the Creative Commons Attribution 4.0 License.
the Creative Commons Attribution 4.0 License.
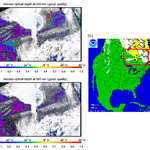
Screening for snow/snowmelt in SNPP VIIRS aerosol optical depth algorithm
Jingfeng Huang
Istvan Laszlo
Lorraine A. Remer
Hongqing Liu
Hai Zhang
Pubu Ciren
Shobha Kondragunta
The Visible Infrared Imaging Radiometer Suite (VIIRS) on board the Suomi National Polar-orbiting Partnership (S-NPP) spacecraft provides validated daily global aerosol optical depth (AOD) retrievals; however, a close examination of the VIIRS aerosol product identified residual snow and snowmelt contamination, resulting generally in an overestimation of AOD. The contamination was particularly evident over Northern Hemisphere high-latitude regions during the spring thaw. To improve the product performance, we introduced a new empirical snow and snowmelt screening scheme that combines a normalized difference snow index (NDSI)- and brightness temperature (BT)-based snow test, a snow adjacency test and a spatial homogeneity test (a.k.a. spatial filter). Testing of retrievals for 18 May 2014 indicated that, compared to the previous, visible reflectance anomaly (VRA)-based snow test, the new NDSI- and BT-based snow test screened out an additional 3.44 % of VIIRS AOD retrievals, most of which were over high latitudes experiencing snowmelt. The new snow adjacency test and the homogeneity test degraded another 5.57 % and 0.26 %, respectively, otherwise “good”-quality AOD retrievals. For the VIIRS–AERONET (Aerosol Robotic Network) matchups over Northern Hemisphere high-latitude regions during 3 years of spring (2013–2015), the new scheme also effectively screened out a significant number of the matchups that had anomalously high positive biases attributable to snow and snowmelt contamination. The new snow and snowmelt screening scheme was transferred to the Interface Data Processing Segment (IDPS) VIIRS aerosol algorithm on 22 June 2015. Subsequently no significant snow and snowmelt contamination was found during spring 2016. The scheme is also implemented in the new Enterprise VIIRS aerosol algorithm in the National Oceanic and Atmospheric Administration (NOAA) Enterprise Processing System (EPS) that became operational in 2017.
Nowadays with increasing public awareness of air pollution and aerosol climatic effects, satellite observations of global aerosol loading and transport provide valuable information for improving our understanding of the impact of aerosols on weather, climate and public health (Kaufman et al., 2002; Quaas et al., 2008; Al-Saadi et al., 2005; van Donkelaar et al., 2010; Kloog et al., 2011). Although satellite retrievals of aerosol optical properties in cloud-free and even in cloudy scenes have advanced tremendously over the past decades (Lenoble et al., 2013; Shi et al., 2014; Jethva et al., 2016; Meyer et al., 2015; Sayer et al., 2016), aerosol observations over snow scenes are still not feasible by passive sensors.
Most overland aerosol algorithms from passive sensors make assumptions about surface reflectance properties in the retrieval scene in order to separate the signal from aerosol scattering in the atmosphere from the signal originating from reflectance from Earth's surface (Remer et al., 2005; Levy et al., 2013; Jackson et al., 2013). Snow reflectance properties vary significantly with snow grain size (Wiscombe and Warren, 1980; Warren, 1982) and impurities in or on the snow (Doherty et al., 2010; Hadley and Kirchstetter, 2012), all of which change rapidly in time as the snow ages, and especially as it melts. The quickly changing optical properties of snow introduce too much uncertainty for global operational aerosol retrieval algorithms (Li et al., 2005). Therefore, screening out snow pixels is a necessary procedure in almost every aerosol retrieval algorithm, yet it remains a daunting challenge, particularly if the scene is complicated with sub-pixel or melting snow. Therefore the aerosol optical depth (AOD) retrievals adjacent to snow still have large uncertainties due to potential snow contamination (Lyapustin et al., 2012). Note that this snow contamination introduces a positive bias in the aerosol retrieval, as snow is bright in the visible and very dark in the near and shortwave infrared. Many passive satellite aerosol retrieval algorithms interpret the brighter-than-assumed visible surface reflectance as extra aerosol loading (Li et al., 2005). Even a small amount of sub-pixel snow in the retrieval scene can introduce positive bias in the AOD.
The Visible Infrared Imaging Radiometer Suite (VIIRS) aboard the Suomi National Polar-orbiting Partnership (S-NPP), launched on 28 October 2011, is the first satellite in the series of the United States' next-generation polar-orbiting operational environmental satellite system, the Joint Polar Satellite System (JPSS). The VIIRS aerosol products, following the aerosol products from heritage sensors such as the Moderate Resolution Imaging Spectrometer (MODIS) and the Multi-angle Imaging Spectroradiometer (MISR), continue to succeed in providing daily global aerosol observations for operational and scientific user communities (Jackson et al., 2013; Liu et al., 2014; Huang et al., 2016; Zhang et al., 2016). When the VIIRS aerosol products were compared to to the Aerosol Robotic Network (AERONET; Holben et al., 1998) ground measurements and the MODIS aerosol products, the validation results indicated that the AOD over land reached validated maturity on 23 January 2013 and the AOD over ocean reached validated maturity on 2 May 2012 (Liu et al., 2014; Huang et al., 2016).
With more than 3 years of the validated S-NPP VIIRS aerosol products publicly available and used in various user applications, further in-depth data analyses show that the VIIRS retrievals are consistently overestimating the AOD, when compared with AERONET, over high-latitude regions in the Northern Hemisphere, especially during the spring thaw, when snow is melting. This implies potential snow and snowmelt contamination in the products.
The VIIRS aerosol products are generated operationally in the so-called Interface Data Processing Segment (IDPS), which is one of the segments of the NPP project that processes the raw observations into environmental data records (geophysical parameters). Versions of the system, representing increasing maturity and algorithm updates, are referred to as Mx builds in a given block (In IDPS a block is similar to a collection in the MODIS processing.) The original at-launch snow test, summarized in the next section, was implemented in the IDPS VIIRS aerosol algorithm from 9 December 2011 to 22 June 2015, until the Mx8.8 build in Block 1.x. The new snow and snowmelt mask described in this paper was implemented in the Mx8.10 build on 22 June 2015 and has been running in the IDPS ever since. The new snow mask was further refined by tuning threshold values, and it has been implemented in the National Oceanic and Atmospheric Administration (NOAA), Enterprise Processing System (EPS) VIIRS aerosol algorithm, which is replacing the IDPS algorithm.
