the Creative Commons Attribution 4.0 License.
the Creative Commons Attribution 4.0 License.
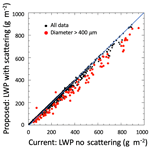
Ground-based observations of cloud and drizzle liquid water path in stratocumulus clouds
Maria P. Cadeddu
Virendra P. Ghate
Mario Mech
The partition of cloud and drizzle water path in precipitating clouds plays a key role in determining the cloud lifetime and its evolution. A technique to quantify cloud and drizzle water path by combining measurements from a three-channel microwave radiometer (23.8, 30, and 90 GHz) with those from a vertically pointing Doppler cloud radar and a ceilometer is presented. The technique is showcased using 1 d of observations to derive precipitable water vapor, liquid water path, cloud water path, drizzle water path below the cloud base, and drizzle water path above the cloud base in precipitating stratocumulus clouds. The resulting cloud and drizzle water path within the cloud are in good qualitative agreement with the information extracted from the radar Doppler spectra. The technique is then applied to 10 d each of precipitating closed and open cellular marine stratocumuli. In the closed-cell systems only ∼20 % of the available drizzle in the cloud falls below the cloud base, compared to ∼40 % in the open-cell systems. In closed-cell systems precipitation is associated with radiative cooling at the cloud top and a liquid water path >200 g m−2. However, drizzle in the cloud begins to exist at weak radiative cooling and liquid water path g m−2. Our results collectively demonstrate that neglecting scattering effects for frequencies at and above 90 GHz leads to overestimation of the total liquid water path of about 10 %–15 %, while their inclusion paves the path for retrieving drizzle properties within the cloud.
- Article
(3915 KB) - Full-text XML
- BibTeX
- EndNote
Marine stratocumulus clouds have a significant impact on the Earth's radiation balance as they reflect a greater amount of solar radiation back to space compared to the ocean surface and emit a similar amount of longwave radiation as the surface. The processes affecting their highly organized spatial structure and their spatial and temporal variability are a topic of active research (Wood et al., 2015). Precipitation is hypothesized to play an important role in the transition between different mesoscale organizations of boundary layer clouds (Feingold and McComiskey, 2016; Wang and Feingold, 2009). Similarly, precipitation together with entrainment impact the cloud microphysical properties that determine the cloud radiative effects (Wood, 2012; Yamaguchi et al., 2017). Hence, characterizing the properties of drizzling stratocumulus clouds through observations and high-resolution models for furthering our understanding of the precipitation processes has been a focus of several previous studies (e.g., Ahlgrimm and Forbes, 2014; Zheng et al., 2017). Using ground-based instrumentation, the study of microphysical and macro-physical cloud properties involves combining data from multiple instruments to retrieve parameters of the hydrometeor drop size distribution (DSD). For example, the radar reflectivity is proportional to the sixth moment of the DSD and was used to retrieve liquid water content that is the third moment of DSD by Frisch et al. (2002). For this purpose, new algorithms are developed that can extract key cloud and drizzle properties such as liquid water content and drop effective radius from a combination of active (e.g., radar, lidar), and passive (broadband or narrowband radiometers) sensors (e.g., Frisch et al., 1995; Fielding et al., 2014). Microwave radiometers have been extensively used in the past in such retrieval techniques to obtain the total column (i.e., cloud and drizzle) liquid water path of a precipitating cloud. By adding a 90 or 183 GHz channel to the traditional 23 and 30 GHz channels, the uncertainty in the retrieved liquid water path (LWP) (and column water vapor) can been reduced significantly (Löhnert and Crewell, 2003). Ground-based retrievals in precipitating or even drizzling conditions are however still an area of active research. Granted that heavy precipitation does affect the measurements by altering the dielectric properties of the surface over which water deposits, the degree to which light precipitation affects the retrieval outcome is still unclear (Wall et al., 2017; Bosisio et al., 2013). Recent theoretical studies (Cadeddu et al., 2017) have shown that drizzle-sized hydrometeors (larger than 90 µm in diameter) significantly scatter the radiation at 90 GHz and could also be used to derive separate estimates of integrated drizzle water and cloud water.
In this work we propose a technique to retrieve column-integrated values of (i) drizzle water path below the cloud base (DWPbc), (ii) drizzle water path above the cloud base (DWPac), and (iii) cloud water above the cloud base (CWP) by combining the data from vertically pointing cloud radar, lidar, and a microwave radiometer. The technique is applied to 20 d of data collected at the Atmospheric Radiation Measurement (ARM) Eastern North Atlantic (ENA) site during light to moderately precipitating stratocumulus cloud conditions. In Sect. 2 an overview of the methodology is provided followed by application to 1 d of data. In Sect. 3 the results are qualitatively assessed by comparison with radar-observed Doppler spectra. The entire dataset of 20 d is examined in Sect. 4 through averages of in-cloud and below-cloud-base drizzle properties for the precipitating shafts, and the relation between LWP, turbulence, and drizzle production is shown. The results are summarized and briefly discussed in Sect. 5.
In Sect. 2.1 an overview of the instrumentation and the radiative transfer models is provided. The use of active sensors to derive microphysical properties of drizzle below cloud base is well established and is used in the first part of the algorithm, the active module, described in Sect. 2.2. In the second part of the algorithm, named the passive module, the novel approach of using scattering properties of drizzle drops to separate cloud and drizzle water path within the cloud is followed. The passive module is described in Sect. 2.3.
