the Creative Commons Attribution 4.0 License.
the Creative Commons Attribution 4.0 License.
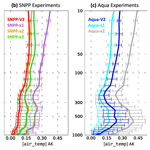
An information content approach to diagnosing and improving CLIMCAPS retrieval consistency across instruments and satellites
Christopher D. Barnet
The Community Long-term Infrared Microwave Combined Atmospheric Product System (CLIMCAPS) characterizes the atmospheric state as vertical profiles of temperature, water vapor, CO2, CO, CH4, O3, HNO3 and N2O, together with a suite of Earth surface and cloud properties. The CLIMCAPS record spans more than 2 decades (2002–present) because it utilizes measurements from a series of hyperspectral infrared sounders on different satellite platforms. In this paper, we take a stepwise approach to diagnosing CLIMCAPS V2 with the goal of identifying which Bayesian retrieval components to improve for a future V3 release. CLIMCAPS is based on the NASA (National Aeronautics and Space Administration) heritage retrieval approach and is the first system to extend the Aqua record with sounders on next-generation platforms in the same orbit. With the baseline quality of CLIMCAPS V2 soundings well-established, the objective of a V3 upgrade is to improve retrieval consistency across the different instruments and platforms for the sake of a seamless global record of atmospheric soundings. We demonstrate how the retrieval averaging kernels (AKs) are key metrics in diagnosing a multi-instrument system such as CLIMCAPS, and we conclude with the recommendation to upgrade the channel subsets and radiative transfer model error spectrum used in defining the Bayesian measurement error covariance matrix.
- Article
(10930 KB) - Full-text XML
-
Supplement
(411 KB) - BibTeX
- EndNote
The launch of Aqua on 4 May 2002 heralded in a new era for satellite sounding of the Earth atmosphere with the hyperspectral Atmospheric Infrared Sounder (AIRS; Aumann et al., 2003; Chahine et al., 2006). AIRS was the first of its kind in space, and it measures emitted infrared (IR) radiance with hundreds of spectrally narrow channels and low instrument noise. Its high spectral resolution allows AIRS to measure a wide range of parameters about the thermodynamic structure and chemical composition of the atmosphere (Susskind et al., 2003). More than 21 years later and still in operational orbit, Aqua continues to contribute important Earth system measurements (Parkinson, 2022). But, with only a few years before the spacecraft is decommissioned, our focus switched to ways that would see the Aqua record continue with next-generation instruments and platforms.
The Community Long-term Infrared Microwave Combined Atmospheric Product System (CLIMCAPS) retrieves atmospheric soundings from hyperspectral infrared (IR) measurements, like those made by AIRS. CLIMCAPS soundings are profiles of temperature, water vapor and a host of minor gases (CO2, CO, CH4, O3, N2O, HNO3) as well as cloud and Earth surface properties (Smith and Barnet, 2023a). CLIMCAPS augments measurements from AIRS with those from AMSU (Advanced Microwave Sounding Unit), also on the Aqua spacecraft, to help distinguish whether a retrieval scene is uniformly clear or uniformly cloudy. CLIMCAPS builds on decades of investment in sounding science to extend the Aqua retrieval record with CrIS (Cross-track Infrared Sounder) and ATMS (Advanced Technology Microwave Sounder) on the Suomi National Polar-orbiting Partnership (SNPP) and Joint Polar Satellite System series (JPSS-1 to JPSS-4, denoted JPSS+). CLIMCAPS is the first system to explicitly address instrument differences in its retrieval approach for the sake of a continuous record between AIRS+AMSU and CrIS+ATMS (Smith and Barnet, 2023a). We use the term “continuous” to mean a data record that is consistent in its characterization of natural variation despite changes in source instrumentation.
The CLIMCAPS retrieval approach has its origin in the NASA sounder retrieval method (Susskind et al., 2003), which deviates from traditional Bayesian optimal estimation (OE; Rodgers, 2000) most notably in its dynamic, scene-dependent regularization approach that applies singular value decomposition (SVD) to the signal-to-noise ratio (SNR) matrix (see Smith and Barnet, 2020, for details). Our focus in this paper is on the CLIMCAPS V2 product suite released by GES DISC (Goddard Earth Sciences Data and Information Science Center) in 2020, which is the only data record to date that spans the combined lifetimes of AIRS+AMSU and CrIS+ATMS with a consistent retrieval approach.
CLIMCAPS has been supported by a series of competitive NASA grants, each funding, at most, the full-time equivalent (FTE) of one expert for 3 years. Product roll-out, evaluation and improvement, therefore, have to manifest within a collaborative community. Each CLIMCAPS sounding is made up of many Earth system parameters (see Table 1 in Smith and Barnet, 2023a) that collectively characterize the global atmosphere twice daily. With such a data load that already spans more than 2 decades, typical validation studies (Nalli et al., 2018a, b; Wang et al., 2020; Yue et al., 2022) are not only resource-intensive, but also fall far short of providing a comprehensive analysis of the overall retrieval quality, especially within an ever-changing climate system. For CLIMCAPS to meaningfully support its wide range of science applications (e.g., Gaudel et al., 2024; Ouyed et al., 2023; Prange et al., 2023), end users need to be equipped with the diagnostic metrics and documentation to enable correct interpretation within data applications (e.g., Smith and Barnet, 2023b). The work described in this paper is testimony to the value of such an interactive science community that fosters dialogue between developer and stakeholder. What we learned is that a future CLIMCAPS V3 release should, first and foremost, improve upon the baseline consistency we established in V2 (Smith and Barnet, 2020) for the sake of higher product accuracy in support of long-term, large-scale studies.
The format of this paper is unconventional in that we do not simply list our experimental methodology, but instead we discuss how we arrived at the methodology in the first place. CLIMCAPS is a complex retrieval system with many component parts, so it is not straightforward to identify which components to address first, given our goals for a V3 upgrade. We, therefore, include a discussion of the systematic approach we took to diagnosing and improving retrieval consistency to help foster confidence in the product and promote accurate interpretation. So, in Sect. 2 we summarize the CLIMCAPS components that afford us a diagnostic evaluation of its retrieval products. In Sect. 3, we first justify then outline our experimental design, and in Sect. 4 we discuss results. Section 5 summarizes our conclusions for future system improvements. The analysis presented in this paper uses CLIMCAPS retrievals from Suomi-NPP CrIS+ATMS and Aqua AIRS+ATMS measurements made in 2016 when both instrument suites were in operational orbit at full spectral resolution. Henceforth, we distinguish these two systems as “CLIMCAPS-Aqua” and “CLIMCAPS-SNPP”. Note that a demonstration of continuity between CLIMCAPS-Aqua and CLIMCAPS-SNPP implies continuity with CLIMCAPS-JPSS+, since all four JPSS platforms, JPSS-1 through JPSS-4, have the same CrIS+ATMS instruments. This means that the CLIMCAPS sounding record has the potential to span at least 4 decades, 2002 to ∼ 2040.
The full CLIMCAPS record was made publicly available for the first time in 2020 as Version 2 (Table S1 in the Supplement). Where the initial development of CLIMCAPS drew on decades of stovepiped efforts at NASA and NOAA (National Oceanic and Atmospheric Administration), the full CLIMCAPS V2 record, now in the public domain, allows us to make targeted upgrades using diagnostic criteria suited to known product applications. This section describes the algorithm elements we employed to address systematic instrument differences in the CLIMCAPS V2 record. Detailed descriptions of the CLIMCAPS retrieval approach can be found elsewhere (Smith and Barnet, 2019, 2020, 2023a, b). Of interest here is the fact that CLIMCAPS generates a myriad of quantitative metrics for in-depth evaluation of the retrieval system and a sounder science approach to algorithm upgrades.
2.1 Instrument-specific algorithm components
CLIMCAPS is instrument agnostic at its core, which enables the same code to run all configurations, eliminating many potential discontinuities due to version control. Instrument design directly affects measurement information content and, therefore, retrieval quality. For example, spectral coverage determines which atmospheric gases can be retrieved, while spectral resolution affects their retrieval quality. Many of the Level-1 product differences are addressed and neutralized in the CLIMCAPS pre-processor, but fundamental instrument differences that affect measurement SNR propagate systematically into the retrieved quantities. As a result, instrument biases can disrupt the geophysical consistency of a long-term data record even if a retrieval system is instrument agnostic. AIRS is a grating spectrometer (Aumann et al., 2003; Chahine et al., 2006) and CrIS an interferometer (Glumb et al., 2002; Strow et al., 2013). Smith and Barnet (2019) summarized these two instruments from a retrieval perspective, and we also elaborate on a few key aspects in Table S1 of this paper. Given these fundamental instrument differences, one has to consider AIRS and CrIS spectral resolutions and how their instrument design affects the fecundity of spectral channels. But IR spectra do not provide the only source of information. CLIMCAPS additionally harvests spatial information from the 3 × 3 cluster of instrument fields of view (FOVs) making up each retrieval's field of regard (FOR), as well as spectral information from the colocated microwave (MW) sounders, AMSU on Aqua and ATMS on SNPP and JPSS+. The reason for this is that retrieval parameters can be mathematically degenerate within a single source of measurement. Adding other sources can help break this degeneracy to allow for the retrieval of discrete parameters (see Table 1 in Smith and Barnet, 2023a, for a full list). A good example is the degeneracy of cloud and surface parameters within the IR radiances. Adding MW and spatial information to the retrieval process helps CLIMCAPS to distinguish between cloud tops and Earth's surface. While the IR sounders provide the primary source of spectral information, one should always keep in mind that the spatial arrangement of IR FOVs and the quality of colocated MW measurements also affect CLIMCAPS retrieval quality.