This paper presents the identification of the snow contamination in Sect. 2, the development of a new snow and snowmelt screening scheme in Sect. 3, and the evaluation of the new scheme in the VIIRS operational aerosol products in Sect. 4, followed by summary and discussion in Sect. 5.
Note that all snow screening tests discussed in this paper are designed to prevent the aerosol algorithm from making retrievals in inappropriate snow cover conditions. For a true snow product, users are directed to the S-NPP VIIRS Snow/Ice products (Key et al., 2013).
According to Jackson et al. (2013), the snow screening in the VIIRS land aerosol algorithm consists of two parts: the snow flag from the upstream VIIRS Cloud Mask (VCM) product, and the internal snow test within the VIIRS aerosol algorithm itself. When aerosol observations are feasible under daytime conditions, the VCM uses the VIIRS Gridded Snow Cover product in conjunction with a reflectance-based snow detection algorithm to check for snow surfaces. However, to avoid significant AOD overestimation caused by snow pixels or sub-pixel snow conditions, the snow screening for aerosol observations needs to be stricter than the general snow detection in the VCM. Thus an internal snow detection scheme within the aerosol algorithm becomes necessary to complement the VCM snow flag. Prior to 22 June 2015, the internal snow detection in the operational IDPS VIIRS aerosol algorithm was based on three tests: (1) the visible reflectance anomaly (VRA), (2) the ratio of top of the atmosphere (TOA) reflectance in the 1240 nm channel (ρ1240, VIIRS band M8) to the 865 nm channel (ρ865, VIIRS band M7) and (3) the surface temperature derived from the split-window technique (Walton et al., 1998). This at-launch internal snow test is referred to as the “VRA-based snow test” hereafter in the paper. VRA is defined as
where and are 488 nm (VIIRS band M3) and 672 nm (VIIRS band M5) surface reflectances. Equation (1) is based on the retrieval assumption that the surface reflectance of the blue wavelength is roughly half that of the red wavelength (Kaufman et al., 1997; Jackson et al., 2013). A significant deviation from this well-established surface reflectance relationship indicates a surface outside of our range of assumptions and should not be used for aerosol retrievals.
In the VIIRS aerosol algorithm with the VRA-based snow test, as shown in Table 1, if the reflectances in the required bands are available and the following conditions are met, the internal snow test sets the snow flag and no aerosol retrievals are reported in the VIIRS aerosol product:
-
VRA > 0.02;
-
;
-
surface temperature <278 K;
-
no cirrus is detected;
-
cloud mask is confidently or probably clear.
When applying this VRA-based snow test, the AOD retrievals over high-latitude geographic regions were, however, consistently overestimated during the boreal spring thaw, when snow was melting (Jackson et al., 2013; Liu et al., 2014; Huang et al., 2016). Neither the external VCM snow tests nor the internal VRA-based snow test was able to effectively detect and screen out those snow- and snowmelt-contaminated pixels, and anomalously high AODs were reported as good-quality retrievals. Those unfiltered snow or snowmelt pixels are usually more reflective in the visible and thus can result in falsely high AOD retrievals if they are mistaken as aerosols. The global validation of the S-NPP VIIRS AOD product reported that such snow and snowmelt contamination frequently happened during spring thaw over high-latitude geographic regions, such as northern Canada and northern Russia. This widespread residual snow and snowmelt contamination caused a significant high bias in the AOD product. Using the AERONET ground measurement, the validation of the VIIRS AOD during February–May of 2013–2015 showed a strong positive bias of +0.073, not meeting the requirement of ±0.06 when AOD ≤ 0.8 (NOAA JPSS Level 1 Requirements Document, https://www.jpss.noaa.gov/assets/pdfs/technical_documents/level_1_requirements.pdf, last access: 1 October 2018). This required an alternative internal snow test in the VIIRS aerosol algorithm to replace the VRA-based snow test.
Table 1Criteria for the VRA-based snow test, the NDSI-based snow test, the snow adjacency test and the homogeneity test.
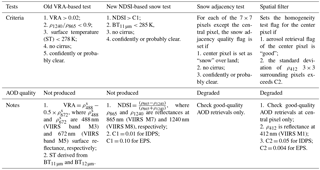
An empirical snow detection technique using near-infrared reflectances at 860 and 1240 nm and brightness temperature (BT) at 11 µm was proposed by Li et al. (2005). This scheme was implemented in the MODIS operational aerosol algorithm beginning with Collection 5 (Levy et al., 2007, 2009, 2013). This paper explores the applicability of a similar approach in the VIIRS aerosol algorithm by using the VIIRS bands. Snow adjacency and spatial homogeneity tests complement this snow test to form a systematic snow and snowmelt screening scheme in the VIIRS aerosol algorithm.
The new empirical snow and snowmelt screening scheme consists of three separate tests: snow test, snow adjacency test and spatial homogeneity test.
3.1 The NDSI- and BT-based snow test
The snow test follows the approach of Li et al. (2005). Similar to step 3 in the VRA-based snow test, this snow test also uses near-infrared and shortwave infrared TOA reflectances, but instead of their simple ratio it calculates the ratio of their difference to their sum, the so-called normalized difference snow index (NDSI) (defined below) and compares it to a threshold value. Normalization is used as a means to adjust for the effects of the solar and view zenith angles because the normalized form is less sensitive to changes in the two angles than the simple ratio form (i.e., Walter-Shea et al., 1997). Again, similar to step 4 in the VRA-based snow detection, it also uses a thermal-infrared brightness temperature test but with a different threshold value. In our adaptation we substitute the following VIIRS spectral bands for the MODIS bands in Li et al. (2005). We calculate the NDSI from ρ865 and ρ1240 (VIIRS bands M7 and M8) and the BT at 10.76 µm (BT11 µm, VIIRS band M15). To differentiate this test from the VRA-based snow test, we call this new snow test the “NDSI-based snow test” hereafter.
