the Creative Commons Attribution 4.0 License.
the Creative Commons Attribution 4.0 License.
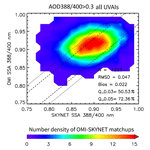
A comparative evaluation of Aura-OMI and SKYNET near-UV single-scattering albedo products
Omar Torres
The aerosol single-scattering albedo (SSA) retrieved by the near-UV algorithm applied to the Aura Ozone Monitoring Instrument (OMI) measurements (OMAERUV) is compared with an independent inversion product derived from the sky radiometer network SKYNET – a ground-based radiation observation network with sites in Asia and Europe. The present work continues previous efforts to evaluate the consistency between the retrieved SSA from satellite and ground sensors. The automated spectral measurements of direct downwelling solar flux and sky radiances made by the SKYNET Sun-sky radiometer are used as input to an inversion algorithm that derives spectral aerosol optical depth (AOD) and single-scattering albedo (SSA) in the near-UV to near-IR spectral range. The availability of SKYNET SSA measurements in the ultraviolet region of the spectrum allows, for the first time, a direct comparison with OMI SSA retrievals eliminating the need of extrapolating the satellite retrievals to the visible wavelengths as is the case in the evaluation against the Aerosol Robotic Network (AERONET). An analysis of the collocated retrievals from over 25 SKYNET sites reveals that about 61 % (84 %) of OMI–SKYNET matchups agree within the absolute difference of ±0.03 (±0.05) for carbonaceous aerosols, 50 % (72 %) for dust aerosols, and 45 % (75 %) for urban–industrial aerosol types. Regionally, the agreement between the two inversion products is robust over several sites in Japan influenced by carbonaceous and urban–industrial aerosols; at the biomass burning site Phimai in Thailand; and the polluted urban site in New Delhi, India. The collocated dataset yields fewer matchups identified as dust aerosols mostly over the site Dunhuang with more than half of the matchup points confined to within ±0.03 limits. Altogether, the OMI–SKYNET retrievals agree within ±0.03 when SKYNET AOD (388 or 400 nm) is larger than 0.5 and the OMI UV Aerosol Index is larger than 0.2. The remaining uncertainties in both inversion products can be attributed to specific assumptions made in the retrieval algorithms, i.e., the uncertain calibration constant, assumption of spectral surface albedo and particle shape, and subpixel cloud contamination. The assumption of fixed and spectrally neutral surface albedo (0.1) in the SKYNET inversion appears to be unrealistic, leading to underestimated SSA, especially under lower aerosol load conditions. At higher AOD values for carbonaceous and dust aerosols, however, retrieved SSA values by the two independent inversion methods are generally consistent in spite of the differences in retrieval approaches.
Satellite-based remote sensing of aerosols has become an essential tool to detect, quantify, and routinely monitor the aerosol optical and size properties over the globe. An accurate representation of aerosols in the climate models is an essential requirement for reducing the uncertainty in the aerosol-related impact on the Earth's radiation balance (direct and semidirect effects) and cloud microphysics (indirect effect) (IPCC, 2013). The fundamental aerosol parameters determining the strength and sign of the radiative forcing are the aerosol optical depth (AOD) and single-scattering albedo (SSA) in addition to the reflective properties of the underlying surface. While the columnar AOD represents the total extinction (scattering and absorption) resulting from the interactions with solar radiation, SSA describes the relative strength of scattering to the total extinction. Together, both AOD and SSA determine the magnitude and sign of the aerosol radiative forcing at the top of the atmosphere. For example, a decrease in SSA from 0.9 to 0.8 can often change the sign of radiative forcing from negative (cooling) to positive (warming) that also depends on the albedo of the underlying surface and the altitude of the aerosols (Hansen et al., 1997). Thus, an accurate estimate of both quantities is a prime requirement for reliable estimates of the net effect of atmospheric aerosols produced with the anthropogenic as well as natural activities.
Launched in July 2004, the Ozone Monitoring Instrument (OMI) on board NASA's Aura satellite has produced a more than a decade long global record of observations of reflected radiation from Earth in the 270–500 nm wavelength range of the spectrum on a daily basis. OMI scans the entire Earth in 14 to 15 orbits with its cross-track swath of ∼2600 km at ground level at a nadir ground pixel spatial resolution of 13 km×24 km. OMI observations of the top-of-atmosphere (TOA) reflected light at 354 and 388 nm wavelengths are used to derive the UV Aerosol Index (UVAI) as well as the AOD and SSA using the OMAERUV algorithm that takes advantage of the well-known sensitivity to the aerosol absorption in the UV spectral region (Torres et al., 1998). While a general description of the OMAERUV algorithm is presented in Torres et al. (2007), the recent algorithmic upgrades are documented in Torres et al. (2013, 2018). The most important changes applied in the latest OMAERUV algorithm upgrade include (1) the use of new carbonaceous aerosol models that account for the presence of organics in the carbonaceous aerosols by assuming the wavelength-dependent imaginary part of the refractive index (Jethva and Torres, 2011), (2) an implementation of the robust scheme to identify aerosol type (smoke, dust, urban/industrial) that combined uses the information on carbon monoxide (CO) observations from the Atmospheric Infrared Sounder (AIRS) and UVAI from OMI (Torres et al., 2013), (3) the use of the aerosol height climatology dataset derived from the Cloud-Aerosol Lidar with Orthogonal Polarization (CALIOP) lidar-based measurements of the vertical profiles of aerosol for the carbonaceous and dust aerosols (Torres et al., 2013), and (4) better treatment of dust particles assuming a realistic spheroidal shape distribution (Torres et al., 2018). Additionally, the upgraded OMAERUV algorithm has adopted a new method to calculate UVAI, which now accounts for the angular scattering effects of clouds and significantly reduces a scan-angle-related asymmetry in UVAI in cloudy scenes (Torres et al., 2018).
The present work continues previous efforts to evaluate the consistency between ground-based SSA measurements and satellite retrievals from near-UV observations. On the first attempt to intercompare space-based and surface near-UV SSA measurements, Earth Probe TOMS retrievals were compared to AERONET observations acquired during the SAFARI 2000 field campaign (Torres et al., 2005). The OMAERUV near-UV aerosol product of AOD and SSA has been continually assessed and validated against the ground-based measurements acquired from the globally distributed Aerosol Robotic Network (AERONET; Torres et al., 2007; Ahn et al., 2008, 2014; Jethva and Torres, 2011; Jethva et al., 2014). While the OMAERUV AOD product was directly validated against the AERONET measurements made in the near-UV (340–380 nm), as carried out in Ahn et al. (2014), the SSA retrievals have been evaluated by comparison with the AERONET ground inversion product (Jethva et al., 2014). The latter analysis required OMI retrievals of SSA to be extrapolated to the shortest visible wavelength of 440 nm of the AERONET inversion product to make the comparison possible. Such adjustment in the wavelength of retrievals can introduce uncertainty in the comparison arising from the inaccuracy of the spectral dependence of absorption assumed in the wavelength conversion.
A direct comparison of the column-integrated SSA at 388 nm retrieved from OMI requires equivalent ground-based columnar retrievals in the near-UV region. The international network of scanning Sun-sky radiometers (SKYNET) fulfills this requirement as it performs the direct Sun and sky measurements in the near-UV (340–380 nm) as well as visible/near-IR (400–1020 nm) regions of the spectrum and derives spectral AOD and SSA. Taking advantage of the availability of ground-based SSA inversions in the near-UV from SKYNET, we intercompare the OMI and SKYNET SSA products at several SKYNET sites in Asia and Europe. Since both retrieval approaches are based on inversion algorithms that rely on assumptions, the resulting level of agreement can only be interpreted as a measure of consistency (or lack thereof) in the measurement of the same physical parameter by fundamentally different remote sensing approaches.
