the Creative Commons Attribution 4.0 License.
the Creative Commons Attribution 4.0 License.
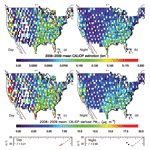
A bulk-mass-modeling-based method for retrieving particulate matter pollution using CALIOP observations
Travis D. Toth
Jeffrey S. Reid
Mark A. Vaughan
In this proof-of-concept paper, we apply a bulk-mass-modeling method using observations from the NASA Cloud-Aerosol Lidar with Orthogonal Polarization (CALIOP) instrument for retrieving particulate matter (PM) concentration over the contiguous United States (CONUS) over a 2-year period (2008–2009). Different from previous approaches that rely on empirical relationships between aerosol optical depth (AOD) and PM2.5 (PM with particle diameters less than 2.5 µm), for the first time, we derive PM2.5 concentrations, during both daytime and nighttime, from near-surface CALIOP aerosol extinction retrievals using bulk mass extinction coefficients and model-based hygroscopicity. Preliminary results from this 2-year study conducted over the CONUS show a good agreement (r2∼0.48; mean bias of −3.3 µg m−3) between the averaged nighttime CALIOP-derived PM2.5 and ground-based PM2.5 (with a lower r2 of ∼0.21 for daytime; mean bias of −0.4 µg m−3), suggesting that PM concentrations can be obtained from active-based spaceborne observations with reasonable accuracy. Results from sensitivity studies suggest that accurate aerosol typing is needed for applying CALIOP measurements for PM2.5 studies. Lastly, the e-folding correlation length for surface PM2.5 is found to be around 600 km for the entire CONUS (∼300 km for western CONUS and ∼700 km for eastern CONUS), indicating that CALIOP observations, although sparse in spatial coverage, may still be applicable for PM2.5 studies.
Please read the corrigendum first before continuing.
-
Notice on corrigendum
The requested paper has a corresponding corrigendum published. Please read the corrigendum first before downloading the article.
-
Article
(7700 KB)
-
The requested paper has a corresponding corrigendum published. Please read the corrigendum first before downloading the article.
- Article
(7700 KB) - Full-text XML
- Corrigendum
- BibTeX
- EndNote
During the last decade, an extensive number of studies have researched the feasibility of estimating PM2.5 (particulate matter with particle diameters smaller than 2.5 µm) pollution with the use of passive satellite-derived aerosol optical depth (AOD; e.g., Liu et al., 2007; Hoff and Christopher, 2009; van Donkelaar et al., 2015). Monitoring of PM concentration from space observations is needed, as PM2.5 pollution is one of the known causes of respiratory-related diseases as well as other health-related issues (e.g., Liu et al., 2005; Hoff and Christopher, 2009; Silva et al., 2013). Yet, ground-based PM2.5 measurements are often inconsistent or have limited availability over much of the globe.
In some earlier studies, empirical relationships of PM2.5 concentrations and AODs were developed and used for estimating PM2.5 concentrations from passive-sensor-retrieved AODs (e.g., Wang and Christopher, 2003; Engel-Cox et al., 2004; Liu et al., 2005; Kumar et al., 2007; Hoff and Christopher, 2009). One of the limitations of this approach is that vertical distributions and the thermodynamic state of aerosol particles vary with space and time. Especially for regions with elevated aerosol plumes, deep boundary layer entrainment zones, or strong nighttime inversions, column-integrated AODs are not a good approximation of surface PM2.5 concentrations at specific points and times (e.g., Liu et al., 2004; Toth et al., 2014; Reid et al., 2017). Indeed, Kaku et al. (2018) recently showed that surface PM2.5 had longer spatial correlation lengths than AOD, even in the “well behaved” southeastern United States where previous studies showed good correlation between PM2.5 and AOD (e.g., Wang and Christopher, 2003). To account for variability in aerosol vertical distribution, several studies have attempted the use of chemical transport models, or CTMs (e.g., van Donkelaar et al., 2015). Satellite data assimilation of AOD has become commonplace, vastly improving AOD analyses and short-term prediction (e.g., Zhang et al., 2014; Sessions et al., 2015). Yet, PM2.5 simulations remain poor (e.g., Reid et al., 2016). Uncertainties in such studies are unavoidable due to uncertainties in CTM-based aerosol vertical distributions, and no nighttime AODs are currently available from passive satellite retrievals.
It is arguable that from a climatological and long-term average perspective, the use of AOD as a proxy for PM2.5 concentrations nevertheless has certain qualitative skill (e.g., Toth et al., 2014; Reid et al., 2017) due to the averaging process that suppresses sporadic aerosol events with highly variable vertical distributions. Still, as illustrated in Fig. 1, in which 2-year (2008–2009) means of Moderate Resolution Imaging Spectroradiometer (MODIS) AOD are plotted against PM2.5 concentrations throughout the contiguous United States (CONUS), although a linear relationship is plausibly shown, a low r2 value of 0.08 is found. To construct Fig. 1, Aqua MODIS Collection 6 (C6) Optical_Depth_Land_And_Ocean data (0.55 µm), restricted to “very good” retrievals as reported by the Land_Ocean_Quality_Flag, are first collocated with daily surface PM2.5 measurements in both space and time (i.e., within 40 km in distance and the same day) and then collocated daily pairs are averaged into 2-year means (for each PM2.5 site). Figure 1 may indicate that even from a long-term mean perspective, aerosol vertical distributions are not uniform across the CONUS, which is also confirmed by other studies (e.g., Toth et al., 2014). AOD retrievals themselves, with known uncertainties due to cloud contamination and assumptions in the retrieval process (e.g., Levy et al., 2013), may also introduce uncertainties to that task.
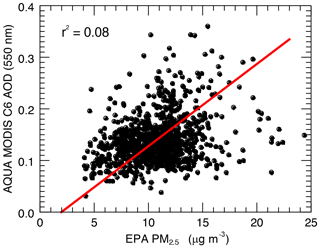
Figure 1For 2008–2009, scatter plot of mean PM2.5 concentration from ground-based U.S. EPA stations and mean column AOD (550 nm) from collocated Collection 6 (C6) Aqua MODIS observations. The red line represents the Deming regression fit.
On board the Cloud-Aerosol Lidar and Infrared Pathfinder Satellite Observations (CALIPSO) satellite, the Cloud-Aerosol Lidar with Orthogonal Polarization (CALIOP) instrument provides observations of aerosol and cloud vertical distributions during both day and night (Hunt et al., 2009; Winker et al., 2010). Given that CALIOP provides aerosol extinction retrievals near the ground, it is interesting and reasonable to raise the following question: can near-surface CALIPSO extinction be used as a better physical quantity than AOD for estimating surface PM2.5 concentrations? This is because unlike AOD, which is a column-integrated value, near-surface CALIPSO extinction is, in theory, a more realistic representation of near-surface aerosol properties. Yet, in comparing with passive sensors such as MODIS, which has a swath width on the order of ∼2000 km, CALIOP is a nadir-pointing instrument with a narrow swath of ∼70 m and a repeat cycle of 16 days (Winker et al., 2009). Thus, the spatial sampling of CALIOP is sparse on a daily basis and temporal sampling or other conditional or contextual biases are unavoidable if CALIOP observations are used to estimate daily PM2.5 concentrations (Zhang and Reid, 2009; Colarco et al., 2014). Also, there are known uncertainties in CALIPSO-retrieved extinction values due to uncertainties in the retrieval process, such as the lidar ratio (extinction-to-backscatter ratio), calibration, and the “retrieval fill value” (RFV) issue (Young et al., 2013; Toth et al., 2018).
Even with these known issues, especially the sampling bias, it is still compelling to investigate if near-surface CALIOP extinction can be utilized to retrieve surface PM2.5 concentrations with reasonable accuracy from a long-term (i.e., 2-year) mean perspective. CALIOP data have been successfully used in PM2.5 studies in the past but primarily for assisting passive AOD and PM2.5 analyses using aerosol vertical distribution as a constraint (e.g., Glantz et al., 2009; van Donkelaar et al., 2010; Val Martin et al., 2013; Toth et al., 2014; Li et al., 2015; Gong et al., 2017). However, the question of the efficacy of the direct use of CALIOP retrievals remained. To demonstrate a concept, we developed a bulk mass scattering scheme for inferring PM concentrations from near-surface aerosol extinction retrievals derived from CALIOP observations. The bulk method used here is based upon the well-established relationship between particle light scattering and PM2.5 aerosol mass concentration (e.g., Charlson et al., 1968; Waggoner and Weiss, 1980; Liou, 2002; Chow et al., 2006), discussed further, with the relevant equations, in Sect. 2.
In this study, using 2 years (2008–2009) of CALIOP and United States (U.S.) Environmental Protection Agency (EPA) data over the CONUS, the following questions are addressed:
-
Can CALIOP extinction be used effectively for estimating PM2.5 concentrations through a bulk mass scattering scheme from a 2-year mean perspective for both daytime and nighttime?
-
Can CALIOP extinction be used as a better parameter than AOD for estimating PM2.5 concentrations from a 2-year mean perspective?
