the Creative Commons Attribution 4.0 License.
the Creative Commons Attribution 4.0 License.
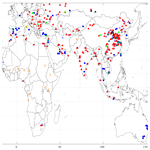
Tracking down global NH3 point sources with wind-adjusted superresolution
Martin Van Damme
Cathy Clerbaux
Pierre-François Coheur
As a precursor of atmospheric aerosols, ammonia (NH3) is one of the primary gaseous air pollutants. Given its short atmospheric lifetime, ambient NH3 concentrations are dominated by local sources. In a recent study, Van Damme et al. (2018) have highlighted the importance of NH3 point sources, especially those associated with feedlots and industrial ammonia production. Their emissions were shown to be largely underestimated in bottom-up emission inventories. The discovery was made possible thanks to the use of oversampling techniques applied to 9 years of global daily IASI NH3 satellite measurements. Oversampling allows one to increase the spatial resolution of averaged satellite data beyond what the satellites natively offer. Here we apply for the first time superresolution techniques, which are commonplace in many fields that rely on imaging, to measurements of an atmospheric sounder, whose images consist of just single pixels. We demonstrate the principle on synthetic data and on IASI measurements of a surface parameter. Superresolution is a priori less suitable to be applied on measurements of variable atmospheric constituents, in particular those affected by transport. However, by first applying the wind-rotation technique, which was introduced in the study of other primary pollutants, superresolution becomes highly effective in mapping NH3 at a very high spatial resolution. We show that plume transport can be revealed in greater detail than what was previously thought to be possible. Next, using this wind-adjusted superresolution technique, we introduce a new type of NH3 map that allows tracking down point sources more easily than the regular oversampled average. On a subset of known emitters, the source could be located within a median distance of 1.5 km. We subsequently present a new global point-source catalog consisting of more than 500 localized and categorized point sources. Compared to our previous catalog, the number of identified sources more than doubled. In addition, we refined the classification of industries into five categories – fertilizer, coking, soda ash, geothermal and explosives industries – and introduced a new urban category for isolated NH3 hotspots over cities. The latter mainly consists of African megacities, as clear isolation of such urban hotspots is almost never possible elsewhere due to the presence of a diffuse background with higher concentrations. The techniques presented in this paper can most likely be exploited in the study of point sources of other short-lived atmospheric pollutants such as SO2 and NO2.
- Article
(9378 KB) - Full-text XML
- BibTeX
- EndNote
As one of the primary forms of reactive nitrogen, NH3 is essential in many of the Earth's biogeochemical processes. It is naturally present along with the nitrogen oxides in the global nitrogen cycle (Canfield et al., 2010; Fowler et al., 2013). However, the discovery of ammonia synthesis through the Haber–Bosch process in the early 1900s has made this vital compound available in almost unlimited quantities, supporting the explosive population growth in the last century (Erisman et al., 2008). As a result, the nitrogen cycle is currently perturbed beyond the safe operating space for humanity, which has led to a host of environmental and societal problems (Steffen et al., 2015). The most obvious direct impact of excess NH3 is that on air quality, as atmospheric NH3 is one of the main precursors of secondary particulate matter, which has important adverse health impacts (Lelieveld et al., 2015; Bauer et al., 2016). Emissions of the two other important precursors (SO2 and NOx), thanks to effective legislation, drastically decreased in the past 20 years in Europe and North America and have started to level off in eastern Asia (Aas et al., 2019; Georgoulias et al., 2019). In contrast, no such decreases are observed or expected in the near future for NH3 (e.g., Warner et al., 2017; Sutton et al., 2013). Unlike the other precursors, NH3 emissions are not well regulated, and in fact, the focus on decreasing NOx and SO2 has already led to increased NH3 emissions (Chang et al., 2016) and concentrations (Lachatre et al., 2018; Liu et al., 2018).
The lack of a global regulative framework stems in part from the historical relative difficulty in measuring NH3 concentrations. Satellite-based measurements of NH3, which were discovered about a decade ago, offer an attractive complementary means of monitoring NH3. Satellite datasets have now reached sufficient maturity to be directly exploitable, even when the individual measurements come with large and variable uncertainties. Using satellite observations we have recently shown the importance of ammonia point sources on regional scales (Van Damme et al., 2018). In total, over 240 of the world's strongest point sources were identified, categorized and quantified. Somewhat expectedly, many of these point sources (or clusters thereof) were found to be associated with “concentrated animal feeding operations” (CAFOs; Zhu et al., 2015; Yuan et al., 2017). However, much more surprisingly was the number of identified industrial emitters and in particular those associated with ammonia and urea-based fertilizer production. An evaluation of the EDGAR inventory additionally showed that emission inventories vastly underestimate the majority of all point-source emissions, even when a conservative average NH3 lifetime of 12 h is assumed in the calculation of the satellite-derived fluxes. Industrial processes could therefore be extremely important, especially on a regional scale. Altogether, these findings were made possible due to the availability of the large multiyear NH3 dataset (Whitburn et al., 2016; Van Damme et al., 2017) derived from measurements of the IASI spaceborne instrument (Clerbaux et al., 2009) and the oversampling technique that was applied to sufficiently resolve localized emitters.
Oversampling techniques applied on measurements of satellite sounders allow obtaining average distributions of atmospheric constituents at a higher spatial resolution than the original measurements (Sun et al., 2018). They exploit the fact that the footprint on the ground of satellite measurements varies in location, size and shape each time the satellite samples an area. When pixels partially overlap, some information becomes available on their (subpixel) intersection. High-resolution mapping can, however, only be obtained by combining typically many hundreds of measurements. A crucial condition on which oversampling relies is that the pixel center and ground instantaneous field of view (GIFOV) of satellite measurements is known with a high accuracy (typically <1 km, as opposed to the coarse spatial resolution of the extent of the satellite pixel, which is typically >10 km). Practical implementation of oversampling is relatively straightforward once the footprint is known: a fine subgrid is constructed in which the value of each cell of the grid is obtained as the average value of all overlapping GIFOVs. Optionally, the averaging can be weighted to take into account measurement error, total pixel surface area and spatial response function. We refer to Sun et al. (2018) and Van Damme et al. (2018) for comprehensive reference material on averaging and oversampling, detailed algorithmic descriptions, and practical considerations for their implementation.
Oversampling has gradually become commonplace in the field of atmospheric remote sensing, especially in the study of short-lived pollutants such as NO2 (Wenig et al., 2008; Russell et al., 2010), SO2 (Fioletov et al., 2011, 2013), HCHO (Zhu et al., 2014) and NH3 (Van Damme et al., 2014, 2018). The increased spatial resolution enables in the first instance a much better identification of emission (point) sources, quantification of their emissions (Streets et al., 2013), and study of transport and plume chemistry (de Foy et al., 2009). Oversampling applied to the study of point sources becomes even more useful when wind fields are taken into account. Beirle et al. (2011) showed that binned averaging per wind direction allows simultaneous estimates of both emission strengths and atmospheric residence times. Valin et al. (2013) and Pommier et al. (2013) introduced the wind-rotation technique whereby each observation is rotated around the presumed point source according to the horizontal wind direction, effectively yielding a distribution in which the winds blow in the same direction. As we will also demonstrate (see Sect. 3), this reduces the overall spread of the transported pollutants and reduces contributions of nearby sources. Combining plume rotation with oversampling has proven to be a very successful technique for the study of NO2 and SO2 point sources, leading to massively improved inventories and emission estimates and better constraints on the atmospheric lifetime of these pollutants (Fioletov et al., 2015, 2016, 2017; Wang et al., 2015; de Foy et al., 2015; Lu et al., 2015; Liu et al., 2016; McLinden et al., 2016).
