the Creative Commons Attribution 4.0 License.
the Creative Commons Attribution 4.0 License.
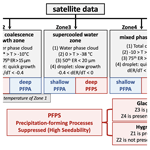
An analysis of cloud microphysical features over United Arab Emirates using multiple data sources
Vesta Afzali Gorooh
Duncan Axisa
Chandrasekar Radhakrishnan
Eun Yeol Kim
Venkatachalam Chandrasekar
Luca Delle Monache
Water is a precious resource and is important for human health, agriculture, industry, and the environment. When water is in short supply, monitoring and predicting the current and future occurrence of precipitating clouds is essential. In this study, we investigate the cloud microphysical features in several convective cloud systems in the United Arab Emirates (UAE) using multiple data sources, including aircraft measurements, satellite observations, weather radar observations, and reanalysis data. The aircraft observation dataset is from an airborne research campaign conducted in August 2019 in the UAE. The cloud cases were identified through analysis of cloud spectrometers mounted on the aircraft. Then, we investigated the microphysical features of those cloud cases, with a focus on precipitation microphysics. The effective radius of the cloud particles retrieved from geostationary satellite data was compared with the aircraft in situ measurement. Using the effective radius retrieved from satellite data, we developed a framework to identify five microphysical zones: the diffusional droplet growth zone, droplet coalescence growth zone, supercooled-water zone, mixed-phase zone, and glaciated zone. The identified zones were verified using the aircraft observations, and the transferability of the five-zone concept was tested using additional cloud cases. The results show that our five-zone concept successfully detects the microphysical features related to precipitation using satellite data in the UAE. This study provides scientific support for the development of an applicable framework to examine cloud precipitation processes and detect suitable cloud features that could be tracked for further precipitation analysis and nowcasting.
- Article
(12996 KB) - Full-text XML
-
Supplement
(847 KB) - BibTeX
- EndNote
Water remains a vital resource globally, with its significance heightened by climate change and an increased frequency of extreme weather events. The availability and sustainability of water resources affect every sector (e.g., Pimentel et al., 2004), particularly in arid or semi-arid regions (Wehbe and Temimi, 2021). In the United Arab Emirates (UAE), the absence of precipitation in the context of the growing population in this region in recent years raises concerns about food security and coastline resilience (e.g., Murad et al., 2007). Understanding the physical processes in clouds that trigger precipitation formation is critical for sustainable water management and effective preparation for potential water-related risks.
Most regions in the UAE are arid or semi-arid, and its surrounding areas, except to the north (bordered by the Persian Gulf), are tropical and subtropical deserts (Niranjan Kumar and Ouarda, 2014). The UAE has four climate zones: the desert foreland, east coast, gravel plains, and mountains (Sherif et al., 2014). Most of these zones are characterized by scarce rainfall and a high evaporation rate, except for certain coastal regions. Rainfall distribution within the UAE exhibits large spatial and temporal variation, with the maximum and minimum precipitation occurring in the mountains and on the east coast and in the desert foreland, respectively (Wehbe et al., 2017). The latter covers the largest portion of the area in the UAE. The wet season generally occurs from November to April. The average monthly rainfall received by the entire country ranges from approximately 2 mm (e.g., in June) to 15 mm (e.g., in March) (Hussein et al., 2021). The average annual rainfall is generally less than 100 mm, varying from 60 to 140 mm (Ouarda et al., 2014; Wehbe et al., 2020). Half of the annual precipitation can fall in a single day during mesoscale convective events (Wehbe et al., 2019; Kumar and Suzuki, 2019).
Due to the extremely low occurrence of rainfall and the dry climate, rainfall enhancement is one of the active areas of research in the UAE (Wehbe et al., 2023). While new technologies for weather modification can improve the operational efficiency of rainfall enhancement activities, identifying suitable targets is always a priority (Axisa and DeFelice, 2016; DeFelice and Axisa, 2016; DeFelice et al., 2023; Hirst et al., 2023). Therefore, it is essential to monitor and detect current and future cloud microphysical features related to precipitation processes.
Within convective clouds, precipitation particles are produced by small-scale microphysical processes that are active in different parts of the cloud. These processes initiate precipitation through multiple physical pathways at different rates. The efficiency with which clouds produce precipitation varies greatly and is a function of the dominant physical process under specific thermodynamic conditions. Growth of precipitation particles can occur through collision and coalescence, the ice multiplication process, or a combination of the two. Raindrops cannot form by diffusional growth alone in convective clouds. The growth of cloud droplets from a radius of 10–20 µm to raindrop size (>100 µm) requires an active collision–coalescence process (Bartlett, 1966). In the absence of collision–coalescence, droplets that form by diffusional growth remain small (i.e., radii<15 µm), and their size distribution is composed of a high number concentration of small droplets (Pruppacher et al., 1998). When these droplets reach temperatures colder than 0 °C, they become supercooled, and ice can develop through different microphysical pathways. Ice multiplication activity within supercooled clouds is active in the −5 to −8 °C region (Hallett and Mossop, 1974), and the rate of production of ice depends upon not only the concentration of large drops (>24 µm diameter) but also the concentration of small drops (<13 µm) in the cloud (Mossop, 1978). Therefore, the cloud droplet size distribution and parameters derived from it, along with the cloud temperature, are critical to understanding cloud microphysical processes and the dominant physical pathways that lead to precipitation.
Advancement of in situ and remote sensing technology has provided the cloud physics community with much-improved research tools to study aerosol–cloud–precipitation interactions. A combination of satellite cloud top temperature and effective droplet radii retrieved from the Advanced Very High Resolution Radiometer (AVHRR) has been used to infer the suppression of coalescence and precipitation processes by smoke (Rosenfeld and Lensky, 1998) and desert dust (Rosenfeld et al., 2001). Tropical Rainfall Measuring Mission (TRMM) multi-sensor satellite observations have been used to detect the presence of non-precipitating supercooled liquid water near cloud tops associated with the heavy seeding from smoke over Indonesia (Rosenfeld, 1999) and urban pollution over Australia (Rosenfeld, 2000). The time series of precipitation formation processes within convective storms over the eastern Mediterranean were tracked by Meteosat Second Generation (MSG) to investigate the cloud response to aerosol loading. A strong correlation was found between the aerosol loading and the depth above cloud base required for the onset of precipitation (Lensky and Shiff, 2007). Aircraft in situ measurements of continental convective clouds seeded with finely milled salt powder detected a broadening of the cloud drop size distribution (Rosenfeld et al., 2010), indicating an acceleration of the warm-rain process. In addition, aircraft measurements have provided evidence that dust particles extend the tail of the cloud droplet size distribution spectra, increasing the droplet effective radii and triggering the formation of warm rain (Pósfai et al., 2013).
Rosenfeld and Lensky (1998) used the AVHRR satellite data to analyze the vertical profiles of the cloud particle effective radii to investigate the precipitation formation processes in convective clouds and introduced five distinct vertical cloud zones, including (1) the diffusional droplet growth zone, (2) the droplet coalescence growth zone, (3) the rainout zone, (4) the mixed-phase zone, and (5) the glaciated zone, which characterize the microphysical features of the cloud from the precipitation formation perspective. Lensky and Drori (2007) followed the Rosenfeld and Lensky (1998) approach and defined the temperature of precipitation onset as the temperature where the median effective radius exceeds a threshold of 15 µm. A recent study by Wang et al. (2019) focused on identifying supercooled-water clouds and developed a method to detect them based on the cloud phase, effective radius, optical thickness, and cloud top temperature from the Advanced Himawari Imager and aircraft in situ cloud measurements.
During the last 2 decades, there has been a continuous effort focused on rainfall enhancement science in the UAE (Mazroui and Farrah, 2017; Al Hosari et al., 2021). However, the recent enhanced observations, including the airborne measurements over the UAE (Wehbe et al., 2021, 2023) and remote sensing from geostationary satellites (Meteosat-10 and Meteosat-8; Kumar and Suzuki 2019), provide unique data sources to examine the cloud microphysical features and the dominant physical pathways that lead to precipitation in the UAE. Kumar and Suzuki (2019) evaluate the spatial and seasonal occurrence of cloud cover from Meteosat-10 and Meteosat-8 in the UAE and analyze the cloud phase distribution to determine the potential for precipitation enhancement through cloud seeding with aerosols. In general, cloud seeding is applied using aerosol that is active as cloud condensation nuclei (CCNs) in the warm part of the cloud (called hygroscopic seeding; Mather et al., 1997; Cooper et al., 1997; Bruintjes, 1999; Terblanche et al., 2000; Silverman, 2000, 2003; Rosenfeld et al., 2010; Flossmann et al., 2019) and aerosol that is active as an ice nucleating particle (INP) around supercooled liquid water clouds (called glaciogenic seeding; Bruintjes, 1999; Silverman, 2001; Woodley et al., 2003a, b; Flossmann et al., 2019). In both hygroscopic and glaciogenic seeding, seeding material must be properly applied to be effective (Geresdi et al., 2021). This is often referred to as targeting. In practice, this is often the most challenging part of operational seeding programs.
In this study, we investigated the microphysical features of cloud cases over the UAE using multiple data sources focusing on the cloud microphysics of precipitation. We examined these features using aircraft observations, introduced a new five-zone framework to detect the cloud microphysical zones using satellite data, and used aircraft measurements to validate the cloud zones detected. The corresponding synoptic conditions and the radar reflectivity features for cloud cases were also explored. This study aims to develop an applicable framework that detects cloud features that correspond to microphysical pathways that are active in different parts of the cloud to characterize precipitation processes in the UAE. One application of this framework is the development of a tool for further analysis of precipitation and nowcasting and to assist with cloud targeting in operational seeding programs.
2.1 Aircraft observations
The aircraft data are from the UAE 2019 airborne campaign (Wehbe et al., 2021; Morrison et al., 2022). The scientific flights in this campaign were conducted by the Stratton Park Engineering Company (SPEC) Learjet 35A in August 2019. This SPEC Learjet 35A aircraft was equipped with state-of-the-art cloud physics instruments. The list of instruments includes the following: a cloud particle imager (CPI; Lawson et al., 2001), a two-dimensional stereo probe (2DS; Lawson et al., 2006), a high-volume precipitation spectrometer (HVPS; Lawson et al., 1998), a fast forward-scattering spectrometer probe (FFSSP; Brenguier et al., 1998), a fast cloud droplet probe (FCDP; Lawson et al., 2017; Woods et al., 2018), and a Nevzorov hot-wire probe (Korolev et al., 1998). The FCDP, FFSSP, 2DS, and HVPS were all equipped with probe tips to reduce the effects of ice crystals shattering (Korolev et al., 2011; Lawson, 2011), and data were post-processed using an inter-arrival time algorithm to remove shattered particles (Lawson, 2011). When combined, the cloud particle probes can measure size distributions in the range of 2 µm to 2 cm in diameter. In this campaign, there were 11 scientific flights (SFs), which are listed in Table 1. Figure 1 shows some examples of the flight tracks, including SF01 on 12 August, SF02 on 13 August, and SF03 on 18 August 2019. Wehbe et al. (2021) study the evolution of growing convective cloud tops during this campaign and present aerosol and cloud microphysical measurements from SF01 and SF04. Morrison et al. (2022) examine microphysical processes with a focus on studying activation and growth of cloud droplets in a bin model and on comparing modeled droplet size distributions to observations for the SF01 case. In this study, we focus on the microphysical properties of SF03, with a focus on the evolution of the drop size distribution and precipitation microphysics.
