optimal model used, providing the highest classification accuracy compared to employing models using only the holography or fluorescence response separately. The results provide a foundation for significantly improving the understanding of the properties and types of MPs present in the atmosphere.
- Article
(6494 KB) - Full-text XML
-
Supplement
(1041 KB) - BibTeX
- EndNote
Plastics composed of synthetic or semi-synthetic polymer materials are ubiquitous in nearly all areas of contemporary society. From packaging to consumer products to roadway materials, plastics are utilized because of their low cost of production and material properties. Due to these factors and rising demands, plastic production has been increasing by approximately 8.4 % annually, where only ∼ 9 % of plastics are recycled, 12 % are incinerated, and the rest accumulate in landfills and in the environment (Geyer et al., 2017). In recent years, public awareness and concerns about plastic pollution as a global environmental crisis have been increasing (Davison et al., 2021), while, concurrently, the amount of plastic pollution in the environment more than doubled in the period from 2000 to 2019 (Agrawala et al., 2022).
Plastics may be introduced into the environment through their origin as “primary” particles, i.e., purposefully manufactured particles for specific applications, such as personal care products (Fendall and Sewell, 2009) or industrial abrasives and paints (Verschoor et al., 2016). Once in the environment, plastics may undergo physical (e.g., mechanical), radiative, chemical, and biological degradation, which alters their size, shape, and mobility within their environment (Brandon et al., 2016; Mao et al., 2020; Othman et al., 2021; Zhang et al., 2021). This degradation produces “secondary” fragments or particles. Primary or secondary particles are categorized into various size classes: macroplastics (> 1 cm), mesoplastics (between 1 and 10 mm), microplastics (referred to throughout this publication as MPs; 1–1000 µm), and nanoplastics (1–1000 nm) (Hartmann et al., 2019; International Organization for Standardization, 2023). While MPs have been a known source of contamination in aquatic ecosystems (Barnes et al., 2009; Cole et al., 2011; Cózar et al., 2014), an interest in research to better understand airborne particles has been on the rise (Beaurepaire et al., 2021; Brahney et al., 2021; Enyoh et al., 2019). Because of their size, shape, and material characteristics (such as their low density; Driedger et al., 2015), MPs and nanoplastics may be emitted into the atmosphere and transported long distances (Brahney et al., 2021), similar to other coarse-mode (maximum length > 1 µm) particles such as mineral dust (Schepanski, 2018; Weinzierl et al., 2017), reaching even the most pristine and remote areas of Earth (Aves et al., 2022; Bergmann et al., 2019; Brahney et al., 2020; Evangeliou et al., 2020). In addition, airborne MPs may cause significant health impacts if inhaled, as some particles can be in the respirable size range (Gasperi et al., 2018; Stuart, 1984), toxic (Prata et al., 2020), and bio-persistent (Mammo et al., 2020). Understanding of the health impacts of microplastic particles is still evolving, and knowing their concentration, size distribution, and polymer type is imperative for addressing this growing concern (Prata, 2018).
The atmosphere remains the least understood environmental compartment for the fate of MPs (Akdogan and Guven, 2019; Zhang et al., 2020). The ubiquity of MPs in the environment and this lack of understanding have created the need for reliable, fast, and quantitative analysis methods. In particular, significant progress in studying the impact of atmospheric MPs is hindered by the lack of analytical methods that can effectively characterize particles in situ and in the size range relevant to atmospheric transport. As particle size decreases, the time and effort required for identification of the plastic particles increase (Shim et al., 2017) and the size limits of detection for common, robust microplastic identification instruments are reached (such as 10–25 µm for FTIR and Raman spectroscopy) (Primpke et al., 2020). Additionally, most conventional methods of MP detection and/or characterization are offline (i.e., they do not measure continuously) and require tedious sample preparation (Primpke et al., 2020). Many standard analysis protocols are also limited in the information they can provide about the MPs. For example, some methods may be limited to only providing information connected to the chemical signature of the material being analyzed, while others – such as the popular methods utilizing optical microscopy – may only provide limited information about MP size and relative abundance (Primpke et al., 2020; Shim et al., 2017).
An often-overlooked material property of airborne microplastics that has the potential to specify the particle type is their natural ability to fluoresce, or autofluoresce, which results from the spontaneous emission of light at one wavelength by the fluorophores (a molecule or compound capable of fluorescence) of the polymers from excited electromagnetic states when exposed to higher-energy, lower-wavelength light (Lakowicz, 2006). For polymers, this can strictly be due to their molecular structure, containing aromatic rings, conjugated double bonds, or other fluorophores; stabilizers, additives, or impurities unintentionally added to the substance during the polymerization process or after production; or some combination thereof. Most studies examine extrinsic fluorescence of MPs, which is a method of applying a dye stain that adheres to plastics (Capolungo et al., 2021; Primpke et al., 2020) and only provides a means of distinguishing MPs from other non-fluorescing materials when viewed on filter media from an optical microscope (Erni-Cassola et al., 2017; Maes et al., 2017). This technique may be prone to misidentification (Beaurepaire et al., 2021) and, like other popular MP identification methods, is offline and labor-intensive. Some commercially available polymers were previously examined for their autofluorescence (Allen et al., 1976; Asfour et al., 2020; Hawkins and Yager, 2003; Könemann et al., 2018; Lionetto et al., 2022; Monteleone et al., 2021a, b; Ornik et al., 2020; Piruska et al., 2005; Spizzichino et al., 2016), but identification of polymer types using their autofluorescence has been limited. For example, Ornik et al. (2020) examined the fluorescence spectra of eight large commercially obtained polymer samples – including polypropylene, polyethylene, polyethylene terephthalate, and two polyamides – and demonstrated that their emission spectra are generally distinguishable from non-polymer samples. They acknowledged that these same principles can be applied to microplastics of various sizes and shapes while leveraging advanced analysis methods such as machine learning for high-accuracy classification.
One recent work has shown promising ability to classify airborne MPs using their autofluorescence (Gratzl et al., 2024). Here, Gratzl et al. (2024) leveraged the Wideband Integrated Bioaerosol Sensor (WIBS; Droplet Measurement Technologies, Longmont, CO, USA) to detect microplastics based on specific fluorescence signatures excited at two wavelengths and detected in two emission wavelength bands. While their approach provides a promising step towards greater understanding of MPs in the atmosphere, the recent introduction of the SwisensPoleno air-flow cytometer (Swisens AG; Emmen, Switzerland) was recently shown to classify biological aerosol particles with high accuracy (Erb et al., 2023, 2024; Sauvageat et al., 2020), expanding the spectral capabilities of the WIBS, and combines additional particle information to strengthen the classification ability of MPs and other atmospheric coarse-mode aerosols. The SwisensPoleno (Jupiter model) characterizes single particles by combining sensor information from digital holography from two orthogonal holographic imagers and steady-state spectrally resolved fluorescence intensity. The multi-method platform is complemented by state-of-the-art machine learning algorithms that provide classification of airborne particle types in near real time.
The objective of this study is to assess the fluorescence responses of various common microplastics and to identify whether this information, together with holographic images of the individual particles measured using the SwisensPoleno, can be used to distinguish MPs from other particle types. This fluorescence and the other measured parameters, such as the particle morphology, are compared to the data of other airborne, coarse-mode particles, including mineral dust, several taxa of pollen, and water droplets. These comparisons yield an estimation of viability for the online in situ detection and classification of airborne MPs using the SwisensPoleno's multi-sensor approach.
2.1 SwisensPoleno
The SwisensPoleno (Jupiter model, manufactured by Swisens AG; Emmen, Switzerland) is an air-flow cytometer providing continuous in situ characterization of single, coarse-mode aerosol particles using multiple measurement methods in a single instrument. It combines sensor information to characterize single particles using digital holography from two orthogonal holographic imagers and spectrally resolved fluorescence intensity measurements. In addition, the instrument provides a measurement of elastic forward and polarized side-scattering of each particle; for this study, however, we focus on using only the fluorescence and holographic imaging systems of the SwisensPoleno. The final component of this multi-method instrument is an integrated machine learning classification model, allowing the instrument to identify particle types in near real time by training models using all measured properties of individual airborne particles. According to the manufacturer, the instrument has an effective flow rate of 40 L min−1, and particles in the size range 0.5–300 µm can be detected in their multi-sensor system.
The SwisensPoleno resolves two digital holograms of the same single particle in the sample stream using digital in-line holography (Berg, 2022; Berg and Videen, 2011), with imaging sensors placed perpendicularly to each other and the imaging plane perpendicular to the sample flow. Using only holographic imaging and image analysis, the SwisensPoleno has been used to detect and classify pollen particles from several different plant species in the size range between 10 and 200 µm with high accuracy (Sauvageat et al., 2020), later adapted to identify fungal spores during ambient monitoring in Switzerland (Erb et al., 2023) and recently shown to be successful when combining holography and fluorescence information for pollen classification (Erb et al., 2024). After hologram reconstruction and processing, each particle image is 200 × 200 px, with a resolution of 0.595 µm px−1. For size and shape statistics, each holographic image is binarized and analyzed using the scikit-image software package (van der Walt et al., 2014) to determine a wide range of characteristic image properties (e.g., mean pixel intensity) and morphological features for each particle, including shape (e.g., eccentricity, solidity) and size (e.g., major and minor axis lengths, area-equivalent diameter).
For fluorescence measurements, the SwisensPoleno uses LEDs at 280 and 365 nm and a 405 nm laser diode for fluorescence excitation. The excitation sources are collimated (405 nm) or focused (280 and 365 nm) and filtered using bandpass filters to narrow their emission spectrum around their center wavelength. The wavebands for detecting fluorescence emissions are 333–381, 411–459, 465–501, 539–585, and 658–694 nm (referred to further using their center wavelengths 357, 435, 483, 562, and 676 nm). Note that the = and nm channels are not included in the SwisensPoleno measurement or analysis, because the fluorescence emission detection wavelengths are longer than the excitation wavelengths. Thus, the combination of excitation sources and measurement channels provides 13 viable measurements for each particle, which we will refer to using the notation of for each excitation and emission channel. The instrument's fluorescence system covers the excitation and emission range typical of bioaerosols (Pöhlker et al., 2012). Importantly, the SwisensPoleno does not differentiate between natural particles that are inherently autofluorescent, such as some bioaerosols and particles derived from synthetic materials such as microplastics. Further details of the SwisensPoleno fluorescence system can be found in the Supplement.