However, as pointed out in Sun et al. (2018), while oversampling offers an increased resolution, it still yields a smoothed representation of the true distributions. There exists a large field of research, collectively referred to as superresolution (Milanfar, 2010), that attempts to construct high-resolution images from several possibly moving or distorted low-resolution representations of the same reality. Oversampling is in essence the simplest way of performing superresolution but in a way that does not fully exploit the spatial information of the measurements. Superresolution has been applied before in the field of remote sensing of land or land cover (e.g., Boucher et al., 2008; Xu et al., 2017), but even though it is theoretically possible, it has not been applied to atmospheric-sounding measurements. In this case, the “images”, as taken by sounders, are of the lowest resolution; i.e., they correspond to single, uniformly colored pixels. Perhaps the main reason why superresolution has not been attempted before on atmospheric sounders is that these rely on the fact that the low-resolution samples should be derived from an underlying distribution that does not change in time (de Foy et al., 2009). When this is not the case, the smoothing introduced by oversampling is actually desirable. With the arrival of the wind-rotation technique, most of the variability observed for point-source emitters can be corrected for, and therefore superresolution becomes viable for short-lived species as NH3.
In Sect. 2 we introduce superresolution and demonstrate its effectiveness on measurements of the IASI sounder for a parameter related to (constant) surface emissivity. Next we illustrate the application of what we coin “wind-adjusted supersampling” on an industrial point source of NH3. In Sect. 3 we use ideas from McLinden et al. (2016) to provide a new type of NH3 map, one that is supersampled and wind-corrected at the same time. This map enables the identification of many new point sources in addition to the ones reported in Van Damme et al. (2018). We performed a detailed global analysis of this new map, which led to the identification and categorization of more than 500 point sources and which we present in Sect. 4.
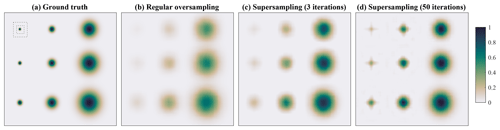
Figure 1Illustration of the supersampling technique on synthetic data. Panel (a) depicts an imaginary ground truth, made up of two-dimensional Gaussian distributions, each with a different spread (0.5 to 40 pixels). The rectangles in (a) indicate the assumed footprint size of the measurements, varying between 7 and 13 pixels. Panel (b) shows the results of the common oversampling approach applied to 100 000 measurements scattered randomly over the area. Panels (c, d) provide the results of the supersampling technique after respectively 3 and 50 iterations.
The general superresolution problem does not have a unique solution, as the available low-resolution measurements typically do not hold all the required information content (i.e., the problem is underdetermined). It is usually also overdetermined because of measurement noise and, for our case especially, because of temporal variability. As a consequence, there is no unique best algorithm, and a myriad of alternatives exist. For this study, we chose the iterative back-projection (IBP) algorithm (Irani and Peleg, 1993), as it takes a particular intuitive and simple form for single-pixel satellite observations and allows addressing the ill-determined nature of the problem. It proceeds as follows. Suppose we have a set of single-pixel measurements M0 of a spatially variable quantity. For the first iteration, the solution of the algorithm corresponds to the regular oversampling, which we will write as (SSi stands for the solution of the supersampling obtained in iteration i, and OS stands for the oversampling operator). From this oversampled average, we then calculate simulated observations for each of the original individual observations, corresponding to what the instrument would see if the ground truth were SS1. The entire set of these simulated measurements will be denoted by M1=M(SS1) (with M the operator that simulates the measurements). If the oversampled average OS1=SS1 corresponds to the ground truth, then M1 would clearly coincide with M0. However, as oversampling typically smooths out the observations, this is generally not the case. An improved estimate of the average (SS2) can be obtained by adding OS(M0−M1) to the oversampled average, therefore correcting (partially) the observed differences. This process then is repeated to obtain increasingly better estimates. The entire algorithm thus reads
The solution converges to an average that is maximally consistent with the observations, i.e., M0≈Mk for sufficiently large k (as shown in Elad and Feuer, 1997, IBP converges to the maximum likelihood estimate whereby M0−Mk is minimized). Figure 1 illustrates the algorithm on synthetic data with an idealized ground truth made up of nine point sources (Fig. 1a), with a Gaussian spread between 0.5 and 40 pixels. The measurement footprint was assumed to be variable between 7 and 13 pixels. The SS1 (Fig. 1b), SS3 (Fig. 1c) and SS50 (Fig. 1d) averages illustrate the convergence and strengths of the algorithm well, which reproduces most of the point sources near perfectly and even partly resolves the smallest feature (compare also with Sun et al., 2018, Fig. 8). Some small ringing effects are noticeable though after 50 iterations (best visible on a screen), which are the result of the undetermined nature of the problem (Dai et al., 2007).
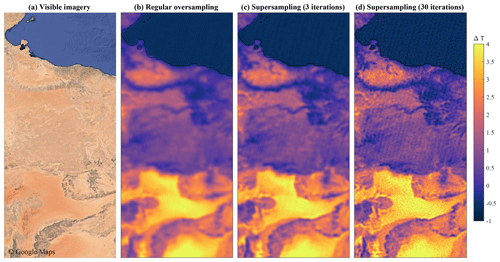
Figure 2Illustration of the IBP superresolution technique on IASI observations of a BTD sensitive to surface quartz. All cloud-free observations of IASI for the period 2007–2018 were used for the averages. Panel (a) shows the corresponding visible imagery from Google Maps.
An example applied to real data is shown in Fig. 2, which shows part of the Sahara and Mediterranean Sea. The quantity on which the oversampling is applied is the brightness temperature difference (BTD) between the IASI channels at 1157 and 1168 cm−1. This BTD, located in the atmospheric window, is sensitive to the sharp change in surface emissivity due to the presence of quartz (see Takashima and Masuda, 1987, who also illustrate that the relevant feature is not seen in airborne dust). Being related to the surface, it can be assumed to be reasonably constant for each overpass of IASI (note that it is not entirely the case: sand dunes do undergo changes over time, and surface emissivities can depend on the viewing angle and can be affected by changes in moist content). Comparison with visible imagery (Fig. 2a) shows, as expected, that the largest BTD values (>4 K) are associated with the most sandy areas. The other desert areas exhibit widely varying values, and oceans are slightly negative. The oversampled average (Fig. 2b) captures most large features down to about 5 km in size. Recalling that the footprint of IASI is a 12 km diameter circle at nadir, and elongates to an ellipse of up to 20 km×39 km at off-nadir angles, this example illustrates why oversampling is such a powerful technique. However, the additional resolution brought by the supersampling is clear, even after three iterations. The smallest features that can be distinguished are about 3–4 km (after three iterations; Fig. 2c) and 2–3 km (after 30 iterations; Fig. 2d) in diameter. That said, with increasing iterations, artifacts start to appear due to enhancements of noise and the specific sampling of IASI (in particular, stripes parallel to the orbit track become apparent). Such overfitting to the data and a sensitivity to outliers is often seen in maximum likelihood optimizations (Milanfar, 2010). It can therefore be advantageous to stop the algorithm after a few iterations (which can also be required for computational reasons).
