the Creative Commons Attribution 4.0 License.
the Creative Commons Attribution 4.0 License.
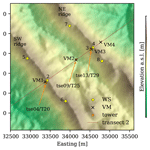
Exploring dual-lidar mean and turbulence measurements over Perdigão's complex terrain
Isadora L. Coimbra
Jakob Mann
José M. L. M. Palma
Vasco T. P. Batista
To assess the accuracy of lidars in measuring mean wind speed and turbulence at large distances above the ground as an alternative to tall and expensive meteorological towers, we evaluated three dual-lidar measurements in virtual-mast (VM) mode over the complex terrain of the Perdigão-2017 campaign. The VMs were obtained by overlapping two coordinated range height indicator scans, prioritising continuous vertical measurements at multiple heights at the expense of high temporal and spatial synchronisation. Forty-six days of results from three VMs (VM1 on the SW ridge, VM2 in the valley, and VM3 on the NE ridge) were compared against sonic readings (at 80 and 100 m a.g.l.) in terms of 10 min means and variances to assess accuracy and the influence of atmospheric stability, vertical velocity, and sampling rate on VM measurements. For mean flow quantities – wind speed (Vh) and u and v velocity components – the r2 values were close to 1 at all VMs, with the lowest equal to 0.948, whereas in the case of turbulence measurements ( and ), the lowest was 0.809. Concerning differences between ridge and valley measurements, the average RMSE for the wind variances was 0.295 m2 s−2 at the VMs on the ridges. In the valley, under a more complex and turbulent flow, smaller between-beam angle, and lower lidars' synchronisation, VM2 presented the highest variance RMSE, 0.600 m2 s−2 for . The impact of atmospheric stability on VM measurements also varied by location, especially for the turbulence variables. VM1 and VM3 exhibited better statistical metrics of the mean and turbulent wind under stable conditions, whereas at VM2, the better results with a stable atmosphere were restricted to the wind variances. We suspect that with a stable and less turbulent atmosphere, the scan synchronisation in the dual-lidar systems had a lower impact on the measurement accuracy. The impact of the zero vertical velocity assumption on dual-lidar retrievals at 80 and 100 m a.g.l. in Perdigão was minimal, confirming the validity of the VM results at these heights. Lastly, the VMs' low sampling rate contributed to 33 % of the overall RMSE for mean quantities and 78 % for variances at 100 m a.g.l., under the assumption of a linear influence of the sampling rate on the dual-lidar error. Overall, the VM results showed the ability of this measurement methodology to capture mean and turbulent wind characteristics under different flow conditions and over mountainous terrain. Upon appraisal of the VM accuracy based on sonic anemometer measurements at 80 and 100 m a.g.l., we obtained vertical profiles of the wind up to 430 m a.g.l. To ensure dual-lidar measurement reliability, we recommend a 90° angle between beams and a sampling rate of at least 0.05 Hz for mean and 0.2 Hz for turbulent flow variables.
- Article
(2538 KB) - Full-text XML
- BibTeX
- EndNote
To evaluate the wind at higher heights (>100 m), measurements from equipment other than anemometric towers are usually employed, as the costs associated with the installation and maintenance of masts scale with height. An alternative to the use of towers at great heights is the wind lidar.
Lidars measure the wind radial velocity up to kilometres of distance, and when employing a single lidar, a homogeneous flow assumption is needed to retrieve the wind vector components. However, under complex wind flow, this may not be a valid assumption, and measurements may present high systematic errors and inaccurate turbulence parameter estimations (Bingöl et al., 2009a, b; Sathe et al., 2011; Pauscher et al., 2016). For turbulence measurements, relevant to wind turbine load calculations, lidar retrievals are susceptible to cross-contamination and volume-averaging errors (Davies et al., 2005).
To reduce wind measurement uncertainty when using a single lidar in complex terrain, some authors have employed wind models to correct for flow distortion in profiling lidar measurements (Pitter et al., 2012; Klaas et al., 2015; Kim and Meissner, 2017). This approach, however, highly depends on the model's configurations and parameterisations (Klaas et al., 2015).
A more reliable solution to a single lidar is using two or more lidars configured to measure the same control volume simultaneously. In the case of three lidars, the three wind vector components can be retrieved from the radial velocities and azimuth and elevation angles (Mann et al., 2008; Sjöholm et al., 2009; Choukulkar et al., 2017). When two lidars are employed, one wind component, such as the vertical velocity, is assumed to be zero, and the other two are estimated. However, a multi-lidar approach implies high equipment costs and difficulties in coordinating and synchronising the lidar beams (Vasiljević et al., 2016). The scan strategy when employing multi-lidars can vary according to the study's objective. Triple-lidar setups were used by Wildmann et al. (2018) to investigate wind turbine wake and by Newman et al. (2016) to assess turbulence measurements. Coplanar range height indicator (RHI) scans were employed to evaluate rotor structures in a valley by Hill et al. (2010), while Calhoun et al. (2006) overlapped RHI scans to retrieve horizontal wind speed profiles in an urban site.
The association of at least two non-collocated lidars measuring multiple heights in a vertical line is called a virtual mast (VM) or virtual tower. Lidars can be configured with stop-and-stare (Damian et al., 2014; Pauscher et al., 2016; Newman et al., 2016; Debnath et al., 2017b; Wittkamp et al., 2021; Liu et al., 2024) or RHI scans (Calhoun et al., 2006; Ng and Hon, 2022; Newsom et al., 2005; Debnath et al., 2017a). Usually, the stop-and-stare scan has a higher spatial and temporal synchronisation but needs more time to measure at different heights as the equipment accelerates and decelerates from one measurement height to the next. Conversely, continuous vertical measurements of overlapping RHIs cover several heights more quickly, although usually with less accuracy, due to the scans not being entirely temporally and spatially synchronised, which is mainly a problem in an unstable atmosphere (Wittkamp et al., 2021; Choukulkar et al., 2017).
Rothermel et al. (1985) were the first to assess the feasibility of the dual-lidar methodology. Recent studies include experiments in complex terrain (Hill et al., 2010; Cherukuru et al., 2015; Santos et al., 2020; Duscha et al., 2023) and urban environments (Collier et al., 2005; Newsom et al., 2005; Calhoun et al., 2006; Wittkamp et al., 2021). The effect of atmospheric stability on virtual-mast measurements was evaluated by Newman et al. (2016) and Choukulkar et al. (2017) over flat terrains. Under stable atmospheric conditions, Newman et al. (2016) found that 10 min turbulent fluctuations from a triple-lidar VM setup aligned closely with Doppler beam swinging (DBS) (Strauch et al., 1984) estimations and diverged in an unstable atmosphere. However, the study did not include sonic measurements at the same height as the virtual mast, later addressed by Choukulkar et al. (2017), who evaluated triple-lidar VM mean measurements against mean sonic observations (at 50–300 m a.g.l., in 50 m increments). The VM results under stable conditions showed smaller errors than in an unstable atmosphere, which was attributed to the higher wind variability in unstable conditions, potentially leading to greater measurement uncertainty.
Despite previous efforts to evaluate multi-lidar measurements, no study has assessed the mean horizontal wind components obtained from two lidar-coordinated RHI scans in a VM mode, with reference sonic anemometer readings, or investigated second-order wind statistics from dual-lidar RHI retrievals or the influence of atmospheric stability and sampling rate on these data. Therefore, this study explores coordinated dual-lidar RHI measurements, in a VM mode, of the mean and turbulent flow under different wind conditions over Perdigão's complex terrain. The virtual-mast results are evaluated against sonic anemometer data at one or more matching heights in terms of coefficient of determination (r2) and statistical errors (RMSE and bias).
The VM measurements come from the Perdigão-2017 campaign (Fernando et al., 2019), a field experiment that was part of the New European Wind Atlas (NEWA) (Mann et al., 2017). During the campaign, profiler (8) and scanning (18) lidars were deployed (Fernando et al., 2019). The latter operated with different scanning schemes, including RHIs along the ridges, RHIs across the ridges (in three transects), and coordinated setups forming dual-lidar measurements. This work focuses on four virtual masts from the experiment, positioned in a transect almost perpendicular to Perdigão's double ridge and formed by seven WindScanners (WSs) – not previously analysed. Thus, we needed to assess the measurements' quality compared to reference data, develop a processing and filtering methodology, and explore the capabilities and limitations of these VMs in Perdigão.
The performance of WindScanners in dual and triple measurement setups, staring at a single point, was evaluated by Pauscher et al. (2016), who compared the results with a sonic anemometer (at 188 m a.g.l.) and DBS readings. The study focused on first- and second-order statistics of horizontal wind components measured by three dual-lidar configurations and one triple-lidar configuration. However, the analysis was limited to a single point, correlating the WS measurements without error quantification.
Previous virtual-mast-based studies in Perdigão combined scanning lidars at different positions than those examined here and with a different focus. Bell et al. (2020) evaluated RHI dual- and triple-lidar measurements in four locations along the Perdigão valley in a VM mode (from 50–600 m a.g.l.), focusing on the analysis of the valley flow. However, since the lidars were not coordinated, the VM analysis was based on 15 min mean values, and a time window of 60 s between lidar scans was imposed, which restricted the result analysis to only mean quantities. Triple-lidar VM measurements at different distances within Perdigão's wind turbine wake were investigated by Wildmann et al. (2019), who proposed a new approach to retrieve the turbulence dissipation rate from RHI lidar retrievals.