The integrated instrument software makes use of a machine learning classification model for real-time, single-particle classification using its holographic images. The model used for real-time or “live” particle classification during instrument deployment is developed, trained, and tested offline on particle types the user expects the instrument to encounter. The SwisensPoleno is shipped with a default model trained by MeteoSwiss with supervised learning on a subset of common central European pollen taxa and water droplets. However, users can train, evaluate, and update their instrument with a classification model prepared on other data. For this study, machine learning classification models were created, trained, and evaluated in a separate Python programming environment decoupled from the instrument. The details of the machine learning models used in this study are outlined in Sect. 2.4 and in the Supplement.
To create individual particle datasets for this study, the SwisensPoleno instrument inlet was coupled to a particle atomizer (SwisensAtomizer) – also manufactured by Swisens AG – that entrains solid, dry test particles into the sample flow of the instrument in laboratory or test environments. The atomizer uses a small (∼ 5 cm) acoustic speaker to apply mechanical vibrations of user-specified frequencies and amplitudes to a small volume of test particles (typically < 1.5 mL). The sample volume is physically in contact with the speaker, so that the acoustical vibrations are transferred directly to the test material inside the sample volume, sometimes inducing granular convection. Particles at the top of the volume are aerosolized because of this vibration, and a small amount of air is introduced into the sample volume to encourage the aerosolized particles to exit the sample volume and enter the sample stream of the SwisensPoleno instrument.
2.2 Materials and material preparation
A total of 15 particle types were analyzed using the SwisensPoleno instrument in a laboratory setting, assessing their fluorescence response and morphology through fluorescence spectroscopy and holographic imaging, respectively. An overview of the particles used in this study can be found in Table 1, including their class names, which are referred to throughout this work for simplicity. In addition, the total number of events (number of individual particles successfully detected with both holographic imaging and fluorescence) for each class are shown. The investigated particle types are categorized as “microplastic”, “pollen”, and “other”. The five microplastic particle types tested were polyamide 12 (PA), polyethylene (PE), polyethylene terephthalate (PET), poly(methyl methacrylate) (PMMA), and polypropylene (PP), which represent common polymers used in society and frequently found as microplastics in the environment (Koelmans et al., 2022; Plastics Europe AISBL, 2022; Zhang et al., 2020). All microplastic particles were commercially purchased and tested in the dry state. To the best of the authors' knowledge, the polymer samples used in this study are free from solvents, additives, or colorants. In addition to these MPs, the other particle types tested included Arizona Test Dust, a volcanic ash sample from Iceland, water droplets, glass reference microspheres, and pollen samples from six different taxa. Although not all particle types in this study are atmospherically relevant for ambient particle classification (e.g., glass microspheres), they were selected to represent a mixture of overlapping morphology, size, and/or fluorescence properties to assess the instrument's ability to differentiate between similarly featured aerosol particles.
Table 1Overview of the particles tested in this study and their properties.
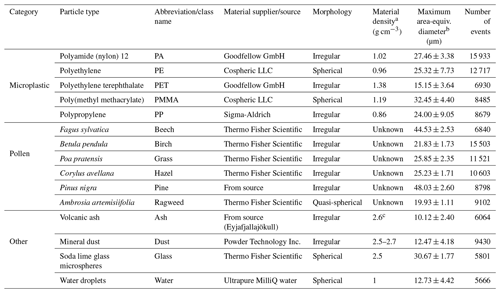
a Provided by the manufacturer, unless otherwise noted.
b Defined as the diameter of a circle with the same area as the imaged particle, taking the maximum value from the two holographic images of each particle. The values represent the mean of each dataset ±1 standard deviation.
c Schumann et al. (2011).
PA, also known as nylon 12 or PA12, was purchased in powder form (Goodfellow GmbH; Hamburg, Germany). The listed particle size range was 10–50 µm with a reported density of 1.020 g cm−3. PA has many practical applications, including product packaging, electrical insulating materials, and sports-related materials (Griehl and Ruesteivi, 1970), and is a common pollutant of the environment (Sun et al., 2019).
Low-density PE microspheres in the nominal size range of 10–106 µm were purchased from Cospheric LLC (Santa Barbara, CA, USA). The reported density is 0.96 g cm−3. PE is used, for example, in reusable bags, rigid trays and containers, and agricultural and food packing films and is made up of approximately 14.4 % of the 2022 global plastic production (Plastics Europe AISBL, 2022). Because of its high commercial use and potential environmental impact (Royer et al., 2018), PE remains a potentially important atmospheric microplastic to characterize.
PET is one of the most common polymer types in use and has applications in textiles, beverage bottles, packaging materials, and other common uses (De Vos et al., 2021). While PET remains one of the most recyclable polymer materials (Plastics Europe AISBL, 2022), much of it ends up in the environment (Schmid et al., 2021). For this study, PET MPs were generated by milling larger PET granules (Goodfellow GmbH; Hamburg, Germany) using a Retsch ZM200 rotor mill. The MPs were sieved through a 50 µm stainless steel mesh, yielding a size fraction of < 50 µm for the sample.
A sample of PMMA microspheres was purchased from Cospheric LLC (Santa Barbara, CA, USA). According to the manufacturer, the density is 1.19 g cm−3, and more than 90 % of the purchased PMMA microspheres are reported to lie in the size range of 27–45 µm. PMMA, also known as acrylic, has a wide variety of practical uses (Ali et al., 2015), including use as a transparent plastic alternative to glass (i.e., Plexiglas). PMMA can be found in environmental pollution (Brahney et al., 2020; Thompson, 2004), reaching even the most remote regions of the world (Aves et al., 2022), but represents a polymer with low demand from plastic converters (Plastics Europe AISBL, 2022).
PP microplastics were produced by milling larger granules purchased from Sigma-Aldrich (reference no. 427888; isotactic, average Mw ∼ 250 000). Briefly, the granules were melted into thin (∼ 1 mm) cuboids at 180 °C for 1 h and then frozen at −70 °C. The frozen cuboids were then milled in ice-cold ethanol for seven 30 s cycles with a knife mill (Retsch GmbH) and size-fractionated using a vibratory sieve shaker (Retsch GmbH). The fraction taken from the vibratory sieve shaker was between 38 and 50 µm. This fraction was dried prior to use.
While the pollen taxa in this study represent a small subset of other fluorescent airborne bioaerosols (Pöhlker et al., 2013), pollen particles are included in this study to assess the ability of the instrument to distinguish between aerosol particle types beyond those previously analyzed with the SwisensPoleno (Erb et al., 2024; Sauvageat et al., 2020). The six different pollen samples tested in the SwisensPoleno were measured in a desiccated state. The bulk densities of these samples are unknown. Betula pendula (birch), Fagus sylvatica (beech), Corylus avellana (hazel), Ambrosia artemislifolia (ragweed), and Poa pratensis (grass) source materials were purchased from Allergon AB (Ängelholm, Sweden) and were introduced into the SwisensPoleno instrument using the SwisensAtomizer as described above. A sample of pine pollen presented in this study was sampled directly from a recent cutting of a flowering pine tree (Pinus nigra). The cutting with male flowers was placed within a sealed chamber that was continuously flushed with particle-free air and directly connected to the SwisensPoleno. Pollen shedding was encouraged by blowing air at the flowers using a small fan.
Arizona Test Dust (Powder Technology Inc., Arden Hills, MN, USA) was investigated with the SwisensPoleno for its response to a reference mineral dust sample. In the figures, the class name for this sample is “dust”. For this study, the A2 “fine” size fraction was tested in the instrument, where the manufacturer reports a nominal size range of up to 80 µm and a composition of multiple mineral components dominated by silicates. Mineral dust and microplastics may share emission pathways (Brahney et al., 2021), and the use of mineral dust in this study represents a particle type with a similar size and morphological features to microplastic fragments. The mineral dust particles contain a variety of mineral compositions which depend greatly on their geographical location (Engelbrecht et al., 2016), some of which have been shown to autofluoresce (Savage et al., 2017). The autofluorescence of Arizona Test Dust was previously measured (Pöhlker et al., 2012), which showed a relatively low autofluorescence intensity with no discernable spectral features.
A sample of volcanic ash was collected following the 2010 volcanic eruption of Eyjafjallajökull in Iceland. This polydisperse sample represents an additional coarse-mode particle type with similar morphologies and sizes to the microplastic fragments found in the atmosphere.
Water droplets were produced through the nebulization of Milli-Q 18.2 MΩ-cm ultrapure water using a medical nebulizer. Ultrapure water is expected to have no fluorescence response (see the Supplement for more information about the SwisensPoleno fluorescence system); however, the spherical morphology presents an opportunity to test the classification accuracy alongside other spherical or quasi-spherical particles.
Glass microspheres, purchased from Thermo Fisher Scientific Inc., represent a common NIST-traceable particle standard for use in aerosol instrument calibration and testing (Dollner et al., 2024; Pinnick et al., 1981). Here, we tested glass microspheres with a nominal mean diameter of 30 ± 1.9 µm as reported by the manufacturer. While the fluorescence information of glass microspheres will not be relevant for ambient coarse-mode aerosol monitoring, the microspheres share a morphology of other common spherical microplastic beads used in, for example, personal care products (Rochman et al., 2015), and they will provide useful information in assessing the instrument's ability to discern different quasi-spherical particles.
2.3 Dataset creation and cleaning
The SwisensAtomizer was physically coupled to the inlet system of the SwisensPoleno, and each class of tested particles was introduced into the instrument by adjusting the atomizer's vibrational frequency and amplitude and the amount of air introduced into the sample volume. Particles were generated in this manner for each particle type until a suitable number (> 5000) of them were successfully detected by both the holographic imaging and fluorescence systems, referred to henceforth as an event. A total of 142 072 events were used in this study. After a dataset for one particle type is recorded, further processing is needed to filter unwanted events from the dataset. These unwanted events, for example, can include events for which a particle lies outside a suitable position for holographic image reconstruction, which results in a blurred, out-of-focus particle image; events clearly consisting of particle aggregation; or unambiguous contamination by particles of types not intended for measurement and visible through holographic imaging or detectable through unexpected fluorescence spectra of individual particles. For example, while training for the mineral dust dataset, a pine pollen particle event can be unambiguously filtered out due to its distinct shape and fluorescence response measured by the SwisensPoleno. During dataset preparation for machine learning training and testing, corrections for stray light (i.e., measurements without particles present in the measurement volume) are applied to the individual events in each dataset.
