the Creative Commons Attribution 4.0 License.
the Creative Commons Attribution 4.0 License.
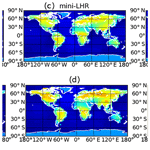
Potential improvements in global carbon flux estimates from a network of laser heterodyne radiometer measurements of column carbon dioxide
Emily L. Wilson
Geronimo L. Villanueva
Giuliano Liuzzi
Liang Feng
Anthony J. DiGregorio
Jianping Mao
Lesley Ott
Bryan Duncan
We present observing system simulation experiments (OSSEs) to evaluate the impact of a proposed network of ground-based miniaturized laser heterodyne radiometer (mini-LHR) instruments that measure atmospheric column-averaged carbon dioxide (XCO2) with a 1 ppm precision. A particular strength of this passive measurement approach is its insensitivity to clouds and aerosols due to its direct sun pointing and narrow field of view (0.2∘). Developed at the NASA Goddard Space Flight Center (GSFC), these portable, low-cost mini-LHR instruments were designed to operate in tandem with the sun photometers used by the AErosol RObotic NETwork (AERONET). This partnership allows us to leverage the existing framework of AERONET's global ground network of more than 500 sites as well as providing simultaneous measurements of aerosols that are known to be a major source of error in retrievals of XCO2 from passive nadir-viewing satellite observations. We show, using the global 3-D GEOS-Chem chemistry transport model, that a deployment of 50 mini-LHRs at strategic (but not optimized) AERONET sites significantly improves our knowledge of global and regional land-based CO2 fluxes. This improvement varies seasonally and ranges 58 %–81 % over southern lands, 47 %–76 % over tropical lands, 71 %–92 % over northern lands, and 64 %–91 % globally. We also show significant added value from combining mini-LHR instruments with the existing ground-based NOAA flask network. Collectively, these data result in improved a posteriori CO2 flux estimates on spatial scales of ∼10 km2, especially over North America and Europe, where the ground-based networks are densest. Our studies suggest that the mini-LHR network could also play a substantive role in reducing carbon flux uncertainty in Arctic and tropical systems by filling in geographical gaps in measurements left by ground-based networks and space-based observations. A realized network would also provide necessary data for the quinquennial global stocktakes that form part of the Paris Agreement.
- Article
(7523 KB) - Full-text XML
- BibTeX
- EndNote
Two recent satellite instruments have made significant contributions to globally characterizing XCO2: the Japanese Greenhouse gases Observing SATellite (GOSAT) or “IBUKI” launched in 2009 (Kuze et al., 2009) and the Orbiting Carbon Observatory-2 (OCO-2; Crisp et al., 2017; Eldering et al., 2017) launched in 2014. Both the Fourier transform spectrometer (FTS) in GOSAT and the grating spectrometer in OCO-2 have multiple viewing geometries (nadir, glint, and target) to observe absorption of XCO2, but OCO-2 offers significant improvements in global surface coverage. While GOSAT and OCO-2 have made important advances in observing greenhouse gases from space, any uncharacterized systematic errors can compromise the accuracy of their data (Wunch et al., 2017) and limit the utility of such data sets for inferring surface flux distributions (Basu et al., 2013). Ground-based networks of accurate and precise XCO2 measurements such as Total Carbon Column Observing Network (TCCON) (Wunch et al., 2017) therefore play an important role in helping to validate these space-borne missions. We describe how we can improve knowledge of the carbon cycle by establishing a network of low-cost, portable mini-LHR (miniaturized laser heterodyne radiometer) instruments that measure XCO2 to fill in gaps left by existing column ground-based networks and space-borne observations. These instruments can be quickly deployed (to be collecting data within a few hours) and can run autonomously in the field with little or no maintenance over a period of months or years.
Ground-based, broad spectral column measurements of XCO2 from the TCCON FTS network have been used to minimize regional systematic errors and serve as a gold standard to validate satellite measurements. In 2010, the TCCON FTS instruments reported an accuracy of ∼1 ppm due to bias errors from uncertainties in spectroscopic parameters (Wunch et al., 2010). They resolved this limitation at five of their sites by tying their column-averaged dry-air mole fractions to the World Meteorological Organization (WMO) in situ trace gas measurement scales using aircraft profiles and indicated that they planned to eventually perform similar calibrations at the remainder of their sites. While TCCON products are well characterized, the majority of the 32 TCCON sites are in the Northern Hemisphere, leaving important monitoring gaps in regions where our knowledge of the drivers of carbon cycling is uncertain (Shuur et al., 2008; Commane et al., 2017; Saunois et al., 2016; Le Quéré et al., 2016).
The NASA mini-LHRs are designed to be deployed in tandem with AErosol RObotic NETwork (AERONET) sun photometers (Holben et al., 1998), taking advantage of their sun trackers. This partnership provides a pathway for establishing a global network of mini-LHRs by leveraging AERONET's network of over 500 sites and offers a simultaneous measure of aerosol optical depth (AOD) that is a necessary input for satellite retrievals (Butz et al., 2009). Similar to TCCON, mini-LHRs can collect data during breaks in cloud coverage, thereby offering the potential for new data products in formerly under-represented regions such as the Amazon Basin, southern Asian monsoon areas, and the Arctic. These vulnerable geographic regions are not well covered by OCO-2 and GOSAT. Here, using numerical experiments, we simulate a strategic (but not optimized) deployment of 50 mini-LHR instruments to AERONET sites and evaluate how this increase in measurement density impacts knowledge of regional and global carbon fluxes.
The mini-LHR is a ground-based, passive, sun-viewing instrument that observes trace gases in the atmospheric column. It has been under development at the NASA Goddard Space Flight Center (GSFC) since 2009 (Melroy et al., 2015; Clarke et al., 2014; Wilson et al., 2014; Wilson and McLinden, 2012), and while earlier versions exclusively measured XCO2, the current version observes both XCO2 and XCH4. Current challenges associated with our understanding of emissions of CH4 (Wolf et al., 2017) will result in a different network design. The mini-LHR has been tested at altitudes ranging from sea level to 3400 m and in climates that include tropical, subtropical, and temperate zones, extending to just below the Arctic Circle, and has shown consistent precisions of 1 ppm in XCO2 and 10 ppb in XCH4 for hourly data products. Figure 1 shows a mini-LHR monitoring XCO2 and XCH4 over thawing permafrost at a remote site in the Bonanza Creek Research Forest near Fairbanks, Alaska. The goal of these field tests was to both improve the quality of the data product as well as to test the durability of commercial components that were intended for indoor lab use.
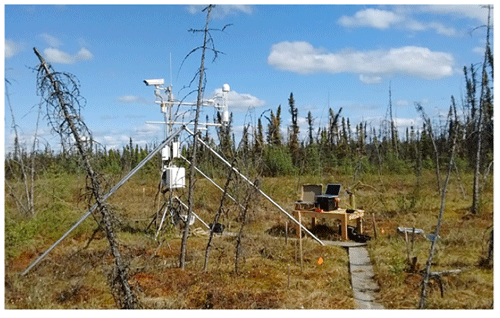
Figure 1The mini-LHR (right) is portable and can be deployed to remote locations where larger TCCON installations are not possible due to the fragile ground conditions. Shown here are the mini-LHR monitors XCO2 and XCH4 alongside an eddy-covariance tower (left) in a collapse-scar bog permafrost site in Alaska.