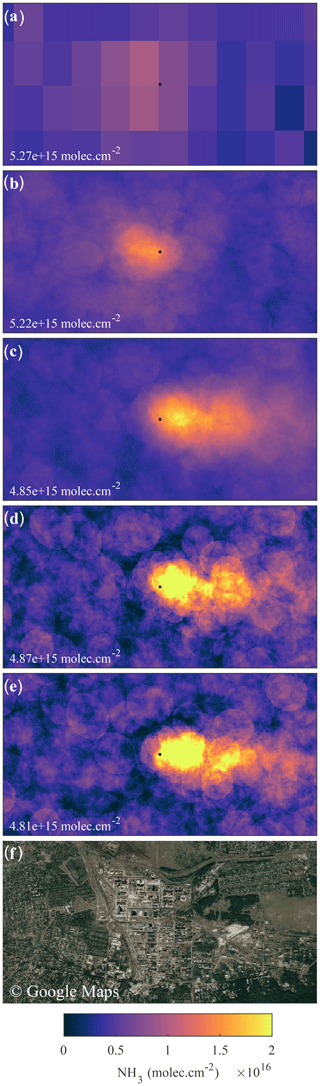
Figure 3Averaging techniques illustrated on the ammonia plant at Horlivka on IASI NH3 data between 2007 and 2013. From top to bottom: (a) gridded average; (b) oversampled average; (c) wind-rotated oversampling, with the rotation center located at the maximum of (b); (d) wind-rotated supersampling, with the rotation center located at the maximum of (b); and (e) wind-adjusted supersampling around the assumed source (center of the plant). (f) Zoomed-in view (factor of 20) over the ammonia plant (data: © Google Maps). For each subpanel, the number in the bottom left corner is the total average NH3 column amount over the entire area.
In this section we illustrate the previously introduced supersampling on a wind-rotated NH3 average centered around a point source. The ammonia plant at Horlivka (Gorlovka), Ukraine, was chosen as a test case. This plant made the news in 2013 because of the major NH3 leak that occurred on 6 August, killing five people and injuring many more. We refer to the Wikipedia article for a detailed description of the event and a list of related newspaper articles (Wikipedia, 2019). The accident itself was not detected by IASI, but an abrupt drop in the average concentrations after the incident is seen in the satellite observations. In fact, after 2013 NH3 enhancements are no longer detected at or near the plant. Figure 3 illustrates the processes of oversampling, supersampling and wind rotation on IASI data from 2007 to 2013. Each subpanel depicts the 120 km×60 km area centered around the plant, from top to bottom.
- a.
Gridded average. In the regular gridded average, each grid cell is assigned the arithmetic average of all observations whose center falls into the grid cell. This method only gives a faithful representation for larger grid-cell sizes, whereas smaller grid sizes provide a higher resolution at the cost of larger noise. Here a grid size of was chosen. NH3 enhancements are seen in a wide area around the plant, with a maximum west of the plant of 1×1016 molec. cm−2.
- b.
Oversampled average. Oversampling the daily maps before averaging increases the resolution and reveals the point-source nature of the emission, with a maximum close to the plant (around 1.6×1016 molec. cm−2).
- c.
Wind-rotated oversampling. The wind-rotation technique (Valin et al., 2013; Fioletov et al., 2015) consists of rotating the map of daily observations around a presumed point source and along the direction of the wind direction at that point. The rotation was applied here to align the winds in the x direction. Daily horizontal wind fields were taken from the ERA5 reanalysis (ERA5, 2019) and interpolated at an altitude equal to half of the boundary layer height. The NH3 average shown in this panel was obtained via oversampling applied to all the daily wind-rotated maps. It is important to note that such a distribution can no longer be interpreted as a geographical map, since each pixel is an average of measurements taken at different places. The only map element that is preserved is the distance to the point source. However, looking at the resulting distribution, the advantages brought by wind rotation are obvious. Whereas in the normal oversampled average the NH3 enhancements are scattered across, aligning the winds significantly enhances both the source and transport (with a maximum of 2×1016 molec. cm−2).
- d.
Wind-adjusted supersampling (i). The figure in this panel was obtained from wind-rotated daily maps, as in the previous panel, but this time the average was calculated with three iterations of the IBP supersampling algorithm. As explained above, supersampling offers most benefits when the underlying distribution can be assumed to be reasonably constant, which is in part achieved by aligning the winds. The resolution is further increased, and as the plume is much less smoothed out, maximum observed columns are also much higher (3.3×1016 molec. cm−2). Note that in general for NH3, three iterations of the IBP algorithm seem to offer a good compromise of increasing the resolution of the average without introducing artifacts related to overfitting.
- e.
Wind-adjusted supersampling (ii). In Fig. 3c and d, the point-source location was taken from Van Damme et al. (2018), where the locations were determined based on the location of the maxima in the oversampled averages. In this last panel, the rotation was applied around the center of the presumed source (the chemical plant). The performance of the wind rotation is further enhanced, yielding a distribution fully consistent with that of a single emitting point source whose emissions undergo transport in a fixed direction. The part of the plume located furthest from the source is a bit off axis, which is probably caused by inhomogeneities in the wind fields across the entire scene. This panel also illustrates the sensitivity of the rotation method to small shifts in the location of the center, a fact that we will exploit in the next section.
One useful property of the different procedures is that they all approximately conserve the quantity that is being averaged; i.e., the averaged quantity in each grid is approximately the same as the average quantity in the grid representing the ground truth given that there is a sufficient number of measurements across the entire grid. When the number of measurements is low, this can break down dramatically, as can be seen with the extreme example of a single high-value measurement over an isolated point source. When a gridded average is made from this single measurement onto a coarse grid (e.g., ), the entire grid cell containing the measurement will be associated with this high value, thus overestimating reality. A strict conservation is therefore not possible in general, as not enough information is contained in the original measurements to reconstruct the ground-truth perfectly even on average. That being said, supersampling conserves quantity with respect to the original measurements, when the number of iterations is large enough. This is a consequence of the fact that the back-projected measurements converge to the actual measurements and therefore also their averages. Finally note that wind rotation does not alter the grid average, as rotation simply redistributes the measurements to different locations on the grid. The average total NH3 columns are indicated in each subpanel of Fig. 3. The average of the ungridded measurements within the considered box equals 5.23×1015 molec. cm−2. As can be seen, the largest change in the average column is caused by the rotation procedure, but this is simply an artifact caused by limiting the average to a square box around a point source (instead of a circle). This example illustrates that in practice, with differences smaller than 1 %, the different gridding procedures can be assumed to conserve quantity.