AIRS and CrIS each have hundreds of spectral channels measuring IR radiation in narrow intervals. In CLIMCAPS, we do not use all the available IR channels for each parameter retrieval, because there is no reason to include channels insensitive to the target parameter that would be filtered out during dynamic SVD regularization anyway. So, for the sake of retrieval speed and improved SNR, we pre-select subsets of channels for each target variable as discussed in Gambacorta and Barnet (2013). The channel subsets we implemented in CLIMCAPS V2 for AIRS and CrIS reflect best practices currently at NASA and NOAA for each instrument configuration (Table S2). But persistent differences in averaging kernels (AKs) between CLIMCAPS-Aqua and CLIMCAPS-SNPP (Smith and Barnet, 2020) reflect systematic effects in SNR that could be traced back to CLIMCAPS V2 channel subsets. We, therefore, revisit the V2 channel sets in this paper and make recommendations for upgrades to improve the consistency in CLIMCAPS retrieval quality across AIRS+AMSU and CrIS+ATMS. Note that we select the CLIMCAPS channel sets from the full range that is available for CrIS (2211 in total). For AIRS, however, the full channel set (2378 in total) is first reduced into a pristine list (less than 1600 after subsetting) ahead of channel selection to remove channels with measurable noise effects due to, for example, large thermal cycles in orbit or on Earth (Manning et al., 2020).
2.2 Diagnostic metrics
CLIMCAPS yields a number of diagnostic metrics that can be used to evaluate its data record. For this study, we predominantly employ three types of metrics to analyze the degree to which retrievals from the two different instrument suites, AIRS+AMSU and CrIS+ATMS, are consistent in space and time. These are (i) cloud-clearing metrics that quantify the random and systematic error introduced by clouds; (ii) AKs and degrees of freedom of signal (DOFS) that quantify measurement SNR; and (iii) the difference between the final retrieval and a priori estimate, which we will refer to as ADIFF from here on. AKs quantify the degree to which a solution depends on the measurement. It is a unitless quantity where zero means that the solution is equal to the a priori estimate and where one means that the solution is entirely derived from the measurements without any contribution from the a priori estimate. A metric that complements the AKs is the degree to which the solution deviates from the a priori estimate, i.e., ADIFF. This is an especially informative metric in CLIMCAPS where all the a priori estimates are independent of the instrument measurements (Smith and Barnet, 2019). For temperature, water vapor and O3, the CLIMCAPS a priori estimate is dynamically defined by the reanalysis model, MERRA-2 (Gelaro et al., 2017; GMAO, 2015), that CLIMCAPS interpolates in time and space to each instrument footprint ahead of retrieval. For the well-mixed gases (CO2, N2O and CH4), we use estimates of their long-term trends across seasons and hemispheres. The CLIMCAPS CO a priori estimate is a seasonal and inter-hemispheric climatology, and for HNO3 and SO2 we have a single static profile at this time. As knowledge of these gases grows, we can consider developing new climatologies and/or employing other chemistry or reanalysis models. Having a priori estimates that are instrument independent is one of the key aspects that enables continuity in the CLIMCAPS record since they help avoid abrupt changes when new instrument measurements are introduced or when SNR is low. A CLIMCAPS retrieval typically deviates from its a priori estimate when the measurements add new information about the true state of the atmosphere within the FOR. On rare occasions, however, a retrieval can be dominated by noise when SNR is very low. One can diagnose CLIMCAPS retrievals to better understand the reasons for > 0 values using the AK matrices that are reported for every retrieval parameter at every FOR in the Level 2 product. These are discussed in detail in Smith and Barnet (2020) but are worth summarizing here:
- 1.
High AK, high ADIFF. Measurement sensitivity is high and updates the a priori estimate with new information about the target variable. This is by far the largest category, defining ∼ 79 % of all retrievals on any given day of measurements. These retrievals are typically flagged as “successful” in that they pass all CLIMCAPS quality control (QC) thresholds.
- 2.
High AK, low ADIFF. Measurement sensitivity is high and agrees with the a priori representation of the target variable. This is the second largest category (∼ 17 %) of CLIMCAPS retrievals.
- 3.
Low AK, low ADIFF. Measurement sensitivity is low, so the retrieval predominantly resembles the a priori estimate. This is the smallest category, defining only ∼ 1% of retrievals on any given day.
- 4.
Low AK, high ADIFF. Measurement sensitivity to the target variable is low, and the spurious effects visible in ADIFF are predominantly errors. The CLIMCAPS QC filters typically flag retrievals in this category as “failed”.
When we evaluate retrieval continuity between CLIMCAPS-Aqua and CLIMCAPS-SNPP, we typically want to see similar space-time patterns in these metrics as it would mean that CLIMCAPS maintains consistency in retrieval SNR despite changes in instrumentation.
3.1 Background and rationale
CLIMCAPS retrieves its large suite of atmospheric state parameters sequentially in a series of steps (Smith and Barnet, 2023a). This stepwise approach holds many advantages, not least because it helps minimize the size of the a priori error covariance matrix employed during retrieval for the sake of a computationally efficient algorithm that can support both real-time and full mission applications. Of primary concern to the CLIMCAPS V2 data record, however, is how such a sequential approach enables targeted updates to the measurement error covariance matrix as knowledge of the true state and retrieval uncertainty grows (Smith and Barnet, 2019). The CLIMCAPS measurement error covariance matrix, Sm, is the sum of the radiance error covariance matrix, δRδRT, and radiance uncertainty due to the background atmospheric state, KTSbK, where K is the Jacobian and Sb the background state error covariance matrix (Smith and Barnet, 2019, 2020). All atmospheric state parameters held constant during any given retrieval step are considered background parameters. CLIMCAPS retrieves clouds first, followed by temperature Tair and water vapor H2Ovap in that order (Smith and Barnet, 2023a). Being first, the cloud properties are retrieved using only a priori, not retrieved, estimates of the clear atmospheric state. Similarly, Sm is, at first, defined only by a priori estimates of radiance and background state uncertainty. After retrieval of the cloud parameters, the IR radiance measurements are cloud cleared (Smith and Barnet, 2023b) to allow for sounding retrievals of the full, clear atmospheric column (i.e., characterization of atmospheric state past, not through, clouds). During this step (and each subsequent step), retrieval uncertainty is quantified and then propagated to the next step. Note that cloud clearing is the only step that updates the radiance uncertainty directly. In all subsequent steps, Sm will incorporate the cloud-cleared radiance uncertainty instead of the a priori measurement uncertainty δRδRT (see Eq. 5 in Smith and Barnet, 2023b). Once Tair is retrieved, KTSbK is updated with the retrieved uncertainty of Tair. More generally, a CLIMCAPS retrieval at step x + 3 uses a background state with a priori estimates replaced by the retrieved parameters from steps x, x + 1 and x + 2. In other words, the stepwise CLIMCAPS approach uses scene-specific signal and noise estimates as soon as they become available so that the a priori state is gradually updated to represent retrieved conditions within the FOR. The main purpose of this stepwise retrieval approach is to improve the efficiency with which the radiance measurement can be decomposed into discrete signals (Smith and Barnet, 2019).
The sequence and number of CLIMCAPS retrieval steps are not fixed but can be arranged to suit target applications. The CLIMCAPS V2 sequence broadly progresses as follows:
- i.
Clouds are by far the largest spectral signature and affect most of the measured spectral range, so cloud-cleared radiances are retrieved first.
- ii.
Tair is spectrally the most linear of all sounding parameters, and it is important to have a stable, accurate representation of Tair before attempting H2Ovap and trace gas retrievals, so Tair is retrieved ahead of all trace gases.
- iii.
H2Ovap is the largest perturbation after clouds and Tair. Water vapor is also pervasive in the whole spectrum. This is why we retrieve H2Ovap after the first Tair retrieval and why it precedes all the other gases.
- iv.
O3 is somewhat nonlinear but has second-order effects on Tair and H2Ovap, so it is retrieved next.
- v.
CO is highly linear with negligible impacts on other parameters, so it is retrieved ahead of all the remaining minor gases.
- vi.
HNO3 is linear and impacts Tair in polar regions, so it follows the CO retrieval.
- vii.
Tair is then retrieved a second time from the same MERRA-2 a priori estimate to capture nonlinearities due to retrieved (not a priori) knowledge of the value and error in H2Ovap and O3.
- viii.
Finally, CO2, N2O and S2O are retrieved in this sequence. Their spectral signatures are mostly linear but very weak (i.e., with low information content) relative to Tair, H2Ovap, O3, CO and HNO3.