2.1 Instrumentation and radiative transfer models
The ARM ENA site has been operational since the summer of 2015 and is located at the northern tip of the northernmost island Graciosa (39∘ N, 28∘ W, 15 m) in the Azores. The site has many instruments, and here we describe those used in this work. A vertically pointing Ka-band Doppler radar named Ka-band ARM Zenith Radar (KAZR) continuously records the raw reflectivity-weighted Doppler spectrum and its first three moments at 2 s temporal and 20 m range resolution. Collocated with the KAZR is a laser ceilometer (lidar) that operates at the 905 nm wavelength and reports the first three optical cloud-base heights and the raw attenuated backscatter at 15 s temporal and 30 m range resolution. A three-channel microwave radiometer is also present at the site that records the calibrated brightness temperatures at 23.8, 30, and 90 GHz frequencies at 10 s temporal resolution. Balloon-borne radiosondes are launched at the site every 12 h at 00:00 and 12:00 UTC. Due to the sparseness of the radiosonde launches, the radiosonde data are interpolated with those from the ECMWF model to deduce profiles of temperature, pressure, humidity, and winds at a uniform 1 min temporal and 50 m vertical resolution. The visible imagery and cloud-top temperature reported by the Spinning Enhanced Visible and Infrared Imager (SEVIRI) on board the geostationary Meteosat satellite were used to confirm the presence of similar cloud conditions around the site as those observed at the site.
The ceilometer attenuated backscatter was filtered for noise using the technique proposed by Kotthaus et al. (2016) and was calibrated following O'Connor et al. (2005) using data collected on 7 March 2016. More details about the ceilometer calibration are mentioned in the Appendix of Ghate and Cadeddu (2019), referred to as GC19 from here on. The KAZR was calibrated by comparing its reflectivity with that from the Ka-band Scanning ARM Cloud Radar that was calibrated using a corner reflector. The KAZR calibration hence is good within 1 dB. The KAZR and ceilometer data were combined to produce estimates of the first three moments of Doppler spectra and of ceilometer attenuated backscatter on a uniform 1 min temporal and 50 m range resolution following Clothiaux et al. (2000). These were further used to calculate cloud boundaries. Microwave radiometer data are collected by a three-channel radiometer (23.8, 30, 90 GHz). The radiometer is calibrated using tip curves (Han and Westwater, 2000) resulting in a calibrated brightness temperature uncertainty of about 0.3 K in the K-band and 1 K in the W-band. The resulting uncertainty in the derived products is about 0.4 kg m−2 for precipitable water vapor (PWV) and 15 g m−2 for LWP. Precipitable water vapor and liquid water path derived using a neural network algorithm (Cadeddu et al., 2009) are provided in the data file. These retrievals are derived with an absorption-only radiative transfer model, MonoRTM (Clough et al., 2005), and are used as a priori information in the algorithm described in this work.
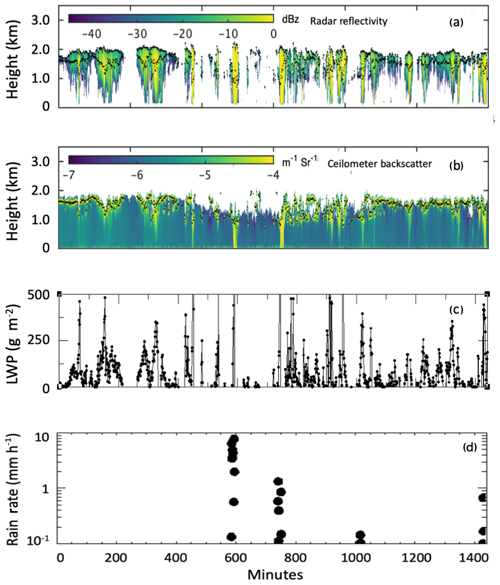
Figure 1(a) Time–height profiles of KAZR-reported reflectivity (shades) together with cloud boundaries from KAZR (cloud top) and ceilometer (cloud base) in black, (b) time–height profiles of ceilometer attenuated backscatter (shades), (c) time series of microwave-radiometer-reported LWP from MWRRETv2 (Turner, 2007), and (d) rain rate at the surface from the video disdrometer (log scale). The data were collected on 21 November 2016. Data in (a) and (b) are 1 min averaged; data in (c) are smoothed with a 5 min running average.
We use the Passive and Active Microwave TRAnsfer (PAMTRA) package (Mech et al., 2018) available at https://github.com/igmk/pamtra (last access: 18 March 2020), a scattering microwave radiative transfer model that simulates active and passive measurements in plane-parallel geometry between 1 and 800 GHz. The calculations are based on the fully polarized model of Evans and Stephens (1995) for nonspherical and oriented particles. The model simulates passive measurements in upward and downward geometry at a given height and allows the choice between different assumptions and models in the calculations of surface emissivity, ice crystal habit, size distribution, and calculation of scattering properties. The Rapid Radiative Transfer Model (RRTM) (Iacono et al., 2000) was used to calculate the radiative fluxes and heating rates. We refer the reader to GC19 regarding the details of the setup and inputs of RRTM.
Examples of the noise-filtered profiles of KAZR-reported reflectivity, ceilometer-reported attenuated backscatter, and the concurrent retrievals of LWP from MWRRET2 (Turner, 2007) in non-scattering approximation are shown in Fig. 1a–c. Moderately to heavily precipitating stratocumulus clouds were observed throughout the day, with most of the precipitation evaporating before reaching the surface. Precipitation measurements at the surface from the video disdrometer are shown in Fig. 1d.
2.2 The active module
The active module of the retrieval technique is similar to that proposed by O'Connor et al. (2005) and applied to the ARM data by GC19 with some subtle differences. Drizzle below the cloud base is assumed to have a three-parameter gamma drop size distribution. The ceilometer attenuated backscatter, radar reflectivity, mean Doppler velocity, and width of the Doppler spectra were used in an iterative manner to retrieve the three parameters of the gamma distribution. Details of the radar–lidar microphysical retrievals of drizzle properties below the cloud base are given in GC19 together with an extensive discussion of the range of validity of the algorithm. The lidar signal attenuates at the cloud base as the lidar ratio (extinction to backscatter) of cloud drops is 50–60 Sr compared to 19 Sr or lower of drizzle drops at the 905 nm wavelength. Hence, the ceilometer attenuated backscatter peaks at the cloud base due to the presence of smaller but more numerous cloud drops in addition to the drizzle drops. The returns at the cloud base from pixels containing both cloud and drizzle drops were neglected by GC19. In this work we assume the DSD of this cloud and drizzle mix to have a lognormal shape with a width of 0.38 and we retrieve the modal diameter and number concentration. These serve as a priori information in the retrieval framework.