The distribution of events among the particle types, along with a count distribution of each particle's maximum area-equivalent diameter (defined as the diameter of a circle with the same area as the imaged particle, taking the maximum value from the two holographic images of each particle), is illustrated in Fig. 1.
2.4 Machine learning
The combination of measurement methods from the SwisensPoleno creates a unique set of data for each particle event. These particle event data can then be used to train a supervised machine learning classification model to predict particle types in near real time. A supervised machine learning classification model is one that maps predefined, discrete categories or classes to the input data corresponding to that output (Müller and Guido, 2016); in this study, the input data are represented by the two holographic images and/or the fluorescence spectra for each particle, and the output is the known particle type from that event. The SwisensPoleno has already demonstrated high-accuracy pollen taxa classification using its holographic imaging system, using its holographic imaging system with a supervised machine learning classification model (Sauvageat et al., 2020), and, by combining holographic images with more information, such as fluorescence, the classification accuracy of pollen can increase (Erb et al., 2024). This is especially important if the features that are used to describe the particle overlap across different particle types, such as particle autofluorescence (Pöhlker et al., 2012). In such cases, the use of machine learning can be particularly useful for finding relationships between particle types and measured particle data that traditional analysis methods cannot distinguish.
In this study, two convolutional neural network (CNN) models and a multilayer perceptron (MLP) model were trained and tested using the Keras (https://keras.io/, last access: 12 September 2023) and TensorFlow (Abadi et al., 2016) frameworks in the Python programming language to understand the ability of the SwisensPoleno's single-particle holography and fluorescence measurements to accurately predict the particle type. One CNN model (“Holo.-Only”) used only the two holographic images of a particle as input, an MLP model used only the fluorescence spectra as input (“Fl.-Only”), and the third, hybrid CNN and MLP model used both images and fluorescence as input (“Holo.+Fl.”). Each of the three models was evaluated on the same set of particle events. The two models that contained the holographic images as an input layer (Holo.-Only and Holo.+Fl.) additionally employed transfer learning using EfficientNet (Tan and Le, 2019) to improve the model performance by increasing the generalization and efficiency and greatly reducing the resources needed for training. The dataset for this study was divided into training and testing subsets using a random 60 %/40 % split. This partitioning resulted in 56 824 events distributed across the 15 datasets that were subsequently used for model evaluation. The classification accuracy was evaluated using a weighted average f score (Müller and Guido, 2016), which will be reported as an accuracy in this work. Further details of the model architecture and other specifications can be found in the Supplement.
3.1 Morphology through digital holography
Figure 2 shows two representative events acquired using the instrument's imaging system for each particle type, displaying the range of sizes and morphological features of particles used in this study. The maximum area-equivalent diameter means (±1 standard deviation) for each class are shown in Table 1. The distributions of the particle measurements for the data of each class, including the maximum area-equivalent diameters, maximum major axis lengths, maximum eccentricity, and maximum solidity, are shown in Figs. S1–S4 in the Supplement. The ash particle type represented, on average, the smallest particles measured in this study, with a mean maximum area-equivalent diameter of 10.12 ± 2.40 µm; pine particles contained the largest mean size of 48.03 ± 2.60 µm. However, the PP MP class had the largest single particles and greatest range for their measured size, with the major axis lengths ranging from ∼ 5 to 100 µm. Despite sieving during sample preparation, the milling of PP and PET particles from larger granules yielded an unexpectedly large number of particles smaller than 10 µm, which – because the samples were untreated – may have aggregated to form large clusters to create the resulting wide size distributions. The PE, PMMA, ragweed, glass, and water particle types represent the (quasi-)spherical particles tested in this study, while the PA, PET, PP, ash, mineral dust, and remaining “pollen” types are non-spherical and irregular in shape. The eccentricity (a measure of how elliptical a particle is, where a value of 0 indicates a circle and values approaching 1 indicate that a particle is becoming more elliptical) of PMMA, glass, PE, water, and ragweed are the lowest of the different types, with mean minimum eccentricities of 0.16 ± 0.05, 0.16 ± 0.06, 0.22 ± 0.08, 0.25 ± 0.12, and 0.25 ± 0.08, respectively. The PP, PET, mineral dust, and ash types represent irregular, asymmetric, and rough-edged particles, and their size distributions are similar to each other but much broader compared to the other types (Fig. 1). Solidity, a measure of a particle's 2D projected roughness (Liu et al., 2015; Sinkhonde et al., 2022) for PP, PET, mineral dust, and ash, is the lowest of all the types (0.91 ± 0.04, 0.91 ± 0.04, 0.92 ± 0.04, and 0.94 ± 0.03, respectively). As expected, the various pollen types tested were more homogenous in morphology compared to the other types, as indicated by their narrow maximum area-equivalent diameter size distribution (Fig. 1).
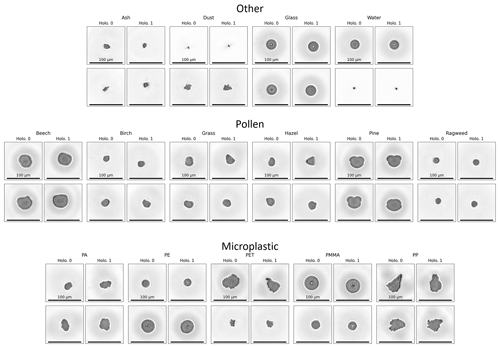
Figure 2Representative holographic images of two particles from each particle category and each particle type. For each valid imaging event, two images are produced per particle, labeled here as “Holo. 0” and “Holo. 1”. Each image is 200 × 200 px at 0.595 µm px−1; 100 µm scale bars are shown for each image.
3.2 Absolute fluorescence spectra
The mean absolute fluorescence response as measured by the SwisensPoleno for the different particle types is shown in Fig. 3. Here, the water dataset is shown to represent the baseline fluorescence response of the instrument, as the ultrapure water is expected to have no detectable autofluorescence beyond an instrument background signal.
The “other” category of particles (i.e., ash, mineral dust, glass, and water) shows generally low and featureless fluorescence across the excitation and emission channels. The glass microspheres have an enhanced fluorescence response in all the channels with the 280 nm excitation source and in the = 405/676 nm channel, which has been shown to be non-negligible in a previous investigation (Boiko et al., 2015). Mineral dust shows a slightly enhanced fluorescence response above the baseline that is broadly spread across the excitation and emission channels, coinciding with a previous investigation (Pöhlker et al., 2012). The ash sample displayed little to no fluorescence above the water (background) signal.
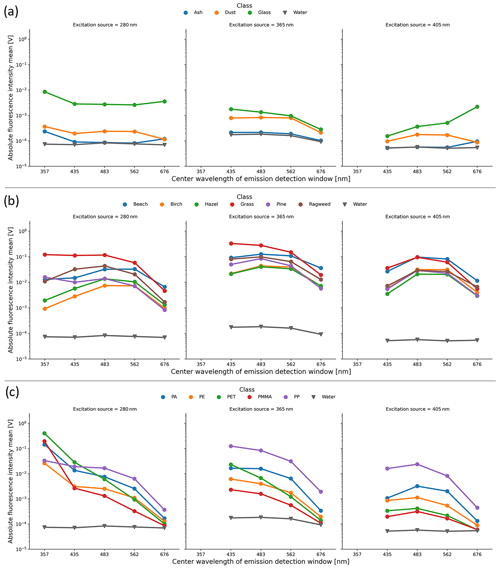
Figure 3Mean absolute fluorescence intensity (Volt) measured by the SwisensPoleno for all the particle classes, where error bars are omitted for plot clarity. The columns represent three excitation sources, and the x axis of each subplot shows the center wavelengths of the emission channels (not to scale). In each subplot, the “water” class represents the instrument background fluorescence signal, and a logarithmic y axis is used. (a) “Other” category, (b) “pollen” category, and (c) “microplastic” category, where the enhanced fluorescence of MP particles in the 280/375 nm excitation/emission can be seen, several orders of magnitude above the water background signal.
Pollen particles show an enhanced fluorescence response in all the channels. For the 365 and 405 nm excitation sources, the average fluorescence response is more similar among the pollen types, exhibiting a broad “hump” across the detection wavelengths, where the intensities are highest in the 483 nm emission detection channel for most pollen types. Generally, the grass pollen (Poa pratensis) showed the highest absolute signal response compared to the other pollen species, similar to previous studies (Lichtenthaler and Schweiger, 1998; Pöhlker et al., 2013).
For MPs, the mean fluorescence in the = 280/357 nm channel exhibits the highest response compared to the other particle types tested, where the absolute intensity is several orders of magnitude higher than the instrument background (water) signal. Conversely, the signal from the 658–694 nm waveband for all excitation sources was about 1 order of magnitude lower for MPs compared to the tested pollen species. Thus, for the 280 and 365 nm excitation sources, the mean intensity of the absolute fluorescence signal decreased with increasing wavelength. For the = 280/357 nm channel, the mean measured absolute fluorescence response for PET was highest (0.41 ± 0.19 V) compared to the other datasets tested. For the other two excitation sources, the highest absolute fluorescence response among the MPs was from the PP class. However, this is due to the largest particles found in the PP dataset, where measured absolute fluorescence intensity increases with particle size following a power law relationship (Hill et al., 2002). In order to address this and other dependencies, the SwisensPoleno calculates a relative fluorescence for each detected particle, as described in the Supplement.
3.3 Relative fluorescence spectra
Figure 4 details the differences between absolute and relative fluorescence for the 280 nm excitation source across all the detection wavebands for the five MP particle types. The size dependence for this excitation source and the measurement channels of the absolute fluorescence shows a power law relationship with the measured intensity; that is, the relationship between absolute fluorescence intensity and size is linear in the log–log space, and the slope of this relationship typically varies between ∼ 2 and 3 (Hill et al., 2015; Könemann et al., 2018). This relationship holds for all the MPs tested in this study except for PET, which has a slope of ∼ 1.5 for the 280 nm excitation source response. After applying the normalization technique to calculate a relative fluorescence, the size dependence (among other non-idealities) was largely eliminated from the measurements (Fig. 4b).