Of primary importance in the CLIMCAPS product suite are the atmospheric profile retrievals from cloud-cleared radiances, so our experiment will start with an evaluation of cloud-clearing uncertainty metrics that propagate and affect measurement SNR in all subsequent steps. Cloud clearing is a well-established method for addressing the way clouds affect IR radiation through the atmosphere (Chahine, 1977; Susskind et al., 1998) and is implemented in CLIMCAPS V2 as discussed in Smith and Barnet (2023b). In short, cloud clearing aggregates the clear-sky radiance signal from each cluster of 3 × 3 FOVs (∼ 15 km at nadir) to retrieve a single cloud-cleared radiance spectrum that represents the atmosphere within the larger FOR (∼ 45 km at nadir). Figure 1 depicts the key uncertainty metrics CLIMCAPS generates during its cloud-clearing step, namely, etarej
and ampl_eta. The former quantifies the bias in retrieved cloud-cleared radiance and the latter the degree to which random instrument noise is affected within the aggregated FOR. If there are differences in the degree to which instruments measure atmospheric clouds, we expect to see them amplified in these uncertainty metrics. The swath width of CLIMCAPS-SNPP (Fig. 1a and c) is slightly wider than CLIMCAPS-Aqua (Fig. 1b and d), hence the smaller data gaps at lower latitudes. Otherwise, we see that etarej
and ampl_eta are largely consistent between CLIMCAPS-SNPP (Fig. 1a and c) and CLIMCAPS-Aqua (Fig. 1b and d) when aggregated onto a 1° equal-angle grid. Note the similarities in the range of values as well as the absence of any view angle effects (i.e., uncertainty does not increase with view angle). Cloud clearing introduces systematic uncertainty (etarej
> 0) and amplifies random instrument noise (ampl_eta > 1) wherever clouds are present. However, cloud clearing reduces random instrument noise (ampl_eta < 1) when clouds are absent, and a cluster of measurement FOVs is simply averaged into a single FOR radiance spectrum (Smith and Barnet, 2023b). Figure 1c and d show that ampl_eta < 1 for much of the globe on any given day.
The differences that do exist between CLIMCAPS-SNPP and CLIMCAPS-Aqua with respect to ampl_eta and etarej
can primarily be attributed to the time difference in observation between the two satellites, as clouds can change significantly over short times. While Aqua and SNPP both have local overpass times of 01:30/13:00 UTC equatorial crossing times, the difference in their orbital altitude means their measurements are offset by a few minutes. In addition, there are small spatial shifts in how AIRS and CrIS observe clouds across their fields of regard (FORs) since the AIRS and CrIS FOVs are not spatially coregistered. What we mean by this is that the 3 × 3 AIRS FOVs could observe a large radiance gradient compared to CrIS simply because of the sampling differences between the two instruments. Moreover, the CrIS FOVs rotate with respect to each other as a function of view angle, while the AIRS FOVs maintain their alignment with the cross-track scan direction irrespective of view angle. This means that AIRS and CrIS do not necessarily observe the same cloud structure within each FOR. Even if the instruments are exactly the same (such as CrIS on SNPP and JPSS+), one cannot compare their retrievals directly due to differences in instrument sampling, both spatially and temporally. This is true for all retrieval approaches, whether they employ cloud clearing or not. In CLIMCAPS, we only provide products in the overlap period between satellites (see Table 2) for product evaluation where this aspect is properly accounted for. Overall, the large-scale similarities observed in Fig. 1 make sense, because cloud clearing neither depends on spectral information content where most of the instrument differences manifest nor depends on any a priori knowledge of clouds that would amplify sampling differences. Instead, cloud clearing uses spatial information content (quantified as measurement variation across a cluster of FOVs) to derive an aggregate cloud-cleared spectrum (Smith and Barnet, 2023b). AIRS and CrIS FOVs are ∼ 14 km at nadir, and each instrument has nine FOVs, making up an ∼ 50 km cloud-cleared FOR. Moreover, both instruments have their FOVs arranged in 3 × 3 arrays, so they capture the available spatial information content in similar ways.
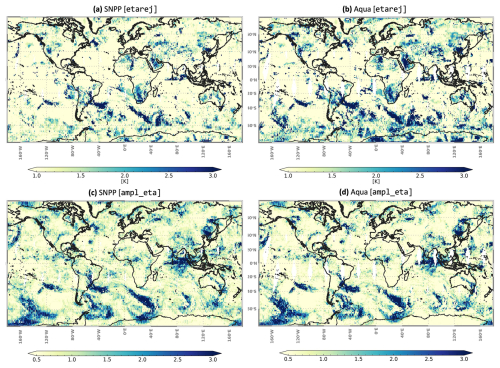
Figure 1CLIMCAPS V2 cloud-clearing error metrics between (a, c) SNPP and (b, d) Aqua Level 2 retrievals on 30 August 2016. These error metrics were binned and then averaged to a uniform 1° equal-angle global grid. (a, b) etarej
represents the systematic error due to an incomplete removal of cloud signals from the Level-1B radiances, and (c, d) ampl_eta
quantifies the degree to which random instrument noise is amplified (or reduced) due to cloud clearing.
For the purpose of this evaluation, there is no need to diagnose differences at finer spatial scales, because our objective at this stage is to identify (and address) the large-scale, systematic differences between CLIMCAPS-SNPP and CLIMCAPS-Aqua. Addressing differences at finer scales will be the focus of future work.
Moving on to the next retrieval step, Fig. 2 depicts CLIMCAPS Tair in the lower troposphere for the two instrument configurations. CLIMCAPS employs the Stand-alone AIRS Radiative Transfer Algorithm (SARTA; Strow et al., 2003) to simulate top-of-atmosphere radiances. SARTA was originally developed for AIRS but later adapted for CrIS. It is possible that SARTA introduces subtle effects into the retrieval product as a result of how it treats differences in instrument spectral correlations. We should note that SARTA is not funded to be maintained consistently for AIRS and CrIS. This means we can expect SARTA to introduce retrieval differences, which we will group under the umbrella of “instrument effects” for the sake of simplicity and clarity of argument in this paper. Another instrument difference that may manifest in the retrievals is the fact that AIRS requires a frequency correction – which is a function of orbital position and season (see Table S1 for more details). Figure 2 depicts no significant difference in the spatial patterns of Tair gradients, maxima and minima, between these two CLIMCAPS configurations.
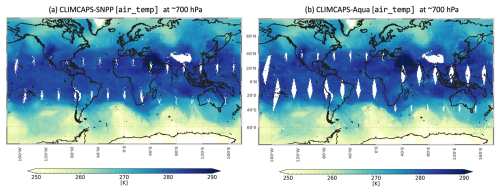
Figure 2CLIMCAPS V2 Tair retrievals from (a) SNPP and (b) Aqua measurements acquired on 30 August 2016. The CLIMCAPS Tair retrievals are made on 100 pressure levels spanning the vertical atmospheric column from Earth's surface to the top of the atmosphere (∼ 0.05 hPa). These panels represent all retrievals that passed quality control [ispare_2 = 0] and were made at ∼ 700 hPa. For display purposes, the values were binned and averaged to a 1° equal-angle global grid.
The results in Fig. 2 alone, however, do not satisfy the requirement for consistency, nor does it demonstrate that we have mitigated the differences between the Aqua and SNPP systems sufficiently. CLIMCAPS employs dynamic regularization, which means that it populates the true null space within each FOR with the a priori estimate. A measurement with large null space (or low information content and weak sensitivity to the target parameter) will yield a retrieval that approximates the a priori estimate, a feature that generally applies to all OE retrieval systems. CLIMCAPS prepares its a priori estimate for Tair by selecting the two MERRA-2 reanalysis fields on either side of the measurement in space and time and linearly interpolating them to the exact location. The CLIMCAPS V2 a priori estimate for Tair provides a good estimate of prevailing conditions even if no new information is contributed by the measurement. At this scale, we thus expect the MERRA-2 a priori estimate to neutralize any differences in observing capability between SNPP and Aqua. For an evaluation of retrieval consistency, we instead turn our attention to diagnosing the CLIMCAPS uncertainty metrics that quantify retrieval SNR where Aqua and SNPP instruments would typically manifest. For this reason, all the results discussed in subsequent sections focus on an evaluation of CLIMCAPS V2 uncertainty, not its retrievals. This is a major departure from standard validation techniques that compare geophysical quantities from multiple, independent sources (e.g., Nalli et al., 2018a, b.; Wang et al., 2020). It is important to distinguish these two approaches and recognize their divergent end goals. This said, the evaluation of uncertainty metrics presented in this paper is made possible largely because of the CLIMCAPS algorithm and product design. Prior to 2020, we did not have access to more than 20 years of AKs from the same system across different instrument suites. Moreover, the fact that CLIMCAPS employs instrument-independent a priori estimates means that retrieval AKs and ADIFF can be interpreted in a straightforward manner.