-
What sampling biases can be expected in CALIOP estimates of PM2.5?
-
How do uncertainties in bulk properties compare to overall CALIOP-retrieved PM2.5 uncertainty?
Details of the methods and datasets used are described in Sect. 2. Section 3 shows the preliminary results using 2 years of EPA PM2.5 and CALIOP data, including an uncertainty analysis. The conclusions of this paper are provided in Sect. 4.
Since 1970, the U.S. EPA has monitored surface PM using a number of Federal Reference/Equivalent Methods (FRMs/FEMs), which employ gravimetric, tapered element oscillating microbalance (TEOM), and beta gauge instruments (Federal Register, 1997; Greenstone, 2002). A total of 2 years (2008–2009) of daily PM2.5 local conditions (EPA code = 88101) data were acquired from the EPA Air Quality System for use in this investigation, consistent with our previous PM2.5 study (Toth et al., 2014). These data represent PM2.5 concentrations over a 24 h period and include two scenarios: one sample is taken during the 24 h duration (i.e., filter-based measurement) or an average is computed from hourly samples within this time period (every hour may not have an available measurement, however).
Note that uncertainties have been reported for hourly PM measurements (Kiss et al., 2017). Examples of some uncertainties in these PM2.5 measurements depend upon the instrument/method used: gravimetric (e.g., transport to the lab/human error and volatization of PM during the drying process; Patashnick et al., 2001), TEOM (e.g., errors due to improper inlet tube temperature; Eatough et al., 2003), and beta attenuation monitors (e.g., changes in the sample flow rate due to variations in temperature and moisture; Spagnolo, 1989). Also, it has been found that beta attenuation monitors may be more accurate than TEOM, as TEOM can underestimate PM2.5 at low temperatures (e.g., Chung et al., 2001). Still, as suggested by Kiss et al. (2017), PM data collected over a longer period of time are much less likely to be biased. Thus, we expect lower uncertainties from data collected over 24 h than from daily data generated by averaging hourly observations. Fully quantifying the differences from the two different PM observing methods, however, is the subject for a future study.
CALIOP, flying aboard the CALIPSO platform within the A-Train satellite constellation, is a dual-wavelength (0.532 and 1.064 µm) lidar that has collected profiles of atmospheric aerosol particles and clouds since summer 2006 (Winker et al., 2007). In this study, daytime and nighttime extinction coefficients retrieved at 0.532 µm from the version 4.10 CALIOP Level 2 5 km aerosol profile (L2_05kmAPro) product were used. Using parameters provided in the L2_05kmAPro product, as well as the corresponding Level 2 5 km aerosol layer (L2_05kmALay) product, a robust quality-assurance (QA) procedure for the aerosol observations was implemented (Table 1). Further information on the QA metrics and screening protocol are discussed in detail in previous studies (Kittaka et al., 2011; Campbell et al., 2012; Toth et al., 2013, 2016). Once the QA procedure was applied, the aerosol profiles were linearly re-gridded from 60 m vertical resolution (above mean sea level, a.m.s.l.) to 100 m segments (i.e., resampled to 100 m resolution) referenced to the local surface (above ground level, a.g.l.; Toth et al., 2014, 2016). The choice of 100 m was arbitrary, and the profiles were re-gridded in order to obtain a dataset corrected for a.g.l., as opposed to the a.m.s.l.-referenced profiles provided by the L2_05kmAPro product. Surface elevation and relative humidity (RH) were taken from collocated model data included in the CALIPSO L2_05kmAPro product (Vaughan et al., 2018; RH was taken from the Modern Era Retrospective-Analysis for Research, or MERRA-2 reanalysis product). To limit the effects of signal attenuation and increase the chances of measuring aerosol presence near the surface, the atmospheric volume description parameter within the L2_05kmAPro dataset is used to cloud-screen each aerosol profile as in Toth et al. (2018).
Table 1The parameters, and corresponding values, used to assure the quality of the CALIOP aerosol extinction profile.
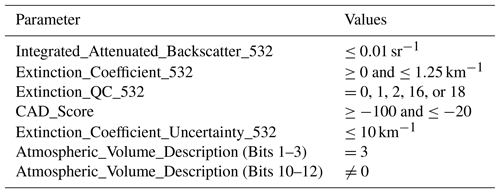
In this study, near-surface PM mass concentration (Cm) is derived from near-surface CALIOP extinction based on a bulk formulation as in Eq. (1) (e.g., Liou, 2002; Chow et al., 2006):
where β is CALIOP-derived near-surface extinction per kilometer, Cm is the PM mass concentration in micrograms per cubic meter, ascat and aabs are dry mass scattering and absorption efficiencies in square meters per gram, and frh represents the light scattering hygroscopicity. As a preliminary study, for the purpose of demonstrating this concept, we assume the dominant aerosol type over the CONUS is pollution aerosol (i.e., the most prevalent near-surface aerosol type reported in the CALIOP products for the CONUS during 2008–2009 is polluted continental) with ascat and aabs values of 3.40 and 0.37 m2 g−1 (Hess et al., 1998; Lynch et al., 2016), respectively. These values are similar to those reported in Hand and Malm (2007) and Kaku et al. (2018) but are interpolated to 0.532 µm from values at 0.450 and 0.550 µm obtained from the Optical Properties of Aerosols and Clouds (OPAC) model (Hess et al., 1998). Still, both ascat and aabs have regional and species-related dependencies. Also, only 2-year averages are used in this study, and we assume that sporadic aerosol plumes are smoothed out in the averaging process and that bulk aerosol properties are similar throughout the study region. We have further explored the impact of aerosol types on PM2.5 retrievals in a later section. Furthermore, to aid in focusing this study on fine-mode and anthropogenic aerosols, those aerosol extinction range bins classified as dust by the CALIOP typing algorithm were excluded from the analysis.
Also, surface PM concentrations are dry mass measurements. To account for the impact of humidity on ascat (it is assumed that aabs is not affected by moisture; Nessler et al., 2005; Lynch et al., 2016), we estimated the hygroscopic growth factor for pollution aerosol based on Hänel (1976), as shown in Eq. (2):
where frh is the hygroscopic growth factor, RH is the relative humidity, and RHref is the reference RH and is set to 30 % in this study (Lynch et al., 2016). Γ is a unitless value (a fit parameter describing the amount of hygroscopic increase in scattering) and is assumed to be 0.63 (i.e., sulfate aerosol) in this study (Hänel, 1976; Chew et al., 2016; Lynch et al., 2016).
Additionally, the CALIOP-derived PM density is for all particle sizes. To convert from mass concentration of PM (Cm) to mass concentration of PM2.5 (Cm2.5), which represents mass concentration for particle diameters smaller than 2.5 µm, we adopted the PM2.5-to-PM10 (PM with diameters less than 10 µm) ratio (φ) of 0.6 as measured during the Studies of Emissions and Atmospheric Composition, Clouds and Climate Coupling by Regional Surveys (SEAC4RS) campaign over the US (Kaku et al., 2018). Again, the ratio of PM2.5 to PM10 can also vary spatially; however we used a regional mean to demonstrate the concept. Analyses in a later section using 2 years (2008–2009) of surface PM2.5 to PM10 data suggest that 0.6 is a rather reasonable number to use for the CONUS for the study period. Here we assume that mass concentrations for particle diameters larger than 10 µm are negligible over the CONUS. Thus, we can rewrite Eq. (1) as
where Cm2.5 is the CALIOP-derived PM2.5 concentration in units of micrograms per cubic meter.
Lastly, we note that most of the results are shown in the form of scatter plots with fits from a Deming regression (Deming, 1943). Due to uncertainties in PM2.5 data, we show slopes computed from Deming regression analyses instead of those from simple linear regression. Deming regression in particular is appropriate here, as it accounts for errors in both the independent and dependent variables (Deming, 1943) and has been used in past PM2.5-related studies (e.g., Huang et al., 2014).
3.1 Regional analysis
Figure 2a shows the mean PM2.5 concentration using 2 years (2008–2009) of daily surface PM2.5 data from the U.S. EPA (PM2.5_EPA), not collocated with CALIOP observations. A total of 1091 stations (some operational throughout the entire period; others only partially) are included in the analysis, and observations from those stations are further used in evaluating CALIOP-derived PM2.5 concentrations (Cm2.5), as later shown in Fig. 3. PM2.5 concentrations of ∼10 µg m−3 are found over the eastern CONUS. In comparison, much lower PM2.5 concentrations of ∼5 µg m−3 are exhibited for the interior CONUS, over states including Montana, Wyoming, North Dakota, South Dakota, Utah, Colorado, and Arizona. For the west coast of the CONUS, and especially over California, higher PM2.5 concentrations are observed, with the maximum 2-year mean near 20 µg m−3. Note that the spatial distribution of surface PM2.5 concentrations over the CONUS as shown in Fig. 2a is consistent with reported values from several studies (e.g., Hand et al., 2013; Van Donkelaar et al., 2015; Di et al., 2017).