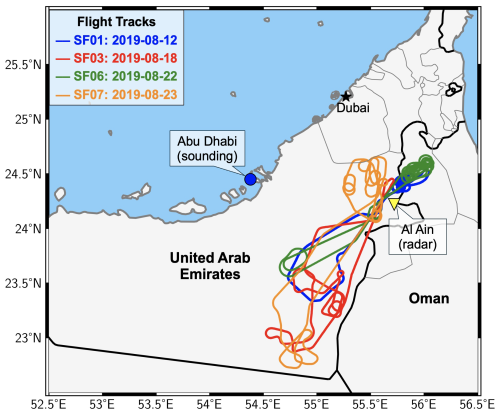
Figure 1A map showing the flight tracks for SF01 (blue line), SF03 (red line), SF06 (green line), and SF07 (orange line) from the UAE 2019 airborne campaign. The yellow triangle is the location of the weather radar in Al Ain, and the blue circle is the location of the Abu Dhabi airport where sounding observations are available.
Table 1A summary of the 11 scientific flights (SFs) in the UAE 2019 airborne campaign, including the flight date, number of cloud penetrations (CPs) identified, the cloud temperature range of the CPs, and the mean effective radius range of the CPs.
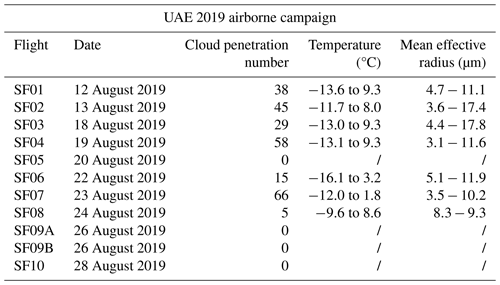
indicates that the temperature range and mean effective radius are not available because the CP was not identified.
The aircraft typically targeted growing young turrets in the early stages of the cloud lifetime because they are the most valuable to sample for studying the formation of precipitation. Young growing turrets are also the most valuable as targets for cloud seeding. Inspection of the forward-facing video from the aircraft confirmed that the clouds sampled are young targets in the early- to mid-life-cycle convection. Figure S2 in the Supplement is an example from flight SF03, showing that the aircraft was penetrating a relatively young turret. In addition, we also examined the vertical profiles of radar reflectivity associated with different cloud cases (at the same time and same location as those cases), which suggest that these clouds are at a relatively young point in their lifetimes. Figure S1a shows the vertical profiles of radar reflectivity for the SF03 cloud case. The radar reflectivity ranges from 0 to ∼ 30 dBz, indicating the characteristics of early- to mid-life-cycle convection. Our radar observations do not show the high reflectivity (higher than 40 dBz) typical of mature convection (Zipser et al., 2006; Feng et al., 2018).
During the flight, if the turret was growing, then the turret was profiled vertically through subsequent cloud penetrations in vertical increments in its early- to mid-life-cycle stage. Once the cloud top was reached and the cloud transitioned to past the mid-life cycle (or rainout stage), a different and younger cloud target within the same cloud field was selected. This can be distinguished from a change in the altitude of the aircraft within a relatively small radius from the previous location. The forward-facing videos from the aircraft confirm this fact.
The effective radius (ER) is a weighted average of the size distribution of cloud droplets. In this study, we used the combined cloud particle size distributions from FCDP, 2DS, and HVPS to calculate ER following the previous studies (e.g., Rosenfeld and Lensky, 1998; Fu et al., 2022). We use 40 µm as a fixed break point to combine the FCDP and 2DS particle size distributions (Fu et al., 2022). The break point between 2DS and HVPS is 1000 µm. Only size distributions with total number concentrations greater than 10 cm−3 are included in the calculation of ERs (Fu et al., 2022).
For better utilization of the aircraft observations, we first identified the measurements of the cloud for each cloud penetration by the aircraft. Figure 2a and b are time segments of the observed total water content (LWTA) from the Nevzorov hot-wire probe and cloud droplet concentrations from FCDP (ConFCDP) during SF03. When the aircraft penetrated a cloud, the LWTA and ConFCDP rapidly increased, as highlighted in the dashed boxes. In this study, if the LWTA is at least 0.05 g m−3 and ConFCDP is at least 20 cm−3 for 1 s or longer, it is considered one cloud penetration (CP). Using this definition, we identified the CPs in all 11 flights, and the numbers of CPs in each flight are listed in the third column of Table 1. Out of the 11 flights, 7 have at least five CPs. Several sensitivity tests were conducted to examine the impacts of the thresholds on defining CPs. Different thresholds in the minimum LWTA (0.01 and 0.1 g m−3) and ConFCDP (10 and 30 cm−3) did not have any significant impacts on the number of detected CPs since the values of those two parameters in CPs are usually substantially higher than the minimum thresholds (e.g., Figs. 2a, b and 5).
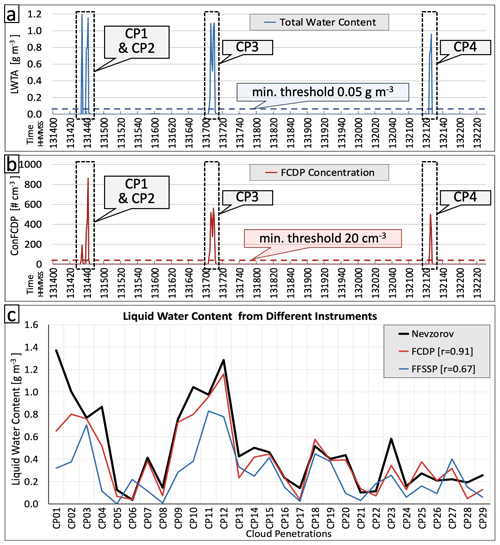
Figure 2Panels (a) and (b) show examples of cloud penetration for flight segments when the aircraft penetrated clouds. (a) Time series of total water content (LWTA) and the minimum LWTA threshold (dashed blue line) for cloud penetration. (b) Time series of cloud particle concentration from FCDP and the minimum FCDP concentration threshold (dashed red line) for cloud penetration. (c) Comparison of liquid water content from different instruments.
After identifying the CPs, we compared selected parameters from different instruments. Figure 2c shows a comparison of liquid water content (LWC) from the FCDP and FFSSP probes to the LWC measured by the Nevzorov probe for the CPs identified in SF03. The Nevzorov probe is a constant-temperature hot-wire probe designed to measure the cloud ice and liquid water content, which can provide a relatively more accurate measurement of the water content (Korolev et al., 1998). The LWC from FCDP has better agreement with the Nevzorov LWC, and their correlation is 0.91, significantly higher than the correlations between the FFSSP and the Nevzorov probes (0.67). Possible reasons for the difference in correlation could be related to sampling bias and/or instrument response, as well as the droplet collection efficiency of the Nevzorov probe. In this study, we focus on the comparison of the FCDP and FFSSP, both forward-scattering spectrometers, to a different instrument that uses other physical properties of droplet sizing, in this case the hot-wire mechanism in the Nevzorov LWC. The finding that the FCDP correlates better with the Nevzorov probe gives us confidence that the FCDP provides higher accuracy compared to the FFSSP.
A vertical distribution of the mean effective radius for all the CPs identified in the flights is shown in Fig. 3. The mean effective radius here is defined as the average effective radius of all observed values in each cloud penetration. SF03 has a cloud base at 9.0 °C, around 3.5 km in elevation, and the highest and coldest CP is at about −13.0 °C, near 6.9 km, indicating a relatively deep cloud. It is worth noting that the cloud top could be higher than the highest CP measured by the aircraft, and other, colder cloud tops could have been present. The cloud droplet effective radius (ER) is about 4.8 µm at the cloud base and increases with height (decreases with temperature) with a maximum ER of 17.8 µm at −12.9 °C. While many cases have a cloud base temperature similar to that of SF03, at around 8.0–9.0 °C, SF07 and SF06 have relatively high measured cloud bases, with temperatures at 1.8 and −3.3 °C, respectively. All the cloud penetration data for these cases were analyzed, and SF03 was utilized as a prime example to demonstrate our analysis using the aircraft observations in Sect. 3.
2.2 Satellite products
2.2.1 High-rate SEVIRI level 1.5 image data
Our study uses the near-real-time high spatiotemporal resolution data from the Spinning Enhanced Visible and Infrared Imager (SEVIRI) sensor on board the Meteosat Second Generation (MSG) satellite (Meteosat-8) with Indian Ocean data coverage (IODC; EUMETSAT High-Rate SEVIRI Level 1.5 Image Data). The visible (VIS), near-infrared (NIR), and infrared (IR) bands are geolocated using a standardized projection, resulting in images containing calibrated, radiance-linearized, and Earth-located information, which is appropriate for deriving meteorological products and conducting additional meteorological processing. We use the cloud brightness temperature (T) derived from one IR channel with a center wavelength of 10.8 µm, known as a clean longwave IR window. This channel is characterized by lower sensitivity to water vapor absorption, enhancing atmospheric moisture correction and facilitating continuous day/night cloud and convection feature identification. The nominal IR image sampling distance (i.e., spatial resolution) is 3 km × 3 km at the sub-satellite point, and the temporal resolution is 15 min (96 data points per day).
2.2.2 Optimal cloud analysis
This study includes the use of the upper-layer cloud effective radius (ER) and cloud phase retrievals from the SEVIRI optimal cloud analysis (OCA; EUMETSAT Optimal Cloud Analysis – MSG) algorithm. The OCA method uses reflectance from the VIS and NIR channels, radiances from the IR channels, the European Centre for Medium-range Weather Forecasts (ECMWF) forecast variables, surface reflectance maps, and cloud mask products to provide ERs ranging from 1 to 31 µm. The SEVIRI OCA scheme is based on an optimal estimation (OE; King et al., 1997; Watts et al., 2011), and it is beneficial for convective cloud monitoring over the Middle East (Mecikalski et al., 2011; Lazri et al., 2014; Hadizadeh et al., 2019). These products rely on the principle that the cloud's optical thickness predominantly determines the reflection function of clouds at a non-absorbing band. In contrast, the reflection function at a water (or ice) absorbing band mainly depends on the size of cloud particles.
Originally formed as a research initiative at the Rutherford Appleton Laboratory (RAL) in 1997, the OCA product has evolved into an operational tool developed by EUMETSAT to deliver timely cloud parameter retrievals from the MSG SEVIRI instrument (Watts et al., 1998). Notably, the OCA product distinguishes itself from alternative retrieval methods by relying on a comprehensive cost function value, indicating consistency between modeling and reality. While some challenges remain, including nighttime performance limitations and constraints in detecting multi-layer conditions for moderate cirrus optical depths, the OCA algorithm is fundamental in advancing our understanding of cloud dynamics and their broader impacts. OCA approaches cloud retrieval as an inverse problem, utilizing a forward model using a radiative transfer model (RTM) to simulate satellite radiances based on a parameterized cloud/atmosphere/surface model and defined observing conditions. The OE method is then employed to obtain the cloud parameters that best match observed radiances, considering measurement errors and prior knowledge (Rodgers, 2000). The OE maximizes the probability of the retrieved state (e.g., cloud effective radius) conditional on the value of the measurements and any a priori knowledge (Poulsen et al., 2012; Watts et al., 2011).
This iterative process aims to minimize the cost function by adjusting the state vector, utilizing the Levenberg–Marquardt scheme for optimization. To initiate the minimization process, the model begins with an initial guess state, typically set to the value of the a priori without additional information. Subsequently, the model iteratively adjusts the state vector in the direction that decreases the cost function at each step, converging toward a minimum. Convergence is reached when the cost function changes minimally between iterations, with unreached convergence deemed to be invalid retrievals. The value of the cost function serves as a measure of the solution state consistency with observations and prior knowledge, with high or low values indicating potential overestimation or underestimation of error, respectively. It is assumed that measurement errors, a priori parameters, and the forward model follow a Gaussian distribution with a zero mean and covariances.