The theoretical basis of the NDSI-based snow test lies in the fact that the spectral dependence of snow reflectance is very different from that of vegetation and soils. The reflectance of snow decreases rapidly with increasing wavelength from visible to shortwave infrared in the 800–1300 nm wavelength range due to strong ice absorption features centered near 1050 and 1240 nm. The reflectance of green vegetation decreases only slightly with increasing wavelength due to weak liquid water absorption bands centered near 960 and 1180 nm. The reflectances of soil and dry vegetation, however, typically increase with wavelength in the same spectral region. Therefore the reflectance of snow is higher than that of green vegetation and soil in visible bands but much lower in shortwave infrared bands (Fig. 3 in Li et al., 2005). Based on the spectral properties of snow, vegetation and soil, the NDSI, which was first used in Gao (1996), is defined as follows:
where ρ865 and ρ1240 are reflectances at 865 nm (VIIRS M7) and 1240 nm (VIIRS M8), respectively. It is noteworthy that, while the IDPS algorithm uses TOA reflectances in NDSI, the EPS algorithm uses Rayleigh-scattering and gas-absorption-corrected reflectances to minimize these effects on NDSI.
Similarly to Li et al. (2005), to avoid over-screening of vegetated pixels, BT11 is also used for further stratification.
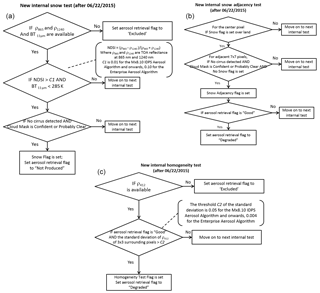
Figure 1Flow charts of the new snow and snowmelt snow screening scheme in the VIIRS aerosol algorithm: (a) internal snow test; (b) internal snow adjacency test; and (c) internal homogeneity test or spatial filter. The tests were transferred to operation to the Mx8.10 IDPS algorithm on 22 June 2015 at 19:43 UTC, and they are also used in the new Enterprise VIIRS aerosol algorithm. “Move on to next internal test” means no actions are taken for the current internal test if any of the criteria are not met, and the algorithm continues to the next internal test.
As Table 1 and the flowchart in Fig. 1a show, this NDSI-based snow test will set a snow flag when the required reflectance and brightness temperature in respective bands are available and the following criteria are met:
-
NDSI > C1 (see below for threshold values used);
-
BT11 µm < 285 K;
-
no cirrus is detected;
-
cloud mask is confidently or probably clear.
C1 has been set to 0.01 in Mx8.10 and newer versions of the IDPS aerosol algorithm. However, evaluation of retrievals over pixels with heavy smog, particularly over eastern China during the boreal spring season, showed that this threshold value resulted in over-screening of such pixels. This happened because some heavy-smog pixels also exhibited an NDSI data range (>0.01) and lower brightness temperature (BT11 µm<285 K) similar to those for snow pixels, indicating stronger absorption of smog particles in the shortwave infrared than in the near infrared and relatively low brightness temperatures at 11 µm. Thus the criteria of NDSI > 0.01 prevented potentially good AOD retrievals of these China smog events (Huang et al., 2017). Adjusting the NDSI threshold value showed that a higher threshold of 0.10 helps to regain most AOD retrievals over the heavy-air-pollution pixels that previously went missing in the IDPS algorithm. Therefore in the EPS algorithm the higher C1 value (0.1) has been adopted. At the same time, the threshold in the spatial filter had to be adjusted as well (in Sect. 3.3) to ensure the new threshold values will not re-introduce artifacts due to snow contamination in other areas (Huang et al., 2017).
Because snow is one of the conditions that prevent meaningful aerosol retrievals, there are no aerosol retrievals over pixels with snow flags (Jackson et al., 2013). Therefore, in the VIIRS aerosol product, those pixels are filled with the fill value, and the quality of the retrievals over those pixels is set as “not produced”.
3.2 Snow adjacency test
Although the NDSI-based snow test improves the snow pixel detection significantly, some residual snowmelt contamination surrounding the snow pixels still exists when we verify AOD retrievals over snow scenes. We attribute this contamination to unidentified sub-pixel snow. To minimize such contamination, a snow adjacency test is introduced as an additional quality assurance procedure in the aerosol algorithm. If the test shows a pixel is within an area of 7×7 pixels surrounding a snow pixel, the aerosol retrieval for that pixel is likely susceptible to snowmelt contamination and the condition becomes unfavorable for meaningful aerosol retrievals.
As shown in Table 1 and Fig. 1b, the new snow adjacency test loops through the adjacent 7×7 pixels surrounding the central snow pixel. For each of the 7×7 pixels except the central pixel, the snow adjacency quality flag is set if the following criteria are met:
-
center pixel is set as “snow” over land;
-
no cirrus is detected;
-
cloud mask is confidently or probably clear.
However, unlike the snow test, the snow adjacency test is designated as one of the “degradation” retrieval conditions rather than a “not-produced” retrieval condition. After the snow adjacency quality flag is set for a particular pixel, the snow adjacency test continues to check whether the aerosol retrieval quality is “good”. If the aerosol retrieval quality is “good”, the snow adjacency test degrades the aerosol retrieval quality from “good” to “degraded”; otherwise, the aerosol retrieval quality is not changed.
3.3 Spatial homogeneity test (spatial filter)
The third test, like the snow adjacency test, is meant to caution users that retrieved AOD may be susceptible to sub-pixel snow and snowmelt situations. This alert becomes especially important during spring thaw, when snowmelt pixels can spread over large geographic areas. Pixels containing a surface with a mixture of exposed soil, vegetation, old snow and soggy slush introduce a level of spatial inhomogeneity not seen during other seasons. To identify this situation, an internal spatial homogeneity test is introduced in the aerosol algorithm. The spatial filter calculates the standard deviation of the TOA 412 nm reflectance (ρ412, VIIRS band M1) in a grouping of 3×3 pixels to assess the spatial homogeneity. If the assessment shows large spatial heterogeneity of the M1 TOA reflectance within the surrounding 3×3 pixel area, the condition becomes unfavorable for meaningful aerosol retrievals. The 412 nm reflectance is used because of the generally low reflectance of snow-free land surfaces at this wavelength.
As shown in Table 1 and Fig. 1c, the spatial filter sets the homogeneity test flag for the center pixel when the following criteria are met:
-
Aerosol retrieval flag of the center pixel is “good”;
-
the standard deviation of ρ412 of 3×3 surrounding pixels exceeds C2.