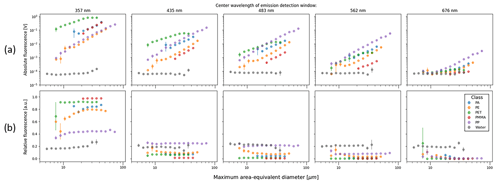
Figure 4(a) Absolute and (b) relative fluorescence of the 280 nm excitation source for MP classes and water droplets, indicating how the size dependence of the fluorescence is mostly eliminated using a relative metric. For each dataset shown, the fluorescence values are averaged for each discrete size bin, and the error bars represent the calculated standard error for the means in each bin.
The mean relative fluorescence response for the various tested particle types is shown in Fig. 5. The relative fluorescence spectra for MPs exhibit a noticeably higher response in the = 280/357 nm channel compared to the other particles tested, which do not display this spectral feature: the mean = 280/357 nm relative fluorescence values for MPs are greater than ∼ 0.44, whereas for all the other particle types tested the mean values are less than 0.33. Across all the excitation and emission channels, the mean relative fluorescence values for the tested pollen types remain below ∼ 0.5, indicating that no one channel contributed to the majority of the spectral response of the respective excitation source. Because water, ash, mineral dust, and glass particles exhibit relatively low fluorescence and little variation across the detection wavelength bands, their relative fluorescence spectra are generally flat.
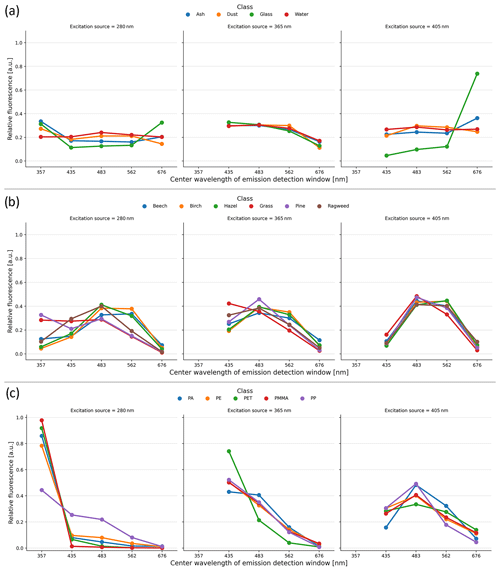
Figure 5Relative fluorescence intensities for each particle type category, where the spectral “signature” of the various particles tested is more apparent and where error bars are omitted for plot clarity. (a) “Other” category, (b) “pollen” category, and (c) “microplastic” category.
The relative fluorescence spectra represent 13 pieces of data for each valid event in the SwisensPoleno, and it becomes difficult to discern common patterns and relationships in this multidimensional dataset. We employed Uniform Manifold Approximation and Projection (UMAP) analysis (McInnes et al., 2018) to better understand the similarities and differences of the relative fluorescence spectra. UMAP is a nonlinear dimensionality reduction technique that aims to preserve the local and global structures of high-dimensional data in a lower-dimensional space (McInnes et al., 2018). The algorithm builds a weighted nearest-neighbor graph, where the weights of the connections are determined by the local density of points and their distances in the original high-dimensional space. UMAP then optimizes the embedding by finding a low-dimensional representation that minimizes the difference between the distances of connected points in the graph and their distances in the lower-dimensional space, capturing the inherent underlying structure of the data and highlighting the relationships and similarities or differences between neighboring points. This 2D representation can then be used to aid visualization and highlight these relationships between the data. Figure 6 shows the results of the UMAP algorithm applied to the relative fluorescence for all events of each data type used in this study, projected into two dimensions. The spacing of the data points in the UMAP plot reflects their similarities or differences: points that are close together indicate that they are more similar based on their spectral characteristics or fluorescence spectra; conversely, points that are far apart in the UMAP plot suggest greater dissimilarity or differences in their spectral properties. As expected, the events from each dataset form relatively tight clusters, and datasets which share relative fluorescence spectral features have clusters in the UMAP plot that are close together or overlap. For example, water, ash, mineral dust, and glass particles overlap in the center of the plot, indicating that their relative fluorescence spectral features also overlap. The birch and hazel pollen datasets share similar relative fluorescence spectral shapes (Fig. 5b), and this is reflected in the UMAP representation with slightly overlapping clusters. For all the other particle types, clustering in the UMAP plot is more distinct, which leads to the interpretation that the underlying relationships in the relative fluorescence spectral features are also quite distinct from one another.
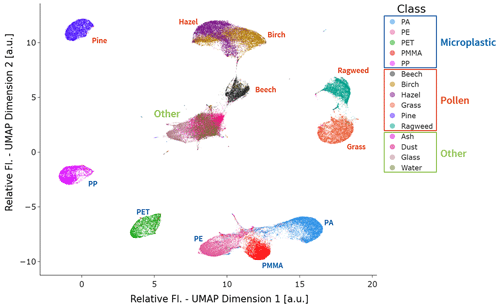
Figure 6UMAP plot of the relative fluorescence spectra for every event in this study. The UMAP analysis depicts the high-dimensional relative fluorescence spectra in a low-dimensional (2D) representation, where each dot represents one event in the study. This 2D representation also provides insight into the relative similarity and difference between the relative fluorescence spectra: the closer each event is, the more similar their relative fluorescence spectra are; conversely, events that are further apart represent relative fluorescence spectra that are more dissimilar. Each dot is colored according to its class name in the legend; the text for each class is colored according to the category of particle types. The events from the particle classes in the “other” category (i.e., ash, dust, glass, and water) are clustered and overlap near the center of the UMAP plot, indicating the underlying similarity of relative fluorescence in this study.
3.4 Particle classification using machine learning
An integrated component of the SwisensPoleno workflow is the ability to classify particle types in near real time by applying a trained machine learning model. This capability was assessed using the measurements in this study by employing three different machine learning model architectures utilizing holographic images and relative fluorescence spectra of the particles as input parameters for particle type classification.
The first model investigated uses a CNN that employs only the two holographic images as input (Holo.-Only). This model differs from the models used in previous studies of bioaerosol identification (Erb et al., 2024; Sauvageat et al., 2020) by expanding the classified particle types beyond bioaerosols and evaluating a different model architecture. Supervised learning classification models often employ a confusion matrix to convey model performance. The values in a normalized confusion matrix show the classification or misclassification for different classes in a classification model, where the values are expressed as percentages or proportions relative to the total number of particles in each true class. The diagonal values represent the correct classification for each class, while the off-diagonal values represent the misclassification percentages. The confusion matrix and performance for the Holo.-Only model can be seen in Fig. 7. The model training resulted in an overall accuracy of 90 % for the test dataset. Particle types that share size and shape characteristics perform worse than those with defining features, such as pollen. For example, the ash, mineral dust, hazel, PET, and PP particle types had an individual classification accuracy of less than 81 % that resulted from their shared irregular morphologies and/or similar size distributions. PET particles were incorrectly classified in 21 % of the 2773 events used in the test dataset as either ash or mineral dust particles, while PP was incorrectly classified as PET in 12 % of the 3458 test dataset events. Interestingly, the spherical particle types (glass, water, ragweed, PE, and PMMA) performed surprisingly well (accuracy > 96 %) considering the overlap in their general morphological characteristics and the size of the tested particles. Of the pollen types, hazel particles were most frequently classified incorrectly with an accuracy of 76 %, where nearly all the misclassified particles (23 %) were classified as birch, highlighting an existing challenge in identifying these two particular pollen taxa based on their very similar morphologies alone.
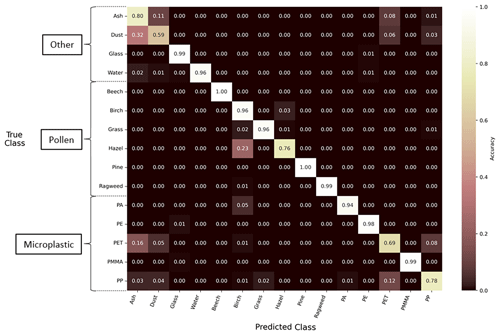
Figure 7Performance of the Holo.-Only machine learning model using a normalized confusion matrix. The diagonal values in the matrix represent the proportion of true positives or the percentage of correctly classified particles for the respective true class on the y axis. The off-diagonal values represent false positives, indicating the misclassification of particles into their respective predicted classes on the x axis. The matrix is normalized along each row.
The second machine learning classification model was a multilayer perceptron using only the relative fluorescence spectra as input (Fl.-Only). Here, the Fl.-Only model had an overall classification accuracy of 94 %, and the distribution of the prediction accuracy is shown in Fig. 8. The accuracy for all the pollen and MP particle types was greater than 92 %, improving on deficiencies when using only the Holo.-Only model for these classes. When assessing MP particle types alone, the Fl.-Only model performed with an accuracy of greater than 98 %. In contrast, the accuracy for correct classification of water, ash, mineral dust, and glass particles had a mixed performance, with accuracies of greater than 95 % for glass particles but less than 74 % for ash, mineral dust, and water particles.
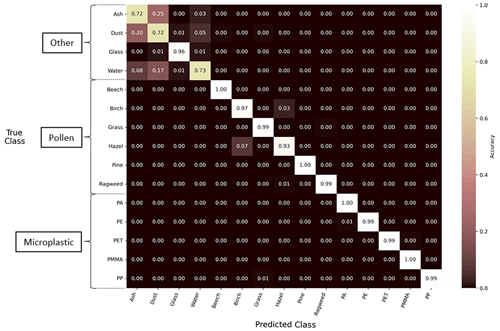
Figure 8Performance of the Fl.-Only machine learning model using a normalized confusion matrix. The diagonal values in the matrix represent the proportion of true positives or the percentage of correctly classified particles for the respective true class on the y axis. The off-diagonal values represent false positives, indicating the misclassification of particles into their respective predicted classes on the x axis. The matrix is normalized along each row.
The third model tested combined the holographic images and relative fluorescence approaches into a single, multi-input model (Holo.+Fl.). An overall prediction accuracy of 98 % was found for this model when using the particle types tested in this study. Figure 9 shows the normalized confusion matrix for these results, indicating the prediction accuracy across all the particle types. An accuracy of less than 95 % was observed only for the ash and mineral dust particle types (85 % and 82 %, respectively). All MP particles were correctly classified at least 98.5 % of the time. Comparing the classification accuracies in Figs. 8 and 9, all the particle types improved their classification performance compared to the models using only their relative fluorescence or holographic images.