Phase determination is a crucial aspect of cloud property retrieval, although it is not directly included in the state vector due to its binary nature. In the EUMETSAT OCA approach, the cloud phase is initially assumed to be either ice or liquid based on the calculated overcast brightness temperature of the 11 µm channel, with a threshold of 260 K distinguishing between the two (the mixed phase is not explicitly accounted for in the OCA approach). Throughout the retrieval iteration, the phase may be switched based on specific criteria: a change from liquid to ice occurs when the estimated effective radius exceeds 23 µm, prompting a restart of the retrieval assuming the ice phase; conversely, if the effective radius for ice clouds falls below 20 µm, the retrieval is restarted assuming the liquid phase.
We acknowledge the uncertainties in satellite ER and phase retrievals. According to the algorithm theoretical basis document (ATBD) for the optimal cloud analysis product (ATBD for Optimal Cloud Analysis Product, 2016) and supporting algorithm documentation, noise exists in ice ER values due to limitations associated with broken or sub-pixel clouds, as well as mixed-phase or heterogeneous clouds. This noise can result in anomalous ice ER values, such as unusually large ER values (>25 µm) or extremely low ER values across all temperatures. In our study, we consider the clouds ice (hereafter ice clouds), liquid (hereafter water clouds), and total clouds (without any classification). To address the potential misclassification of ice clouds, we implemented a filtering step to exclude any data points where ice retrievals appear at temperatures above 0 °C. This adjustment ensures alignment with expected physical characteristics. Additionally, we invite the readers to refer to the OCA product guides to contextualize and clarify any observed irregularities in the ER data.
2.3 Other datasets
There are six C-band weather radars covering the UAE region. In this study, the dual-polarization vertical profiles from the Al Ain radar (Fig. 1) were used to analyze cloud characteristics. The observation area of that radar overlaps with the aircraft observation region. The quality control (QC) procedure was performed before generating the radar's dual-polarization vertical profiles. The QC procedure includes detecting and removing radio frequency interference (RFI), sea clutter, and noise from the data. RFI and sea clutter removal are based on a fuzzy-logic algorithm (Liu and Chandrasekar, 2000), and the censoring of noisy data is performed using the signal-to-noise ratio (SNR) and normalized coherent power (NCP). After the QC process, the radar data were converted from polar to Cartesian coordinates, with both horizontal and vertical resolutions set at 0.5 km. In the final step, using the location and time of the cloud penetrations conducted by the aircraft, we identified coincident observations from the gridded radar data. We applied a 1 km spatial and a 5 min temporal threshold in the collocation procedure. The radar vertical profiles are generated from the coinciding observations.
The latest version of the reanalysis dataset from the European Centre for Medium-range Weather Forecasts (ERA5; Hersbach et al., 2020) was used in this study to provide an overview of weather conditions for the cloud cases, including the total cloud cover, total-column water, and convective available potential energy (CAPE). The ERA5 data are on a horizontal resolution of about 31 km and 137 vertical levels from the surface up to 0.01 hPa (∼ 80 km). The data used in this study are obtained at a 0.25° horizontal resolution and an hourly temporal resolution. In addition, the temperature sounding profiles observed at the Abu Dhabi airport (Fig. 1) are used to identify temperature inversions.
3.1 Meteorology
First, a synoptic overview for 18 August 2019 (SF03) was conducted using ERA5 and satellite data (Fig. 4) to understand the overall weather conditions, including the total cloud cover, total-column water, and convective available potential energy (CAPE) at 13:00 UTC from ERA5 and the 3 h precipitation amount from satellite data between 12:00 and 15:00 UTC. Meanwhile, SF03 was conducted from 12:53 to 14:31 UTC, and all the CPs are located within the small black box in Fig. 4. The atmosphere within this observation area (the small black box) contained a substantial amount of water vapor, measured as 40–45 mm of total-column water (Fig. 4a). The CPs were located on the east side of a strong convection zone (Fig. 4b), and the total cloud cover was 40 %–70 % (Fig. 4c). Meanwhile, there was a strong temperature inversion layer around 6 km in altitude at a temperature of −5 to −7 °C over this area according to the temperature sounding profile observed at the Abu Dhabi airport at 12:00 UTC and the ERA5 reanalysis (not shown). Temperature inversions are frequently observed during summer in the UAE (Weston et al., 2020), which can suppress convection. Based on the precipitation amount derived from satellite data between 12:00 and 15:00 UTC, the SF03 CPs were over the southeast region of a precipitation area (Fig. 4d). In this study, we only used the reanalysis to explore the synoptic conditions for the clouds sampled by the aircraft. To identify potential cloud targets for rainfall enhancement applications, high-resolution short-term numerical weather forecasts, or nowcasts, provide useful information (total-column water, CAPE, total cloud cover, etc.) to locate potential areas for convective cloud development.
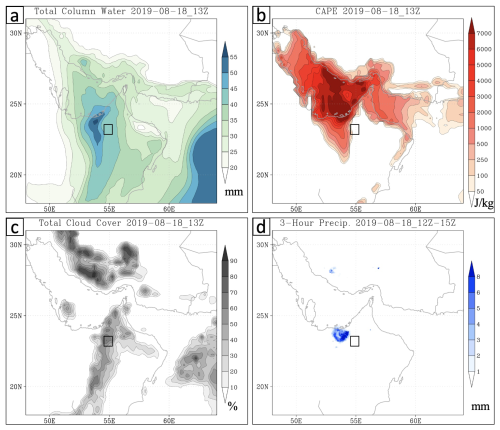
Figure 4(a) The total-column water (mm) at 13:00 UTC on 18 August 2019 from the ERA5 reanalysis. (b) Same as (a) but for the convective available potential energy (CAPE, J kg−1). (c) Same as (a) but for the total cloud cover (%). (d) The precipitation amount (mm) between 12:00 and 15:00 UTC on 18 August 2019 from satellite data. The small black box in each panel shows the location of cloud penetrations identified in SF03.
3.2 Analysis of cloud microphysical parameters from aircraft observations
Aircraft observations provide detailed measurements of the cloud microphysical properties within clouds. The time series of a few selected parameters from SF03 on 18 August 2019 is shown in Fig. 5 as an example. This flight took off at 12:53 UTC and landed at 14:31 UTC. The coincidence of the peak values in total water content from the Nevzorov hot-wire probe and FCDP concentrations showed good agreement in detecting CPs. The first CP occurred at 13:14:34 UTC, with observed peaks in both total water content (1.19 g m−3) and FCDP concentration (860 cm−3) at a temperature of −4.6 °C and a height of 5.8 km. The highest (coldest) CP was conducted at 13:26:35 UTC at a height of 6.9 km and a temperature of −13 °C, with total water content of 0.24 g m−3 and FCDP concentration of 48 cm−3. At 14:05:34 UTC, CPs were detected at around 3.6 km in height, with a temperature of around 9.1 °C. Below that, no other CPs were detected, which indicates a cloud base of 3.6 km, with a total water content of 0.25 g m−3 and FCDP concentration of 1286 cm−3. In total, 29 CPs were identified for this case. The mean effective radii (see Fig. 3) range from 4.6 to 8.9 µm throughout the vertical profile of the cloud and are smaller than the 15 µm threshold for the onset of warm rain based on satellite measurements (Lensky and Drori, 2007). Meanwhile, Freud and Rosenfeld (2012) defined 14 µm as the precipitation threshold for warm rain in convective clouds based on aircraft-retrieved data. Although some CPs occurred very close to each other (a few seconds away), all the CPs were identified and processed as independent CP measurements.
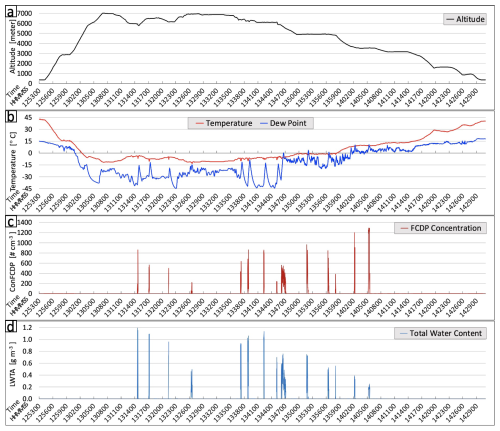
Figure 5The aircraft observations from SF03, including (a) altitude, (b) temperature and dew point, (c) cloud particle concentration from FCDP, and (d) total water content from the Nevzorov probe.
The cloud particle size and the growth rate of the size with height are critical to the formation of rain-sized droplets (Freud and Rosenfeld, 2012). Overall, the ERs of the CPs from SF03 increase with the decrease in temperature (left column of Fig. 6). Four CPs from the cloud base to the cloud top are selected for further analysis, including the cloud particle size distributions (middle column) and 2DS and CPI images (right column) from the research aircraft. The first CP (first row of Fig. 6) is around the cloud base, with a temperature of 9.1 °C. Figure 6b shows the corresponding cloud particle size distribution from three instruments: FCDP, 2DS, and HVPS. The FCDP measures the size and number concentration of cloud droplets in the range of 2–50 µm in diameter (Lawson et al., 2017). The cloud particle size distribution for this CP has the highest concentration at the particle diameter 7–9 µm, decreasing with increasing size (red line in Fig. 6b). The minimum detectable cloud particle size of 2DS is about 10 µm in diameter (Lawson et al., 2006; Baker et al., 2009), and number concentrations are in good agreement with the FCDP for cloud particle sizes larger than 20 µm. Overall, the cloud base penetration at a temperature of 9.1 °C has a high droplet concentration at a relatively small size (<20 µm). This is consistent with the 2DS images for this CP, where large droplets are absent in the 2DS image strips (e.g., Fig. 6c). At colder temperatures of −0.7 °C (second row of Fig. 6), the particle size distribution shifts to larger sizes, with a maximum concentration of around 10 µm in the FCDP, showing very little droplet growth in the main body of the size distribution in the warm part of the cloud. At a temperature of −5.2 °C (third row of Fig. 6), the size distribution has a peak concentration at 11 µm, and the 2DS detects a small number concentration (21 L−1) of particles at 50–200 µm in diameter. The 2DS records a few particles around 100 µm in diameter, and the inspection of the CPI shows that these are spherical and around 100 µm in diameter (Fig. 6i). At a temperature of −12.1 °C (fourth row of Fig. 6), there are significantly more cloud particles around or larger than 100 µm based on 2DS with a concentration of 137 L−1 (Fig. 6k). The particles in the 2DS and CPI images are ice and are larger than 100 µm in diameter (Fig. 6l). The observed ice particles indicate an ice production process that is active around −12 °C in the SF03 case.
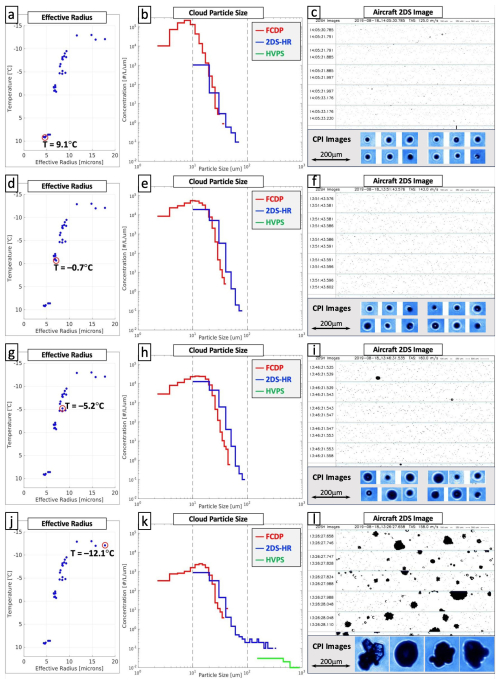
Figure 6The first row is for the cloud penetration at 9.1 °C: (a) the effective radius of each cloud penetration from SF03. The penetration at 9.1 °C is highlighted in a red circle. (b) The distribution of cloud particle size. (c) The 2DS image (top) and CPI image (bottom). Panels (d)–(l) are the same as panels (a)–(c) but for cloud penetration with temperatures of −0.7, −5.2, and −12.1 °C, respectively.