C2 has been set to 0.05 in the Mx8.10 and newer versions of the IDPS aerosol algorithm. As has been mentioned above, in the EPS algorithm the NDSI threshold was relaxed from its IDPS value to regain retrievals over heavy-smog pixels (see Sect. 3.1), and consequently the value of C2 had to be adjusted accordingly to ensure a stricter homogeneity requirement to compensate. This is because smog events are usually homogeneous at these spatial scales, and thus an adjustment of the spatial filter threshold does not cause over-screening of smog pixels or other retrieval scenes yet does manage to eliminate the spatially variable sub-pixel snow that appeared once the NDSI threshold was relaxed. The adjustment helps minimize the potential false aerosol retrievals over pixels with sub-pixel snow and NDSI values in the range of 0.01–0.10. However, to verify the new NDSI threshold will not introduce snow and snowmelt contamination, we closely examined 24 granules with smog pixels on smog-prevalent days over China from 29 November 2015 to 29 February 2016, and another 30 granules with snow and snowmelt pixels over Canada on 27–31 March and 28–29 May 2015. With same sets of threshold values of NDSI and spatial filter applied to both heavy smog and snow scenes, a new C2 = 0.004 was carefully selected to achieve a balance between screening for sub-pixel snow and allowing retrievals of heavy smog. More justification of the threshold changes can be found in Sect. 4, where the aerosol retrievals from the EPS algorithm are discussed.
Due to the nature of the spatial filter, the homogeneity test not only helps detecting sub-pixel snow pixels but also helps screening partial cloudy pixels that contain sub-pixel cloud. The test is particularly effective for those low-level “popcorn” cumulus clouds, which are so small in spatial scale that they cause higher spatial variability. Since both cloud and snow conditions are unfavorable conditions for meaningful satellite aerosol retrievals, the homogeneity test provides additional quality assurances to the VIIRS aerosol retrievals in terms of both snow and cloud screenings.
Similar to the snow adjacency test, the homogeneity test is designated as one of the “degradation” conditions rather than a “not-produced” condition. However, for efficient aerosol retrieval the homogeneity test is only conducted when the aerosol retrieval quality of the center pixel is “good”. If this flag is “good” and the above criteria are met, the homogeneity test quality flag is set and the quality of the aerosol retrieval is degraded from “good” to “degraded”.
To demonstrate the effectiveness of the new snow and snowmelt screening scheme, we apply old and new schemes to the same granule. In this test the new scheme uses the thresholds in the Mx8.10 IDPS algorithm (C1 = 0.01 and C2 = 0.05). As seen in Fig. 2a, an example of “good” quality VIIRS AOD retrievals clearly shows anomalously high AOD values over areas to the east and south of the Hudson Bay in Canada on 19 May 2015 when the snowpack was melting, as indicated by the snow cover map in Fig. 2c from the NOAA National Centers for Environmental Information (NCEI) (https://www.ncdc.noaa.gov/snow-and-ice/snow-cover/us/20150519, last access: 1 October 2018). Comparing the results of the new NDSI-based scheme in Fig. 2b with the VRA-based snow test of Fig. 2a clearly shows that the amount of anomalously high AOD retrievals are significantly reduced, implying the new scheme has effectively reduced the snow and snowmelt contamination in the good-quality AOD retrievals.
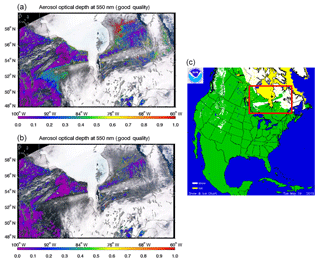
Figure 2VIIRS “good”-quality AOD retrievals on 19 May 2015: (a) with the old VRA-based internal snow test; (b) with the new snow and snowmelt screening scheme; and (c) the snow cover map produced by the NOAA National Centers for Environmental Information (NCEI) (https://www.ncdc.noaa.gov/snow-and-ice/snow-cover/us/20150519, last access: 1 October 2018). The red square in (c) shows the areas covered in (a) and (b). Note the snow cover to the east and south of the Hudson Bay in (c) and the associated anomalously high AOD values over the same areas in (a) but not in (b).
We can better understand the workings of the new scheme by comparing the spectral reflectance of different populations of pixels found in the granules of Fig. 2. Five populations of pixels are shown in Fig. 3: (1) snow pixels identified by the VRA-based snow test; (2) snow pixels identified by the NDSI-based snow test; (3) snow or snowmelt pixels flagged by the spatial filter; (4) snow adjacent pixels flagged by the snow adjacency test; and (5) pixels with good-quality aerosol retrievals from both versions of the algorithm, where the retrieval conditions should be less susceptible to snow and snowmelt contamination.
The averages of TOA reflectances at 11 VIIRS spectral bands, the NDSI and the BT11 µm are calculated for each population. The TOA reflectance ρ865 and ρ1240 are connected by a line segment to show the slopes better. Because ice particles have much stronger absorption at 1240 nm than soil and vegetation, the steeper the negative slope from 865 to 1240 nm, the higher the NDSI value and the more likely that snow exists in those pixels. While this slope is negative for snow and slightly positive for soil, as explained in Sect. 3.1, the pixels with sub-pixel snow should feature a reduced steepness of the slope depending on the relative coverage of soil and snow within the pixel. Similarly, because the absolute temperature of snow is generally lower than that of vegetation or soil, the more snow that exists in the pixels, the lower the radiation emitted by the pixels and thus the lower the brightness temperature at 11 µm (i.e., Gutman et al., 1995; Hori et al., 2006; Li et al., 2013).
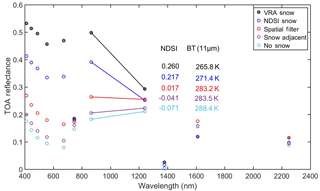
Figure 3Spectral curve of the TOA reflectance at 11 VIIRS wavelengths in five populations of pixels selected based on internal tests.
As shown in Fig. 3, the NDSI and BT values for pixels identified as snow by the VRA-based snow test are 0.260 and 265.8 K, respectively, and those for pixels identified as snow by the NDSI-based snow test are 0.217 and 271.4 K. Thus, the NDSI-based snow test has been more aggressive, categorizing pixels as snow despite their spectral signature and temperature being less characteristically snow-like (that is, darker and warmer) than was required by the VRA-based snow test. The snow adjacency and spatial filter tests continue in this vein identifying more pixels with even less snow-like characteristics: NDSI and BT11 µm values are 0.017 and 283.2 K for the spatial filter category and −0.041 and 283.5 K for the snow adjacency category, respectively. For the category with good-quality aerosol retrieval after both schemes were applied, the NDSI is lowest (−0.071) and BT11 µm is highest (288.4 K), indicating surface conditions with the least snow-like characteristics.
