the Creative Commons Attribution 4.0 License.
the Creative Commons Attribution 4.0 License.
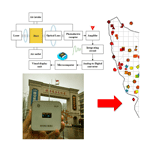
Strategies of method selection for fine-scale PM2.5 mapping in an intra-urban area using crowdsourced monitoring
Shan Xu
Bin Zou
Yan Lin
Xiuge Zhao
Shenxin Li
Chenxia Hu
Fine particulate matter (PM2.5) is of great concern to the public due to its significant risk to human health. Numerous methods have been developed to estimate spatial PM2.5 concentrations in unobserved locations due to the sparse number of fixed monitoring stations. Due to an increase in low-cost sensing for air pollution monitoring, crowdsourced monitoring of exposure control has been gradually introduced into cities. However, the optimal mapping method for conventional sparse fixed measurements may not be suitable for this new high-density monitoring approach. This study presents a crowdsourced sampling campaign and strategies of method selection for 100 m scale PM2.5 mapping in an intra-urban area of China. During this process, PM2.5 concentrations were measured by laser air quality monitors through a group of volunteers during two 5 h periods. Three extensively employed modelling methods (ordinary kriging, OK; land use regression, LUR; and regression kriging, RK) were adopted to evaluate the performance. An interesting finding is that PM2.5 concentrations in micro-environments varied in the intra-urban area. These local PM2.5 variations can be easily identified by crowdsourced sampling rather than national air quality monitoring stations. The selection of models for fine-scale PM2.5 concentration mapping should be adjusted according to the changing sampling and pollution circumstances. During this project, OK interpolation performs best in conditions with non-peak traffic situations during a lightly polluted period (holdout validation R2: 0.47–0.82), while the RK modelling can perform better during the heavily polluted period (0.32–0.68) and in conditions with peak traffic and relatively few sampling sites (fewer than ∼100) during the lightly polluted period (0.40–0.69). Additionally, the LUR model demonstrates limited ability in estimating PM2.5 concentrations on very fine spatial and temporal scales in this study (0.04–0.55), which challenges the traditional point about the good performance of the LUR model for air pollution mapping. This method selection strategy provides empirical evidence for the best method selection for PM2.5 mapping using crowdsourced monitoring, and this provides a promising way to reduce the exposure risks for individuals in their daily life.
- Article
(4940 KB) -
Supplement
(246 KB) - BibTeX
- EndNote
Fine particulate matter (PM2.5) has been associated with an increased risk of morbidity and mortality in both the long term and the short term (Beverland et al., 2012; Cohen et al., 2017; Di et al., 2017; Lelieveld et al., 2015). The persistent cumulative effects from exposure in daily activities, especially daily travelling, are critical (Kingham et al., 2013; Hankey et al., 2017). If individuals could consciously choose the location and time of their outdoor activities based on detailed knowledge about the spatio-temporal variation in PM2.5 concentration, then their health protection could be improved.
In situ measurement is the most reliable way to capture the PM2.5 concentrations across every corner of a city in real time. However, fixed monitoring stations in conventional air quality monitoring networks are sparse. As a result, site-based observations encounter challenges in capturing spatio-temporal variations in air pollutants, especially in intra-urban areas with unevenly distributed emission sources and dispersion conditions (Kumar et al., 2015; Zou et al., 2015; Apte et al., 2017). Spatial mapping methods, including air dispersion modelling, spatial interpolation, satellite remote sensing (RS), and empirical models, have been increasingly employed to estimate concentrations of PM2.5 in unobserved locations over the past 2 decades (Jerrett et al., 2005; Henderson et al., 2007; El-Harbawi, 2013; Saraswat et al., 2013; Kim et al., 2014; Rice et al., 2015; Fang et al., 2016; Zou et al., 2017; Zhai et al., 2018; Xu et al., 2018; Liu et al., 2018). The outputs of a dispersion model considerably depend on detailed emission inventories and meteorological information, which are not usually available for many cities. The coarse spatial resolution (≥1–10 km) of satellite instruments and the missing data problem due to the cloud cover prohibit the widespread use of RS in PM2.5 concentration mapping in urban environments (Zou et al., 2015; Apte et al., 2017).
Conversely, geostatistical and empirical models can estimate concentrations at high spatial resolution with a rather low requirement for data. The most commonly employed models are ordinary kriging (OK) interpolation and land use regression (LUR) modelling. Some studies have improved the estimating accuracy by combining these two technologies (Mercer et al., 2011; De Hoogh et al., 2018). While they have been successfully applied to map the spatial variability of PM2.5 concentrations in various geographic areas, their accuracy varies as the concentration levels and sample sizes change (Wang et al., 2012; Mercer et al., 2011; Lee et al., 2014; Zou et al., 2015; Gillespie et al., 2016; Choi et al., 2017; De Hoogh et al., 2018).
Due to an increase in low-cost sensing for air pollution monitoring, the real-time strategies for exposure control in cities have been further developed (Kumar et al., 2015). Crowdsourced monitoring that enables citizens to produce geospatial data is constantly growing and shows considerable potential (Heipke, 2010). Large and diverse groups of people who lack formal training can easily describe their environments with a mobile phone or smartphone and upload data via informal social networks and web technology. Unlike traditional fixed monitoring stations that are usually mounted on roofs (i.e. 3 to 20 m a.g.l.) for the sake of instrument protection, crowdsourced monitoring provides real-time PM2.5 monitoring that reflects the real exposure for individuals who live and work on the ground. Although crowdsourced monitoring tends to produce observations with questionable quality, it enables us to obtain measurements of ambient air pollution in dense networks at relatively low cost. Some studies have employed these data to display the air pollution concentration and investigate the exposure risks (Thompson, 2016; Miskell et al., 2017; Jerrett et al., 2017). These observations are still point measurements that are only representative of the limited area around the site and cannot satisfy the demand of obtaining the air pollution concentration whenever and wherever we want.
One way to address the previously mentioned challenge is to combine high-density crowdsourced observations with spatial mapping methods. An important investigation was performed by Schneider et al. (2017) in Oslo, Norway. They presented a universal kriging technique for urban NO2 concentration mapping that combines near-real-time crowdsourced observations of urban air quality with output from an air pollution dispersion model. However, high-density crowdsourced measurements may vary among urban micro-environments with different human daily activities and among sparsely distributed conventional in situ measurements. Using the elected mapping methods from previous studies to depict the variation in air pollution on a very fine spatial and temporal scale with new monitoring ways may cause the misclassification of exposure and an underestimation of risk. As the number of valid crowdsourced observations may significantly change due to instrument faults, human error, and other quality issues, the applicability of mapping methods to different sampling sizes needs sound scientific evidence.
In this study, we presented strategies of method selection for PM2.5 concentration mapping based on crowdsourced datasets with varying size. The intra-urban crowdsourced sampling campaign was conducted in the city of Changsha, China, over two periods in different pollution scenarios. The performance of OK, LUR, and regression kriging (RK) in estimating PM2.5 pollution was evaluated and compared with an increasing number of training sites. The best performing method was employed to plot the variation in the hourly PM2.5 concentration and identify the pollution hotspots in the intra-urban area. The results from this study will provide evidence for the method selection of PM2.5 mapping using crowdsourced monitoring and significantly contribute to efficient air pollution mapping and exposure assessment in intra-urban areas.