The mini-LHR measures XCO2 by scanning the CO2 absorption feature near 1.61 µm. Figure 2 shows the current configuration of the system, and Table 1 lists key system parameters. Sunlight is collected with a fibre-coupled, 0.2∘ field-of-view collimator that is non-invasively connected to an AERONET sun tracker. Once collected, sunlight is modulated with a fibre switch, superimposed with infrared laser light from a distributive feedback laser in a single-mode fibre coupler, and then mixed in a fast photoreceiver and InGaAs detector to produce an radio frequency (RF) beat signal. The RF receiver separates RF and direct current (DC) outputs, and the RF signal is amplified, filtered, and then detected with a square-law detector. The resulting signal is measured with a lock-in amplifier referenced to the fibre switch frequency as the laser scans across an absorption feature. A microprocessor controls the laser scanning and data collection. The mini-LHR has spectral sampling resolution of ∼0.013 cm−1, which is 15 times higher than GOSAT (∼0.2 cm−1), 20 times higher than OCO-2 (∼0.3 cm−1), and slightly higher than TCCON (∼0.02 cm−1). Individual scans of the CO2 feature are collected at 2 min intervals throughout the day during sunlight hours when clouds are not present and averaged into hourly data products.
Table 1Parameters used to calculate mini-LHR averaging kernels for the Bonanza Creek site. The molecules analysed in the 72-layer atmosphere included H2O, CO2, O3, N2O, CO, CH4, O2, and N2, and the level of variability was 0.05.
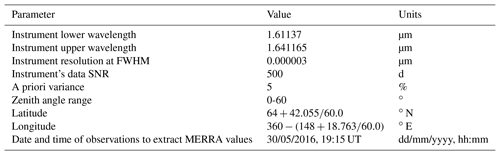
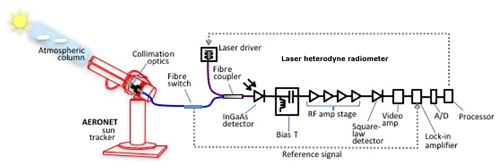
Figure 2Schematic of a mini-LHR. Sunlight is collected with collection optics that are non-invasively connected to the AERONET sun tracker. Sunlight is then modulated with a fibre switch, superimposed with infrared laser light from a distributive feedback laser in a single-mode fibre coupler, and mixed in a fast photoreceiver and InGaAs detector to produce a radio frequency (RF) beat signal. In the RF receiver (custom), a bias tee separates RF and DC outputs. The RF signal passes through a gain stage and is then detected with a square-law detector. The signal is measured with a lock-in amplifier that is referenced to the modulation frequency as the laser scans across an absorption feature. A microprocessor controls the laser scanning and data collection.
Averaged absorption scans are analysed to extract column mole fractions of CO2 using custom analysis software developed at GSFC that is similar to the approach used by TCCON. There are two main steps involved in processing data: (1) simulating the spectra (mathematically simulating what the mini-LHR observes in the atmosphere) and (2) fitting the simulation to the data to extract the abundance of XCO2.
We simulate the spectra using the Planetary Spectrum Generator (PSG), which is an online tool developed at NASA–GSFC (Villanueva et al., 2015, 2016) for synthesizing Earth and planetary spectra (atmospheres and surfaces) for a broad range of wavelengths (0.1 µm to 100 mm, spanning UV to radio wavelengths) from any observatory, orbiter, or lander. This is achieved by combining several state-of-the-art radiative transfer models, spectroscopic databases, and planetary databases. The PSG code includes refraction of sunlight through the atmosphere as well as a computationally efficient scattering package that incorporates the latest radiative transfer numerical methods (Villanueva et al., 2015; Smith et al., 2009) and is parameterized for LTE (local thermodynamic equilibrium) calculations. While scattering is not required for direct sun-viewing measurements, the scattering package contains a treatment of aerosols; the extinction portion of this treatment is needed to properly model the continuum shape. The PSG is operated remotely by employing a versatile online application program interface (API). The API operates by sending a configuration file to the PSG servers. Upon reception of the configuration file, the PSG computes and returns the spectra.
Part of this simulation includes the Modern-Era Retrospective analysis for Research and Applications, Version 2 (MERRA-2) data set, which provides meteorological inputs (Reichle et al., 2011; Rienecker et al., 2011) and provides a 72-layer model of the atmosphere. The retrieval employs the MERRA-2 database to define the state and a priori values for the atmosphere. We “perturb” the CO2 profile by a scaler, which is the value that is actually being retrieved by the retrieval algorithm. MERRA is the Modern-Era Retrospective Analysis for Research and Applications database, which is the latest atmospheric reanalysis of the modern satellite era produced by NASA's Global Modeling and Assimilation Office. MERRA has incorporated information from hundreds of orbiters and ground stations since 1980 and provides global three-dimensional analyses of atmospheric parameters (e.g. temperature, abundance profiles, and aerosols). Specifically, our retrieval works with the M2I3NVASM component, which provides assimilated meteorological fields (pressure, temperature, water vapour, ozone, and water ice clouds) from the surface to ∼80 km (72 layers), with a cadence of 180 min and spatial resolution of ∼0.5∘ (576×361). The values are further refined temporally and spatially to a resolution of better than 1 km, employing the USGS-GTOPO30 topographic maps and considering a hydrostatic equilibrated atmosphere within every bin. Our code computes temperature (T) and pressure (P) abundances for Earth by first selecting a set of six standard profiles based on season and latitude: “Tropical”, “Midlatitude-Summer”, “Midlatitude-Winter”, “Subarctic-Summer”, “Subarctic-Winter”, and “US-Standard” (Anderson et al., 1986). These profiles provide abundances for a myriad of species and basic temperature and pressure profiles. The code then extracts P, T, O3, H2O, and water ice abundances from the MERRA-2 database for this location and time. The MERRA-2 grid is described on a coarse grid, and it does not contain fine elevation information; therefore the GTOPO30 topography database (∼1 km resolution) is also used to derive the exact elevation of the mini-LHR site location. The information from MERRA-2 at a particular geolocation is then refined in elevation, e.g. using scale heights, using this high-resolution topographic map.
Our code generates an initial configuration file that establishes the location, date, and time of the measurement. Using this configuration file, the code calls the PSG–API, and this returns all of the geometry parameters (air mass, phase angle, etc.) and an a priori vertical profile based on the date and location. Then, using this configuration file, the program goes into the fitting routine that calls the PSG–API to calculate spectra by fitting the CO2 abundance using an optimal estimation approach. The fit perturbs the CO2 abundance and obtains a fit based on the Levenberg–Marquardt algorithm, which is an iterative least-squares curve-fitting procedure.
Calibration and validation of mini-LHR data
Mini-LHR instruments periodically undergo a calibration–validation procedure at NASA–GSFC to track performance and establish documented traceability of column data products. In particular, we calculate and report measurement precision, measurement error, and measurement bias, as defined by the Vocabulaire International de Métrologie (VIM; Meaures, 2012).
We estimate measurement precision (standard deviation) by routine laboratory calibrations. In the calibration procedure, the mini-LHR instrument scans a NIST (National Institute of Standards and Technology) traceable atmospheric mixture of gases (NIST Traceable Reference Material Program for Gas Standards, https://doi.org/10.6028/NIST.SP.260-126rev2013) in a 36 m Herriot absorption cell. The NIST traceable atmospheric mixture of gases fulfils the criteria of a measurement standard with a negligible measurement uncertainty. The calibration gas standards are specified in the Code of Federal Regulations (CFR) to calibrate instruments used to monitor regulated emissions. The standard deviation of the points in the scan provides an estimate of precision, while regular calibrations track any measurement bias (systematic measurement error).