The paper is organized as follows: Sect. 2 describes the satellite- and ground-based datasets assessed in this analysis along with the collocation methodology; the results of OMI–SKYNET SSA comparison over individual sites, combined for each aerosol type, and diagnosis of differences between them are presented in Sect. 3; the possible sources of uncertainty in both inversion products are discussed in Sect. 4; the paper is summarized and concluded in Sect. 5.
2.1 The OMI–OMAERUV aerosol product
The entire record of OMI observations (October 2004 to present) has been reprocessed recently with the refined OMAERUV algorithm (PGEVersion V1.8.9.1) to derive a comprehensive aerosol product that includes retrievals of the UV Aerosol Index (UVAI), AOD, SSA, and AAOD (388 nm) at a pixel resolution of 13 km×24 km at nadir viewing geometry. The retrieved parameters are also reported at 354 and 500 nm wavelengths following the spectral dependence of aerosols assumed in the chosen model. The dataset is available in the HDF-EOS5 format and can be obtained at no cost from the NASA Goddard Earth Sciences (GES) Data and Information Services Center (DISC) server at http://daac.gsfc.nasa.gov/ (last access: 19 November 2019). The recent upgrade has been documented in detail in the work of Jethva and Torres (2011), Torres et al. (2013, 2018), and Ahn et al. (2014). Here, we use the OMAERUV Level 2 Collection 003 (V1.8.9.1) aerosol product processed in July 2017. The expected uncertainty limits in the OMAERUV SSA retrievals are determined to be ±0.03 and ±0.05, based on its comparison with AERONET SSA inversion and sensitivity analysis carried out during the development of the OMAERUV algorithm (Torres et al., 2007). Following an early evaluation of the OMI aerosol product for a handful of sites, Jethva et al. (2014) conducted a global evaluation of the SSA product and also carried out a detailed uncertainty test considering different sources of errors, such as aerosol model, surface albedo, and aerosol layer height. The results of the sensitivity analysis further confirmed the uncertainty budget estimated earlier during the early development of the OMAERUV algorithm. However, note that the errors could attain larger magnitudes when algorithmic assumptions are far off from the real atmospheric conditions.
Post-2007, the OMI observations have been affected by a possible external obstruction that perturbs both the measured solar flux and Earth radiance. This obstruction affecting the quality of radiance at all wavelengths for a particular viewing direction is referred to as the “row anomaly” (RA) since the viewing geometry is associated with the row numbers on the charge-coupled device detectors. The RA issue was detected for the first time in mid-2007 with a couple of rows, which during the later period of operation expanded to other rows in 2008 and later. At present, about half of the total 60 rows across the track are identified and flagged as row-anomaly-affected positions for which no physical retrievals are performed (Schenkeveld et al., 2017). The details about this issue can be found at http://www.knmi.nl/omi/research/product/rowanomaly-background.php (last access: 19 November 2019). The RA has significantly affected the sampling during post-2008 OMI measurements, where row anomaly flags blanket about half of the OMI swath. As a result, the availability of the number of retrievals since 2009 over a particular site is reduced. Therefore, the OMI–SKYNET matchups are also expected to be lower during the row-anomaly-affected period. The OMAERUV algorithm assigns quality flags to each pixel which carries information on the quality of the retrieval depending upon the observed condition. We used aerosol retrievals free of RA and flagged as quality flag “0”, which are considered reliable due to higher confidence in detecting aerosols in a scene with minimal cloud contamination.
2.2 The SKYNET aerosol inversion product
The SKYNET is an international network of scanning Sun-sky radiometers (manufactured by Prede Co. Ltd., Japan) performing routine and long-term measurements of direct and diffuse solar radiations at several wavelengths spanning the UV (340 and 380 nm), visible (400, 500, 675 nm), and near-IR (875, 1020 nm) region, as well as in the shortwave IR (1627 and 2200 nm) of the spectrum. The automated measurements of direct and diffuse solar radiations are used to measure spectral AOD and retrieve SSA and other aerosol optical–microphysical properties (volume size distribution, refractive index, phase function, and asymmetry parameter) at the same standard wavelengths of AOD following an inversion algorithm packaged in the SKYRAD.pack software (Nakajima et al., 1996; Hashimoto et al., 2012). Cloudy observations are screened using the Cloud Screening Sky Radiometer code (Khatri and Takamura, 2009).
The SKYNET radiometers come in two flavors, model POM-01 and model POM-02. The POM-01 instrument carries a total of five wavelength filters covering visible to near-IR (400–1020 nm), whereas the POM-02 instrument has two additional filters in the UV region (340 and 380 nm) along with the other filters in the visible to shortwave-IR (including 1627 and 2200 nm) part of the spectrum. The calibration of each SKYNET radiometer is performed on-site on a monthly basis using the improved Langley method (Nakajima et al., 1996; Campanelli et al., 2004a, 2007). Occasionally, the intercalibration of radiometers is carried out against the master instrument well-calibrated using the Langley method on a high mountain site, e.g., Mauna Loa. The SKYNET radiometers are also intercompared with AERONET Cimel Sun photometers and precision filter radiometers at three observation sites, i.e., Chiba University, Valencia (Estelles et al., 2016), and Rome (Campanelli et al., 2018).
Studies in the past have compared AODs (Estellés et al., 2012a) and SSAs (Estellés et al., 2012b) measured/retrieved from SKYNET and AERONET and shown that AODs are well-correlated and in good agreement, but the SKYNET SSAs are found to be higher than those of AERONET (Che et al., 2008; Hashimoto et al., 2012). Khatri et al. (2016) further pinpoints the factors, such as the quality of input data attributed to different calibration and observation protocols, different quality assurance criteria, the calibration constant for sky radiances, differences in measured AOD, and surface albedo, responsible for the inconsistent aerosol SSA between AERONET and SKYNET using observations from the four representative sites, i.e., Chiba (Japan), Pune (India), Valencia (Spain), and Seoul (South Korea). More discussion on the sources of uncertainties is presented in Sect. 4.
Table 1A list of SKYNET sites and the corresponding dataset used in the present analysis. Sensor type POM-02 consists of a total of seven wavelength filters, including near-UV bands, i.e., 340, 380, 400, 500, 675, 870, and 1020 nm, whereas POM-01 sensors have a total of five wavelength filters, i.e., 400, 500, 675, 870, and 1020 nm. The rightmost four columns enlist the statistical measures of OMI–SKYNET single-scattering albedo matchups. Abbreviations: N is the number of satellite–ground matchups, RMSD is the root-mean-square difference between OMI and SKYNET, Q_0.03 and Q_0.05 are the percent matchups within an absolute difference of 0.03 and 0.05.
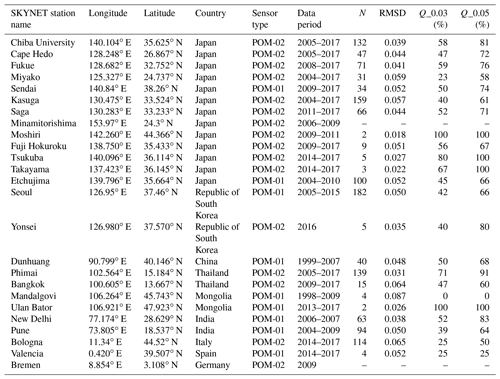
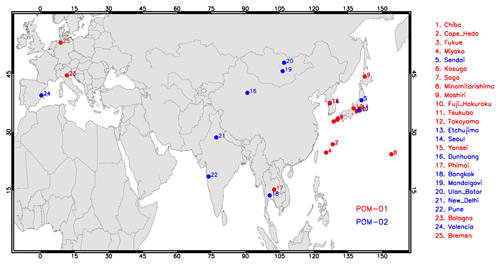
Figure 1Geographical placement of ground-based SKYNET sensors (POM-01 in blue, POM-02 in red) over sites in Asia and Europe. The SKYNET datasets for these sites are freely accessible from the Center for Environmental Remote Sensing (CEReS), Chiba University, Japan (http://atmos3.cr.chiba-u.jp/skynet/data.html, last access: 19 November 2019).
