the Creative Commons Attribution 4.0 License.
the Creative Commons Attribution 4.0 License.
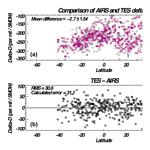
Characterization and evaluation of AIRS-based estimates of the deuterium content of water vapor
Susan S. Kulawik
Dejian Fu
Vivienne H. Payne
Alan E. Lipton
Igor Polonsky
Yuguang He
Karen Cady-Pereira
Jean-Luc Moncet
Robert L. Herman
Fredrick W. Irion
Kevin W. Bowman
Single-pixel tropospheric retrievals of HDO and H2O concentrations are retrieved from Atmospheric Infrared Sounder (AIRS) radiances using the optimal estimation algorithm developed for the Aura Tropospheric Emission Spectrometer (TES) project. We evaluate the error characteristics and vertical sensitivity of AIRS measurements corresponding to 5 d of TES data (or five global surveys) during the Northern Hemisphere summers between 2006 and 2010 (∼600 co-located comparisons per day). We find that the retrieval characteristics of the AIRS deuterium content measurements have similar vertical resolution in the middle troposphere as TES but with slightly less sensitivity in the lowermost troposphere, with a typical degrees of freedom (DOFS) in the tropics of 1.5. The calculated measurement uncertainty is ∼30 ‰ (parts per thousand relative to the deuterium composition of ocean water) for a tropospheric average between 750 and 350 hPa, the altitude region where AIRS is most sensitive, compared to ∼15 ‰ for the TES data. Comparison with the TES data also indicates that the uncertainty of a single target AIRS HDO ∕ H2O measurement is ∼30 ‰. Comparison of AIRS and TES data between 30∘ S and 50∘ N indicates that the AIRS data are biased low by ‰ with a latitudinal variation of ∼7.8 ‰. This latitudinal variation is consistent with the accuracy of TES data compared to in situ measurements, suggesting that both AIRS and TES have similar accuracy.
- Article
(3518 KB) - Full-text XML
- BibTeX
- EndNote
The author's copyright for this publication is transferred to the Jet Propulsion Laboratory, California Institute of Technology.
Measurements of the isotopic composition of water can help identify the source of the water and provide knowledge about its condensation and evaporation history (e.g., Galewsky et al., 2016, and references therein). Through most of the twentieth century, most isotopic measurements of water have been of precipitation (e.g., Craig, 1961). Near global measurements of the isotopic composition of water vapor became possible with the advent of spectroscopic techniques applied to in situ measurements (e.g., Noone et al., 2011) using lasers and for passive ground-based and satellite measurements (e.g., Worden et al., 2006; Frankenberg et al., 2009; Schneider et al., 2012; Lacour et al., 2012). These data have in turn been used to evaluate the role of convection, large-scale dynamics, and evapotranspiration in the tropical water cycle (e.g., Worden et al., 2007; Frankenberg et al., 2009; Wright et al., 2017) and tropical convection (e.g., Lacour et al., 2018, and refs therein) and the role of plants in global evapotranspiration (Good et al., 2015).
In this paper we demonstrate a retrieval algorithm, based upon the Aura TES optimal estimation retrieval algorithm (e.g., Worden et al., 2012) that can provide robustly characterized measurements of the deuterium content of water vapor (HDO and H2O) from the AIRS measurements. Our goal is to create a multi-decadal Earth Science Data Record (ESDR) using the AIRS and TES data; the TES global record spans ∼6 years (2005–2010) and the AIRS data span 17+ years starting in 2002. This ESDR could potentially be used for evaluating the changing water cycle (e.g., Bailey et al., 2017) and its coupling to the carbon cycle (e.g., Zhou et al., 2014; Wright et al., 2017).
We first characterize the vertical resolution and uncertainties for estimates of HDO and H2O, and their ratio, using AIRS radiance observations corresponding to boreal summertime TES global survey's between 2006 and 2010, which is the time period when TES observations sample the (near) global atmosphere, and the calibration approach for TES measurements remained the same. We make only these comparisons due to current processing limitations but expect additional overlap between TES and AIRS data sets in the coming years. We then compare the AIRS and TES data to evaluate the calculated uncertainties of the AIRS data.
The AIRS instrument is a nadir-viewing, scanning infrared spectrometer (Aumann et al., 2003; Pagano et al., 2003; Irion et al., 2018; DeSouza-Machado et al., 2018) that is on board the NASA Aqua satellite and was launched in 2002. AIRS measures the thermal radiance between approximately 3 and 12 µm with a resolving power of approximately 1200. For the 8 µm spectral range used for the HDO∕H2O retrievals, the spectral resolution is ∼1 cm−1 with a gridding of ∼0.5 cm−1; the signal-to-noise ratio (SNR) ranges from ∼400 to ∼1000 over the 8 µm region for a typical tropical scene. A single footprint has a diameter of ∼15 km in the nadir; with the ∼1650 km swath, the AIRS instrument can measure nearly the whole globe in a single day. The Aqua satellite is part of the “A-Train” that consists of multiple satellites, including TES, in a Sun-synchronous orbit at 705 km with an approximately 13:30 Equator crossing time.
The Aura TES instrument is a Fourier transform spectrometer that originally was designed to measure the thermal infrared (IR) radiances in both the limb and nadir viewing in order to obtain vertically resolved trace gas profiles of ozone, CO, CH4, HDO, and H2O and several ozone pre-cursors such as ammonia, methanol, and PAN (e.g., Beer et al., 2001; Worden et al., 2004, 2006, 2012; Luo et al., 2007; Beer et al., 2008; Payne et al., 2014). Several of these trace gases, such as CO, CH4, and ammonia, have also been quantified using AIRS radiances (e.g., McMillan et al., 2010; Xiong et al., 2008; Warner et al., 2016). In comparison to the AIRS instrument, TES has a spectral resolution of ∼0.12 cm−1 (apodized) with a spectral gridding of 0.06 cm−1. The SNR is ∼300 in the 8 µm spectral region. The Aura TES instrument, after the summer of 2005, observes one nadir scene every 100 km along the orbit path. The effective length of the record is approximately 5 years, between September 2005 and November 2009, after which instrument degradation problems resulted in interruptions and a decrease in sampling. The AIRS instrument has nearly 1000 times the sampling of TES and near-continuous operation between 2002 and the present and therefore can be used to construct several composition-based ESDRs.