Having demonstrated the effectiveness of both the wind-rotation and supersampling approaches in revealing point sources, we are now in a position to introduce a new type of NH3 map, specifically designed to track down point sources. It is based on a similar map presented in McLinden et al. (2016) for SO2, but some important differences were introduced here to make it work for NH3. The main idea of McLinden et al. (2016) is to treat each location on Earth as a potential point source and to assign it a value proportional to the downwind (the source) minus the upwind (the background) column. In particular, for a given location, a wind-rotated average is constructed first, similar to Fig. 3c. Representative average columns are then obtained downwind and upwind from the potential source (e.g., in boxes of 10 km×10 km). Finally, the difference of the upwind and downwind average is calculated, and this value is then used to represent the point-source column at that specific location. While the method works nicely for SO2, this method proved to be only moderately successful when we applied it to the IASI NH3 data. In particular, for those places where area sources dominate or where point sources are clustered over too large an area, local variation in the columns produces a noisy map, with many fictitious point sources.
We found that instead of the differences, the downwind average alone produced a more representative point-source map. In addition, applying the method not on the oversampled average but on the supersampled one allows increasing the resolution. There are two key advantages offered by a point-source map constructed in this way as opposed to a regular oversampled average: brighter point sources and smoother (lower) values over the background. The fact that point sources appear brighter is a direct consequence of the plume concentration achieved with wind-rotated supersampling, as shown in the previous section. Smoothing of the background is accomplished by the process of averaging the area downwind. However, by applying the method not on an oversampled average but on a supersampled one, this smoothing is partially offset for point sources. The resulting point-source map has a similar horizontal resolution to the oversampled map, but with increased averaged columns at the point sources and a smoother background distribution.
Examples over two selected regions in North America are shown in Fig. 4b and d. In these examples, the downwind averages were calculated in boxes extending from −5 to 5 km in the y direction and 0 to 20 km in the x direction. Figure 4a and c correspond to the regular oversampled averages. In Fig. 4a, which shows the oversampled average of the southern part of the Saskatchewan province of Canada, no point sources are apparent in the patchy NH3 distribution. The corresponding point-source map, shown in Fig. 4, is smoother over areas dominated by the diffuse sources, where column variations are close to the measurement uncertainty. In addition, two bright spots are evident, which upon investigation coincide with the location of an ammonia plant (Belle Plaine) and a very large feedlot (>2 km in length) near the town of Lanigan. Looking back at the oversampled average, even with the advantage of hindsight, these sources can hardly be singled out. Figure 4c and d show the southwestern part of Kansas, US. It is an area well known for its cattle (Harrington and Lu, 2002). In Van Damme et al. (2018) several point sources associated with feedlots were isolated in Kansas and the rest of the High Plains region, but most of the area was found to be too diffuse to allow identification of individual point sources. The NH3 point-source map facilitates greatly the attribution of these. This is due to the reduction in noise and the fact that the main point sources contrast much more with the background. An added benefit of this is that the location of the maxima in the map is in general closer to the actual emission source than is the case in the oversampled map, making it easier to track down the suspected source with visible imagery and therefore also to assign and identify the point source.
Displaced maxima that are seen in regular averages (wind-adjusted or not) can also be the result of transport, as noted by Van Damme et al. (2018), who found that especially for coastal sites, the shift can be as much as 20 km. The suspected reason is vertical uplift during transport, which makes NH3 easier to detect and to measure (as can be seen in Fig. 3c) downwind of the source. The way in which the point-source map is set up corrects for the effects of transport, as the columns are partially reallocated back to their source by assigning the average downwind column to the point source. We have quantified the ability to locate sources on a careful selection of 36 industrial emitters. These were all chosen to be relatively isolated, with no nearby other industries or other sources, so that the actual emitting source is known with confidence. In addition, only small- to medium-sized plants were chosen (<1 km across) so that the precise location of the emission is known within a distance of about 500 m. For the regular oversampled map, the sources were found within a median distance of 3.9 km and a mean of 5.4±3.7 km. The furthest distance was 15.2 km. For the point-source map, all but five sites were located within 3 km (with a median of 1.5 km, a mean of 2.1±1.7 km and a maximum of 7.3 km), which confirms its improved performance in geo-allocation of the sources.
A final advantage of the point-source map is its performance in areas mildly affected by fires (e.g., in southeastern Asia, Mexico and parts of South America). Certain hotspots due to fires, with a plume center of around 25 km, can look just like actual point sources. In the point-source map, these often appear less bright and are blurred out over a wider area, with lower columns compared to the oversampled average. On the other hand, as before, actual point sources appear brighter and can emerge from the patchy NH3 distribution that is characteristic for areas affected by fires. For that reason, comparing the oversampled and the point-source map was found to be very useful for singling out point sources, especially in those areas with larger background values. Example point sources are the ammonia plant in Campana (Argentina) and Bastos (Brazil), an important center of egg production. These were previously difficult to detect but are now easily identified.
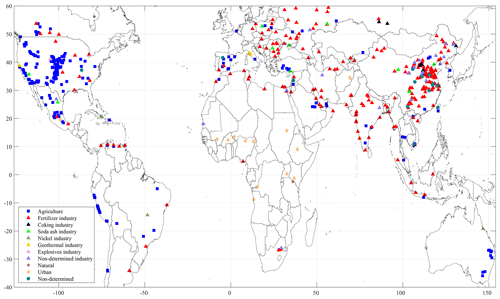
Figure 5Global distribution of NH3 point sources and their categorization. The total numbers per category are as follows: agriculture (215), coking industry (9), explosives industry (1), fertilizer industry (217), geothermal industry (3), non-determined industry (21), nickel industry (4), soda ash industry (11), natural (1), non-determined (15) and urban (13).
Using the methodology presented in the previous section, NH3 point-source maps of the world (land only) were constructed at a resolution of (corresponding to a horizontal resolution of the order of 1–2 km). A few such maps were constructed by varying the size of the averaging box and the applied wind speeds (either in the middle of the boundary layer or at 100 m). While oversampling and back projection are computationally not that demanding, we recall that the construction is based on the treating each grid cell of the map as a potential point source and therefore relies on the construction of wind-adjusted supersampled maps like Fig. 3e for each grid cell. Therefore, producing a world map at that resolution entails the generation of over 100 000 000 maps similar to Fig. 3e, each at a resolution of 1 km and each using more than 250 000 IASI measurements. A single point-source map therefore takes more than a month of computation. We decided to use all the available 2007–2017 NH3 data both from IASI/Metop A (2007–2017) and IASI/Metop B (2013–2017), which helps in reducing the noise even though it creates averages which are biased towards the last 5 years. The maps were then analyzed to provide an update of the point-source catalog presented in Van Damme et al. (2018). We refer to it for a detailed description of the methodology for the identification and categorization of the point sources, as we used the same method here. In brief, first, the global map is analyzed region per region in search of NH3 hotspots that are no larger than 50 km across and that exhibit localized and concentrated enhancements compatible with a point source or dense cluster of point sources. Areas dominated by fires are excluded from the analysis. Analysis of areas with many sources or large ambient background concentrations, such as the Indo-Gangetic Plain, is severely hampered and reveals only the very large point sources. Isolated point sources in remote areas, on the other hand, can easily be picked up. The presence of a point source in the catalog should therefore not be seen as a quantitative indicator of its emission strength. Note that in this study we did not attempt to quantify the emission strengths of each. The categorization of the suspected point sources is performed using Google Earth imagery and third-party information (mainly inventories of fertilizer plants and online resources). The original categories were as follows: “agriculture”, “fertilizer industry”, “other industry”, “natural” and “non-determined”. Here we expanded the number of categories considerably and in particular introduced an urban category and subdivided “other industry” into five new categories, as detailed below.