In this study, we include the SKYNET data acquired over a total of 25 sites distributed mostly across Asia and a few in Europe. The dataset is freely accessible from the data portal of the Center for Environmental Remote Sensing (CEReS), Chiba University, Japan (http://atmos3.cr.chiba, last access: 19 November 2019). Figure 1 shows the geographic distribution of selected sites, whereas Table 1 lists the geocoordinates of these sites with the associated sensor type (POM-01 or POM-02) and data periods. The SKYNET aerosol product is derived using two different Skyrad packs: version 4.2 and version 5, the differences of which are explained in Hashimoto et al. (2012). In this study, we use the SKYNET Level 2 product retrieved using version 5 of Skyrad pack. SKYNET retrievals assigned with cloud flag “0” are included in the analysis since these measurements are believed to be free of cloud contamination and considered to be higher-quality retrievals. A careful examination of the SKYNET inversion dataset revealed some irregularities in the measurements for many sites, such as irregular patterns in the shape of spectral SSAs, identical values of SSA at near-UV and visible wavelengths, and much larger standard deviation (> 0.1) in SSA within a few hours. These spurious measurements were excluded from the present analysis.
2.3 The collocation of OMI and SKYNET measurements
OMI retrievals correspond to a spatial scale of 13 km×24 km at nadir representing the atmospheric conditions over an area. Unlike the direct measurements of the spectral AOD, which correspond to columnar point measurements, the retrievals made by SKYNET use the sky radiances measured at several discrete angles azimuthally, therefore representing the sky condition observed over a station which is associated with an approximately 5 km radius surrounding the Sun photometer site. SKYNET retrieves aerosol optical–microphysical properties, including spectral SSA, under all cloud-free conditions and at all aerosol loadings. It is expected that the inversion of retrieved parameters from sky radiances offers better accuracy at larger solar zenith angles owing to the longer optical path and better aerosol absorption signal (Dubovik et al., 2000). These conditions are best satisfied with the measurements made during the early morning and late afternoon hours. On the other hand, Aura-OMI overpasses a station during the afternoon hours with the local Equator-crossing time 13:30. Therefore, the collocation of the measurements was carried out within a time window of ±3 h around OMI overpass time to get sufficient high-quality SKYNET retrievals particularly from early morning/late afternoon measurements. The OMI retrievals of SSA were spatially averaged in a grid area of 0.5∘ by 0.5∘ centered at the SKYNET site. Though the spatial averaging area for the OMI retrieval is about 50 km2, due to its larger footprint, the actual area intercepted by OMI pixels around the SKYNET site is likely to be larger.
OMI performs retrievals at 354 and 388 nm wavelengths, whereas the SKYNET POM-02 instrument reports SSA at nearby wavelengths of 340, 380, and 400 nm. To compare both SSA products at the same wavelength, SKYNET SSA was linearly interpolated at 388 nm, to match with the wavelength of the OMI retrieval, using the measurements at the two nearest wavelengths, i.e., 380 and 400 nm. The SKYNET POM-01 instruments do not carry UV wavelength filters but report the retrievals at the shortest wavelength 400 nm and other visible/near-IR wavelengths. In this case, the OMI retrievals are extrapolated from 388 to 400 nm, to match with the wavelength of SKYNET inversion, following the spectral dependence of SSA associated with the chosen aerosol model in the OMI algorithm. It is reasonably fair to assume that the extrapolation of OMI SSA in a narrow window of 12 nm, i.e., from 388 to 400 nm, should not be a major source of uncertainty in comparing SSA from OMI and SKYNET.
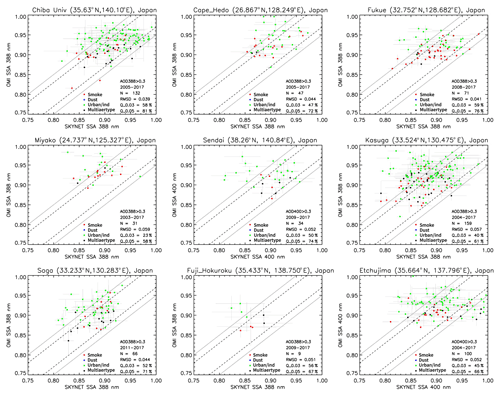
Figure 2OMAERUV versus SKYNET single-scattering albedo comparison for different sites in Japan. Legends with different colors represent the aerosol type selected by the OMAERUV algorithm for the colocated matchups (N). RMSD is the root-mean-square difference between the two retrievals; Q_0.03 and Q_0.05 are the percents of total matchups (N) that fall within the absolute difference of 0.03 and 0.05, respectively. OMI–SKYNET matchups with AOD > 0.3 (388 or 400 nm) in both measurements are used for comparison.
3.1 OMI–SKYNET comparison over individual stations
Figure 2 displays the OMAERUV versus SKYNET SSA scatterplots for selected sites in Japan. The comparison was made at 388 or 400 nm depending upon the availability of the SKYNET inversion at those wavelengths, i.e., POM-01 or POM-02 sensors. Legends with different colors represent the aerosol type selected by the OMAERUV algorithm for the colocated matchups (N). RMSD is the root-mean-square difference between the two retrievals; Q_0.03 and Q_0.05 are the percent of total matchups (N) that fall within the absolute difference of 0.03 and 0.05, respectively; the horizontal and vertical lines for each matchup are the standard deviation of temporally and spatially averaged SKYNET and OMI SSAs. The comparison includes OMI–SKYNET matchups with AOD > 0.3 (388 or 400 nm) in both measurements simultaneously. The scatterplots reveal a good level of agreement for matchups identified as carbonaceous/smoke aerosols over Chiba University, Cape Hedo, Fukue, Saga, and Etchujima with the majority of points confined within the absolute difference of 0.03. The OMI–SKYNET combined dataset is dominated with matchup points identified as the urban/industrial aerosols in the OMAERUV algorithm for which the measured UVAI falls below 0.5, representing lower aerosol loading in the boundary layer with weakly absorbing properties. Under such observed conditions, the uncertainties in both kinds of measurements are prone to be larger due to the lower absorption signal relative to the instrumental noise and errors in algorithmic assumptions, such as surface albedo, that could further amplify the overall uncertainty in the retrievals. Despite these inherent uncertainties, an agreement within the difference of ±0.03 for more than half of the collocated retrievals is encouraging.
Figure 3 shows the scatterplots of OMI–SKYNET SSA for the remaining sites located in South Korea, China, Thailand, India, and Italy. For the Seoul site in South Korea, OMI tends to overestimate SSA for a number of matchups assigned as the urban/industrial aerosols and for a few with the carbonaceous/smoke aerosol type such that about 42 % of total matchups fall within the difference of 0.03. For the Dunhuang site located in the desert area of China, a majority of collocated data points were identified as dust aerosol type, providing an overall better agreement with 50 % and 68 % of matchups bounded within ±0.03 and ±0.05 differences, respectively. The Phimai site in Thailand is known to be influenced by the springtime biomass burning activities, where OMI and SKYNET SSAs are found to agree the most among all 25 sites, providing 71 % and 91 % of the matchups restricted within the ±0.03 and ±0.05 limits, respectively. The agreement between the two sensors was robust for the carbonaceous/smoke aerosol type followed by the urban/industrial aerosols. Over the megacity of New Delhi in the Indo-Gangetic Plain in India, which is seasonally influenced by the smoke and desert dust aerosols in addition to the local source of urban pollution, the OMI–SKYNET matchups are found to agree within ±0.03 and ±0.05 for 52 % and 83 % of the evaluated data points, respectively. Over the Pune station located near the western boundary of India and the Bologna site in Italy, OMI retrieves higher SSA compared to that of SKYNET, yielding 39 % and 64 % as well as 25 % and 50 % matchups, respectively, within the two uncertainty limits. Table 1 lists the statistical measures of the OMI–SKYNET SSA comparison for all 25 sites. A more detailed description of the different sources of uncertainty is presented in Sect. 4.