Having established the target metrics for our evaluation in this paper, Fig. 3 addresses questions regarding view angle and time of day, both of which potentially affect signal strength and thus continuity across instruments. We use CLIMCAPS-Aqua on 30 August 2016 as illustration, but the same holds for CLIMCAPS-SNPP on the same day (not shown). The DOFS of a retrieval system with respect to a target variable is the sum total of all corresponding AK peaks (or the trace of the AK matrix). When mapped out, as in Fig. 3a and b, DOFS provide an efficient way of summarizing information content patterns. It is immediately obvious that the CLIMCAPS-Aqua DOFS for Tair do not increase with view angle, nor do they change dramatically between the AM and PM orbits. We can attribute this consistency in CLIMCAPS DOFS across all view angles and times of day to the efficiency with which CLIMCAPS applies dynamic regularization. In a retrieval system without such an ability, one may see the DOFS increase with view angle, because at high angles the measurements observe a larger portion of the atmosphere and have more spectral channels sensitive to conditions in the middle to lower troposphere. One can think of this as the channels traversing a thicker atmosphere at higher view angles due to a larger slant path, which causes the AK sensitivities to move higher up in the atmosphere. Channel sensitivity to the boundary layer at nadir may be sensitive to the mid-troposphere at the edge of the scan (50° for CrIS, 49.5° for AIRS). This means that the channels we select for profile retrievals need to have sensitivities that span the full vertical column at all view angles. At nadir, many of the channels may become sensitive to surface conditions where CLIMCAPS error estimates are also high (due to larger errors in cloud, surface skin temperature and emissivity). For such channels, their information contribution may be minimal at nadir. At higher view angles, however, their SNR may improve due to decreasing errors in emissivity and surface skin temperature at higher altitudes (lower atmospheric pressure). There is similar variability in channel SNR with changes in atmospheric conditions (e.g., lapse rate) and seasonal cycles. The dynamic regularization approach we adopted in CLIMCAPS allows for access to channel information content whenever and wherever it is available. Stated differently, retrieval systems that employ static regularization (e.g., Rodgers, 2000) limit instrument spectral information in many cases, because their a priori assumptions and parameterization may be outdated or fail to reflect localized conditions.
The ADIFF for CLIMCAPS temperature at 700 hPa (Fig. 3c and d) complements the DOFS maps (Fig. 3a and b) and illustrates that the degree to which CLIMCAPS adjusts the MERRA-2 a priori with information from the measurements is consistent across land and ocean, night and day, and nadir and the edge of the scan. Figure 3e depicts the vertical information content for seven CLIMCAPS retrieval variables: Tair, H2Ovap, O3, CO, CH4, CO2 and HNO3. These profiles represent the global average of the AK peaks (i.e., diagonal vector of AK matrix), with the error bars depicting the standard deviation of SNR across all types of conditions on a global day. For each of the variables represented in Fig. 3e, the AK statistics are reported separately for the 01:30 and 13:30 orbits to demonstrate how time of day does not impose significant differences. Therefore, we focus our evaluation for the remainder of this paper on large ensembles of AKs that include all view angles and orbits to optimize continuity in the CLIMCAPS V3 product for all scenes across multiple satellites.
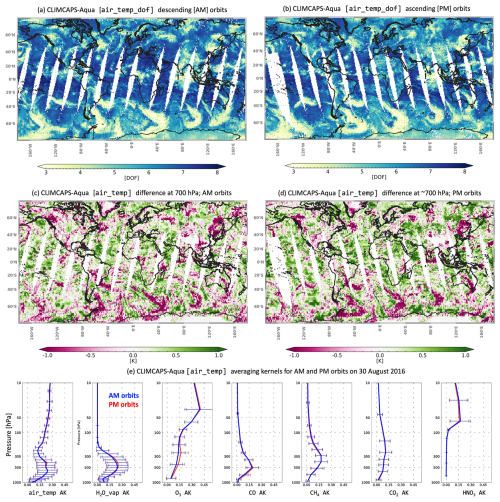
Figure 3CLIMCAPS information content as a function of (a–d) view angle and (e) time of day. (a) CLIMCAPS-Aqua DOFS for Tair from all FORs in descending orbits (13:30 UTC equatorial crossing time) on 30 August 2016. (c) CLIMCAPS-Aqua Tair difference between retrieval and a priori (MERRA-2) at ∼ 700 hPa for all descending orbits. (b, d) Same as (a, c) but for all ascending orbits (01:30 UTC equatorial crossing time). CLIMCAPS DOFS are independent of view angle and time of day (e) CLIMCAPS-Aqua Tair AK mean and standard deviation (error bars) from all (red) ascending FORs and (blue) descending FORs. The AKs represent the average (and standard deviation) of the diagonal vectors from the retrieval AK matrices. CLIMCAPS AK structure and variance are independent of time of day.
3.2 Experimental design
Table 1 summarizes the algorithm components that we identified as directly affecting retrieval consistency, specifically those of Tair, CO2 and O3 where instrument-specific inconsistencies have been reported (Smith and Barnet, 2020). In total, there are six algorithm components (column 1) reported for the two CLIMCAPS configuration currently implemented for V2 as well as how we propose to change them for a future version, V3. The values reported in columns x1, x2 and x3 represent the experimental configurations we analyze and discuss in Sect. 4. In summary, the six algorithm components we tested in this paper are as follows:
-
Bmax
is employed during CLIMCAPS regularization (see Smith and Barnet, 2020, for details). In short,Bmax
is an empirical term that informs the eigenvalue (λ) threshold as follows: . During regularization, CLIMCAPS applies SVD to the measurement SNR matrix, . All functions with λ ≥ λc are used in the retrieval without any regularization. Those functions with 0.05 < λ < λc are damped and all with λ < 0.05 are excluded from the retrieval. A largerBmax
imposes a lower threshold value (λc), which means a greater number of eigenfunctions pass into the retrieval without any regularization.Bmax
should not be too large because all measurements have noise to filter out, nor shouldBmax
be too small because all successful measurements have some information to contribute. Note that when we talk aboutBmax
in this paper, we mean theBmax
value employed specifically for Tair retrievals. -
RTAerr
quantifies the radiative transfer algorithm (RTA) bias that propagates into CLIMCAPS retrievals, whenever a radiative transfer calculation is made.RTAerr
applies to each channel individually and varies according to the accuracy of transmittance and radiance calculation within the RTA. TheRTAerr
associated with a specific RTA will typically decrease as the RTA matures. Historically, theRTAerr
is calculated offline using a large ensemble of data for specific instrument configurations. CLIMCAPS-SNPP uses the NOAARTAerr
calculated for CrIS, whereas CLIMCAPS-Aqua uses the NASARTAerr
calculated for AIRS. CLIMCAPS-Aqua and CLIMCAPS-SNPP use different versions of SARTA (due to funding limitations that inhibits synchronized SARTA upgrades across all instrument configurations), so we do not expectRTAerr
to be universal for all CLIMCAPS configurations. We list theRTAerr
values, averaged across all spectral channels, for CLIMCAPS-SNPP and CLIMCAPS-Aqua in Table 1 below. Note how the V2RTAerr
is, on average, an order of magnitude larger for CLIMCAPS-SNPP (∼ 0.5 K) than CLIMCAPS-Aqua (∼ 0.05 K). We investigate this disparity in Sect. 4 and demonstrate how a lowerRTAerr
for CLIMCAPS-SNPP improves retrieval quality. -
apodcor
defines the degree to which adjacent channels are correlated due to apodization of the CrIS interferograms (or radiance measurements). AIRS is a grating spectrometer (Table A1) and its measurements, therefore, are naturally apodized. CLIMCAPS applies Hamming apodization to CrIS measurements to impose a localized spectral response function and thus remove a significant portion of the geophysical noise otherwise present in the CrIS interferograms (Barnet et al., 2000, 2023). -
The last three rows summarize the number of channels within each spectral subset that CLIMCAPS uses in the retrieval of Tair, CO2 and O3, respectively. As with
RTAerr
, CLIMCAPS V2 development benefited from existing efforts at NOAA and NASA to inform these channel selections. We critically evaluate these V2 channel sets here and make recommendations for future upgrades.
Table 1Summary of the CLIMCAPS algorithm configurations discussed in this paper. Each configuration is unique with respect to the six algorithm parameters depicted in column 1. The V2 configurations represent the CLIMCAPS V2 record available via NASA GES DISC (Table 2). The x1, x2 and x3 configurations depict the experiments we performed, and V3 is the configuration we propose for the next CLIMCAPS release for AIRS+AMSU and CrIS+ATMS. Since CrIS+ATMS data are identical on the SNPP and JPSS platforms, the SNPP-V3 configuration depicted here also applies to JPSS-V3. The number of Tair channels reported here represent those selected from the longwave (LW) IR band only. As shown in Table S2, CLIMCAPS uses channels from all three bands for Tair retrievals.
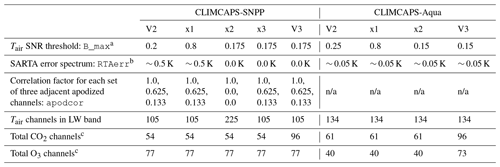
a Smith and Barnet (2020). b See Fig. 4c and Table S1 for more details. c See Fig. 5.
n/a: not applicable.
Table 2Summary of the full CLIMCAPS V2 record that is publicly available via the NASA data and information service center at the Goddard Spaceflight Center (or GES DISC).
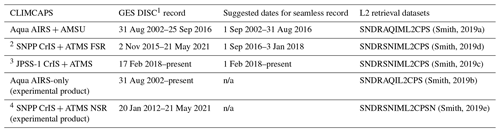
1 NASA Goddard Earth Sciences Data and Information Services Center, https://disc.gsfc.nasa.gov/. 2 SNPP Full Spectral Resolution (FSR) CrIS mode introduced to allow science-quality CO retrievals (Gambacorta et al., 2014). 3 The first of four Joint Polar Satellite System (JPSS) payloads in space. In operational mode, JPSS-1 is known as NOAA-20. 4 Suomi National Polar orbiting Partnership (SNPP) Normal Spectral Resolution (NSR) CrIS mode.