The new point-source catalog is listed in Table A1 and illustrated on a world map in Fig. 5. Agricultural point sources were found to be invariably associated with CAFOs. Their number more than doubled, from 83 to 216, largely due to the increased attribution in areas of densely located point sources. For many of the previously tagged “source regions”, it was possible to resolve large individual emitters. This was the case in the US (particularly in the geographical region that corresponds to the High Plains Aquifer), Mexico and along the coast of Peru. Also notable are several newly exposed large feedlots in Canada and in eastern Australia. For the first time, agricultural point sources were also identified in China and Russia.
Industrial point sources, as before, are mainly associated with ammonia or urea-based fertilizer production (216, coming from 132) in Europe, northern Africa and Asia. Industrial hotspots were categorized as fertilizer industry as soon as evidence was found of fertilizer production, even when there are clearly other industries present that may contribute. Separate categories were introduced for the previously identified soda ash, geothermal, nickel-mining and coking industries, as additional examples were found for each. One ammonia plant in the US, associated with the manufacturing of explosives, was also assigned a separate category. Emissions over unidentified industries were labeled as a non-determined industry.
An important new category is the urban one. Previously, localized emissions near Mexico City, Bamako (Mali) and Niamey (Niger) were noted. While these hotspots represent diffuse sources, they have been included in the catalog, as the extent of the emissions in the relevant cities is sufficiently local and sufficiently in excess of background values. Thanks to the improved source representation, clear enhancements were found in Kabul (Afghanistan) and 12 African urban agglomerations: Ouagadougou (Burkina Faso), Bamako (Mali), Kano (Nigeria), Niamey (Niger), Maiduguri (Nigeria), Khartoum–Omdurman (Republic of the Sudan), Luanda (Angola), Kinshasa (Congo), Nairobi (Kenya), Addis Ababa (Ethiopia), Bamako (Mali) and Kampala (Uganda). Especially in Asia, atmospheric NH3 is found in excess over most megacities and with much larger columns than found in these African megacities. However, because of the much larger background columns, and much denser clusters of cities, these could not be singled out as was the case in Africa. Apart from industry, known urban sources of NH3 include emissions from vehicles, human waste (waste treatment and sewers), biological waste (garbage containers) and domestic fires (including waste incineration) (Adon et al., 2016; Sun et al., 2017; Reche et al., 2015). At least the hotspot at Bamako is consistent with in situ measurements (Adon et al., 2016), which report very high concentrations year-round, between 28 and 73 ppb on a monthly averaged basis. Local conditions surely are key in explaining why some cities in Africa exhibit much larger concentrations than others. Johannesburg (South Africa) for instance blends in completely in the background, with ambient values barely larger than in the rest of South Africa. While this is outside the scope of the paper, there is no doubt that the IASI NH3 data could be further exploited to better understand the driving factors of urban emission on a global scale.
Other than at Lake Natron (Clarisse et al., 2019), no other natural NH3 hotspots have been identified. For a number of presumed point sources, no likely source could be attributed; however given their location (central US, Middle East and eastern Asia), these are most likely anthropogenic.
Oversampling is a technique now commonplace in the field of atmospheric sounding for achieving hyperresolved spatial averages beyond what the satellites natively offer. There is a class of algorithms referred to as superresolution that goes beyond oversampling, but these have until now only been applied to measurements of satellite imagers for surface parameters. Here, we have shown that it is a viable method that can also be applied to the single-pixel images taken by atmospheric sounders for short-lived gases. We demonstrated this with measurements of a quartz emissivity feature over the Sahara, for which a spatial resolution down to 2–3 km could be achieved.
Superresolution is a priori less suitable for measurements of atmospheric gases because of variations in their distribution related to variations in transport. However, by aligning the winds around point-source emitters, much of this variability can be removed. In Sect. 3, we have shown the advantage of applying IBP superresolution on such wind-corrected data. The resulting averaged plumes originating from point sources not only reveal more detail, but maximum concentrations and gradients are also larger and presumably more realistic. Studies of atmospheric lifetime (e.g., Fioletov et al., 2015), which rely on the precise shape of the dispersion, could potentially benefit from this increase in accuracy.
Wind-adjusted superresolution images around point sources form the basis of the NH3 point-source map, which is an NH3 average that simultaneously corrects for wind transport, accentuates point sources and smooths area sources. It was inspired by the SO2 “difference” map presented in McLinden et al. (2016), but as we do not look at differences, the NH3 map still looks like an NH3 total column distribution. However, other than for the identification of point sources, such a map is not easily exploitable, as it is a distorted representation of the reality that favors point sources. In-depth analysis allowed us to perform a major update of the global catalog of point sources presented in Van Damme et al. (2018), with more than 500 point sources identified and categorized. As a whole, this study further highlights the importance of point sources on local scales. The world map shows distinct patterns, with agricultural point sources completely dominant in America, in contrast to Europe and Asia, where industrial point sources are prevalent. In Africa, NH3 hotspots are mainly found near urban agglomerations.
While the point-source catalog was established with a great deal of care, given its size, mistakes will inevitably be present both in the localization of the point sources (due to, for example, noise in the data or NH3 in transport) and in the categorization. Improvements can probably best be achieved with feedback from the international community, with complementary knowledge on regional sources. For this reason, and to keep track of emerging emission sources, we have set up a website with an interactive global map, visualizing the distribution, type and time evolution of the different point sources (http://www.ulb.ac.be/cpm/NH3-IASI.html, last access: 11 October 2019). With the help of the community, we hope it can become a useful resource for information on global NH3 point sources.
The IASI NH3 product is available from the Aeris data infrastructure (http://iasi.aeris-data.fr, last access: 11 October 2019). It is also planned to be operationally distributed by EUMETCast under the auspices of the Eumetsat Atmospheric Monitoring Satellite Application Facility (AC-SAF; http://ac-saf.eumetsat.int, last access: 11 October 2019).
Table A1Updated point-source catalog. The categories are abbreviated as A for agriculture, CI for the coking industry, EI for the explosives industry, FI for the fertilizer industry, GI for the geothermal industry, NDI for a non-determined industry, NI for the nickel industry, SI for the soda ash industry, N for natural, ND for non-determined and U for urban.