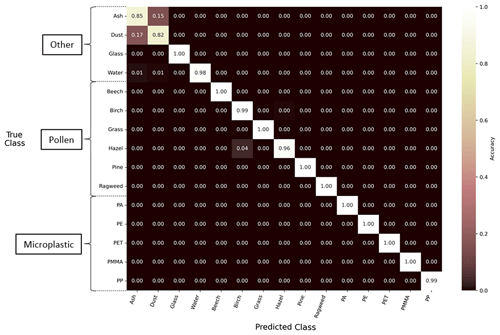
Figure 9Performance of the Holo.+Fl. machine learning model using a normalized confusion matrix. The diagonal values in the matrix represent the proportion of true positives or the percentage of correctly classified particles for the respective true class on the y axis. The off-diagonal values represent false positives, indicating the misclassification of particles into their respective predicted classes on the x axis. The matrix is normalized along each row. For example, 4 % of all the hazel particles in the test dataset were misclassified as birch using the Holo.+Fl. model.
Digital holography can provide improved information about aerosol particle size and shape beyond other light-scattering methods (Berg et al., 2017) and has been demonstrated for various coarse-mode particles, including bioaerosol (Erb et al., 2024; Sauvageat et al., 2020), ice crystals (Touloupas et al., 2020), and more (Berg et al., 2017). The SwisensPoleno is a powerful instrument for capturing a diverse range of single-particle morphologies in near real time. The MP particles tested in this study closely represent two common MP morphologies – spherical beads and fragments – found in the environment (Cowger et al., 2020; Helm, 2017; Yu et al., 2023). However, particles that share morphological features and size distributions may be misclassified by a machine learning model that uses 2D images as the only training data input, as was demonstrated in this work. For example, fragmented, irregular particle types in this study that had similar size distributions – such as PP, PET, volcanic ash, and mineral dust – performed with lower accuracies (accuracies < 81 %) when using a machine learning model employing holographic images as the only input, where including additional, concurrent measurement information may increase the accuracy of real-time particle identification. On the other hand, spherical and quasi-spherical particle types – such as ragweed pollen, water droplets, glass beads, PE microspheres, and PMMA microspheres – performed well (accuracies > 96 %) when considering their holographic images only, indicating that this machine learning model can find distinctive features not easily identifiable by the eye.
The MP particles tested in this study have an absolute fluorescence response greater than or on the same order as pollen particles. The exceptionally strong fluorescence observed for PET particles aligns with expectations, as PET contains an aromatic ring in its composition acting as a strongly emitting fluorophore. PET MPs and nanoplastics were previously observed to exhibit autofluorescence, due to their strong absorption in the UV region (Lionetto et al., 2022). While PET exhibits fluorescence when excited at longer wavelengths (i.e., in the visible spectrum), the results from this study showed that, as the excitation wavelength increases, the fluorescence intensity decreases. However, polymers which lack aromatic or highly conjugated double-bond structures (i.e., PA, PMMA, PP, and PE) are not traditionally associated with strong autofluorescence (Shadpour et al., 2006); nonetheless, the PA, PMMA, PP, and PE microplastics used in this study displayed fluorescence intensities on the same order as the primary biological particles tested. These results may suggest the presence of other factors that contribute to their measured fluorescence, such as the unintended presence of impurities or additives (i.e., unintentionally added substances; Bridson et al., 2023). Additionally, while polyolefins like PE and PP do not contain fluorophores in their chemical structure, photooxidation or thermal oxidation (Allen et al., 1977; Zhao et al., 2022), impurities (Bridson et al., 2023; Laatsch et al., 2023), fiber structural defects (Poszwa et al., 2016), or formation of high-molecular-weight clusters (Laatsch et al., 2023) can cause PE and PP to become fluorescent. For example, during the photooxidation process, enones and dienones can be formed (Allen et al., 1977), which makes those polymers gain fluorescent properties. Further investigation is required to understand the specific mechanisms driving the fluorescent properties observed.
While the autofluorescence properties of other airborne particles (such as polycyclic aromatic hydrocarbons – PAHs –, mineral dust, or pollen) may overlap (Pöhlker et al., 2012; Savage et al., 2017), the use of the SwisensPoleno instrument is a very promising method to overcome the challenge of distinguishing MPs from other airborne particles due to the combined information of particle morphology and fluorescence provided by the instrument. The relative fluorescence spectra for the tested particles show distinct spectral features that can be distinguishable from each other, as demonstrated by, for example, the UMAP dimensionality reduction technique (Fig. 6). The relative fluorescence measurement system, combined with a machine learning classification model, allows for particles that share morphological characteristics to be distinguished by a high degree of accuracy, such as the spherical particles used in this study (water droplets, ragweed pollen, glass, PE, and PMMA microspheres). When using the relative fluorescence of the particles in a machine learning model, the overall classification accuracy was enhanced compared to when particle holographic images were only used for model inference, increasing from 90 % to 94 %. Particles that exhibit a distinct fluorescence spectral pattern can be differentiated from other particle types with high accuracy using the machine learning model; conversely, particles that have low relative fluorescence and indistinct spectral features – such as water and mineral dust – were more often misclassified in model evaluation. This result could prove problematic for any ambient measurements that rely strictly on fluorescence in environments where the interaction of water droplets and mineral dust is possible. Here, too, future work using the SwisensPoleno may help classify these ambiguous fluorescence events by including the polarized scattering information for each event.
It is important to acknowledge that the atmosphere contains a wide variety of aerosols in terms of composition, size, and shape (Seinfeld and Pandis, 2016). This study only considers specific subsets of particle types that the SwisensPoleno instrument might encounter during ambient monitoring; therefore, while the machine learning models in this study exhibited generally high classification accuracies, generalizing them to ambient measurements with the SwisensPoleno will likely lead to misclassifications. For example, while not addressed in this study, future work should assess how the SwisensPoleno's fluorescence response is affected by different variables, such as the source and age of various pollen taxa, both commercially available reference pollens and freshly collected samples. Additionally, spores of various bacteria and fungi – known to be an important atmospheric bioaerosol that autofluoresces (Hill et al., 2009) – are not considered here and would certainly be misclassified if the models used in this study – lacking the necessary training data – were used in ambient particle identification. For MPs, while the MPs tested in this study were assumed to be without additives, many plastics are produced with additives that enhance their performance or functionality (Hahladakis et al., 2018). Thus, it can be assumed that many of the MPs in the environment also contain additives, which could alter their measured fluorescence in the SwisensPoleno. Additionally, further investigation is required to understand how components of airborne microplastics found in the environment – such as particles comprised of multiple components (i.e., tire and road wear particles; Kreider et al., 2010), those containing pollutants adsorbed onto the surface (e.g., Fu et al., 2021; Gao et al., 2021), or those that have undergone environmental weathering processes such as photooxidation (Sun et al., 2020) – contribute to changes in measured fluorescence and how this may impact their measurement in the SwisensPoleno.
In this study, the high-performance capabilities of the SwisensPoleno's measurement system and application of a machine learning classification model were evaluated to accurately characterize and identify five different polymer types of MP particles under controlled laboratory conditions. The instrument's ability to identify and differentiate MPs from similarly featured coarse-mode aerosol particles, including mineral dust, various pollen taxa, and water droplets, was demonstrated. This was achieved through the application of a machine learning model that was trained and validated on separate datasets consisting of holographic images and fluorescence spectral data for each particle type. The high classification accuracy of the model affirmed the instrument's effectiveness in distinguishing between single coarse-mode particles.
The microplastics tested in this study represent common polymer types for microplastics found in environmental pollution. They display sufficient fluorescence intensities that can be measured with the SwisensPoleno and have distinct spectral features, aiding in distinguishing particle types between both MPs and non-MPs. In the machine learning classification model configurations used in this study, model performance increased when combining holographic images of single microplastic particles with their measure relative fluorescence, expanding on previous studies using the instrument for bioaerosol identification. Future work is required to understand how increasing sample complexity can affect instrument performance and particle typing accuracy. For example, more particle types with varying morphologies and compositions need to be tested, such as MP fibers, MP particles that have experienced atmospheric processing or weathering, and MP particles with additives or other chemical composition differences. The prediction accuracy of these various other MPs needs to be evaluated alongside other autofluorescing aerosol particles, including additional bioaerosol types such as spores, combustion byproducts such as PAHs, and tire and road wear particles.
While an improvement to the comprehensiveness of the data used can improve future studies, all MPs tested in this study demonstrated detectable fluorescence, falling within the measurement range of the SwisensPoleno. The combination of fluorescence and holographic imaging enabled the machine learning models to distinguish various MP types in the study, between one another and other coarse-mode particles, suggesting the potential suitability of the instrument for monitoring airborne MPs under ambient conditions. The ability to monitor and accurately classify MPs in situ and in near real time would provide a substantial increase in the understanding of the abundance, distribution, properties, and potential impact MP particles could have on humans and the environment.
The data and code used in this study are available for research purposes on request from the authors.
The supplement related to this article is available online at: https://doi.org/10.5194/amt-17-6945-2024-supplement.
NDB and BW conceptualized the study. NDB, JB, and BW designed the experiments. NDB, JB, and LAD prepared the materials for measurement. NDB and JB performed the measurements. EG and YZ developed the data processing and machine learning software, which was edited and implemented by NDB. NDB analyzed the data and prepared the manuscript with contributions from BW and LAD, and all the co-authors contributed to the reviewing and editing of the manuscript.
EG and YZ are employees of Swisens AG, yet their employment at Swisens AG does not impact the results presented or influence the interpretation of the results. The research was conducted with good scientific rigor and impartiality. The authors have no other competing interests to declare.
Publisher’s note: Copernicus Publications remains neutral with regard to jurisdictional claims made in the text, published maps, institutional affiliations, or any other geographical representation in this paper. While Copernicus Publications makes every effort to include appropriate place names, the final responsibility lies with the authors.
The authors acknowledge the support of Hans Moosmüller by supplying the Arizona Test Dust sample and Erny Niederberger and Maximilian Dollner for their valuable discussions regarding the study design and manuscript preparation. Additionally, the authors acknowledge and thank Itziar Otazo Aseguinolaza and Lukas Wimmer for their assistance in the preparation of the polypropylene and polyethylene terephthalate samples used in this study. The authors acknowledge the funding by the University of Vienna, the Gottfried and Vera Weiss Foundation, and FWF. The University of Vienna's investment program is acknowledged for purchasing the SwisensPoleno instrument. Lee Ann Dailey would like to acknowledge the funding from the IMPTOX project.