To assess accuracy of the mini-LHR during its development, two short-duration side-by-side comparisons were completed between the mini-LHR and TCCON stations at Park Falls, Wisconsin, in 2012 and at Caltech in Pasadena, California, in 2014. Sample scans from these comparisons are shown in Fig. 3 with fits from the PSG retrieval. Data from 2014 showed a significant improvement in agreement with the TCCON CO2 measurements because it was possible to collect more scans within a shorter timeframe; the 2012 data are the average of three scans collected over the period of 1 h and the 2014 data are the average of five scans collected over the period of a half hour. A longer-duration side-by-side comparison is planned with the TCCON located at the NASA Armstrong Flight Research Center (AFRC). In addition to laboratory calibrations, potential bias between mini-LHR instruments will also be addressed by regularly comparing a “standard” mini-LHR that is co-located at the NASA–AFRC TCCON with all other mini-LHR instruments. TCCON FTS instruments measure column CH4 and CO2 at the same wavelengths but at lower resolution than the mini-LHR. While TCCON has a well-documented history of characterization, we refer to this as an “estimate” of measurement error due to differences in resolution and because there are known biases between TCCON sites (1 % for CO2 at US sites and 1.1±0.2 % at European sites; Wunch et al., 2010).
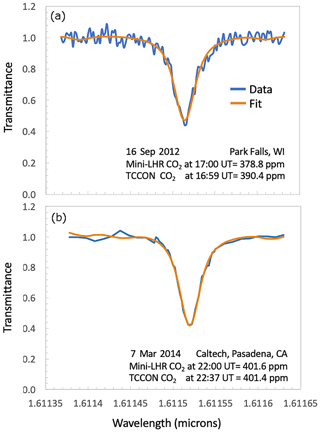
Figure 3Side-by-side comparisons of mini-LHR and TCCON instruments (a) at Park Falls on 16 September 2012 and (b) at Caltech on 7 March 2014. Mini-LHR data are shown in blue with the PSG retrieval fit in orange. The 2012 data are the average of three scans collected over the period of an hour, and the 2014 data are the average of five scans collected over the period of a half hour. The resulting XCO2 value for the mini-LHR and the nearest corresponding value for TCCON are shown in the inset.
For passive satellite observations, scattering from clouds and aerosols is known to be a significant source of retrieval error for XCO2 (Mao and Kawa, 2004; Aben et al., 2007; Uchino et al., 2012; Yoshida et al., 2013). This is primarily because these are nadir-pointing instruments that view sunlight reflected on a portion of ground that is illuminated by direct sunlight as well as scattered sunlight from clouds and aerosols. In contrast, ground-passive measurements have a narrow field of view (FOV) and point directly at the sun. The TCCON at Park Falls, Wisconsin, for example, has an FOV of ∼0.14∘, and mini-LHRs have an FOV of ∼0.2∘ (compared to the sun which has a field of view of ∼0.5∘). Because the FOVs of these instruments are narrower than those of the sun, their light collection optics do not accept the scattered light outside of this FOV. Consequently, the mini-LHR and TCCON are mainly impacted by extinction, resulting in lower levels of sunlight reaching these ground instruments and lower signal-to-noise levels. Solar intensity variations that impact TCCON are corrected by dividing the interferograms by the unmodulated DC signal (Keppel-Aleks et al., 2007). For the mini-LHR, transmittance scans are corrected for extinction by dividing scans by a fitted baseline that tracks fluctuations in solar irradiance.
We use numerical experiments to provide an upper limit on the theoretical potential of the proposed network on reducing the uncertainty of regional carbon flux estimates. The approach we take is to define a closed-loop experiment, often called observing system simulation experiments (OSSEs), in which we define the true atmospheric state using a global 3-D model of atmospheric chemistry and transport driven by true surface fluxes. This true atmospheric state is then sampled as it would be by the mini-LHRs (e.g. time, location, and vertical sensitivity). We then generate a complementary set of model values that are generated from an independent surface flux inventory, including differences in the magnitude and distribution of fluxes; we use this independent inventory as our a priori for the OSSEs. We infer the a posteriori fluxes from measurements using an ensemble Kalman filter.
We use version 9.02 of the GEOS-Chem model of atmospheric chemistry and transport (http://geos-chem.org, last access: 24 April 2019) driven by GEOS-5-analysed meteorological fields that include a simulation of atmospheric CO2 that has been evaluated with a range of ground-based, aircraft, and satellite observations (Feng et al., 2009, 2011, and 2016). For our experiments, we use the model at a horizontal resolution of 4∘ latitude × 5∘ longitude for an arbitrary year, which is 2014 in our experiments. We use monthly ODIAC fossil fuel emissions (Oda and Maksyutov, 2011), monthly ocean biosphere fluxes (Takahashi et al., 2009), and weekly biomass burning emissions from the Global Fire Emission Database (GFEDv3; van der Werf et al., 2010).
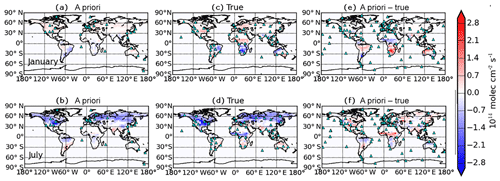
Figure 4Distribution of land biosphere CO2 fluxes (1014 molec cm−2 s−1) for January (a, c, e) and July (b, d, f) in our study year, described on the 4∘ latitude × 5∘ longitude GEOS-Chem model grid. (a, b) show the CASA model that we used as our a priori, (c, d) show output from the ORCHIDEE land surface model that we use to define the true state, and (e, f) show the difference between ORCHIDEE and the CASA model. The cyan triangles represent observation sites from (1) current and future TCCON networks (a, b), (2) the enhanced mini-LHR network (c, d), and (3) the subset of the NOAA ground-based network (e, f).
Currently, it is common practice to assume that most of the uncertainty in atmospheric CO2 stems from natural fluxes, so other sources are typically assumed to be well described by existing inventories (e.g. Gurney et al., 2003). While this practice is slowly being challenged by the community, we retain these assumptions for the purpose of our theoretical calculations. To define our true atmospheric state, we use three-hourly land biosphere fluxes from the ORCHIDEE land surface model (Krinner et al., 2005); in a separate model calculation, to define our a priori state, we use three-hourly land biosphere fluxes from CASA (Olsen and Randerson, 2004). Figure 4 shows that there are significant seasonal differences in the magnitudes and distributions of ORCHIDEE and CASA land biosphere CO2 fluxes so that our OSSE provides a rigorous test of the theoretical data.
For the purposes of inter-comparability of impacts of different data, we have ignored any source of systematic error in the different measurements or the transport model that links a priori information to 4-D atmospheric mole fractions of CO2. Even sub-parts-per-million levels of uncharacterized systematic error in atmospheric measurements will significantly compromise our ability to infer unbiased regional CO2 flux estimates.
Our retrieval simulation requires a matrix of averaging kernels (A) that describes the sensitivity of the retrieved state vector (in this case, a vector describing the vertical profile of CO2 described over n atmospheric layers) to the “true” state vector x, for different values of solar zenith angle throughout the day. Using the standard convention, upper-case and lower-case emboldened variables denote a matrix and vector, respectively, and superscripts −1 and T denote matrix inverse and transpose operations, respectively. The averaging kernel is calculated as (Rodgers, 2000; Liuzzi et al., 2016)
where Sa is the a priori error covariance matrix (size n×n), Sϵ is the measurement error covariance matrix (size m×m, where m denotes the length of the radiance vector), and K is the matrix of weighting functions that describes the derivative of the radiance with respect to a change in the CO2 profile (size m×n). The matrix of averaging kernel is used here to describe the instrument sensitivity to changes in CO2 so that we can simulate mini-LHR XCO2 column measurements in GEOS-Chem. For simplicity, we assume that Sa and Sϵ are diagonal and represent the square of the background variability of CO2 concentration in each atmospheric layer and the square of the instrument noise, respectively.
The sum of the rows of A corresponds to summing the retrieval sensitivities to the CO2 in each atmosphere layer and describes the sensitivity of the atmospheric column to a change in atmospheric CO2 in the vertical profile, i.e. the column averaging kernel a. In the ideal case, each summed row would be close to unity. Figure 5 shows that for a variety of solar zenith angles, a >0.8 in the troposphere but falls off quickly in the stratosphere, consistent with TCCON averaging kernels (Wunch et al., 2011).