Figures 4 and 5 provide graphic depictions of the algorithm elements summarized in Table 1. Figure 4a contrasts the V2 channel subsets for Tair in CLIMCAPS-SNPP (red) and CLIMCAPS-Aqua (orange). This is not the full channel set but rather just those channels from the 660–760 cm−1 spectral range with sensitivity to temperature at pressure levels from Earth's surface to the top of the atmosphere. It is immediately obvious that there are significant differences in the channels sets for Tair between SNPP and Aqua. For the CLIMCAPS V2 implementation in 2019, we combined best practices at NOAA and NASA. The goal with all present and future upgrades to CLIMCAPS is to evolve the system to a place where the retrievals represent the observing capability common to all instruments making up the full record. The blue lines in Fig. 4a represent the x2 configuration we tested for CLIMCAPS-SNPP. For a full list of the CLIMCAPS V2 channel sets currently used for all retrieval parameters, see Table S2.
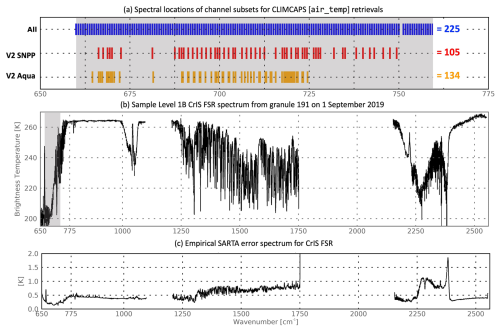
Figure 4(a) CLIMCAPS V2 spectral channel subsets used in Tair retrievals for Aqua (orange) and SNPP (red) in the (grey area) 1.4 µm CO2 absorption band. An experimental subset of channels used in the CLIMCAPS-SNPP x3 configuration (see Table 1) is in (blue). (b) Sample of CrIS full spectral resolution (FSR) measurement given by Level 1B product granule 191 on 1 September 2019 to contextualize the location of the 1.4 µm region in the CrIS longwave band (∼ 650–1100 cm−1). (c) CLIMCAPS V2 empirically derived error spectrum of SARTA forward model bias for CrIS FSR spectra.
In addition to channel set differences that affect CLIMCAPS SNR, there is also the SARTA forward model error, RTAerr
, typically calculated by the respective retrieval teams. Similar to the channel sets, RTAerr
evolved separately for SNPP and Aqua at NOAA and NASA, respectively. Figure 4c illustrates the NOAA RTAerr
for SNPP that we implemented in CLIMCAPS V2. Also in Fig. 4c is the RTAerr
for Aqua, but this spectrum is invisible due to it being orders of magnitude smaller than the SNPP RTAerr
, and thus off the scale in Fig. 4c (see Table 1, row 2). This tells us that RTAerr
is a potential source of discontinuity in the CLIMCAPS V2 record and needs to be updated and normalized across both system configurations for the sake of retrieval consistency across instrument suites. Of importance here is the large disparity in RTAerr
across the Aqua and SNPP configurations and not a detailed depiction of each RTAerr
individually.
Given known differences in CLIMCAPS V2 AKs for SNPP and Aqua (Smith and Barnet, 2020), we additionally diagnosed channel subsets for CO2 and O3 in this paper. Figure 5 illustrates differences in the SNPP and Aqua V2 channel sets for O3 and CO2 (orange) and how we propose to standardize them for a future V3 implementation (blue).
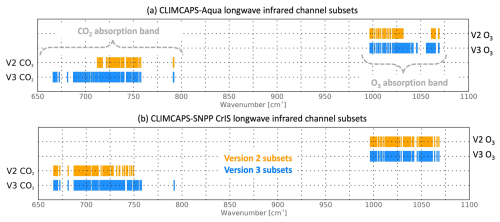
Figure 5Illustration of the channel subsets used for CO2 and O3 retrievals in the (orange) V2 and (blue) proposed V3 configurations for (a) CLIMCAPS-Aqua and (b) CLIMCAPS-SNPP (see Table 1).
In summary, we introduced and explained our experimental design, because the evaluation of retrieval consistency across different instrument suites is not straightforward. There is no universal retrieval approach that satisfies the SNR requirements of all applications, nor do we know exactly how instrument differences manifest under all conditions. The fact is that hyperspectral IR instruments measure a large array of atmospheric parameters that together characterize thermodynamic structure (i.e., temperature and water vapor profiles) and chemical composition (e.g., pollutant gas concentrations). But instead of a series of distinct spectral signatures, the result is a convolved IR measurement with complex inter-dependencies of both geophysical signal and noise. CLIMCAPS mitigates these complexities to a large degree with its sequential retrieval approach and subsets of channels that maximize the SNR for each target parameter. But even then, the result is not clear-cut, because instrument differences can cause spectral effects that are difficult to disassociate from the convolved geophysical signals. This means that one-to-one comparisons with independent datasets can be difficult to interpret. For a transparent evaluation of CLIMCAPS V2 retrieval consistency, we therefore focus on the analysis of CLIMCAPS uncertainty metrics instead. Table 1 summarizes all the algorithm components we tested, the results of which we present and discuss in Sect. 4 below.
In this section, we present results for each experimental configuration (Table 1) using available uncertainty metrics: AKs, DOFs and ADIFF. CLIMCAPS AKs typically have a large dynamic range across a day of retrievals, because measurement SNR varies from scene to scene with prevailing conditions, and CLIMCAPS regularization minimizes a priori dependence according to the information content of measurements.
Figure 6 depicts Tair AKs for the existing V2 record as well as each of the experimental configurations listed in Table 1. The profiles in Fig. 6 represent the average of all Tair AKs on 1 September 2019 (321 300 in total). The error bars represent the AK standard deviation. It is immediately obvious in Fig. 6a that there are systematic SNR differences between CLIMCAPS-SNPP (red) and CLIMCAPS-Aqua V2 (blue). Not only do the SNPP Tair AKs lack vertical structure, but they are also much lower in value and dynamic range compared to the Aqua Tair AKs. The cause of this disparity is not immediately obvious and could be due to fundamental instrument differences in spectral resolution, over-damping of the measurement within retrieval system or a combination of both.
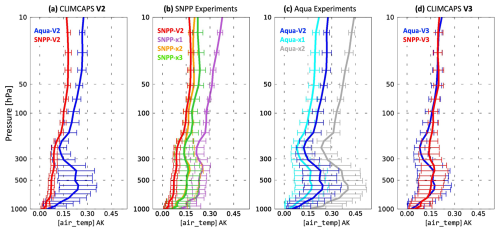
Figure 6Comparison of AKs to illustrate disparities in Tair information content structure and variance for (a) CLIMCAPS V2 (blue) Aqua and (red) SNPP, (b) three CLIMCAPS-SNPP experimental configurations (purple+orange+green) contrasted against the (red) V2 SNPP configuration, (c) two CLIMCAPS-Aqua experimental configurations (cyan+grey) against the (blue) V2 Aqua configuration, and (d) the proposed CLIMCAPS V3 configurations (blue) Aqua and (red) SNPP to improve Level 2 data continuity. (b, c) The experimental configurations referenced here are detailed in Table 1.
We can diagnose the causal factors influencing V2 Tair AK disparities by changing the retrieval configurations as listed in Table 1. Figure 6b contrasts the SNPP V2 Tair AK against three experimental configurations: x1, x2 and x3. When the SNPP Bmax
threshold is increased from 0.2 to 0.8 (i.e., V2 vs. x1), we see a dramatic jump in AK values across all pressure levels as well as a larger dynamic range in the mid-troposphere. As discussed in Sect. 3, Bmax
is derived empirically and informs the eigenvalue threshold (λc) that determines the number of eigenfunctions CLIMCAPS will damp (or leave undamped) in the retrieval step. Bmax
is considered too large (and its corresponding λc too small) when the AKs exhibit spurious effects and retrieval accuracy is low. This happens when CLIMCAPS uses too many eigenfunctions without any damping. An SVD of the measurement sensitivity matrix, , results in eigenfunctions arranged in order of signal strength such that higher-order eigenfunctions are associated with signal and lower-order functions are dominated by noise. When lower-order eigenfunctions are not sufficiently damped, then their noise propagates into the retrievals as error. Similarly, a Bmax
value is considered too small (and λc too large) when too many higher-order eigenfunctions are damped and not enough measurement information passes on to the retrieval. In such a case, the AKs will be small with a low dynamic range, and retrievals will mostly represent the a priori.
What is evident in Fig. 6b is that Bmax
alone does not explain the low AK values for CLIMCAPS-SNPP V2 (red). Both the x2 and x3 configurations for CLIMCAPS-SNPP (green and orange) have slightly lower Bmax
values (0.175), yet higher AKs with larger variance. This is the oppositive of what we expect for smaller Bmax
values. Using the SNPP-V2 configuration (Bmax
= 0.2) and setting RTAerr
= 0.0 K, we see a significant change in the AK structure, magnitude and variance (not shown). This tells us that the RTAerr
we adopted for CLIMCAPS-SNPP V2 is much too large (Fig. 4c). In fact, it is an order of magnitude larger, on average, than the RTAerr
for CLIMCAPS-Aqua V2 (∼ 0.05 K). We, therefore, propose that the RTAerr
should be carefully recomputed for all CLIMCAPS V3 CrIS+ATMS configurations. For the sake of this paper, however, we list RTAerr
= 0.0 K as a placeholder value for future upgrades, since the effort required to derive an accurate estimate of RTAerr
is out of the scope of this paper.