The authors have been funded through the University of Vienna and the Gottfried-and-Vera Weiss Foundation and FWF in the context of the PlasticSphere project (FWF project no. AP36417; https://doi.org/10.55776/P36417). The SwisensPoleno instrument was purchased under the University of Vienna’s investment program (IP734015). Lea Ann Dailey has been funded from the IMPTOX project (European Union Horizon 2020 program grant no. 965173).
This paper was edited by Francis Pope and reviewed by two anonymous referees.
Abadi, M., Barham, P., Chen, J., Chen, Z., Davis, A., Dean, J., Devin, M., Ghemawat, S., Irving, G., Isard, M., Kudlur, M., Levenberg, J., Monga, R., Moore, S., Murray, D. G., Steiner, B., Tucker, P., Vasudevan, V., Warden, P., Wicke, M., Yu, Y., and Zheng, X.: Tensorflow: a system for large-scale machine learning, in: Proceedings of the 12th USENIX Symposium on Operating Systems Design and Implementation (OSDI'16), Savannah, GA, USA, 2–4 November 2016, USENIX Association, 265–283, ISBN 978-1-931971-33-1, 2016.
Agrawala, S., Dubois, M., Börkey, P., and Lanzi, E. (Eds.): Global Plastics Outlook: Economic Drivers, Environmental Impacts, and Policy Options, OECD, https://doi.org/10.1787/de747aef-en, 2022.
Akdogan, Z. and Guven, B.: Microplastics in the environment: A critical review of current understanding and identification of future research needs, Environ. Pollut., 254, 113011, https://doi.org/10.1016/j.envpol.2019.113011, 2019.
Ali, U., Karim, K. J. B. A., and Buang, N. A.: A Review of the Properties and Applications of Poly (Methyl Methacrylate) (PMMA), Polym. Rev., 55, 678–705, https://doi.org/10.1080/15583724.2015.1031377, 2015.
Allen, N. S., Homer, J., and McKellar, J. F.: The use of luminescence spectroscopy in aiding the identification of commercial polymers, Analyst, 101, 260–264, https://doi.org/10.1039/an9760100260, 1976.
Allen, N. S., Homer, J., and McKellar, J. F.: Origin and role of the luminescent species in the photo-oxidation of commercial polypropylene, J. Appl. Polym. Sci., 21, 2261–2267, https://doi.org/10.1002/app.1977.070210823, 1977.
Asfour, H., Otridge, J., Thomasian, R., Larson, C., and Sarvazyan, N.: Autofluorescence properties of balloon polymers used in medical applications, J. Biomed. Opt., 25, 1–18, https://doi.org/10.1117/1.jbo.25.10.106004, 2020.
Aves, A. R., Revell, L. E., Gaw, S., Ruffell, H., Schuddeboom, A., Wotherspoon, N. E., LaRue, M., and McDonald, A. J.: First evidence of microplastics in Antarctic snow, The Cryosphere, 16, 2127–2145, https://doi.org/10.5194/tc-16-2127-2022, 2022.
Barnes, D. K. A., Galgani, F., Thompson, R. C., and Barlaz, M.: Accumulation and fragmentation of plastic debris in global environments, Philos. T. Roy. Soc. B, 364, 1985–1998, https://doi.org/10.1098/rstb.2008.0205, 2009.
Beaurepaire, M., Dris, R., Gasperi, J., and Tassin, B.: Microplastics in the atmospheric compartment: a comprehensive review on methods, results on their occurrence and determining factors, Curr. Opin. Food Sci., 41, 159–168, https://doi.org/10.1016/j.cofs.2021.04.010, 2021.
Berg, M. J.: Tutorial: Aerosol characterization with digital in-line holography, J. Aerosol Sci., 165, 106023, https://doi.org/10.1016/j.jaerosci.2022.106023, 2022.
Berg, M. J. and Videen, G.: Digital holographic imaging of aerosol particles in flight, J. Quant. Spectrosc. Ra., 112, 1776–1783, https://doi.org/10.1016/j.jqsrt.2011.01.013, 2011.
Berg, M. J., Heinson, Y. W., Kemppinen, O., and Holler, S.: Solving the inverse problem for coarse-mode aerosol particle morphology with digital holography, Sci. Rep., 7, 9400, https://doi.org/10.1038/s41598-017-09957-w, 2017.
Bergmann, M., Mützel, S., Primpke, S., Tekman, M. B., Trachsel, J., and Gerdts, G.: White and wonderful? Microplastics prevail in snow from the Alps to the Arctic, Sci. Adv., 5, eaax1157, https://doi.org/10.1126/sciadv.aax1157, 2019.
Boiko, V., Dovbeshko, G., Dolgov, L., Kiisk, V., Sildos, I., Loot, A., and Gorelik, V.: Angular shaping of fluorescence from synthetic opal-based photonic crystal, Nanoscale Res. Lett., 10, 1–7, https://doi.org/10.1186/s11671-015-0781-y, 2015.
Brahney, J., Hallerud, M., Heim, E., Hahnenberger, M., and Sukumaran, S.: Plastic rain in protected areas of the United States, Science, 368, 1257–1260, https://doi.org/10.1126/science.aaz5819, 2020.
Brahney, J., Mahowald, N., Prank, M., Cornwell, G., Klimont, Z., Matsui, H., and Prather, K. A.: Constraining the atmospheric limb of the plastic cycle, P. Natl. Acad. Sci. USA, 118, e2020719118, https://doi.org/10.1073/pnas.2020719118, 2021.
Brandon, J., Goldstein, M., and Ohman, M. D.: Long-term aging and degradation of microplastic particles: Comparing in situ oceanic and experimental weathering patterns, Mar. Pollut. Bull., 110, 299–308, https://doi.org/10.1016/j.marpolbul.2016.06.048, 2016.
Bridson, J. H., Abbel, R., Smith, D. A., Northcott, G. L., and Gaw, S.: Release of additives and non-intentionally added substances from microplastics under environmentally relevant conditions, Environmental Advances, 12, 100359, https://doi.org/10.1016/j.envadv.2023.100359, 2023.
Capolungo, C., Genovese, D., Montalti, M., Rampazzo, E., Zaccheroni, N., and Prodi, L.: Photoluminescence-Based Techniques for the Detection of Micro- and Nanoplastics, Chem.-Eur. J., 27, 17529–17541, https://doi.org/10.1002/chem.202102692, 2021.
Cole, M., Lindeque, P., Halsband, C., and Galloway, T. S.: Microplastics as contaminants in the marine environment: A review, Mar. Pollut. Bull., 62, 2588–2597, https://doi.org/10.1016/j.marpolbul.2011.09.025, 2011.
Cowger, W., Booth, A. M., Hamilton, B. M., Thaysen, C., Primpke, S., Munno, K., Lusher, A. L., Dehaut, A., Vaz, V. P., Liboiron, M., Devriese, L. I., Hermabessiere, L., Rochman, C., Athey, S. N., Lynch, J. M., De Frond, H., Gray, A., Jones, O. A. H., Brander, S., Steele, C., Moore, S., Sanchez, A., and Nel, H.: Reporting Guidelines to Increase the Reproducibility and Comparability of Research on Microplastics, Appl. Spectrosc., 74, 1066–1077, https://doi.org/10.1177/0003702820930292, 2020.
Cózar, A., Echevarría, F., González-Gordillo, J. I., Irigoien, X., Úbeda, B., Hernández-León, S., Palma, Á. T., Navarro, S., García-de-Lomas, J., Ruiz, A., Fernández-de-Puelles, M. L., and Duarte, C. M.: Plastic debris in the open ocean, P. Natl. Acad. Sci. USA, 111, 10239–10244, https://doi.org/10.1073/pnas.1314705111, 2014.
Davison, S. M. C., White, M. P., Pahl, S., Taylor, T., Fielding, K., Roberts, B. R., Economou, T., McMeel, O., Kellett, P., and Fleming, L. E.: Public concern about, and desire for research into, the human health effects of marine plastic pollution: Results from a 15-country survey across Europe and Australia, Global Environ. Chang., 69, 102309, https://doi.org/10.1016/j.gloenvcha.2021.102309, 2021.
De Vos, L., Van de Voorde, B., Van Daele, L., Dubruel, P., and Van Vlierberghe, S.: Poly(alkylene terephthalate)s: From current developments in synthetic strategies towards applications, Eur. Polym. J., 161, 110840, https://doi.org/10.1016/j.eurpolymj.2021.110840, 2021.
Dollner, M., Gasteiger, J., Schöberl, M., Gattringer, A., Beres, N. D., Bui, T. P., Diskin, G., and Weinzierl, B.: The Cloud Indicator: A novel algorithm for automatic detection and classification of clouds using airborne in situ observations, Atmos. Res., 308, 107504, https://doi.org/10.1016/j.atmosres.2024.107504, 2024.
Driedger, A. G. J., Dürr, H. H., Mitchell, K., and Van Cappellen, P.: Plastic debris in the Laurentian Great Lakes: A review, J. Great Lakes Res., 41, 9–19, https://doi.org/10.1016/j.jglr.2014.12.020, 2015.
Engelbrecht, J. P., Moosmüller, H., Pincock, S., Jayanty, R. K. M., Lersch, T., and Casuccio, G.: Technical note: Mineralogical, chemical, morphological, and optical interrelationships of mineral dust re-suspensions, Atmos. Chem. Phys., 16, 10809–10830, https://doi.org/10.5194/acp-16-10809-2016, 2016.
Enyoh, C. E., Verla, A. W., Verla, E. N., Ibe, F. C., and Amaobi, C. E.: Airborne microplastics: a review study on method for analysis, occurrence, movement and risks, Environ. Monit. Assess., 191, 668, https://doi.org/10.1007/s10661-019-7842-0, 2019.
Erb, S., Berne, A., Burgdorfer, N., Clot, B., Graber, M.-J., Lieberherr, G., Sallin, C., Tummon, F., and Crouzy, B.: Automatic real-time monitoring of fungal spores: the case of Alternaria spp., Aerobiologia, 40, 123–127 https://doi.org/10.1007/s10453-023-09780-z, 2023.
Erb, S., Graf, E., Zeder, Y., Lionetti, S., Berne, A., Clot, B., Lieberherr, G., Tummon, F., Wullschleger, P., and Crouzy, B.: Real-time pollen identification using holographic imaging and fluorescence measurements, Atmos. Meas. Tech., 17, 441–451, https://doi.org/10.5194/amt-17-441-2024, 2024.