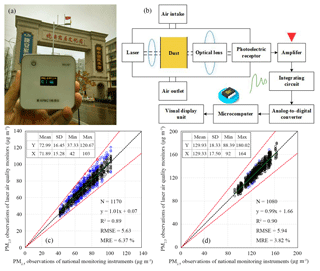
Figure 1Laser air quality monitor: (a) exterior, (b) measuring principle, and (c, d) scatter plots of hourly PM2.5 concentration from the laser air quality monitor (Y) and national monitoring instrument (X). The black, blue, and red dots indicate PM2.5 observations with a relative error of < 10 %, 10 %–20 %, and > 20 %, respectively. The black and red dotted lines are the 1:1 line and 1:1.2 line as references.
2.1 PM2.5 sampling
2.1.1 Measurement instrument
The portable laser air quality monitor SDL307 (produced by Nova Fitness Co., Ltd.) is employed to perform sampling. The monitor manual can be downloaded from http://www.inovafitness.com/index.html (last access: 18 February 2019). This monitor can be conveniently carried with a total size of 7.3 cm × 7.3 cm×2.0 cm (Fig. 1a) and has a resolution of 0.1 µg m−3. It has two monitoring modes, Mode One and Mode Two. Under Mode One, the monitor observes PM2.5 concentration in real time and measurement updates and automatically stores every second, but only has a battery life of 5 h; that is, there are 60 min, a total of 3600 s of measurements if we continuously observe PM2.5 for 1 h in Mode One. Under Mode Two, the monitor repeats the procedure of observing and storing every second for 1 min and then sleeping 5 min (i.e. 10 min, a total of 600 s of measurements per hour), and the battery life is 30 h. According to the test report provided by the Center for Building Environment Test at Tsinghua University, the laser monitors were compared with the regulatory monitor in the lab in the air quality monitoring mode at concentration levels of 21, 63, 106, 212, 454, and 1060 µg m−3 and the relative errors were rather low (within ±20 %) and demonstrated similar patterns between concentration levels except for the 1060 µg m−3 level (http://www.inovafitness.com/a/minyongchanpin/jianceyilei/2015/0522/31.html, last access: 1 April 2019). The concentration of particulate matter is measured using the light-scattering method (Fig. 1b). The monitor contains a special laser module, and the signals are recorded by a photoelectric receptor when particulate matter passes through laser light. The count and size of particulate matter are then analysed by a microcomputer after the signals are amplified and converted. Their mass concentrations are automatically calculated using a built-in algorithm based on the conversion factor between the light-scattering method and the tapered element oscillating microbalance technology.
To ensure the low inter-sensor variability (i.e. the measurement difference under the same condition) of sampling monitors, we placed 115 laser air quality monitors in the same environment and continuously observed them in Mode Two for 1 week during each of the four seasons. If the absolute value of relative error between the observation of one monitor and the average observations of the other monitors exceeded 5 %, this monitor fell into disuse. This procedure was conducted both indoors and outdoors. Subsequently, 86 monitors with rather stable performance and a small difference between each observation remained. In addition, we randomly selected 30 portable laser air quality monitors to compare with the national monitoring instruments to further guarantee the reliability of the sampling data. First, for ease of operation, three national air quality monitoring stations were selected. Second, for each station, 10 monitors were observed in Mode Two (i.e. 600 s of measurements per hour) next to the national monitoring instrument (∼15 m a.g.l. in the study area) from 08:00 to 20:00 UTC+8 (for all times throughout) on 20–22 December 2015 and from 08:00 to 20:00 on 29–31 December 2015. The hourly PM2.5 concentrations of the evaluation periods were the mean values of 10 min measurements. The weather on 20–22 December was overcast with patchy drizzle and light rain at times, and the relative humidity (RH) ranged from 77 % to 94 %, while the weather on 29–31 December was cloudy with some sunshine and a RH that ranged from 38 % to 67 %.
The scatter plots and descriptive statistics of the valid hourly average PM2.5 concentrations from the 30 laser air quality monitors and the three national monitoring instruments for 20–22 and 29–31 December were presented in Fig. 1c and d, respectively. The hourly average PM2.5 concentrations for two types of instruments generally showed good agreement with a correlation coefficient R2 of 0.89 and 0.90. The root-mean-square errors (RMSEs) for the former time period were lower than the RMSE for the latter time period (5.63 vs. 5.94 µg m−3), while the mean relative error (MRE) was higher than the MRE for the latter time period (6.37 % vs. 3.82 %). The latter time period demonstrated a smaller difference in hourly average PM2.5 concentrations between laser air quality monitors and the national monitoring instruments with mean values and standard deviations (SD) of 72.99±16.45 µg m−3 vs. 71.89±15.28 µg m−3 and 129.93±18.33 µg m−3 vs. 129.33±17.50 µg m−3. The relationship between RH and the ratio of laser monitor measurements to the national instrument measurements for two evaluation periods was presented as Fig. S1a and b in the Supplement. The ratio of laser monitor measurements to the national instrument measurements roughly increased exponentially with the increase in the RH for 20–22 December (R2=0.2186), while the ratio was uncorrelated with RH for 29–31 December (R2=0.0457). When the RH correction is made with an empirical equation for 20–22 December, the R2 between hourly PM2.5 concentration from laser monitor measurements and the national instrument measurements improved from 0.89 to 0.90.
2.1.2 Sampling design
The sampling area is located in the Changsha metropolitan area (112∘49′–113∘14′ E, 27∘58′–28∘24′ N), which covers an area of approximately 920 km2 and seven districts (refer to Fig. 2). Changsha is the capital of Hunan Province with a population that exceeds 7 million people. The area experienced high-level exposure to air pollutants due to an increase in anthropogenic activities and intensive energy consumption.
To ensure that the sampling sites exhibit a relatively even and typical distribution for different urban micro-environments (i.e. residential community, building site, school, and park), a series of rules were designed to determine the potential PM2.5 sampling sites based on the distribution of potential emission sources (refer to Table 1). The data that support the sampling design consist of important points of interest (POIs), dust surfaces, and main road networks. POI data include industrial parks, enterprises, factories, depots, hospitals, schools, and parks. Dust surfaces refer to natural and artificial bare surfaces with vegetation that covers less than 10 %, which easily produce atmospheric particulate matter, such as construction sites, stacked substance, and natural bare land. These data were collected from the Information Center of Land and Resources of Hunan Province. More than three observations of PM2.5 concentrations are required every hour for each potential sampling site to improve the reliability of the sampling data. Given that the number of laser air quality monitors and the distance that a volunteer can walk in 1 h are limited, only two to four sites can be set in the area in which a volunteer can cover during the sampling. Therefore, a total of 208 potential PM2.5 sampling sites were selected. The centres of each area covered by a monitor were numbered in sequence (i.e. 1–86). The monitors were also numbered and labelled.