The retrieved modal diameter and rain rate for the case shown in Fig. 1 are shown in Fig. 2a and b. During this day, the drizzle modal diameter was between 100 and 800 µm and rain rate was around 2.5 mm d−1 with brief peaks greater than 10 mm d−1. Precipitation shafts were identified using the criteria explained in G19 and shown as black solid lines in Fig. 2a. In this specific case 24 drizzle shafts were identified with measurable precipitation detected at the surface for some of the drizzle shafts. Although this does not constitute a problem for the active instrumentation, it does affect the passive module because excessive water deposition on the radiometer can affect the data. At the cloud base the average modal diameter of the mixed drizzle–cloud DSD was 77.8 µm.
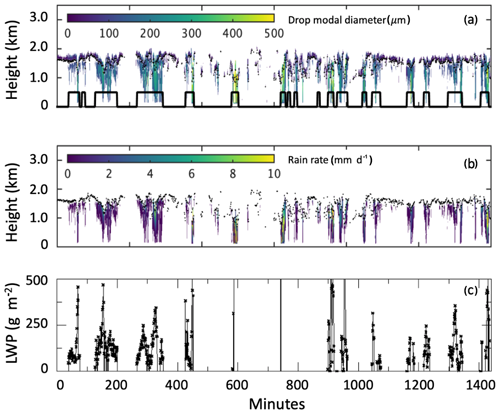
Figure 2(a) Time–height profiles of retrieved drizzle drop modal diameter below cloud (shades) and identified drizzle shafts (black line), (b) time–height profiles of rain rate, and (c) time series of retrieved LWP during precipitating shafts using PAMTRA. The data were collected on 21 November 2016. Data in (a) and (b) are 1 min averaged; data in (c) are smoothed with a 5 min running average.
2.3 The passive module
Table 1The passive module of the retrieval algorithm. Mean values and SDs of a priori retrieved quantities for all the cases where the retrieval converged are shown in bold.
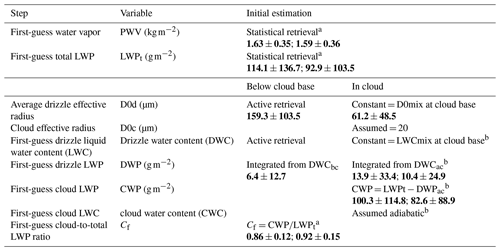
a Retrieved with passive module. b Adjusted during the retrieval to be consistent with integrated amounts.
The output from the active (radar–lidar) module is used as input to the microwave radiative transfer model. The theoretical basis for the retrieval is provided in Cadeddu et al. (2017). In this operational implementation only three quantities are retrieved: PWV, total liquid water path (LWPt), and Cf, the ratio of cloud to total water path. The radiative transfer code, PAMTRA, used in the passive module requires information on the cloud and drizzle DSD, specifically liquid water content, the shape parameter, and effective diameter. Because the microwave measurements are insensitive to the gamma parameter of the DSD, this last is set to zero in the passive module denoting exponential distribution. The below-cloud drizzle water content (DWCbc), below-cloud drizzle water path (DWPbc), and average drizzle effective radius below cloud base calculated from the active module are provided to the radiative transfer model. These properties of drizzle below are kept intact during the entire iterative process within the passive module. Figure 3 shows a flowchart of the active and passive modules with the quantities provided as input, the intermediate outputs, and the final output. Additional details of the passive module are provided in Table 1.
Because in-cloud properties are not easily derived and the active module is only valid at and below cloud base, several assumptions had to be made about the in-cloud DSD parameters. The drizzle water content above cloud base (DWCac) is assumed constant with value equal to the drizzle water content at the cloud base (Wood, 2005), and the cloud water content (CWC) is assumed to follow an adiabatic profile (Zuidema et al., 2005). The initial adiabatic profile is determined by subtracting the initial drizzle water path (Table 1, row 6) from the initial total LWP (LWPt in Table 1, row 2) and distributing the resulting cloud water path adiabatically between cloud base and top. These estimates of CWP and the first-guess LWPt are used to provide the first-guess estimate of Cf as shown in the flowchart (Table 1, row 9). At each iteration the drizzle water path above cloud base (DWPac) and CWP are adjusted based on LWPt and Cf to ensure consistency with the drizzle below cloud base by scaling the liquid water content accordingly. Once the retrieval converges, the diagonal elements of the covariance matrix can provide information on the reduction of the uncertainty of the three retrieved parameters.
The retrieval of the Cf parameter depends on how much the scattering information affects the measurement and is therefore dependent on the drop size distribution. It is expected that the retrieval will be more effective during precipitation characterized by drops larger than 100 µm in diameter. The advantage of having larger drops is however offset by the fact that they usually reach the surface, which impacts the convergence because of water deposition on the radiometer window. This limitation of the ground-based instrument is evident in Fig. 2c where the total LWP from this work is shown during precipitating shafts. On 21 November 2016 the retrieval converged in 367 out of the 484 min identified in the drizzle shafts. Using the proposed technique from aircraft or satellites will enable the study of a wider range of precipitating conditions and take better advantage of the scattering information. In fact, based on a similar principle, Jacob et al. (2019) applied a neural network retrieval to microwave measurements collected from aircraft to separate cloud from drizzle water path over the Atlantic Ocean.