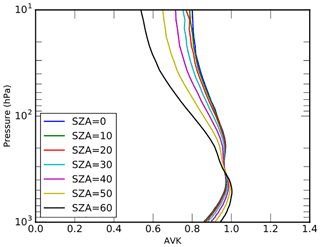
Figure 5Averaging kernel () values for CO2 for different values of solar zenith angles. Calculations for CO2 have been done assuming a mini-LHR SNR of 500 (although in reality SNR varies with solar zenith angle (SZA) and is highest near an SZA of 0) and assuming a background variability of 5 %.
We also calculate the number of degrees of freedom (DOF) of the retrieval as the trace of A, which estimates the number of independent pieces of information that can be derived from retrieval. In the case of the column XCO2 retrieval, this should be close to or higher than 1; values less than 1 indicate the influence of a priori information (Camy-Peyret et al., 2017). We find that with an signal-to-noise ratio (SNR) value of 500 and an assumed a priori variance of 5 %, for CO2, concentrations result in a DOF between 0.88 and 2.10.
To infer regional fluxes of CO2 from the measurements, we use an established ensemble Kalman filter approach (Feng et al., 2009, 2016, 2017). For brevity, we refer the reader to Feng et al. (2009) for a detailed description of the approach and its application within GEOS-Chem. We adopt a uniform 50% a priori uncertainty and assume a conservative 1.5 ppm for individual measurement and model transport errors. To characterize the impact of the mini-LHR measurements on the a priori knowledge we use a metric that describes how uncertainty of fluxes has reduced after the a priori has been informed by the following measurements: , where and denote the diagonal elements of the a posteriori and a priori CO2 flux error covariance matrices, respectively. A larger value for γ denotes a larger scientific impact of the observations. We also report comparisons between true, a priori, and a posteriori CO2 fluxes over key geographical regions. Together, our use of two independent land biosphere flux inventories, the γ metric and the inter-comparison of fluxes provide a transparent theoretical assessment of the LHR data to quantify geographical fluxes of CO2.
We performed three experiments to estimate true CO2 fluxes using the following: (1) TCCON measurements with their current measurement configuration (Fig. 4), (2) mini-LHR measurements collected at an enhanced number (50) of sites, and (3) the combined data sets from mini-LHR measurements and selected surface flask sites to study the added value of mini-LHR data to the existing NOAA Earth System Research Laboratory ground-based network of mole fraction data that is commonly used to infer regional CO2 fluxes (e.g. Peylin et al., 2013). We conservatively assume a mini-LHR measurement precision of 1.5 ppm in our experiments; however, the current precisions for the mini-LHR and TCCON data products are <1 ppm for 1 h data products (Wunch et al., 2010; Messerschmidt et al., 2011; Wilson et al., 2017; Melroy et al., 2015). Instrument biases were not included in these OSSE runs because our focus is the relative performance of different ground-based remote-sensing networks.
Table 2 lists the proposed enhanced distribution of mini-LHR instruments. These sites were initially chosen to target regions where AERONET sites already existed and where there are gaps in the existing in situ measurements, TCCON measurements, and satellite observations. Consideration was also given to accessibility, acknowledging evolving political environments. Consequently, the enhanced network has not been optimized to minimize carbon flux uncertainties.
Table 3 reports the TCCON sites used in our numerical experiments. We simulate TCCON XCO2 observations using the same approach as we use for the mini-LHR instruments. For TCCON, we use CO2 averaging kernels from the latest TCCON XCO2 retrievals of version GCC2014 (Wunch et al., 2011).
Table 4 shows the surface flask sites used in our joint assimilation experiment. They are a subset of the NOAA ground-based network and are chosen mainly based on their data continuity in recent years (i.e. 2009–2016). In our experiments, we use the real availability of the flask data in the compiled surface data set (GLOBALVIEW-v3.2) while simulating the observation values by sampling model surface CO2 concentrations at the observation location and time.
Figure 6 compares the annual mean deviation between true (ORCHIDEE) and a posteriori fluxes inferred from the TCCON, the enhanced mini-LHR network, and the mini-LHR and NOAA flask observations. We acknowledge that the primary purpose of TCCON is to provide a ground truth for satellite observations, which are not optimized for surface flux estimation. Nevertheless, we find that the current TCCON network can generally reduce the systematic bias between the a priori and the true state, particularly over the northern mid-latitude land region that reflects observation coverage. As expected, the uneven and coarse coverage over tropical and southern land regions results in a large-scale dipole effect between tropical South America and other tropical and extratropical regions, e.g. Australia. Sensitivity experiments (not shown) show that the performance of the mini-LHR instruments distributed using the current TCCON measurement configuration is comparable with the TCCON instruments, despite larger assumed random errors. We find this is primarily due to the comparable role of instrument and atmospheric transport model errors (1.5 ppm), particularly at the 4∘ latitude × 5∘ longitude horizontal resolution employed here. Using finer-scale meteorology could very well reduce model error, but knowledge of this error is poorly defined, with no robust quantitative method currently available.
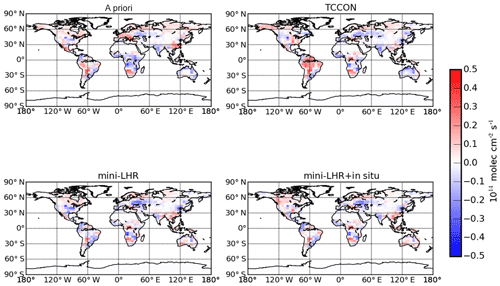
Figure 6Distribution of annual mean residuals that describe the true state minus a priori and a posteriori CO2 fluxes (1014 molec cm−2 s−1), described on the 4∘ latitude × 5∘ longitude GEOS-Chem model grid.
Table 2List of 50 selected AERONET and NASA sites with mini-LHR installed in the future for the OSSE study.
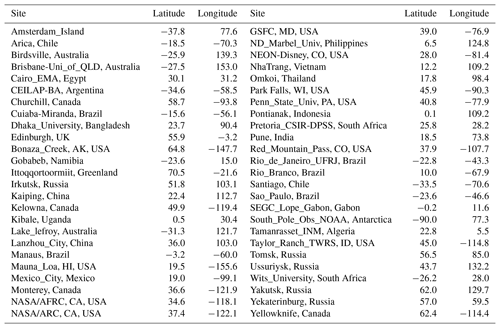
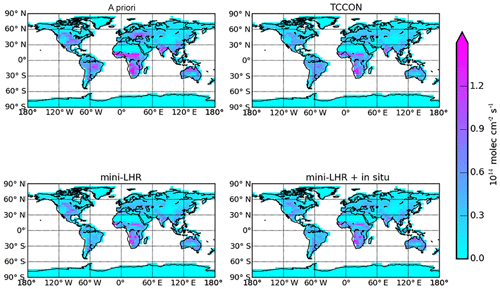
Figure 7Distribution of annual mean root-mean-square errors between the true state and a priori and a posteriori CO2 fluxes (1014 molec cm−2 s−1), described on the 4∘ latitude × 5∘ longitude GEOS-Chem model grid.
A posteriori fluxes inferred from the enhanced mini-LHR network significantly improve agreement with true fluxes compared to TCCON measurement configuration, particularly over tropical land regions. Annual mean deviations are generally smaller than 2×1013 molec cm−2 s−1 and vary on the grid scale throughout the year for many continental regions. We find that including the NOAA flask data helps with reducing these variations, in particular over North America and Europe, where the coverage by the surface data is densest (Fig. 6). Figure 7 compares the root-mean-square error (RMSE) for our three inversion experiments relative to the true state. First, all our inversions show much smaller deviations compared to the a priori values. The enhanced mini-LHR network performs significantly better over tropical lands such as tropical South America and tropical North Africa.