Beyond the difficulties in multi-lidar measurements, an additional one lies in measuring the complex wind flow above the mountainous terrain of Perdigão. With wind turbines increasingly being placed in complex terrains due to the depletion of flatland and more site constraints, a greater understanding and mapping of the wind in such areas are required. Furthermore, with the growth in height and rotor of modern wind turbines, it is crucial to assess the wind potential and characteristics at greater heights.
2.1 Field campaign
Located in Portugal's mainland, the Perdigão site is characterised by two parallel ridges (SW and NE) with an elevation of about 250 m above the nearby terrain, separated by 1.4 km, and extending over 4 km (Fig. 1). The SW ridge averages 231.2 m, with a slope of around 33.3°; the NE ridge is about 217.6 m, with an inclination of 28.5°; and the valley floor is 41.9 m. The terrain coverage is non-homogeneous, with a mixture of low vegetation and eucalyptus and pine tree patches (Palma et al., 2020).
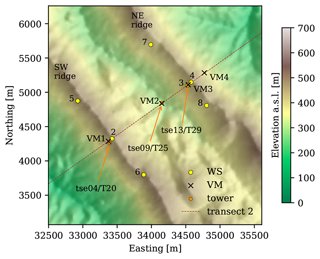
Figure 1Perdigão terrain (Farr et al., 2007) and measuring device locations (ETRS89/PT-TM06).
In the Perdigão-2017 campaign, multiple measuring devices worked simultaneously to obtain a high-resolution dataset from 1 May until 15 June 2017. This is called the intensive observational period (IOP) and is the study period of this work. Among the installed equipment, the sensors employed here are those installed in the three 100 m masts and seven WindScanners operated by the Technical University of Denmark (DTU; Fig. 1).
The wind flow in Perdigão was initially assumed to be two-dimensional, with the predominant wind direction perpendicular to its double ridge (Fernando et al., 2019). However, the measurements revealed Perdigão's intricate wind flow. Despite the uniform perpendicular flow on the synoptic scale, on smaller scales, the wind exhibits two main directions (Fig. 2). In the valley, the wind direction aligns with the valley (tse09/T25 wind rose), while on the ridges (tse04/T20 and tse13/T29 wind roses), it is perpendicular to the valley.
2.2 Towers
The three 100 m towers were located along transect 2 (Menke et al., 2019b), almost perpendicular to the ridges: tse04/T20 on the SW ridge, tse09/T25 in the valley, and tse13/T29 on the NE ridge (Fig. 1 and Table 1). The tower equipment provided wind speed and temperature measurements that were used in this study to evaluate the VM wind speed retrievals and classify the atmospheric stability.
Gill 3D WindMaster Pro sonic anemometers were operated at a frequency of 20 Hz, with sensor heights shown in Table 3 and Fig. 3. NCAR SHT75 temperature and humidity sensors were installed at seven levels: 2, 10, 20, 40, 60, 80, and 100 m a.g.l. The post-processed (quality-controlled, tilt-corrected, and in a geographic coordinate system) data from these instruments were downloaded from UCAR/NCAR – Earth Observing Laboratory (2019a). The sonic anemometer data were tilt-corrected using laser survey measurements (Menke and Mann, 2017) to determine the azimuth, pitch, roll, and height of each anemometer, ensuring that the post-processed wind components were represented in geographical coordinates (UCAR/NCAR – Earth Observing Laboratory, 2019b).
2.3 WindScanners
Eight WindScanners (WS1–8), four on each ridge and operated by DTU (Vasiljević et al., 2016; Menke et al., 2019a), were employed in the Perdigão-2017 campaign. In terms of settings, the range gate separation (15 m), full width at half maximum of the spatial weighting function (30 m), spatial coverage (from 100 to 3000 m away from the equipment), elevation step (0.75°), accumulation time (500 ms), and pulse length (200 ns) were identical for all WindScanners. WS1–4, WS6, and WS8 had an elevation range of 36°, while WS5 and WS7 covered an angular range of 18°. WindScanners 1–4 performed RHI measurements along transect 2, and WindScanners 5–8 operated in a sequence of three scan types, each with a 10 min duration: along the ridge, virtual-mast, and transect scans. By crossing WS2–4 RHI measurements with WS5–8 virtual-mast scan (also RHI), four virtual masts (VM1–4) were reconstructed with the campaign measurements (Fig. 1 and Table 2).
To guarantee the quality of the WS measurements, before the dual-lidar processing, the WS data were initially filtered out according to the equipment's radial velocity limits ( m s−1) and the carrier-to-noise ratio (CNR), where a threshold equal to −22 dB (determined from CNR versus radial velocity plots of the multiple WindScanners) was imposed. The WS spectrum data were not stored in the Perdigão campaign; only the processed signal results were. Other filters were employed while processing the VM measurements (Sect. 3.1).
During the Perdigão-2017 experiment, four virtual masts (VM1–4) were configured (Menke et al., 2019a) according to the intersection point between two non-collocated WindScanners (WSa and WSb; Table 2). Two virtual masts (VM1 and VM3) were located on the top of the SW and NE ridges, another VM was located in the valley (VM2), and the last one was located downhill from the NE ridge (VM4; Fig. 1). VM1–3 were located at distances of 32.4, 9.4, and 3.3 m, respectively, from tse04/T20, tse09/T25, and tse13/T29 100 m towers to compare VM results with reference equipment at overlapping heights and to map the vertical profile of the wind from 10 to around 430 m a.g.l.
3.1 Dual-lidar processing and filtering
The processing and filtering of the dual-lidar measurements in Perdigão required the following steps.
-
Step 1. The radial velocities of WSa () and WSb () were interpolated along the beam direction at the VM coordinates (Table 2).
-
Step 2. The VM heights (Table 3 and Fig. 3) were calculated as the average of the closest WSa and WSb measurement heights.
-
Step 3. Likewise, the VM measurement timestamps were determined by averaging the WSa and WSb nearest timestamps.
-
Step 4. The Cartesian velocity components in the x (u) and y directions (v) were obtained from the radial velocities (vr) and the azimuth (θ) and elevation (ϕ) angles of WSa and WSb, assuming that the vertical wind component is zero (w=0), by
Subsequently, the horizontal wind speed (Vh) was calculated.
-
Averages and variances of wind velocity components and wind speed were calculated within 10 min intervals.
-
-
Step 5. The VM measurements were filtered in two steps.
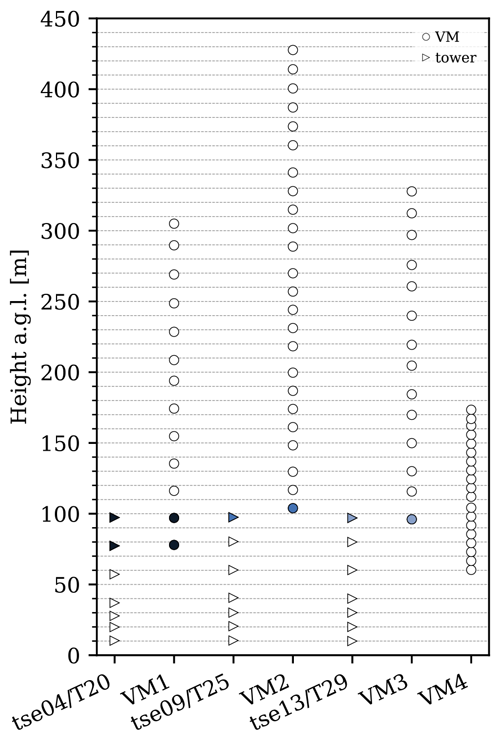
Figure 3Tower and VM heights of wind speed measurements (matching heights in coloured markers: dark blue for tse04/T20 and VM1, medium blue for tse09/T25 and VM2, and light blue for tse13/T29).
After these processing steps, we ended up with dual-lidar measurements that spanned the atmosphere from 80 to 305 m a.g.l. in VM1, 100 to 430 m in VM2, 100 to 330 m in VM3, and 60 to 170 m in VM4 – i.e. more than 4 times the height of conventional tall meteorological towers (100 m a.g.l.). We focused our analysis on the measurements from VM1 at 80 and 100 m, VM2 at 100 m, and VM3 at 100 m, as these were the only measurements obtained at the same height as the sonic anemometer readings, enabling the evaluation of the VM data's reliability.
Upon validating their accuracy, we can use the entire VM dataset in further studies. However, at higher heights, the assumption of zero vertical velocity (Step 4) can reduce the accuracy of the horizontal wind components obtained from dual-lidar measurements, since the increase in beam elevation angles causes the lidar beams to be more aligned with the vertical component of the wind.