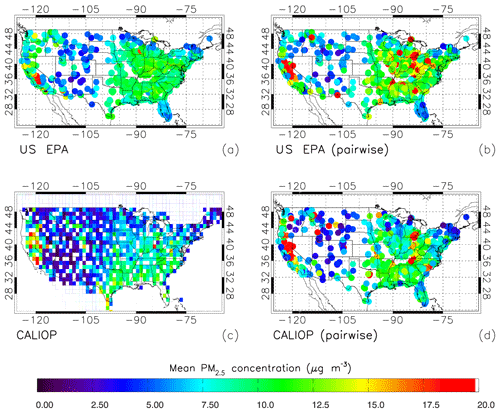
Figure 2For 2008–2009 over the CONUS, (a) mean PM2.5 concentration (µg m−3) for those U.S. EPA stations with reported daily measurements, and (c) 1∘ × 1∘ average CALIOP-derived PM2.5 concentrations for the 100–1000 m a.g.l. atmospheric layer, using Eq. (3), for combined daytime and nighttime conditions. Also shown are the pairwise PM2.5 concentrations from (b) EPA daily measurements and (d) those derived from CALIOP (day and night combined), both averaged for each EPA station for the 2008–2009 period. For all four plots, values greater than 20 µg m−3 are colored red.
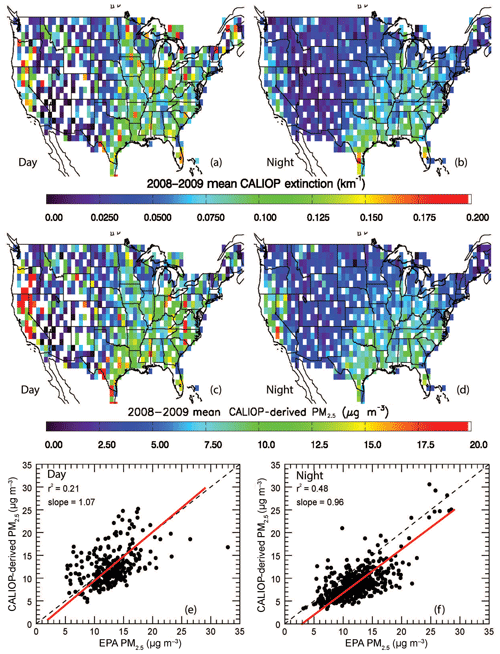
Figure 3For 2008–2009 over the CONUS, 1∘ × 1∘ average CALIOP extinction, relative to the number of cloud-free 5 km CALIOP profiles in each 1∘ × 1∘ bin, for the 100–1000 m a.g.l. atmospheric layer, for (a) daytime and (b) nighttime measurements. Also shown are the corresponding CALIOP-derived PM2.5 concentrations, using Eq. (3) for (c) daytime and (d) nighttime conditions. Values greater than 0.2 km−1 and 20 µg m−3 for (a, b) and (c, d), respectively, are colored red. Scatter plots of mean PM2.5 concentration from ground-based U.S. EPA stations and those derived from collocated near-surface CALIOP observations are shown in the bottom row, using (e) daytime and (f) nighttime CALIOP data. The red lines represent the Deming regression fits.
Figure 3a shows the 2-year averaged 1∘ × 1∘ (latitude and longitude) gridded daytime CALIOP aerosol extinction over the CONUS using CALIOP observations from 100 to 1000 m, referenced to the number of cloud-free L2_05kmAPro profiles in each 1×1∘ bin. The lowest 100 m of CALIOP extinction data are not used in the analysis due to the potential of surface return contamination (e.g., Toth et al., 2014), although this has been improved for the version 4 CALIOP products but may still be present in some cases. Here the averaged extinction from 100–1000 m is used to represent near-surface aerosol extinction. This selection of the 100–1000 m layer is somewhat arbitrary, even though it is estimated from the mean CALIOP-based aerosol vertical distribution over the CONUS (Toth et al., 2014). Thus, a sensitivity study is provided in a later section to understand the impact of this aerosol layer selection on CALIOP-based PM2.5 retrievals. As shown in Fig. 3a, a higher mean near-surface CALIOP extinction of 0.1 km−1 is found for the eastern CONUS and over California, while lower values of 0.025–0.05 km−1 are found for the interior CONUS. Figure 3b shows a plot similar to Fig. 3a but using nighttime CALIOP observations only. Although similar spatial patterns are found during both day and night, the near-surface extinction values are overall lower for nighttime than daytime, and nighttime data are less noisy than daytime. These findings are not surprising, as daytime CALIOP measurements are subject to contamination from background solar radiation (e.g., Omar et al., 2013).
To investigate any diurnal biases in the data, Fig. 3c and d show the derived PM2.5 concentration using daytime and nighttime CALIOP data, respectively, based on the method described in Sect. 2. Both Fig. 3c and d suggest a higher PM2.5 concentration of ∼10–12.5 µg m−3 over the eastern CONUS and a much lower PM2.5 concentration of ∼2.5–5 µg m−3 over the interior CONUS. High PM2.5 values of 10–20 µg m−3 are also found over the west coast of the CONUS, particularly over California. The spatial distribution of PM2.5 concentrations, as derived using near-surface CALIOP data (Fig. 3c and d, as well as the combined daytime and nighttime perspective shown in Fig. 2c), is remarkably similar to the spatial distribution of PM2.5 values as estimated based on ground-based observations (Fig. 2a). Still, day and night differences in PM2.5 concentrations are also clearly visible, as higher PM2.5 values are found, in general, during daytime, based on CALIOP observations. The high daytime PM2.5 values, as shown in Fig. 3c, may represent stronger near-surface convection and more frequent anthropogenic activities during daytime. However, they may also be partially contributed by solar radiation contamination. Another possibility is that the daytime mean extinction coefficients (from which the mean PM2.5 estimates are derived) appear artificially larger than at night due to high daytime noise limiting the ability of CALIOP to detect fainter aerosol layers during daylight operations. Note that, for context, maps of the number of days and CALIOP Level 2 5 km aerosol profiles used in the creation of Fig. 3a–d are shown in Appendix Fig. A1.
Figure 3e shows the intercomparison between PM2.5_EPA and PM2.5_CALIOP concentrations. Note that only CALIOP and ground-based PM2.5 data pairs, which are within 100 km of each other and have reported values for the same day (i.e., year, month, and day), are used to generate Fig. 3e. Still, although only spatially and temporally collocated data pairs are used, ground-based PM2.5 data represent 24 h averages, while CALIOP-derived PM2.5 concentrations are instantaneous values over the daytime CALIOP overpass. To reduce this temporal bias, 2 years (2008–2009) of collocated CALIOP-derived and measured PM2.5 concentrations are averaged and only the 2-year averages are used in constructing Fig. 3e. Also, to minimize the abovementioned temporal sampling bias, ground stations with fewer than 100 collocated pairs are discarded. This leaves a total of 276 stations for constructing Fig. 3e.
As shown in Fig. 3e, an r2 value of 0.21 (with slope of 1.07) is found between CALIOP-derived and measured surface PM2.5 concentrations, with a corresponding mean bias of −0.40 µg m−3 (PM2.5_CALIOP−PM2.5_EPA). In comparison, Fig. 3f shows results similar to Fig. 3e, but for nighttime CALIOP data. A much higher r2 value of 0.48 (with slope of 0.96) is found between CALIOP-derived and measured PM2.5 values from 528 EPA stations, with a corresponding mean bias of −3.3 µg m−3 (PM2.5_CALIOP−PM2.5_EPA). This may be related to the diurnal variability of PM2.5 concentrations, as the daily mean EPA measurement might be closer to the CALIOP morning retrieval than to its afternoon counterpart. Still, data points are more scattered in Fig. 3e in comparison with Fig. 3f, which again indicates that daytime CALIOP data are noisier, possibly due to daytime solar contamination as well as other factors such as biases in relative humidity. Details of these biases are further explored in Sect. 3.2.
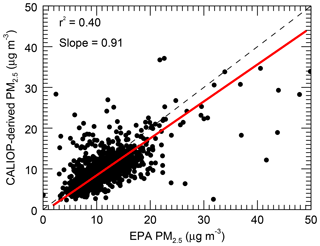
Figure 4Scatter plot of mean PM2.5 concentration from ground-based U.S. EPA stations and those derived from collocated near-surface CALIOP observations using combined daytime and nighttime CALIOP data. The red line represents the Deming regression fit.
To supplement this analysis, a pairwise PM2.5_EPA and PM2.5_CALIOP (day and night CALIOP combined) analysis is presented in the spatial plots of Fig. 2b and d. Here, however, we lift the requirement of 100 collocated pairs to increase data samples for better spatial representativeness. The spatial variability of PM2.5 over the CONUS is consistent with the observed patterns of non-collocated data (i.e., Fig. 2a and c), but with generally higher values due to differences in sampling. Also, comparing Fig. 2b and d, PM2.5_EPA spatial patterns match well with those of PM2.5_CALIOP, yet with larger values for PM2.5_EPA (consistent with the biases discussed above). Lastly, a scatter plot of the pairwise analysis shown in Fig. 2b and d is provided in Fig. 4. An r2 value of 0.40 is found between EPA and CALIOP-derived PM2.5 concentrations from a combined daytime and nighttime CALIOP perspective. Overall, Figs. 2, 3, and 4 indicate that near-surface CALIOP extinction data can be used to estimate surface PM2.5 concentrations with reasonable accuracy.