The radiative transfer forward model used for this work is the optimal spectral sampling (OSS) fast radiative transfer model (RTM) (Moncet et al., 2008, 2015). The OSS approach is integrated in the operational Cross-Track Infrared Sounder (CrIS; Han et al., 2013) processing system (Divarkala et al., 2014) and has also been utilized for trace gas retrievals from CrIS (e.g., Shephard and Cady-Pereira, 2015). OSS uses a series of approximations tailored to a specific frequency range and spectral resolution to increase the radiative transfer calculation performance by approximately a factor of 20–100 relative to a line-by-line calculation (http://rtweb.aer.com, last access: 8 April 2019). OSS can be trained to user-defined accuracy relative to the line-by-line model used for training. Here, the training threshold was set to 20 % of the AIRS noise level. The line-by-line model used as a reference in the training and to build the absorption coefficient look-up tables (LUTs) used by the fast RTM is the Line-By-Line Radiative Transfer Model (LBLRTM) (Clough et al., 2005; Alvarado et al., 2013). The OSS version used in this work is based on LBLRTM v12.4, using the TES_v2.0 spectroscopic line parameter database. The TES_v2.0 line parameter database follows the HITRAN 2012 compilation (Rothman et al., 2013), with the following exceptions.
-
H2O positions and intensities are taken from the aer_v_3.4 line parameter database (http://rtweb.aer.com, last access: 8 April 2019), closely following the measured and calculated values published in Coudert et al. (2008).
-
CH4 includes first-order line mixing coefficients (as supplied in the aer_v_3.4 line parameter database). These were calculated using the approach of Tran et al. (2006).
-
CO2 line parameters are from the database of Lamouroux et al. (2015). This database takes most of its line positions, intensities, and lower state energies from the HITRAN 2012 database, but the values for air-broadening half-widths and their temperature dependences are adjusted from the HITRAN 2012 values to be consistent throughout the bands, and the air-induced pressure shifts (not given for a majority of transitions in HITRAN 2012) were added. The TES_v2.0 database includes first-order line mixing coefficients (as supplied in the aer_v_3.4.1 line parameter database), calculated using the software of Lamouroux et al. (2015).
Further information on the AER line parameter databases can be found at http://rtweb.aer.com (last access: 8 April 2019). OSS is adapted for use with AIRS radiances using the version 4 AIRS spectral response function (SRF) (Strow et al., 2003) that is interpolated to a uniform grid of 0.004 cm−1 centered on the channel center frequencies. The OSS radiative transfer code provides speedup of 20–100× over the original TES operational radiation transfer model (Clough et al., 2006).
The optimal estimation algorithm used in this analysis for quantifying CH4, HDO, H2O, temperature, cloud properties, and emissivity is extensively discussed in Worden et al. (2004, 2012) and Bowman et al. (2006). We therefore refer the reader to those papers for a description of the retrieval algorithm, with a suggestion that they start with the Worden et al. (2012) paper; however, we will briefly summarize the retrieval approach here. This retrieval algorithm, now called the MUlti-SpEctra, MUlti-SpEcies, MUlti-Sensors (MUSES) algorithm (Worden et al., 2007; Fu et al., 2013, 2016, 2018; Worden et al., 2013), can use radiances from multiple instruments including TES, CrIS, OMI, OMPS, TROPOMI, and MLS to quantify geophysical observables that affect the corresponding radiance.
For the AIRS retrievals discussed here, we simultaneously estimate not just CH4, CO, HDO, and H2O but also temperature (surface and atmosphere), emissivity (if over land), and a spectrally varying gray body cloud (e.g., Kulawik et al., 2006; Eldering et al., 2008). As in Worden et al. (2006, 2012) the constraint matrix used to regularize the HDO and H2O components of the retrieval includes off-diagonal components that reflect a priori knowledge about the variability of HDO with respect to H2O in order to ensure that retrieval of the ratio of HDO to H2O is optimized, as opposed to either HDO or H2O alone. The prior information used for this covariance is derived from monthly climatologies using the NCAR Global Climate Model as discussed in Worden et al. (2006). The a priori profile used for the HDO∕H2O ratio is set to be constant over the whole globe, and represents the mean tropical a priori profile from the NCAR model. However, the H2O a priori profile is allowed to vary by latitude and is based on reanalysis (Worden et al., 2006); therefore the HDO profile is the mean tropical profile of the HDO∕H2O ratio from the NCAR model multiplied by the H2O a priori profile.
We use single-pixel radiances that have not been transformed through “cloud clearing” in order to preserve the original well-characterized radiance noise characteristics for use in our estimates (Irion et al., 2018; DeSouza-Machado et al., 2018) and because we find that single-pixel AIRS radiances have sufficient information about cloud pressure and optical depth to be retrieved jointly with the trace gases, as demonstrated empirically through validation of these AIRS-based composition retrievals with TES retrievals (e.g., Figs. 1–4). We assume the noise in any given pixel is uncorrelated with that from adjacent pixels. However, these correlations are known to exist (e.g., Pagano et al., 2008) and the impact of ignoring them is that our calculated uncertainties will be larger than expected and therefore our noise-related uncertainty should be considered a conservative estimate.
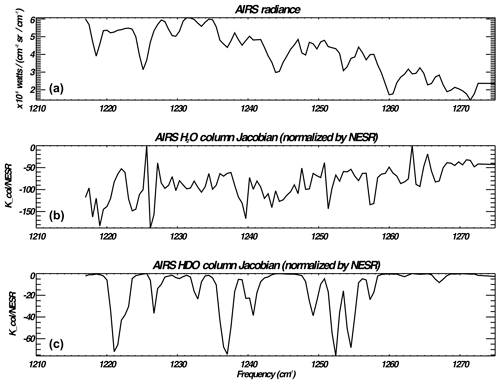
Figure 1(a) AIRS radiance at approximately 8 µm for a typical tropical scene. (b) The total column (log) Jacobian for H2O normalized by the AIRS NESR. (c) The total column (log) Jacobian for HDO normalized by the AIRS NESR.