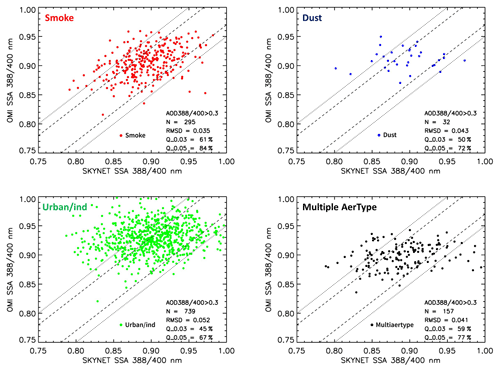
Figure 4Composite scatterplots of OMAERUV versus SKYNET single-scattering albedo (388 or 400 nm) for the three distinct aerosol types, i.e., smoke, dust, and urban/industrial, as identified by the OMAERUV algorithm. OMI–SKYNET matchups with AOD > 0.3 (388 or 400 nm) in both measurements are used for the comparison. The matchup points labeled “multiaertype” represent conditions when collocated OMI pixels are identified with more than one aerosol type.
3.2 Composites for each aerosol type
Figure 4 displays the composite scatterplots of OMI versus SKYNET SSA derived by segregating the matchup points for each aerosol type from all 25 sites. The intention here is to evaluate the consistency between the two retrieval methods for each aerosol type separately and understand their relative differences. When identified as the carbonaceous/smoke aerosol type, the OMI–SKYNET matchups reveal the best comparison relatively among the three major aerosol types, with 61 % and 84 % of data points falling within the absolute difference of 0.03 and 0.05, respectively, and providing the lowest (0.035) root-mean-square difference between the two retrievals. The collocation procedure yields the lowest number of matchups (N=32) for desert dust aerosol type obtained mostly over the site of Dunhuang in China, resulting in 50 % and 72 % of data points within the stated uncertainty limits. Among the three aerosol types, the collocated points assigned with the urban/industrial aerosol type (Fig. 4 bottom left) yield the maximum number of matchups (N=739) with the relatively weakest agreement (RMSD =0.052), where OMI tends to overestimate SSA for a significant number of instances, resulting in about 45 % and 67 % of data points falling within the two limits of expected uncertainties. When more than one prescribed aerosol type is selected for OMI pixels around the SKYNET stations, the matchups between the two sensors resulted in 59 % and 77 % retrievals within the uncertainty limits with an RMSD of 0.041 – a comparison slightly poorer than the smoke-only case but better than the dust-only and urban/industrial-only retrieval cases. Combined, all three distinct aerosol types simultaneously yield the total number of matchups (N=1223) with an RMSD of 0.047 between OMI and SKYNET, resulting in 51 % and 72 % of collocated data points falling within the absolute difference of 0.03 and 0.05 difference respectively. When the restriction of AOD > 0.3 is removed from the collocation procedure, allowing all matchups regardless of their respective AOD values, the total number of collocated data points was increased to more than twice (N=2691), albeit with a relatively weaker agreement yielding an RMSD of 0.06 and percent data points within the uncertainty limits reducing to 38 % and 59 %, respectively.
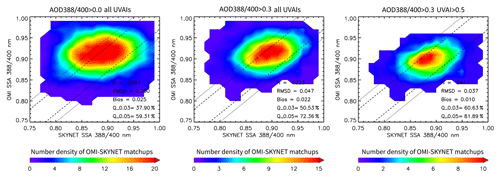
Figure 5Composite number density plots of the SSA comparison between OMI and SKYNET for different aerosol loading conditions. The resultant statistics of the comparison are depicted in the lower right in each plot. Note that the scale used for the number density of satellite–ground matchups for the three sets of comparisons is different.
3.3 Composites for varying aerosol loading and POM-01 versus POM-02
Figure 5 shows the number density plots comparing OMI–SKYNET SSA matchups obtained from all sites combined and for varying aerosol loading conditions. The best set of comparison is achieved under the most restrictive scenario when corresponding OMI-retrieved AOD and UVAI are constrained to > 0.3 and > 0.5, respectively, albeit with a significantly reduced number of matchups compared to the other two cases with fewer (middle) or no (left) restrictions. The improved comparison reflected in statistical parameters is a result of avoiding retrievals with lower aerosol loading when both kinds of measurements might be subjected to larger uncertainties due to algorithmic assumptions.
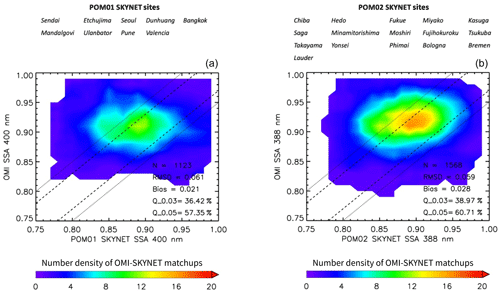
Figure 6Composite number density contour plots of the SSA comparison between OMI and SKYNET for POM-01 (a) and POM-02 (b) sensors separately. The resultant statistics of the comparison are depicted in the lower right of both plots.
Figure 6 shows the number density plot comparing SSA between SKYNET and OMI for POM-01 and POM-02 sensors separately. Overall, no major difference is noticed in the derived statistics between the two sets of comparison, except that the number of matchups obtained with the POM-02 sensor is 39 % more than those with POM-01 sensors, and the POM-02 dataset offers marginally better comparison (except bias, which is higher with POM-02) with OMI SSA retrievals. This analysis indicates that the interpolation of OMI SSA from 388 to 400 nm for its comparison with POM-01 data is not a significant source of discrepancy between the two SSA datasets.
3.4 Diagnosis of OMAERUV versus SKYNET SSA
The SKYNET algorithm inverts the spectral sky radiances in conjunction with the direct AOD measurements to retrieve the real and imaginary parts of the refractive index and particle size distribution of cloud-free observations under all aerosol loading conditions. These inversion products are believed to be more stable and accurate at higher aerosol loadings and solar zenith angles due to stronger aerosol absorption signal and longer optical path (Dubovik et al., 2000). Similarly, a sensitivity analysis of the two-channel OMAERUV retrievals suggests that the retrieved AOD and SSA are susceptible to the small change in surface albedo at lower aerosol loading (Jethva et al., 2014). For instance, an absolute difference of 0.01 in the surface albedo leads to a change in AOD approximately by 0.1 and SSA by ∼0.02.
Figure 7a shows the absolute difference in collocated SSA between OMI and SKYNET as a function of concurrent SKYNET direct AOD (388 or 400 nm) measurements for all aerosol types. All OMI–SKYNET matchup data obtained from a total of 25 sites under all AOD conditions are included here. The data are shown in the box-and-whisker format, where the horizontal lines represent the median value of each bin of sample size 150, the filled circle represents the mean value, and shaded vertical bars cover the 25th and 75th percentiles of the population in each data bin. While for most bins the mean and median values of SSA difference were restricted to within ±0.03, OMI tends to overestimate SSA relative to that of SKYNET at lower AODs giving larger differences and spread in the data population. Similar patterns were observed when the difference in SSA was related to the OMI-retrieved AOD (Fig. 7b). In both cases, the differences in SSA minimize at larger AOD values (> 0.5), suggesting a convergence in both retrievals. Figure 7c shows a similar plot of SSA difference against the concurrent OMI UVAI. Notably, the differences in SSA exhibit a well-defined relationship with UVAI compared to the AOD case (Fig. 7a and b). For UVAI lesser than zero, the differences in the retrieval are found to be beyond the expected uncertainty in both inversions, at least in the mean sense. For the lower range of UVAI, the OMI algorithm mostly employs the urban/industrial model for the retrieval where all aerosols are assumed to be confined within the boundary layer (< 2 km) with a vertical profile that follows an exponential distribution. On the other hand, the mean and median values of the SSA difference for UVAI larger than 0.2 for all bins fall within the 0.03 uncertainty range. The SSA differences approach to near-zero with a reduced spread at larger magnitudes. Notably, both inversions are found to be in closer agreement for UVAI measurements > 0.3.