3.2 Uncertainty analysis
In this section, uncertainties in the CALIOP-derived 2-year averaged PM2.5 concentrations are explored as functions of aerosol vertical distribution, PM2.5-to-PM10 ratio, RH, aerosol type, and cloud presence above. Spatial-sampling-related biases as well as prognostic errors are also studied.
3.2.1 Prognostic errors in Cm2.5
As a first step for the uncertainty analysis, we estimated the prognostic error of 2-year averaged PM2.5_CALIOP. Figure 5 shows the root-mean-square error (RMSE) of CALIOP-based PM2.5 concentrations against those from EPA stations as a function of CALIOP-based PM2.5 for the 2008–2009 period over the CONUS. RMSEs were computed for five equally sampled bins, determined from a cumulative histogram analysis. Each point in Fig. 5, from left to right, represents the RMSE and mean PM2.5 concentration derived from CALIOP for 0 %–20 %, 20 %–40 %, 40 %–60 %, 60 %–80 %, and 80 %–100 % cumulative frequencies. A mean combined daytime and nighttime RMSE of ∼4 µg m−3 is found, with a mean value slightly greater for nighttime (∼ 4.3 µg m−3) than daytime (∼3.7 µg m−3). While most bins exhibit larger nighttime RMSEs, daytime RMSEs are larger for the greatest mean CALIOP-derived PM2.5 concentrations.
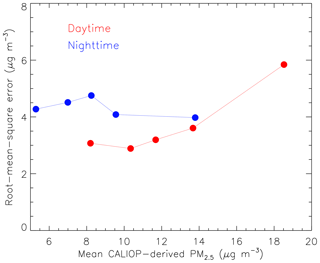
Figure 5Root-mean-square errors of CALIOP-derived PM2.5 against EPA PM2.5 as a function of CALIOP-derived PM2.5, using both daytime (in red) and nighttime (in blue) CALIOP observations. The five bins are equally sampled based upon a cumulative histogram analysis, and each point from left to right represents the RMSE and mean PM2.5 concentration derived from CALIOP for 0 %–20 %, 20 %–40 %, 40 %–60 %, 60 %–80 %, and 80 %–100 % cumulative frequencies.
Table 2Statistical summary of a sensitivity analysis varying the height of the surface layer, including R2, slope from Deming regression, mean bias (CALIOP−EPA) of PM2.5 in micrograms per cubic meter, and percent error change in derived PM2.5, defined as . The row in bold represents the results shown in the remainder of the paper.
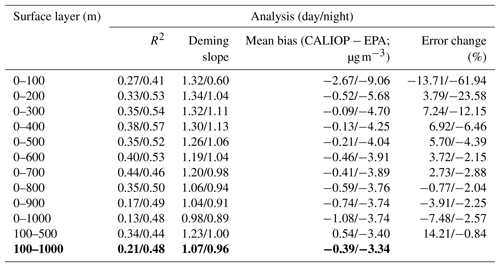
3.2.2 Surface layer height sensitivity study
A sensitivity study was conducted for which PM2.5 was derived from near-surface CALIOP aerosol extinction by varying the height of the surface layer in increments of 100 m from the ground to 1000 m. Note that the surface layer (0–100 m) is included for this sensitivity study only. The statistical results of this analysis, for both daytime and nighttime conditions, are shown in Table 2. Four statistical parameters were computed, consisting of r2, slope from Deming regression, mean bias (CALIOP−EPA) of PM2.5, and percent error change in derived PM2.5, defined as . For context, the bottom row of Table 2 shows the results from the original analysis. In terms of r2 and slope, optimal values peak at different surface layer heights between daytime and nighttime. For example, for daytime, the largest correlations are found for the 0–600 and 0–700 m layers, while for nighttime these are found for the 0–300 and 0–400 m layers. However, the 0–300 m layer exhibits the lowest mean bias for the daytime analysis, and the 100–1000 m layer exhibits the lowest mean bias for the nighttime analysis. Overall, marginal changes are found for varying the height of the surface layer. Yet the largest mean bias is found for the 0–100 m layer, indicating the need for excluding the 0–100 m layer in the analysis.
3.2.3 RH sensitivity study
Profiles of RH were taken from the MERRA-2 reanalysis product, as these collocated data are provided in the CALIPSO L2_05kmAPro product. However, biases may exist in this RH dataset. Thus, we examined the impact of varying the RH values by ±10 % on the CALIOP-derived PM2.5 concentrations. For both daytime and nighttime analyses, no significant differences in the r2 and slope values were found. However, a +15 % change in the mean derived PM2.5 values was found by decreasing the RH values by 10 %, while a −15 % change in the mean derived PM2.5 values was found by increasing the RH values by 10 %.
3.2.4 PM2.5-to-PM10 ratio sensitivity study
Another source of uncertainty in this study is the PM2.5∕PM10 ratio. Using surface-based PM2.5 and PM10 data from those EPA stations over the CONUS for 2008–2009 with concurrent PM2.5 and PM10 daily data available (i.e., 409 stations), we computed the mean PM2.5∕PM10 ratio and its corresponding standard deviation. The mean ratio was 0.56 with a standard deviation of 0.32. It is interesting to note that the mean PM2.5∕PM10 ratio estimated from 2 years of surface observations over the CONUS is close to 0.6 (the number used in this study), as reported by Kaku et al. (2018). We also tested the sensitivity of the derived PM2.5 concentrations as a function of PM2.5∕PM10 ratio for two scenarios: ±1 standard deviation of the mean (Table 3). In general, a ±50 % to 60 % change is found with the variation in the PM2.5∕PM10 ratio at the range of ±1 standard deviation of the mean. As suggested from Table 3, the lowest mean daytime bias is found for a ratio of 0.6, and for nighttime the lowest mean bias occurs using a ratio of 0.88.
Table 3Statistical summary of a sensitivity analysis varying the PM2.5-to-PM10 ratio used, including slope from Deming regression, mean bias (CALIOP−EPA) of PM2.5 in micrograms per cubic meter, and percent error change in derived PM2.5, defined as . The row in bold represents the results shown in the remainder of the paper.
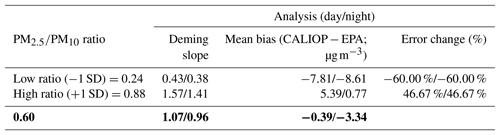
3.2.5 Sampling-related biases
As mentioned in the introduction section, a sampling bias, due to the very small footprint size and ∼16-day repeat cycle of CALIOP, can exist when using CALIOP observations for PM2.5 estimates (Zhang and Reid, 2009). This sampling-induced bias is investigated from a 2-year mean perspective by comparing histograms of PM2.5_EPA and Cm2.5 concentrations as shown in Fig. 6. To generate Fig. 6, all available daily EPA PM2.5 data are used to represent the “true” 2-year mean spectrum of PM2.5 concentrations over the EPA sites. The aerosol extinction data spatially collocated to the EPA sites (Sect. 3.1), but not temporally collocated, are used for estimating the 2-year mean spectrum of PM2.5 concentrations as derived from CALIOP observations. To be consistent with the previous analysis, only cloud-free CALIOP profiles are considered. The PM2.5_EPA concentrations peak at ∼10 µg m−3 (standard deviation of ∼3 µg m−3), and CALIOP-derived PM2.5 peaks at ∼9 µg m−3 (daytime; standard deviation of ∼4 µg m−3) and ∼7 µg m−3 (nighttime; standard deviation of ∼2 µg m−3). The distribution shifts towards smaller concentrations for CALIOP, more so for nighttime than daytime (possibly due to CALIOP daytime versus nighttime detection differences).
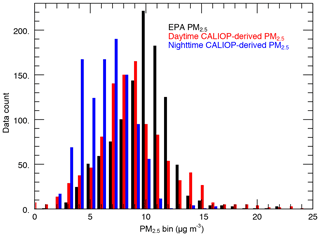
Figure 6The 2-year (2008–2009) histograms of mean PM2.5 concentrations from the U.S. EPA (in black) and those derived from aerosol extinction using nighttime (in blue) and daytime (in red) CALIOP data. The U.S. EPA data shown are not collocated, while those derived using CALIOP are spatially (but not temporally) collocated, with EPA station observations.