2.1.3 Sampling and data processing
Sampling was performed in two time periods in the winter of 2015 to examine the effect of air quality grades on the mapping results. The first period fell between 08:00 and 12:00 on 24 December. In this period, the official air pollution levels were “good” and “moderate” (i.e. Period 1, lightly polluted period). The weather was overcast with occasional rain or drizzle, and the relative humidity (RH) ranged from 95 % to 98 %. The second period extended between 14:00 and 18:00 on 25 December, when an orange warning signal of haze (i.e. official air pollution level was “heavily polluted”) was released by the Changsha Meteorology Bureau (i.e. Period 2, heavily polluted period). The weather was cloudy with some sunshine, and the RH ranged from 39 % to 43 %.
Before sampling started, every volunteer received one monitor and went to the corresponding area. At each potential monitoring site, the volunteer lifted the monitor (∼2 m above the ground) and constantly measured PM2.5 concentration in Mode One for 3 min. During this process, after the first 60 s, we observed the screen and uploaded the measurement using a smartphone application (app) that we developed to verify the reliability of the stored data. For each hour, we eliminated the sampling sites observed less than three times. As the sites take turns measuring PM2.5 concentration, there are at least 3 min of measurements every 20 min of every sampling hour for those sites left. The valid observations for every sampling hour (i.e. 9–12 min, a total of 540–720 s measurements per hour) were then averaged at each site. The geographic coordinates of the sampling sites were also uploaded. As some volunteers quit after the sampling of the first period, the sampling sites in Period 2 were concentrated in the central study area. A total of 179–208 samples were successfully collected at each hour in Period 1, and 105–118 samples were successfully collected in Period 2. The official observations at 10 national monitoring stations in the study area were also obtained (China Environmental Monitoring Center, CEMC: http://106.37.208.233:20035/, last access: 31 December 2015) and averaged for comparison purposes.
2.2 Mapping method selection
We divided sampling data into a training set and a validation set (holdout validation) for each hour to evaluate the performance of OK, LUR, and RK with an increasing number of training sites. The training datasets were divided into groups based on the percentages of 20 %, 30 %, 40 %, 50 %, 60 %, 70 %, 80 %, and 90 % of the total number of monitoring sites. Therefore, a series of groups of training samples (36–42, 54–62, 72–83, 90–104, 107–125, 125–146, 143–166, 161–189 sites in Period 1 and 31–35, 42–47, 52–59, 63–71, 73–83, 84–94, 94–106 in Period 2) were extracted using the Subset Features tool of ArcGIS (version 10.0). We repeated this process 100 times for each training set size for Period 1 and 50 times for Period 2. Statistics including the coefficient R2, RMSE, and MRE between the predicted concentrations and observed concentrations of PM2.5 in the independent validation set were employed to evaluate and compare their performance.
2.2.1 Ordinary kriging
OK estimates the target variable at an unsampled location as a linear combination of neighbouring observations. OK relies on a weighting scheme, where closer observations have a greater impact on the final prediction. The weighting scheme is dictated by the variogram (Pang et al., 2010; Zou et al., 2015) and can be described as
where Z∗(X0) is the estimation of an unknown sample point; Z(Xi) and ωi are the values of the ith known sample point surrounding the unknown sample point and its corresponding weight, respectively; and n is the number of known sample points.
2.2.2 Land use regression
LUR modelling predicts the air pollution concentration by linking measurements of monitoring sites and geographic elements around them using the least-squares method. LUR is composed of predictor variable extraction and selection and regression modelling and validation.
Geographic factors including pollution sources (dust surface and pollution industries), road networks, and land use/cover were employed to indirectly characterise the PM2.5 emissions in this study. These data were generated using multiple ring buffers with different radii (50–1000 m) at each monitoring site. Meteorological data including wind speed, atmospheric pressure, relative humidity, and temperature of 107 sites in and around the sampling area, which may affect the dispersion of PM2.5, were also obtained. Geographic factors were made available by the Information Centre of Department of Land and Resources of Hunan Province. Meteorological data were released by the Hunan Meteorology Bureau. All variables (Table 2) were extracted using ArcGIS (version 10.0). The optimal buffer radius for the percentage of dust surfaces and land use, pollution industry density, and road density were defined based on the maximum Pearson correlation coefficients.
An automatic forward–backward stepwise regression procedure was employed to select the best-fitting LUR models based on the screened-out predictors. The final LUR models in this study were determined based on the criteria of the lowest Akaike information criterion (AIC) value and the highest fitting R2. The model structure can be expressed as
where PM2.5,s is the estimation of the hourly averaged PM2.5 concentration of site s, Xi,s () represents independent variables, a0 is a constant, ai () represents regression coefficients, and μ is the random error estimated using the least-squares method. This process was conducted in R statistical software (version 3.3.2) (Fox and Weisberg, 2011; R Core Team, 2016).
2.2.3 Regression kriging
RK is a two-stage statistical procedure in this study. First, separate standard LUR models were developed based on crowdsourced observations in the training dataset for each hour. Second, the residuals for the LUR models were calculated and interpolated for each hour using OK technology. Finally, the estimations of the residuals at the validation sites were extracted and added to the LUR estimations.
In this study, OK was performed using the Geostatistical Analyst tool of ArcGIS (version 10.0), and interpolated residuals were obtained using the Extract Values to Point tool. The entire process was implemented with Python scripts.
2.3 PM2.5 concentration mapping
The method that performed best with 90 % training sites was chosen as the mapping method. Using this method, the spatial distributions of the PM2.5 concentration for each hour were estimated with all samples. In this study, nearest-neighbour distances between two sampling sites ranged from 15 to 60 m for Period 1 and 54 to 98 m for Period 2. Considering the resolutions of the potential predictors, 100 m was used as the mapping grid size. The spatial distributions of the PM2.5 concentration for each hour with measurements of 10 national monitoring stations were estimated using the same method for comparison.
3.1 Descriptive statistics of PM2.5 concentrations
Table 3 shows the descriptive statistics of hourly PM2.5 concentrations for the crowdsourced sampling sites and the national monitoring stations. For Period 1, the mean values and SD of the PM2.5 concentrations for the crowdsourced sampling sites ranged from (69.67±18.81) to (76.45±14.55) µg m−3. These values were substantially higher than those for the national monitoring stations (i.e. (36.9±10.97) – (41.2±8.68) µg m−3). The maximum and minimum values of crowdsourced PM2.5 concentrations were higher than the national values. However, the mean values and SD of PM2.5 concentrations of the crowdsourced sites are lower than those of the national stations in Period 2. The former values ranged from (162.72±15.96) to (171.89±21.5) µg m−3, while the latter values ranged from (177.8±16.91) to (188.3±22.4) µg m−3. Although the minimum values of crowdsourced PM2.5 concentrations were also lower than those of the national stations, the maximum values were higher. The average PM2.5 concentrations of Period 2 were substantially higher than those of Period 1, and the highest values occurred when traffic and emissions from cooking had peaked (i.e. 12:00 and 18:00) for both periods.