A primary difference between the retrieval approach shown in this paper versus the TES methane and HDO retrievals (Worden et al., 2012) and those from previous efforts using AIRS radiances (e.g., Xiong et al., 2008) is that we retrieve these trace gas profiles using the AIRS radiances from ∼8 and ∼12 µm instead of radiances from the 8 µm region alone in order to provide a stronger constraint on atmospheric temperature and hence reduce uncertainty from knowledge of temperature on the HDO and H2O retrievals. The 8 µm region used (∼1217 to 1315 cm−1) for these retrievals has the most sensitivity to HDO and H2O, whereas the 12 µm band (∼650 to 900 cm−1) is primarily sensitive to temperature and H2O. All channels are used within this spectra unless flagged as poor during calibration.
While H2O is quantified using radiances from both the 12 and 8 µm spectral regions, the primary absorption lines used here to quantify HDO are in the 8 µm region. There are other HDO (and H2O) lines available to use from the AIRS radiance but for now we only use the 8 µm region to ensure consistency between AIRS and TES data. Figure 1a shows the 8 µm radiance and the Jacobian, or sensitivity of the radiance to variations in the (log) H2O and (log) HDO, respectively (Fig. 1b, c). These Jacobians are normalized by the instrument noise. For example, a value of 1 means that it would take a 100 % change in the corresponding species to distinguish between two similar radiances (everything about the observed scene and radiance is the same except for the species of interest) above the noise level. A value of therefore means that only a 2 % variation is required (or 1∕50).
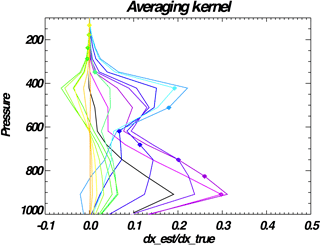
Figure 2The rows of the averaging kernel matrix for the HDO retrieval corresponding to the radiance shown in Fig. 1. The different colors and symbols are used to indicate the pressure levels corresponding to each row of the averaging kernel matrix.
Figure 2 shows the averaging kernel matrix for the HDO component of the joint retrieval. The averaging kernel describes the response of the estimate, or log(HDO), relative to variations in the true state; consequently it can also be used to evaluate the vertical resolution and sensitivity of the estimate. For example, if HDO varies by 100 % at 908 hPa, then the AIRS estimate would be able to observe about 30 % of the variability because the averaging kernel is approximately 0.3 at that level. The averaging kernel at 908 hPa also depends on the deuterium content at several other pressure levels below and above, indicating that the estimate at 908 hPa depends on the deuterium content variations at these other levels. Not shown are the dependencies of the (log) HDO estimate on those from the (log) H2O estimate. These dependencies between the HDO averaging kernels and with the H2O averaging kernels are accounted for when constructing the HDO∕H2O ratio; however a residual uncertainty called the “smoothing” error is imparted when comparing the HDO∕H2O ratio to independent data; this smoothing error is part of the error budget shown in Fig. 3. As discussed in Worden et al. (2012) and Schneider et al. (2012), the sensitivity of the estimated HDO∕H2O ratio is limited by the sensitivity of the estimate to HDO. Users of these data should note that this ratio is typically used with that of H2O in order to better evaluate their joint variation (HDO∕H2O, H2O) against simple mixing and rainfall models (Noone et al., 2011). However, the sensitivity of the radiance to H2O variations is much stronger than that for HDO, although the altitude region of the HDO sensitivity typically overlaps with the H2O sensitivity. Schneider et al. (2012) discuss how to created HDO∕H2O, H2O pairs to mitigate this component of the smoothing error when comparing these data against the simple models described in Noone et al., (2011). For comparison to more complex global climate models the user of these data also needs to apply the HDO and H2O averaging kernels to the corresponding model fields (e.g., Risi et al., 2012).
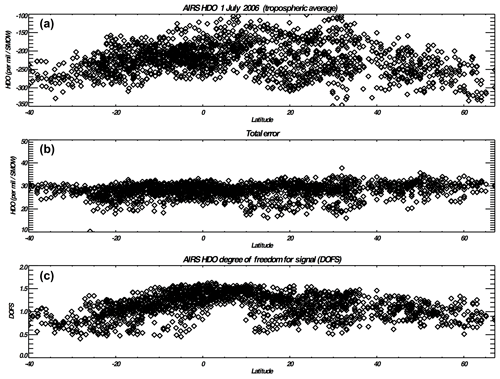
Figure 3(a) The mean tropospheric deuterium content (in “per mil” or units of parts per thousand relative to the deuterium content of the ocean or SMOW) for 1 June 2006 as inferred from AIRS radiance measurements. (b) The total error for the measurements in (a) (also in units of per mil relative to SMOW). (c) The DOFS for the retrieval.
Figure 3a shows the tropospheric deuterium content (or HDO∕H2O ratio) derived from AIRS observations on 1 July 2006. Despite the improved computational performance of the OSS radiative transfer calculation relative to the TES algorithm line-by-line calculation (Clough et al., 2005), the retrieval is still sufficiently expensive such that we can only process a subset of the AIRS retrievals. Considering the computational cost, for the purpose of constructing a record we currently only process AIRS retrievals from between 45∘ S and 65∘ N that coincide with the nearest TES observation but with an additional two observations within 100 km of the TES track over the continents; this ad hoc sampling strategy is based on experience with previous studies using the TES deuterium and methane measurements. The traditional notation for this quantity is called “delta-D” , or “δ-D” with units of “per mil” or parts per thousand relative to the Standard Mean Ocean Water (SMOW) deuterium content, which is molecules of HDO per molecule of H2O. The observations shown represent the deuterium content for the pressures between 750 and 350 hPa, where we find the AIRS and TES observations have maximal overlap in their vertical resolution.