3.1.1 Hard target filter
Some WS measurements had interference from hard targets, such as terrain, vegetation, and masts, and were, therefore, filtered out. As a result, VM2 and VM3 presented only one measuring height that overlapped with the sonic heights, at around 100 m a.g.l., while VM1 had two measuring heights that matched the tse04/T20 sonics, at ∼ 80 m and ∼ 100 m a.g.l.
3.1.2 Minimum quantity of measurement filter
Although the WSs were configured to perform approximately 22 VM scans in each 10 min measurement period, device restrictions and filtering led to periods with fewer valid scans, as shown in Fig. 4 for VMs' measurements at 100 m a.g.l. To evaluate the impact of the number of valid scans per 10 min period on VM measurement accuracy, we computed error indicators for VM1–3 datasets under various filtering thresholds (Table 4). Starting with unfiltered data (0 % filter), we defined the minimum number of scans (threshold) required for a 10 min measurement to be considered valid, progressively increasing the filter criteria (as represented by the percentage values in Table 4) up to a 90 % filter. For example, with the 20 % filter, a 10 min measurement was considered valid and included in the analysis if it contained at least 20 % of the total scan quantity, i.e. four valid scans for a maximum of 22.
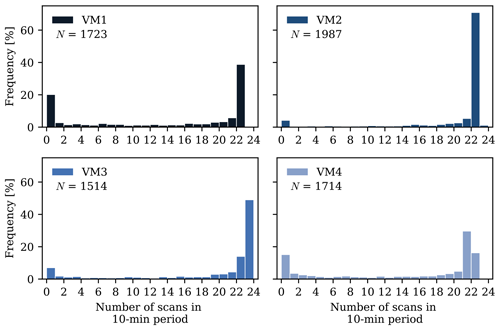
Figure 4Histogram of the number of valid scans in 10 min periods for all virtual masts at ∼ 100 m a.g.l., before the MQM filter. N represents the total number of valid 10 min measurements at ∼ 100 m a.g.l. during the IOP, before the MQM filter.
Table 4Errors between VMs and towers according to the minimum quantity of measurements (MQM) in 10 min periods for u, v, , and .
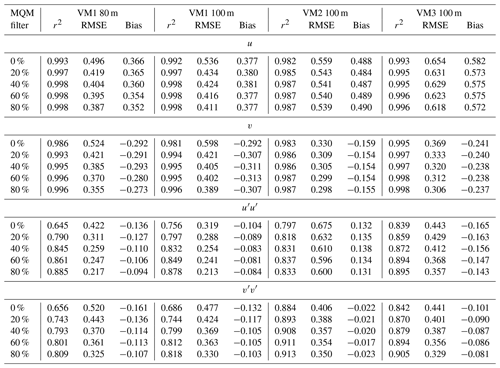
The RMSE and bias units are m s−1 for u and v variables, while for and the units are m2 s−2. r2 is unitless.
The turbulence measurements ( and ) were more sensitive to the MQM filter than the mean values (u and v), as evidenced mainly by the RMSE (Table 4). Consequently, this metric was chosen as the criterion for identifying the optimal MQM filter value, which retrieves a VM dataset with low errors while avoiding a significant data loss, caused by a too-constrained filter.
The balance between low RMSE and low data loss occurs when . Here, ΔRMSE is the percentage difference in RMSE between any MQM filter above 0 % and the raw data (0 % filter), and ΔN is the percentage difference in the number of samples between the two datasets. By averaging across all VMs, we determined that the optimal MQM filter is 50 % for the mean and 80 % for the turbulence VM measurements. Applying a filter higher than 50 % (80 %) can reduce the dataset size to a point where the remaining data become less representative of the mean (turbulent) wind flow. Therefore, subsequent mean and turbulence results will be presented using 50 % and 80 % MQM filters.
Since VM4 is the only virtual mast with no reference measurement nearby, the filtering procedure determined through the VM1–3 analysis was replicated at VM4.
3.2 Dual-lidar measurement constraints and error sources
As two simultaneous WSs are required to produce a VM measurement, the VM is constrained by the availability of both WindScanners. WS2–4 (WSa in Table 2) continuously performed RHI scans, while WS5–8 (WSb in Table 2) only did the intercepting RHI scan twice per hour. Thus, the VM measurements occurred twice per hour within 10 min. During the 10 min period, each WS performed a maximum of 22 or 23 scans (Fig. 4); i.e. a maximum sampling rate of 0.038 Hz ( Hz), approximately 500 times lower than the sonic anemometer frequency (equal to 20 Hz).
Another constraint was the dependence of VM data availability on concurrent measurements from both WindScanners, which, at specific periods, depicted limited data due to equipment downtime or filtering (low CNR, hard targets, and MQM filter). The data availability for each VM at 100 m a.g.l. during the IOP is detailed in Table 5. For mean wind components, the average data availability for all heights was 46.2 % for VM1, 76.3 % for VM2, 54.1 % for VM3, and 56.9 % for VM4. For and , on the other hand, availability was 37.5 %, 69.8 %, 47.8 %, and 49.2 % for VM1–4.
The interception angle (Δχ) between lidars' beams (Table 6), with directions and , influences the accuracy of VM results. This is because the dual-lidar error of a retrieved wind field component (σDD(uj)) is (Stawiarski et al., 2013)
where is the error prefactor, αj is the angle between the direction of the wind field component () and the mean lidar direction (), and is the radial velocity error, assuming that is identical in both lidars (). While the radial velocity error depends on several factors, such as the specific lidar, atmospheric backscatter, distance from the instrument, focus position, and instrument temperature, we assume it to be identical in both lidars because the angle between the beams is a more significant contributor to the dual-lidar error.
Table 6Average angle between lidars' beams (Δχ) and prefactors of the dual-lidar propagation error for the horizontal velocity components (u and v).
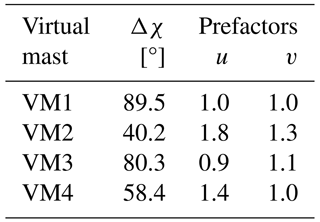
The prefactor is directly influenced by the between-beam angle and the direction of the wind component, namely u and v, and indirectly by the VM height (Fig. 5), as Δχ varies with the beams' elevation angles. Ideally, the angle between the beams would be close to 90°, which results in prefactors equal to 1, regardless of the wind component direction. At Perdigão's four virtual masts, only VM1 and VM3 had Δχ close to the optimal angle (∼ 89.5 and ∼ 80.3°), while the angles at VM2 and VM4 were 40.2 and 58.4°, on average (Table 6). This means that the prefactors and the propagation of the radial velocity error at VM2 and VM4 are greater than at VM1 and VM3.
When retrieving the u velocity, the dual-lidar propagation error is about 1.0, 1.8, 0.9, and 1.4 times the error of the radial velocity for VM1–4, respectively (Table 6). For the v velocity, the prefactors are around 1.0, 1.3, 1.1, and 1.0 for VM1–4. On the other hand, the dual-lidar error of the horizontal wind speed is a combination of the σDD(u), σDD(v), and wind speed components:
assuming that the errors in u and v are not correlated.
With regard to height variation (Fig. 5), the prefactors varied little and generally showed higher values with increasing height, except for the v wind component measured by VM1.
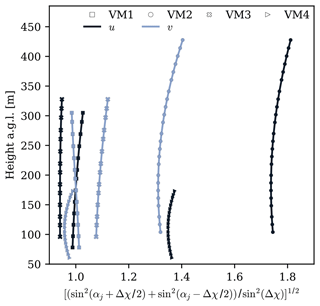
Figure 5Dual-lidar error prefactor of a retrieved wind field component as a function of the beam height for VM1–4.
Another source of error when combining radial velocities from different lidars can arise when there is a mismatch in their range gate heights (Stawiarski et al., 2013). Such mismatch can cause the lidars to measure different wind structures, mainly under high-vertical-wind-shear conditions. For the Perdigão-2017 campaign, the height difference between the range gate centers, after the radial interpolation, varied for each height and virtual mast. At VM1–4, the displacements went up to 4.4, 6.8, 8.7, and 1.6 m, respectively. However, given that the spatial resolution of the WindScanners was approximately 30 m, this mismatch is not expected to impact the virtual-mast results substantially.
In addition, the lidars' scans were not fully synchronised in time (Fig. 6). This means that measurements from WSa and WSb occurred at slightly different times, which can lead to time-average errors in the dual-lidar measurements (Stawiarski et al., 2013) due to the stationary atmospheric assumption (Choukulkar et al., 2017). At VM1, the predominant time differences between WSa and WSb ranged from 0 to 2 s, accounting for 53.7 % of all VM1 measurements. At VM2, WSb consistently recorded measurements later than WSa, leading to time lags of 8–10 s in 69.8 % of VM2's measurements. For VM3, 51.1 % of the measurements depicted a time difference between 3 and 5 s. Meanwhile, at VM4, the time difference for 62.8 % of the measurements fell in the [1 s, 3 s) interval. While these desynchronisations may impact the retrieval of turbulent variables, their influence is expected to be insignificant for mean quantities.