The VRA-based and NDSI-based snow screen schemes were implemented into the aerosol algorithm and applied globally for the testing date of 18 May 2014. Figure 4 shows the good-quality VIIRS AOD retrievals with the old (Fig. 4a) and new tests (Fig. 4b), and their differences (Fig. 4c). Most of the reduced good-quality AOD retrievals were found over high-latitude snowmelt-prevalent regions. They either became “not produced” because of the stricter new snow test or were degraded from “good” to “degraded” by the new snow adjacency test and the spatial filter.
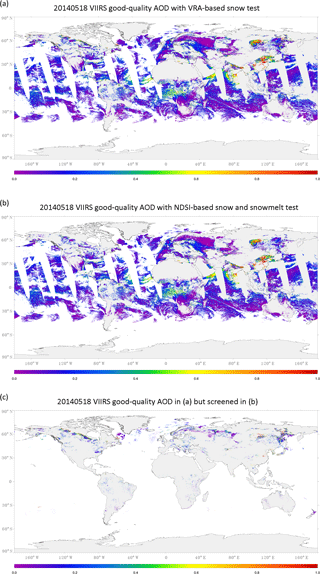
Figure 4The good-quality VIIRS AOD retrievals on 18 May 2014: (a) with the old internal VRA-based snow test; (b) with the new NDSI-based snow and snowmelt screening scheme; and (c) the difference between (b) and (a) that either were removed because of the new snow test or were degraded by the new snow adjacency test and the spatial homogeneity test.
To demonstrate the impact of the tests on the number of retrievals, statistics of sample size and percentage change in the number of AOD retrievals were calculated for 18 10∘ latitude bins, and the results are shown in Fig. 5. As expected, the new snow, adjacency and spatial homogeneity tests have largest impact over high-latitude regions in the Northern Hemisphere, where snow rapidly melts during boreal spring thaw. Overall, global statistics indicate that the new snow test screened out an additional 3.44 % of VIIRS AOD retrievals, and the new snow adjacency test and the homogeneity test degraded another additional 5.57 % and 0.26 % “good”-quality AOD retrievals, respectively. Although these percentages may change from day to day, they provide rough estimates of the magnitudes of the percentage change in the number of good aerosol retrievals when the VRA-based snow test is replaced by the new snow and snowmelt screening scheme.
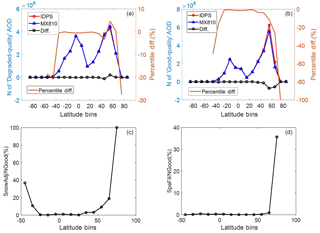
Figure 5Statistics in the sample size and percentage change in the AOD retrievals on 18 May 2014 as a function of 10∘ latitude bin: (a) number of “degraded”-quality AOD retrievals, produced by the algorithm running the old VRA-based snow mask (red line with squares) and the new NDSI-based snow mask (blue line with triangles), and the difference between the two (black line with open circles). The brownish-red line displays the difference as a percentage that is read along the right-hand axis. (b) Same as (a) but for “good”-quality AOD retrievals only; (c) the percentage of the pixels flagged as adjacent to an identified snow pixel over the total number of “good”-quality AOD IP retrievals; and (d) the percentage of the pixels flagged by the homogeneity test (spatial filter) over the total number of “good”-quality AOD retrievals.
The effectiveness of the new snow and snowmelt screening scheme is also verified from the validation of the VIIRS AOD products with AERONET ground measurements. Figure 6 compares the VIIRS–AERONET matchups over 50–90∘ N, 50–130∘ W during boreal spring, February–May 2013–2015, during which period the VRA-based snow test was still in operation in the VIIRS aerosol algorithm. A matchup is defined as the mean good-quality VIIRS AOD retrievals within 27.5 km from an AERONET site and the mean AERONET AOD observations within ±30 min of the VIIRS overpass time.
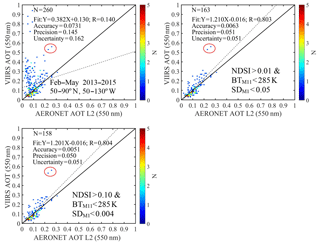
Figure 6Matchups of the VIIRS good-quality AOD retrievals with AERONET over 50–90∘ N, 50–130∘ W during boreal spring February–May 2013–2015: (a) with the old VRA-based internal snow test; (b) with the new snow and snowmelt screening scheme and the thresholds used in the Mx8.10 IDPS algorithm; and (c) with the new snow and snowmelt screening scheme but the thresholds used in the EPS algorithm. The two anomalous points with higher positive biases in the red circles were found to be more related to the AOD retrieval itself rather than snow under-screening.
A significant number of anomalously high VIIRS AOD retrievals with positive biases were found in the retrievals plotted in Fig. 6a. Then for each matchup, the corresponding NDSI, BT, snow adjacency and spatial standard deviations were calculated, so that we could apply the various tests of the NDSI-based snow screen using the different threshold values for the various versions of the algorithm. The VIIRS–AERONET matchups in Fig. 6a were removed where the new snow test would have prevented the retrieval or the new snow adjacency test or homogeneity test would have degraded the quality of the retrieval. Figure 6b and c showed two screening conditions; one uses thresholds from the Mx8.10 IDPS algorithm, and the other uses thresholds from the EPS algorithm. Out of 260 matchups in Fig. 6a, using the IDPS thresholds, 43 were identified as snow, 94 were found with the adjacency test and none were found with the spatial filter test. Because some of the snow pixels were also adjacent to other snow pixels, resulting in redundancy, 97 pixels were screened out, leaving 163 remaining matchups after the screening, with much improved accuracy, much lower uncertainty and much better agreement to AERONET.
Similarly, the same level of improvement was also achieved with the EPS thresholds. Thirty matchups were found with snow, 94 with the adjacency test and 81 with the spatial filter test because of the tighter threshold for the standard deviation. Allowing for pixels with multiple conditions, the screening with the EPS thresholds resulted in 158 remaining matchups in Fig. 6c, having better agreement to AERONET. There are two anomalous points in Fig. 6a–c with higher positive biases in the red circles that were not screened out. With the same snow screening scheme, we ran the EPS algorithm on the same granule and found that the same two matchups with higher positive biases in the red circles were retrieved by the EPS algorithm as good-quality retrievals, but their biases were significantly reduced by ∼0.2 from 0.5–0.6 to 0.3–0.4. This means the positive biases of these two anomalies seemed to be related to the AOD retrieval itself rather than under-screening snow.