The errors are calculated during the optimal estimation retrieval (Bowman et al., 2006; Worden et al., 2012) and depend on the expected noise of the AIRS radiances and the parameters that are co-retrieved with the AIRS HDO∕H2O ratio such as temperature, surface emissivity, clouds, and methane. As noted in Worden et al. (2012) these co-retrieved parameters affect both the precision and accuracy whereas the noise only affects the precision. The total error (Fig. 3b) is given in units of per mil and ranges between 25 ‰ and 30 ‰. The DOFS, or trace of the averaging kernel, are shown in Fig. 3c and indicate that many of the HDO∕H2O retrievals can resolve different parts of the troposphere, at least in the tropics, because (as demonstrated in Fig. 2) the rows of the averaging kernels are separated between the boundary layer region (surface to ∼750 hPa) and the free troposphere (∼600 to 300 hPa). However, these observations cannot completely resolve the total variability in these two regions of the atmosphere because the total DOFS is typically 1.5 or less and for the measurement to be able to resolve the variability (to within the calculated error) of the two regions there would need to be at least 2 DOFS.
Figure 4 shows a comparison between overlapping AIRS and TES estimates of the HDO∕H2O ratio for 1 June 2006. The AIRS and TES measurements effectively overlap in space and within a few seconds in time as the instruments are in the same orbit. However not all the comparisons shown in Fig. 4 overlap as retrievals may be rejected due to poor quality. We therefore compare all data that are within 200 km in the free troposphere. We do not expect substantive error to occur due to spatial mismatch of 2∘ or less because air parcels in the free troposphere have length scales that are several hundred kilometers long (e.g., Worden et al., 2013). The average between approximately 750 and 350 hPa is shown for when the DOFS are larger than 1 for this altitude region. There is a slight bias of ‰ between TES and AIRS as shown in Fig. 4a. The calculated and actual (RMS difference between AIRS and TES) uncertainties are shown and are approximately 30 ‰, primarily driven by the uncertainty in the AIRS-based estimates as the TES-based estimates have an uncertainty of approximately 15 ‰. Figure 5 shows a direct comparison of the AIRS and TES data. The correlation is about 0.89 and the one-to-one line (solid line) overlaps this distribution. However the lowest values likely diverge from the one-to-one line, possibly because the vertical distribution in the sensitivity depends on the amount of HDO and hence we should expect differences between the TES and AIRS deuterium measurements for these lower-sensitivity retrievals.
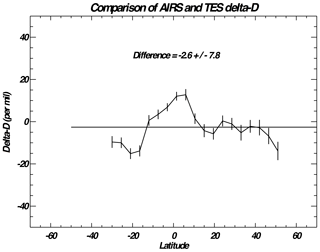
Figure 6The latitudinal differences between TES and AIRS delta-D using co-located observations for 5 d (approximately 600 observations per day) of data, spaced over five Northern Hemisphere summers between 2006 and 2010.
A comparison of the AIRS and TES HDO∕H2O ratio for five single global surveys taken between 2006 and 2010 (one global survey per year during boreal summer) is shown in Table 1 and indicates that the overall bias varies between −2.7 ‰ and 3.7 ‰. Using all five TES global surveys that are summarized in Table 1 we can construct how AIRS and TES compare as a function of latitude as shown in Fig. 6. Figure 6 is constructed by averaging the difference between TES and AIRS observations within 5∘ latitudinal bins. The mean bias across latitudes is ‰. The error bars shown on the difference is the error on the mean, which is the root mean square (RMS) of the differences divided by the square root of the number of co-located observations; as this error bar is a measure of precision for each latitude bin, this comparison demonstrates that there are variations in the comparison that are larger than the precision and are therefore related to systematic errors in either the TES data or AIRS data or both. Variations in these systematic errors can be seen in the latitudinal variability, which has an RMS variation of ∼7.8 ‰ for the different latitude bins but can vary by as much as ‰ to ‰ in the tropics. Typically these variations are due to a combination of uncertainties in the spectroscopy along with temperature, water vapor, and surface properties; they may also be due to smoothing error, which is related to how differences in the vertical resolution affect the tropospheric average of the deuterium content shown in these figures (e.g., Worden et al., 2004). This 7.8 ‰ variation across latitudes is about the same as the reported accuracy of the Aura TES delta-d observations that are based on comparisons of TES data with surface and aircraft measurements (Worden et al., 2011; Herman et al., 2014). We therefore report the current accuracy of the AIRS data to be ∼7.8 ‰. We expect future comparisons between these data and those from aircraft or revisions to the AIRS retrieval approach will modify this estimate of the accuracy.
Table 1Comparison between averaged TES and AIRS HDO∕H2O ratio (750–350 hPa). The units are in parts per thousand relative to Standard Mean Ocean Water. The second column shows the expected RMS based on the uncertainties of the TES and AIRS data. The third column shows the actual RMS difference between TES and AIRS. The last column shows the mean difference.
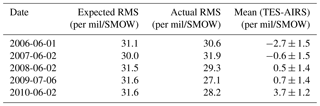
This paper describes the vertical resolution and error characteristics of retrievals of the deuterium content (or the HDO∕H2O ratio) of water vapor using AIRS radiances and then evaluates the consistency between AIRS and TES retrievals of HDO and H2O. We find that the AIRS and TES deuterium content for the lower troposphere (750–350 hPa) is consistent, or within their calculated uncertainties, for the 5-year period in which TES observations span the globe (2006–2010). We find the total uncertainty for a single AIRS observation is ∼30 ‰ with an accuracy of ∼7.8 ‰. These uncertainties can be compared to the observed total variability, which can range from approximately −350 ‰ to −50 ‰ over the whole globe, as observed by the Aura TES data (Worden et al., 2006) and shown in Fig. 3 for AIRS data.