Figure 3 demonstrates the spatial variation in the PM2.5 measurements over the two periods in the study area, and the spatial variations between different sampling sites and two periods can be obtained. For Period 1, the PM2.5 concentrations gradually decreased from north to south and from west to east. Higher concentrations of PM2.5 (> 75 µg m−3) were observed at sampling sites in the northwest corner of the study area. The sampling sites in Changsha County with high levels of green vegetation cover had lower PM2.5 concentrations compared with the sites in the inner city. For Period 2, conversely, sampling sites in the central and eastern parts of the study area had higher PM2.5 concentrations than those in the western part. Monitoring sites had PM2.5 concentrations higher than 150 µg m−3 in most areas, with the exception of the western Yuelu district. In particular, sampling sites in areas along the Xiangjiang River, especially in the higher-education megacentre, experienced extreme PM2.5 pollution (> 210 µg m−3).
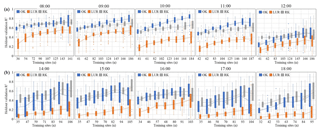
Figure 4Box plots of holdout validation R2 between the observed concentration and predicted concentration of PM2.5 for OK, LUR, and RK with an increase in training sites: (a) Period 1; (b) Period 2. The boundaries of the boxes indicate the 75th percentile and 25th percentile (Q3 and Q1, respectively). The line within the box denotes the median (Q2), and the crosses denote the averages. The error bars above and below indicate the highest datum (Q3+1.5IQR, IQR is the interquartile range, IQR=Q3−Q1) and the lowest datum (Q1−1.5IQR), respectively. Dots above and below the error bars indicate the outliers.
3.2 Model performance for OK, LUR, and RK
The box plots of Fig. 4 show the variation in the holdout validation R2 for the three mapping approaches in relation to the number of training sites. The average and standard deviation of the RMSE and MRE between the observed concentration and predicted concentration of PM2.5 in the holdout validation were presented in the Supplement (Tables S3–S4). The average values and variability ranges of R2 for OK, LUR, and RK were positively associated with an increase in the number of training sites. RK performed best in Period 2 and at 08:00 and 12:00 of Period 1 with fewer than ∼100 training sites. The LUR demonstrated the poorest performance for both periods of the models tested.
For Period 1, the PM2.5 estimating accuracy was generally highest at 09:00 and lowest at 12:00. The average validation R2 ranges for different number training sites of OK at 08:00, 09:00, 10:00, 11:00, and 12:00 were 0.58–0.72, 0.56–0.78, 0.51–0.82, 0.47–0.71, and 0.24–0.48, respectively. Compared with OK, the accuracy of LUR was substantially lower. The ranges were 0.26–0.55, 0.29–0.54, 0.16–0.40, 0.16–0.36, and 0.24–0.34. The average R2 values for RK were weakly smaller than OK at 09:00, 10:00, and 11:00 with ranges of 0.59–0.69, 0.50–0.66, and 0.48–0.60, respectively. The average R2 values of RK at 08:00 and 12:00 were higher than OK when less than ∼100 sampling sites were divided into training datasets (08:00: 0.65–0.69 vs. 0.58–0.68; 12:00: 0.40–0.44 vs. 0.24–0.41). For Period 2, the validation R2 from high to low followed the sequence RK > OK > LUR. The average validation R2 values for a different number of training sites of OK were considerably lower in Period 1. The ranges at 14:00, 15:00, 16:00, 17:00, and 18:00 were 0.25–0.49, 0.34–0.50, 0.40–0.59, 0.27–0.39, and 0.18–0.27, respectively. The average R2 values of LUR were even lower; the lowest values were 0.08, 0.07, 0.15, 0.06, and 0.04, and the highest values were 0.22, 0.25, 0.42, 0.22, and 0.16, respectively. Combining OK and LUR, the performance of RK improved with an average R2 that ranged from 0.43, 0.44, 0.43, 0.36, and 0.32 to 0.60, 0.68, 0.52, 0.54, and 0.57.
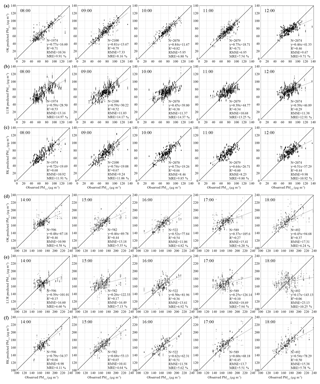
Figure 5Scatter plots of repeated validating results with 90 % training sites for (a) OK, Period 1; (b) LUR, Period 1; (c) RK, Period 1; (d) OK, Period 2; (e) LUR, Period 2; and (f) RK, Period 2. The solid line is the 1:1 line, which is a reference.
Figure 5 shows scatter plots of holdout validation results with 90 % training sites. For Period 1, the lowest total R2 of OK and the highest total R2 of OK were 0.46 for 12:00 and 0.82 for 10:00 (Fig. 5a), respectively, while R2 values of RK were lower with the range of 0.44–0.68 (Fig. 5c); they were both higher than the LUR (0.29–0.53, Fig. 5b). Correspondingly, the RMSE and MRE from low to high were OK (5.95–10.36; 6.80 %–9.91 %) < RK (8.23–10.92; 9.80 %–11.91 %) < LUR (10.68–13.16; 12.91 %–14.97 %). For Period 2, however, the RK presented the highest accuracy with a R2 that ranged from 0.45 (17:00) to 0.66 (14:00) (Fig. 5f). The OK ranked second (R2: 0.27–0.54, Fig. 5d), while the LUR achieved the poorest performance (R2: 0.06–0.36, Fig. 5e).
3.3 Spatial patterns of crowdsourced PM2.5 concentration
Figure 6a and b reveal the spatial distributions of OK-interpreted PM2.5 concentrations for Period 1 from the crowdsourced sampling sites and the national monitoring stations, respectively. Figure 6c and d demonstrate the spatial distributions of the RK-estimated PM2.5 concentrations for Period 2. The crowdsourced hourly PM2.5 concentration maps demonstrate more detailed intra-urban variations than the national monitoring maps, especially for Period 1.
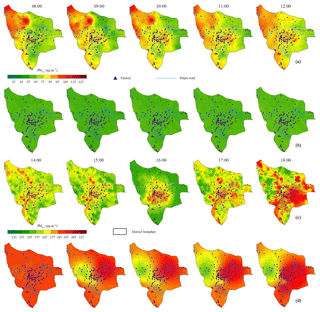
Figure 6Spatial distributions of PM2.5 concentrations from crowdsourced sampling sites and national monitoring stations. (a) Period 1, crowdsourced sampling; (b) Period 1, national monitoring; (c) Period 2, crowdsourced sampling; (d) Period 2, national monitoring.
For Period 1, crowdsourced PM2.5 concentrations generally increased from southeast to northwest with multiple hotspots. In the central and south regions of the study area, areas with a larger number of factories experience a relatively higher PM2.5 concentration than other areas. The national monitoring PM2.5 concentrations, however, were less than 55 µg m−3 with limited spatial variation. For Period 2, with the exception of 14:00, the national monitoring PM2.5 concentration maps showed high-east and low-west patterns. PM2.5 concentrations of the central Yuelu district were rather low (< 175 µg m−3). Crowdsourced PM2.5 concentrations demonstrate extensive cold spots of PM2.5 concentrations in southern Changsha County and the southern Kaifu district, while southern Yuelu and western Tianxin with a high density of factories and roads were hotspots of PM2.5 concentration.