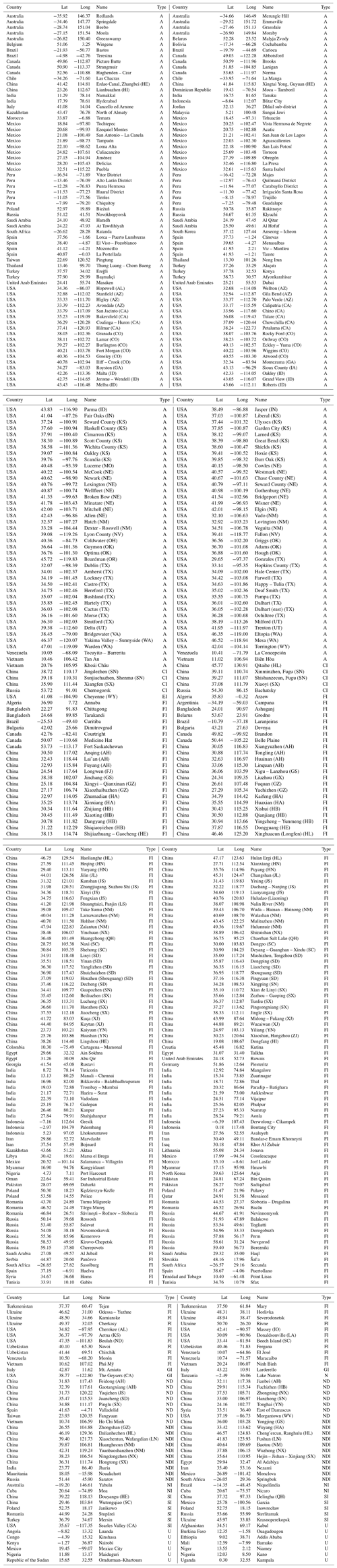
LC conceptualized the study, wrote the code, prepared the figures and drafted the paper. LC and MVD updated the point-source catalog. All authors contributed to the text and interpretation of the results.
The authors declare that they have no conflict of interest.
IASI is a joint mission of EUMETSAT and the Centre National d'Études Spatiales (CNES, France). It is flown aboard the Metop satellites as part of the EUMETSAT Polar System. The IASI L1c and L2 data are received through the EUMETCast near-real-time data distribution service. Lieven Clarisse is a research associate supported by the Belgian F.R.S-FNRS. The IASI NH3 product is available from the Aeris data infrastructure (http://iasi.aeris-data.fr, last access: 11 October 2019).
This research has been supported by the Belgian F.R.S-FNRS and the Belgian State Federal Office for Scientific, Technical and Cultural Affairs (Prodex arrangement IASI.FLOW).
This paper was edited by Diego Loyola and reviewed by two anonymous referees.
Aas, W., Mortier, A., Bowersox, V., Cherian, R., Faluvegi, G., Fagerli, H., Hand, J., Klimont, Z., Galy-Lacaux, C., Lehmann, C. M. B., Myhre, C. L., Myhre, G., Olivié, D., Sato, K., Quaas, J., Rao, P. S. P., Schulz, M., Shindell, D., Skeie, R. B., Stein, A., Takemura, T., Tsyro, S., Vet, R., and Xu, X.: Global and regional trends of atmospheric sulfur, Scientific Reports, 9, 953, https://doi.org/10.1038/s41598-018-37304-0, 2019. a
Adon, M., Yoboué, V., Galy-Lacaux, C., Liousse, C., Diop, B., Doumbia, E. H. T., Gardrat, E., Ndiaye, S. A., and Jarnot, C.: Measurements of NO2, SO2, NH3, HNO3 and O3 in West African urban environments, Atmos. Environ., 135, 31–40, https://doi.org/10.1016/j.atmosenv.2016.03.050, 2016. a, b
Bauer, S. E., Tsigaridis, K., and Miller, R.: Significant atmospheric aerosol pollution caused by world food cultivation, Geophys. Res. Lett., 43, 5394–5400, https://doi.org/10.1002/2016gl068354, 2016. a
Beirle, S., Boersma, K. F., Platt, U., Lawrence, M. G., and Wagner, T.: Megacity Emissions and Lifetimes of Nitrogen Oxides Probed from Space, Science, 333, 1737–1739, https://doi.org/10.1126/science.1207824, 2011. a
Boucher, A., Kyriakidis, P. C., and Cronkite-Ratcliff, C.: Geostatistical Solutions for Super-Resolution Land Cover Mapping, IEEE T. Geosci. Remote, 46, 272–283, https://doi.org/10.1109/tgrs.2007.907102, 2008. a
Canfield, D. E., Glazer, A. N., and Falkowski, P. G.: The Evolution and Future of Earth's Nitrogen Cycle, Science, 330, 192–196, https://doi.org/10.1126/science.1186120, 2010. a
Chang, Y., Zou, Z., Deng, C., Huang, K., Collett, J. L., Lin, J., and Zhuang, G.: The importance of vehicle emissions as a source of atmospheric ammonia in the megacity of Shanghai, Atmos. Chem. Phys., 16, 3577–3594, https://doi.org/10.5194/acp-16-3577-2016, 2016. a
Clarisse, L., Van Damme, M., Gardner, W., Coheur, P.-F., Clerbaux, C., Whitburn, S., Hadji-Lazaro, J., and Hurtmans, D.: Atmospheric ammonia (NH3) emanations from Lake Natron’s saline mudflats, Scientific Reports, 9, 4441, https://doi.org/10.1038/s41598-019-39935-3, 2019. a
Clerbaux, C., Boynard, A., Clarisse, L., George, M., Hadji-Lazaro, J., Herbin, H., Hurtmans, D., Pommier, M., Razavi, A., Turquety, S., Wespes, C., and Coheur, P.-F.: Monitoring of atmospheric composition using the thermal infrared IASI/MetOp sounder, Atmos. Chem. Phys., 9, 6041–6054, https://doi.org/10.5194/acp-9-6041-2009, 2009. a
Dai, S., Han, M., Wu, Y., and Gong, Y.: Bilateral Back-Projection for Single Image Super Resolution, in: 2007 IEEE International Conference on Multimedia and Expo, Beijing, China, 2–5 July 2007, IEEE, https://doi.org/10.1109/icme.2007.4284831, 2007. a
de Foy, B., Krotkov, N. A., Bei, N., Herndon, S. C., Huey, L. G., Martínez, A.-P., Ruiz-Suárez, L. G., Wood, E. C., Zavala, M., and Molina, L. T.: Hit from both sides: tracking industrial and volcanic plumes in Mexico City with surface measurements and OMI SO2 retrievals during the MILAGRO field campaign, Atmos. Chem. Phys., 9, 9599–9617, https://doi.org/10.5194/acp-9-9599-2009, 2009. a, b
de Foy, B., Lu, Z., Streets, D. G., Lamsal, L. N., and Duncan, B. N.: Estimates of power plant NOx emissions and lifetimes from OMI NO2 satellite retrievals, Atmos. Environ., 116, 1–11, https://doi.org/10.1016/j.atmosenv.2015.05.056, 2015. a
Elad, M. and Feuer, A.: Restoration of a single superresolution image from several blurred, noisy, and undersampled measured images, IEEE T. Image Process., 6, 1646–1658, https://doi.org/10.1109/83.650118, 1997. a
ERA5: Copernicus Climate Change Service (C3S): ERA5: Fifth generation of ECMWF atmospheric reanalyses of the global climate, Copernicus Climate Change Service Climate Data Store (CDS), available at: https://cds.climate.copernicus.eu/cdsapp#!/home (last access: 11 October 2019), 2019. a
Erisman, J. W., Sutton, M. A., Galloway, J., Klimont, Z., and Winiwarter, W.: How a century of ammonia synthesis changed the world, Nat. Geosci., 1, 636–639, https://doi.org/10.1038/ngeo325, 2008. a
Fioletov, V., McLinden, C. A., Kharol, S. K., Krotkov, N. A., Li, C., Joiner, J., Moran, M. D., Vet, R., Visschedijk, A. J. H., and Denier van der Gon, H. A. C.: Multi-source SO2 emission retrievals and consistency of satellite and surface measurements with reported emissions, Atmos. Chem. Phys., 17, 12597–12616, https://doi.org/10.5194/acp-17-12597-2017, 2017. a