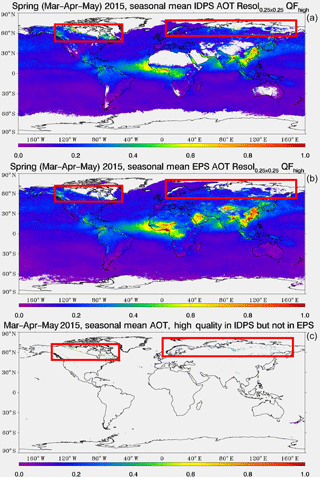
Figure 7S-NPP VIIRS seasonal mean of good-quality AOD during the spring thaw season, March–May 2015: (a) with the old VRA-based snow test in the IDPS aerosol algorithm; (b) with the new snow and snowmelt screening scheme in the EPS aerosol algorithm; and (c) good-quality AOD retrievals in the IDPS product but not in the EPS product, mainly attributable to the improved snow and snowmelt tests. The most outstanding snow and snowmelt under-screening regions over Northern Hemisphere high-latitude regions are highlighted in red squares. In the maps shown, the original 750 m pixel-level AOD retrievals were mapped to 0.25×0.25∘ equal-angle latitude–longitude grids.
The new snow and snowmelt scheme was transferred to operation (TTO) in the Mx8.10 IDPS VIIRS aerosol algorithm on 22 June 2015 at 19:43 UTC. No significant snow or snowmelt contamination was found in the operational IDPS aerosol products during the 2016 spring thaw season, demonstrating the new scheme had improved the quality of the products with much better snow and snowmelt screening. The new snow and snowmelt screening scheme is also implemented in the EPS VIIRS aerosol algorithm, but with its own thresholds (Laszlo and Liu, 2016). As previously discussed, the threshold values of the NDSI and spatial filter were adjusted in the EPS VIIRS aerosol algorithm to regain heavy-smog retrievals while keeping the same level of snowmelt screening as in the Mx8.10 IDPS algorithm (Huang et al., 2017). Global assessment of the new tests in the EPS algorithm were conducted for the boreal spring season of 2015, which had significant snow and snowmelt contamination over Northern Hemisphere high-latitude regions (Fig. 7a). The VIIRS aerosol retrieval in the spring thaw season of 2015, produced from the EPS algorithm in Fig. 7b, was compared to the operational IDPS products during the same season in Fig. 7a. Note that May 2015 preceded the implementation of the new snow scheme, which began in June of that year. Thus Fig. 7a shows high AOD along the edge of the northern snow boundary, but Fig. 7b with the new scheme does not. The new boreal spring seasonal VIIRS AOD retrievals are much improved in terms of the significantly reduced amount of anomalously high AOD values, particularly over northern Canada and northern Russia. At the same time, Fig. 7b also highlights that the EPS aerosol products regained more AOD retrievals of smog events over eastern China during spring 2015, resulting in higher seasonal mean AOD there. This improvement results from the NDSI threshold adjustment. Other advantages to the EPS products – unrelated to the new snow mask, such as retrievals over the bright deserts – can also be seen in Fig. 7b.
Validation of the S-NPP VIIRS operational aerosol products revealed residual snow and snowmelt contaminations during the boreal spring thaw season over high-latitude geographic regions. To reduce such contamination, we proposed a snow and snowmelt screening scheme that combines a new NDSI- and BT-based snow test, a snow adjacency test and a spatial filter based on the standard deviation of reflectance at 412 nm. The pixels flagged as snow by the snow test will become “not produced”, while the “good” AOD retrievals adjacent to identified snow pixels or with higher spatial heterogeneity will be “degraded”. It is noteworthy that in the operational environment the cloud test should be conducted before the snow test because the snow detection requires clear-sky conditions. The snow test should be conducted before the snow adjacency test and the spatial filter because the latter tests need to know whether the central pixel is identified as snow or whether the retrieval is a good-quality one. The order of snow adjacency test and spatial filter, however, does not produce a difference in the aerosol retrieval. Since the spatial filter requires standard deviation calculation, which is more computationally expensive than the snow adjacency test, it is usually arranged as the last test of the snow and snowmelt scheme. The testing of the new scheme demonstrated significant improvements in the VIIRS aerosol retrievals with far fewer anomalously high AOD retrievals due to snow and snowmelt contamination.
A global testing on 18 May 2014, a typical day in the spring thaw season when snow and snowmelt prevail, showed that the new snow test screened out an additional 3.44 % “good”-quality VIIRS AOD retrievals, which were otherwise contaminated by snow and snowmelt, and the new snow adjacency test and the homogeneity test degraded 5.57 % and 0.26 % “good”-quality AOD retrievals to “degraded” quality, respectively. This percentage is expected to be lower in other seasons when AOD retrievals are expected to be less susceptible to snow and snowmelt contamination. In future work, in order to reach more quantitative statistics for a better understanding of the relative contributions from each test, more testing dates at different seasons are needed. The additional testing will not only help find seasonal variability of the tests but will also help identify any residual snow and snowmelt contaminations or any over-screened AOD retrievals, both of which are valuable for further algorithm improvement.
The new snow and snowmelt scheme was also able to screen out a significant number of the VIIRS–AERONET matchups that had anomalously high positive biases in the IDPS VIIRS aerosol products over Canada during the spring thaw season from 2013 to 2015. The new snow and snowmelt screening scheme was transferred to operation (TTO) in the operational IDPS VIIRS aerosol algorithm on 22 June 2015 at 19:43 UTC. No significant snow or snowmelt contamination was found in the operational IDPS aerosol products during the 2016 spring thaw season. The new scheme has also been implemented in the upcoming EPS aerosol algorithm, albeit with fine-tuned threshold values of NDSI and spatial filter tests, in order to regain some AOD retrievals over heavy-smog events and to maintain the snow and snowmelt screening at the same strict level as in the IDPS algorithm. The VIIRS AOD retrievals during spring 2015 produced by the EPS algorithm were much improved from the IDPS AOD product, in terms of the significantly reduced amount of anomalously high AOD values, particularly over northern Canada and northern Russia. The new EPS VIIRS aerosol algorithm became operational in July 2017.
All the operational IDPS VIIRS data used in this study are publicly accessible at the NOAA Comprehensive Large Array-data Stewardship System (CLASS, http://www.class.ngdc.noaa.gov/, last access: 1 October 2018).
The authors declare that they have no conflict of interest.
This study is supported by the NOAA JPSS Program Office (Mitchell D.