While only 5 d of comparisons are shown here for the purpose of evaluating the retrieval approach and error characteristics of these AIRS retrievals, we expect to produce a record of the AIRS-based deuterium content retrievals from the start of the mission (2002) through the present. Because of computational limitations, we expect to process data from 45∘ S to 65∘ N at approximately 4 times the sampling of the Aura TES measurements and with increased sampling () over the continental regions with the goal of increasing this sampling once the initial record is completed and as additional resources become available.
The Aura TES data are publicly available on the Langley Atmospheric Research Center Data Archive https://eosweb.larc.nasa.gov/ (NASA, 2019). We expect the AIRS-based deuterium data to be publicly released by January 2020. Files in IDL format of the data shown are available from the author upon request: john.r.w.worden@jpl.nasa.gov.
JRW led the study and made the comparisons between TES and AIRS. SSK and DF provided the AIRS retrievals. VHP, KWB and RLH supported initial evaluation of the OSS forward model and HDO∕H2O retrievals. AEL, IP, YH, KCP and JLM provided the OSS forward model needed for the HDO∕H2O retrievals. FWI of the AIRS team supported the adoption of the AIRS data.
The authors declare that they have no conflict of interest.
The research was carried out at the Jet Propulsion Laboratory, California Institute of Technology, under a contract with the National Aeronautics and Space Administration.
This paper was edited by Lars Hoffmann and reviewed by Lars Hoffmann and one anonymous referee.
Alvarado, M. J., Payne, V. H., Mlawer, E. J., Uymin, G., Shephard, M. W., Cady-Pereira, K. E., Delamere, J. S., and Moncet, J.-L.: Supplement to “Performance of the Line-By-Line Radiative Transfer Model (LBLRTM) for temperature, water vapor, and trace gas retrievals: recent updates evaluated with IASI case studies”, Atmos. Chem. Phys., 13, https://doi.org/10.5194/acp-13-6687-2013-supplement, 2013.
Aumann, H. H., Chahine, M. T., Gautier, C., Goldberg, M. D., Kalnay, E., McMillin, L. M., Revercomb, H., Rosenkranz, P. W., Smith, W. L., Staelin, D. H., Strow, L. L., and Susskind, J.: AIRS/AMSU/HSB on the aqua mission: design, science objectives, data products, and processing systems, IEEE T. Geosci. Remote, 41, 253–264, https://doi.org/10.1109/TGRS.2002.808356, 2003.
Bailey, A., Blossey, P. N., Noone, D., Nusbaumer, J., and Wood, R.: Detecting shifts in tropical moisture imbalances with satellite-derived isotope ratios in water vapor, J. Geophys. Res.-Atmos., 122, 5763–5779, https://doi.org/10.1029/2010JD015197, 2017.
Beer, R., Glavich, T. A., and Rider, D. M.: Tropospheric emission spectrometer for the Earth Observing System's Aura satellite, Appl. Optics, 40, 2356–2367, 2001.
Beer, R., Shephard, M. W., Kulawik, S. S., Clough, S. A., Eldering, A., Bowman, K. W., Sander, S. P., Fisher, B. M., Payne, V. H., Luo, M., Osterman, G. B., and Worden, J. R.: First satellite observations of lower tropospheric ammonia and methanol, Geophys. Res. Lett., 35, L09801, https://doi.org/10.1029/2008GL033642, 2008.
Bowman, K. W., Rodgers, C. D., Kulawik, S. S., Worden, J., Sarkissian, E., Osterman, G., Steck, T., Lou, M., Eldering, A., and Shephard, M.: Tropospheric emission spectrometer: Retrieval method and error analysis, IEEE T. Geosci. Remote, 44, 1297–1307, 2006.
Clough, S. A., Shephard, M. W., Mlawer, E. J., Delamere, J. S., Iacono, M. J., Cady-Pereira, K., Boukabara, S., and Brown, P. D.: Atmospheric radiative transfer modeling: A summary of the AER codes, J. Quant. Spectrosc. Ra., 91, 233–244, https://doi.org/10.1016/j.jqsrt.2004.05.058, 2005.
Clough, S. A., Shephard, M. W., Worden, J., Brown, P. D., Worden, H. M., Luo, M., Rodgers, C. D., Rinsland, C. P., Goldman, A., and Brown, L.: Forward model and Jacobians for tropospheric emission spectrometer retrievals, IEEE T. Geosci. Remote, 44, 1308–1323, 2006.
Coudert, L., Wagner, G., Birk, M., Baranov, Y. I., Lafferty, W. J., and Flaud, J.-M.: The (H2O)-O-16 molecule: Line position and line intensity analyses up to the second triad, J. Mol. Spectrosc., 251, 339–357, 2008.
Craig, H.: Isotopic variations in meteoric waters, Science, 133, 1702–1703, 1961.
DeSouza-Machado, S., Strow, L. L., Tangborn, A., Huang, X., Chen, X., Liu, X., Wu, W., and Yang, Q.: Single-footprint retrievals for AIRS using a fast TwoSlab cloud-representation model and the SARTA all-sky infrared radiative transfer algorithm, Atmos. Meas. Tech., 11, 529–550, https://doi.org/10.5194/amt-11-529-2018, 2018.
Divakarla, M., Barnet, C., Liu, X., Gu, D., Wilson, M., Kizer, S., Xiong, X., Maddy, E., Ferraro, R., Knuteson, R., Hagan, D., Ma, X.-L., Tan, C., Nalli, N., Reale, A., Mollner, A. K., Yang, W., Gambacorta, A., Feltz, M., Iturbide-Sanchez, F., Sun, B., and Goldberg, M.: The CrIMSS EDR Algorithm: Characterization, Optimization, and Validation, J. Geophys. Res.-Atmos., 119, 4953–4977, https://doi.org/10.1002/2013JD020438, 2014.
Eldering, A., Kulawik, S. S., Worden, J., Bowman, K., and Osterman, G.: Implementation of cloud retrievals for TES atmospheric retrievals: 2. Characterization of cloud top pressure and effective optical depth retrievals, J. Geophys. Res.-Atmos., 113, D16S37, https://doi.org/10.1029/2007JD008858, 2008.