Fioletov, V. E., McLinden, C. A., Krotkov, N., Moran, M. D., and Yang, K.: Estimation of SO2 emissions using OMI retrievals, Geophys. Res. Lett., 38, L21811, https://doi.org/10.1029/2011GL049402, 2011. a
Fioletov, V. E., McLinden, C. A., Krotkov, N., Yang, K., Loyola, D. G., Valks, P., Theys, N., Van Roozendael, M., Nowlan, C. R., Chance, K., Liu, X., Lee, C., and Martin, R. V.: Application of OMI, SCIAMACHY, and GOME-2 satellite SO2 retrievals for detection of large emission sources, J. Geophys. Res.-Atmos., 118, 11399–11418, https://doi.org/10.1002/jgrd.50826, 2013. a
Fioletov, V. E., McLinden, C. A., Krotkov, N., and Li, C.: Lifetimes and emissions of SO2 from point sources estimated from OMI, Geophys. Res. Lett., 42, 1969–1976, https://doi.org/10.1002/2015gl063148, 2015. a, b, c
Fioletov, V. E., McLinden, C. A., Krotkov, N., Li, C., Joiner, J., Theys, N., Carn, S., and Moran, M. D.: A global catalogue of large SO2 sources and emissions derived from the Ozone Monitoring Instrument, Atmos. Chem. Phys., 16, 11497–11519, https://doi.org/10.5194/acp-16-11497-2016, 2016. a
Fowler, D., Coyle, M., Skiba, U., Sutton, M. A., Cape, J. N., Reis, S., Sheppard, L. J., Jenkins, A., Grizzetti, B., Galloway, J. N., Vitousek, P., Leach, A., Bouwman, A. F., Butterbach-Bahl, K., Dentener, F., Stevenson, D., Amann, M., and Voss, M.: The global nitrogen cycle in the twenty-first century, Philos. T. Roy. Soc. B, 368, 20130164, https://doi.org/10.1098/rstb.2013.0164, 2013. a
Georgoulias, A. K., van der A, R. J., Stammes, P., Boersma, K. F., and Eskes, H. J.: Trends and trend reversal detection in 2 decades of tropospheric NO2 satellite observations, Atmos. Chem. Phys., 19, 6269–6294, https://doi.org/10.5194/acp-19-6269-2019, 2019. a
Harrington, L. M. and Lu, M.: Beef feedlots in southwestern Kansas: local change, perceptions, and the global change context, Global Environ. Chang., 12, 273–282, https://doi.org/10.1016/s0959-3780(02)00041-9, 2002. a
Irani, M. and Peleg, S.: Motion Analysis for Image Enhancement: Resolution, Occlusion, and Transparency, J. Vis. Commun. Image R., 4, 324–335, https://doi.org/10.1006/jvci.1993.1030, 1993. a
Lachatre, M., Fortems-Cheiney, A., Foret, G., Siour, G., Dufour, G., Clarisse, L., Clerbaux, C., Coheur, P.-F., Van Damme, M., and Beekmann, M.: The unintended consequence of SO2 and NO2 regulations over China: increase of ammonia levels and impact on PM2.5 concentrations, Atmos. Chem. Phys., 19, 6701–6716, https://doi.org/10.5194/acp-19-6701-2019, 2018. a
Lelieveld, J., Evans, J. S., Fnais, M., Giannadaki, D., and Pozzer, A.: The contribution of outdoor air pollution sources to premature mortality on a global scale, Nature, 525, 367–371, https://doi.org/10.1038/nature15371, 2015. a
Liu, F., Beirle, S., Zhang, Q., Dörner, S., He, K., and Wagner, T.: NOx lifetimes and emissions of cities and power plants in polluted background estimated by satellite observations, Atmos. Chem. Phys., 16, 5283–5298, https://doi.org/10.5194/acp-16-5283-2016, 2016. a
Liu, M., Huang, X., Song, Y., Xu, T., Wang, S., Wu, Z., Hu, M., Zhang, L., Zhang, Q., Pan, Y., Liu, X., and Zhu, T.: Rapid SO2 emission reductions significantly increase tropospheric ammonia concentrations over the North China Plain, Atmos. Chem. Phys., 18, 17933–17943, https://doi.org/10.5194/acp-18-17933-2018, 2018. a
Lu, Z., Streets, D. G., de Foy, B., Lamsal, L. N., Duncan, B. N., and Xing, J.: Emissions of nitrogen oxides from US urban areas: estimation from Ozone Monitoring Instrument retrievals for 2005–2014, Atmos. Chem. Phys., 15, 10367–10383, https://doi.org/10.5194/acp-15-10367-2015, 2015. a
McLinden, C. A., Fioletov, V., Shephard, M. W., Krotkov, N., Li, C., Martin, R. V., Moran, M. D., and Joiner, J.: Space-based detection of missing sulfur dioxide sources of global air pollution, Nat. Geosci., 9, 496–500, https://doi.org/10.1038/ngeo2724, 2016. a, b, c, d, e
Milanfar, P.: Super-Resolution Imaging, CRC Press, Boca Raton, USA, https://doi.org/10.1201/9781439819319, 2010. a, b
Pommier, M., McLinden, C. A., and Deeter, M.: Relative changes in CO emissions over megacities based on observations from space, Geophys. Res. Lett., 40, 3766–3771, https://doi.org/10.1002/grl.50704, 2013. a
Reche, C., Viana, M., Karanasiou, A., Cusack, M., Alastuey, A., Artiñano, B., Revuelta, M. A., López-Mahía, P., Blanco-Heras, G., Rodríguez, S., de la Campa, A. M. S., Fernández-Camacho, R., González-Castanedo, Y., Mantilla, E., Tang, Y. S., and Querol, X.: Urban NH3 levels and sources in six major Spanish cities, Chemosphere, 119, 769–777, https://doi.org/10.1016/j.chemosphere.2014.07.097, 2015. a
Russell, A. R., Valin, L. C., Bucsela, E. J., Wenig, M. O., and Cohen, R. C.: Space-based Constraints on Spatial and Temporal Patterns of NOx Emissions in California, 2005–2008, Environ. Sci. Technol., 44, 3608–3615, https://doi.org/10.1021/es903451j, 2010. a
Steffen, W., Richardson, K., Rockstrom, J., Cornell, S. E., Fetzer, I., Bennett, E. M., Biggs, R., Carpenter, S. R., de Vries, W., de Wit, C. A., Folke, C., Gerten, D., Heinke, J., Mace, G. M., Persson, L. M., Ramanathan, V., Reyers, B., and Sorlin, S.: Planetary boundaries: Guiding human development on a changing planet, Science, 347, 1259855, https://doi.org/10.1126/science.1259855, 2015. a
Streets, D. G., Canty, T., Carmichael, G. R., de Foy, B., Dickerson, R. R., Duncan, B. N., Edwards, D. P., Haynes, J. A., Henze, D. K., Houyoux, M. R., Jacob, D. J., Krotkov, N. A., Lamsal, L. N., Liu, Y., Lu, Z., Martin, R. V., Pfister, G. G., Pinder, R. W., Salawitch, R. J., and Wecht, K. J.: Emissions estimation from satellite retrievals: A review of current capability, Atmos. Environ., 77, 1011–1042, https://doi.org/10.1016/j.atmosenv.2013.05.051, 2013. a