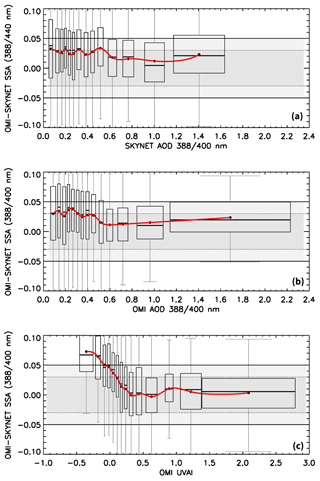
Figure 7Difference in SSA between OMI and SKYNET as a function of the coincident SKYNET-measured (a) and OMI-retrieved (b) aerosol optical depth and OMI-measured UVAI (c). Filled circles in black are the mean of the difference for each AOD and UVAI bin with an equal sample size of 200 matchups; horizontal lines represent the median of the bin samples; the shaded area in gray encompasses data within the 25th (lower) to 75th (higher) percentile range, whereas vertical lines in gray represent 1.5 times the interquartile range (25th to 75th percentile). The dotted and solid horizontal lines are the uncertainty range of ±0.03 and ±0.05, respectively. The width of each box represents the 2 standard deviations of the data contained in the respective bins.
4.1 Uncertainties in the ground-based SKYNET inversion product
The standard SKYNET inversion algorithm assumes a wavelength-independent surface albedo of 0.1 at all wavelengths across the UV-to-visible part of the spectrum. However, the algorithm code allows flexibility to alter the value surface albedo in time and wavelength (Campanelli et al., 2015). The diffuse light reflected from the ground plays a second-order role in the measured sky radiances in most situations; however, it has the potential to affect the SSA inversion; e.g., overestimated (underestimated) surface albedo can underestimate (overestimate) SSA (Dubovik et al., 2000; Khatri et al., 2012). Using simultaneous inversion data from SKYNET and AERONET for four representative sites, Khatri et al. (2016) have shown that the difference in the prescribed surface albedo between SKYNET and AERONET results in a difference of ∼0.04 in SSA at red (675 nm) and near-IR wavelengths retrieved from the two collocated ground sensors. The difference in SSA can also reach as high as ∼0.08 when surface albedo differed by 0.3. The assumed surface albedo value of 0.1 at near-UV (340 and 380 nm) and shorter visible wavelength (400 nm) seems to be unrealistic for the vegetated and urban surfaces. The surface albedo database at 354 and 388 nm derived from multiyear observations from OMI suggests that the vegetated surfaces and urban centers are characterized with the lower values of surface albedo, i.e., ∼0.02–0.03 and ∼0.05, respectively; for desert surfaces, the albedo could be as high as 0.08–0.10. Significant differences in the assumed surface albedo values between OMI and SKYNET at shorter wavelengths could be one of the responsible factors for discrepancies in SSA noted over several sites, particularly at lower aerosol loading when the uncertainty in surface characterization can amplify the error in the SSA inversion.
To further investigate this effect, the difference in SSA between OMI and SKYNET as a function of the simultaneous difference in surface albedo is analyzed and shown in Fig. 8a. The data are presented in a standard box-and-whisker plot format. The analysis reveals a link between differences in SSA and surface albedo, where increasing differences in SSA (OMI > SKYNET) are associated with significant negative biases in surface albedo between OMI and SKYNET. In other words, a large overestimation in SKYNET surface albedo causes an underestimation of retrieved SSA, which is consistent with the findings of Dubovik et al. (2000) and Khatri et al. (2012, 2016), thereby resulting in a substantial positive difference in SSA between OMI and SKYNET. Recently, Mok et al. (2018) have shown that the use of the AERONET surface albedo dataset at 440 nm in the SKYNET algorithm for the South Korea region produces SSA values larger by ∼0.01 at near-UV wavelengths. Notably, differences in SSA tend to be lower when the differences in surface albedo are also minimal, such that the mean and median values of those bins remain within the expected uncertainties of ±0.03 in both retrievals. This result, along with the previous findings cited above, convincingly points out that the SSA inversion from ground-based sensors, especially at lower aerosol loadings, is likely susceptible to the prescribed surface albedo. The assumption of a fixed value of spectral surface albedo of 0.1 in the SKYNET algorithm appears to be inappropriate, requiring a revision using more accurate datasets of spectral reflectance or albedo such as from MODIS and OMI.
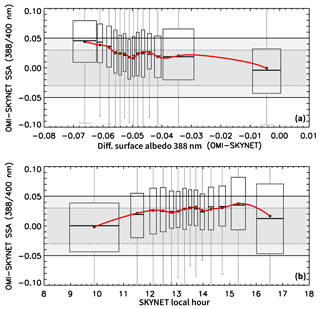
Figure 8Same as in Fig. 7 but the difference in SSA between OMI and SKYNET is related to (a) the difference in surface albedo assumed by the two algorithms and (b) the local measurement hour of SKYNET.
The dependence of the SSA difference on the local hour of SKYNET measurements is quantified in Fig. 8b. The SKYNET dataset accessed from the data server at Chiba University does not contain information on the solar zenith angle. However, the local time of measurements reported in the data file for each station can serve as a proxy for the solar zenith angle. The OMI–SKYNET matchups exhibit a systematic dependency, where the differences between the two datasets become relatively minimal when early morning and late afternoon inversions of SKYNET associated with higher solar zenith angle are collocated with OMI overpass time around 13:30 Equator-crossing time. Owing to a longer atmospheric optical path at higher solar zenith angles, and thereby better aerosol absorption signal, the ground-based aerosol inversions, such as from AERONET and SKYNET, are expected to be more reliable for sky measurements carried out during the early morning/late afternoon.
The SKYNET inversion algorithm (SKYRAD.pack version 4.2 and version 5) assumes aerosols of spherical shape regardless of the actual aerosol type observed in the scene. Following a detailed analysis of the effect of nonsphericity of the particles on the difference between the retrievals carried out assuming spherical and spheroidal size distribution, Khatri et al. (2016) concluded that the assumed shape of particles has a nonsignificant impact on the retrieved SSA. Their study revealed an SSA difference of ±0.01 for measurements having a maximum scattering angle < 120∘ and a difference of up to ±0.02 at a scattering angle > 120∘, where the difference in the phase function is significant between spherical and spheroidal size distributions (Torres et al., 2018). The OMI–SKYNET collocation procedure, as shown in Fig. 4, yields relatively fewer matchups that are identified as dust aerosol type according to the OMAERUV aerosol type identification scheme. A majority of the collocated data points were derived over the desert site of Dunhuang in China, showing a reasonable agreement in SSA between OMI and SKYNET for dust aerosols further supporting the findings of Khatri et al. (2016) that the SSA retrievals are not significantly impacted by the assumption of the shape of particles, i.e., spherical or spheroidal.
Apart from the algorithmic assumptions, the calibration constant used for sky radiances measured by SKYNET instruments can be a potential source of errors in the inversion. The Khatri et al. (2016) study suggests that the calibration constant for sky radiances determined from the disk scan method using a solar disk scan area of (Boi et al., 1999) may be underestimated, resulting in overestimated sky radiance and thus relatively higher SSA. Some of the larger differences in SSA between OMI and SKYNET, where OMI underestimates SSA relative to the SKYNET, can be attributed to the imperfect calibration applied to the SKYNET sensors.
4.2 Possible sources of uncertainties in OMAERUV retrievals
Like other satellite-based remote sensing algorithms, OMAERUV also relies on assumptions about the atmospheric and surface properties for the retrieval of aerosol properties. The single largest known source of error in the OMI retrievals is the subpixel cloud contamination within the OMI footprint. Given the footprint of size 13 km×24 km for near-nadir pixels, which intercept an area of about 338 km2 on the ground, the presence of subpixel clouds may not be avoided entirely. Currently, the algorithm assigns quality flags to each pixel, which carries information on the quality of the retrieval depending upon the observed conditions (Torres et al., 2013). Aerosol retrievals with the quality flag “0” are considered reliable as this category of flag scheme largely avoids cloud-contaminated pixels by choosing the appropriate thresholds in reflectivity and UVAI measurements.