Goldberg, JPSS Program scientist, and Lihang Zhou, JPSS STAR Program
manager) under a grant to the Cooperative Institute for Climate and
Satellites – Maryland (CICS-Maryland; award no.: NA14NES4320003; title
of award: CICS cooperative agreement 2014–2019). The manuscript
contents are solely the opinions of the authors and do not constitute a
statement of policy, decision or position on behalf of NOAA or the U.S.
government. The EPS VIIRS aerosol
products used in this study are available from the VIIRS aerosol calibration
and validation team upon request
(http://www.star.nesdis.noaa.gov/smcd/emb/viirs_aerosol/index.php, last access: 1 October 2018). For any
questions related to the VIIRS aerosol dataset, please contact
Istvan.Laszlo@noaa.gov or Shobha.Kondragunta@noaa.gov.
Edited by: Alexander Kokhanovsky
Reviewed by: four anonymous referees
Aerosol ATBD: VIIRS Aerosol optical depth and Particle Size Parameter Algorithm Theoretical Basis Document (Revision B), available at: https://www.star.nesdis.noaa.gov/jpss/documents/ATBD/D0001-M01-S01-020_JPSS_ATBD_VIIRS-AOT-APSP_B.pdf, last access: 1 October 2018.
Aerosol OAD: VIIRS Aerosol Products (AOD, APSP & SM) Intermediate Product (IP)/Environmental Data Records (EDR) Software – OAD (Revision F), available at: http://npp.gsfc.nasa.gov/sciencedocs/2015-09/474-00073_OAD-VIIRS-Aerosols-IP-EDR_H.pdf, last access: 1 October 2018.
Al-Saadi, J., Szykman, J., Pierce, R. B., Kittaka, C., Neil, D., Chu, D. A.,Remer, L. A., Gumley, L., Prins, E., Weinstock, L., MacDonald, C., Wayland, R., Dimmick, F., and Fishman, J.: Improving national air quality forecasts with satellite aerosol observations, B. Am. Meteorol. Soc., 86, 1249–1261, https://doi.org/10.1175/BAMS-86-9-1249, 2005.
Doherty, S. J., Warren, S. G., Grenfell, T. C., Clarke, A. D., and Brandt, R. E.: Light-absorbing impurities in Arctic snow, Atmos. Chem. Phys., 10, 11647–11680, https://doi.org/10.5194/acp-10-11647-2010, 2010.
Gao, B. C.: NDWI – A normalized difference water index for remote sensing of vegetation liquid water from space, Remote Sens. Environ., 58, 257–266, https://doi.org/10.1016/S0034-4257(96)00067-3, 1996.
Gutman, G., Tarpley, D., Ignatov, A., and Olson, S.: The enhanced NOAA global land data set from the advanced very high resolution radiometer, B. Am. Meteorol. Soc., 76, 1141–1156, https://doi.org/10.1175/1520-0477(1995)076<1141:TENGLD>2.0.CO;2, 1995.
Hadley, O. L. and Kirchstetter, T. W.: Black-carbon reduction of snow albedo, Nat. Clim. Change, 2, 437–440, https://doi.org/10.1038/nclimate1433, 2012.
Holben, B. N., Eck, T. F., Slutsker, I., Tanré, D., Buis, J. P., Setzer, A., Vermote, E., Reagan, J. A., Kaufman, Y. J., Nakajima, T., Lavenu, F., Jankowiak, I., and Smirnov, A.: AERONET – A federated instrument network and data archive for aerosol characterization, Remote Sens. Environ., 66, 1–16, https://doi.org/10.1016/S0034-4257(98)00031-5, 1998.
Hori, M., Aoki, T., Tanikawa, T., Motoyoshi, H., Hachikubo, A., Sugiura, K., Yasunari, T., Eide, H., Storvold, R., Nakajima, Y., and Fumihiro, T.: In-situ measured spectral directional emissivity of snow and ice in the 8–14 µm atmospheric window, Remote Sens. Environ., 100, 486–502, https://doi.org/10.1016/j.rse.2005.11.001, 2006.
Huang, J., Kondragunta, S., Laszlo, I., Liu, H., Remer, L. A., Zhang, H., Superczynski, S., Ciren, P., Holben, B. N., and Petrenko, M.: Validation and expected error estimation of Suomi-NPP VIIRS aerosol optical depth and Ångström exponent with AERONET, J. Geophys. Res.-Atmos., 121, 7139–7160, https://doi.org/10.1002/2016JD024834, 2016.
Huang, J., Liu, H., Zhang, H., Kondragunta, S., Laszlo, I., Ciren, P., Remer, L. A., and Superczynski, S.: Detecting Air Pollution Events Over China Using Two Different Aerosol optical depth Products Derived from S-NPP VIIRS Observations, Abstract #303078, 13th Annual Symposium on New Generation Operational Environmental Satellite Systems, AMS Annual Meeting 2017, Seattle, 22–26 January 2017.
Jackson, J., Liu, H., Laszlo, I., Kondragunta, S., Remer, L. A., Huang, J., and Huang, H.: Suomi-NPP VIIRS aerosol algorithms and data products, J. Geophys. Res.-Atmos., 118, 12673–12689, https://doi.org/10.1002/2013JD020449, 2013.
Jethva, H., Torres, O., Remer, L., Redemann, J., Livingston, J., Dunagan, S., Shinozuka, Y., Kacenelenbogen, M., Rosenheimer, M. S., and Spurr, R.: Validating MODIS above-cloud aerosol optical depth retrieved from “color ratio” algorithm using direct measurements made by NASA's airborne AATS and 4STAR sensors, Atmos. Meas. Tech., 9, 5053–5062, https://doi.org/10.5194/amt-9-5053-2016, 2016.
Kaufman, Y. J., Tanre, D., Remer, L. A., Vermote, E. F., Chu, A., and Holben, B. N.: Operational remote sensing of tropospheric aerosol over land from EOS moderate resolution imaging spectroradiometer, J. Geophys. Res.-Atmos., 102, 17051–17067, https://doi.org/10.1029/96jd03988, 1997.
Kaufman, Y. J., Tanré, D., and Boucher, O.: A satellite view of aerosols in the climate system, Nature, 419, 215–23, https://doi.org/10.1038/nature01091, 2002.
Key, J. R., Mahoney, R., Liu, Y., Romanov, P., Tschudi, M., Appel, I., Maslanik, J., Baldwin, D., Wang, X., and Meade, P.: Snow and ice products from Suomi NPP VIIRS, J. Geophys. Res.-Atmos., 118, 12816–12830, https://doi.org/10.1002/2013JD020459, 2013.