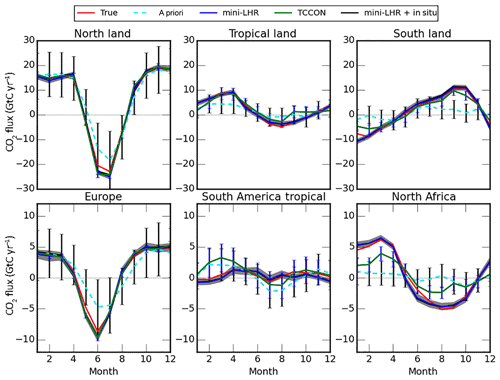
Figure 8True, a priori, and a posteriori monthly CO2 fluxes (GtC yr−1), described over large-scale geographical regions for our study year. Vertical lines and the grey shading denote uncertainties associated with the a priori fluxes and the a posteriori fluxes inferred from the mini-LHR and NOAA in situ flask networks, respectively.
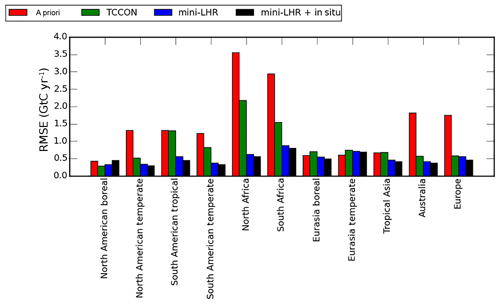
Figure 9Annual mean root-mean-square errors (RMSEs; GtC yr−1) associated with (red) a priori and a posteriori flux estimates inferred from (green) TCCON and (blue) mini-LHR CO2 columns and from the (black) combined mini-LHR–NOAA in situ flask networks.
Figures 8 and 9 summarize the agreement between a priori, true, and a posteriori CO2 fluxes from our three inversions for hemispheric-scale land regions. TCCON broadly reproduces true fluxes, but the current TCCON configuration is insensitive to some geographical regions (e.g. tropical South America and North Africa), as expected. Fluxes inferred from data from the mini-LHR network, independently and in combination with NOAA flask data, are closer to the true state over most parts of the world, e.g. north land summer months, as expected. Figures 8 and 9 also show that on the large spatial scales we have studied, the NOAA flask data provide a modest amount of additional information to the mini-LHR network. This suggests that a ground-based remote-sensing network that provides calibrated, high-frequency observations of column CO2 has comparable performance with the existing in situ network on larger continental spatial scales.
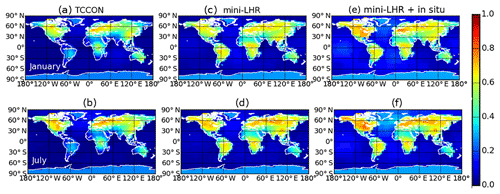
Figure 10Theoretical improvement in the knowledge of CO2 fluxes for a nominal (a, c, e) January and (b, d, f) July as determined by the γ factor, defined in the main text, for the (a, b) mini-LHR, (c, d) TCCON, and (e, f) combined mini-LHR–NOAA in situ flask measurement networks.
Figure 10 shows that the mini-LHR enhanced network of 50 sites results in global and significant improvements in our knowledge of CO2 fluxes. Significant values of the error reduction γ are found over most of North America and Eurasia as well as over South America and central and southern Africa. There is a similar geographical distribution of improvements during boreal summer months, but with larger values over North America and Eurasia, including the northernmost latitudes. Similar calculations for the TCCON network show comparable levels of improvement but are more spatially limited, particularly over the Northern Hemisphere. We show there is clear value in combining in situ flask data with the mini-LHR network, with significant improvements in CO2 fluxes, particularly over North American and Eurasia.
The mobility of mini-LHR sensors allows us to locate them in remote environments where an AERONET site is already established. This includes, in particular, tropical ecosystems where the physical environment is challenging for large-scale instrument installations and at polar latitudes where space-borne measurements are compromised because of low solar illumination and low surface reflectance over snow or ice. This suggests that the mini-LHR network could play a substantive role in an Arctic monitoring network, particularly during spring and autumn months.
Our results demonstrate the complementarity of the mini-LHR and in situ flask networks. Calibrating sensors from the TCCON and mini-LHR networks represent additional observational constraints on CO2 fluxes that rival knowledge inferred from the current in situ observation network over large-scale geographical regions (e.g. North America and Eurasia) and outperform for other regions (e.g. tropics). The in situ networks provide an invaluable record on the changing carbon cycle by putting present-day changes in a historical context, whose value is reduced if they are terminated.
The development of the mini-LHR technology is ongoing, but this computational study already builds on a growing body of work that has characterized its error budgets and its in-field performance (Wilson et al., 2013; Clarke et al., 2014; Melroy et al., 2015).
With a modest deployment of mini-LHR instruments to 50 sites, numerical experiments with the GEOS-Chem model indicate that the resulting XCO2 data products lead to improvements of carbon flux uncertainties ranging from 58 % to 81 % over southern lands, 47 % to 76 % over tropical lands, 71 % to 92 % over northern lands, and 64 % to 91 % globally. Because mini-LHRs leverage AERONET's global network of more than 500 sites worldwide, additional instruments can be rapidly added to target specific areas of uncertainty, such as thawing permafrost emissions in the Arctic or tropical ecosystems in the mid-latitudes. In addition to infrastructure, co-location of these instruments provides a simultaneous measurement of aerosol optical depth which is necessary for evaluating and correcting aerosol scattering effects in XCO2 satellite retrievals and consequent uncertainty in local and regional carbon flux estimates. Sun-viewing mini-LHR instruments are not impacted by some of the issues that degrade the quality of airborne or space-borne techniques that use reflected sunlight: surface reflectivity (e.g. darkness and angular dependence), surface roughness (sunlight path length), geolocation error, and scattering from clouds and aerosols. Together with the capability of measuring through gaps in cloud cover and continuous observation during daylight hours, the mini-LHR surface network in tandem operation with AERONET could provide full global and seasonal observation coverage and offer a necessary validation product for orbital missions.
However, the modelling study is largely agnostic to the underlying technology. Consequently, a similar result would be obtained, for example, by using a network of Bruker EM27/SUN instruments after they have been modified to withstand inclement weather and can run exclusively on solar power. A growing network of inter-calibrated ground-based remote-sensing units, as part of a global carbon measurement system, must strike a balance between a diffuse network of gold-standard spectrometers (TCCON), a larger network of intermediate (cost–performance) spectrometers (COCCON), and an even larger network of cheaper, less precise autonomous spectrometers that can be deployed in high-risk or high-reward environments where we remain data poor (e.g. tropics).
Code and data used in this study are available from the authors upon request.
PIP and ELW conceived the study and wrote the paper. LF, with support from JM, led the calculations. All co-authors provided comments on the paper.
The authors declare that they have no conflict of interest.
Development of the mini-LHR was supported by the NASA–GSFC Internal Research and Development (IRAD) programme, the NASA–GSFC Science Innovation Fund (SIF), and the NASA Interdisciplinary Science (IDS) programme (NNH127DA001N). Work at the University of Edinburgh was partly funded by the NERC National Centre for Earth Observation (NCEO). Paul I. Palmer gratefully acknowledges funding from his Royal Society Wolfson Research Merit Award. We would also like to thank Brent Holben and the AERONET team for their ongoing support and Laura Iraci and Jim Podolske from NASA Ames Research Center (ARC) for support with TCCON access and data at NASA–AFRC.
This paper was edited by John Worden and reviewed by two anonymous referees.
Aben, I., Hasekamp, O., and Hartmann, W.: Uncertainties in the space-based measurements Of CO2 columns due to scattering in the Earth's atmosphere, J. Quant. Spectros. Ra., 104, 450–459, https://doi.org/10.1016/j.jqsrt.2006.09.013, 2007.