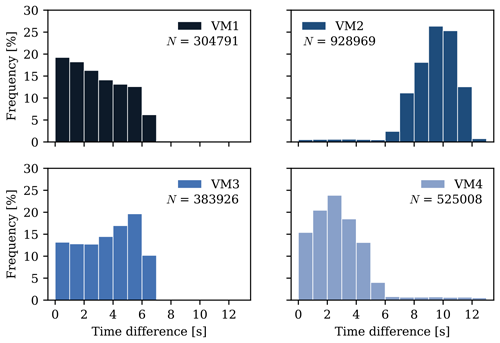
Figure 6Time difference histogram of the mean flow measurements at all heights between the lidars constituting the virtual masts. N represents the total number of valid 10 min measurements at all heights during the IOP.
Lastly, the horizontal position of each VM differed from the corresponding tower locations. This can affect the VM results when nearby tower measurements are used as a reference due to the underlying assumption of a spatially homogeneous atmosphere. This is most pronounced for VM1, located 32.4 m apart from tse04/T20. Meanwhile, VM2 was 9.4 m from tse09/T25, and VM3 was 3.3 m from tse13/T29.
This section compares virtual-mast and sonic measurements and how atmospheric stability, vertical velocity, and sampling rate influence the VM wind velocity and turbulence retrievals. The analyses are based on 10 min averages of the horizontal wind speed (Vh) and its components (u and v), as well as their variances ( and ). The virtual-mast and sonic comparisons also cover radial velocity means (vr) and variances (). All results are in local time, equal to UTC + 1 h in the summer period, and in the ETRS89/PT-TM06 coordinate system.
4.1 Virtual-mast and sonic comparisons
Virtual-mast and tower measurements were compared at their closest heights, with no vertical interpolation: VM1 at 77.9 and 97.0 m with tse04/T20 at 77.3 and 97.3 m, VM2 at 103.9 m with tse09/T25 at 97.5 m, and VM3 at 96.0 m with tse13/T29 at 97.0 m. For simplification, the comparison heights were rounded to 80 and 100 m.
As a first analysis, and from the WindScanners of VM1–3 were compared against sonic measurements projected in the laser beam direction to assess the measurements of each WS equipment without introducing uncertainties related to the dual-lidar methodology (Sect. 3).
Care must be taken when comparing VM results in the valley (VM2) with those on the ridges (VM1 and VM3), since the flows are intrinsically different at the comparison heights (80 and 100 m a.g.l.). In the valley, the main wind direction is along the valley, whereas on the ridges the main wind direction is cross-valley; the wind speeds are lower (Fig. 2); and the turbulence intensity is 2.7 times higher than on the ridges.
4.1.1 Mean flow measurements
In the comparison between VM and sonic vr (Table 7), the fit of the linear regressions for all WindScanners was almost perfect, with r2 values close to 1. The lowest r2 was equal to 0.989 (WS6 at VM2 100 m). In the linear regression equation (), despite the coefficients (m) being approximately 1, the constants (b), determined by where the line intercepts the y axis, assumed positive (WS5, WS2, and WS7) and negative (WS3 and WS6) values according to the WS, meaning an overall overestimation and underestimation of vr. In addition, b values higher than 0.4 m s−1 were observed in WS5 (0.414 m s−1 at 80 m and 0.445 m s−1 at 100 m a.g.l.) and WS7 (0.492 m s−1 at 100 m a.g.l.). These WindScanners also showed higher RMSE and bias errors in their radial velocities at 100 m: 0.509 m s−1 and 0.436 m s−1 in WS5 and 0.586 m s−1 and 0.523 m s−1 in WS7.
Table 7Statistical parameters from VM and tower comparisons for mean and variance variables.
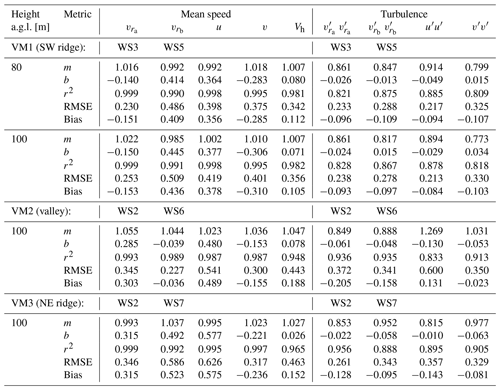
The units of b, RMSE, and bias are m s−1 for mean variables and m2 s−2 for variances. m and r2 are unitless. m is the coefficient, and b is the constant of the linear regression equation ().
When WS5 and WS7 form VM1 and VM3, their beams align with the direction of the ridges (Fig. 1), and at the top of the hills, the main wind directions are perpendicular to the ridge's orientation (Fig. 2). Thus, due to a lidar's inherent limitation to directly measure the wind component perpendicular to its beam orientation, WS5 and WS7 setups contribute to their wind speed measurement errors.
For the horizontal wind speed (Vh) and u and v wind components obtained from the dual lidars, besides the beam orientation of each WS regarding the position of the wind, the intersection angle between the two beams is also important (Table 6). At VM1 and VM3, Δχ was close to 90°, the optimal angle to retrieve u and v, whereas at VM2, the angle was about 40°, yielding higher dual-lidar propagation error in the u and v components, with mean prefactors equal to 1.8 and 1.3 (Table 6 and Fig. 5).
The coefficients of determination were close to 1 for the mean wind variables at all virtual masts (Table 7 and Fig. 7), with the lowest values equal to 0.987 for u and v and 0.948 for Vh at VM2. The lower r2 values at VM2 are attributed to the smaller angle between WS2 and WS6 beams and to the turbulent flow in the valley, which may require a greater VM sampling rate than 0.038 Hz. The highest errors, however, occurred at VM3 for u (0.626 m s−1 RMSE and 0.575 m s−1 bias) and at VM1 for v (0.401 m s−1 RMSE and −0.310 m s−1 bias), while for the horizontal wind speed, VM3 obtained the highest RMSE, equal to 0.463 m s−1, and VM2 the highest bias, 0.188 m s−1. Additionally, all VM results overestimated the anemometer readings of the mean east–west wind component and Vh (positive bias), and they underestimated the north–south wind component (negative bias).
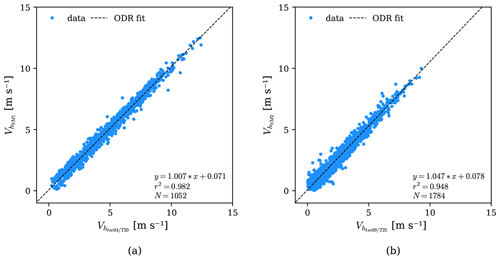
Figure 7Mean flow measurements of virtual masts against sonic anemometer data: (a) VM1 and tse04/T20 Vh at 100 m a.g.l. and (b) VM2 and tse09/T25 Vh at 100 m a.g.l.
The average magnitude of the VM error (RMSE) did not follow the trend observed in the dual-lidar propagation errors. Contrary to the prefactor values (Table 6), VM2's u variable did not show the highest RMSE value among the VMs, and the x wind component in VM3 did not exhibit the lowest, indicating that factors beyond the error coefficient influenced the VMs' RMSE.
The Vh errors of the VMs generally fell within the range of those for the u and v components. The r2, on the other hand, showed lower values (0.969 on average) than for u and v (0.994 on average).
Compared to Pauscher et al. (2016), the horizontal wind speed results of Perdigão's VMs showed lower r2 values against reference sonic anemometer measurements, ∼ 3 % lower on average. The difference between both results is due to the scanning mode and the underlying assumptions in each scan. Pauscher et al. (2016) employed a staring configuration, recording data at 0.5 Hz, whereas in our analysis, the virtual-mast measurements were formed by combining two RHI scans with a maximum sampling rate of 0.038 Hz. In the latter, the lidar beams were constantly moving and not perfectly synchronised in time and space, resulting in a lower measurement frequency and forcing a greater flow homogeneity assumption compared to the staring approach.
4.1.2 Turbulence measurements
For the radial velocity variances ( and ), the r2 values were consistently lower than for the mean radial velocities ( and ), going from 0.994 in the means to 0.888 in the variances, on average (Table 7). The lowest coefficient of determination for between lidar and sonic measurements was 0.821 at WS3 in VM1 80 m, whereas the highest was 0.956 at WS2 in VM3 100 m.
The radial velocity variance errors averaged 0.294 m2 s−2 for RMSE and −0.123 m2 s−2 for bias on the ridges. In the valley, under a more turbulent flow and with a low measurement rate, the average errors for were higher than those on the ridges, with an RMSE of 0.357 m2 s−2 and bias of −0.182 m2 s−2. However, independent of the measurement location, all WindScanners underestimated the turbulence measurements (negative bias).
For and , the VMs' low sampling rate led to a weaker linear correlation against sonic measurements than for u and v. The r2 results, which were higher than 0.987 (VM2 u and v) for the mean wind speed components, assumed values as low as 0.809 (VM1 at 80 m a.g.l.) in the variances (Table 7 and Fig. 8). This means the VM turbulence measurements did not portray the wind variability, represented by r2, as the sonic anemometer readings and the VM averages.