Over the desert regions, e.g., the Dunhuang SKYNET site in China, the frequency of occurrence of clouds is expected to be minimal. Therefore, it is less likely that the SSA retrievals over these sites are affected by cloud contamination. A reasonable agreement between the two retrieval datasets (Fig. 3) supports this assumption. The quality flag scheme, however, cannot entirely rule out the presence of small levels of subpixel cloud contamination or the presence of thin cirrus in the OMI footprint, which can cause overestimation in the retrieval of SSA, such as noted over the SKYNET sites in Kasuga, Etchujima, Seoul, Bologna, and Pune. Larger uncertainties observed over these sites are associated with the urban–industrial aerosol type, possibly because the AODs for this aerosol type are the lowest in the analysis and, therefore, subject to the lower sensitivity to absorption and possibly more affected by subpixel cloud contamination.
Another possible source of uncertainty can be the assumption of the aerosol layer height. The climatology of the aerosol layer height derived from CALIOP measurements adequately describes the observed mean layer of carbonaceous and desert dust aerosols (Torres et al., 2013). It is particularly robust over the arid and semiarid areas where large numbers of cloud-free observations were used in the calculation. However, note that the temporal and spatial coverage of CALIOP is limited to a 16 d repeat cycle over the same location. Variations in the aerosol layer height not observed by CALIOP, therefore, will be missed in the derived climatology and thus can be a source of uncertainty. Sensitivity analysis of the OMAERUV retrievals suggests that an overestimation (underestimation) in the aerosol layer height results in an overestimated (underestimated) SSA. This is because an increase (decrease) in the assumed aerosol layer height from the actual one enhances (reduces) absorption in the radiance lookup table (not in the actual TOA measurements), which the OMAERUV algorithm compensates for by retrieving lower (higher) AOD and higher (lower) SSA to match with the observations.
The third source of uncertainty that can affect SSA retrieval is the accuracy of the prescribed surface albedo. For the surface characterization, the OMAERUV algorithm uses a near-UV surface albedo database derived using the multiyear OMI reflectivity observations. The method adopts a minimum reflectivity approach, ensuring minimal or no contamination from the atmosphere, i.e., aerosols and clouds, in the measurements. Afterward, the minimum reflectivity dataset derived from the OMI observations was adjusted in the temporal domain to the seasonality of surface albedo retrieved in the visible wavelengths from MODIS. The dataset contains surface albedo values at 354 and 388 nm at a grid resolution of . Compared to the previous OMAERUV dataset using the TOMS-based surface albedo product at 1∘ grid resolution, the new OMI-based dataset is expected to be more accurate to within 0.005 to 0.01, owing to its higher spatial resolution and the fact that it is contemporary to the OMI operation. A sensitivity study of the OMAERUV retrievals to the change in surface albedo described in Jethva et al. (2014) suggests that an increase in surface albedo by 0.01 in the near-UV region over desert areas results in a decrease in the magnitude of retrieved SSA by . The effect of uncertain surface albedo can be more pronounced at lower aerosol loading, where the reduced signal from the atmosphere makes OMAERUV retrieval more susceptible to the uncertainty in surface albedo.
The assumed aerosol microphysical and optical properties could be additional sources of uncertainty. The particle size distributions assumed in the OMAERUV models are adopted from long-term AERONET inversion statistics (Dubovik et al., 2002), representing areas influenced by smoke, dust, and urban/industrial aerosols, and therefore are considered realistic representations of the total atmospheric column. The carbonaceous smoke aerosols are assumed to be spherical in shape with a bimodal lognormal size distribution and characterized with a steep absorption gradient, such that the absorption Ångström exponent (AAE) in the near-UV lies in the range 2.5–3.0, to adequately represent the organics in the biomass burning smoke particles (Kirchstetter et al., 2004; Jethva and Torres, 2011). The desert dust aerosol model follows the bimodal lognormal size distribution with particles comprised of randomly oriented spheroids with an axis ratio (shape factor) distribution adopted from Dubovik et al. (2006). The sensitivity study followed by an actual inversion of OMI data presented in Torres et al. (2018) demonstrates that the change in dust particle shape from spherical to spheroidal distribution improved the AOD retrievals significantly and brought the equivalence between the retrievals over the left and right sides of the OMI swath for the oceanic dust belt region of the tropical Atlantic. The associated changes in SSA retrievals were noted within ±0.01 and −0.02 for the scattering angle up to 100–150 and > 160∘, respectively. The OMAERUV version 1.8.9.1 data product used in the present study adopts a new dust model representing spheroidal particles based on the work of Dubovik et al. (2006) and Torres et al. (2018). The spectral dependence of the refractive index in the near-UV assumed in the dust aerosol model is generally consistent with the in situ laboratory measurements (Wagner et al., 2012). For instance, retrieval of AOD and SSA for carbonaceous aerosols using the smoke model with an AAE of 1.90 (10 % relative spectral dependence in the imaginary index between 354 and 388 nm) and 1.0 (no spectral dependence in the imaginary index), instead of the standard AAE assumption of 2.7, results in a decrease in SSA up to −0.07, respectively, suggesting a marked sensitivity of the SSA retrieval to the significant changes in the spectral aerosol absorption. Due to the shortage of ground-based characterization of absorption in the near-UV part of the spectrum, the regional representation of the spectral absorption properties in the OMAERUV models is limited. Therefore, spatial and temporal variations in the spectral properties of aerosols can be a potential source of error in the SSA retrieval.
We presented a comparative analysis of the aerosol SSA retrieved from the OMI's two-channel aerosol algorithm (OMAERUV) against an independent ground-based inversion made by the SKYNET Sun photometers over the selected 25 sites located mainly in Asia and Europe. This study follows our previous efforts of evaluating the OMI near-UV SSA product carried out using the ground-based AERONET dataset (Jethva et al., 2014). The capability of SKYNET sensors to measure the Sun and sky radiance at near-UV wavelengths (340–380–400 nm) and subsequently retrieve the aerosol optical properties, including SSA, at these wavelengths provides a unique opportunity to directly compare the two near-UV SSA products from ground and satellite. Ground-based inversion of SSA at the near-UV wavelengths eliminates the need to adjust and extrapolate satellite retrieval to the visible wavelengths such as in the case with the comparison against AERONET. Since the SSAs inferred from two different platforms are essentially retrieved from two fundamentally different inversion algorithms, the present study does not stand as a validation exercise for either retrieval datasets. Instead, the purpose of this analysis was to check the consistency (or lack thereof) between the two retrieved quantities of the same physical parameter regarding standard statistical comparison, i.e., RMSD and percent of matchups within the expected uncertainties.
Unlike the AERONET Level 2 inversion product that reports spectral SSA when AOD (440 nm) exceeds a value of 0.4, the SKYNET Level 2 dataset delivers spectral SSA in the near-UV and visible parts of the spectrum under all cloud-free observations for all AOD conditions. The collocation procedure that matched temporal inversion data from SKYNET with spatial retrievals from OMI resulted in a total of 2691 collocated data points for AOD > 0.0 and 1223 when AOD > 0.3 collected from 25 sites representing the biomass burning region of Southeast Asia, desert in China, and urban/industrial areas in Japan, India, and Europe. Combined for all 25 sites and under all AOD conditions, we find 38 % and 59 % of the total SKYNET–OMI SSAs agree within their estimated uncertainty range of ±0.03 and ±0.05, respectively, with an overall root-mean-square difference of 0.06. When restricted with the condition AOD > 0.3 in both measurements, the agreement of the comparison improved to 51 % and 72 % with a root-mean-square difference of 0.047. When segregated by aerosol type, the agreement between the two sensors is found to be robust for matchups identified as the carbonaceous aerosols over several sites in Japan, Seoul in South Korea, Phimai in Thailand, and New Delhi in India, yielding 61 % and 84 % of data points falling within the limits of ±0.03 and ±0.05 with an overall RMSD of 0.035. The collocation procedure found few matchups for desert dust aerosol, mostly over the Dunhuang site in China, showing a reasonable comparison with 50 % and 68 % of data points within expected uncertainty limits. Among the three major aerosol types, the urban/industrial type aerosols provide the maximum number of matchup data points with a relatively poorer comparison, where 45 % and 67 % of data are found to be within the uncertainty limits.