The noticeable features of these observations are that the cloud base temperature, effective radii, droplet number concentrations, and total water content are typical of high-based continental convective clouds composed of a high number concentration of small droplets formed by the diffusional growth of droplets. These droplets have low collision efficiency, and the collision–coalescence process is suppressed, as evidenced by the particle size distributions in Fig. 6b where the peak concentration and the effective radius are smaller than the 15 µm threshold for warm rain (Lensky and Drori, 2007). The cloud penetration at −12 °C indicates that ice production is active at warmer temperatures, which is consistent with ice multiplication within supercooled clouds in the −5 to −8 °C region (Hallett and Mossop, 1974) that depends on the ratio of small (diameter < 13 µm) to large (diameter > 24 µm) cloud droplets.
In general, the analysis of cloud microphysical parameters observed in the SF03 case agrees with Wehbe et al. (2021) for SF01 and SF04, where the dominance of small-sized particles with diameters less than 10 µm and the minimal concentrations of intermediate sizes (10–30 µm) indicate that an active collision–coalescence process was not achieved. Wehbe et al. (2021) postulated that strong updrafts in SF04 may have carried a limited number of large particles aloft to serve as INPs at −10.6 °C, but these were not present in SF01. Although the occurrence of first ice cannot be linked to a specific ice nucleation process, ice production is active in SF03. There are many uncertainties associated with the number concentration of ice particles expected within high-based continental convective clouds within a certain time, especially in a dusty boundary layer, where INP concentrations in the Arabian Basin have a range of up to 2 orders of magnitude at −15 °C, between and L−1 (Beall et al., 2022). However, the tail in the particle size distribution larger than 100 µm (see Fig. 6k) in SF03 is indicative of an active ice production process that is dominant compared to a suppressed collision–coalescence process (see Fig. 6b), where the size distribution shows a high number concentration of small droplets.
3.3 Effective radius from satellite data
While aircraft observations can provide detailed measurements to examine the microphysical features of the cloud, they has a limited sample size of measurements and are usually not available for routine assessment of the dominant physical pathways that lead to precipitation. Thus, more accessible observation data are needed for real-time applications, such as satellite data. As described in Sect. 2.2, we retrieved the cloud particle ERs for each cloud case using the satellite data from the SEVIRI–Meteosat 2nd Generation Indian Ocean dataset.
Before utilizing the ER values retrieved from satellite data, they are validated with the ER measured by aircraft. To ensure the accuracy and relevance of our comparative analysis, the following steps are taken to match the aircraft cloud penetration data with the corresponding satellite data.
- 1.
Temporal collocation. We applied a temporal threshold of 5 min to the aircraft cloud penetrations and the satellite observations to ensure that the measurements were as close in time as possible. This was necessary because of the 15 min temporal resolution of the products from the SEVIRI sensor on board the MSG satellite.
- 2.
Spatial collocation. For spatial collocation, we applied a spatial threshold of 3 km, which allowed us to match the cloud features observed by the aircraft to the satellite data. The aircraft data provide a high-resolution, detailed view of specific cloud penetrations, and this proximity ensures that the satellite data used for comparison represent the same cloud features.
- 3.
Verification of collocation. The collocated data points were further verified by comparing the retrieved ERs from both satellite and aircraft data.
Figure 7 shows the comparison of ERs between the satellite and aircraft datasets for the SF03 case. In each CP from the research aircraft, the 25th, 50th, and 75th percentiles of the ER values are very close to each other because the ERs measured from most aircraft CPs are in a short time period (1 s to several seconds/measurements per CP). Therefore, only the 50th percentiles of ER values are plotted as black dots in Fig. 7. Compared to the satellite-retrieved ERs for total cloud, the aircraft-measured ERs are around the 25th percentile of the satellite ERs (Fig. 7a). This is similar to the results from Rosenfeld and Lensky (1998), who showed that the ERs measured by aircraft were mostly around the 25th percentile of the satellite measurements. The ERs measured by aircraft are further compared to the ERs of water clouds and ice clouds from the satellite. The results show that the aircraft ERs are close to the 50th percentile of the satellite ERs for water clouds when the temperature is relatively warm, indicating fair agreement between these two datasets (Fig. 7c). That is because the aircraft-derived ERs are highly sensitive to the mode of the size distribution that occurs in the range of the FCDP, which measures the size of particles in the 2–50 µm diameter range and is sensitive to water droplets. It is not surprising that the aircraft ERs tend to be smaller than the satellite ERs for ice clouds due to the lack of sensitivity of the FCDP to ice particles. Overall, the ERs from aircraft and satellite datasets have fair agreement, which gives us the confidence to use ERs retrieved from satellite data to analyze the relevant cloud features.
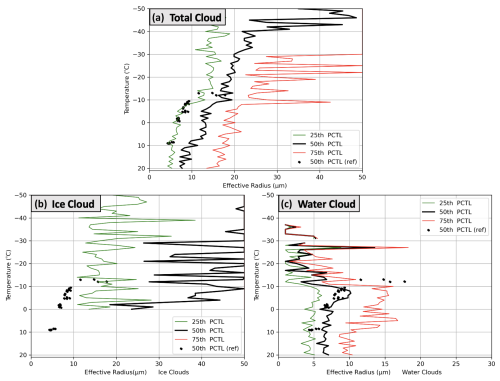
Figure 7Panel (a) shows the 25th (green line), 50th (black line), and 75th (red line) percentiles of the effective radius (ER) for the total cloud in SF03 retrieved from satellite data. Panels (b) and (c) are the same as (a) but for the ice and water clouds from the satellite data, respectively. The black dots are the 50th percentiles of the ER values in each cloud penetration from aircraft observations.
This validation is crucial because the ER products are fundamental to understanding cloud microphysical processes, particularly in assessing phase transitions and particle growth mechanisms across different cloud zones. Furthermore, it is important to note that satellite retrievals, especially at certain wavelengths, may introduce artifacts in satellite-derived ER values due to phase misclassification, as discussed in the ATBD for OCA product (ATBD for Optimal Cloud Analysis product, 2016). To address this, we implemented a filtering step in the satellite data to remove any data points where ice retrievals appear at temperatures above 0 °C, ensuring that the ER values remain within physically realistic limits. This adjustment aligns with findings in Rosenfeld and Lensky (1998), reinforcing the robustness of satellite-derived ER values for microphysical characterization.
3.4 Definition of the cloud zones
When there is sufficient data, the next step is characterizing the cloud microphysical features that are indicators of the dominant microphysical processes leading to precipitation. Rosenfeld and Lensky (1998) introduced a five-zone concept for some cloud cases based on their microphysical features, including the diffusional droplet growth zone, droplet coalescence growth zone, rainout zone, mixed-phase zone, and glaciated zone. In this study, we follow the Rosenfeld and Lensky (1998) concept and propose a refinement to their methodology. To better represent the early development of convective clouds, we replaced the rainout zone with a supercooled-water zone, where supercooled droplets are a hydrometeor type associated with ice production and the growth of ice particles to precipitation sizes in mixed-phase convective clouds. In addition, we add the thresholds of brightness temperature, ER, the growth rate of ER, and the cloud phase to define the zones corresponding to the basic cloud physics principles described in Sect. 1.
The accurate classification of these five zones relies on physically consistent retrieval of ER values and cloud phases. To enhance reliability, we applied phase-specific thresholds, excluding anomalous ER measurements attributed to phase misclassification (e.g., ice retrievals at temperatures > 0 °C) and ensuring that satellite data represent cloud microphysics. In our analysis, we consider the number of samples for each zone to provide insight into data density, enhancing our understanding of data consistency within each classification. These adjustments are integrated throughout the zones, especially in differentiating water-dominated and ice-dominated regions, and contribute to a clearer distinction between microphysical processes.
Figure 8 is a framework to detect the five zones using satellite data. The thresholds in the framework were determined based on the analysis of our SF03 case, then tested using different cloud cases (SF01, SF06, and SF07) and validated using aircraft observations (Sect. 4). The definitions of the five zones and the corresponding thresholds for satellite data are listed below.
- 1.
Zone 1 – diffusional droplet growth zone. This zone is usually close to the cloud base, with relatively small particle size and very slow growth of particle size. Thus, it is detected using the satellite data of the water-phase cloud and identified when brightness temperature (T) > 0 °C and the 50th percentile of ER < 10 µm. When this zone exists and is relatively deep, the precipitation-forming processes are suppressed, indicating the potential for rainfall enhancement. Our choice of diffusional droplet growth at T > 0 °C and ER < 10 µm is based on the cloud ER in the UAE during the summer (August 2019) and aims to identify extreme cases of diffusional growth to also eliminate cases when coalescence may be marginally active. This is to identify the best targets for cloud seeding using hygroscopic particles, which is most effective in cases when coalescence is not active. When we reference hygroscopic seeding, we assume that the objective is to enhance precipitation. In this case, the hygroscopic particles are ultra-giant cloud condensation nuclei (UGCCNs), which, when introduced at the cloud base and in the updraft region, enhance the coalescence of warm-based clouds, accelerating the warm-rain process (Rosenfeld et al., 2010).
- 2.
Zone 2 – the droplet coalescence growth zone. The particle size's growth rate in this zone is relatively large, indicating a quick cloud particle growth above the cloud base through a collision–coalescence process. Thus, it is detected using the water-phase cloud and identified when T is lower than the coldest temperature in Zone 1 (TZ1) and higher than −10 °C, when the 75th percentile of ER is between 15 and 20 µm, and when the growth rate is relatively large ( < −0.4 µm °C−1). The 75th percentile of ER is used to identify the relatively large droplets developed by collision and coalescence. Although there are still many small droplets, some droplets may start to grow quickly due to coalescence, which will result in a long tail in the droplet size distribution. When Zone 2 exists and is relatively deep, the precipitation-forming processes are active, and the potential for rainfall enhancement using hygroscopic particles (e.g., ultra-giant cloud condensation nuclei) is low.
- 3.
Zone 3 – the supercooled-water zone. This zone has water particles at a temperature considerably below the freezing temperature, and the growth rate of the particle size is relatively slow. Thus, it is detected using the water-phase cloud and identified when 0 °C > T > −38 °C, when the 50th percentile of ER < 20 µm, and when the growth rate () is between −0.4 and 0.0 µm °C−1. The supercooled-water zone is designed according to the requirements of the cloud seeding operator for conducting hygroscopic and glaciogenic seeding. In glaciogenic seeding, the operators will target supercooled water, even when this supercooled region overlaps with the presence of high coalescence activity. The main difference between Zone 2 and Zone 3 is the growth rates (). When Zone 3 exists and is sufficiently deep, the precipitation-forming processes are usually suppressed, indicating the potential for rainfall enhancement by hygroscopic and/or glaciogenic seeding.
- 4.
Zone 4 – the mixed-phase zone. This zone has a mixed phase with relatively large particles and rapid particle size growth that usually occurs at relatively low temperatures. Thus, it is detected using satellite data from the total cloud and is identified when −10 °C > T > −38 °C, when the 75th percentile of ER > 20 µm, and when the growth rate < −0.4 µm °C−1. Based on the aircraft observations in this UAE campaign in August 2019, the warmest temperature when ice particles were measured is at least −10 °C. Therefore, we use −10 °C as the minimum threshold. A deep Zone 4 usually indicates suppressed precipitation-forming processes and the potential for rainfall enhancement by glaciogenic seeding.
- 5.