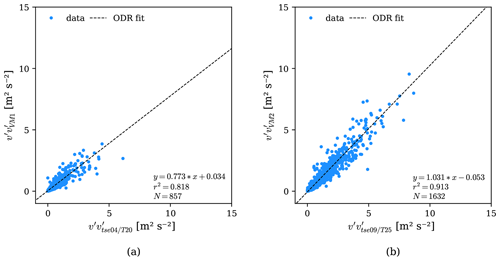
Figure 8Turbulence measurements of virtual masts against sonic anemometer data: (a) VM1 and tse04/T20 at 80 m a.g.l. and (b) VM2 and tse09/T25 at 100 m a.g.l.
In the linear regression equation between VM and sonic turbulence measurements, b was close to zero in all VMs, with the highest value of −0.130 m2 s−2 for at VM2, while the slope coefficient (m) ranged from 0.799 at 80 m VM1 () to 1.269 at 100 m VM2 (). The steeper slope for VM2's turbulence measurements (both above 1) indicated greater sensitivity to changes in turbulence compared to the other VMs, where m was less than 1. However, this did not translate into better accuracy, as VM2 had the highest RMSE for turbulence measurements.
Regarding errors, on the ridges, the average RMSE for the turbulent wind components (0.295 m2 s−2) was lower than in the valley (0.475 m2 s−2), as also observed in the radial velocity results. The RMSE at VM2 for turbulence measurements was the highest (0.600 m2 s−2 for ), while the highest bias was at VM3 (−0.143 m2 s−2 for ), closely followed by VM2 (0.131 m2 s−2 for ), in absolute values. The high errors in VM2 turbulence measurements are attributed to the approximately 9 s mismatch between the lidars. Other contributing factors are the small interception angle between the lidars' beams and the measurement sampling rate, which may be insufficient for the valley complex flow, as also observed in the VM2 mean flow results. Consistently with the distinct valley flow, measured by VM2 uniquely overestimated the sonic measurements (positive bias), despite the negative bias in the radial velocity variances of WS2 and WS6.
Overall, the VM turbulence measurements showed a high mean r2 value (0.867) and low mean errors (0.340 m2 s−2 RMSE and −0.063 m2 s−2 bias), despite the average r2 being lower than that of the mean wind components (0.994), the imperfect synchronisation of the scans, and the low sampling rate. The relatively high accuracy of the VM results in capturing the turbulent flow, even with measurement constraints, indicates that in Perdigão, synoptic and mesoscale systems dominate the atmospheric circulation at the site, and small-scale phenomena played a minor role in the wind patterns.
In Pauscher et al. (2016), the r2 values of () were equal to 0.954 (0.966), 0.887 (0.903), and 0.782 (0.861) for the three different dual-lidar combinations. On average, their r2 values were ∼ 1 % (∼ 6 %) higher than the ones depicted here. This difference is again related to the nature of the scans (staring versus RHI combination), which affects the temporal–spatial synchronisation and the measurement frequency.
4.2 Influences on the dual-lidar results
Besides the inherent differences between point-based sonic readings and volumetric-based VM measurements, additional factors can cause the VM results to diverge further from the reference readings. Our analysis focused on three potential factors: atmospheric stability, vertical velocity, and sampling rate.
4.2.1 Atmospheric stability
To assess the atmospheric stability influence on mean and turbulence measurements in a multi-lidar setup, we categorised VM1–3 measurements according to the atmospheric stability of the nearby 100 m towers, estimated by the bulk Richardson number (RiB), similar to Menke et al. (2019b), being assigned as stable (RiB>0) or unstable (RiB≤0). While previous studies focused on the stability influence on VMs in flat terrains (Newman et al., 2016; Choukulkar et al., 2017), the virtual masts in Perdigão were located in mountainous terrain, where the complex wind flow can disrupt a direct correlation between stability and dual-lidar measurements.
The bulk Richardson number (RiB) was calculated with the 10 min average horizontal mean wind speed components measured at 100 m a.g.l. (u100 and v100) and assuming relatively dry air conditions, i.e. using the 10 min average potential temperature at 2 m (Θ2) and 100 m (Θ100) height rather than the virtual potential temperature (Θv) (Stull, 1988):
The gravitational acceleration is g=9.81 m s−2, m, and the wind speed at 2 m a.g.l. was assumed equal to zero. The 10 min average potential temperature was approximated by , where K m−1 and T is the 10 min average air temperature (Stull, 1988) measured by the temperature sensors.
We assumed relatively dry air conditions (Θv≈Θ) due to the lack of pressure measurements on Perdigão's 100 m towers and the limited availability of barometric data from nearby towers, which reduced the number of periods for which we could calculate RiB and classify atmospheric stability. This assumption proved valid because the differences between the 10 min average Θv and the 10 min average Θ at the three 100 m towers did not exceed 3.8 K at 100 m a.g.l. during the entire IOP.
The distribution of the RiB values at the three 100 m towers (Fig. 9) further highlights the different conditions between ridge and valley wind flow. For tse04/T20 and tse13/T29, the histograms peak around zero RiB with nearly symmetrical distributions, showing similar quantities of unstable and stable conditions. The valley tower, on the other hand, has a broader distribution with a significant spread towards positive RiB values, indicating greater variability in stability compared to the ridge towers and a prevalence of stable atmospheric conditions.
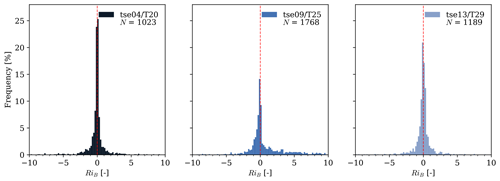
Figure 9Histogram of the bulk Richardson number from 10 min average measurements at tse04/T20 (SW ridge), tse09/T25 (valley), and tse13/T29 (NE ridge) at 100 m a.g.l. during the VM measurement periods of the mean flow. The RiB values are constrained to the −10 to 10 interval, with a bin width of 0.2.
From the data collected by the 100 m towers, the following number of 10 min periods were classified as unstable (stable) at VM1–3: 526 (497), 780 (988), and 617 (572) for the mean wind components at 100 m a.g.l. For the variances, the respective quantities were 447 (383) at VM1, 719 (898) at VM2, and 552 (514) at VM3.
The influence of atmospheric stability on the dual-lidar results was affected by the distinct wind flows between the ridges and the valley in Perdigão (Table 8), as well as by the different spatial (WSs' interception angle) and temporal (WSs' desynchronisation) configurations among the VMs. On the ridges, VM1 and VM3 showed slightly better r2 values and slightly lower errors under stable compared to unstable atmospheric conditions, especially for turbulent flow variables. The average r2, RMSE, and bias for the mean wind components (u and v) were 0.997, 0.414 m s−1, and 0.082 m s−1 in stable conditions, while under unstable conditions, these were equal to 0.996, 0.434 m s−1, and 0.075 m s−1. For turbulence variables ( and ), the statistical metrics assumed mean values of 0.853, 0.235 m2 s−2, and −0.055 m2 s−2 for stable, and 0.836, 0.339 m2 s−2, and −0.140 m2 s−2 for unstable conditions.
Table 8Statistical parameters from VM and tower comparisons according to the atmospheric stability.
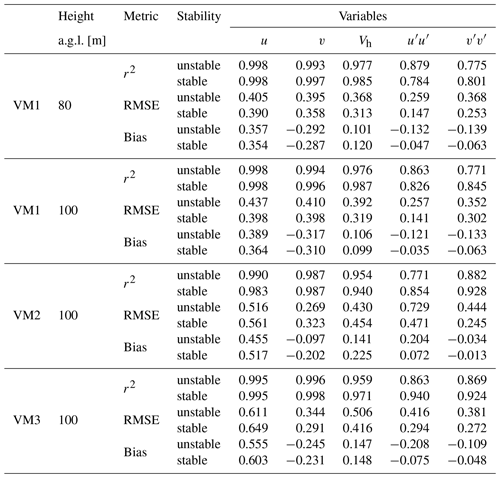
The RMSE and bias units are m s−1 for u, v, and Vh variables, while for and the units are m2 s−2. r2 is unitless.
Conversely, at the valley VM, higher r2 values and lower errors with a stable atmosphere were restricted to and . The variances r2, RMSE, and bias under stable conditions were 0.891, 0.358 m2 s−2, and 0.029 m2 s−2, on average. In comparison, the average and metrics during unstable conditions were equal to 0.827, 0.587 m2 s−2, and 0.085 m2 s−2. Another distinct result at VM2 was that regardless of the atmospheric conditions; the turbulence measurement overestimated the tse09/T25 sonic anemometer readings at 100 m a.g.l.
The overall better results from the VMs under stable compared to unstable atmospheric conditions indicate that when the air is more stable and less turbulent, the temporal and spatial synchronisation between the scans of a multi-lidar system becomes less critical, without compromising the accuracy of the measurements. Additionally, while the statistical metrics for the 10 min mean values changed slightly according to stability, the metrics for the 10 min variances were more affected by atmospheric conditions. In terms of wind direction, there was no clear relationship between the VM wind direction error (i.e. the difference between the VM's 10 min average horizontal wind direction and the tower's 10 min average horizontal wind direction) and atmospheric stability (not shown here).