Total, cloud, and drizzle water path during the first 4 h of 21 November 2016 (minutes 1–240) are shown in Fig. 4a and b. Although the below-cloud drizzle is well defined in the active retrieval process, the information that can be gained from the microwave retrieval on the partition of cloud and drizzle depends on how much information is available from the measurements. The CWP constitutes the largest portion of the total LWP, and the resulting total drizzle water path (in cloud and below cloud) is in this case about twice the precipitating drizzle. In the next section the in-cloud partition between drizzle and cloud water path is closely examined next to the radar Doppler spectra on 21 November 2016.
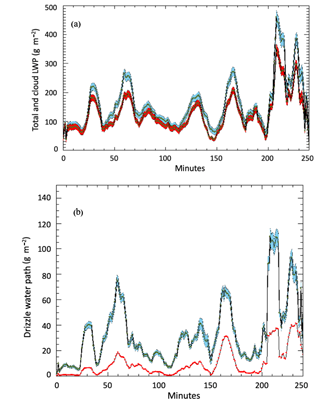
Figure 4(a) Total (blue) and cloud (red) LWP. (b) Total drizzle water path (blue) and below-cloud drizzle water path (red) between 00:00 and 04:00 UTC on 21 November 2016. The data are smoothed with a 10 min boxcar average for better readability. Shaded regions represent the 1-sigma uncertainty provided by the optimal estimation algorithm.
Due to lack of other coincident retrievals of cloud and drizzle water within the cloud layer, here we qualitatively evaluate them by separating the cloud and drizzle contributions in the Doppler spectra. Possible ways and the challenges of quantitatively evaluating these retrievals are discussed in the last section.
3.1 Radar spectra processing
Doppler spectra from cloud radars have been previously used to gain insight into the onset and evolution of drizzle in clouds (Kollias et al., 2011a, b; Luke and Kollias, 2013; Acquistapace et al., 2019). The methodology is based on the fact that the Doppler spectra of a non-precipitating cloud are centered on the zero-mean velocity due to their movement with turbulence, while those containing falling drizzle drops are negatively skewed due to their fall velocity. Hence, the presence of drizzle drops in a cloud introduces a negative skewness in the cloud Doppler spectra. In this section, cloud Doppler spectra are analyzed with the intent of separating the cloud and drizzle components to qualitatively evaluate their co-variability.
In the following analysis the Doppler spectra were averaged for 1 min to reduce the effect of turbulence and they were denoised using the technique of Hildebrand and Sekhon (1974). Doppler spectra for six drizzle shafts that lasted for more than 20 min on 21 November 2016 and for which the microwave retrieval converged at least 75 % of the times are analyzed. Figure 5 shows examples of Doppler spectra from the drizzle shaft that developed between 04:22 and 05:50 UTC (minutes 262–350 in Figs. 1 and 2). The shift in the location of the peak towards negative velocity near the cloud base (Fig. 5a) indicates the presence of drizzle drops that dominate the radar signal. Gates near the cloud top on the other hand have peaks centered around the zero velocity, indicating the presence of cloud drops. In Fig. 5a the increase in the power of the signal as drizzle drops become the dominant contribution to the radar reflectivity is also noticeable. To separate the drizzle from the cloud contribution in the power spectra, the assumption was made that the signal originating near the cloud top is mostly generated by cloud droplets. This assumption holds true in weak and moderate drizzling conditions; however it fails in heavily precipitating clouds when the Doppler spectra at the cloud top are as negatively skewed as the Doppler spectra at cloud base. The spectra for layers near the cloud top were vertically averaged and fitted to a Gaussian distribution. The terminal fall velocity of cloud droplets is very small, and their observed Doppler velocity distribution is a result of turbulence. The standard deviation of the near-cloud-top Gaussian distribution was taken as representative of the velocity spread of the cloud droplet distribution through the cloud. Cloud-only spectra near the cloud top at 04:29, 04:35, and 04:56 UTC are shown in blue in Fig. 5b–d. Note that the vertical velocity was converted into drop diameter using the relation between fall velocity and diameter from Frisch et al. (1995) and Gossard et al. (1990). To isolate the cloud component, the right shoulder of the curve is fitted to a Gaussian distribution with standard deviation given by the cloud-only distribution (red curve). When this estimated cloud component is subtracted from the cloud-averaged spectra, the resulting distribution (shown in purple) is considered representative of the drizzle-only signal. The areas under the final cloud and drizzle spectra (indicated by the red and purple stripes, respectively) are proportional to the total mass of cloud and drizzle water responsible for the radar signal under the Rayleigh scattering regime with some modifications during the Mie scattering regime. Although the analysis is qualitative, it can be seen that the procedure captures the evolution of the drizzle from its initial stage to a stage where the drizzle component becomes more prominent in the cloud.
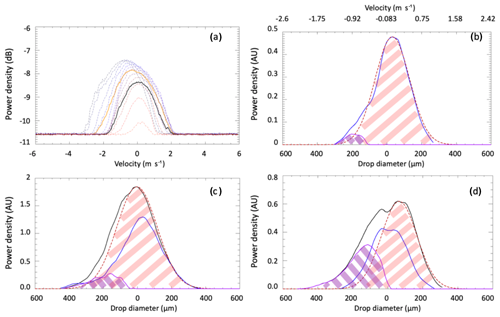
Figure 5(a) Radar Doppler spectra between cloud base and cloud top at 04:30 UTC on 21 November 2016. (b–d) Doppler spectra averaged between cloud base and cloud top (black); averaged over cloud-only layers (blue); Gaussian fitted curve (red); drizzle component (purple) at 04:29 (b), 04:35 (c), and 04:56 (d) UTC. The black line in (b) is entirely under the blue line. All Doppler spectra are minute averaged. On the top x axis of (b) the velocity corresponding to the calculated diameter is shown. Negative velocities refer to downward motion. The drop diameter on the x axis was derived using the size–velocity relation in Gossard et al. (1990).