Zone 5 – the glaciated zone. It is a nearly stable zone of ER, and the glaciated particle size is usually large. Thus, it is detected using the ice-phase cloud and identified when T is lower than −10 °C and when the 75th percentile of ER > 25 µm. If Zone 5 exists, the cloud has active precipitation-forming processes, which indicates that the potential for rainfall enhancement is low.
The 75th percentile of ER is used to identify zones 2, 4, and 5 to detect the relatively large particles in the tail of the particle size distribution that form by coalescence or ice formation. Figure S3 shows an example from one cloud penetration at a temperature of −5.2 °C from SF03. The 75th percentile is more sensitive to those large particles compared to the 50th percentile.
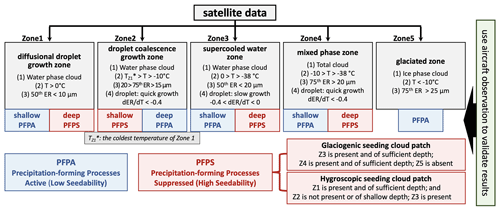
Figure 8The flowchart of the five-zone framework, including (1) the diffusional droplet growth zone, (2) the droplet coalescence growth zone, (3) the supercooled-water zone, (4) the mixed-phase zone, and (5) the glaciated zone. The blue boxes indicate that precipitation-forming processes are active (PFPA), and the red boxes indicate that precipitation-forming processes are suppressed (PFPS).
Overall, the five zones have different microphysical features, and their definitions are motivated by the requirements of the cloud seeding operator to conduct hygroscopic and/or glaciogenic seeding, as labeled in Fig. 8. Generally, when the cloud is identified as having suppressed precipitation-forming processes, it could be a suitable target for precipitation enhancement. Conversely, when the cloud is identified as having active precipitation-forming processes, it may not be suitable for precipitation enhancement. Figure 8 defines zones by their microphysical features, where active precipitation-forming processes (PFPA) and suppressed precipitation-forming processes (PFPS) are indicated, and the corresponding identification of hygroscopic seeding and glaciogenic seeding patches are shown in the bottom-right corner, which will be discussed in the last section, “Summary and discussion”.
An example of a cloud patch at a specific time from SF03 is utilized to demonstrate how to use the five-zone framework to identify the zones. Figure 9a shows some cloud patches (different colors) from the satellite data on 18 August 2019, and the one outlined by a red circle is selected to detect the zones. Figure 9c and d show the ERs for the water, ice, and total-cloud phases. As mentioned before, to provide further context and transparency, we also plotted the number of samples corresponding to each percentile in the figures, which enables a clearer interpretation of the sample size and variability across different cloud types and phases. This inclusion helps highlight the robustness of our satellite-derived ER values, particularly in cases with sufficient data density. In the water cloud, the algorithm detects deep Zone 1 (vertical purple bar) and Zone 3 (vertical cyan bar) layers; Zone 2 is not detected. In the total cloud, the 75th percentile of ER grows quickly between the temperatures of −37 and −11 °C, identified as Zone 4 (vertical yellow bar). Meanwhile, Zone 5 is identified at temperatures lower than −39 °C (vertical orange bar) using the ice cloud satellite data. Overall, due to the present and sufficient depth of zones 1, 3, and 4, this cloud patch is categorized as having precipitation-forming processes suppressed, indicating that it is a potential target for rainfall enhancement.
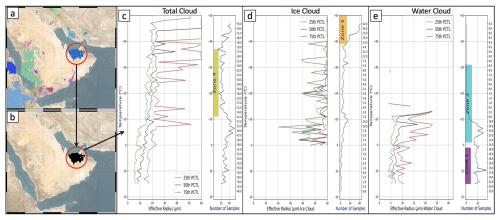
Figure 9(a) Examples of cloud patches (colors) detected in satellite data. (b) The cloud patch selected for the analysis of effective radius. (c) The effective radius from satellite data for the total cloud (left) and the number of data samples (right); the vertical yellow bar represents Zone 4, the mixed-phase zone. Panel (d) is the same as (c) but for the ice cloud, and the vertical orange bar represents Zone 5, the glaciated zone. Panel (e) is the same as (c) but for the water cloud, and the vertical purple and cyan bars represent Zone 1 (diffusional growth zone) and Zone 3 (supercooled-water zone), respectively. The data were excluded if they were below the lifted condensation level (LCL, which approximates the cloud base; 15 °C according to the skew-T observed at Abu Dhabi at 00:00 UTC on the same day of this cloud case).
It is worth noting that the satellite data below the cloud base were excluded in Fig. 9. The cloud base in this case captured by the aircraft had a temperature of about 9.1 °C (Fig. 6a). Meanwhile, according to the skew-T chart (not shown) from the sounding observed in Abu Dhabi at 00:00 UTC on the same day as this cloud case (the closest sounding observation), the lifted condensation level (LCL, approximating the cloud base) was 15 °C. That is warmer than the cloud base temperature captured by the aircraft (∼ 9.1 °C), possibly due to the differences in time and location between the sounding and aircraft observations. If the cloud base temperature from a sounding observation at the same time and location as the cloud case is available, it can be used to accurately exclude the satellite data below the cloud base and inhibit the corresponding uncertainties in the depth of the diffusional growth zone (Zone 1) if it is identified.
In the previous section, we focused on the SF03 cloud case and introduced the five-zone framework to identify different cloud microphysical zones. In this section, the five-zone framework is tested in more cloud cases, and the results are validated using aircraft observations to evaluate the transferability of the five-zone concept.
First, more examples of the zone detection at specific time points are provided. Figure 10 shows the identification of the zones at two different time points for a developing cloud on 18 August 2019. In Fig. 10a, the black object in the top panel is the cloud patch from the satellite data at 13:57 UTC, and Fig. 10b–d show the zones 1 and 5 that were identified from the satellite data of water and ice clouds, respectively. After 2 h of development, this cloud patch covers a larger area at 15:57 UTC (Fig. 10e), and in addition to zones 1 and 5, zones 2, 3, and 4 are also detected based on the water and total-cloud data (Fig. 10f–h). For each cloud zone, we have also included plots of the number of samples, which visually represent sample size across the defined thresholds. In addition to SF03, Fig. 11 shows the identification of the zones for the SF07 cloud case on 23 August 2019. At 10:12 UTC, the cloud is mainly over the southwest side of the Hajar Mountains, and zones 1, 3, and 4 are detected in the water and total-cloud data (Fig. 11a–d). After 2.5 h, the cloud target becomes significantly smaller, but zones 1, 3, and 4 still exist at 12:42 UTC (Fig. 11e–h).
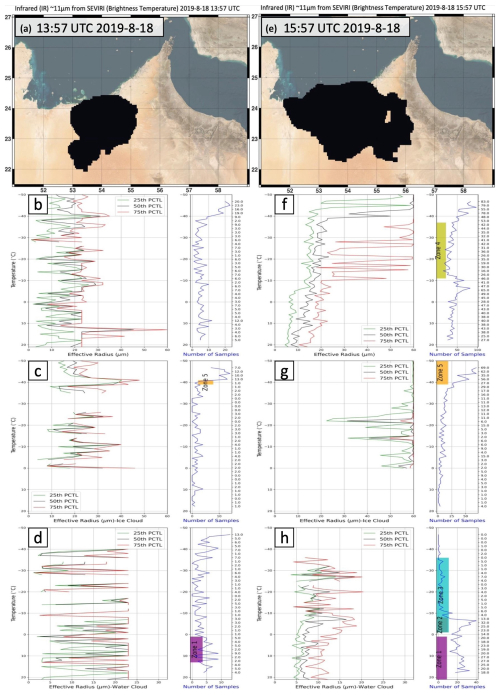
Figure 10The zones detected in one cloud patch on 18 August 2019. (a) The object of the cloud patch at 13:57 UTC. (b–d) The effective radius (left) and the number of data samples (right) for the total-, ice, and water cloud phases, respectively. Panels (e)–(h) are the same as panels (a)–(d) but for the same cloud patch at 15:57 UTC.
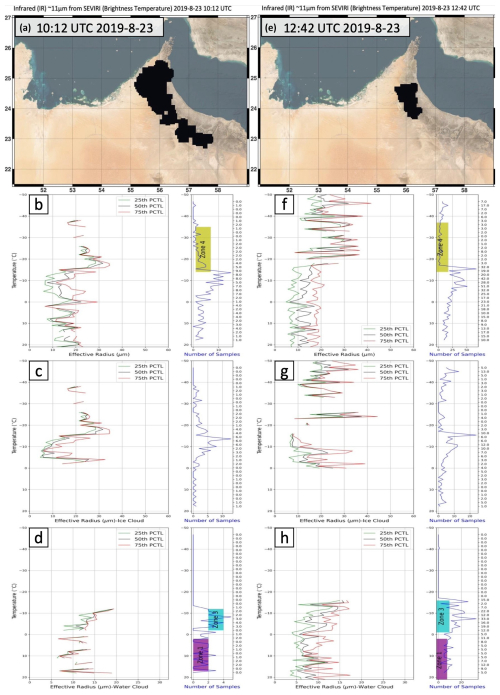
Figure 11The same as Fig. 10 but for a cloud patch at (a–d) 10:12 UTC and (e–h) 12:42 UTC on 23 August 2019 (SF07).
The same detection processes can be repeated for any cloud patch through its life cycle to examine the microphysical evolution of the cloud. To test the transferability of the five-zone concept, the cloud zones are detected using the satellite data from the time periods of the SF01, SF03, SF06, and SF07 cloud cases (Fig. 12). These four cases were selected because they have sufficient satellite data to identify the zones and have aircraft measurements to validate the results (Fig. 13). SF02 and SF04 are not presented here because of limited collocated aircraft data samples and the cloudy pixels/points in the satellite images for the ice and water phases.
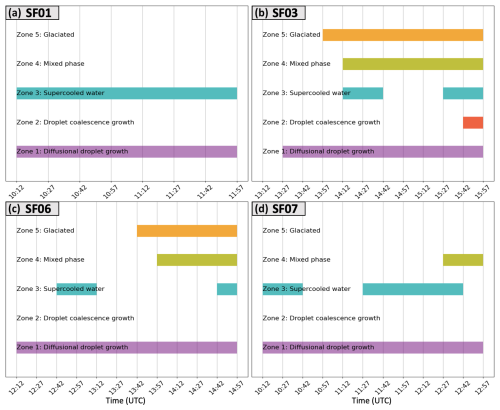
Figure 12The evolution of the five zones in (a) the SF01 cloud case, (b) the SF03 cloud case, (c) the SF06 cloud case, and (d) the SF07 cloud case. The x axis is time, and the y axis denotes the five zones.
Although all the cloud cases sampled are young targets in the early- to mid-life-cycle convection, as previously discussed, there are differences in the zones detected across different cases. In the SF01 cloud case on 12 August 2019, only zones 1 and 3 are detected. These zones are detected continuously from 10:12 to 11:57 UTC (Fig. 12a), indicating suppressed precipitation-forming processes for this case. The SF03 and SF06 cloud cases have a similar evolution of the zones, including a continuous Zone 1, discontinuous Zone 3, and zones 4 and 5 during the middle and later periods. The difference is that in the SF03 case, zones 4 and 5 develop earlier and last longer, indicating that the SF03 case has a more active ice production process. In the SF07 case, only zones 1 and 3 are detected for a significant time period, suggesting suppressed precipitation-forming processes.