4.2.2 Vertical velocity
Another possible influence on VM retrievals was the assumption of a zero vertical wind velocity (w) made to obtain the horizontal wind components from the WindScanners' radial velocities (Step 4 in Sect. 3.1). The coefficient of determination of the linear regression between the 10 min average w values measured by sonic anemometers and the 10 min horizontal wind speed errors of the VMs (i.e. the difference between the VM's 10 min average horizontal wind speed and the anemometer's 10 min average horizontal wind speed) around 100 m a.g.l. in Perdigão was lower than 0.060 at all measurement locations. For turbulence measurements, the highest r2 between the 10 min average w values and the 10 min VM measurement errors was 0.110 at VM1.
These low r2 values mean that the assumption of zero vertical wind velocity had a minimal impact on the VM measurements at 80 and 100 m a.g.l. in Perdigão, confirming the validity of the VM results at these heights. This minimal impact is attributed to the small elevation angles of the lidars' beams (Table 2) and the low vertical velocity at the site, which did not exceed 3.6 m s−1 at 100 m a.g.l. during the IOP.
At heights above 100 m, however, the elevation angles of the beams will be higher, causing the lidar beams to be more aligned with the vertical component of the wind. Thus, in a strong convective atmosphere at higher heights, the vertical velocity can influence the virtual-mast results more significantly. In Perdigão, the maximum elevation angles of the VMs were 21.6° at VM1, 15.6° at VM2, and 23.0° at VM3.
4.2.3 Sampling rate
We turned to the sonic data to assess how the VM sampling rate affected the results. Results at progressively lower sampling rates were compared against the 20 Hz measurements in terms of r2, RMSE (Fig. 10), and bias. The data were downsampled by selecting every nth sample for frequencies between 1 and 20 Hz (e.g. for 2 Hz, every 10th sample) and by selecting the nth time step for frequencies below 1 Hz (e.g. for 0.5 Hz, every second time step). Following downsampling, variances and averages were calculated over 10 min intervals. Then, to assess the influence of the sampling rate in the VM retrievals, the statistical metrics of the sonic data were linearly interpolated at the VMs' acquisition rates, between 0.018–0.038 Hz for the means and 0.030–0.038 Hz for the variances (shaded area in Fig. 10).
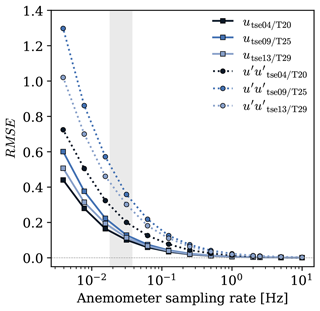
Figure 10RMSE of sonic measurements by the sampling rate, for the mean (u) and turbulent () x-axis wind speed component, on the three 100 m towers at 100 m a.g.l. The RMSE units are m s−1 for u and m2 s−2 for .
Similar to the previous results, the mean wind flow (u, v, and Vh) and the metrics r2 and bias showed less sensitivity to measurement frequency than the variances ( and ) and RMSE at the three 100 m towers. Additionally, the sampling rate had a similar influence on the wind components of the same moment, evidenced by the comparable results for u and v and for and at Table 9. Consequently, Fig. 10 displays only the RMSE for mean and turbulent x-axis wind speed component at 100 m a.g.l.
Table 9Averaged statistical metrics due to sampling rates in the virtual-mast measurement range for the mean (0.018–0.038 Hz) and turbulent (0.030–0.038 Hz) flow, based on sonic readings at 100 m a.g.l.
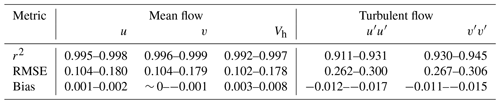
The units of RMSE and bias are m s−1 for mean variables, while for variances they are m2 s−2. r2 is unitless.
At 100 m a.g.l., the estimated average RMSE of the VMs, due solely to their sampling rate, ranged between 0.102 and 0.180 m s−1 for the mean flow quantities and 0.262 and 0.306 m2 s−2 for the turbulence variables (Table 9). Considering the overall RMSE values for all virtual masts at 100 m a.g.l. (0.434 m s−1 for the average of u and v and 0.363 m2 s−2 for the average of and ), around 33 % of the VMs' RMSE for the mean wind components and 78 % for the variances can be attributed to their measurement frequency, assuming a linear influence of this factor. For the mean horizontal wind velocity, 33 % of the VMs' average RMSE at 100 m a.g.l. can be attributed to their measurement frequency. Additionally, to accurately measure the wind flow in the valley, a higher sampling rate is required than above the hills, especially to retrieve the wind variances. Within the VM sampling rate range, the average RMSE error for turbulence measurements at 100 m a.g.l. is about 61 % and 19 % higher in the valley than on the SW and NE ridge.
Therefore, when aiming for dual-lidar readings with errors due to the sampling rate lower than those presented here, one should evaluate the elevation range covered in the RHI mode, the lidar's acquisition time, and the type of scan. Additionally, the influence of the sampling rate on measurements should be considered when planning new experimental campaigns, particularly in the selection of equipment and measurement frequency of targeted wind variables. For a minimum RMSE increase (below 0.1 m s−1 and 0.1 m2 s−2) compared to the 20 Hz frequency, the VM sampling rate should be at least 0.05 Hz for mean quantities and 0.2 Hz for turbulence measurements.
Dual-lidar measurements of range height indicator (RHI) scans in a virtual-mast (VM) mode were compared against sonic anemometer readings at three 100 m towers over the Perdigão complex terrain to evaluate the VM measurement uncertainty and validate its use over large distances above the ground. The study focused on 10 min means and variances of radial velocity (vr), wind speed (Vh), and wind velocity (u and v), retrieved by dual-lidar and sonic anemometers at 80 and 100 m a.g.l. A methodology for processing the virtual-mast dataset was also devised.
In the analysis of the mean flow, a high correlation was found between VM and sonic measurements, with r2 values close to 1 at all VMs. Notably, the lowest r2 were observed at VM2 (0.987 for u and v, and 0.948 for Vh), attributed to the small angle (∼ 40.2°) between the lidars' beams (leading to high dual-lidar error propagation) and to the more turbulent flow in the valley. Regarding the errors, the average RMSE and bias for u and v was 0.422 m s−1 and 0.102 m s−1 for all VMs, with the highest values occurring at VM3, 0.626 m s−1 and 0.575 m s−1, for the u component. The error magnitudes were consistent for all mean flow variables (u, v, and Vh) within each virtual mast. However, the average r2 for Vh (0.969) was lower than that for the wind components (0.994).
The low measuring frequency (0.038 Hz maximum) and the VM location mainly impacted the turbulence measurements ( and ). The average r2 that was equal to 0.994 for the mean wind components was 0.867 for the variances. In the linear regression equation, the constants (b) took on values close to zero for all VMs, while the slope coefficients (m) varied from 0.799 for VM1 to 1.269 for VM2. The greater sensitivity of VM2 to turbulence changes, however, did not translate into better accuracy. The RMSE for and across all VMs averaged 0.340 m2 s−2, with the highest value observed in the valley (VM2), reaching 0.600 m2 s−2 for , due to the worse lidars' synchronisation (about 9 s), the smaller between-beam angle, and the complex valley flow. Overall, the VM correlations against reference turbulence measurements were still high, and the average errors were low (0.340 m2 s−2 RMSE and −0.063 m2 s−2 bias), indicating that small-scale phenomena play a smaller role at 80 and 100 m a.g.l. in Perdigão.
The influence of atmospheric stability also depended on the VM location. The virtual masts on the ridges (VM1 and VM3) showed higher correlations and lower errors under stable compared to unstable conditions. Namely for the variances, where the average r2, RMSE, and bias for VM1 and VM3 under stable (unstable) conditions were equal to 0.853 (0.836), 0.235 m2 s−2 (0.339 m2 s−2), and −0.055 m2 s−2 (−0.140 m2 s−2). In the valley (VM2), the better statistical metrics with stable conditions were restricted to the variance measurements of the wind, showing average r2, RMSE, and bias of 0.891 (0.827), 0.358 m2 s−2 (0.587 m2 s−2), and 0.029 m2 s−2 (0.085 m2 s−2) with stable (unstable) atmosphere. Although atmospheric stability differently affected the accuracy of VM measurements on the ridges and in the valley, the results indicate that in a stable, less turbulent atmosphere, synchronisation between the scans of a multi-lidar system becomes less critical for maintaining measurement accuracy than in unstable conditions. Regarding the VM wind direction, no correlation between its errors and atmospheric stability could be drawn.
The impact of the zero vertical velocity assumption on dual-lidar retrievals at 80 and 100 m a.g.l. in Perdigão was minimal, confirming the validity of the VM results at these heights. The r2 results were lower than 0.060 for the 10 min average w values from sonic anemometer readings and the 10 min horizontal wind speed errors from the VM measurements, and they were lower than 0.110 for the 10 min average w values and the 10 min variance errors from the VMs.
Lastly, the influence of the VM sampling rate accounted for 33 % of the overall RMSE for the mean quantities and 78 % for the variances at 100 m a.g.l. when assuming a linear influence of this factor on the dual-lidar error. The impact of sampling rate on measurements, including those from dual lidars, is crucial when selecting and configuring equipment to ensure accurate recording of target variables.