3.2 Radar and radiometer
The areas under the red and purple curves shown in Fig. 5b–d are shown in Fig. 6a and b for two entire drizzle shafts (04:22–05:50 UTC and 21:41–22:24 UTC). The radiometer-retrieved CWP and DWPac (black and red lines in Fig. 6c and d) follow a similar time evolution. The missing points are times when the passive retrieval failed to converge. It should be noted that, as explained in Sect. 2, the drizzle water path below cloud base derived by the active module is used, together with an initial estimate of total LWP, to estimate the a priori partition between cloud and drizzle water path. During the retrieval process the algorithm adjusts the PWV and total and cloud water path (Cf) to achieve convergence based on the microwave radiometer measurements. During this process both the cloud water and in-cloud drizzle water path are adjusted. Therefore, a correlation between the radar information and the radiometer retrieval is expected. Figure 6 shows that the retrieval process conserves the information provided by the radar, and, while adjusting the total liquid water path to be consistent with the scattering properties of the hydrometeors, it provides final estimates of CWP that are consistent with the radar in-cloud information and with the radar-provided retrievals below cloud base. In the two examples below, the radar and radiometer both show that the CWP component is dominant through the drizzle shaft and the DWPac increases to reach a maximum after about 10 min. The retrieved total LWP in these two drizzle shafts shows that during the times of maximum drizzle development the DWPac reaches at the most 10 %–15 % of the CWP. The quantification of the DWP in relation to the total LWP and CWP is examined in the next section.
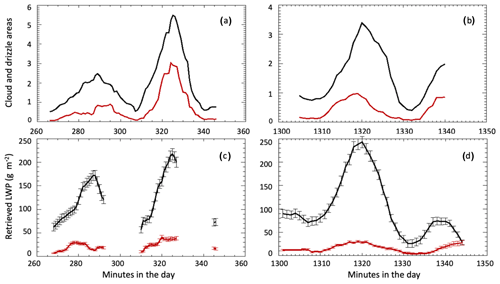
Figure 6Cloud (black) and drizzle (red) areas derived from Doppler spectra during two precipitating shafts at 04:22–05:50 UTC (a) and 21:41–22:24 UTC (b) on 21 November 2016. In the bottom panels corresponding cloud LWP (black) and in-cloud drizzle water path (red) estimated by the passive module are shown for the same drizzle shafts (c, d).
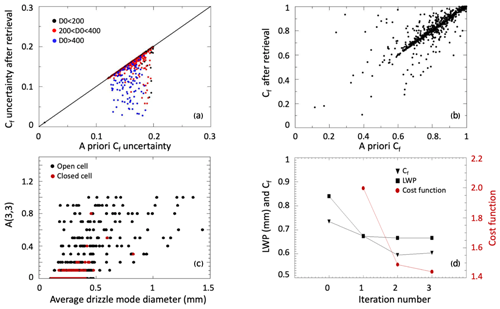
Figure 7Scatter plot between (a) a priori Cf uncertainty and Cf uncertainty after the retrieval. (b) A priori Cf and Cf estimated with the retrieval for samples with LWP greater than 150 g m−2. (c) A(3,3) dependence on the drizzle average mode diameter. (d) Changes in total LWP (black squares), Cf (black triangles), and cost function (red circles) during the convergence process for one retrieval point.
In this section cloud and drizzle water path derived on 10 d each of open cellular and closed cellular stratocumulus cloud conditions observed at the ENA site are analyzed and discussed. The purpose of this section is to evaluate whether the results are consistent with the current state of knowledge of stratocumulus clouds and to provide ideas for possible applications of these results to the study of turbulence, drizzle production, drizzle formation, and cloud–aerosol interaction.
Before proceeding with the details of the drizzle and cloud water path partition, some general features of the retrieval applied to the 10 open-cell cases are shown. The open-cell cases are selected as they contain larger drizzle drops, leading to greater scattering of the microwave signal. However similar conclusions can be drawn for the closed-cell data. Figure 7a shows the reduction in the uncertainty of Cf (ratio of CWP to total LWP) after the retrieval converges. The retrieval has a larger impact in cases where the drizzle diameter below cloud base is larger than 200 µm (Fig. 7a). A Cf value of unity corresponds to no drizzle drops present within the cloud layer, and a value of zero corresponds to absence of any cloud-sized drops in the cloud layer. The final retrieved Cf varies between 0.5 and 1 (no drizzle) and is shown in Fig. 7b vs. the a priori Cf for clouds with LWP greater than 150 g m−2. Collectively Fig. 7a and b demonstrate the reduction in the uncertainty of Cf due to the retrieval process. After the retrieval converges, the averaging kernel matrix A from Eq. (5) in Cadeddu et al. (2017) is related to the independent pieces of information (or degrees of freedom of the system) provided by the measurements. The third diagonal element A(3,3) of the matrix, shown in Fig. 7c, represents the varying contribution of the measurements to the retrieval of Cf. Finally, an example of the convergence process for one retrieval point is shown in Fig. 7d. The retrieval starts with a first guess and adjusts the three retrieved parameters until the convergence criteria specified in Eq. (4) of Cadeddu et al. (2017) are satisfied. The process minimizes a cost function that is monitored at each iteration to ensure proper convergence. The convergence process is very quick and is usually completed after two or three iterations as shown in Fig. 7d.
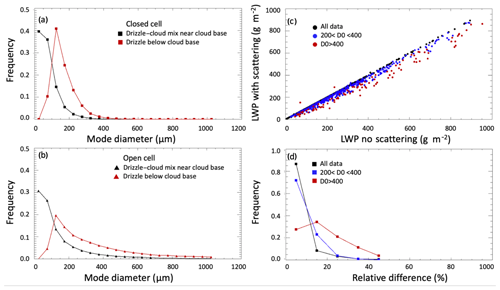
Figure 8Distributions of retrieved mode diameter for closed-cell (a) and open-cell (b) cases. Red symbols represent drizzle below cloud base, and black symbols represent cloud–drizzle mix immediately above the cloud base. (c) Scatterplot of total LWP retrieved with and without scattering effects. (d) Relative difference between total LWP retrieved without and with scattering segregated by below-cloud drizzle mode diameter.