To validate the results of the zones based on satellite data, we examined the aircraft observations for those four cloud cases. The SF03 case is intensively examined in Sect. 3 as an example. Here, to conduct a comparison among the four cases, we selected an aircraft CP with a similar temperature (−12.1 °C for the CPs from SF03 and SF07, −12.3 °C for SF01, and −12.0 °C for SF06) from each case and examined the cloud particle distribution and the 2DS images (Fig. 13). A relatively cold temperature (around −12 °C) is selected because the main difference among those four cases based on our five-zone framework is that SF03 and SF06 have zones 4 and 5 but SF01 and SF07 do not, indicating a difference in the ice production process. The CPs around −12 °C are relatively cold for these clouds at the early- to mid-life-cycle stage, which presents the best validation for the differences in properties of zones 4 and 5. The cloud particle sizes from SF01 and SF07 are more concentrated at the relatively small size (Fig. 13a and d). They have a higher concentration (close to or over ) than SF03 (below ) and SF06 (below ) from FCDP in the range of 10–20 µm, which implies that the droplet growth in the SF01 and SF07 cloud cases is suppressed. The particle size distribution from 2DS and HVPS in SF03 has a long tail toward the large size, 100–1000 µm (Fig. 13b), while the distribution in SF06 indicates large particles around 100 µm (Fig. 13c). The 2DS image examples in the right column of Fig. 13 are in agreement with the cloud particle distribution for particles greater than 20 µm. The 2DS image for SF03 shows many ice particles significantly larger than 100 µm, consistent with the continuous zones 4 and 5 detected based on satellite data. The 2DS image for SF06 shows a few large particles around or larger than 100 µm, which has fair agreement with zones 4 and 5, which exist for a relatively short period of time. The CPI images for the corresponding CPs for the SF01, SF03, and SF06 cloud cases show similar results (right column in Fig. 13). The CPI images capture the large ice particles in SF03 and SF06, while the image for SF01 does not have any large particles. The CPI image for SF07 is not included since it does not have any images available around the time of that CP (within 10 s before and after that CP).
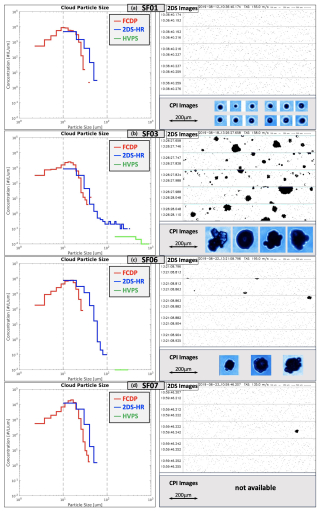
Figure 13(a) The distributions of cloud particle size (left), 2DS images (top right), and CPI images (bottom right) for the cloud penetration at a temperature of −12.3 °C in SF01. (b) Same as (a) but for the cloud penetration at a temperature of −12.1 °C in SF03. (c) Same as (a) but for the cloud penetration at a temperature of −12.0 °C in SF06. (d) Same as (a) but for the cloud penetration at a temperature of −12.1 °C in SF07; there are no CPI images.
Overall, the five-zone framework identifies the microphysical evolution of all four cloud cases, and the aircraft observations support the zones identified based on satellite data.
In this study, we investigated the microphysical features of clouds in the UAE using aircraft observations, introduced a five-zone framework to identify cloud microphysical zones using satellite data, and validated the zones detected by satellite data using aircraft measurements. Our study aims to provide scientific support to develop an applicable framework to examine cloud microphysical processes and detect cloud features that could be targeted for precipitation enhancement in the UAE. A summary of this study is listed below.
-
The UAE 2019 airborne campaign provides a unique aircraft sensor dataset, which is analyzed to examine the microphysical features of some cloud cases in the UAE.
-
The effective radius (ER) retrieved from satellite data is in fair agreement with the ER measured by aircraft, boosting our confidence in the use of ER data from satellites to analyze the cloud microphysical features.
-
Following Rosenfeld and Lensky (1998), a new five-zone framework was developed to identify the cloud microphysical zones using satellite data, which can be used to describe the cloud microphysical processes and rainfall enhancement potential.
-
The five-zone framework can successfully detect the cloud microphysical zones, including the glaciated zone with large ice particles. The results were validated with aircraft measurements for four cloud cases.
In addition to satellite data, radar data are often used to examine the impacts of cloud seeding (e.g., Vujović and Protić, 2017; Zaremba et al., 2024; Wang et al., 2021). Meanwhile, the radar data might be a potential data source, providing additional information to refine the detection of precipitation-forming processes. We considered radar reflectivity as a supplementary data source to characterize the cloud features related to precipitation. However, only the radar in Al Ain (Fig. 1) overlaps with the observation area of the three research flights (SF03, SF06, and SF07). We explored the potential relationship between the radar reflectivity and the cloud's microphysical features, as summarized in the Supplement. Due to the limited number of available samples, it is difficult to connect the radar data and the cloud microphysical zones. More samples are needed to investigate the potential usage of radar data in detecting the cloud microphysical zones.
The five-zone framework used in this study is based on the five-zone concept from Rosenfeld and Lensky (1998), but it uses a supercooled-water zone instead of the rainout zone since this study focuses on the microphysical processes related to precipitation of convective clouds. In addition, the thresholds of temperature, ER, ER growth rate, and cloud phase are added for each zone in our framework. We used previous studies (Rosenfeld and Lensky, 1998; Wang et al., 2019) as conceptual references and the data from intensive analysis of the SF03 cloud case to determine those thresholds and then refined them through a case study analysis of other cloud cases. Since all these cloud cases occur during summer in the UAE, the thresholds determined in this study are considered specific to the summer (primarily convective systems) over the UAE. If this five-zone framework is utilized for different seasons or climate zones, a corresponding modification of those thresholds is needed due to the differences in the cloud microphysical features and the environmental aerosols. However, this framework presents a methodology that could be tuned to different seasons and climate zones to identify other cloud types using the threshold parameters identified here.
The five-zone framework presents a concept to identify the cloud microphysical zones and diagnose the cloud microphysical processes that affect precipitation. Summarized in Fig. 14, this framework focuses on the growth of cloud particle size, which can be quantified using the effective radius retrieved from satellite data. When the precipitation-forming processes are active (Fig. 14a), such as a deep coalescence zone or the presence of a deep glaciated zone, precipitation may occur efficiently. On the other hand, the precipitation-forming processes are suppressed (Fig. 14b) when a deep diffusional growth zone (the coalescence process is suppressed) is present or a deep supercooled-water zone (the ice particle production process is not active) is present. In the five-zone framework, we included thresholds to guide the mode of precipitation enhancement for the cases when suppressed precipitation-forming processes are detected, as shown in red at the bottom of Fig. 8. The clouds with suppressed precipitation-forming processes could be glaciogenic (cold cloud) seeding targets if the supercooled-water zone is present and has sufficient depth, the mixed-phase zone is present and has sufficient depth, or the glaciated zone is absent, which indicate that the ice particle production process is not active. On the other hand, the cloud could be a hygroscopic (warm cloud) seeding target if the diffusional droplet growth zone is present and has sufficient depth, the droplet coalescence growth zone is not present or is shallow, or the supercooled-water zone is present, which indicate that the cloud droplet collision–coalescence process is suppressed. In addition to determining rainfall enhancement targets for climatological analysis or feasibility studies, this information about seeding mode (hygroscopic and/or glaciogenic) is advantageous to guide cloud seeding operations in near-real time.
In conclusion, this study has successfully introduced and tested a five-zone framework to identify cloud microphysical zones using satellite data, focusing on cloud microphysical processes related to precipitation and the potential for rainfall enhancement in the UAE during summer. The performance of the framework was demonstrated through the analysis of cloud cases and validated with aircraft measurements. Future work will aim to enhance this approach by incorporating a machine-learning-based cloud tracking algorithm applied to MSG data, allowing for a more detailed examination of microphysical zones in near-real time within individually tracked cloud clusters. This advancement will further our understanding of cloud precipitation processes and improve the identification of suitable targets for precipitation enhancement.
The ERA5 data can be accessed at the ECMWF Climate Data Store: https://doi.org/10.24381/cds.143582cf (Hersbach et al., 2017). The high-rate SEVIRI level 1.5 image data can be assessed from the EUMETSAT Data Centre at https://data.eumetsat.int/product/EO:EUM:DAT:MSG:HRSEVIRI-IODC (EUMETSAT, 2025a). The SEVIRI optimal cloud analysis products can be assessed from the EUMETSAT Data Centre at https://user.eumetsat.int/catalogue/EO:EUM:DAT:MSG:OCA-IODC (EUMETSAT, 2025b). The aircraft observations are archived at the UAE National Center of Meteorology. Readers can request the dataset by contacting research@ncms.ae.
The supplement related to this article is available online at https://doi.org/10.5194/amt-18-1981-2025-supplement.
ZZ analyzed cloud microphysical features using aircraft observation data, examined the meteorological conditions using ERA5 data, and wrote the paper with contributions from all co-authors. VAG performed the analysis of cloud particle effective radius using satellite data and developed the code to detect the five zones using satellite data. DA conceptualized and supervised this study and guided the analysis. LDM supervised this study and reviewed the paper. CR, EYK, and VC processed the radar data and helped with the interpretation of the radar data.
The contact author has declared that none of the authors has any competing interests.
Any opinions, findings and conclusions or recommendations expressed in this material are those of the author(s) and do not necessarily reflect the views of the National Center of Meteorology, Abu Dhabi, UAE, funder of the research.
Publisher's note: Copernicus Publications remains neutral with regard to jurisdictional claims made in the text, published maps, institutional affiliations, or any other geographical representation in this paper. While Copernicus Publications makes every effort to include appropriate place names, the final responsibility lies with the authors.
The authors acknowledge Paul Lawson and Brad Baker from the Stratton Park Engineering Company (SPEC) for providing the aircraft observation data from the UAE 2019 airborne campaign. The authors also acknowledge Youssef Wehbe and Michael Weston from NCM UAE for their review and valuable comments on this paper.
This research has been supported by the National Center of Meteorology, Abu Dhabi, UAE (award no. 30128758). This material is based on work supported by the National Center of Meteorology, Abu Dhabi, UAE, under the UAE Research Program for Rain Enhancement Science.
This paper was edited by Cuiqi Zhang and reviewed by Daniel Rosenfeld and two anonymous referees.
Al Hosari, T., Al Mandous, A., Wehbe, Y., Shalaby, A., Al Shamsi, N., Al Naqbi, H., Al Yazeedi, O., Al Mazroui, A., and Farrah, S.: The UAE cloud seeding program: A statistical and physical evaluation, Atmosphere-Basel, 12, 1013, https://doi.org/10.3390/atmos12081013, 2021.
ATBD for Optimal Cloud Analysis Product: https://user.eumetsat.int/s3/eup-strapi-media/pdf_mtg_atbd_oca_b1ba8fc34c.pdf (last access: 18 November 2024), 2016.
Axisa, D. and DeFelice, T. P.: Modern and prospective technologies for weather modification activities: A look at integrating unmanned aircraft systems, Atmos. Res., 178, 114–124, https://doi.org/10.1016/j.atmosres.2016.03.005, 2016.
Baker, B., Mo, Q., Lawson, R. P., O'Connor, D., and Korolev, A.: The effects of precipitation on cloud droplet measurement devices, J. Atmos. Ocean. Tech., 26, 1404–1409, https://doi.org/10.1175/2009JTECHA1191.1, 2009.
Bartlett, J. T.: The growth of cloud droplets by coalescence, Q. J. Roy. Meteor. Soc., 92, 93–104, https://doi.org/10.1002/qj.49709239108, 1966.
Beall, C. M., Hill, T. C. J., DeMott, P. J., Köneman, T., Pikridas, M., Drewnick, F., Harder, H., Pöhlker, C., Lelieveld, J., Weber, B., Iakovides, M., Prokeš, R., Sciare, J., Andreae, M. O., Stokes, M. D., and Prather, K. A.: Ice-nucleating particles near two major dust source regions, Atmos. Chem. Phys., 22, 12607–12627, https://doi.org/10.5194/acp-22-12607-2022, 2022.