Still, Fig. 6 may reflect the diurnal difference in PM2.5 concentrations as well as the retrieval bias in Cm2.5 values. Thus, we have re-performed the exercise shown in Fig. 6 using spatially and temporally collocated PM2.5_EPA and Cm2.5 data as shown in Fig. 7. To construct Fig. 7, PM2.5_EPA and Cm2.5 data are collocated following the steps mentioned in Sect. 3.1, with CALIOP and EPA PM2.5 representing 2-year mean values for each EPA station. Again, only cloud-free CALIOP profiles are considered for this analysis. As shown in Fig. 7a, the PM2.5_EPA concentrations peak at ∼12 µg m−3 (standard deviation of ∼4 µg m−3), and daytime Cm2.5 peaks at ∼10 µg m−3 (standard deviation of ∼4 µg m−3). In comparison, with the use of collocated nighttime Cm2.5 and PM2.5_EPA data as shown in Fig. 7b, the peak PM2.5_EPA value is about 5 µg m−3 higher than the peak Cm2.5 value (with similar standard deviations as found in the analyses of Fig. 7a). Considering both Figs. 6 and 7, it is likely that the temporal sampling bias seen in Fig. 6 is at least in part due to retrieval bias as well as the difference in PM2.5 concentrations during daytime and nighttime.
3.2.6 CALIOP AOD analysis
Most past studies focused on the use of column AODs as proxies for surface PM2.5 (e.g., Liu et al., 2005; Hoff and Christopher, 2009; van Donkelaar et al., 2015). Therefore, it is interesting to investigate whether near-surface CALIOP extinction values can be used as a better physical quantity to estimate surface PM2.5 in comparing with column-integrated CALIOP AOD. To achieve this goal, we have compared CALIOP column AOD and PM2.5 from EPA stations, as shown in Fig. 8. Similar to the scatter plots of Fig. 3, each point represents a 2-year mean for each EPA site and was created from a dataset following the same spatial–temporal collocation as described above. As shown in Fig. 8, r2 values of 0.04 and 0.13 are found using CALIOP daytime and nighttime AOD data, respectively, similar to the MODIS-based analysis shown in Fig. 1. This is expected, as elevated aerosol layers will negatively impact the relationship between surface PM2.5 and column AOD. The derivation of surface PM2.5 from near-surface CALIOP extinction, as demonstrated from this study, however, provides a much better spatial matching between the quantities being compared, with potential error terms that can be well quantified and minimized in later studies.
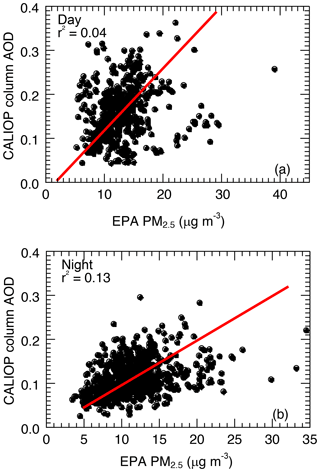
Figure 8For 2008–2009, scatter plots of mean PM2.5 concentration from ground-based U.S. EPA stations and mean column AOD from collocated CALIOP observations, using (a) daytime and (b) nighttime CALIOP data. The red lines represent the Deming regression fits.
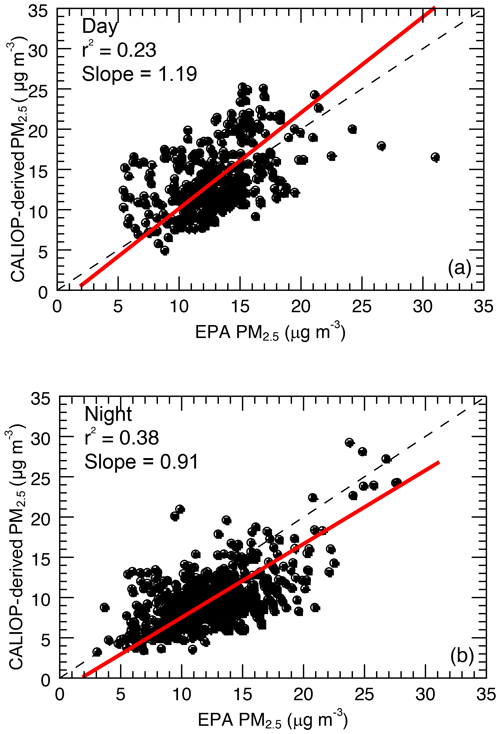
Figure 9For 2008–2009, scatter plots of mean PM2.5 concentration from ground-based U.S. EPA stations and those derived from collocated all-sky (including cloud-free and cloudy profiles) near-surface CALIOP observations, using (a) daytime and (b) nighttime CALIOP data. The red lines represent the Deming regression fits.
3.2.7 Cloud flag sensitivity study
For most of this paper, a strict cloud screening process is implemented, during which no clouds are allowed in the entire CALIOP profile. However, in contrast to passive sensor capabilities (e.g., MODIS), near-surface aerosol extinction coefficients can be readily retrieved from CALIOP profiles even when there are transparent cloud layers above. Therefore, we conducted an additional analysis for which no cloud flag was set (i.e., all-sky conditions). Results are shown in scatter plot form in Fig. 9, in a manner similar to in Fig. 3e and f, with an additional 97 points for the daytime analysis and 156 points for the nighttime analysis. Comparing the all-sky results with those of Fig. 3e and f (cloud-free conditions), the r2 values are similar. This is also true in terms of mean bias, with similar values of 0.70 µg m−3 found for daytime, and −2.68 µg m−3 for nighttime, all-sky scenarios. This indicates that our method performs reasonably well from an all-sky perspective. However, we note that restricting the analysis to solely those cases that are cloudy (not shown), the method does not perform as well. For example, the r2 value decreases by 71 % for the daytime analysis compared to the cloud-free results (Fig. 3e). The corresponding nighttime r2 value decreases by 90 %. This is expected, as any errors made in estimating the optical depths of the overlying clouds will propagate (as biases) into the extinction retrievals for the underlying aerosols.
3.2.8 Aerosol type analysis
Also, for this study, we assume that the primary aerosol type over the CONUS is pollution (i.e., sulfate) aerosol, which is generally composed of smaller (fine mode) particles that tend to exhibit mass extinction efficiencies ∼4 m2 g−1. However, even after implementing our dust-free restriction, the study region can also be contaminated with non-pollution aerosols, which can have a larger particle size and exhibit lower mass extinction efficiencies (e.g., Hess et al., 1998; Malm and Hand, 2007; Lynch et al., 2016). The use of PM2.5 versus PM10 somewhat mitigates this size dependency, but nevertheless coarse mode dust or sea salt can dominate PM2.5 mass values (e.g., Atwood et al., 2013).
Thus, in this section, the impact of aerosol types on the derived PM2.5 concentrations was explored by varying the mass scattering and absorption efficiencies and gamma values associated with each aerosol type. The three aerosol types chosen for this sensitivity study were dust, sea salt, and smoke, based upon Lynch et al. (2016). The mass scattering and absorption values for dust and sea salt were interpolated to 0.532 µm from values at 0.450 and 0.550 µm from OPAC (as was performed for the sulfate case; Hess et al., 1998). For smoke, these values were interpolated to 0.532 µm from values at 0.440 and 0.670 µm as provided by Reid et al. (2005) for smoke cases over the US and Canada. The gamma values were taken from Lynch et al. (2016) and the references within. These values, as well as the results from this sensitivity study, are shown in Table 4. If we assume all aerosols within the study region are smoke aerosols, no major changes in the retrieved CALIOP PM2.5 values are found. However, significant uncertainties on the order of ∼200 % are found if sea salt, or ∼800 % if dust, aerosol mass scattering and absorption efficiencies and gamma values are used instead. Clearly, this study suggests that accurate aerosol typing is necessary for future applications of CALIOP observations for surface PM2.5 estimations.
Table 4Statistical summary of a sensitivity analysis varying the aerosol type assumed in the derivation of PM2.5, including R2, slope from Deming regression, mean bias (CALIOP−EPA) of PM2.5 in micrograms per cubic meter, and percent error change in derived PM2.5, defined as . The row in bold represents the results shown in the remainder of the paper.
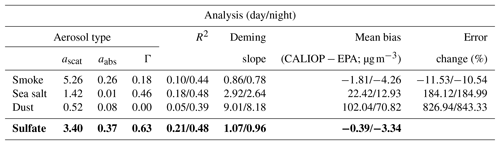
3.3 E-folding correlation length for PM2.5 concentrations over the CONUS
As a last study, we also estimated the spatial e-folding correlation length for PM2.5 concentrations over the CONUS. This provides us an estimation of the correlation between a CALIOP-derived and actual PM2.5 concentration for a given location as a function of distance between the CALIOP observation and the given location. To accomplish this, all EPA stations over the CONUS with at least 50 days of daily data available for the 2008–2009 period were first determined. Next, the distances between each pair of these EPA stations, and their corresponding correlation of daily PM2.5 concentrations, were computed. Results are shown in Fig. 10 as a scatter plot, with individual points in gray and the black curve representing the exponential fit to the data. A decrease in PM2.5 correlation with distance between EPA stations is found, and the e-folding length in correlation (e.g., correlation reduced to 1∕e, or 0.37) is ∼600 km (from an AOD standpoint, this value is 40–400 km, as suggested by Anderson et al., 2003).