The differences in SSA between OMI and SKYNET are found to be larger at lower aerosol loading, where OMI retrieves significantly higher SSA compared to that of SKYNET. However, the differences are minimized at larger AOD values (> 0.5), suggesting a convergence in both retrievals at moderate to larger aerosol loading. Similarly, the differences in SSA exhibit a stronger relationship to UVAI showing larger discrepancies beyond expected uncertainty limits at lower UVAIs (< 0) but nearing zero with a reduced spread in matchups at larger magnitudes of UVAI (> 0.2–0.3).
Much of the inconsistency observed between OMI and SKYNET at lower aerosol loading indicates retrieval issues due to reduced signal-to-noise ratio and uncertain algorithmic assumptions. For instance, the OMAERUV retrievals are more susceptible to the changes in surface albedo at lower AODs and to the spectral absorption at higher AODs (Jethva and Torres, 2011). On the other hand, the SKYNET inversion algorithm assumes a wavelength-independent surface albedo of 0.1 across the UV to visible-near-IR wavelengths, which appears to be unrealistic, especially in the UV region where OMI surface albedo dataset reports much lower values (< 0.05) over land. Though the reflected light from surface plays a second-order role in the ground-based retrievals, previous studies and results derived in the present work (Fig. 8) show that the uncertainty in surface albedo can cause non-negligible errors in SSA retrievals that likely exceed the expected accuracy level of ±0.03.
Despite the inherent uncertainties associated with both satellite and ground inversion products, a good level of agreement between the two independent techniques over SKYNET sites under the favorable conditions, i.e., at higher aerosol loading, higher solar zenith angle, and when the surface albedo assumption is consistent, is encouraging. We intend to extend the present analysis to other SKYNET sites whose data are still not directly accessible in the public domain. Continuing the evaluation of inversion products, both from satellite and ground, is an important exercise to track the changes and improvements in the algorithms and resulting data products and to establish the consistency (or lack thereof) that can help to diagnose further and improve the accuracy of retrievals.
The SKYNET aerosol dataset was accessed from the data repository of the Center for Environmental Remote Sensing (CEReS), Chiba University, Japan (http://atmos3.cr.chiba-u.jp/skynet/data.html, Takamura and Nakajima, 2004). The OMI/OMAERUV aerosol retrieval dataset (https://doi.org/10.5067/Aura/OMI/DATA2004, Torres, 2006) was obtained from the NASA Goddard Earth Sciences (GES) Data and Information Services Center (DISC) server at https://aura.gesdisc.eosdis.nasa.gov/data/Aura_OMI_Level2/OMAERUV.003/ (last access: 19 November 2019). The comparison results of OMI–SKYNET single-scattering albedo products presented in this paper are freely available from the first author on request.
HJ, the leading author, conceptualized the study and wrote the paper. He conducted a comparative data analysis of OMI- and SKYNET-retrieved single-scattering albedo products presented in the paper. OT (2nd author) brought his expertise in interpreting the results and helped improve the manuscript write-up.
The authors declare that they have no conflict of interest.
This article is part of the special issue “SKYNET – the international network for aerosol, clouds, and solar radiation studies and their applications (AMT/ACP inter-journal SI)”. It is not associated with a conference.
We thank the Center for Environmental Remote Sensing (CEReS), Chiba University, Japan (http://atmos3.cr.chiba-u.jp/skynet/data.html), for the online availability of the SKYNET dataset for several sites in Japan, South Korea, China, India, Italy, and Germany. Acknowledgments are also due to the principal investigators and their staff for establishing and maintaining respective SKYNET sites, whose data are used in the present work. We acknowledge the support of NASA GES-DISC, the NASA Earth Science data center, for the online availability of the OMI aerosol product assessed in this analysis. Thanks are due to the two anonymous reviewers for offering constructive comments leading to the improvements in the article.
This paper was edited by Stelios Kazadzis and reviewed by two anonymous referees.
Ahn, C., Torres, O., and Bhartia, P. K.: Comparison of Ozone Monitoring Instrument UV Aerosol Products with Aqua/Moderate Resolution Imaging Spectroradiometer and Multiangle Imaging Spectroradiometer observations in 2006, J. Geophys. Res., 113, D16S27, https://doi.org/10.1029/2007JD008832, 2008.
Ahn, C., Torres, O., and Jethva, H.: Assessment of OMI near-UV aerosol optical depth over land, J. Geophys. Res.-Atmos., 119, https://doi.org/10.1002/2013JD020188, 2014.
Boi, P., Tonna, G., Dalu, G., Nakajima, T., Olivieri, B., Pompei, A., Campanelli, M., and Rao, R.: Calibration and data elaboration procedure for sky irradiance measurements, Appl. Optics, 38, 896–907, 1999.
Campanelli, M., Nakajima, T., and Olivieri, B.: Determination of the solar calibration constant for a sun-sky radiometer, Appl. Optics, 43, 651-659 https://doi.org/10.1364/AO.43.000651, 2004.
Campanelli, M., Estelles, V., Tomasi, C., Nakajima, T., Malvestuto, V., and Martinez-Lozan, J. A.: Application of the SKYRAD improved Langley plot method for the in situ calibration of CIMEL sun-sky photometers, Appl. Optics, 46, 2688–2702, https://doi.org/10.1364/AO.46.002688, 2007.
Campanelli, M., Estellés, V., Colwell, S., Shanklin, J., and Ningombam S. S.: Analysis of aerosol optical properties from continuous sun-sky radiometer measurements at Halley and Rothera, Antarctica over seven years, Geophysical Research Abstracts, Vol. 17, EGU2015-2768, EGU General Assembly, Vienna, Austria, 12–17 April 2015.
Campanelli, M., Iannarelli, A. M., Kazadzis, S., Kouremeti, N., Vergari, S., Estelles, V., Diemoz, H., di Sarra, A., and Cede, A.: The QUATRAM Campaign: QUAlity and TRaceabiliy of Atmospheric aerosol Measurements, The 2018 WMO/CIMO Technical Conference on Meteorological and Environmental Instruments and Methods of Observation (CIMO TECO-2018) “Towards fit-for-purpose environmental measurements”, 8–11 October 2018, Amsterdam, the Netherlands, 2018.
Che, H., Shi, G., Uchiyama, A., Yamazaki, A., Chen, H., Goloub, P., and Zhang, X.: Intercomparison between aerosol optical properties by a PREDE skyradiometer and CIMEL sunphotometer over Beijing, China, Atmos. Chem. Phys., 8, 3199–3214, https://doi.org/10.5194/acp-8-3199-2008, 2008.
Dubovik, O., Smirnov, A., Holben, B. N., King, M. D., Kaufman, Y. J., Eck, T. F., and Slutsker, I.: Accuracy assessments of aerosol optical properties retrieved from Aerosol Robotic Network (AERONET) Sun and sky radiance measurements, J. Geophys. Res., 105, 9791–9806, https://doi.org/10.1029/2000JD900040, 2000.
Dubovik, O., Holben, B. N., Eck, T. F., Smirnov, A., Kaufman, Y. J., King, M. D., Tanre, D., and Slutsker, I.: Variability of absorption and optical properties of key aerosol types observed in worldwide locations, J. Atmos. Sci., 59, 590–608, 2002.