Aimed at efficiently mapping the PM2.5 concentration in an intra-urban area at a fine scale using crowdsourced monitoring, a high-density crowdsourced sampling campaign and strategies of the popular mapping method selection with an increase in training sites were presented in China for the first time.
The number of sampling sites were 18 and 10 per 100 km2 for Period 1 and Period 2, respectively. These data comprise a considerable improvement compared with a density of approximately 0.015 sites per 100 km2 in the national air quality monitoring network in China. As expected, crowdsourced PM2.5 measurements demonstrated detailed spatial variation among urban micro-environments, and these variations can hardly be disclosed by sparse national air quality monitoring stations. This finding suggests that crowdsourced sampling can effectively improve the density of PM2.5 monitoring at a rather low monetary cost and can be supportive of the short-term air pollution exposure assessment for epidemiologic studies at a fine scale. However, persuading the general public to continuously observe and upload PM2.5 concentrations during their activities of daily living through a designed study is difficult. We employed a batch of volunteers to model their behaviours on the general public's behaviour and simultaneously collect data. This approach is a preliminary practice of crowdsourced monitoring and can be further developed and improved in the long-term exposure assessment at the fine scale in the future with progress in low-cost wearable air quality monitors and automatic processing techniques of crowdsourced data.
During the sampling, as the number and the battery life of the monitors are limited, we cannot continuously observe PM2.5 concentration at one site for the whole sampling period. For all potential sites in one area that a volunteer can cover, 27–36 min is needed to walk through three times. That means 24–33 min is left in 1 h to observe two to four potential sites three times. Therefore, at each potential monitoring site, 3 min is designed for PM2.5 concentration constantly monitoring every observation (i.e. Mode One). Although the hourly sampling concentrations are only averages from 9 to 12 min, a total of 540–720 s of measurements, we believe it is generally equal to the hourly averages for the following reasons: (1) the three to four times of 3 min observation happened at rather regular intervals, which can reflect the temporal variation in PM2.5 measurements for one location during 1 h to a certain extent. (2) There are 10 min, a total of 600 s, of measurements per hour for each monitor during the comparison experiments with the national monitoring instruments; the numbers of measurements per hour for crowdsourced campaign and comparison experiments are close, and averages from the 600 s of measurements during the comparison experiments demonstrate a relatively good agreement with the national hourly PM2.5 observations (R2: 0.89–0.90), which provides empirical evidence for this assumption. (3) Several studies have employed sampling periods of 10 min to measure the within-day variability of PM concentrations (Godri et al., 2010; Griffiths et al., 2018) and few studies have proven that the performance characteristics of some low-cost sensors on 1 min and 1 h scales were rather similar (Zheng et al., 2018). However, it needs to be noted that this assumption was proposed under the circumstance that the weather condition and emission sources of the study area demonstrate no extreme variation in the short term. It is inapplicable to some special cases such as a dust storm when the PM2.5 can increase tens and even hundreds of times to a high concentration within a few minutes (Zhang et al., 2010).
The hourly PM2.5 concentrations between crowdsourced sampling sites and national monitoring stations were rather different; this difference varied as the official air quality level changed. The crowdsourced PM2.5 concentrations were substantially larger than the national concentrations in Period 1 (lightly polluted) and slightly lower in Period 2 (heavily polluted). One possible reason is that the national monitoring stations in the study area were installed on the roofs of mid-rise buildings (i.e. ∼15 m) with ventilation and spaciousness, while crowdsourced sampling was conducted on the real ground (i.e. ∼2 m). The PM2.5 concentration may experience a drop above ∼15 m from the ground (Quang et al., 2012; Sajani et al., 2018). The change in the major pollution sources and meteorological conditions in the study area may contribute to the difference between two periods; the major contribution of local sources, especially the vehicle emission and the very high RH (95 %–98 %) during the lightly polluted period, may cause the accumulation of PM2.5 near the ground; and the sources of long-range transport of regional pollution during the heavily polluted period can increase the concentration of PM2.5 in the upper layer. Another possible reason can be the inaccuracies in measurements the low-cost sensor and the use of optical particle detection may cause. For Period 1, the very high RH may lead to an overestimate of PM2.5 concentration. The ratio of laser monitor measurements to the national instrument measurements generally increased exponentially with the increase in the RH for 20–22 December (i.e. the first evaluation period with a weather condition similar to that of the lightly polluted sampling period, RH: 77 %–94 %) (R2=0.2186). This is consistent with the findings of Zheng et al. (2018) and Badura et al. (2018), who discovered that low-cost sensors tend to overestimate the PM2.5 concentrations when RH is high (> ∼80 %). However, the agreement between laser monitor and the national instrument was rather good (R2=0.89) and the improvement after RH correction was insignificant (0.01); the potential effect of RH on hourly PM2.5 concentration during very-high-RH events could be consistent because of the low inter-sensor variability (i.e. the measurement difference under the same condition, < 5 %) of sampling monitors selected from the preliminary experiments and the small spatial variability of RH in intra-urban areas. In view of the above-mentioned reasons, we believe the hourly PM2.5 concentration for the lightly polluted sampling period could generally disclose the air pollution variation in different urban micro-environments although the very-high-RH conditions (RH > 95 %) were not experienced in the evaluation period. For Period 2, the high-concentration environment may cause the underestimates of the low-cost sensor (Zheng et al., 2018; Johnson et al., 2018). However, the monitor experienced few high-concentration environments (> 160 µg m−3) for 29–31 December (i.e. the second evaluation period with a weather condition similar to that of the heavily polluted sampling period). The monitor was compared with the regulatory monitor at concentration levels of 106, 212, and 454 µg m−3 in the test of Tsinghua University and the relative errors were rather low (within ±20 %) and demonstrated similar patterns between concentration levels. In consideration of this and the low inter-sensor variability of sampling monitors, we assume the responses of sampling monitor to the national monitoring instrument in the heavily polluted sampling period are consistent in the study area and the spatial variation in air pollution could be revealed to some extent. As the three methods we compared were performed with the same sampling dataset, the uncertainty in measurements associated with the monitor, RH, and high-concentration environments may cause a limited influence on the method comparison results. We therefore did not correct the measurements in this study. However, more efforts are needed in the intended use environment evaluation, uncertainty analysis, and bias correction of low-cost sensors for applications that require more accurate measurements in the future, such as very-high-resolution mapping of air pollution and accurate exposure assessment, especially under extreme weather conditions and very-high- and very-low-concentration environments.
Unlike previous studies that conducted performance comparisons of OK, LUR, and RK in estimating air pollution concentration on an annual and seasonal scale based on measurements from sparse regulatory stations (Mercer et al., 2011; Lee et al., 2014; Zou et al., 2015; Choi et al., 2017; De Hoogh et al., 2018), this research is the first study to evaluate and compare their performance with an increase in the number of training sites at an hourly scale using crowdsourced monitoring.