As mentioned in Sect. 2.1, the a priori total liquid water path (LWPt) used to start the convergence process is derived with a neural network algorithm (Cadeddu et al., 2009) with no-scattering assumptions. The present retrieval generally reduces the LWPt with respect to the a priori, and the reduction is more pronounced for cases affected by scattering to a larger extent. However, for a better understanding of the overall impact of the scattering effect on the total LWP, the same retrievals were performed without scattering, assuming that the LWP is distributed entirely in the cloud layer. Figure 8a and b show distributions of the retrieved drizzle mode diameter below (red) and above (black) cloud base for the closed-cell (a) and open-cell (b) cases. In Fig. 8c and d the effect of the drizzle diameter on the retrieved LWP is examined by looking at the relative differences between the LWP retrieved with scattering (LWPsc) and without scattering (LWPnosc). The relative differences in Fig. 8d are computed as . Accounting for scattering effects reduces the total liquid water path by about 8 %–20 % depending on the drizzle diameter. This result provides a quantification of the uncertainty that can be expected from neglecting scattering effects during precipitating conditions. For thicker clouds with , neglecting the scattering effects of drizzle drops when using the 90 GHz channel can potentially lead to an overestimation of LWP by ∼100 g m−2, far higher than the accuracy needed for characterizing the aerosol–cloud interactions.
A summary of the average cloud and drizzle characteristics in the drizzle shafts for open-cell and closed-cell days analyzed is reported in Tables 2 to 5. The cloud optical thickness was broadly estimated assuming a constant cloud drop effective radius of 10 µm using the relation (Painemal and Zuidema, 2011). From Tables 2 and 4 it is evident that in closed cellular stratocumuli 70 %–80 % of the total drizzle is found in the cloud, and less than 30 % of the total drizzle in a shaft falls below the cloud base. In open cellular stratocumuli, on average 30 %–50 % of the total drizzle precipitates with most of it falling below cloud base. The modal drizzle diameter found within the cloud in open cellular stratocumuli is almost twice the modal diameter found in closed cellular stratocumuli. The ratio of below-cloud drizzle drop diameter to in-cloud drizzle drop diameter is ∼2 for open cellular stratocumuli and ∼3 for closed cellular stratocumuli, confirming drizzle being ubiquitous in these clouds with only some of it falling below the cloud base in both mesoscale organizations. There are two main limitations that affect the results shown in Tables 2 and 4. First there is the lack of sensitivity of the microwave channels to drop sizes smaller than ∼100 µm, which increases the uncertainty in the retrieved DWP. This limitation affects both open and closed-cell cases; however as the number of small drops is higher in closed cellular stratocumuli than in open cellular stratocumuli, a larger underestimation of DWP in the cloud can be expected in closed cellular stratocumuli. The second limitation concerns the inability of the microwave radiometer to measure brightness temperatures during intense precipitation due to water deposition on the radome. This will only affect the open-cell cases and will result in an underestimation of the average drizzle shaft DWP in the cloud. As expected, the total LWP is larger in the open-cell cases compared to the closed cell, even accounting for the retrieval underestimation due to lack of convergence during times with the highest precipitation.
Table 3Above- and below-cloud drizzle diameter, cloud-top temperature (CTT), optical depth, and geometrical thickness for open-cell cases.
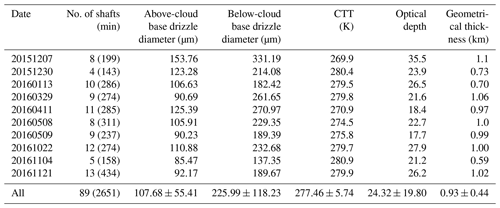
Table 5Above- and below-cloud drizzle diameter, cloud-top temperature, optical depth, and geometrical thickness for closed-cell cases.
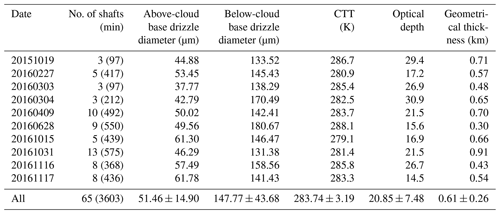
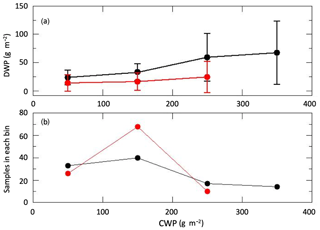
Figure 9(a) Mean and standard deviation of cloud and drizzle water path for open-cell (black) and closed-cell (red) drizzle shafts. (b) Number of samples in each bin for open-cell (black) and closed-cell (red) drizzle shafts.
The co-variability of the total (in-cloud + below-cloud) DWP and the CWP is explored in Fig. 9. Shaft averaged values of DWP and CWP are binned in bins centered at 50, 150, 250, and 350 g m−2 with a width of 100 g m−2. The total (in-cloud + below-cloud) drizzle water path in the shaft is a small fraction (generally less than 30 %) of the CWP and increases with the cloud water path. This behavior is consistent with the findings of Lebsock et al. (2011). The DWP increase is more pronounced in the open-cell (shown in black) than in the closed-cell (shown in red) systems, and for a similar amount of CWP greater amount of drizzle is present in the open cellular drizzle shafts. This is further examined in Fig. 10 where the cumulative distribution of the ratio of precipitating to total drizzle water path in the shaft is shown segregated by the average drizzle diameter at the cloud base. The figure shows that the fraction of drizzle water path leaving the cloud is higher in shafts that, on average, have larger droplets. Virtually all closed-cell cases (blue line) have a drizzle diameter less than 200 µm (GC19) and for 90 % of them the fraction of drizzle water path below the cloud is less than 0.2. In the same range of drizzle diameter, open-cell drizzle shafts (black line) show higher precipitation fraction with 90 % of the shafts having a ratio of below-cloud to total drizzle water path of 0.4 or less. Finally, in 80 % of the drizzle shafts with larger average drop sizes (red line) the ratio of below-cloud to total drizzle water path is 0.6 or less.