Overall, Perdigão's VMs obtained accurate mean flow measurements, and their turbulence estimations, despite displaying lower correlations against reference data, also showed low errors, demonstrating the VMs' ability to capture mean and turbulent wind characteristics under different flow conditions, at great heights, and in complex terrain. From the VM measurements and sonic readings, the construction of vertical profiles of the wind enables the analysis of Perdigão's complex flow at heights up to 430 m a.g.l.
For greater data accuracy and reliability in future dual-lidar campaigns, the lidars must be positioned to form an approximately 90° angle between their beams to minimise error propagation and operated at a sampling frequency of at least 0.05 Hz for mean quantities and 0.2 Hz for turbulence. These frequencies yield a minimal RMSE increase (below 0.1 m s−1 and 0.1 m2 s−2) compared to the 20 Hz frequency.
Perdigão-2017 data are available on the websites https://perdigao.fe.up.pt/ (University of Porto, 2020) and https://data.eol.ucar.edu/master_lists/generated/perdigao/ (UCAR/NCAR – Earth Observing Laboratory, 2019c). The anemometric tower measurements are available at https://doi.org/10.26023/8X1N-TCT4-P50X (UCAR/NCAR – Earth Observing Laboratory, 2019a). The lidar data measured by the Technical University of Denmark are also available at https://doi.org/10.11583/DTU.7228544.V1 (Menke et al., 2018).
IC and VB developed the initial methodology, validation, and code. IC refined the methodology, further processed and analysed the data, and wrote the first manuscript draft under the guidance of JM and JP. All authors participated actively in reviewing the work and rewriting multiple versions of the manuscript.
The contact author has declared that none of the authors has any competing interests.
Publisher’s note: Copernicus Publications remains neutral with regard to jurisdictional claims made in the text, published maps, institutional affiliations, or any other geographical representation in this paper. While Copernicus Publications makes every effort to include appropriate place names, the final responsibility lies with the authors.
We thank the anonymous referee and Joachim Reuder for their valuable feedback and suggestions.
This research was carried out within the scope of the LIdar Knowledge Europe (LIKE) project, H2020-MSCAITN-2019, funded by the European Union (grant no. 858358). Jakob Mann's work was supported by the European Union's Horizon Europe Framework programme HORIZON-CL5-2021-D3-03-04 under grant agreement no. 10108420 (FLOW). Vasco Batista and José Palma have received funding from the European Union's Horizon Europe research and innovation programme (HORIZON-MSCA-2022-DN-01-01) under the Marie Sklodowska-Curie grant agreement no. 101119550 (AptWind) and from a research contract with Renewable Energy Systems Ltd and Natural Power Consultants Ltd.
This paper was edited by Leonie von Terzi and reviewed by Joachim Reuder and one anonymous referee.
Bell, T. M., Klein, P., Wildmann, N., and Menke, R.: Analysis of flow in complex terrain using multi-Doppler lidar retrievals, Atmospheric Measurement Techniques, 13, 1357–1371, https://doi.org/10.5194/amt-13-1357-2020, 2020. a
Bingöl, F., Mann, J., and Foussekis, D.: Conically scanning lidar error in complex terrain, Meteorol. Z., 18, 189–195, https://doi.org/10.1127/0941-2948/2009/0368, 2009a. a
Bingöl, F., Mann, J., and Foussekis, D.: Lidar performance in complex terrain modelled by WAsP Engineering, in: Proceedings EWEC, 16–19 pp., Marseille, France, https://backend.orbit.dtu.dk/ws/portalfiles/portal/3744984/2009_41.pdf (last access: 20 December 2024) , 2009b. a
Calhoun, R., Heap, R., Princevac, M., Newsom, R., Fernando, H., and Ligon, D.: Virtual towers using coherent Doppler lidar during the Joint Urban 2003 Dispersion Experiment, J. Appl. Meteorol. Climato., 45, 1116–1126, 2006. a, b, c
Cherukuru, N. W., Calhoun, R., Lehner, M., Hoch, S. W., and Whiteman, C. D.: Instrument configuration for dual-Doppler lidar coplanar scans: METCRAX II, J. Appl. Remote Sens., 9, 096090, https://doi.org/10.1117/1.JRS.9.096090, 2015. a
Choukulkar, A., Brewer, W. A., Sandberg, S. P., Weickmann, A., Bonin, T. A., Hardesty, R. M., Lundquist, J. K., Delgado, R., Iungo, G. V., Ashton, R., Debnath, M., Bianco, L., Wilczak, J. M., Oncley, S., and Wolfe, D.: Evaluation of single and multiple Doppler lidar techniques to measure complex flow during the XPIA field campaign, Atmos. Meas. Tech., 10, 247–264, https://doi.org/10.5194/amt-10-247-2017, 2017. a, b, c, d, e, f
Collier, C. G., Davies, F., Bozier, K. E., Holt, A. R., Middleton, D. R., Pearson, G. N., Siemen, S., Willetts, D. V., Upton, G. J. G., and Young, R. I.: Dual-Doppler Lidar Measurements for Improving Dispersion Models, B. Am. Meteorol. Soc., 86, 825–838, https://doi.org/10.1175/BAMS-86-6-825, 2005. a
Damian, T., Wieser, A., Träumner, K., Corsmeier, U., and Kottmeier, C.: Nocturnal Low-level Jet evolution in a broad valley observed by dual Doppler lidar, Meteorol. Z., 23, 305–313, https://doi.org/10.1127/0941-2948/2014/0543, 2014. a
Davies, F., Collier, C. G., and Bozier, K. E.: Errors associated with dual-Doppler-lidar turbulence measurements, J. Optics A, 7, S280–S289, https://doi.org/10.1088/1464-4258/7/6/005, 2005. a
Debnath, M., Iungo, G. V., Ashton, R., Brewer, W. A., Choukulkar, A., Delgado, R., Lundquist, J. K., Shaw, W. J., Wilczak, J. M., and Wolfe, D.: Vertical profiles of the 3-D wind velocity retrieved from multiple wind lidars performing triple range-height-indicator scans, Atmos. Meas. Tech., 10, 431–444, https://doi.org/10.5194/amt-10-431-2017, 2017a. a
Debnath, M., Iungo, G. V., Brewer, W. A., Choukulkar, A., Delgado, R., Gunter, S., Lundquist, J. K., Schroeder, J. L., Wilczak, J. M., and Wolfe, D.: Assessment of virtual towers performed with scanning wind lidars and Ka-band radars during the XPIA experiment, Atmos. Meas. Tech., 10, 1215–1227, https://doi.org/10.5194/amt-10-1215-2017, 2017b. a
Duscha, C., Pálenik, J., Spengler, T., and Reuder, J.: Observing atmospheric convection with dual-scanning lidars, Atmos. Meas. Tech., 16, 5103–5123, https://doi.org/10.5194/amt-16-5103-2023, 2023. a
Farr, T. G., Rosen, P. A., Caro, E., Crippen, R., Duren, R., Hensley, S., Kobrick, M., Paller, M., Rodriguez, E., Roth, L., Seal, D., Shaffer, S., Shimada, J., Umland, J., Werner, M., Oskin, M., Burbank, D., and Alsdorf, D.: The Shuttle Radar Topography Mission, Rev. Geophys., 45, 2005RG000183, https://doi.org/10.1029/2005RG000183, 2007. a
Fernando, H. J. S., Mann, J., Palma, J. M. L. M., Lundquist, J. K., Barthelmie, R. J., Belo-Pereira, M., Brown, W. O. J., Chow, F. K., Gerz, T., Hocut, C. M., Klein, P. M., Leo, L. S., Matos, J. C., Oncley, S. P., Pryor, S. C., Bariteau, L., Bell, T. M., Bodini, N., Carney, M. B., Courtney, M. S., Creegan, E. D., Dimitrova, R., Gomes, S., Hagen, M., Hyde, J. O., Kigle, S., Krishnamurthy, R., Lopes, J. C., Mazzaro, L., Neher, J. M. T., Menke, R., Murphy, P., Oswald, L., Otarola-Bustos, S., Pattantyus, A. K., Rodrigues, C. V., Schady, A., Sirin, N., Spuler, S., Svensson, E., Tomaszewski, J., Turner, D. D., van Veen, L., Vasiljević, N., Vassallo, D., Voss, S., Wildmann, N., and Wang, Y.: The Perdigão: peering into microscale details of mountain winds, B. Am. Meteorol. Soc., 100, 799–819, https://doi.org/10.1175/BAMS-D-17-0227.1, 2019. a, b, c