Another question that often arises with regard to CLIMCAPS-SNPP/JPSS+ is the issue of apodization. We will not explain apodization in this paper or cover the reasons for apodizing CrIS radiances ahead of retrievals. Others cover this in great detail (Barnet et al., 2000, 2023). What we do wish to illustrate here is how spectral correlation due to apodization has no significant impact on CLIMCAPS AKs and, therefore, retrieval SNR. The SNPP-x2 and SNPP-x3 configurations differ only in the number of longwave (LW) IR channels used in Tair retrievals. SNPP-x2 uses all available CrIS channels in the 660–760 cm−1 range (225 channels in total), while SNPP-x3 uses the V2 105 channel subset (Fig. 4a). In addition, SNPP-x2 sets apodcor
= 0 for all adjacent channels to indicate the absence of spectral correlation due to apodization. A comparison of the AKs (Fig. 6b) and Tair and evaluation of Tair retrievals (not shown) indicate no significant difference between SNPP-x2 and SNPP-x3. We, therefore, do not recommend any changes to the apodization of CrIS radiances or the V2 Tair channel subsets for future CLIMCAPS upgrades. The SNPP-x1 and Aqua-x1 configurations yielded very high AK values, but because their Bmax
values forced CLIMCAPS to use a large number of eigenfunctions undamped, their retrieval quality deteriorated relative to the operational V2 product (not shown).
We can next turn our attention to evaluating ADIFF (Fig. 7). As discussed, ADIFF quantifies the difference between retrieval and a priori. When we look at a global map of ADIFF at a specific pressure level, we can gain insight into the retrieval system. For example, an ADIFF with a consistent speckle pattern across large areas would indicate random retrieval SNR, or an ADIFF with a strong latitudinal pattern would indicate systematic bias in retrieval SNR. What we want to see in a global map of ADIFF, instead, is consistency across latitudes with a spatial pattern corresponding to known geophysical features, like clouds.
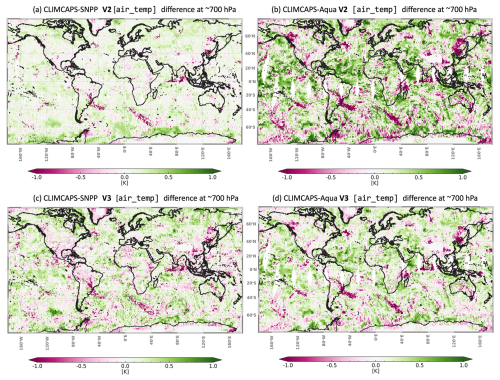
Figure 7CLIMCAPS Tair difference between retrieval and a priori at ∼ 700 hPa on 30 August 2016 for all ascending and descending orbits aggregated to a uniform 1° equal-angle global grid. Comparison of Tair differences, ADIFF, between (a, b) CLIMCAPS V2 and the proposed (c, d) CLIMCAPS V3 configurations for (a, c) SNPP and (b, d) Aqua.
Figure 7a and b depict V2 Tair ADIFF at 700 hPa for the SNPP and Aqua configurations, while Fig. 7c and d show what ADIFF would look like for a V3 system. It is immediately obvious that the CLIMCAPS retrieval SNR is neither truly random nor alarmingly biased. This said, the V2 Tair ADIFF has large differences between the two instrument systems, both in magnitude and dynamic range. This indicates that there are systematic differences in the way CLIMCAPS V2 is configured for CLIMCAPS-SNPP and CLIMCAPS-Aqua, but that these are largely resolved in the proposed V3 Tair configuration. A stronger correlation between CLIMCAPS-SNPP and CLIMCAPS-Aqua in V3 Tair ADIFF tells us that the upgrades we suggest here will improve continuity in the CLIMCAPS record across CrIS+ATMS and AIRS+AMSU. Even so, we do not expect to fully resolve retrieval continuity across all parameters from CrIS+ATMS and AIRS+AMSU, given the list of known differences in instrumentation (Table S1). Some of the other changes we suggest for a CLIMCAPS V3 upgrade (see Table 1) pertain to the channel subsets for O3 and CO2 retrievals, which we illustrate in Fig. 8.
Figure 8 depicts the global average (and standard deviation) of CLIMCAPS AKs for seven retrieval parameters – Tair, H2Ovap, O3, CO, CH4, CO2 and HNO3 – for the different configurations evaluated in this paper (Table 1). Figure 8a represents the V2 retrieval capability we discussed in previous work (Smith and Barnet, 2020). Apart from Tair and H2Ovap, we also notice disparities in CLIMCAPS V2 AKs for CO2 and HNO3. The disparities that exist for the CO AKs across CLIMCAPS-Aqua and CLIMCAPS-SNPP, on the other hand, are predominantly due to known IR instrument differences; the AIRS shortwave (SW) IR band does not cover the full spectral absorption region for CO, while CrIS captures all spectral channels sensitive to CO in the SW IR band (Table 1; Smith and Barnet, 2019). There is, thus, a physical limit to the signal that exists for CO in the AIRS sounder. We argue that it is important to capture as much of the CO signal as possible, given the present-day need for information about air quality and fire emissions. We, therefore, propose to make no algorithm changes to CLIMCAPS-SNPP/JPSS+ that would reduce the CO AK for the sake of mimicking CLIMCAPS-Aqua CO capability. This is the only retrieval parameter for which we make this exception. All other retrieval parameters are supported by comparable instrument observing capabilities.
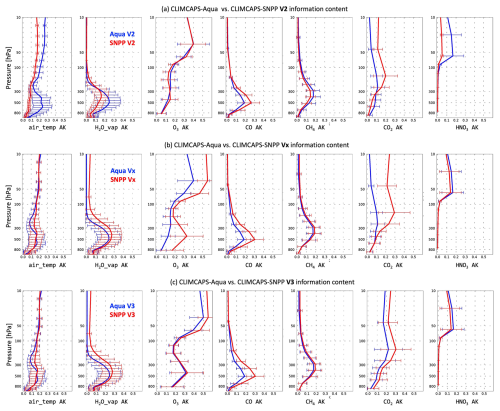
Figure 8CLIMCAPS AKs for Tair as well as six atmospheric gases (H2Ovap, O3, CO, CH4, CO2 and HNO3) as the mean and standard deviation (error bars) for all retrievals in ascending and descending orbits that passed quality control on 1 September 2016. Comparisons of CLIMCAPS AKs from (blue) Aqua and (red) SNPP using three different configurations, (a) V2, (b) experimental SNPP-x3 and Aqua-x2, and the proposed (c) V3 for both instrument suites.
With this paper, we demonstrated how decadal continuity can be achieved in a Level 2 product using the same retrieval system and measurements from two satellites with different instruments, AIRS and CrIS. Achieving retrieval consistency across instruments is not a trivial task and requires knowledge of instrument design as well as calibration. Like all atmospheric sounding observations, CLIMCAPS retrievals are not direct measurements but, instead, indirect observations derived from inverting the top-of-atmosphere IR and MW spectra. Many signal inversion techniques exist depending on the type of measurement and target application. For CLIMCAPS, we employed the method originally developed by Susskind et al. (2003) for the AIRS instrument. We emphasized how the approach to dynamically regularize each retrieval, based on scene-dependent measurement information content, benefits CLIMCAPS retrievals and contrasts with the static, generalized regularization promoted in Rodgers (2000). CLIMCAPS uses a space and time colocated reanalysis model, MERRA-2 (Gelaro et al., 2017), as a priori estimate for Tair, H2Ovap and O3. Not only does this provide a priori estimates that are largely independent of the measurements, but it also allows for the calculation of representative a priori error covariance matrices for use in the Bayesian retrieval steps. Statistical a priori estimates that are derived from the full set of spectral measurements (e.g., Goldberg et al., 2003; Milstein and Blackwell, 2016) confound the analysis of AKs since instrument information is contained in the a priori estimate as well as the final retrieval. One could argue that reanalysis models, like MERRA-2, already assimilate spectral information from AIRS, CrIS, AMSU and ATMS to derive their model fields. But, as argued in Smith and Barnet (2019), not only do reanalysis models, like MERRA-2, assimilate only small subsets of spectral channels, they also apply rigorous spatial thinning to avoid any interference from clouds, aerosols or smoke. In addition, MERRA-2 assimilates spectral information from a large array of sources so the contribution from any individual instrument at a specific space-time location is always low, if not absent. The CLIMCAPS design, with its instrument-independent a priori and representative error covariance matrices, enables the systematic propagation of error through all retrieval steps to yield accurate retrievals with high yield of successful results across the globe (Smith and Barnet, 2019).
The Level-2 CLIMCAPS V2 product contains the AK matrices for each retrieved parameter, at each instrument FOR, which enables the SNR analysis. The validation of retrieved quantities alone does not advance knowledge of a retrieval system. Instead, the analysis of SNR we presented in this paper offers a more honest, in-depth assessment of the contribution that IR measurements make to the retrieved solution. This, in turn, helps promote retrieval consistency since the results can be used to optimize CLIMCAPS to have consistency in measurement contribution (as characterized by the shape, magnitude and variance of the AKs) across a wide range of environmental conditions and despite instrument differences.