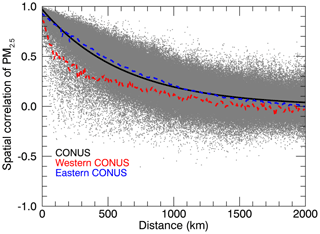
Figure 10For 2008–2009 over the CONUS, scatter plot of distance (km) between any two U.S. EPA stations and the corresponding spatial correlation of PM2.5 concentration between each pair of stations. The black curve represents the exponential fit to the data for the entire CONUS, and the red and blue dashed lines represent 10 km bin averages for the western and eastern CONUS, respectively.
Also included in Fig. 10 are results from a corresponding regional analysis, with the red and blue lines showing bin averages (10 km) for the western and eastern CONUS, respectively (regions partitioned by the −97∘ longitude line). The e-folding length is ∼300 km for the western CONUS and ∼700 km for the eastern CONUS, indicating a much shorter correlation length for pollution over the western CONUS, possibly due to a more complex terrain such as mountains. Overall, these PM2.5 e-folding lengths suggest that CALIOP-derived PM2.5 concentrations could still have some representative skill within a few hundred kilometers of a given location.
In this paper, we have demonstrated a new bulk-mass-modeling method for retrieving surface particulate matter (PM) with particle diameters smaller than 2.5 µm (PM2.5) using observations acquired by the NASA Cloud-Aerosol Lidar with Orthogonal Polarization (CALIOP) instrument from 2008 to 2009. For the purposes of demonstrating this concept, only regionally averaged parameters, such as mass scattering and absorption coefficients, and PM2.5-to-PM10 (PM with particle diameters smaller than 10 µm) conversion ratio, are used. Also, we assume the dominant type of aerosols over the study region is pollution aerosols (supported by the occurrence frequencies of aerosol types determined by the CALIOP algorithms) and exclude aerosol extinction range bins classified as dust from the analysis. Even with the highly averaged parameters, the results from this paper are rather promising and demonstrate a potential for monitoring PM pollution using active observations from lidars. Specifically, the primary results of this study are as follows.
-
CALIOP-derived PM2.5 concentrations of ∼10–12.5 µg m−3 are found over the eastern contiguous United States (CONUS), with lower values of ∼2.5–5 µg m−3 over the central CONUS. PM2.5 values of ∼10–20 µg m−3 are found over the west coast of the CONUS, primarily California. The spatial distribution of 2-year mean PM2.5 concentrations derived from near-surface CALIOP aerosol data compares well to the spatial distribution of in situ PM2.5 measurements collected at the ground-based stations of the U.S. Environmental Protection Agency (EPA). The use of nighttime CALIOP extinction to derive PM2.5 results in a higher correlation (r2=0.48; mean bias µg m−3) with EPA PM2.5 than daytime CALIOP extinction data (r2=0.21; mean bias µg m−3).
-
Correlations between CALIOP aerosol optical depth (AOD) and EPA PM2.5 are much lower (r2 values of 0.04 and 0.13 for daytime and nighttime CALIOP AOD data, respectively) than those obtained from derived PM2.5 using near-surface CALIOP aerosol extinction. A similar correlation is also found between Moderate Resolution Imaging Spectroradiometer (MODIS) AOD and EPA PM2.5 from 2-year (2008–2009) means. This suggests that CALIOP extinction may be used as a better parameter for estimating PM2.5 concentrations from a 2-year mean perspective. Also, the algorithm proposed in this study is essentially a semi-physical-based method, and thus the retrieval process can be improved, upon a careful study of the physical parameters used in the process.
-
Spatial and temporal sampling biases, as well as a retrieval bias, are found. Also, several sensitivity studies were conducted, including surface layer height, cloud flag, PM2.5∕PM10 ratio, relative humidity, and aerosol type. The sensitivity studies highlight the need for accurate aerosol typing for estimating PM2.5 concentrations using CALIOP observations.
-
Using surface-based PM2.5 at EPA stations alone, the e-folding correlation length for PM2.5 concentrations was found to be about 600 km for the CONUS. A regional analysis yielded values of ∼300 and ∼700 km for the western and eastern CONUS, respectively. Thus, while limited in spatial sampling, measurements from CALIOP may still be used for estimating PM2.5 concentrations over the CONUS.
As noted earlier, CALIOP observations are still rather sparse, and concerns related to reported CALIOP aerosol extinction values also exist, such as solar and surface contamination and the retrieval fill value issue (e.g., Toth et al., 2018). Yet, the future High Spectral Resolution Lidar (HSRL) instrument on board the Earth Clouds, Aerosol, and Radiation Explorer (EarthCARE) satellite (Illingworth et al., 2015), as well as forthcoming space-based lidar missions in response to the 2017 Decadal Survey, offer opportunities to further explore aerosol extinction-based PM concentrations. Ultimately the results from this study show that the combined use of several lidar instruments for monitoring regional and global PM pollution is potentially achievable.
The CALIPSO Level 2 5 km aerosol profile (Vaughan et al., 2018; https://doi.org/10.5067/CALIOP/CALIPSO/LID_L2_05kmAPro-Standard-V4-10; last access: 26 September 2018) and aerosol layer (Vaughan et al., 2018; https://doi.org/10.5067/CALIOP/CALIPSO/LID_L2_05kmALay-Standard-V4-10; last access: 26 September 2018) products were obtained from NASA Langley Research Center Atmospheric Science Data Center. MODIS data were obtained from NASA Goddard Space Flight Center (http://ladsweb.nascom.nasa.gov; last access: 12 March 2019). The PM2.5 data were obtained from the EPA AQS site (https://aqs.epa.gov/aqsweb/airdata/download_files.html; last access: 12 March 2019).
JZ and TDT designed the experiment, TDT performed data analysis, and JSR and MAV made extensive suggestions and revised the study. All authors were involved in the writing of the paper.
The authors declare that they have no conflict of interest.
This research was funded with the support of the NASA Earth and Space
Science Fellowship program (NNX16A066H). Author Jianglong Zhang acknowledges the support
from NASA grant NNX17AG52G. Jeffrey S. Reid was supported by ONR 322. We thank the two anonymous
reviewers for their constructive and valuable comments in improving the paper.
Edited by: Vassilis Amiridis
Reviewed by: two anonymous referees
Anderson, T. L., Charlson, R. J., Winker, D. M., Ogren, J. A., and Holmén, K.: Mesoscale Variations of Tropospheric Aerosols, J. Atmos. Sci., 60, 119–136, https://doi.org/10.1175/1520-0469(2003)060<0119:MVOTA>2.0.CO;2, 2003.
Atwood, S. A., Reid, J. S., Kreidenweis, S. M., Cliff, S. S., Zhao, Y., Lin, N.-H., Tsay, S.-C., Chu, Y.-C., and Westphal, D. L.: Size resolved measurements of springtime aerosol particles over the northern South China Sea, Atmos. Environ., 78, 134–143, https://doi.org/10.1016/j.atmosenv.2012.11.024, 2013.
Campbell, J. R., Tackett, J. L., Reid, J. S., Zhang, J., Curtis, C. A., Hyer, E. J., Sessions, W. R., Westphal, D. L., Prospero, J. M., Welton, E. J., Omar, A. H., Vaughan, M. A., and Winker, D. M.: Evaluating nighttime CALIOP 0.532 µm aerosol optical depth and extinction coefficient retrievals, Atmos. Meas. Tech., 5, 2143–2160, https://doi.org/10.5194/amt-5-2143-2012, 2012.
Charlson, R. J., Ahlquist, N. C., and Horvath, H.: On the generality of correlation of atmospheric aerosol mass concentration and light scatter, Atmos. Environ., 2, 455–464, https://doi.org/10.1016/0004-6981(68)90039-5, 1968.
Chew, B. N., Campbell, J. R., Hyer, E. J., Salinas, S. V., Reid, J. S., Welton, E. J., Holben, B. N., and Liew, S. C.: Relationship between aerosol optical depth and particulate matter over Singapore: Effects of aerosol vertical distributions, Aerosol Air Qual. Res., 16, 2818–2830, https://doi.org/10.4209/aaqr.2015.07.0457, 2016.
Chow, J. C., Watson, J. G., Park, K., Robinson, N. F., Lowenthal, D. H., Park, K., and Magliano, K. A.: Comparison of particle light scattering and fine particulate matter mass in central California, J. Air Waste Manage., 56, 398–410, https://doi.org/10.1080/10473289.2006.10464515, 2006.
Chung, A., Chang, D. P., Kleeman, M. J., Perry, K. D., Cahill, T. A., Dutcher, D., McDougall, E. M., and Stroud, K.: Comparison of real-time instruments used to monitor airborne particulate matter, J. Air Waste Manage., 51, 109–120, 2001.
Colarco, P. R., Kahn, R. A., Remer, L. A., and Levy, R. C.: Impact of satellite viewing-swath width on global and regional aerosol optical thickness statistics and trends, Atmos. Meas. Tech., 7, 2313–2335, https://doi.org/10.5194/amt-7-2313-2014, 2014.
Deming, W. E.: Statistical Adjustment of Data, Wiley, New York, 1943.