Hill, M., Calhoun, R., Fernando, H. J. S., Wieser, A., Dörnbrack, A., Weissmann, M., Mayr, G., and Newsom, R.: Coplanar Doppler lidar retrieval of rotors from T-REX, J. Atmos. Sci., 67, 713–729, https://doi.org/10.1175/2009JAS3016.1, 2010. a, b
Kim, H.-G. and Meissner, C.: Correction of LiDAR measurement error in complex terrain by CFD: Case study of the Yangyang pumped storage plant, Wind Eng., 41, 226–234, https://doi.org/10.1177/0309524X17709725, 2017. a
Klaas, T., Pauscher, L., and Callies, D.: LiDAR-mast deviations in complex terrain and their simulation using CFD, Meteorol. Z., 24, 591–603, https://doi.org/10.1127/metz/2015/0637, 2015. a, b
Liu, X., Zhang, H., Wang, Q., Wang, X., Zhang, X., Li, R., Qin, S., Yin, J., and Wu, S.: Inter-comparison study of wind measurement between the three-lidar-based virtual tower and four lidars using VAD techniques, Geo-spatial Information Science, 1–17 pp., https://doi.org/10.1080/10095020.2024.2307930, 2024. a
Mann, J., Cariou, J.-P., Courtney, M. S., Parmentier, R., Mikkelsen, T., Wagner, R., Lindelöw, P., Sjöholm, M., and Enevoldsen, K.: Comparison of 3D turbulence measurements using three staring wind lidars and a sonic anemometer, IOP Conference Series: Earth and Environmental Science, 1, 012012, https://doi.org/10.1088/1755-1315/1/1/012012, 2008. a
Mann, J., Angelou, N., Arnqvist, J., Callies, D., Cantero, E., Arroyo, R. C., Courtney, M., Cuxart, J., Dellwik, E., Gottschall, J., Ivanell, S., Kühn, P., Lea, G., Matos, J. C., Palma, J. M. L. M., Pauscher, L., Peña, A., Rodrigo, J. S., Söderberg, S., Vasiljevic, N., and Rodrigues, C. V.: Complex terrain experiments in the New European Wind Atlas, Philos. T. R. Soc. A, 375, 20160101, https://doi.org/10.1098/rsta.2016.0101, 2017. a
Menke, R. and Mann, J.: Perdigao 2017: Laser survey of measurement masts, Tech. rep., DTU Wind Energy, https://perdigao.fe.up.pt/documents/file/238 (last access: 20 December 2024), 2017. a
Menke, R., Mann, J., and Vasiljevic, N.: Perdigão-2017: multi-lidar flow mapping over the complex terrain site, Technical University of Denmark [data set], https://doi.org/10.11583/DTU.7228544.V1, 2018. a
Menke, R., Vasiljevic, N., and Mann, J.: Perdigão 2017: DTU's scanning lidar measurements, Tech. rep., DTU Wind Energy, Denmark, https://windsptds.fe.up.pt/thredds/fileServer/upperAir/Lidar/DTU Scanning Lidar Data/netcdf/DTU_Lidar_Measurement_Report_v1.0.pdf (last access: 7 March 2024), 2019a. a, b
Menke, R., Vasiljević, N., Mann, J., and Lundquist, J. K.: Characterization of flow recirculation zones at the Perdigão site using multi-lidar measurements, Atmos. Chem. Phys., 19, 2713–2723, https://doi.org/10.5194/acp-19-2713-2019, 2019b. a, b
Newman, J. F., Bonin, T. A., Klein, P. M., Wharton, S., and Newsom, R. K.: Testing and validation of multi-lidar scanning strategies for wind energy applications, Wind Energy, 19, 2239–2254, https://doi.org/10.1002/we.1978, 2016. a, b, c, d, e
Newsom, R. K., Ligon, D., Calhoun, R., Heap, R., Cregan, E., and Princevac, M.: Retrieval of microscale wind and temperature fields from single- and dual-Doppler lidar data, J. Appl. Meteorol., 44, 1324–1345, https://doi.org/10.1175/JAM2280.1, 2005. a, b
Ng, C. W. and Hon, K. K.: Fast dual-doppler LiDAR retrieval of boundary layer wind profiles, Weather, 77, 134–142, https://doi.org/10.1002/wea.3800, 2022. a
Palma, J. M. L. M., Silva, C. A. M., Gomes, V. C., Silva Lopes, A., Simões, T., Costa, P., and Batista, V. T. P.: The digital terrain model in the computational modelling of the flow over the Perdigão site: the appropriate grid size, Wind Energy Sci., 5, 1469–1485, https://doi.org/10.5194/wes-5-1469-2020, 2020. a
Pauscher, L., Vasiljevic, N., Callies, D., Lea, G., Mann, J., Klaas, T., Hieronimus, J., Gottschall, J., Schwesig, A., Kühn, M., and Courtney, M.: An inter-comparison study of multi- and DBS lidar measurements in complex terrain, Remote Sens., 8, 782, https://doi.org/10.3390/rs8090782, 2016. a, b, c, d, e, f
Pitter, M., Abiven, C., Vogstad, K., Harris, M., Barker, W., and Brady, O.: Lidar and computational fluid dynamics for resource assessment in complex terrain, in: Proceedings EWEA, Copenhagen, Denmark, https://www.zxlidars.com/wp-content/uploads/2021/07/EWEA-Lidar-and-CFD-in-complex-terrain-paper-v6.pdf (last access: 20 December 2024), 2012. a
Rothermel, J., Kessinger, C., and Davis, D. L.: Dual-Doppler lidar measurement of winds in the JAWS experiment, J. Atmos. Ocean. Technol., 2, 138–147, https://doi.org/10.1175/1520-0426(1985)002<0138:DDLMOW>2.0.CO;2, 1985. a
Santos, P., Mann, J., Vasiljević, N., Cantero, E., Sanz Rodrigo, J., Borbón, F., Martínez-Villagrasa, D., Martí, B., and Cuxart, J.: The Alaiz experiment: untangling multi-scale stratified flows over complex terrain, Wind Energy Sci., 5, 1793–1810, https://doi.org/10.5194/wes-5-1793-2020, 2020. a
Sathe, A., Mann, J., Gottschall, J., and Courtney, M. S.: Can Wind Lidars Measure Turbulence?, J. Atmos. Ocean. Technol., 28, 853–868, https://doi.org/10.1175/JTECH-D-10-05004.1, 2011. a
Sjöholm, M., Mikkelsen, T., Mann, J., Enevoldsen, K., and Courtney, M.: Spatial averaging-effects on turbulence measured by a continuous-wave coherent lidar, Meteorol. Z., 18, 281–287, https://doi.org/10.1127/0941-2948/2009/0379, 2009. a
Stawiarski, C., Träumner, K., Knigge, C., and Calhoun, R.: Scopes and challenges of dual-Doppler lidar wind measurements – An error analysis, J. Atmos. Ocean. Technol., 30, 2044–2062, https://doi.org/10.1175/JTECH-D-12-00244.1, 2013. a, b, c
Strauch, R. G., Merritt, D. A., Moran, K. P., Earnshaw, K. B., and De Kamp, D. V.: The Colorado Wind-Profiling Network, J. Atmos. Ocean. Technol., 1, 37–49, https://doi.org/10.1175/1520-0426(1984)001<0037:TCWPN>2.0.CO;2, 1984. a
Stull, R. B.: An introduction to boundary layer meteorology, Springer Netherlands, Dordrecht, https://doi.org/10.1007/978-94-009-3027-8, 1988. a, b
UCAR/NCAR – Earth Observing Laboratory: NCAR/EOL Quality Controlled High-rate ISFS surface flux data, geographic coordinate, tilt corrected, Version 1.1, UCAR/NCAR – Earth Observing Laboratory [data set], https://doi.org/10.26023/8X1N-TCT4-P50X, 2019a. a
UCAR/NCAR – Earth Observing Laboratory: Perdigão-ISFS Data Report, https://www.eol.ucar.edu/content/perdigao-isfs-data-report (last access: 20 December 2024), 2019b. a
UCAR/NCAR – Earth Observing Laboratory: Perdigao Data Sets, https://data.eol.ucar.edu/master_lists/generated/perdigao/ (last access: 20 December 2024), 2019c. a
University of Porto: Perdigão field experiment, https://perdigao.fe.up.pt/ (last access: 7 March 2024), 2020. a
Vasiljević, N., Lea, G., Courtney, M., Cariou, J.-P., Mann, J., and Mikkelsen, T.: Long-Range WindScanner System, Remote Sens., 8, 896, https://doi.org/10.3390/rs8110896, 2016. a, b
Wildmann, N., Vasiljevic, N., and Gerz, T.: Wind turbine wake measurements with automatically adjusting scanning trajectories in a multi-Doppler lidar setup, Atmos. Meas. Tech., 11, 3801–3814, https://doi.org/10.5194/amt-11-3801-2018, 2018. a
Wildmann, N., Bodini, N., Lundquist, J. K., Bariteau, L., and Wagner, J.: Estimation of turbulence dissipation rate from Doppler wind lidars and in situ instrumentation for the Perdigão 2017 campaign, Atmos. Meas. Tech., 12, 6401–6423, https://doi.org/10.5194/amt-12-6401-2019, 2019. a
Wittkamp, N., Adler, B., Kalthoff, N., and Kiseleva, O.: Mesoscale wind patterns over the complex urban terrain around Stuttgart investigated with dual-Doppler lidar profiles, Meteorol. Z., 30, 185–200, https://doi.org/10.1127/metz/2020/1029, 2021. a, b, c