We proposed a series of changes that can be implemented in CLIMCAPS V3, should future funding allow, to improve its multi-decadal sounding record. This is especially important for a system, such as CLIMCAPS, that observes the planetary boundary layer (PBL). The PBL affects human health and well-being first and foremost, so it is one of the primary considerations in CLIMCAPS. Over and above the issues we consider in this paper for V3 upgrades, CLIMCAPS allows ample opportunity for targeted upgrades to its PBL observing capability in future. Our paper is additionally relevant to other sounding systems and instruments, such as the NOAA Unique Combined Atmospheric Processing System (NUCAPS; Barnet et al., 2021). If NOAA optimizes NUCAPS for consistency across all hyperspectral instruments, then their soundings can depict real-time diurnal changes to help inform severe weather forecasts. Moreover, the modern-era approach is to de-aggregate large multi-sensor satellites into smaller IR-only and MW-only satellites, as well as to merge measurements from low-Earth orbiting and geostationary satellites alike. This means that there is a greater need for the ability to algorithmically handle and evaluate these new dynamic types of representation error in retrievals. Also, it is expected that future instruments will have new kinds of instrument errors due to the higher demands on spatial and spectral resolution. The ability to robustly and efficiently account for instrument errors along with the ability to diagnose their impact on the retrieval products is the future of sounding science. CLIMCAPS and the analysis approach we presented here discuss the types of upgrades that are relevant to sounding science itself, and we argue that improvements in one product can translate into the other products in the multi-dimensional sounding space.
Table 2 summarizes the CLIMCAPS V2 product suite that is maintained, archived and distributed by the NASA Goddard Earth Sciences Data and Information Services Center (GES DISC). The primary CLIMCAPS product suite spans instruments on three platforms to provide a continuous record of satellite soundings from 2002 to the present day. These are (rows 1–3) AIRS+AMSU on Aqua and CrIS+ATMS on SNPP as well as the JPSS series. In addition, CLIMCAPS generates two experimental products that span the full lifetimes of the two early satellites (rows 4 and 5), namely, (i) Aqua by predominantly using AIRS channels to overcome the loss of key AMSU channels in 2016 and (ii) SNPP by using CrIS normal (or low) spectral resolution (NSR) radiances. Between 20 January 2012 and 2 November 2015, the SNPP CrIS measurements were exclusively available in NSR mode; a second, full spectral resolution (FSR) CrIS Level 1B product was later added to better match the spectral information content of AIRS. The CLIMCAPS V2 record includes these two experimental datasets to support sounding science and instrument investigations, but they will not be released or maintained in future CLIMCAPS versions.
The supplement related to this article is available online at https://doi.org/10.5194/amt-18-1823-2025-supplement.
CDB and NS, together, conceptualized this work and designed the experiments. CDB is the architect and developer of the CLIMCAPS software. NS performed the formal analysis and generated all graphics. NS wrote the original draft and CDB reviewed the original and revised manuscripts with NS editing the final version.
The contact author has declared that neither of the authors has any competing interests.
Publisher’s note: Copernicus Publications remains neutral with regard to jurisdictional claims made in the text, published maps, institutional affiliations, or any other geographical representation in this paper. While Copernicus Publications makes every effort to include appropriate place names, the final responsibility lies with the authors.
The work reported in this paper requires dedicated expertise and effective teamwork within a collaborative environment. The authors, Nadia Smith (NS) and Chris D. Barnet (CDB), therefore wish to express their sincere gratitude to NASA for funding the science and development of CLIMCAPS. Each milestone was met within the confines of the NASA ROSES (Research Opportunities in Space and Earth Science) competitive grant system spanning 9 years altogether. Each grant funded at most 1 FTE yr−1 for a total of 3 years.
-
2015–2018 (principal investigator CDB): ROSES grant no. NH15CM66C enabled development of the retrieval code as well as the set of a priori estimates.
-
2018–2021 (principal investigator CDB): ROSES grant no. 80NSSC18K0975 enabled the setup and operationalization of CLIMCAPS for instruments on Aqua, SNPP and JPSS.
-
2021–2024 (principal investigator NS): ROSES grant no. 80NSSC21K1959 supported the maintenance of CLIMCAPS, which included engagement with stakeholders to improve product design and develop applications.
The authors would like to acknowledge Joel Susskind who transformed techniques used in numerical quantum mechanics to provide the adaptive method of regularization that enables the robust utilization of hyperspectral satellite soundings employed in the operational AIRS Science Team, NUCAPS and CLIMCAPS algorithms. This approach continues to be refined and is still evolving, as demonstrated in this paper. The authors would also like to acknowledge some of the early influential work by Barney Conrath (e.g., Conrath, 1977) to understand the physical nature of information content. Chris D. Barnet had the honor to work closely with both Joel and Barney for many years and values their desire to maximize the exploitation of space sounding assets.
This research has been supported by the National Aeronautics and Space Administration (grant no. 80NSSC21K1959).
This paper was edited by Ralf Sussmann and reviewed by two anonymous referees.
Aumann, H. H., Chahine, M. T., Gautier, C., Goldberg, M. D., Kalnay, E., McMillin, L. M., Revercomb, H., Rosenkranz, P. W., Smith, W. L., Staelin, D. H., Strow, L. L., and Susskind, J.: AIRS/AMSU/HSB on the aqua mission: design, science objectives, data products, and processing systems, IEEE T. Geosci. Remote, 41, 253–264, https://doi.org/10.1109/TGRS.2002.808356, 2003.
Barnet, C. D., Blaisdell, J. M., and Susskind, J.: Practical methods for rapid and accurate computation of interferometric spectra for remote sensing applications, IEEE T. Geosci. Remote, 38, 169–183, https://doi.org/10.1109/36.823910, 2000.
Barnet, C. D., Divakarla, M., Gambacorta, A., Iturbide-Sanchez, F., Tan, C., Wang, T., Warner, J., Zhang, K., and Zhu, T.: NOAA Unique Combined Atmospheric Processing System (NUCAPS) algorithm theoretical basis document, National Oceanic and Atmospheric Administration, Washington, D.C., USA, https://www.star.nesdis.noaa.gov/jpss/documents/ATBD/ATBD_NUCAPS_v3.1.pdf (last access: 4 April 2025), 2021.
Barnet, C. D., Smith, N., Ide, K., Garrett, K., and Jones, E.: Evaluating the Value of CrIS Shortwave-Infrared Channels in Atmospheric-Sounding Retrievals, Remote Sensing, 15, 547, https://doi.org/10.3390/rs15030547, 2023.
Chahine, M. T.: Remote sounding of cloudy parameters II. Multiple cloud formations, J. Atmos. Sci., 34, 744–757, https://doi.org/10.1175/1520-0469(1977)034<0744:RSOCAI>2.0.CO;2, 1977.
Chahine, M. T., Pagano, T. S., Aumann, H. H., Atlas, R., Barnet, C. D., Blaisdell, J., Chen, L., Divakarla, M., Fetzer, E. J., Goldberg, M., Gautier, C., Granger, S., Hannon, S., Irion, F. W., Kakar, R., Kalnay, E., Lambrigtsen, B. H., Lee, S.-Y., Le MARSHALL, J., Mcmillan, W. W., Mcmillin, L., Olsen, E. T., Revercomb, H., Rosenkranz, P., Smith, W. L., Staelin, D., Strow, L. L., Susskind, J., Tobin, D., Wolf, W., and Zhou, L.: AIRS: Improving Weather Forecasting and Providing New Data on Greenhouse Gases, B. Am. Meteorol. Soc., 87, 911–926, https://doi.org/10.1175/BAMS-87-7-911, 2006.
Conrath, B. J.: Backus-Gilbert theory and its application to retrieval of ozone and temperature profiles, in: Inversion Methods in Atmospheric Remote Sounding, Elsevier, 155–193, https://doi.org/10.1016/B978-0-12-208450-8.50012-6, 1977.
Gambacorta, A. and Barnet, C. D.: Methodology and information content of the NOAA NESDIS operational channel selection for the Cross-Track Infrared Sounder (CrIS), IEEE T. Geosci. Remote, 51, 3207–-3216, https://doi.org/10.1109/TGRS.2012.2220369, 2013.
Gambacorta, A., Barnet, C., Wolf, W., King, T., Maddy, E., Strow, L., Xiaozhen Xiong, Nalli, N., and Goldberg, M.: An Experiment Using High Spectral Resolution CrIS Measurements for Atmospheric Trace Gases: Carbon Monoxide Retrieval Impact Study, IEEE Geosci. Remote S., 11, 1639–1643, https://doi.org/10.1109/LGRS.2014.2303641, 2014.
Gaudel, A., Bourgeois, I., Li, M., Chang, K.-L., Ziemke, J., Sauvage, B., Stauffer, R. M., Thompson, A. M., Kollonige, D. E., Smith, N., Hubert, D., Keppens, A., Cuesta, J., Heue, K.-P., Veefkind, P., Aikin, K., Peischl, J., Thompson, C. R., Ryerson, T. B., Frost, G. J., McDonald, B. C., and Cooper, O. R.: Tropical tropospheric ozone distribution and trends from in situ and satellite data, Atmos. Chem. Phys., 24, 9975–10000, https://doi.org/10.5194/acp-24-9975-2024, 2024.