As expected, the performance of three methods improved with an increase in the number of training sites. Compared with former studies that normally developed in other fields (e.g. spatial variability analysis of soil components in the environmental sciences) (Li and Heap, 2014), this study further confirmed the better performance of OK interpolation with larger training datasets in air pollution estimation. We substantiated the findings of Johnson et al. (2010), who discovered that LUR models developed with fewer sampling sites may perform poorly using real-ground PM2.5 measurements. However, average holdout validation R2 values (0.04–0.55) between the observed concentration and predicted concentration of PM2.5 in this study were smaller than the results in Johnson et al. (2010) (0.29–0.67) and similar studies of NO2 presented by Wang et al. (2012) and Gillespie et al. (2016) (0.44–0.85). The variations in the hourly average PM2.5 concentration between two sampling sites were generally sharper compared with the annual average values. The meteorological condition had a more sensitive role in the short-term transmission and diffusion of PM2.5 than the long-term processes. These findings suggest that the most effective way to improve the accuracy of the mapping method continues to increase the number of sampling sites and confirm the necessity of developing high-density crowdsourced sampling for PM2.5 monitoring. However, the increased variability ranges of R2 and the standard deviation of RMSE and MRE with an increase in the number of training sites also suggest that the performance of these methods was affected by more than sampling size. The spatial distribution of the samples, for example, may influence their estimating accuracy (Li and Heap, 2014).
Contrary to the findings of Zou et al. (2015) and Choi et al. (2017) conducted at the annual scale, OK interpolation surprisingly showed a better performance in estimating the PM2.5 concentrations compared with the LUR modelling with a substantially higher average R2 and lower RMSE and MRE. RK also performed better than LUR (0.32–0.71 vs. 0.04–0.55), which is consistent with the findings of Mercer et al. (2011) (0.67–0.75 vs. 0.48–0.74) and De Hoogh et al. (2018) (0.66 vs. 0.59). RK had the highest accuracy in Period 2 and at 08:00 and 12:00 of Period 1 with fewer than ∼100 training sites. These results suggest that OK interpolation based on crowdsourced sampling is the best strategy for the PM2.5 mapping in the intra-urban area when the official air pollution levels are good and moderate for non-peak traffic conditions in this study, while RK is the best strategy when the pollution levels are heavily polluted. These findings challenge the traditional point on the LUR model's good performance in air pollution mapping and verify that the applicability of mapping methods varies as the monitoring technology and sampling density change. In addition, the accuracy of OK and LUR were distinctly higher for Period 1 (0.24–0.82; 0.13–0.55) than for Period 2 (0.18–0.59; 0.04–0.42), while that for RK was rather stable (0.40–0.71 vs. 0.32–0.68). This finding indicates the robustness and generalisation capability of RK in estimating the PM2.5 concentration.
Using the selected mapping method, the spatial distributions of the hourly PM2.5 concentration based on crowdsourced sampling data and national air quality observations were successfully plotted and compared. The former distribution provides more information about the intra-urban PM2.5 variations than the latter distribution. The nearest-neighbour distances that range from 15 to 60 m between two crowdsourced sampling sites enable PM2.5 concentration mapping to attain the 100 m scale. In the lightly polluted period, this phenomenon was more pronounced. These findings not only suggest the support of crowdsourced activities in PM2.5 monitoring on a fine scale but also prompt us to pay more attention to the scenarios with low-level air pollution. This outcome is critical to the long-term future of air pollution prevention and control and public health protection for China since the main emphasis has gradually shifted from the control of heavy pollution to the prevention of exposure risks.
As the crowdsourced PM2.5 concentration maps revealed, areas with a larger number of factories and high density of roads experienced relatively higher PM2.5 concentrations, while areas with high levels of green vegetation cover had lower PM2.5 concentrations. The relatively high concentration in the northwest corner of the study area with few factories in Period 1 may be attributed to the dust deposition from construction activities promoted by a high RH in this newly developed zone. This finding suggests that optimising the distribution of land use may improve the air quality to some extent, and strengthening the control of local emission may be the primary way to reduce pollution in the lightly polluted period. As the urban air quality grade has an important effect on the spatial distribution of samples (spatial autocorrelation and heterogeneity), which may also be affected by sample size, the mechanism for this influence is somewhat equivocal and needs further research.
This study presented strategies of method selection for efficient PM2.5 concentration mapping with an increasing number of training sites using crowdsourced monitoring. The results confirmed that PM2.5 concentrations in micro-environments varied across the intra-urban area in China's cities. These variations can be clearly disclosed by the crowdsourced PM2.5 sampling rather than the national air quality monitoring stations. The selection of models for fine-scale PM2.5 concentration mapping should be adjusted with changing sampling and pollution circumstances. During this project, ordinary kriging (OK) interpolation performs the best in conditions with non-peak traffic situations in the lightly polluted period, while regression kriging (RK) can perform better in the heavily polluted period and conditions with peak traffic and relatively few sampling sites in the lightly polluted period. Additionally, note that the land use regression (LUR) model demonstrates a limited ability in estimating PM2.5 concentrations at a very fine scale in this study. This method selection strategy provides empirical evidence for the method selection of PM2.5 mapping using crowdsourced monitoring and a promising way to reduce the exposure risks for individuals in their daily lives.
The national monitoring dataset of PM2.5 is available from the China Environmental Monitoring Center (CEMC), hosted by the Ministry of Ecology and Environment of the People's Republic of China, http://106.37.208.233:20035/ (last access: 31 December 2015). The important point of interest (POI) data, dust surfaces, and road networks for sampling design and land use/cover data for LUR modelling were collected from the Information Center of Land and Resources of Hunan Province. Meteorological data for LUR modelling were derived from the Hunan Meteorology Bureau. These data are not available online due to the non-disclosure agreement between us and relevant departments. The PM2.5 sampling data are not available online according to the local environmental disclosure regulation on information provision; that is, citizens, legal persons, and other organizations cannot release the uncensored environmental information to the public without authorization.
The supplement related to this article is available online at: https://doi.org/10.5194/amt-12-2933-2019-supplement.
SX performed the experiments and wrote the paper text. BZ supervised and designed the research and helped with the paper. YL and XZ helped with the discussion and revisions. SL and CH participated in the data processing.
The authors declare that they have no conflict of interest.
Special thanks go to the volunteers who participated in this crowdsourced sampling campaign. Our deepest gratitude goes to the editor and the anonymous reviewers for their careful work and thoughtful suggestions.
This research has been supported by the National Key Research and Development Program of China (grant no. 2016YFC0206201), the National Key Research and Development Program of China (grant no. 2016YFC0206205), the National Nature Science Foundation of China (grant no. 41871317), and the Innovation Driven Program of Central South University (grant no. 2018CX016).
This paper was edited by Pierre Herckes and reviewed by four anonymous referees.
Apte, J. S., Messier, K. P., Gani, S., Brauer, M., Kirchstetter, T. W., Lunden, M. M., Marshall, J. D., Portier, C. J., Vermeulen, R. C., and Hamburg, S. P.: High-resolution air pollution mapping with google street view cars: exploiting big data, Environ. Sci. Technol., 51, 6999–7009, https://doi.org/10.1021/acs.est.7b00891, 2017.