Anderson, G. P., Chetwynd, J. H., Clough, S. A., Shettle, E. P., and Kneizys, F. X.: AFGL Atmospheric Constituent Profiles (0–120 km), Air Force Geophysics Laboratory, Hanscom AFB, USA, 43 pp., available at: https://apps.dtic.mil/dtic/tr/fulltext/u2/a175173.pdf (last access: 24 April 2019), 1986.
Basu, S., Guerlet, S., Butz, A., Houweling, S., Hasekamp, O., Aben, I., Krummel, P., Steele, P., Langenfelds, R. L., Torn, M., Biraud, S. C., Stephens, B. B., Andrews, A., and Worthy, D.: Global CO2 fluxes estimated from GOSAT retrievals of total column CO2, Atmos. Chem. Phys., 13, 8695–8717, https://doi.org/10.5194/acp-13-8695-2013, 2013.
Butz, A., Hasekamp, O. P., Frankenberg, C., and Aben, I.: Retrievals of atmospheric CO2 from simulated space-borne measurements of backscattered near-infrared sunlight: accounting for aerosol effects, Appl. Optics, 48, 3322–3336, 2009.
Camy-Peyret, C., Liuzzi, G., Masiello, G., Serio, C., Venafra, S., and Montzka, S.: Assessment of IASI capability for retrieving carbonyl sulphide (OCS), J. Quant. Spect. Ra., 201, 197–208, https://doi.org/10.1016/j.jqsrt.2017.07.006, 2017.
Clarke, G. B., Wilson, E. L., Miller, J. H., and Melroy, H. R.: Uncertainty analysis for the miniaturized laser heterodyne radiometer (mini-LHR), Meas. Sci. Technol., 25, 055204–055209, https://doi.org/10.1088/0957-0233/25/5/055204, 2014.
Commane, R., Lindaas, J., Benmergui, J., Luus, K. A., Change, R. Y.-W., Daube, B. C., Euskirchen, E. S., Henderson, J. M., Kariong, A., Miller, J. B., Miller, S. M., Parazoo, N. C., Randerson, J. T., Sweeney, C., Tans, P., Thoning, K., Veraverbeke, S., Miller, C. E., and Wofsy, S. C.: Carbon dioxide sources from Alaska driven by increasing early winter respiration from Arctic tundra, P. Natl. Acad. Sci. USA, 114, 5361–5366, https://doi.org/10.1073/pnas.1618567114, 2017.
Crisp, D., Pollock, H. R., Rosenberg, R., Chapsky, L., Lee, R. A. M., Oyafuso, F. A., Frankenberg, C., O'Dell, C. W., Bruegge, C. J., Doran, G. B., Eldering, A., Fisher, B. M., Fu, D., Gunson, M. R., Mandrake, L., Osterman, G. B., Schwandner, F. M., Sun, K., Taylor, T. E., Wennberg, P. O., and Wunch, D.: The on-orbit performance of the Orbiting Carbon Observatory-2 (OCO-2) instrument and its radiometrically calibrated products, Atmos. Meas. Tech., 10, 59–81, https://doi.org/10.5194/amt-10-59-2017, 2017.
Eldering, A., O'Dell, C. W., Wennberg, P. O., Crisp, D., Gunson, M. R., Viatte, C., Avis, C., Braverman, A., Castano, R., Chang, A., Chapsky, L., Cheng, C., Connor, B., Dang, L., Doran, G., Fisher, B., Frankenberg, C., Fu, D., Granat, R., Hobbs, J., Lee, R. A. M., Mandrake, L., McDuffie, J., Miller, C. E., Myers, V., Natraj, V., O'Brien, D., Osterman, G. B., Oyafuso, F., Payne, V. H., Pollock, H. R., Polonsky, I., Roehl, C. M., Rosenberg, R., Schwandner, F., Smyth, M., Tang, V., Taylor, T. E., To, C., Wunch, D., and Yoshimizu, J.: The Orbiting Carbon Observatory-2: first 18 months of science data products, Atmos. Meas. Tech., 10, 549–563, https://doi.org/10.5194/amt-10-549-2017, 2017.
Feng, L., Palmer, P., Bosch, H., and Dance, S.: Estimating surface CO2 fluxes from space-borne CO2 dry air mole fraction observations using an ensemble Kalman Filter, Atmos. Chem. Phys., 9, 2619–2633, https://doi.org/10.5194/acp-9-2619-2009, 2009.
Feng, L., Palmer, P. I., Yang, Y., Yantosca, R. M., Kawa, S. R., Paris, J.-D., Matsueda, H., and Machida, T.: Evaluating a 3-D transport model of atmospheric CO2 using ground-based, aircraft, and space-borne data, Atmos. Chem. Phys., 11, 2789–2803, https://doi.org/10.5194/acp-11-2789-2011, 2011.
Feng, L., Palmer, P. I., Parker, R. J., Deutscher, N. M., Feist, D. G., Kivi, R., Morino, I., and Sussmann, R.: Estimates of European uptake of CO2 inferred from GOSAT XCO2 retrievals: sensitivity to measurement bias inside and outside Europe, Atmos. Chem. Phys., 16, 1289–1302, https://doi.org/10.5194/acp-16-1289-2016, 2016.
Feng, L., Palmer, P. I., Bösch, H., Parker, R. J., Webb, A. J., Correia, C. S. C., Deutscher, N. M., Domingues, L. G., Feist, D. G., Gatti, L. V., Gloor, E., Hase, F., Kivi, R., Liu, Y., Miller, J. B., Morino, I., Sussmann, R., Strong, K., Uchino, O., Wang, J., and Zahn, A.: Consistent regional fluxes of CH4 and CO2 inferred from GOSAT proxy XCH4 : XCO2 retrievals, 2010–2014, Atmos. Chem. Phys., 17, 4781–4797, https://doi.org/10.5194/acp-17-4781-2017, 2017.
Gurney, K. R., Law, R. M., Denning, A. S., Rayner, P. J., Baker, D., Bousquet, P., Bruhwiler, L., Chen, Y.-H., Ciais, P., Fan, S., Fung, I. Y., Gloor, M., Heimann, M., Higuchi, K., John, J., Kowalczyk, E., Maki, T., Maksyutov, S., Peylin, P., Prather, M., Pak, B. C., Sarmiento, J., Taguchi, S., Takahashi, T., and Yuen, C.-W.: TransCom 3 CO2 inversion intercomparison: 1. Annual mean control results and sensitivity to transport and prior flux information, Tellus B, 55, 555–579, https://doi.org/10.3402/tellusb.v55i2.16728, 2003.
Holben, B. N., Eck, T. F., Slutsker, I., Tanre, D., Buis, J. P., Setzer, A., Vermote, E., Reagan, J. A., Kaufman, Y. J., Nakajima, T., Lavenue, F., Jankowiak, I., and Smirnov, A.: AERONET - A federated instrument network and data archive for aerosol characterization, Remote Sens. Environ., 66, 1–16, 1998.
Keppel-Aleks, G., Toon, G. C., Wennberg, P. O., and Deutscher, N. M.: Reducing the impact of source brightness fluctuations on spectra obtained by Fourier-transform spectrometry, Appl. Optics, 46, 4774–4779, 2007.
Krinner, G., Viovy, N., de Noblet-Ducoudré, N., Ogée, J., Polcher, J., Friedlingstein, P., Ciais, P., Sitch, S., and Prentice, I. C.: A dynamic global vegetation model for studies of the coupled atmosphere–biosphere system, Global Biogeochem. Cy., 19, GB1015, https://doi.org/10.1029/2003GB002199, 2005.
Kuze, A., Suto, H., Nakajima, H., and Hamazaki, T.: Thermal and near infrared sensor for carbon observation Fourier-transform spectrometer on the greenhouse gases observing satellite for greenhouse gases monitoring, Appl. Optics, 48, 6716–6733, 2009.