Gelaro, R., McCarty, W., Suárez, M. J., Todling, R., Molod, A., Takacs, L., Randles, C. A., Darmenov, A., Bosilovich, M. G., Reichle, R., Wargan, K., Coy, L., Cullather, R., Draper, C., Akella, S., Buchard, V., Conaty, A., da Silva, A. M., Gu, W., Kim, G.-K., Koster, R., Lucchesi, R., Merkova, D., Nielsen, J. E., Partyka, G., Pawson, S., Putman, W., Rienecker, M., Schubert, S. D., Sienkiewicz, M., and Zhao, B.: The modern-era retrospective analysis for research and applications, Version 2 (MERRA-2), J. Climate, 30, 5419–5454, https://doi.org/10.1175/JCLI-D-16-0758.1, 2017.
Glumb, R. J., Jordan, D. C., and Mantica, P.: Development of the Crosstrack Infrared Sounder (CrIS) sensor design, in: Proc. SPIE 4486, Infrared Spaceborne Remote Sensing IX, International Symposium on Optical Science and Technology, San Diego, CA, 29 July–3 August 2001, SPIE, 411–424, https://doi.org/10.1117/12.455124, 2002.
GMAO: MERRA-2 inst3_3d_asm_Nv: 3d,3-Hourly,Instantaneous,Model-Level,Assimilation,Assimilated Meteorological Fields V5.12.4, Goddard Earth Sciences Data and Information Services Center (GES DISC) [data set], Greenbelt, MD, USA, https://doi.org/10.5067/WWQSXQ8IVFW8, 2015.
Goldberg, D. G., Qu, Y., McMillim, L. M., Wolf, W., Zhou, L., and Divakarla, G.: AIRS near-real-time products and algorithms in support of operational numerical weather prediction, IEEE T. Geosci. Remote, 41, 379–389, https://doi.org/10.1109/TGRS.2002.808307, 2003.
Manning, E. M., Aumann, H. H., Pagano, T. S., Broberg, S. E., Schindler, R. A., and Overoye, K. R.: Causes and effects of AIRS optics temperature cycles, in: Earth Observing Systems XXV, 20 August 2020, Online Only, SPIE, 115010B, https://doi.org/10.1117/12.2567458, 2020.
Milstein, A. B. and Blackwell, W. J.: Neural network temperature and moisture retrieval algorithm validation for AIRS/AMSU and CrIS/ATMS, J. Geophys. Res.-Atmos., 121, 1414–1430, https://doi.org/10.1002/2015JD024008, 2016.
Nalli, N. R., Gambacorta, A., Liu, Q., Barnet, C. D., Tan, C., Iturbide-Sanchez, F., Reale, R., Sun, B., Wilson, M., Borg, L., and Morris, V. R.: Validation of Atmospheric Profile Retrievals From the SNPP NOAA-Unique Combined Atmospheric Processing System. Part 1: Temperature and Moisture, IEEE T. Geosci. Remote, 56, 180–190, https://doi.org/10.1109/TGRS.2017.2744558, 2018a.
Nalli, N. R., Gambacorta, A., Liu, Q., Tan, C., Iturbide-Sanchez, F., Barnet, C. D., Joseph, E., Morris, V. R., Oyola, M., and Smith, J. W.: Validation of Atmospheric Profile Retrievals from the SNPP NOAA-Unique Combined Atmospheric Processing System. Part 2: Ozone, IEEE T. Geosci. Remote, 56, 598–607, https://doi.org/10.1109/TGRS.2017.2762600, 2018b.
Ouyed, A., Smith, N., Zeng, X., Galarneau, T., Su, H., and Dixon, R. D.: Global Three-Dimensional Water Vapor Feature-Tracking for Horizontal Winds Using Hyperspectral Infrared Sounder Data From Overlapped Tracks of Two Satellites, Geophys. Res. Lett., 50, e2022GL101830, https://doi.org/10.1029/2022GL101830, 2023.
Parkinson, C. L.: The Earth-Observing Aqua Satellite Mission: 20 Years and Counting, Earth and Space Science, 9, e2022EA002481, https://doi.org/10.1029/2022EA002481, 2022.
Prange, M., Buehler, S. A., and Brath, M.: How adequately are elevated moist layers represented in reanalysis and satellite observations?, Atmos. Chem. Phys., 23, 725–741, https://doi.org/10.5194/acp-23-725-2023, 2023.
Rodgers, C. D.: Inverse methods for atmospheric sounding: theory and practice, World Scientific, Singapore; Hackensack, N.J., ISBN 978-981-02-2740-1, 2000.
Smith, N: Sounder SIPS Aqua AIRS IR + MW Level 2 CLIMCAPS retrieved atmospheric state V2.1, NASA [data set], https://doi.org/10.5067/J7GVFM11OGIX, 2019a.
Smith, N: Sounder SIPS Aqua AIRS IR-only Level 2 CLIMCAPS retrieved atmospheric state V2.1, NASA [data set], https://doi.org/10.5067/WZVJ68EXNLK7, 2019b.
Smith, N: Sounder SIPS JPSS-1 CrIMSS Level 2 CLIMCAPS retrieved atmospheric state V2.1, NASA [data set], https://doi.org/10.5067/5GHJWKUXQSP6, 2019c.
Smith, N: Sounder SIPS Suomi NPP CrIMSS Level 2 CLIMCAPS Full Spectral Resolution retrieved atmospheric state V2.1, NASA [data set], https://doi.org/10.5067/SD6WORV4GX8P, 2019d.
Smith, N: Sounder SIPS Suomi NPP CrIMSS Level 2 CLIMCAPS Normal Spectral Resolution retrieved atmospheric state V2.1, NASA [data set], https://doi.org/10.5067/KU5L6AXRNQX8, 2019e.
Smith, N. and Barnet, C. D.: Uncertainty Characterization and Propagation in the Community Long-Term Infrared Microwave Combined Atmospheric Product System (CLIMCAPS), Remote Sensing, 11, 1227, https://doi.org/10.3390/rs11101227, 2019.
Smith, N. and Barnet, C. D.: CLIMCAPS observing capability for temperature, moisture, and trace gases from AIRS/AMSU and CrIS/ATMS, Atmos. Meas. Tech., 13, 4437–4459, https://doi.org/10.5194/amt-13-4437-2020, 2020.
Smith, N. and Barnet, C. D.: CLIMCAPS – A NASA Long-Term Product for Infrared + Microwave Atmospheric Soundings, Earth and Space Science, 10, e2022EA002701, https://doi.org/10.1029/2022EA002701, 2023a.
Smith, N. and Barnet, C. D.: Practical Implications of CLIMCAPS Cloud Clearing and Derived Quality Metrics, Earth and Space Science, 10, e2023EA002913, https://doi.org/10.1029/2023EA002913, 2023b.
Strow, L. L., Hannon, S. E., De Souza-Machado, S., Motteler, H. E., and Tobin, D.: An overview of the AIRS radiative transfer model, IEEE T. Geosci. Remote, 41, 303–313, https://doi.org/10.1109/TGRS.2002.808244, 2003.
Strow, L. L., Motteler, H., Tobin, D., Revercomb, H., Hannon, S., Buijs, H., Predina, J., Suwinski, L., and Glumb, R.: Spectral calibration and validation of the Cross-track Infrared Sounder on the Suomi NPP satellite: CrIS spectral calibration, J. Geophys. Res.-Atmos., 118, 12486–12496, https://doi.org/10.1002/2013JD020480, 2013.
Susskind, J., Barnet, C. D., and Blaisdell, J. M.: Determination of atmospheric and surface parameters from simulated AIRS/AMSU/HSB sounding data: Retrieval and cloud clearing methodology, Adv. Space Res., 21, 369–384, https://doi.org/10.1016/S0273-1177(97)00916-2, 1998.
Susskind, J., Barnet, C. D., and Blaisdell, J. M.: Retrieval of atmospheric and surface parameters from AIRS/AMSU/HSB data in the presence of clouds, IEEE T. Geosci. Remote, 41, 390–409, https://doi.org/10.1109/TGRS.2002.808236, 2003.
Wang, T., Roman, J., Yue, Q., and Wong, S. (Eds.): Test Report of Performance of CLIMCAPS-SNPP and CLIMCAPS-JPSS1 Retrievals, Jet Propulsion Laboratory, California Institute of Technology, CA, https://docserver.gesdisc.eosdis.nasa.gov/public/project/Sounder/CLIMCAPS.V2.Test.Report.pdf (last access: 1 August 2024), 2020.
Yue, Q., Fetzer, E. J., Wang, L., Kahn, B. H., Smith, N., Blaisdell, J. M., Meyer, K. G., Schreier, M., Lambrigtsen, B., and Tkatcheva, I.: Evaluating the consistency and continuity of pixel-scale cloud property data records from Aqua and SNPP (Suomi National Polar-orbiting Partnership), Atmos. Meas. Tech., 15, 2099–2123, https://doi.org/10.5194/amt-15-2099-2022, 2022.