Frankenberg, C., Yoshimura, K., Warneke, T., Aben, I., Butz, A., Deutscher, N., Griffith, D., Hase, F., Notholt, J., Schneider, M., Schrijver, H., and Rockmann, T.: Dynamic Processes Governing Lower-Tropospheric HDO∕H2O Ratios as Observed from Space and Ground, Science, 325, 1374–1377, https://doi.org/10.1126/science.1173791, 2009.
Frankenberg, C., Wunch, D., Toon, G., Risi, C., Scheepmaker, R., Lee, J.-E., Wennberg, P., and Worden, J.: Water vapor isotopologue retrievals from high-resolution GOSAT shortwave infrared spectra, Atmos. Meas. Tech., 6, 263–274, https://doi.org/10.5194/amt-6-263-2013, 2013.
Fu, D., Worden, J. R., Liu, X., Kulawik, S. S., Bowman, K. W., and Natraj, V.: Characterization of ozone profiles derived from Aura TES and OMI radiances, Atmos. Chem. Phys., 13, 3445–3462, https://doi.org/10.5194/acp-13-3445-2013, 2013.
Fu, D., Bowman, K. W., Worden, H. M., Natraj, V., Worden, J. R., Yu, S., Veefkind, P., Aben, I., Landgraf, J., Strow, L., and Han, Y.: Supplement to “High-resolution tropospheric carbon monoxide profiles retrieved from CrIS and TROPOMI”, Atmos. Meas. Tech., 9, https://doi.org/10.5194/amt-9-2567-2016-supplement, 2016.
Fu, D., Kulawik, S. S., Miyazaki, K., Bowman, K. W., Worden, J. R., Eldering, A., Livesey, N. J., Teixeira, J., Irion, F. W., Herman, R. L., Osterman, G. B., Liu, X., Levelt, P. F., Thompson, A. M., and Luo, M.: Supplement to “Retrievals of tropospheric ozone profiles from the synergism of AIRS and OMI: methodology and validation”, Atmos. Meas. Tech., 11, https://doi.org/10.5194/amt-11-5587-2018-supplement, 2018.
Galewsky, J., Larsen, H. S., Field, R. D., Worden, J. R., Risi, C., and Schneider, M.: Stable isotopes in atmospheric water vapor and applications to the hydrologic cycle, Rev. Geophys., https://doi.org/10.1002/2015RG000512, 2016.
Good, S. P., Noone, D., and Bowen, G.: Hydrologic connectivity constrains partitioning of global terrestrial water fluxes, Science, 349, 175–177, https://doi.org/10.1126/science.aaa5931, 2015.
Han, Y., Revercomb, H., Cromp, M., Gu, D., Johnson, D., Mooney, D., Scott, D., Strow, L., Bingham, G., Borg, L., Chen, Y., DeSlover, D., Esplin, M., Hagan, D., Jin, X., Knuteson, R., Motteler, H., Predina, J., Suwinski, L., Taylor, J., Tobin, D., Tremblay, D., Wang, C., Wang, L., Wang, L., and Zavyalov, V.: Suomi NPP CrIS measurements, sensor data record algorithm, calibration and validation activities, and record data quality, J. Geophys. Res.-Atmos., 118, 12734–12748, https://doi.org/10.1002/2013JD020457, 2013.
Herman, R. L., Cherry, J. E., Young, J., Welker, J. M., Noone, D., Kulawik, S. S., and Worden, J.: Aircraft validation of Aura Tropospheric Emission Spectrometer retrievals of HDO∕H2O, Atmos. Meas. Tech., 7, 3127–3138, https://doi.org/10.5194/amt-7-3127-2014, 2014.
Irion, F. W., Kahn, B. H., Schreier, M. M., Fetzer, E. J., Fishbein, E., Fu, D., Kalmus, P., Wilson, R. C., Wong, S., and Yue, Q.: Single-footprint retrievals of temperature, water vapor and cloud properties from AIRS, Atmos. Meas. Tech., 11, 971–995, https://doi.org/10.5194/amt-11-971-2018, 2018.
Kulawik, S. S., Worden, J., Eldering, A., Bowman, K., Gunson, M., Osterman, G. B., Zhang, L., Clough, S. A., Shephard, M. W., and Beer, R.: Implementation of cloud retrievals for Tropospheric Emission Spectrometer (TES) atmospheric retrievals: part 1. Description and characterization of errors on trace gas retrievals, J. Geophys. Res.-Atmos., 111, D24204, https://doi.org/10.1029/2005JD006733, 2006.
Lacour, J.-L., Risi, C., Clarisse, L., Bony, S., Hurtmans, D., Clerbaux, C., and Coheur, P.-F.: Mid-tropospheric δD observations from IASI/MetOp at high spatial and temporal resolution, Atmos. Chem. Phys., 12, 10817–10832, https://doi.org/10.5194/acp-12-10817-2012, 2012.
Lacour, J.-L., Risi, C., Worden, J., Clerbaux, C., and Coheur, P.-F.: Importance of depth and intensity of convection on the isotopic composition of water vapor as seen from IASI and TES δD observations, Earth Planet. Sc. Lett., 481, 387–394, https://doi.org/10.1016/j.epsl.2017.10.048, 2018.
Lamouroux, J., Régalia, L., Thomas, X., Vander Auwera, J., Gamache, R. R., and Hartmann, J.-M.: CO2 line-mixing database and software update and its tests in the 2.1 µm and 4.3 µm regions, J. Quant. Spectrosc. Ra., 151, 88–96, 2015.
Luo, M., Rinsland, C. P., Rodgers, C. D., Logan, J. A., Worden, H., Kulawik, S., Eldering, A., Goldman, A., Shephard, M. W., Gunson, M., and Lampel, M.: Comparison of carbon monoxide measurements by TES and MOPITT: Influence of a priori data and instrument characteristics on nadir atmospheric species retrievals, J. Geophys. Res.-Atmos., 112, D09303, https://doi.org/10.1029/2006JD007663, 2007.