Le Quéré, C., Andrew, R. M., Canadell, J. G., Sitch, S., Korsbakken, J. I., Peters, G. P., Manning, A. C., Boden, T. A., Tans, P. P., Houghton, R. A., Keeling, R. F., Alin, S., Andrews, O. D., Anthoni, P., Barbero, L., Bopp, L., Chevallier, F., Chini, L. P., Ciais, P., Currie, K., Delire, C., Doney, S. C., Friedlingstein, P., Gkritzalis, T., Harris, I., Hauck, J., Haverd, V., Hoppema, M., Klein Goldewijk, K., Jain, A. K., Kato, E., Körtzinger, A., Landschützer, P., Lefèvre, N., Lenton, A., Lienert, S., Lombardozzi, D., Melton, J. R., Metzl, N., Millero, F., Monteiro, P. M. S., Munro, D. R., Nabel, J. E. M. S., Nakaoka, S. I., O'Brien, K., Olsen, A., Omar, A. M., Ono, T., Pierrot, D., Poulter, B., Rödenbeck, C., Salisbury, J., Schuster, U., Schwinger, J., Séférian, R., Skjelvan, I., Stocker, B. D., Sutton, A. J., Takahashi, T., Tian, H., Tilbrook, B., van der Laan-Luijkx, I. T., van der Werf, G. R., Viovy, N., Walker, A. P., Wiltshire, A. J., and Zaehle, S.: Global Carbon Budget 2016, Earth Syst. Sci. Data, 8, 605–649, https://doi.org/10.5194/essd-8-605-2016, 2016.
Liuzzi, G., Masiello, G., Serio, C., Venafra, S., and Camy-Peyret, C.: Physical inversion of the full IASI spectra: Assessment of atmospheric parameters retrievals, consistency of spectroscopy and forward modelling, J. Quant. Spectrosc. Ra., 182, 128–157, https://doi.org/10.1016/j.jqsrt.2016.05.022, 2016.
Mao, J.-P. and Kawa, S. R.: Sensitivity studies for space-based measurement of atmospheric total column carbon dioxide by reflected sunlight, Appl. Optics, 43, 914–927, 2004.
Meaures, B. I. D. P. E.: JCM 200:1012: International vocabulary of metrology – Basic and general concepts and associated terms (VIM), 3rd Edn., 108 pp., available at: https://www.bipm.org/en/publications/guides/vim.html (last access: 24 April 2019), 2012.
Melroy, H. R., Wilson, E. L., Clarke, G. B., Ott, L. E., Mao, J.-P., Ramanathan, A. K., and McLinden, M. L.: Autonomous field measurements of CO2 in the atmospheric column with the miniaturized laser heterodyne radiometer (Mini-LHR), Appl. Phys. B, 120, 609–615, 2015.
Messerschmidt, J., Geibel, M. C., Blumenstock, T., Chen, H., Deutscher, N. M., Engel, A., Feist, D. G., Gerbig, C., Gisi, M., Hase, F., Katrynski, K., Kolle, O., Lavric, J. V., Notholt, J., Palm, M., Ramonet, M., Rettinger, M., Schmidt, M., Sussmann, R., Toon, G. C., Truong, F., Warneke, T., Wennberg, P. O., Wunch, D., and Xueref-Remy, I.: Calibration of TCCON column-averaged CO2: the first aircraft campaign over European TCCON sites, Atmos. Chem. Phys., 11, 10765–10777, https://doi.org/10.5194/acp-11-10765-2011, 2011.
Oda, T., and Maksyutov, S.: A very high-resolution (1 km × 1 km) global fossil fuel CO2 emission inventory derived using a point source database and satellite observations of nighttime lights, Atmos. Chem. Phys., 11, 543–556, https://doi.org/10.5194/acp-11-543-2011, 2011.
Olsen, S. C. and Randerson, J. T.: Differences between surface and column atmospheric CO2 and implications for carbon cycle research, J. Geophys. Res.-Atmos., 109, D02301, https://doi.org/10.1029/2003JD003968, 2004.
Peylin, P., Law, R. M., Gurney, K. R., Chevallier, F., Jacobson, A. R., Maki, T., Niwa, Y., Patra, P. K., Peters, W., Rayner, P. J., Rödenbeck, C., van der Laan-Luijkx, I. T., and Zhang, X.: Global atmospheric carbon budget: results from an ensemble of atmospheric CO2 inversions, Biogeosciences, 10, 6699–6720, https://doi.org/10.5194/bg-10-6699-2013, 2013.
Reichle, R. H., Koster, R. D., DeLannoy, G. J. M., Forman, B. A., Liu, Q., Mahanama, S. P. P., and Toure, A.: Assessment and Enhancement of MERRA Land Surface Hydrology Estimates, J. Climate, 24, 6322–6338, 2011.
Rienecker, M. M., Suarez, M. J., Gelaro, R., Todling, R., Bacmeister, J., Liu, E., Bosilovich, M. G., Schubert, S. D., Takacs, L., Kim, G., Bloom, S., Chen, J., Collins, D., Conaty, A., da Silva, A., Gu, W., Joiner, J., Koster, R. D., Lucchesi, R., Molod, A., Owens, T., Pawson, S., Pegion, P., Redder, C. R., Reichle, R., Robertson, F. R., Ruddick, A. G., Sienkiewicz, M., and Woollen, J.: MERRA: NASA's Modern-Era Retrospective Analysis for Research and Applications, J. Climate, 24, 3624–3648, 2011.
Rodgers, C. D.: Inverse Methods for Atmospheric Sounding, Theory and Practice, in: Series on Atmospheric, Oceanic, and Planetary Physics, Oxford Press, Oxford, 2000.
Saunois, M., Bousquet, P., Poulter, B., Peregon, A., Ciais, P., Canadell, J. G., Dlugokencky, E. J., Etiope, G., Bastviken, D., Houweling, S., Janssens-Maenhout, G., Tubiello, F. N., Castaldi, S., Jackson, R. B., Alexe, M., Arora, V. K., Beerling, D. J., Bergamaschi, P., Blake, D. R., Brailsford, G., Brovkin, V., Bruhwiler, L., Crevoisier, C., Crill, P., Covey, K., Curry, C., Frankenberg, C., Gedney, N., Höglund-Isaksson, L., Ishizawa, M., Ito, A., Joos, F., Kim, H. S., Kleinen, T., Krummel, P., Lamarque, J. F., Langenfelds, R., Locatelli, R., Machida, T., Maksyutov, S., McDonald, K. C., Marshall, J., Melton, J. R., Morino, I., Naik, V., O'Doherty, S., Parmentier, F. J. W., Patra, P. K., Peng, C., Peng, S., Peters, G. P., Pison, I., Prigent, C., Prinn, R., Ramonet, M., Riley, W. J., Saito, M., Santini, M., Schroeder, R., Simpson, I. J., Spahni, R., Steele, P., Takizawa, A., Thornton, B. F., Tian, H., Tohjima, Y., Viovy, N., Voulgarakis, A., van Weele, M., van der Werf, G. R., Weiss, R., Wiedinmyer, C., Wilton, D. J., Wiltshire, A., Worthy, D., Wunch, D., Xu, X., Yoshida, Y., Zhang, B., Zhang, Z., and Zhu, Q.: The global methane budget 2000–2012, Earth Syst. Sci. Data, 8, 697–751, https://doi.org/10.5194/essd-8-697-2016, 2016.
Shuur, E. A. G., Bockheim, J., Canadell, J. G., Euskirchen, E., Field, C. B., Goryachkin, S. V., Hagemann, S., Kuhry, P., Lafleur, P. M., Lee, H., Mazhitova, G., Nelson, F. E., Rinke, A., Romanovsky, V. E., Shiklomanov, N., Tarnocai, C., Venevsky, S., Vogel, J. G., and Zimov, S. A.: Vulnerability of permafrost carbon to climate change: Implications for the global carbon cycle, Bioscience, 58, 701–714, https://doi.org/10.1641/B580807, 2008.