Brenguier, J. L., Bourrianne, T., Coelho, A. A., Isbert, J., Peytavi, R., Trevarin, D., and Weschler, P.: Improvements of droplet size distribution measurements with the Fast-FSSP (Forward Scattering Spectrometer Probe), J. Atmos. Ocean. Tech., 15, 1077–1090, https://doi.org/10.1175/1520-0426(1998)015<1077:IODSDM>2.0.CO;2, 1998.
Bruintjes, R. T.: A review of cloud seeding experiments to enhance precipitation and some new prospects, B. Am. Meteorol. Soc., 80, 805–820, https://doi.org/10.1175/1520-0477(1999)080<0805:AROCSE>2.0.CO;2, 1999.
Cooper, W. A., Bruintjes, R. T., and Mather, G. K.: Calculations pertaining to hygroscopic seeding with flares, J. Appl. Meteorol., 36, 1449–1469, https://doi.org/10.1175/1520-0450(1997)036<1449:CPTHSW>2.0.CO;2, 1997.
Defelice, T. P. and Axisa, D.: Developing the framework for integrating autonomous unmanned aircraft systems into cloud seeding activities, J. Aeronaut. Aerospace Eng., 5, 1–6, https://doi.org/10.4172/2168-9792.1000172, 2016.
DeFelice, T. P., Axisa, D., Bird, J. J., Hirst, C. A., Frew, E. W., Burger, R. P., Baumgardner, D., Botha, G., Havenga, H., Breed, D., Bornstein, S., Choate, C., Gomez-Faulk, C., and Rhodes, M.: Modern and prospective technologies for weather modification activities: A first demonstration of integrating autonomous uncrewed aircraft systems, Atmos. Res., 290, 106788, https://doi.org/10.1016/j.atmosres.2023.106788, 2023.
EUMETSAT: High-Rate SEVIRI Level 1.5 Image Data, EUMETSAT Data Centre [data set], https://data.eumetsat.int/product/EO:EUM:DAT:MSG:HRSEVIRI-IODC, last access: 23 April 2025a.
EUMETSAT: Optimal Cloud Analysis – MSG, EUMETSAT Data Centre [data set], https://user.eumetsat.int/catalogue/EO:EUM:DAT:MSG:OCA-IODC, last access: 23 April 2025b.
Feng, Z., Leung, L. R., Houze Jr, R. A., Hagos, S., Hardin, J., Yang, Q., Han, B., and Fan, J.: Structure and evolution of mesoscale convective systems: Sensitivity to cloud microphysics in convection-permitting simulations over the United States, J. Adv. Model. Earth Sy., 10, 1470–1494, https://doi.org/10.1029/2018MS001305, 2018.
Flossmann, A. I., Manton, M., Abshaev, A., Bruintjes, R., Murakami, M., Prabhakaran, T., and Yao, Z.: Review of advances in precipitation enhancement research, B. Am. Meteorol. Soc., 100, 1465–1480, https://doi.org/10.1175/BAMS-D-18-0160.1, 2019.
Freud, E. and Rosenfeld, D.: Linear relation between convective cloud drop number concentration and depth for rain initiation, J. Geophys. Res.-Atmos., 117, D02207, https://doi.org/10.1029/2011JD016457, 2012.
Fu, D., Di Girolamo, L., Rauber, R. M., McFarquhar, G. M., Nesbitt, S. W., Loveridge, J., Hong, Y., van Diedenhoven, B., Cairns, B., Alexandrov, M. D., Lawson, P., Woods, S., Tanelli, S., Schmidt, S., Hostetler, C., and Scarino, A. J.: An evaluation of the liquid cloud droplet effective radius derived from MODIS, airborne remote sensing, and in situ measurements from CAMP2Ex, Atmos. Chem. Phys., 22, 8259–8285, https://doi.org/10.5194/acp-22-8259-2022, 2022.
Geresdi, I., Xue, L., Chen, S., Wehbe, Y., Bruintjes, R., Lee, J. A., Rasmussen, R. M., Grabowski, W. W., Sarkadi, N., and Tessendorf, S. A.: Impact of hygroscopic seeding on the initiation of precipitation formation: results of a hybrid bin microphysics parcel model, Atmos. Chem. Phys., 21, 16143–16159, https://doi.org/10.5194/acp-21-16143-2021, 2021.
Hadizadeh, M., Rahnama, M., Kamali, M., Kazemi, M., and Mohammadi, A.: A new method to estimate cloud effective radius using Meteosat Second Generation SEVIRI over Middle East, Adv. Space Res., 64, 933–943, https://doi.org/10.1016/j.asr.2019.05.035, 2019.
Hallett, J. and Mossop, S.: Production of secondary ice particles during the riming process, Nature, 249, 26–28, https://doi.org/10.1038/249026a0, 1974.
Hersbach, H., Bell, B., Berrisford, P., Hirahara, S., Horányi, A., Muñoz-Sabater, J., Nicolas, J., Peubey, C., Radu, R., Schepers, D., Simmons, A., Soci, C., Abdalla, S., Abellan, X., Balsamo, G., Bechtold, P., Biavati, G., Bidlot, J., Bonavita, M., De Chiara, G., Dahlgren, P., Dee, D., Diamantakis, M., Dragani, R., Flemming, J., Forbes, R., Fuentes, M., Geer, A., Haimberger, L., Healy, S., Hogan, R. J., Hólm, E., Janisková, M., Keeley, S., Laloyaux, P., Lopez, P., Lupu, C., Radnoti, G., de Rosnay, P., Rozum, I., Vamborg, F., Villaume, S., and Thépaut, J.-N.: Complete ERA5 from 1940: Fifth generation of ECMWF atmospheric reanalyses of the global climate, Copernicus Climate Change Service (C3S) Data Store (CDS) [data set], https://doi.org/10.24381/cds.143582cf, 2017.
Hersbach, H., Bell, B., Berrisford, P., Hirahara, S., Horányi, A., Muñoz-Sabater, J., Nicolas, J., Peubey, C., Radu, R., Schepers, D., Simmons, A., Soci, C., Abdalla, S., Abellan, X., Balsamo, G., Bechtold, P., Biavati, G., Bidlot, J., Bonavita, M., De Chiara, G., Dahlgren, P., Dee, D., Diamantakis, M., Dragani, R., Flemming, J., Forbes, R., Fuentes, M., Geer, A., Haimberger, L., Healy, S., Hogan, R. J., Hólm, E., Janisková, M., Keeley, S., Laloyaux, P., Lopez, P., Lupu, C., Radnoti, G., de Rosnay, P., Rozum, I., Vamborg, F., Villaume, S., and Thépaut, J.-N.: The ERA5 global reanalysis, Q. J. Roy. Meteor. Soc., 146, 1999–2049, https://doi.org/10.1002/qj.3803, 2020.
Hirst, C. A., Bird, J. J., Burger, R., Havenga, H., Botha, G., Baumgardner, D., DeFelice, T., Axisa, D., and Frew, E. W.: An autonomous uncrewed aircraft system performing targeted atmospheric observation for cloud seeding operations, Field Robotics, 3, 687–724, https://doi.org/10.55417/fr.2023022, 2023.
Hussein, K. A., Alsumaiti, T. S., Ghebreyesus, D. T., Sharif, H. O., and Abdalati, W.: High-resolution spatiotemporal trend analysis of precipitation using satellite-based products over the United Arab Emirates, Water-Sui, 13, 2376, https://doi.org/10.3390/w13172376, 2021.
King, M. D., Tsay, S.-C., Platnick, S. E., Wang, M., and Liou, K.-N.: Cloud Retrieval Algorithms for MODIS: Optical Thickness, Effective Particle Radius, and Thermodynamic Phase, MODIS Algorithm Theoretical Basis Document No. ATBD-MOD-05 MOD06 – Cloud product, https://cimss.ssec.wisc.edu/dbs/China2011/Day2/Lectures/MOD06OD_Algorithm_Theoretical_Basis_Document.pdf (last access: 23 April 2025), 1997.
Korolev, A. V., Strapp, J. W., Isaac, G. A., and Nevzorov, A. N.: The Nevzorov airborne hot-wire LWC–TWC probe: Principle of operation and performance characteristics, J. Atmos. Ocean. Tech., 15, 1495–1510, https://doi.org/10.1175/1520-0426(1998)015<1495:TNAHWL>2.0.CO;2, 1998.
Korolev, A. V., Emery, E. F., Strapp, J. W., Cober, S. G., Isaac, G. A., Wasey, M., and Marcotte, D.: Small ice particles in tropospheric clouds: Fact or artifact? Airborne Icing Instrumentation Evaluation Experiment, B. Am. Meteorol. Soc., 92, 967–973, https://doi.org/10.1175/2010BAMS3141.1, 2011.
Kumar, K. N. and Suzuki, K.: Assessment of seasonal cloud properties in the United Arab Emirates and adjoining regions from geostationary satellite data, Remote Sens. Environ., 228, 90–104, https://doi.org/10.1016/j.rse.2019.04.024, 2019.
Lawson, R. P.: Effects of ice particles shattering on the 2D-S probe, Atmos. Meas. Tech., 4, 1361–1381, https://doi.org/10.5194/amt-4-1361-2011, 2011.
Lawson, R. P., Stewart, R. E., and Angus, L. J.: Observations and numerical simulations of the origin and development of very large snow-flakes, J. Atmos. Sci., 55, 32092–3229, https://doi.org/10.1175/1520-0469(1998)055<3209:oansot>2.0.co;2, 1998.
Lawson, R. P., Baker, B. A., Schmitt, C. G., and Jensen, T. L.: An overview of microphysical properties of Arctic clouds observed in May and July during FIRE, J. Geophys. Res.-Atmos., 106, 14989–15014, https://doi.org/10.1029/2000JD900789, 2001.
Lawson, R. P., O'Connor, D., Zmarzly, P., Weaver, K., Baker, B., Mo, Q., and Jonsson, H.: The 2D-S (stereo) probe: Design and preliminary tests of a new airborne, high speed, high-resolution particle imaging probe, J. Atmos. Ocean. Tech., 23, 1462–1477, https://doi.org/10.1175/JTECH1927.1, 2006.
Lawson, R. P., Gurganus, C., Woods, S., and Bruintjes, R.: Aircraft observations of cumulus microphysics ranging from the tropics to midlatitudes: Implications for a “new” secondary ice process, J. Atmos. Sci., 74, 2899–2920, https://doi.org/10.1175/JAS-D-17-0033.1, 2017.
Lazri, M., Ameur, S., Brucker, J. M., and Ouallouche, F.: Convective rainfall estimation from MSG/SEVIRI data based on different development phase duration of convective systems (growth phase and decay phase), Atmos. Res., 147, 38–50, https://doi.org/10.1016/j.atmosres.2014.04.019, 2014.
Lensky, I. M. and Drori, R.: A satellite-based parameter to monitor the aerosol impact on convective clouds, J. Appl. Meteorol. Clim., 46, 660–666, https://doi.org/10.1175/JAM2487.1, 2007.
Lensky, I. M. and Shiff, S.: Using MSG to monitor the evolution of severe convective storms over East Mediterranean Sea and Israel, and its response to aerosol loading, Adv. Geosci., 12, 95–100, https://doi.org/10.5194/adgeo-12-95-2007, 2007.
Liu, H. and Chandrasekar, V.: Classification of Hydrometeors Based on Polarimetric Radar Measurements: Development of Fuzzy Logic and Neuro-Fuzzy Systems, and In Situ Verification, J. Atmos. Ocean. Tech., 17, 140–164, https://doi.org/10.1175/1520-0426(2000)017<0140:COHBOP>2.0.CO;2, 2000.