Badura, M., Batog, P., Drzeniecka-Osiadacz, A., and Modzel, P.: Evaluation of low-cost sensors for ambient PM2.5 monitoring, J. Sensors, 2018, 5096540, 1–16, https://doi.org/10.1155/2018/5096540, 2018.
Beverland, I. J., Cohen, G. R., Heal, M. R., Carder, M., Yap, C., Robertson, C., Hart, C. L., and Agius, R. M.: A comparison of short-term and long-term air pollution exposure associations with mortality in two cohorts in Scotland, Environ. Health Persp., 120, 1280–1285, https://doi.org/10.1289/ehp.1104509, 2012.
Choi, G., Bell, M. L., and Lee, J. T.: A study on modeling nitrogen dioxide concentrations using land-use regression and conventionally used exposure assessment methods, Environ. Res. Lett., 12, 044003, https://doi.org/10.1088/1748-9326/aa6057, 2017.
Cohen, A. J., Brauer, M., Burnett, R., Anderson, H. R., Frostad, J., Estep, K., Balakrishnan, K., Brunekreef, B., Dandona, L., Dandona, R., Feigin, V., Freedman, G., Hubbell, B., Jobling, A., Kan, H., Knibbs, L., YangLiu, Y., Martin, R., Morawska, L., Pope III, A., Shin, H., Straif, K., Shaddick, G., Thomas, M., van Dingenen, R., Donkelaar, A., Vos, T., Murray, C. J. L., and Forouzanfar, M. H.: Estimates and 25-year trends of the global burden of disease attributable to ambient air pollution: an analysis of data from the global burden of diseases study 2015, Lancet, 389, 1907–1918, https://doi.org/10.1016/S0140-6736(17)30505-6, 2017.
De Hoogh, K., Chen, J., Gulliver, J., Hoffmann, B., Hertel, O., Ketzel, M., Bauwelinck, M., van Donkelaar, A., Hvidtfeldt, U. A., Katsouyanni, K., Klompmaker, J., Martin, R. V., Samoli, E., Schwartz, P. E., Stafoggia, M., Bellander, T., Strak, M., Wolf, K., Vienneau, D., Brunekreef, B., and Hoek, G.: Spatial PM2.5, NO2, O3 and BC models for Western Europe-Evaluation of spatiotemporal stability, Environ. Int., 120, 81–92, https://doi.org/10.1016/j.envint.2018.07.036, 2018.
Di, Q., Dai, L., Wang, Y., Zanobetti, A., Choirat, C., Schwartz, J. D., and Dominici, F.: Association of short-term exposure to air pollution with mortality in older adults, Jama, 318, 2446–2456, https://doi.org/10.1001/jama.2017.17923, 2017.
El-Harbawi, M.: Air quality modelling, simulation, and computational methods: a review, Environ. Rev., 21, 149–179, https://doi.org/10.1139/er-2012-0056, 2013.
Fang, X., Zou, B., Liu, X., Sternberg, T., and Zhai, L.: Satellite-based ground PM2.5 estimation using timely structure adaptive modeling, Remote Sens. Environ., 186, 152–163, https://doi.org/10.1139/er-2012-0056, 2016.
Fox, J. and Weisberg, S.: An R companion to applied regression, 2nd edn., SAGE Publications, Inc., 2455 Teller Road, Thousand Oaks, California 91320, USA, 2011.
Gillespie, J., Beverland, I., Hamilton, S., and Padmanabhan, S.: Development, evaluation, and comparison of land use regression modeling methods to estimate residential exposure to nitrogen dioxide in a cohort study, Environ. Sci. Technol., 50, 11085–11093, https://doi.org/10.1021/acs.est.6b02089, 2016.
Godri, K. J., Harrison, R. M., Tim, E., Timothy, B., Christina, D., Mudway, I. S., and Kelly, F. J.: Increased oxidative burden associated with traffic component of ambient particulate matter at roadside and urban background schools sites in London, PLoS ONE, 6, e21961, https://doi.org/10.1371/journal.pone.0021961, 2011.
Griffiths, S. D., Chappell, P., Entwistle, J. A., Kelly, F. J., and Deary, M. E.: A study of particulate emissions during 23 major industrial fires: implications for human health, Environ. Int., 112, 310–323, https://doi.org/10.1016/j.envint.2017.12.018, 2018.
Hankey, S., Lindsey, G., and Marshall, J. D.: Population-level exposure to particulate air pollution during active travel: planning for low-exposure, health-promoting cities, Environ. Health Persp., 125, 527–534, https://doi.org/10.1289/EHP442, 2017.
Heipke, C.: Crowdsourcing geospatial data, ISPRS J. Photogramm., 65, 550–557, https://doi.org/10.1016/j.isprsjprs.2010.06.005, 2010.
Henderson, S. B., Beckerman, B., Jerrett, M., and Brauer, M.: Application of land use regression to estimate long-term concentrations of traffic-related nitrogen oxides and fine particulate matter, Environ. Sci. Technol., 41, 2422–2428, https://doi.org/10.1021/es0606780, 2007.
Jerrett, M., Arain, A., Kanaroglou, P., Beckerman, B., Potoglou, D., Sahsuvaroglu, T., Morrison, J. and, Giovis, C.: A review and evaluation of intraurban air pollution exposure models, J. Expo. Anal. Env. Epid., 15, 185–204, https://doi.org/10.1038/sj.jea.7500388, 2005.
Jerrett, M., Donaire-Gonzalez, D., Popoola, O., Jones, R., Cohen, R. C., Almanza, E., de Nazelle, A., Mead, I., Carrasco-Turigas, G., Cole-Hunter, T., Triguero-Mas, M., Seto, E., and Nieuwenhuijsen, M.: Validating novel air pollution sensors to improve exposure estimates for epidemiological analyses and citizen science, Environ. Res., 158, 286–294, https://doi.org/10.1016/j.envres.2017.04.023, 2017.
Johnson, K. K., Bergin, M. H., Russell, A. G., and Hagler, G. S. W.: Field test of several low-cost particulate matter sensors in high and low concentration urban environments, Aerosol Air Qual. Res., 18, 565–578, https://doi.org/10.4209/aaqr.2017.10.0418, 2018.
Johnson, M., Isakov, V., Touma, J. S., Mukerjee, S., and Özkaynak, H.: Evaluation of land-use regression models used to predict air quality concentrations in an urban area, Atmos. Environ., 44, 3660–3668, https://doi.org/10.1016/j.atmosenv.2010.06.041, 2010.
Kim, S. Y., Yi, S. J., Eum, Y. S., Choi, H. J., Shin, H., Ryou, H. G., and Kim, H.: Ordinary kriging approach to predicting long-term particulate matter concentrations in seven major Korean cities, Environ. Health Toxicol., 29, e2014012, https://doi.org/10.5620/eht.e2014012, 2014.