Smith, M. D., Wolff, M. J., Clancy, R. T., and Murchie, S. L.: Compact Reconnaissance Imaging Spectrometer observations of water vapor and carbon monoxide, J. Geophys. Res., 114, E00D03, https://doi.org/10.1029/2008JE003288, 2009.
Takahashi, T., Sutherland, S. C., Wanninkhof, R., Sweeney, C., Feely, R. A., Chipman, D. W., Hales, B., Friederich, G., Chavez, F., Sabine, C., Watson, A., Bakker, D. C. E., Schuster, U., Metzl, N., Yoshikawa-Inoue, H., Ishii, M., Midorikawa, T., Nojiri, Y., Kortzinger, A., Steinhoff, T., Hoppema, M., Olafsson, J., Arnarson, T. S., Tilbrook, B., Johannessen, T., Olsen, A., Bellerby, R., Wong, C. S., Delille, B., Bates, N. R., and de Baar, H. J. W.: Climatological mean and decadal changes in surface ocean pCO2, and net sea-air CO2 flux over the global oceans, Deep-Sea Res. Pt. II, 56, 554–577, https://doi.org/10.1016/j.dsr2.2008.12.009, 2009.
Uchino, O., Kikuchi, N., Sakai, T., Morino, I., Yoshida, Y., Nagai, T., Shimizu, A., Shibata, T., Yamazaki, A., Uchiyama, A., Kikuchi, N., Oshchepkov, S., Bril, A., and Yokota, T.: Influence of aerosols and thin cirrus clouds on the GOSAT-observed CO2: a case study over Tsukuba, Atmos. Chem. Phys., 12, 3393–3404, https://doi.org/10.5194/acp-12-3393-2012, 2012.
van der Werf, G. R., Randerson, J. T., Giglio, L., Collatz, G. J., Mu, M., Kasibhatla, P. S., Morton, D. C., DeFries, R. S., Jin, Y., and van Leeuwen, T. T.: Global fire emissions and the contribution of deforestation, savanna, forest, agricultural, and peat fires (1997–2009), Atmos. Chem. Phys., 10, 11707–11735, https://doi.org/10.5194/acp-10-11707-2010, 2010.
Villanueva, G. L., Mumma, M. J., Novak, R. E., Kaufl, H. U., Hartogh, P., Encrenaz, T., Tokunaga, A., Khayat, A., and Smith, M. D.: Strong water isotopic anomalies in the martian atmosphere: Probing current and ancient reservoirs, Science, 348, 218–221, 2015.
Villanueva, G. L., Smith, M., Wolff, M. J., Protopapa, S., Hewagama, T., Mandell, A. M., and Faggi, S.: Planetary Spectrum Generator (PSG), available at: https://psg.gsfc.nasa.gov/ (last access: 24 April 2019), 2016.
Wilson, E. and McLinden, M.: Miniaturized Laser Heterodyne Radiometer for carbon dioxide methane and carbon monoxide measurements in the atmospheric column, US Patent No. 8699029 (Filed 2012, Awarded 2014), 2012.
Wilson, E. L., Mclinden, M. L., Miller, J. H., Allan, G. R., Ott, L. E., Melroy, H. R., and Clarke, G. B.: Miniaturized laser heterodyne radiometer for measurements of CO2 in the atmospheric column, Applied Phys. B, 114, 385–393, https://doi.org/10.1007/s00340-013-5531-1, 2013.
Wilson, E. L., McLinden, M. L., Miller, J. H., Allen, G. R., Ott, L. E., Melroy, H. R., and Clarke, G. B.: Miniaturized Laser Heterodyne Radiometer for Measurements of CO2 in the Atmospheric Column, Appl. Phys. B, 114, 385–393, https://doi.org/10.1007/s00340-013-5531-1, 2014.
Wilson, E. L., DiGregorio, A. J., Riot, V. J., Ammons, M. S., Bruner, W. W., Carter, D., Mao, J.-P., Ramanathan, A., Strahan, S. E., Oman, L. D., Hoffman, C., and Garner, R. M.: A 4 U laser heterodyne radiometer for methane (CH4) and carbon dioxide (CO2) measurements from an occultation-viewing CubeSat, Meas. Sci. Technol., 28, 035902, https://doi.org/10.1088/1361-6501/aa5440, 2017.
Wolf, J., Asrar, G. R., and West, T. O.: Revised methane emissions factors and spatially distributed annual carbon fluxes for global livestock, Carbon Balance Manage., 12, 16, https://doi.org/10.1186/s13021-017-0084-y, 2017.
Wunch, D., Toon, G. C., Wennberg, P. O., Wofsy, S. C., Stephen, M., Fischer, M. L., Uchino, O., Abshire, J. B., Bernath, P. F., Biraud, S. C., Blavier, F. L., Boone, C., Bowman, K. P., Browell, E. V., Campos, T., Connor, B. J., Daube, B. C., Deutscher, N. M., Diao, M., Elkins, J. W., Gerbig, C., Gottlieb, E., Griffith, D., Hurst, D. F., Jimenez, R., Keppel-Aleks, G., Kort, E. A. I., Park, S., Robinson, J., Roehl, C. M., Sawa, Y., Sherlock, V., Sweeney, C., Tanaka, T., and Zondo, M. A.: Calibration of the Total Carbon Column Observing Network using aircraft profile data, Atmos. Meas. Tech., 3, 1351–1362, https://doi.org/10.5194/amt-3-1351-2010, 2010.
Wunch, D., Toon, G. C., Blavier, -. F., L., Washenfelder, R. A., Notholt, J., Connor, B., Griffith, D. W. T., Sherlock, V., and Wennberg, P. O.: The total carbon column observing network (TCCON), Philos. T. Roy. Soc. A, 369, 2087–2112, 2011.
Wunch, D., Wennberg, P. O., Osterman, G., Fisher, B., Naylor, B., Roehl, C. M., O'Dell, C., Mandrake, L., Viatte, C., Kiel, M., Griffith, D. W. T., Deutscher, N. M., Velazco, V. A., Notholt, J., Warneke, T., Petri, C., De Maziere, M., Sha, M. K., Sussmann, R., Rettinger, M., Pollard, D., Robinson, J., Morino, I., Uchino, O., Hase, F., Blumenstock, T., Feist, D. G., Arnold, S. G., Strong, K., Mendonca, J., Kivi, R., Heikkinen, P., Iraci, L., Podolske, J., Hillyard, P. W., Kawakami, S., Dubey, M. K., Parker, H. A., Sepulveda, E., García, O. E., Te, Y., Jeseck, P., Gunson, M. R., Crisp, D., and Eldering, A.: Comparisons of the Orbiting Carbon Observatory-2 (OCO-2) XCO2 measurements with TCCON, Atmos. Meas. Tech., 10, 2209–2238, https://doi.org/10.5194/amt-10-2209-2017, 2017.
Yoshida, Y., Kikuchi, N., Morino, I., Uchino, O., Oshchepkov, S., Bril, A., Saeki, T., Schutgens, N., Toon, G. C., Wunch, D., Roehl, C. M., Wennberg, P. O., Griffith, D. W. T., Deutscher, N. M., Warneke, T., Notholt, J., Robinson, J., Sherlock, J., Connor, B., Rettinger, M., Sussmann, R., Ahonen, P., Heikkinen, P., Kyro, E., Mendonca, J., Strong, K., Hase, F., Dohe, S., and Yokota, T.: Improvement of the retrieval algorithm for GOSAT SWIR XCO2 and XCH4 and their validation using TCCON data, Atmos. Meas. Tech., 6, 1533–1547, https://doi.org/10.5194/amt-6-1533-2013, 2013.