Mather, G. K., Terblanche, D. E., Steffens, F. E., and Fletcher, L.: Results of the South African cloud-seeding experiments using hygroscopic flares, J. Appl. Meteorol., 36, 1433–1447, https://doi.org/10.1175/1520-0450(1997)036<1433:ROTSAC>2.0.CO;2, 1997.
Mazroui, A. A. and Farrah, S.: The UAE Seeks Leading Position in Global Rain Enhancement Research, J. Wea. Mod., 49, 54–55, https://doi.org/10.54782/jwm.v49i1.562, 2017.
Mecikalski, J. R., Watts, P. D., and Koenig, M.: Use of Meteosat Second Generation optimal cloud analysis fields for understanding physical attributes of growing cumulus clouds, Atmos. Res., 102, 175–190, https://doi.org/10.1016/j.atmosres.2011.06.023, 2011.
Morrison, H., Lawson, P., and Chandrakar, K. K.: Observed and Bin Model Simulated Evolution of Drop Size Distributions in High-Based Cumulus Congestus Over the United Arab Emirates, J. Geophys. Res.-Atmos., 127, e2021JD035711, https://doi.org/10.1029/2021JD035711, 2022.
Mossop, S. C.: The influence of drop size distribution on the production of secondary ice particles during graupel growth, Q. J. Roy. Meteor. Soc., 104, 323–330, https://doi.org/10.1002/qj.49710444007, 1978.
Murad, A. A., Al Nuaimi, H., and Al Hammadi, M.: Comprehensive assessment of water resources in the United Arab Emirates (UAE), Water Resour. Manag., 21, 1449–1463, https://doi.org/10.1007/s11269-006-9093-4, 2007.
Niranjan Kumar, K. and Ouarda, T. B. M. J.: Precipitation variability over UAE and global SST teleconnections, J. Geophys. Res.-Atmos., 119, 10–313, https://doi.org/10.1002/2014JD021724, 2014.
Ouarda, T. B., Charron, C., Kumar, K. N., Marpu, P. R., Ghedira, H., Molini, A., and Khayal, I.: Evolution of the rainfall regime in the United Arab Emirates, J. Hydrol., 514, 258–270, https://doi.org/10.1016/j.jhydrol.2014.04.032, 2014.
Pimentel, D., Berger, B., Filiberto, D., Newton, M., Wolfe, B., Karabinakis, E., Clark, S., Poon, E., Abbett, E., and Nandagopal, S.: Water resources: agricultural and environmental issues, BioScience, 54, 909–918, https://doi.org/10.1641/0006-3568(2004)054[0909:WRAAEI]2.0.CO;2, 2004.
Poulsen, C. A., Siddans, R., Thomas, G. E., Sayer, A. M., Grainger, R. G., Campmany, E., Dean, S. M., Arnold, C., and Watts, P. D.: Cloud retrievals from satellite data using optimal estimation: evaluation and application to ATSR, Atmos. Meas. Tech., 5, 1889–1910, https://doi.org/10.5194/amt-5-1889-2012, 2012.
Pósfai, M., Axisa, D., Tompa, É., Freney, E., Bruintjes, R., and Buseck, P. R.: Interactions of mineral dust with pollution and clouds: An individual-particle TEM study of atmospheric aerosol from Saudi Arabia, Atmos. Res., 122, 347–361, https://doi.org/10.1016/j.atmosres.2012.12.001, 2013.
Pruppacher, H. R., Klett, J. D., and Wang, P. K.: Microphysics of clouds and precipitation, Aerosol Sci. Tech., 28, 381–382, https://doi.org/10.1080/02786829808965531, 1998.
Rodgers, C. D.: Inverse methods for atmospheric sounding: theory and practice, World Scientific Publishing, Singapore-New Jersey-London-Hong Kong, ISBN 13 9789810227401, https://api.semanticscholar.org/CorpusID:60696486 (last access: 23 April 2025), 2000.
Rosenfeld, D.: TRMM observed first direct evidence of smoke from forest fires inhibiting rainfall, Geophys. Res. Lett., 26, 3105–3108, https://doi.org/10.1029/1999GL006066, 1999.
Rosenfeld, D.: Suppression of rain and snow by urban and industrial air pollution, Science, 287, 1793–1796, https://doi.org/10.1126/science.287.5459.1793, 2000.
Rosenfeld, D. and Lensky, I. M.: Satellite-based insights into precipitation formation processes in continental and maritime convective clouds, B. Am. Meteorol. Soc., 79, 2457–2476, https://doi.org/10.1175/1520-0477(1998)079<2457:SBIIPF>2.0.CO;2, 1998.
Rosenfeld, D., Rudich, Y., and Lahav, R.: Desert dust suppressing precipitation: A possible desertification feedback loop, P. Natl. Acad. Sci. USA, 98, 5975–5980, https://doi.org/10.1073/pnas.101122798, 2001.
Rosenfeld, D., Axisa, D., Woodley, W. L., and Lahav, R.: A quest for effective hygroscopic cloud seeding, J. Appl. Meteorol. Clim., 49, 1548–1562, https://doi.org/10.1175/2010JAMC2307.1, 2010.
Sherif, M., Almulla, M., Shetty, A., and Chowdhury, R. K.: Analysis of rainfall, PMP and drought in the United Arab Emirates, Int. J. Climatol., 34, 1318–1328, https://doi.org/10.1002/joc.3768, 2014.
Silverman, B. A.: An independent statistical reevaluation of the South African hygroscopic flare seeding experiment, J. Appl. Meteorol., 39, 1373–1378, https://doi.org/10.1175/1520-0450(2000)039<1373:AISROT>2.0.CO;2, 2000.
Silverman, B. A.: A critical assessment of glaciogenic seeding of convective clouds for rainfall enhancement, B. Am. Meteorol. Soc., 82, 903–924, https://doi.org/10.1175/1520-0477(2001)082<0903:ACAOGS>2.3.CO;2, 2001.
Silverman, B. A.: A critical assessment of hygroscopic seeding of convective clouds for rainfall enhancement, B. Am. Meteorol. Soc., 84, 1219–1230, https://doi.org/10.1175/BAMS-84-9-1219, 2003.
Terblanche, D. E., Steffens, F. E., Fletcher, L., Mittermaier, M. P., and Parsons, R. C.: Toward the operational application of hygroscopic flares for rainfall enhancement in South Africa, J. Appl. Meteorol., 39, 1811–1821, https://doi.org/10.1175/1520-0450(2001)039<1811:TTOAOH>2.0.CO;2, 2000.
Vujović, D. and Protić, M.: The behavior of the radar parameters of cumulonimbus clouds during cloud seeding with AgI, Atmos. Res., 189, 33–46, https://doi.org/10.1016/j.atmosres.2017.01.014, 2017.
Wang, J., Yue, Z., Rosenfeld, D., Zhang, L., Zhu, Y., Dai, J., Yu, X., and Li, J.: The Evolution of an AgI Cloud-Seeding Track in Central China as Seen by a Combination of Radar, Satellite, and Disdrometer Observations, J. Geophys. Res.-Atmos., 126, e2020JD033914, https://doi.org/10.1029/2020JD033914, 2021.
Wang, Z., Letu, H., Shang, H., Zhao, C., Li, J., and Ma, R.: A supercooled water cloud detection algorithm using Himawari-8 satellite measurements, J. Geophys. Res.-Atmos., 124, 2724–2738, https://doi.org/10.1029/2018JD029784, 2019.
Watts, P., Mutlow, C., Baran, A., and Zavody, A.: Study on cloud properties derived from Meteosat Second Generation observations, EUMETSAT ITT no. 97/181, https://www-cdn.eumetsat.int/files/2020-04/pdf_sci_97181_msg-cloud-props.pdf (last access: 23 April 2025), 1998.
Watts, P. D., Bennartz, R., and Fell, F.: Retrieval of two-layer cloud properties from multispectral observations using optimal estimation, J. Geophys. Res.-Atmos., 116, D16203 https://doi.org/10.1029/2011JD015883, 2011.
Wehbe, Y. and Temimi, M.: A remote sensing-based assessment of water resources in the Arabian Peninsula, Remote Sens., 13, 247, https://doi.org/10.3390/rs13020247, 2021.
Wehbe, Y., Ghebreyesus, D., Temimi, M., Milewski, A., and Al Mandous, A.: Assessment of the consistency among global precipitation products over the United Arab Emirates, J. Hydrol. Reg. Stud., 12, 122–135, https://doi.org/10.1016/j.ejrh.2017.05.002, 2017.
Wehbe, Y., Temimi, M., Weston, M., Chaouch, N., Branch, O., Schwitalla, T., Wulfmeyer, V., Zhan, X., Liu, J., and Al Mandous, A.: Analysis of an extreme weather event in a hyper-arid region using WRF-Hydro coupling, station, and satellite data, Nat. Hazards Earth Syst. Sci., 19, 1129–1149, https://doi.org/10.5194/nhess-19-1129-2019, 2019.
Wehbe, Y., Temimi, M., and Adler, R. F.: Enhancing precipitation estimates through the fusion of weather radar, satellite retrievals, and surface parameters, Remote Sens., 12, 1342, https://doi.org/10.3390/rs12081342, 2020.
Wehbe, Y., Tessendorf, S. A., Weeks, C., Bruintjes, R., Xue, L., Rasmussen, R., Lawson, P., Woods, S., and Temimi, M.: Analysis of aerosol–cloud interactions and their implications for precipitation formation using aircraft observations over the United Arab Emirates, Atmos. Chem. Phys., 21, 12543–12560, https://doi.org/10.5194/acp-21-12543-2021, 2021.
Wehbe, Y., Griffiths, S., Al Mazrouei, A., Al Yazeedi, O., and Al Mandous, A.: Rethinking water security in a warming climate: rainfall enhancement as an innovative augmentation technique, NPJ Clim. Atmos. Sci., 6, 171, https://doi.org/10.1038/s41612-023-00503-2, 2023.
Weston, M. J., Temimi, M., Nelli, N. R., Fonseca, R. M., Thota, M. S., and Valappil, V. K.: On the analysis of the low-level double temperature inversion over the United Arab Emirates: a case study during April 2019, IEEE Geosci. Remote S., 18, 346–350, https://doi.org/10.1109/LGRS.2020.2972597, 2020.
Woods, S., Lawson, R. P., Jensen, E., Bui, T. P., Thornberry, T., Rollins, A., Pfister, L., and Avery, M.: Microphysical properties of tropical tropopause layer cirrus, J. Geophys. Res.-Atmos., 123, 6053–6069, https://doi.org/10.1029/2017JD028068, 2018.
Woodley, W. L., Rosenfeld, D., and Silverman, B. A.: Results of on-top glaciogenic cloud seeding in Thailand. Part I: The demonstration experiment, J. Appl. Meteorol. Clim., 42, 920–938, https://doi.org/10.1175/1520-0450(2003)042<0920:ROOGCS>2.0.CO;2, 2003a.
Woodley, W. L., Rosenfeld, D., and Silverman, B. A.: Results of on-top glaciogenic cloud seeding in Thailand. Part II: Exploratory analyses, J. Appl. Meteorol., 42, 939–951, https://doi.org/10.1175/1520-0450(2003)042<0939:ROOGCS>2.0.CO;2, 2003b.
Zaremba, T. J., Rauber, R. M., Girolamo, L. D., Loveridge, J. R., and McFarquhar, G. M.: On the radar detection of cloud seeding effects in wintertime orographic cloud systems, J. Appl. Meteorol. Clim., 63, 27–45, https://doi.org/10.1175/JAMC-D-22-0154.1, 2024.
Zipser, E. J., Cecil, D. J., Liu, C., Nesbitt, S. W., and Yorty, D. P.: Where are the most intense thunderstorms on Earth?, B. Am. Meteorol. Soc., 87, 1057–1072, https://doi.org/10.1175/BAMS-87-8-1057, 2006.