Di, Q., Wang, Y., Zanobetti, A., Wang, Y., Koutrakis, P., Choirat, C., Dominici, F., and Schwartz, J. D.: Air pollution and mortality in the Medicare population, New Engl. J. Med., 376, 2513–2522, https://doi.org/10.1056/NEJMoa1702747, 2017.
Eatough, D. J., Long, R. W., Modey, W. K., and Eatough, N. L.: Semi-volatile secondary organic aerosol in urban atmospheres: meeting a measurement challenge, Atmos. Environ., 37, 1277–1292, 2003.
Engel-Cox, J. A., Holloman, C. H., Coutant, B. W., and Hoff, R. M.: Qualitative and quantitative evaluation of MODIS satellite sensor data for regional and urban scale air quality, Atmos. Environ., 38, 2495–2509, https://doi.org/10.1016/j.atmosenv.2004.01.039, 2004.
Federal Register: National ambient air quality standards for particulate matter, Final Rule Federal Register/vol. 62, no. 138/18 July 1997/Final Rule, 40 CFR Part 50, 1997.
Glantz, P., Kokhanovsky, A., von Hoyningen-Huene, W., and Johansson, C.: Estimating PM2.5 over southern Sweden using space-borne optical measurements, Atmos. Environ., 43, 5838–5846, https://doi.org/10.1016/j.atmosenv.2009.05.017, 2009.
Gong, W., Huang, Y., Zhang, T., Zhu, Z., Ji, Y., and Xiang, H.: Impact and Suggestion of Column-to-Surface Vertical Correction Scheme on the Relationship between Satellite AOD and Ground-Level PM2.5 in China, Remote Sens., 9, 1038, https://doi.org/10.3390/rs9101038, 2017.
Greenstone, M.: The impacts of environmental regulations on industrial activity: Evidence from the 1970 and 1977 clean air act amendments and the census of manufactures, J. Polit. Econ., 110, 1175–1219, https://doi.org/10.1086/342808, 2002.
Hand, J. L. and Malm, W. C.: Review of aerosol mass scattering efficiencies from ground-based measurements since 1990, J. Geophys. Res.-Atmos., 112, D16203, https://doi.org/10.1029/2007JD008484, 2007.
Hand, J. L., Schichtel, B. A., Malm, W. C., and Frank, N. H.: Spatial and temporal trends in PM2.5 organic and elemental carbon across the United States, Adv. Meteorol., 2013, 367674, https://doi.org/10.1155/2013/367674, 2013.
Hänel, G.: The properties of atmospheric aerosol particles as functions of the relative humidity at thermodynamic equilibrium with the surrounding moist air, Adv. Geophys., 19, 73–188, https://doi.org/10.1016/S0065-2687(08)60142-9, 1976.
Hess, M., Koepke, P., and Schult, I.: Optical properties of aerosols and clouds: The software package OPAC, B. Am. Meteorol. Soc., 79, 831–844, https://doi.org/10.1175/1520-0477(1998)079<0831:OPOAAC>2.0.CO;2, 1998.
Hoff, R. M. and Christopher, S. A.: Remote sensing of particulate pollution from space: have we reached the promised land?, J. Air Waste Manage., 59.6, 645–675, https://doi.org/10.3155/1047-3289.59.6.645, 2009.
Huang, X. H., Bian, Q., Ng, W. M., Louie, P. K., and Yu, J. Z: Characterization of PM2.5 major components and source investigation in suburban Hong Kong: a one year monitoring study, Aerosol Air Qual. Res., 14, 237–250, 2014.
Hunt, W. H., Winker, D. M., Vaughan, M. A., Powell, K. A., Lucker, P. L., and Weimer, C.: CALIPSO lidar description and performance assessment, J. Atmos. Ocean. Tech., 26, 1214–1228, https://doi.org/10.1175/2009JTECHA1223.1, 2009.
Illingworth, A. J., Barker, H. W., Beljaars, A., Ceccaldi, M., Chepfer, H., Clerbaux, N., Cole, J., Delanoë, J., Domenech, C., Donovan, D. P., Fukuda, S., Hirakata, M., Hogan, R. J., Huenerbein, A., Kollias, P., Kubota, T., Nakajima, T., Nakajima, T. Y., Nishizawa, T., Ohno, Y., Okamoto, H., Oki, R., Sato, K., Satoh, M., Shephard, M. W., Velázquez-Blázquez, A., Wandinger, U., Wehr, T., and Van Zadelhoff, G.-J.: The EarthCARE satellite: The next step forward in global measurements of clouds, aerosols, precipitation, and radiation, B. Am. Meteorol. Soc., 96, 1311–1332, https://doi.org/10.1175/BAMS-D-12-00227.1, 2015.
Kaku, K. C., Reid, J. S., Hand, J. L., Edgerton, E. S., Holben, B. N., Zhang, J., and Holz, R. E.: Assessing the challenges of surface-level aerosol mass estimates from remote sensing during the SEAC4RS and SEARCH campaigns: Baseline surface observations and remote sensing in the southeastern United States, J. Geophys. Res.-Atmos., 123, 7530–7562, https://doi.org/10.1029/2017JD028074, 2018.
Kiss, G., Imre, K., Molnár, Á., and Gelencsér, A.: Bias caused by water adsorption in hourly PM measurements, Atmos. Meas. Tech., 10, 2477–2484, https://doi.org/10.5194/amt-10-2477-2017, 2017.
Kittaka, C., Winker, D. M., Vaughan, M. A., Omar, A., and Remer, L. A.: Intercomparison of column aerosol optical depths from CALIPSO and MODIS-Aqua, Atmos. Meas. Tech., 4, 131–141, https://doi.org/10.5194/amt-4-131-2011, 2011.
Kumar, N., Chu, A., and Foster, A.: An empirical relationship between PM2.5 and aerosol optical depth in Delhi Metropolitan, Atmos. Environ., 41, 4492–4503, https://doi.org/10.1016/j.atmosenv.2007.01.046, 2007.
Levy, R. C., Mattoo, S., Munchak, L. A., Remer, L. A., Sayer, A. M., Patadia, F., and Hsu, N. C.: The Collection 6 MODIS aerosol products over land and ocean, Atmos. Meas. Tech., 6, 2989–3034, https://doi.org/10.5194/amt-6-2989-2013, 2013.
Li, J., Carlson, B. E., and Lacis, A. A.: How well do satellite AOD observations represent the spatial and temporal variability of PM2.5 concentration for the United States?, Atmos. Environ., 102, 260–273, https://doi.org/10.1016/j.atmosenv.2014.12.010, 2015.
Liou, K.-N.: An introduction to atmospheric radiation, Academic Press, 84, 9, 2002.
Liu, Y., Park, R. J., Jacob, D. J., Li, Q., Kilaru, V., and Sarnat, J. A.: Mapping annual mean ground- level PM2.5 concentrations using Multiangle Imaging Spectroradiometer aerosol optical thickness over the contiguous United States, J. Geophys. Res.-Atmos., 109, D22206, https://doi.org/10.1029/2004JD005025, 2004.
Liu, Y., Sarnat, J. A., Kilaru, V., Jacob, D. J., and Koutrakis, P.: Estimating ground-level PM2.5 in the eastern United States using satellite remote sensing, Environ. Sci. Technol., 39, 3269–3278, https://doi.org/10.1021/es049352m, 2005.
Liu, Y., Franklin, M., Kahn, R., and Koutrakis, P.: Using aerosol optical thickness to predict ground-level PM2.5 concentrations in the St. Louis area: A comparison between MISR and MODIS, Remote Sens. Environ., 107, 33–44, https://doi.org/10.1016/j.rse.2006.05.022, 2007.
Lynch, P., Reid, J. S., Westphal, D. L., Zhang, J., Hogan, T. F., Hyer, E. J., Curtis, C. A., Hegg, D. A., Shi, Y., Campbell, J. R., Rubin, J. I., Sessions, W. R., Turk, F. J., and Walker, A. L.: An 11-year global gridded aerosol optical thickness reanalysis (v1.0) for atmospheric and climate sciences, Geosci. Model Dev., 9, 1489–1522, https://doi.org/10.5194/gmd-9-1489-2016, 2016.
Malm, W. C. and Hand, J. L.: An examination of the physical and optical properties of aerosols collected in the IMPROVE program, Atmos. Environ., 41, 3407–3427, https://doi.org/10.1016/j.atmosenv.2006.12.012, 2007.
Nessler, R., Weingartner, E., and Baltensperger, U.: Effect of humidity on aerosol light absorption and its implications for extinction and the single scattering albedo illustrated for a site in the lower free troposphere, J. Aerosol Sci., 36, 958–972, 2005.
Omar, A. H., Winker, D. M., Tackett, J. L., Giles, D. M., Kar, J., Liu, Z., Vaughan, M. A., Powell, K. A., and Trepte, C. R.: CALIOP and AERONET aerosol optical depth comparisons: One size fits none, J. Geophys. Res.-Atmos., 118, 4748–4766, https://doi.org/10.1002/jgrd.50330, 2013.
Patashnick, H., Rupprecht, G., Ambs, J. L., and Meyer, M. B.: Development of a reference standard for particulate matter mass in ambient air, Aerosol Sci. Technol., 34, 42–45, 2001.