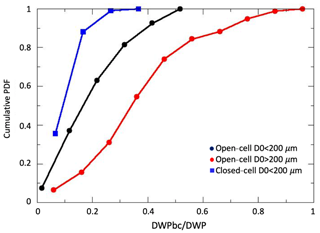
Figure 10(a) Cumulative distribution of below-cloud to total drizzle water path for open and closed-cell cases segregated by drizzle modal diameter (D0) at the cloud base.
The partition of cloud and drizzle water path is also important when studying the relation between turbulence and precipitation. As an example, Fig. 11 shows the total (a), below-cloud-base (b), and above-cloud-base (c) drizzle water binned by the radiative flux divergence at the cloud top and by total LWP for all 1 min averaged closed-cell cases. The figure illustrates the relation between drizzle, LWP, and turbulence. Clouds with strong divergence (less than −100 W m−2) have a high probability of developing drizzle in the cloud when the LWP is above ∼150 g m−2. However, from Fig. 11 precipitation does not develop until the LWP is above ∼200 g m−2. The differences in the values of DWP below and above the cloud base for a similar amount of radiative flux divergence at the cloud top and total LWP suggest drizzle might be present within the cloud before it is detected below the cloud base. In addition, the amount of drizzle water within the cloud is greater than the amount below the cloud base for almost all values of radiative cooling and LWP.
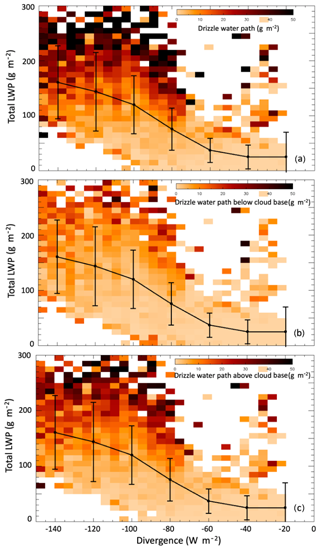
Figure 11(a) Total, (b) below-cloud-base, and (c) in-cloud drizzle water path binned by radiative divergence and total liquid water path. The black circles connected by a solid line represent the total LWP binned by flux divergence and the vertical bars represent the standard deviation of the data in each bin.
In this work Mie scattering by drizzle drops in the microwave spectrum is exploited to partition cloud and drizzle water path using data from active and passive sensors. Brightness temperature observations from a microwave radiometer, profiles of lidar attenuated backscatter, and profiles of the first three moments of the radar Doppler spectra serve as input to the retrieval algorithm. These data together with a radiative transfer code that includes Mie scattering calculations are used to derive parameters of drizzle DSD below the cloud base, total column LWP, and cloud and drizzle water path above the cloud base in marine boundary layer stratocumulus clouds. Due to the lack of coincident observations of in-cloud DWP via aircraft measurements, the retrieved cloud and drizzle water path above the cloud base during 1 d are qualitatively compared with the radar Doppler spectra between cloud base and cloud top. The analysis suggests that the optimal estimation algorithm utilizes the information provided by the radar and ceilometer on the drizzle below the cloud base to adjust the cloud water path and in-cloud drizzle water path to achieve convergence. The converged solution is broadly consistent with the partition between cloud and in-cloud drizzle water path extracted from the radar Doppler spectra.
The retrieval algorithm is applied to 20 d of precipitating stratocumulus cloud conditions at the ARM ENA site. Quantitative analysis of the cloud and drizzle water path during 20 d of precipitating events at the ENA site shows differences between closed- and open-cell scenarios. In the closed-cell systems, only a small fraction (∼20 %) of the available drizzle in the cloud falls below the cloud base compared to the open cell (∼40 %). Precipitation is associated with strong radiative cooling at the cloud top (less than −100 W m−2) and higher liquid water path (higher than 200 g m−2). However, drizzle in the cloud begins to exist at weak radiative cooling (divergence is greater than −80 W m−2) and liquid water path higher than ∼150 g m−2. The amount of available drizzle that falls below the cloud base is higher (30 %–50 %) in open-cell systems than in closed-cell systems and is related to the average drizzle drop size. The average total drizzle water path in open-cell drizzle shafts was fairly high; in all cases analyzed here it was higher than ∼30 g m−2, accounting for at least 20 % of the total liquid water path retrieved by the radiometer. As the algorithm did not converge during the highest precipitating intervals of the open-cell drizzle shafts, it is reasonable to conclude that the estimates provided here are in certain cases an underestimation. Additionally, smaller drizzle drops in the cloud are undetected because their scattering effect is negligible in the microwave, leading to a possible underestimation of the in-cloud DWP even in closed-cell systems.
The technique presented here can be readily applied to derive profiles of drizzle properties below the cloud base, cloud water path, drizzle water path above the cloud base, and total liquid water path under the following conditions, (i) the radar and ceilometer are not severely attenuated by precipitation and are able to adequately detect the cloud base and cloud top, (ii) the radiometer measurements are not affected by precipitation on the radome, (iii) the drizzle droplet diameter is large enough to be detected by the 90 GHz channel, and (iv) the cloud can be considered near adiabatic to assume a priori cloud water content. Only single-layer stratocumulus clouds (closed cell) and precipitating stratocumulus clouds with non-precipitating shallow cumulus below (open cell) were analyzed in this work. However, the technique should be applicable to different atmospheric conditions with observations from aircraft or satellite platforms because the primary limitation in this work is water accumulation on the ground-based radiometer radome.