McMillan, W. W., Pierce, R. B., Sparling, L. C., Osterman, G., Mccann, K., Fischer, M. L., Rappenglück, B., Newsom, R., Turner, D., Kittaka, C., Evans, K., Biraud, S., Lefer, B., Andrews, A., and Oltmans, S.: An observational and modeling strategy to investigate the impact of remote sources on local air quality: A Houston, Texas, case study from the Second Texas Air Quality Study (TexAQS II), J. Geophys. Res, 115, D01301, https://doi.org/10.1029/2009JD011973, 2010.
Moncet, J.-L., Uymin, G., Lipton, A. E., and Snell, H. E.: Infrared radiance modeling by optimal spectral sampling, J. Atmos. Sci., 65, 3917–3934, https://doi.org/10.1175/2008JAS2711.1, 2008.
Moncet, J.-L., Uymin, G., Liang, P., and Lipton, A. E.: Fast and accurate radiative transfer in the thermal regime by simultaneous optimal spectral sampling over all channels, J. Atmos. Sci., 72, 2622–2641, https://doi.org/10.1175/JAS-D-14-0190.1, 2015.
NASA: available at: https://eosweb.larc.nasa.gov/, last access: 10 April 2019.
Noone, D., Galewsky, J., Sharp, Z. D., Worden, J., Barnes, J., Baer, D., Bailey, A., Brown, D. P., Christensen, L., Crosson, E., Dong, F., Hurley, J. V., Johnson, L. R., Strong, M., Toohey, D., Van Pelt, A., and Wright, J. S.: Properties of air mass mixing and humidity in the subtropics from measurements of the D∕H isotope ratio of water vapor at the Mauna Loa Observatory, J. Geophys. Res.-Atmos., 116, D22113, https://doi.org/10.1029/2011JD015773, 2011.
Pagano, T. S., Aumann, H. H., Hagan, D. E., and Overoye, K.: Prelaunch and in-flight radiometric calibration of the Atmospheric Infrared Sounder (AIRS), IEEE T. Geosci. Remote, 41, 265–273, 2003.
Pagano, T. S., Aumann, H. H., Schindler, R., Elliott, D., Broberg, S., Overoye, K., and Weiler, M. H.: Absolute radiometric calibration accuracy of the Atmospheric Infrared Sounder (AIRS), Proc. SPIE, 7081, 70811B, https://doi.org/10.1117/12.795445, 2008.
Payne, V. H., Alvarado, M. J., Cady-Pereira, K. E., Worden, J. R., Kulawik, S. S., and Fischer, E. V.: Satellite observations of peroxyacetyl nitrate from the Aura Tropospheric Emission Spectrometer, Atmos. Meas. Tech., 7, 3737–3749, https://doi.org/10.5194/amt-7-3737-2014, 2014.
Risi, C., Noone, D., Worden, J., Frankenberg, C., Stiller, G., Kiefer, M., Funke, B., Walker, K., Bernath, P., Schneider, M., Wunch, D., Sherlock, V., Deutscher, N., Griffith, D., Wennberg, P. O., Strong, K., Smale, D., Mahieu, E., Barthlott, S., Hase, F., García, O., Notholt, J., Warneke, T., Toon, G., Sayres, D., Bony, S., Lee, J., Brown, D., Uemura, R., and Sturm, C.: Process-evaluation of tropospheric humidity simulated by general circulation models using water vapor isotopologues: 1. Comparison between models and observations, J. Geophys. Res.-Atmos., 117, D05303, https://doi.org/10.1029/2011JD016621, 2012.
Rodgers, C. D. and Connor, B. J.: Intercomparison of remote sounding instruments, J. Geophys. Res.-Atmos., 108, 4116, https://doi.org/10.1029/2002JD002299, 2003.
Risi, C., Noone, D., Frankenberg, C., and Worden, J.: Role of continental recycling in intraseasonal variations of continental moisture as deduced from model simulations and water vapor isotopic measurements, Water Resour. Res., 49, 4136–4156, https://doi.org/10.1002/wrcr.20312, 2013.
Rothman, L. S., Gordon, I. E., Babikov, Y., Barbe, A., Benner, D. C., Bernath, P. F., Birk, M., Bizzocchi, L., Boudon, V., Brown, L. R., Campargue, A., Chance, K., Cohen, E. A., Coudert, L. H., Devi, V. M., Drouin, B. J., Fayt, A., Flaud, J. M., Gamache, R. R., Harrison, J. J., Hartmann, J. M., Hill, C., Hodges, J. T., Jacquemart, D., Jolly, A., Lamouroux, J., Le Roy, R. J., Li, G., Long, D. A., Lyulin, O. M., Mackie, C. J., Massie, S. T., Mikhailenko, S., Müller, H. S. P., Naumenko, O. V., Nikitin, A. V., Orphal, J., Perevalov, V., Perrin, A., Polovtseva, E. R., Richard, C., Smith, M. A. H., Starikova, E., Sung, K., Tashkun, S., Tennyson, J., Toon, G. C., Tyuterev, V. G., and Wagner, G.: The HITRAN 2012 Molecular Spectroscopic Database, J. Quant. Spectrosc. Ra., 130, 4–50, 2013.
Schneider, M., Barthlott, S., Hase, F., González, Y., Yoshimura, K., García, O. E., Sepúlveda, E., Gomez-Pelaez, A., Gisi, M., Kohlhepp, R., Dohe, S., Blumenstock, T., Wiegele, A., Christner, E., Strong, K., Weaver, D., Palm, M., Deutscher, N. M., Warneke, T., Notholt, J., Lejeune, B., Demoulin, P., Jones, N., Griffith, D. W. T., Smale, D., and Robinson, J.: Ground-based remote sensing of tropospheric water vapour isotopologues within the project MUSICA, Atmos. Meas. Tech., 5, 3007–3027, https://doi.org/10.5194/amt-5-3007-2012, 2012.