Dubovik, O., Sinyuk, A., Lapyonok, T., Holben, B. N., Mishcenko, M., Yang, P., Eck, T. F., Volten, H., Munoz, O., Vehelmann, B., van der Zande, W. J., Leon, J. F., Sorokin, M., and Slutsker, I.: Application of spheroid models to account for aerosol particle nonsphericity in remote sensing of desert dust, J. Geophys. Res., 111, D11208, https://doi.org/10.1029/2005JD006619, 2006.
Estellés, V., Campanelli, M., Smyth, T. J., Utrillas, M. P., and Martínez-Lozano, J. A.: Evaluation of the new ESR network software for the retrieval of direct sun products from CIMEL CE318 and PREDE POM01 sun-sky radiometers, Atmos. Chem. Phys., 12, 11619–11630, https://doi.org/10.5194/acp-12-11619-2012, 2012a.
Estellés, V., Campanelli, M., Utrillas, M. P., Expósito, F., and Martínez-Lozano, J. A.: Comparison of AERONET and SKYRAD4.2 inversion products retrieved from a Cimel CE318 sunphotometer, Atmos. Meas. Tech., 5, 569–579, https://doi.org/10.5194/amt-5-569-2012, 2012b.
Estelles, V., Kouremeti, N., Campanelli, M., Grobner, J., Mari nez-Lozano, J. A., and Kazadzis, S.: Preliminary aerosol optical depth comparison between ESR/SKYNET, AERONET and GAW international networks, International SKYNET workshop, Rome (Italy), 20 March 2016.
Hansen, J., Sato, M., and Ruedy, R.: Radiative forcing and climate response, J. Geophys. Res., 102, 6831–6864, https://doi.org/10.1029/96JD03436, 1997.
Hashimoto, M., Nakajima, T., Dubovik, O., Campanelli, M., Che, H., Khatri, P., Takamura, T., and Pandithurai, G.: Development of a new data-processing method for SKYNET sky radiometer observations, Atmos. Meas. Tech., 5, 2723–2737, https://doi.org/10.5194/amt-5-2723-2012, 2012.
IPCC: Climate Change 2013: The Physical Science Basis. Contribution of Working Group I to the Fifth Assessment Report of the Intergovernmental Panel on Climate Change, edited by: Stocker, T. F., Qin, D., Plattner, G.-K., Tignor, M., Allen, S. K., Boschung, J., Nauels, A., Xia, Y., Bex, V., and Midgley, P. M., Cambridge University Press, Cambridge, United Kingdom and New York, NY, USA, 1535 pp., https://doi.org/10.1017/CBO9781107415324, 2013.
Jethva, H. and Torres, O.: Satellite-based evidence of wavelength-dependent aerosol absorption in biomass burning smoke inferred from Ozone Monitoring Instrument, Atmos. Chem. Phys., 11, 10541–10551, https://doi.org/10.5194/acp-11-10541-2011, 2011.
Jethva, H., Torres, O., and Ahn, C.: Global assessment of OMI aerosol single-scattering albedo using ground-based AERONET inversion, J. Geophys. Res.-Atmos., 119, 9020–9040, https://doi.org/10.1002/2014JD021672, 2014.
Khatri, P. and Takamura, T.: An algorithm to screen cloud affected data for sky radiometer data analysis, J. Meteorol. Soc. Jpn., 87, 189–204, 2009.
Khatri, P., Takamura, T., Yamazaki, A., and Kondo, Y.: Reterival of key aerosol optical parameters for spectral direct and diffuse irradiances measured by a horizontal surface detector, J. Atmos. Ocean. Tech., 29, 683–696, 2012.
Khatri, P., Takamura, T., Nakajima, T., Estellés, V., Irie, H., Kuze, H., Campanelli, M., Sinyuk, A., Lee, S.-M., Sohn, B. J., Pandithurai, G., Kim, S.-W., Yoon, S. C., Martinez-Lozano, J. A., Hashimoto, M., Devara, P. C. S., and Manago, N.: Factors for inconsistent aerosol single scattering albedo between SKYNET and AERONET, J. Geophys. Res.-Atmos., 121, 1859–1877, https://doi.org/10.1002/2015JD023976, 2016.
Kirchstetter, T. W., Novakov, T., and Hobbs, P. V.: Evidence that the spectral dependence of light absorption by aerosols is affected by organic carbon, J. Geophys. Res., 109, D21208, https://doi.org/10.1029/2004JD004999, 2004.
Mok, J., Krotkov, N. A., Torres, O., Jethva, H., Li, Z., Kim, J., Koo, J.-H., Go, S., Irie, H., Labow, G., Eck, T. F., Holben, B. N., Herman, J., Loughman, R. P., Spinei, E., Lee, S. S., Khatri, P., and Campanelli, M.: Comparisons of spectral aerosol single scattering albedo in Seoul, South Korea, Atmos. Meas. Tech., 11, 2295–2311, https://doi.org/10.5194/amt-11-2295-2018, 2018.
Nakajima, T., Tonna, G., Rao, R., Boi, P., Kaufman, Y., and Holben, B.: Use of sky brightness measurements from ground for remote sensing of particulate polydispersions, Appl. Optics, 35, 15, 2672–2686, 1996.
Schenkeveld, V. M. E., Jaross, G., Marchenko, S., Haffner, D., Kleipool, Q. L., Rozemeijer, N. C., Veefkind, J. P., and Levelt, P. F.: In-flight performance of the Ozone Monitoring Instrument, Atmos. Meas. Tech., 10, 1957–1986, https://doi.org/10.5194/amt-10-1957-2017, 2017.
Takamura, T. and Nakajima, T. : Overview of SKYNET and its activities, Opt. Pura Apl. 37, 3303–3308, 2004 (data available at: http://atmos3.cr.chiba-u.jp/skynet/data.html, last access: 19 November 2019).
Torres, O.: OMI/Aura Near UV Aerosol Optical Depth and Single Scattering Albedo 1-orbit L2 Swath 13×24 km V003, Greenbelt, MD, USA, Goddard Earth Sciences Data and Information Services Center (GES DISC), https://doi.org/10.5067/Aura/OMI/DATA2004, 2006.
Torres, O., Bhartia, P. K., Herman, J. R., Ahmad, Z., and Gleason, J.: Derivation of aerosol properties from satellite measurements of backscattered ultraviolet radiation: Theoretical basis, J. Geophys. Res., 103, 17099–17110, https://doi.org/10.1029/98JD00900, 1998.
Torres, O., Bhartia, P. K., Sinyuk, A., Welton, E. J., and Holben, B.:Total Ozone Mapping Spectrometer measurements of aerosol absorption from space: Comparison to SAFARI 2000 ground-based observations, J. Geophys. Res., 110, D10S18, https://doi.org/10.1029/2004JD004611, 2005.
Torres, O., Tanskanen, A., Veihelmann, B., Ahn, C., Braak, R., Bhartia, P. K., Veefkind, P., and Levelt, P.: Aerosols and surface UV products from Ozone Monitoring Instrument observations: An overview, J. Geophys. Res., 112, D24S47, https://doi.org/10.1029/2007JD008809, 2007.
Torres, O., Ahn, C., and Chen, Z.: Improvements to the OMI near-UV aerosol algorithm using A-train CALIOP and AIRS observations, Atmos. Meas. Tech., 6, 3257–3270, https://doi.org/10.5194/amt-6-3257-2013, 2013.
Torres, O., Bhartia, P. K., Jethva, H., and Ahn, C.: Impact of the ozone monitoring instrument row anomaly on the long-term record of aerosol products, Atmos. Meas. Tech., 11, 2701–2715, https://doi.org/10.5194/amt-11-2701-2018, 2018.
Wagner, R., Ajtai, T., Kandler, K., Lieke, K., Linke, C., Müller, T., Schnaiter, M., and Vragel, M.: Complex refractive indices of Saharan dust samples at visible and near UV wavelengths: a laboratory study, Atmos. Chem. Phys., 12, 2491–2512, https://doi.org/10.5194/acp-12-2491-2012, 2012.