Erni-Cassola, G., Gibson, M. I., Thompson, R. C., and Christie-Oleza, J. A.: Lost, but Found with Nile Red: A Novel Method for Detecting and Quantifying Small Microplastics (1 mm to 20 µm) in Environmental Samples, Environ. Sci. Technol., 51, 13641–13648, https://doi.org/10.1021/acs.est.7b04512, 2017.
Evangeliou, N., Grythe, H., Klimont, Z., Heyes, C., Eckhardt, S., Lopez-Aparicio, S., and Stohl, A.: Atmospheric transport is a major pathway of microplastics to remote regions, Nat. Commun., 11, 3381, https://doi.org/10.1038/s41467-020-17201-9, 2020.
Fendall, L. S. and Sewell, M. A.: Contributing to marine pollution by washing your face: Microplastics in facial cleansers, Mar. Pollut. Bull., 58, 1225–1228, https://doi.org/10.1016/j.marpolbul.2009.04.025, 2009.
Fu, L., Li, J., Wang, G., Luan, Y., and Dai, W.: Adsorption behavior of organic pollutants on microplastics, Ecotox. Environ. Safe., 217, 112207, https://doi.org/10.1016/j.ecoenv.2021.112207, 2021.
Gao, X., Hassan, I., Peng, Y., Huo, S., and Ling, L.: Behaviors and influencing factors of the heavy metals adsorption onto microplastics: A review, J. Clean. Prod., 319, 128777, https://doi.org/10.1016/j.jclepro.2021.128777, 2021.
Gasperi, J., Wright, S. L., Dris, R., Collard, F., Mandin, C., Guerrouache, M., Langlois, V., Kelly, F. J., and Tassin, B.: Microplastics in air: Are we breathing it in?, Curr. Opin. Environ. Sci. Health, 1, 1–5, https://doi.org/10.1016/j.coesh.2017.10.002, 2018.
Geyer, R., Jambeck, J. R., and Law, K. L.: Production, use, and fate of all plastics ever made, Sci. Adv., 3, 25–29, https://doi.org/10.1126/sciadv.1700782, 2017.
Gratzl, J., Seifried, T. M., Stolzenburg, D., and Grothe, H.: A fluorescence approach for an online measurement technique of atmospheric microplastics, Environmental Science: Atmospheres, 4, 601–610, https://doi.org/10.1039/D4EA00010B, 2024.
Griehl, W. and Ruesteivi, D.: Nylon-12-Preparation, Properties, and Applications, J. Ind. Eng. Chem., 62, 16–22, https://doi.org/10.1021/ie50723a005, 1970.
Hahladakis, J. N., Velis, C. A., Weber, R., Iacovidou, E., and Purnell, P.: An overview of chemical additives present in plastics: Migration, release, fate and environmental impact during their use, disposal and recycling, J. Hazard Mater., 344, 179–199, https://doi.org/10.1016/j.jhazmat.2017.10.014, 2018.
Hartmann, N. B., Hüffer, T., Thompson, R. C., Hassellöv, M., Verschoor, A., Daugaard, A. E., Rist, S., Karlsson, T., Brennholt, N., Cole, M., Herrling, M. P., Hess, M. C., Ivleva, N. P., Lusher, A. L., and Wagner, M.: Are We Speaking the Same Language? Recommendations for a Definition and Categorization Framework for Plastic Debris, Environ. Sci. Technol., 53, 1039–1047, https://doi.org/10.1021/acs.est.8b05297, 2019.
Hawkins, K. R. and Yager, P.: Nonlinear decrease of background fluorescence in polymer thin-films – a survey of materials and how they can complicate fluorescence detection in µTAS, Lab Chip, 3, 248–252, https://doi.org/10.1039/B307772C, 2003.
Helm, P. A.: Improving microplastics source apportionment: A role for microplastic morphology and taxonomy?, Anal. Methods-UK, 9, 1328–1331, https://doi.org/10.1039/c7ay90016c, 2017.
Hill, S. C., Pinnick, R. G., Niles, S., Fell, N. F., Pan, Y.-L., Bottiger, J., Bronk, B. V., Holler, S., and Chang, R. K.: Fluorescence from airborne microparticles: dependence on size, concentration of fluorophores, and illumination intensity: erratum, Appl. Optics, 41, 4432, https://doi.org/10.1364/AO.41.004432, 2002.
Hill, S. C., Mayo, M. W., and Chang, R. K.: Fluorescence of bacteria, pollens, and naturally occurring airborne particles: excitation/emission spectra Army report, ARL-TR-4722, 2009.
Hill, S. C., Williamson, C. C., Doughty, D. C., Pan, Y. Le, Santarpia, J. L., and Hill, H. H.: Size-dependent fluorescence of bioaerosols: Mathematical model using fluorescing and absorbing molecules in bacteria, J. Quant. Spectrosc. Ra., 157, 54–70, https://doi.org/10.1016/j.jqsrt.2015.01.011, 2015.
International Organization for Standardization: Issue Brief: ISO definitions of key terms for plastic pollution, ISO, Geneva, Switzerland, 16 pp., ISBN 978-92-67-11320-3, 2023.
Koelmans, A. A., Redondo-Hasselerharm, P. E., Nor, N. H. M., de Ruijter, V. N., Mintenig, S. M., and Kooi, M.: Risk assessment of microplastic particles, Nature Reviews Materials, 7, 138–152, https://doi.org/10.1038/s41578-021-00411-y, 2022.
Könemann, T., Savage, N. J., Huffman, J. A., and Pöhlker, C.: Characterization of steady-state fluorescence properties of polystyrene latex spheres using off- and online spectroscopic methods, Atmos. Meas. Tech., 11, 3987–4003, https://doi.org/10.5194/amt-11-3987-2018, 2018.
Kreider, M. L., Panko, J. M., McAtee, B. L., Sweet, L. I., and Finley, B. L.: Physical and chemical characterization of tire-related particles: Comparison of particles generated using different methodologies, Sci. Total Environ., 408, 652–659, https://doi.org/10.1016/j.scitotenv.2009.10.016, 2010.
Laatsch, B. F., Brandt, M., Finke, B., Fossum, C. J., Wackett, M. J., Lowater, H. R., Narkiewicz-Jodko, A., Le, C. N., Yang, T., Glogowski, E. M., Bailey-Hartsel, S. C., Bhattacharyya, S., and Hati, S.: Polyethylene Glycol 20k. Does It Fluoresce?, ACS Omega, 8, 14208–14218, https://doi.org/10.1021/acsomega.3c01124, 2023.
Lakowicz, J. R. (Ed.): Principles of Fluorescence Spectroscopy, 3rd edn., Springer US, 954 pp., https://doi.org/10.1007/978-0-387-46312-4, 2006.
Lichtenthaler, H. K. and Schweiger, J.: Cell wall bound ferulic acid, the major substance of the blue-green fluorescence emission of plants, J. Plant Physiol., 152, 272–282, https://doi.org/10.1016/S0176-1617(98)80142-9, 1998.
Lionetto, F., Lionetto, M. G., Mele, C., Corcione, C. E., Bagheri, S., Udayan, G., and Maffezzoli, A.: Autofluorescence of Model Polyethylene Terephthalate Nanoplastics for Cell Interaction Studies, Nanomaterials, 12, 1560, https://doi.org/10.3390/nano12091560, 2022.
Liu, E. J., Cashman, K. V., Rust, A. C., and Gislason, S. R.: The role of bubbles in generating fine ash during hydromagmatic eruptions, Geology, 43, 239–242, https://doi.org/10.1130/G36336.1, 2015.
Maes, T., Jessop, R., Wellner, N., Haupt, K., and Mayes, A. G.: A rapid-screening approach to detect and quantify microplastics based on fluorescent tagging with Nile Red, Sci. Rep., 7, 44501, https://doi.org/10.1038/srep44501, 2017.
Mammo, F. K., Amoah, I. D., Gani, K. M., Pillay, L., Ratha, S. K., Bux, F., and Kumari, S.: Microplastics in the environment: Interactions with microbes and chemical contaminants, Sci. Total Environ., 743, 140518, https://doi.org/10.1016/j.scitotenv.2020.140518, 2020.
Mao, R., Lang, M., Yu, X., Wu, R., Yang, X., and Guo, X.: Aging mechanism of microplastics with UV irradiation and its effects on the adsorption of heavy metals, J. Hazard Mater., 393, 122515, https://doi.org/10.1016/j.jhazmat.2020.122515, 2020.
McInnes, L., Healy, J., and Melville, J.: UMAP: Uniform Manifold Approximation and Projection for Dimension Reduction, https://doi.org/10.48550/ARXIV.1802.03426, 2018.
Monteleone, A., Brandau, L., Schary, W., and Wenzel, F.: Using autofluorescence for microplastic detection – Heat treatment increases the autofluorescence of microplastics, Clin. Hemorheol. Micro., 76, 473–493, https://doi.org/10.3233/CH-209223, 2021a.
Monteleone, A., Wenzel, F., Langhals, H., and Dietrich, D.: New application for the identification and differentiation of microplastics based on fluorescence lifetime imaging microscopy (FLIM), Journal of Environmental Chemical Engineering, 9, 104769, https://doi.org/10.1016/j.jece.2020.104769, 2021b.
Müller, A. C. and Guido, S.: Introduction to Machine Learning with Python, O'Reilly Media, Inc., ISBN: 9781449369897, 2016.
Ornik, J., Sommer, S., Gies, S., Weber, M., Lott, C., Balzer, J. C., and Koch, M.: Could photoluminescence spectroscopy be an alternative technique for the detection of microplastics? First experiments using a 405 nm laser for excitation, Appl. Phys. B, 126, 15, https://doi.org/10.1007/s00340-019-7360-3, 2020.
Othman, A. R., Hasan, H. A., Muhamad, M. H., Ismail, N. 'Izzati, and Abdullah, S. R. S.: Microbial degradation of microplastics by enzymatic processes: a review, Environ. Chem. Lett., 19, 3057–3073, https://doi.org/10.1007/s10311-021-01197-9, 2021.
Pinnick, R. G., Garvey, D. M., and Duncan, L. D.: Calibration of Knollenberg FSSP Light-Scattering Counters for Measurement of Cloud Droplets, J. Appl. Meteorol., 20, 1049–1057, https://doi.org/10.1175/1520-0450(1981)020<1049:COKFLS>2.0.CO;2, 1981.