Shephard, M. W. and Cady-Pereira, K. E.: Cross-track Infrared Sounder (CrIS) satellite observations of tropospheric ammonia, Atmos. Meas. Tech., 8, 1323–1336, https://doi.org/10.5194/amt-8-1323-2015, 2015.
Strow, L. L., Hannon, S., Weiler, M., Overoye, K., Gaiser, S. L., and Aumann, H. H.: Prelaunch spectral calibration of the atmospheric infrared sounder (AIRS), IEEE T. Geosci. Remote, 41, 274–286, https://doi.org/10.1109/TGRS.2002.808245, 2003.
Tran, H., Flaud, J.-M., Gabard, T., Hase, F., Von Clarmann, T., Camy-Peyret, C., Payan, S., and Hartmann, J.-M.: Model, software, and database for line-mixing effects in the n3 and n4 bands of CH4 and tests using laboratory and planetary measurements. I. N2 (and air) broadenings and the Earth atmosphere, J. Quant. Spectrosc. Ra., 101, 284–305, 2006.
Warner, J. X., Wei, Z., Strow, L. L., Dickerson, R. R., and Nowak, J. B.: The global tropospheric ammonia distribution as seen in the 13-year AIRS measurement record, Atmos. Chem. Phys., 16, 5467–5479, https://doi.org/10.5194/acp-16-5467-2016, 2016.
Worden, J., Kulawik, S., Shepard, M., Clough, S., Worden, H., Bowman, K., and Goldman, A.: Predicted errors of tropospheric emission spectrometer nadir retrievals from spectral window selection, J. Geophys. Res.-Atmos., 109, D09308, https://doi.org/10.1029/2004JD004522, 2004.
Worden, J., Bowman, K., Noone, D., Beer, R., Clough, S., Eldering, A., Fisher, B., Goldman, A., Gunson, M., Herman, R., Kulawik, S. S., Lampel, M., Luo, M., Osterman, G., Rinsland, C., Rodgers, C., Sander, S., Shephard, M., and Worden, H.: Tropospheric Emission Spectrometer observations of the tropospheric HDO∕H2O ratio: Estimation approach and characterization, J. Geophys. Res.-Atmos., 111, D16309, https://doi.org/10.1029/2005JD006606, 2006.
Worden, J., Noone, D., Bowman, K., Beer, R., Eldering, A., Fisher, B., Gunson, M., Goldman, A., Herman, R., Kulawik, S. S., Lampel, M., Osterman, G., Rinsland, C., Rodgers, C., Sander, S., Shephard, M., Webster, C. R., and Worden, H.: Importance of rain evaporation and continental convection in the tropical water cycle, Nature, 445, 528–532, https://doi.org/10.1038/nature05508, 2007.
Worden, J., Noone, D., Galewsky, J., Bailey, A., Bowman, K., Brown, D., Hurley, J., Kulawik, S., Lee, J., and Strong, M.: Estimate of bias in Aura TES HDO∕H2O profiles from comparison of TES and in situ HDO∕H2O measurements at the Mauna Loa observatory, Atmos. Chem. Phys., 11, 4491–4503, https://doi.org/10.5194/acp-11-4491-2011, 2011.
Worden, J., Kulawik, S., Frankenberg, C., Payne, V., Bowman, K., Cady-Peirara, K., Wecht, K., Lee, J.-E., and Noone, D.: Profiles of CH4, HDO, H2O, and N2O with improved lower tropospheric vertical resolution from Aura TES radiances, Atmos. Meas. Tech., 5, 397–411, https://doi.org/10.5194/amt-5-397-2012, 2012.
Worden, J., Wecht, K., Frankenberg, C., Alvarado, M., Bowman, K., Kort, E., Kulawik, S., Lee, M., Payne, V., and Worden, H.: CH4 and CO distributions over tropical fires during October 2006 as observed by the Aura TES satellite instrument and modeled by GEOS-Chem, Atmos. Chem. Phys., 13, 3679–3692, https://doi.org/10.5194/acp-13-3679-2013, 2013.
Wright, J. S., Fu, R., Worden, J. R., Chakraborty, S., Clinton, N., Risi, C., sun, Y., and Yin, L.: Rainforest-initiated wet season onset over the southern Amazon, P. Natl. Acad. Sci. USA, 114, 8481–8486, https://doi.org/10.1073/pnas.1621516114, 2017.
Xiong, X., Barnet, C., Maddy, E., Sweeney, C., Liu, X., Zhou, L., and Goldberg, M.: Characterization and validation of methane products from the Atmospheric Infrared Sounder (AIRS), J. Geophys. Res, 113, G00A01, https://doi.org/10.1029/2007JG000500, 2008.
Xiong, X., Barnet, C., Maddy, E., Wofsy, S. C., Chen, L. A., Karion, A., and Sweeney, C.: Detection of methane depletion associated with stratospheric intrusion by atmospheric infrared sounder (AIRS), Geophys. Res. Lett., 40, 2455–2459, https://doi.org/10.1002/grl.50476, 2013.
Zhou, L., Tian, Y., Myneni, R. B., Ciais, P., Saatchi, S., Liu, Y. Y., Piao, S., Chen, H., Vermote, E. F., Song, C., and Hwang, T.: Widespread decline of Congo rainforest greenness in the past decade, Nature, 508, 86–90, https://doi.org/10.1038/nature13265, 2014.
- Abstract
- Copyright statement
- Introduction
- Description of AIRS and TES instruments
- Description of the radiative transfer forward model
- Description of the retrieval approach
- Characterization of HDO∕H2O profiles
- Comparison of AIRS and TES HDO∕H2O retrievals
- Conclusion
- Data availability
- Author contributions
- Competing interests
- Acknowledgements
- Review statement
- References
- Abstract
- Copyright statement
- Introduction
- Description of AIRS and TES instruments
- Description of the radiative transfer forward model
- Description of the retrieval approach
- Characterization of HDO∕H2O profiles
- Comparison of AIRS and TES HDO∕H2O retrievals
- Conclusion
- Data availability
- Author contributions
- Competing interests
- Acknowledgements
- Review statement
- References