Kingham, S., Longley, I., Salmond, J., Pattinson, W., and Shrestha, K.: Variations in exposure to traffic pollution while travelling by different modes in a low density, less congested city, Environ. Pollut., 181, 211–218, https://doi.org/10.1016/j.envpol.2013.06.030, 2013.
Kumar, P., Morawska, L., Martani, C., Biskos, G., Neophytou, M., Di, S. S., Bell, M., Norford, L., and Britter, R.: The rise of low-cost sensing for managing air pollution in cities, Environ. Int., 75, 199–205, https://doi.org/10.1016/j.envint.2014.11.019, 2015.
Lee, J. H., Wu, C. F., Hoek, G., De, H. K., Beelen, R., Brunekreef, B., and Chan, C. C.: Land use regression models for estimating individual NOx and NO2 exposures in a metropolis with a high density of traffic roads and population, Sci. Total Environ., 472, 1163–1171, https://doi.org/10.1016/j.scitotenv.2013.11.064, 2014.
Lelieveld, J., Evans, J. S., Fnais, M., Giannadaki, D., and Pozzer, A.: The contribution of outdoor air pollution sources to premature mortality on a global scale, Nature, 525, 367–371, https://doi.org/10.1038/nature15371, 2015.
Li, J. and Heap, A. D.: Spatial interpolation methods applied in the environmental sciences: a review, Environ. Modell. Softw., 53, 173–189. https://doi.org/10.1016/j.envsoft.2013.12.008, 2014.
Liu, Y., Cao, G. F., Zhao, N. Z., Mulligan, K., and Ye, X. Y.: Improve ground-level PM2.5 concentration mapping using a random forests-based geostatistical approach, Environ. Pollut., 235, 272–282, https://doi.org/10.1016/j.envpol.2017.12.070, 2018.
Mercer, L. D., Szpiro, A. A., Sheppard, L., Lindström, J., Adar, S. D., Allen, R. W., Avol, E. L., Oron, A. P., Larson, T., Liu, L. J., and Kaufman, J. D.: Comparing universal kriging and land-use regression for predicting concentrations of gaseous oxides of nitrogen (NOx) for the multi-ethnic study of atherosclerosis and air pollution (MESA Air), Atmos. Environ., 45, 4412–4420, https://doi.org/10.1016/j.atmosenv.2011.05.043, 2011.
Miskell, G., Salmond, J., and Williams, D. E.: Low-cost sensors and crowd-sourced data: observations of siting impacts on a network of air-quality instruments, Sci. Total Environ., 575, 1119–1129, https://doi.org/10.1016/j.scitotenv.2016.09.177, 2017.
Pang, W., Christakos, G., and Wang, J. F.: Comparative spatiotemporal analysis of fine particulate matter pollution, Environmetrics, 21, 305–317, https://doi.org/10.1002/env.1007, 2010.
Quang, T. N., He, C., Morawska, L., Knibbs, L. D., and Falk, M.: Vertical particle concentration profiles around urban office buildings, Atmos. Chem. Phys., 12, 5017–5030, https://doi.org/10.5194/acp-12-5017-2012, 2012.
Rice, M. B., Ljungman, P. L., Wilker, E. H., Dorans, K. S., Gold, D. R., Schwartz, J., Koutrakis, P., Washko, G. R., O'Connor, G. T., and Mittleman, M. A.: Long-term exposure to traffic emissions and fine particulate matter and lung function decline in the framingham heart study, Am. J. Respir. Crit. Care. Med., 191, 656–64, https://doi.org/10.1164/rccm.201410-1875OC, 2015.
Sajani, S. Z., Marchesi, S., Trentini, A., Bacco, D., Zigola, C., Rovelli, S., Ricciardelli, I., Maccone, C., Lauriola, P., Cavallo, D. M., Poluzzi, V., Cattaneo, A., and Harrison, R.: Vertical variation of PM2.5 mass and chemical composition, particle size distribution, NO2, and BTEX at high rise building, Environ. Pollut., 235, 339–349, https://doi.org/10.1016/j.envpol.2017.12.090, 2018.
Saraswat, A., Apte, J. S., Kandlikar, M., Brauer, M., Henderson, S. B., and Marshall, J. D.: Spatiotemporal land use regression models of fine, ultrafine, and black carbon particulate matter in new Delhi, India, Environ. Sci. Technol., 47, 12903–12911, https://doi.org/10.1021/es401489h, 2013.
Schneider, P., Castell, N., Vogt, M., Dauge, F. R., Lahoz, W. A., and Bartonova, A.: Mapping urban air quality in near real-time using observations from low-cost sensors and model information, Environ. Int., 106, 234–247, https://doi.org/10.1016/j.envint.2017.05.005, 2017.
Thompson, J. E.: Crowd-sourced air quality studies: a review of the literature & portable sensors, T. Environ. Anal. Chem., 11, 23–34, https://doi.org/10.1016/j.teac.2016.06.001, 2016.
R Core Team: R: A language and environment for statistical computing, R foundation for statistical computing, Vienna, Austria, available at: http://www.R-project.org, last access: 10 November 2016.
Wang, M., Beelen, R., Eeftens, M., Meliefste, K., Hoek, G., and Brunekreef, B.: Systematic evaluation of land use regression models for NO2, Environ. Sci. Technol., 46, 4481–4489, https://doi.org/10.1021/es204183v, 2012.
Xu, S., Zou, B., Shafi, S., and Sternberg, T.: A hybrid Grey-Markov/ LUR model for PM10 concentration prediction under future urban scenarios, Atmos. Environ., 187, 401–409, https://doi.org/10.1016/j.atmosenv.2018.06.014, 2018.
Zhai, L., Li, S., Zou, B., Sang, H., Fang, X., and Xu, S.: An improved geographically weighted regression model for PM2.5 concentration estimation in large areas, Atmos. Environ., 181, 145–154, https://doi.org/10.1016/j.atmosenv.2018.03.017, 2018.
Zhang, W., Xu, D., Zhuang, G., Wang, W., and Guo, L.: Characteristics of ambient 1-min PM2.5 variation in Beijing, Environ. Monit. Assess., 165, 137–146, https://doi.org/10.1007/s10661-009-0933-6, 2010.
Zheng, T., Bergin, M. H., Johnson, K. K., Tripathi, S. N., Shirodkar, S., Landis, M. S., Sutaria, R., and Carlson, D. E.: Field evaluation of low-cost particulate matter sensors in high- and low-concentration environments, Atmos. Meas. Tech., 11, 4823–4846, https://doi.org/10.5194/amt-11-4823-2018, 2018.
Zou, B., Luo, Y., Wan, N., Zheng, Z., Sternberg, T., and Liao, Y.: Performance comparison of LUR and ok in PM2.5 concentration mapping: a multidimensional perspective, Sci. Rep., 5, 8698, https://doi.org/10.1038/srep08698, 2015.
Zou, B., Pu, Q., Bilal, M., Weng, Q., Zhai, L., and Nichol, J. E.: High-resolution satellite mapping of fine particulates based on geographically weighted regression, IEEE Geosci. Remote. S., 13, 495–499, https://doi.org/10.1109/LGRS.2016.2520480, 2017.