Kloog, I., Koutrakis, P., Coull, B. A., Lee, H. J., and Schwartz, J.: Assessing temporally and spatially resolved PM2.5 exposures for epidemiological studies using satellite aerosol optical depth measurements, Atmos.. Environ., 45, 6267–6275, https://doi.org/10.1016/j.atmosenv.2011.08.066, 2011.
Laszlo, I. and Liu, H.: EPS Aerosol Optical Depth (AOD) Algorithm Theoretical Basis Document, Version 3.0.1, available at: https://www.star.nesdis.noaa.gov/jpss/documents/ATBD/ATBD_EPS_Aerosol_AOD_v3.0.1.pdf (1 October 2018), 2016.
Lenoble, J., Remer, L. A., and Tanré, D. (Eds.): Aerosol Remote Sensing, Springer Praxis Books, Berlin, Heidelberg, https://doi.org/10.1007/978-3-642-17725-5_1, 2013.
Levy, R. C., Remer, L. A., Mattoo, S. , Vermote, E. F., and Kaufman, Y. J.: Second-generation operational algorithm: Retrieval of aerosol properties over land from inversion of Moderate Resolution Imaging Spectroradiometer spectral reflectance, J. Geophys. Res., 112, D13211, https://doi.org/10.1029/2006JD007811, 2007.
Levy, R. C., Mattoo, S., Munchak, L. A., Remer, L. A., Sayer, A. M., Patadia, F., and Hsu, N. C.: The Collection 6 MODIS aerosol products over land and ocean, Atmos. Meas. Tech., 6, 2989–3034, https://doi.org/10.5194/amt-6-2989-2013, 2013.
Li, R. R, Remer, L., Kaufman, Y. J., Mattoo, S., Gao, B. C., and Vermote, E.: Snow and ice mask for the MODIS aerosol products, IEEE Geosci. Remote S., 2, 306–310, https://doi.org/10.1109/LGRS.2005.847755, 2005
Li, Z.-L., Wu , H., Wang, N., Qiu, S., Sobrino, J. A., Wan, Z., Tang, B., and Yan, G.: Land surface emissivity retrieval from satellite data, Int. J. Remote Sens., 34, 3084–3127, https://doi.org/10.1080/01431161.2012.716540, 2013.
Liu, H., Remer, L. A., Huang, J., Huang, H., Kondragunta, S., Laszlo, I., Oo, M., and Jackson, J.: Preliminary evaluation of S-NPP VIIRS aerosol optical depth, J. Geophys. Res.-Atmos., 119, 3942–3962, https://doi.org/10.1002/2013JD020360, 2014.
Lyapustin, A., Wang, Y., Laszlo, I., and Korkin, S.: Improved cloud and snow screening in MAIAC aerosol retrievals using spectral and spatial analysis, Atmos. Meas. Tech., 5, 843–850, https://doi.org/10.5194/amt-5-843-2012, 2012.
Meyer, K., Platnick, S., and Zhang, Z.: Simultaneously inferring above-cloud absorbing aerosol optical depth and underlying liquid phase cloud optical and microphysical properties using MODIS, J. Geophys. Res.-Atmos., 120, 5524–5547, https://doi.org/10.1002/2015JD023128, 2015.
Quaas, J., Boucher, O., Bellouin, N., and Kinne, S.: Satellite-based estimate of the direct and indirect aerosol climate forcing, J. Geophys. Res.-Atmos., 113, D05204, https://doi.org/10.1029/2007JD008962, 2008.
Remer, L. A., Kaufman, Y. J., Tanré, D., Mattoo, S., Chu, D. A., Martins, J. V., Li, R. R., Ichoku, C., Levy, R. C., Kleidman, R. G., Eck, T. F., Vermote, E., and Holben, B. N.: The MODIS aerosol algorithm, products, and validation, J. Atmos. Sci., 62, 947–973, https://doi.org/10.1175/JAS3385.1, 2005.
Sayer, A. M., Hsu, N. C., Bettenhausen, C., Lee, J., Redemann, J., Schmid, B., and Shinozuka, Y.: Extending “Deep Blue” aerosol retrieval coverage to cases of abC4 AMTD Interactive comment Printer-friendly version Discussion paper sorbing aerosols above clouds: Sensitivity analysis and first case studies, J. Geophys. Res.-Atmos., 121, 4830–4854, https://doi.org/10.1002/2015JD024729, 2016.
Shi, Y., Zhang, J., Reid, J. S., Liu, B., and Hyer, E. J.: Critical evaluation of cloud contamination in the MISR aerosol products using MODIS cloud mask products, Atmos. Meas. Tech., 7, 1791–1801, https://doi.org/10.5194/amt-7-1791-2014, 2014.
Van Donkelaar, A., Martin, R. V., Brauer, M., Kahn, R., Levy, R. C., Verduzco, C., and Villeneuve, P. J.: Global estimates of ambient fine particulate matter concentrations from satellite-based aerosol optical depth: development and application, Environ. Health Persp., 118, 847, https://doi.org/10.1289/ehp.0901623, 2010.
VIIRS Cloud Mask ATBD, available at: http://www.star.nesdis.noaa.gov/jpss/documents/ATBD/D0001-M01-S01-011_JPSS_ATBD_VIIRS-Cloud-Mask_E.pdf, last access: 1 October 2018.
Walter-Shea, E., Privette, J. L., Cornell, D., Mesarch, M. A., and Hays, C.: Relations between Directional Spectral Vegetation Indices and Leaf Area and Absorbed Radiation in Alfalfa, Remote Sens. Environ., 61, 162–177, https://doi.org/10.1016/S0034-4257(96)00250-7, 1997.
Walton, C. C., Pichel, W. G., Sapper, J. F., and May, D. A.: The development and operational application of nonlinear algorithms for the measurement of sea surface temperatures with the NOAA polar-orbiting environmental satellites, J. Geophys. Res., 103, 27999–28012, https://doi.org/10.1029/98JC02370, 1998.
Zhang, H., Kondragunta, S., Laszlo, I., Liu, H., Remer, L. A., Huang, J., Superczynski, S., and Ciren, P.: An enhanced VIIRS aerosol optical depth (AOD) retrieval algorithm over land using a global surface reflectance ratio database, J. Geophys. Res.-Atmos., 121, 10717–10738, https://doi.org/10.1002/2016JD024859, 2016.