Reid, J. S., Eck, T. F., Christopher, S. A., Koppmann, R., Dubovik, O., Eleuterio, D. P., Holben, B. N., Reid, E. A., and Zhang, J.: A review of biomass burning emissions part III: intensive optical properties of biomass burning particles, Atmos. Chem. Phys., 5, 827–849, https://doi.org/10.5194/acp-5-827-2005, 2005.
Reid, J. S., Kaku, K., Xian, P., Benedetti, A., Colarco, P. R., da Silva Jr., A. M., Holben, B. N., Rubin, J., Tanaka, T. Y., and Zhang, J.: Skill of Operational Aerosol Forecast Models in Predicting Aerosol Events and Trends of the Eastern United States, A11B-001, AGU Fall meeting, San Francisco, 12–16 December 2016.
Reid, J. S., Kuehn, R. E., Holz, R. E., Eloranta, E. W., Kaku, K. C., Kuang, S., Newchurch, M. J., Thompson, A. M., Trepte, C. R., Zhang, J., Atwood, S. A., Hand, J. L., Holben, B. N., Minnis, P., and Posselt, D. J.: Ground-based High Spectral Resolution Lidar observation of aerosol vertical distribution in the summertime Southeast United States, J. Geophys. Res.-Atmos., 122, 2970–3004, https://doi.org/10.1002/2016JD025798, 2017.
Sessions, W. R., Reid, J. S., Benedetti, A., Colarco, P. R., da Silva, A., Lu, S., Sekiyama, T., Tanaka, T. Y., Baldasano, J. M., Basart, S., Brooks, M. E., Eck, T. F., Iredell, M., Hansen, J. A., Jorba, O. C., Juang, H.-M. H., Lynch, P., Morcrette, J.-J., Moorthi, S., Mulcahy, J., Pradhan, Y., Razinger, M., Sampson, C. B., Wang, J., and Westphal, D. L.: Development towards a global operational aerosol consensus: basic climatological characteristics of the International Cooperative for Aerosol Prediction Multi-Model Ensemble (ICAP-MME), Atmos. Chem. Phys., 15, 335–362, https://doi.org/10.5194/acp-15-335-2015, 2015.
Silva, R. A., West, J. J., Zhang, Y., Anenberg, S. C., Lamarque, J. F., Shindell, D. T., Collins, W. J., Dalsoren, S., Faluvegi, G., Folberth, G., and Horowitz, L. W.: Global premature mortality due to anthropogenic outdoor air pollution and the contribution of past climate change, Environ. Res. Lett., 8, 034005, https://doi.org/10.1088/1748-9326/8/3/034005, 2013.
Spagnolo, G. S.: Automatic instrument for aerosol samples using the beta-particle attenuation, J. Aerosol Sci., 20, 19–27, 1989.
Toth, T. D., Zhang, J., Campbell, J. R., Reid, J. S., Shi, Y., Johnson, R. S., Smirnov, A., Vaughan, M. A., and Winker, D. M.: Investigating enhanced Aqua MODIS aerosol optical depth retrievals over the mid-to-high latitude Southern Oceans through intercomparison with co-located CALIOP, MAN, and AERONET data sets, J. Geophys. Res.-Atmos., 118, 4700–4714, https://doi.org/10.1002/jgrd.50311, 2013.
Toth, T. D., Zhang, J., Campbell, J. R., Hyer, E. J., Reid, J. S., Shi, Y., and Westphal, D. L.: Impact of data quality and surface-to-column representativeness on the PM2.5∕satellite AOD relationship for the contiguous United States, Atmos. Chem. Phys., 14, 6049–6062, https://doi.org/10.5194/acp-14-6049-2014, 2014.
Toth, T. D., Zhang, J., Campbell, J. R., Reid, J. S., and Vaughan, M. A.: Temporal variability of aerosol optical thickness vertical distribution observed from CALIOP, J. Geophys. Res.-Atmos., 121, 9117–9139, https://doi.org/10.1002/2015JD024668, 2016.
Toth, T. D., Campbell, J. R., Reid, J. S., Tackett, J. L., Vaughan, M. A., Zhang, J., and Marquis, J. W.: Minimum aerosol layer detection sensitivities and their subsequent impacts on aerosol optical thickness retrievals in CALIPSO level 2 data products, Atmos. Meas. Tech., 11, 499–514, https://doi.org/10.5194/amt-11-499-2018, 2018.
Val Martin, M., Heald, C. L., Ford, B., Prenni, A. J., and Wiedinmyer, C.: A decadal satellite analysis of the origins and impacts of smoke in Colorado, Atmos. Chem. Phys., 13, 7429–7439, https://doi.org/10.5194/acp-13-7429-2013, 2013.
Van Donkelaar, A., Martin, R. V., Brauer, M., Kahn, R., Levy, R., Verduzco, C., and Villeneuve, P. J.: Global Estimates of Ambient Fine Particulate Matter Concentrations from Satellite Based Aerosol Optical Depth: Development and Application, Environ. Health Persp., 118, 847–855, https://doi.org/10.1289/ehp.0901623, 2010.
Van Donkelaar, A., Martin, R. V., Spurr, R. J., and Burnett, R. T.: High-resolution satellite-derived PM2.5 from optimal estimation and geographically weighted regression over North America, Environ. Sci. Technol., 49, 10482–10491, https://doi.org/10.1021/acs.est.5b02076, 2015.
Vaughan, M., Pitts, M., Trepte, C., Winker, D., Detweiler, P., Garnier, A., Getzewich, B., Hunt, W., Lambeth, J., Lee, K.-P., Lucker, P., Murray, T., Rodier, S., Tremas, T., Bazureau, A., and Pelon, J.: Cloud-Aerosol LIDAR Infrared Pathfinder Satellite Observations (CALIPSO) data management system data products catalog, Release 4.20, NASA Langley Research Center Document PC-SCI-503, available at: https://www-calipso.larc.nasa.gov/products/CALIPSO_DPC_Rev4x20.pdf, last access: 26 September 2018 (data available at: https://doi.org/10.5067/CALIOP/CALIPSO/LID_L2_05kmAPro-Standard-V4-10 and https://doi.org10.5067/CALIOP/CALIPSO/ LID_L2_05kmALay-Standard-V4-10).
Waggoner, A. P. and Weiss, R. E.: Comparison of fine particle mass concentration and light scattering extinction in ambient aerosol, Atmos. Environ., 14, 623–626, https://doi.org/10.1016/0004-6981(80)90098-0, 1980.
Wang, J. and Christopher, S. A.: Intercomparison between satellite-derived aerosol optical thickness and PM2.5 mass: implications for air quality studies, Geophys. Res. Lett., 30, 21, https://doi.org/10.1029/2003GL018174, 2003.
Winker, D. M., Hunt, W. H., and McGill, M. J.: Initial performance assessment of CALIOP, Geophys. Res. Lett., 34, L19803, https://doi.org/10.1029/2007GL030135, 2007.
Winker, D. M., Vaughan, M. A., Omar, A., Hu, Y., Powell, K. A., Liu, Z., Hunt, W. H., and Young, S. A.: Overview of the CALIPSO Mission and CALIOP Data Processing Algorithms, J. Atmos. Ocean. Tech., 26, 2310–2323, https://doi.org/10.1175/2009JTECHA1281.1, 2009.
Winker, D. M., Pelon, J., Coakley Jr., J. A., Ackerman, S. A., Charlson, R. J., Colarco, P. R., Flamant, P., Fu, Q., Hoff, R. M., Kittaka, C., Kubar, T. L., LeTreut, H., McCormick, M. P., Mégie , G., Poole, L., Powell, K., Trepte, C., Vaughan, M. A., and Wielicki, B. A.: The CALIPSO mission: A global 3D view of aerosols and clouds, B. Am. Meteorol. Soc., 91, 1211–1230, https://doi.org/10.1175/2010BAMS3009.1, 2010.
Young, S. A., Vaughan, M. A., Kuehn, R. E., and Winker, D. M.: The retrieval of profiles of particulate extinction from Cloud–Aerosol Lidar and Infrared Pathfinder Satellite Observations (CALIPSO) data: Uncertainty and error sensitivity analyses, J. Atmos. Ocean. Tech., 30, 395–428, https://doi.org/10.1175/2009JTECHA1281.1, 2013.
Zhang, J. and Reid, J. S.: An analysis of clear sky and contextual biases using an operational over ocean MODIS aerosol product, Geophys. Res. Lett., 36, L15824, https://doi.org/10.1029/2009GL038723, 2009.
Zhang, J., Campbell, J. R., Hyer, E. J., Reid, J. S., Westphal, D. L., and Johnson, R. S.: Evaluating the impact of multisensor data assimilation on a global aerosol particle transport model, J. Geophys. Res.-Atmos., 119, 4674–4689, https://doi.org/10.1002/2013JD020975, 2014.
The requested paper has a corresponding corrigendum published. Please read the corrigendum first before downloading the article.
- Article
(7700 KB) - Full-text XML