Piruska, A., Nikcevic, I., Lee, S. H., Ahn, C., Heineman, W. R., Limbach, P. A., and Seliskar, C. J.: The autofluorescence of plastic materials and chips measured under laser irradiation, Lab Chip, 5, 1348–1354, https://doi.org/10.1039/b508288a, 2005.
Plastics Europe AISBL: Plastics – the Facts 2022, Brussels, Belgium, 81 pp., https://plasticseurope.org/knowledge-hub/plastics-the-facts-2022/ (last access: 2 October 2023), 2022.
Pöhlker, C., Huffman, J. A., and Pöschl, U.: Autofluorescence of atmospheric bioaerosols – fluorescent biomolecules and potential interferences, Atmos. Meas. Tech., 5, 37–71, https://doi.org/10.5194/amt-5-37-2012, 2012.
Pöhlker, C., Huffman, J. A., Förster, J.-D., and Pöschl, U.: Autofluorescence of atmospheric bioaerosols: spectral fingerprints and taxonomic trends of pollen, Atmos. Meas. Tech., 6, 3369–3392, https://doi.org/10.5194/amt-6-3369-2013, 2013.
Poszwa, P., Kędzierski, K., Barszcz, B., and Nowicka, A. B.: Fluorescence confocal microscopy as effective testing method of polypropylene fibers and single polymer composites, Polym. Test., 53, 174–179, https://doi.org/10.1016/j.polymertesting.2016.05.025, 2016.
Prata, J. C.: Airborne microplastics: Consequences to human health?, Environ. Pollut., 234, 115–126, https://doi.org/10.1016/j.envpol.2017.11.043, 2018.
Prata, J. C., da Costa, J. P., Lopes, I., Duarte, A. C., and Rocha-Santos, T.: Environmental exposure to microplastics: An overview on possible human health effects, Sci. Total Environ., 702, 134455, https://doi.org/10.1016/j.scitotenv.2019.134455, 2020.
Primpke, S., Christiansen, S. H., Cowger, W., De Frond, H., Deshpande, A., Fischer, M., Holland, E. B., Meyns, M., O'Donnell, B. A., Ossmann, B. E., Pittroff, M., Sarau, G., Scholz-Böttcher, B. M., and Wiggin, K. J.: Critical Assessment of Analytical Methods for the Harmonized and Cost-Efficient Analysis of Microplastics, Appl. Spectrosc., 74, 1012–1047, https://doi.org/10.1177/0003702820921465, 2020.
Rochman, C. M., Kross, S. M., Armstrong, J. B., Bogan, M. T., Darling, E. S., Green, S. J., Smyth, A. R., and Veríssimo, D.: Scientific Evidence Supports a Ban on Microbeads, Environ. Sci. Technol., 49, 10759–10761, https://doi.org/10.1021/acs.est.5b03909, 2015.
Royer, S.-J., Ferrón, S., Wilson, S. T., and Karl, D. M.: Production of methane and ethylene from plastic in the environment, PLoS One, 13, e0200574, https://doi.org/10.1371/journal.pone.0200574, 2018.
Sauvageat, E., Zeder, Y., Auderset, K., Calpini, B., Clot, B., Crouzy, B., Konzelmann, T., Lieberherr, G., Tummon, F., and Vasilatou, K.: Real-time pollen monitoring using digital holography, Atmos. Meas. Tech., 13, 1539–1550, https://doi.org/10.5194/amt-13-1539-2020, 2020.
Savage, N. J., Krentz, C. E., Könemann, T., Han, T. T., Mainelis, G., Pöhlker, C., and Huffman, J. A.: Systematic characterization and fluorescence threshold strategies for the wideband integrated bioaerosol sensor (WIBS) using size-resolved biological and interfering particles, Atmos. Meas. Tech., 10, 4279–4302, https://doi.org/10.5194/amt-10-4279-2017, 2017.
Schepanski, K.: Transport of Mineral Dust and Its Impact on Climate, Geosciences-Basel, 8, 151, https://doi.org/10.3390/geosciences8050151, 2018.
Schmid, C., Cozzarini, L., and Zambello, E.: Microplastic's story, Mar. Pollut. Bull., 162, 111820, https://doi.org/10.1016/j.marpolbul.2020.111820, 2021.
Schumann, U., Weinzierl, B., Reitebuch, O., Schlager, H., Minikin, A., Forster, C., Baumann, R., Sailer, T., Graf, K., Mannstein, H., Voigt, C., Rahm, S., Simmet, R., Scheibe, M., Lichtenstern, M., Stock, P., Rüba, H., Schäuble, D., Tafferner, A., Rautenhaus, M., Gerz, T., Ziereis, H., Krautstrunk, M., Mallaun, C., Gayet, J.-F., Lieke, K., Kandler, K., Ebert, M., Weinbruch, S., Stohl, A., Gasteiger, J., Groß, S., Freudenthaler, V., Wiegner, M., Ansmann, A., Tesche, M., Olafsson, H., and Sturm, K.: Airborne observations of the Eyjafjalla volcano ash cloud over Europe during air space closure in April and May 2010, Atmos. Chem. Phys., 11, 2245–2279, https://doi.org/10.5194/acp-11-2245-2011, 2011.
Seinfeld, J. H. and Pandis, S. N.: Atmospheric Chemistry and Physics: From Air Pollution to Climate Change, 3rd edn., John Wiley & Sons, Inc., 1152 pp., ISBN: 978-1-118-94740-1, 2016.
Shadpour, H., Musyimi, H., Chen, J., and Soper, S. A.: Physiochemical properties of various polymer substrates and their effects on microchip electrophoresis performance, J. Chromatogr. A, 1111, 238–251, https://doi.org/10.1016/j.chroma.2005.08.083, 2006.
Shim, W. J., Hong, S. H., and Eo, S. E.: Identification methods in microplastic analysis: a review, Anal. Methods-UK, 9, 1384–1391, https://doi.org/10.1039/C6AY02558G, 2017.
Sinkhonde, D., Rimbarngaye, A., Kone, B., and Herring, T. C.: Representativity of morphological measurements and 2-d shape descriptors on mineral admixtures, Results in Engineering, 13, 100368, https://doi.org/10.1016/j.rineng.2022.100368, 2022.
Spizzichino, V., Caneve, L., Colao, F., and Ruggiero, L.: Characterization and discrimination of plastic materials using laser-induced fluorescence, Appl. Spectrosc., 70, 1001–1008, https://doi.org/10.1177/0003702816641267, 2016.
Stuart, B. O.: Deposition and clearance of inhaled particles, Environ. Health Persp., 55, 369–390, https://doi.org/10.1289/ehp.8455369, 1984.
Sun, J., Dai, X., Wang, Q., van Loosdrecht, M. C. M., and Ni, B. J.: Microplastics in wastewater treatment plants: Detection, occurrence and removal, Water Res., 152, 21–37, https://doi.org/10.1016/j.watres.2018.12.050, 2019.
Sun, Y., Yuan, J., Zhou, T., Zhao, Y., Yu, F., and Ma, J.: Laboratory simulation of microplastics weathering and its adsorption behaviors in an aqueous environment: A systematic review, Environ. Pollut., 265, 114864, https://doi.org/10.1016/j.envpol.2020.114864, 2020.
Tan, M. and Le, Q. V.: EfficientNet: Rethinking Model Scaling for Convolutional Neural Networks, in: Proceedings of the 36th International Conference on Machine Learning Research, edited by: Chaudhuri, K. and Salakhutdinov, R., PMLR, 97, 6105–6114, https://proceedings.mlr.press/v97/tan19a.html (last access: 12 Septemebr 2023), 2019.
Thompson, R. C.: Lost at Sea: Where Is All the Plastic?, Science, 304, 838–838, https://doi.org/10.1126/science.1094559, 2004.
Touloupas, G., Lauber, A., Henneberger, J., Beck, A., and Lucchi, A.: A convolutional neural network for classifying cloud particles recorded by imaging probes, Atmos. Meas. Tech., 13, 2219–2239, https://doi.org/10.5194/amt-13-2219-2020, 2020.
van der Walt, S., Schönberger, J. L., Nunez-Iglesias, J., Boulogne, F., Warner, J. D., Yager, N., Gouillart, E., and Yu, T.: scikit-image: image processing in Python, PeerJ, 2, e453, https://doi.org/10.7717/peerj.453, 2014.
Verschoor, A., De Poorter, L., Dröge, R., Kuenen, J., and de Valk, E.: Emission of microplastics and potential mitigation measures: Abrasive cleaning agents, paints and tyre wear, Rijksinstituut voor Volksgezondheid en Milieu RIVM, RIVM Report 2016-0026, 2016.
Weinzierl, B., Ansmann, A., Prospero, J. M., Althausen, D., Benker, N., Chouza, F., Dollner, M., Farrell, D., Fomba, W. K., Freudenthaler, V., Gasteiger, J., Groß, S., Haarig, M., Heinold, B., Kandler, K., Kristensen, T. B., Mayol-Bracero, O. L., Müller, T., Reitebuch, O., Sauer, D., Schäfler, A., Schepanski, K., Spanu, A., Tegen, I., Toledano, C., and Walser, A.: The Saharan Aerosol Long-Range Transport and Aerosol–Cloud-Interaction Experiment: Overview and Selected Highlights, B. Am. Meteorol. Soc., 98, 1427–1451, https://doi.org/10.1175/BAMS-D-15-00142.1, 2017.
Yu, J. T., Diamond, M. L., and Helm, P. A.: A fit-for-purpose categorization scheme for microplastic morphologies, Integr. Environ. Asses., 19, 422–435, https://doi.org/10.1002/ieam.4648, 2023.
Zhang, K., Hamidian, A. H., Tubić, A., Zhang, Y., Fang, J. K. H., Wu, C., and Lam, P. K. S.: Understanding plastic degradation and microplastic formation in the environment: A review, Environ. Pollut., 274, 103118, https://doi.org/10.1016/j.envpol.2021.116554, 2021.
Zhang, Y., Kang, S., Allen, S., Allen, D., Gao, T., and Sillanpää, M.: Atmospheric microplastics: A review on current status and perspectives, Earth-Sci. Rev., 203, 103118, https://doi.org/10.1016/j.earscirev.2020.103118, 2020.
Zhao, Y., Long, J., Zhuang, P., Ji, Y., He, C., and Wang, H.: Transforming polyethylene and polypropylene into nontraditional fluorescent polymers by thermal oxidation, J. Mater. Chem. C, 10, 1010–1016, https://doi.org/10.1039/D1TC05520H, 2022.