Sun, K., Tao, L., Miller, D. J., Pan, D., Golston, L. M., Zondlo, M. A., Griffin, R. J., Wallace, H. W., Leong, Y. J., Yang, M. M., Zhang, Y., Mauzerall, D. L., and Zhu, T.: Vehicle Emissions as an Important Urban Ammonia Source in the United States and China, Environ. Sci. Technol., 51, 2472–2481, https://doi.org/10.1021/acs.est.6b02805, 2017. a
Sun, K., Zhu, L., Cady-Pereira, K., Chan Miller, C., Chance, K., Clarisse, L., Coheur, P.-F., González Abad, G., Huang, G., Liu, X., Van Damme, M., Yang, K., and Zondlo, M.: A physics-based approach to oversample multi-satellite, multispecies observations to a common grid, Atmos. Meas. Tech., 11, 6679–6701, https://doi.org/10.5194/amt-11-6679-2018, 2018. a, b, c, d
Sutton, M. A., Reis, S., Riddick, S. N., Dragosits, U., Nemitz, E., Theobald, M. R., Tang, Y. S., Braban, C. F., Vieno, M., Dore, A. J., Mitchell, R. F., Wanless, S., Daunt, F., Fowler, D., Blackall, T. D., Milford, C., Flechard, C. R., Loubet, B., Massad, R., Cellier, P., Personne, E., Coheur, P. F., Clarisse, L., Van Damme, M., Ngadi, Y., Clerbaux, C., Skjøth, C. A., Geels, C., Hertel, O., Wichink Kruit, R. J., Pinder, R. W., Bash, J. O., Walker, J. T., Simpson, D., Horváth, L., Misselbrook, T. H., Bleeker, A., Dentener, F., and de Vries, W.: Towards a climate-dependent paradigm of ammonia emission and deposition, Philos. T. Roy. Soc. B, 368, 20130166, https://doi.org/10.1098/rstb.2013.0166, 2013. a
Takashima, T. and Masuda, K.: Emissivities of quartz and Sahara dust powders in the infrared region (7–17 µm), Remote Sens. Environ., 23, 51–63, https://doi.org/10.1016/0034-4257(87)90070-8, 1987. a
Valin, L. C., Russell, A. R., and Cohen, R. C.: Variations of OH radical in an urban plume inferred from NO2 column measurements, Geophys. Res. Lett., 40, 1856–1860, https://doi.org/10.1002/grl.50267, 2013. a, b
Van Damme, M., Clarisse, L., Heald, C. L., Hurtmans, D., Ngadi, Y., Clerbaux, C., Dolman, A. J., Erisman, J. W., and Coheur, P. F.: Global distributions, time series and error characterization of atmospheric ammonia (NH3) from IASI satellite observations, Atmos. Chem. Phys., 14, 2905–2922, https://doi.org/10.5194/acp-14-2905-2014, 2014. a
Van Damme, M., Whitburn, S., Clarisse, L., Clerbaux, C., Hurtmans, D., and Coheur, P.-F.: Version 2 of the IASI NH3 neural network retrieval algorithm: near-real-time and reanalysed datasets, Atmos. Meas. Tech., 10, 4905–4914, https://doi.org/10.5194/amt-10-4905-2017, 2017. a
Van Damme, M., Clarisse, L., Whitburn, S., Hadji-Lazaro, J., Hurtmans, D., Clerbaux, C., and Coheur, P.-F.: Industrial and Agricultural Ammonia Point Sources Exposed, Nature, 564, 99–103, https://doi.org/10.1038/s41586-018-0747-1, 2018. a, b, c, d, e, f, g, h, i, j
Wang, S., Zhang, Q., Martin, R. V., Philip, S., Liu, F., Li, M., Jiang, X., and He, K.: Satellite measurements oversee China’s sulfur dioxide emission reductions from coal-fired power plants, Environ. Res. Lett., 10, 114015, https://doi.org/10.1088/1748-9326/10/11/114015, 2015. a
Warner, J. X., Dickerson, R. R., Wei, Z., Strow, L. L., Wang, Y., and Liang, Q.: Increased atmospheric ammonia over the world's major agricultural areas detected from space, Geophys. Res. Lett., 44, 2875–2884, https://doi.org/10.1002/2016gl072305, 2017. a
Wenig, M. O., Cede, A. M., Bucsela, E. J., Celarier, E. A., Boersma, K. F., Veefkind, J. P., Brinksma, E. J., Gleason, J. F., and Herman, J. R.: Validation of OMI tropospheric NO2 column densities using direct-Sun mode Brewer measurements at NASA Goddard Space Flight Center, J. Geophys. Res., 113, D16S45, https://doi.org/10.1029/2007jd008988, 2008. a
Whitburn, S., Van Damme, M., Clarisse, L., Bauduin, S., Heald, C. L., Hadji-Lazaro, J., Hurtmans, D., Zondlo, M. A., Clerbaux, C., and Coheur, P.-F.: A flexible and robust neural network IASI-NH3 retrieval algorithm, J. Geophys. Res., 121, 6581–6599, https://doi.org/10.1002/2016jd024828, 2016. a
Wikipedia: 2013 Chemical accident in Horlivka, available at: https://en.wikipedia.org/wiki/2013_Chemical_accident_in_Horlivka, last access: 27 February 2019. a
Xu, J., Liang, Y., Liu, J., and Huang, Z.: Multi-Frame Super-Resolution of Gaofen-4 Remote Sensing Images, Sensors, 17, 2142, https://doi.org/10.3390/s17092142, 2017. a
Yuan, B., Coggon, M. M., Koss, A. R., Warneke, C., Eilerman, S., Peischl, J., Aikin, K. C., Ryerson, T. B., and de Gouw, J. A.: Emissions of volatile organic compounds (VOCs) from concentrated animal feeding operations (CAFOs): chemical compositions and separation of sources, Atmos. Chem. Phys., 17, 4945–4956, https://doi.org/10.5194/acp-17-4945-2017, 2017. a
Zhu, L., Jacob, D. J., Mickley, L. J., Marais, E. A., Cohan, D. S., Yoshida, Y., Duncan, B. N., Abad, G. G., and Chance, K. V.: Anthropogenic emissions of highly reactive volatile organic compounds in eastern Texas inferred from oversampling of satellite (OMI) measurements of HCHO columns, Environ. Res. Lett., 9, 114004, https://doi.org/10.1088/1748-9326/9/11/114004, 2014. a
Zhu, L., Henze, D. K., Bash, J. O., Cady-Pereira, K. E., Shephard, M. W., Luo, M., and Capps, S. L.: Sources and Impacts of Atmospheric NH3: Current Understanding and Frontiers for Modeling, Measurements, and Remote Sensing in North America, Current Pollution Reports, 1, 95–116, https://doi.org/10.1007/s40726-015-0010-4, 2015. a