Our results primarily highlight the need to account for scattering by drizzle drops while retrieving the column amount of liquid water (LWP) from the brightness temperatures observed by high-frequency microwave radiometers. Precipitation is ubiquitous in marine stratocumulus clouds with much of it evaporating before reaching the surface (Zhou et al., 2015; Remillard et al., 2012; Serpetzoglou et al., 2008). The LWP can be inaccurate from traditional (satellite and ground-based) algorithms that neglect the scattering due to drizzle drops for clouds with LWP greater than 500 g m−2. This can lead to inaccurate quantification of adiabaticity (e.g., Kim et al., 2003, 2008), precipitation susceptibility (e.g., Sorooshian et al., 2009), and aerosol–cloud interactions (e.g., McComiskey et al., 2009). LWP is also one of the primary metrics for evaluating single-column model simulations and large-eddy simulation (LES) models in stratocumulus cloud conditions (e.g., Remillard et al., 2017; McGibbon and Bretherton, 2017). The ARM program has had a strong impact on furthering our understanding of aerosol–cloud–precipitation interactions (Feingold and McComiskey, 2016) and on cloud modeling at various scales (Kruger et al., 2016; Randall et al., 2016). Although preliminary, our analyses have an impact on the conclusions of some of the previous studies. Objective quantification of the overestimation of the LWP by the traditional algorithms is warranted and will be the topic of further study.
The ground-based data used in this study were obtained from the Atmospheric Radiation Measurement (ARM) user facility, a U.S. Department of Energy (DOE) Office of Science user facility managed by the Office of Biological and Environmental Research, and are available as follows: KAZR and CEIL data are available from https://www.arm.gov (last access: 27 March 2020), and MWR and SONDE data are available from https://doi.org/10.5439/1182058 (MWR3C, 2019) and https://doi.org/10.5439/1021460 (SONDES, 2012).
MC prepared the manuscript with contributions from all authors. VG preprocessed, cleaned and calibrated the radar and ceilometer data. MC performed the active and passive retrievals. MM contributed to the development and compilation of PAMTRA and provided support of the use of the PAMTRA radiative transfer model.
The authors declare that they have no conflict of interest.
Virendra P. Ghate was supported by the U.S. Department of Energy (DOE) Atmospheric System Research (ASR), Office of Science, Office of Biological and Environmental Research (BER) program, under contract DE-AC02-06CH11357 awarded to Argonne National Laboratory. Maria P. Cadeddu is supported by the U.S. Department of Energy, Office of Science, Office of Biological and Environmental Research, Atmospheric Radiation Measurement infrastructure, under contract no. DE-AC02-06CH11357.
This research has been supported by the U.S. Department of Energy, Office of Science (grant no. DE-AC02-06CH11357).
This paper was edited by Wiebke Frey and reviewed by three anonymous referees.
Acquistapace, C., Loöhnert, U., Maahn, M., and Kollias, P.: A New Criterion to Improve Operational Drizzle Detection with Ground-Based Remote Sensing, J. Atmos. Ocean. Tech., 36, 781–801, https://doi.org/10.1175/JTECH-D-18-0158.1, 2019.
Ahlgrimm, M. and Forbes, R.: Improving the Representation of Low Clouds and Drizzle in the ECMWF Model Based on ARM Observations from the Azores, Mon. Weather Rev., 142, 668–685, https://doi.org/10.1175/MWR-D-13-00153.1, 2014.
Bosisio A., V., Fionda, E., Ciotti, P., and Martellucci, P.: A sky status indicator to detect rain-affected atmospheric thermal emissions observed at ground, IEEE Trans. Geosci. Remote Sens., 51, 9, 4643–4649, 2013.
Cadeddu, M. P., Turner, D. D., and Liljegren, J. C.: A neural network for real-time retrievals of PWV and LWP from arctic millimeter-wave ground-based observations, IEEE Trans. Geosci. Remote Sens., 47, 7, 1887–1900, 2009.
Cadeddu, M. P., Marchand, R., Orlandi, E., Turner, D. D., and Mech, M.: Microwave Passive Ground-Based Retrievals of Cloud and Rain Liquid Water Path in Drizzling Clouds: Challenges and Possibilities, IEEE Trans. Geosci. Remote. Sens., 55, 11, 6468–6481, 2017.
Clothiaux, E. E., Ackerman, T. P., Mace, G. G., Moran, K. P., Marchand, R. T., Miller, M. A., and Martner, B. E.: Objective Determination of Cloud Heights and Radar Reflectivities Using a Combination of Active Remote Sensors at the ARM CART Sites, J. Appl. Meteor., 39, 645–665, https://doi.org/10.1175/1520-0450(2000)039<0645:ODOCHA>2.0.CO;2, 2000.
Clough, S. A., Shephard, M. W., Mlawer, E. J., Delamere, J. S., Iacono, M. J., Cady-Pereira, K., Boukabara, S., and Brown, P. D.: Atmospheric radiative transfer modeling: a summary of the AER codes, J. Quant. Spectrosc. Radiat. Transf., 91, 233–244, 2005.
Evans K. F. and Stephens, G. L.: Microwave radiative transfer through clouds composed of realistically shaped ice crystals. Part II: Remote sensing of ice clouds, J. Atmos. Sci., 52, 2058–2072, 1995.
Feingold, G. and McComiskey, A.: ARM's Aerosol–Cloud–Precipitation Research (Aerosol Indirect Effects), Meteorol. Monogr., 57, 22.1–22.15, https://doi.org/10.1175/AMSMONOGRAPHS-D-15-0022.1, 2016.
Fielding M. D., Chiu, J. C., Hogan, R. J., Feingold, G., Eloranta, E., O'Connor, E. J., and Cadeddu, M. P.: Joint retrievals of cloud and drizzle in marine boundary layer clouds using ground-based radar, lidar and zenith radiances, Atmos. Meas. Tech., 8, 2663–2683, https://doi.org/10.5194/amt-8-2663-2015, 2015.
Frisch, A. S., Fairall, C. W., and Snider, J. B.: Measurement of Stratus Cloud and Drizzle Parameters in ASTEX with a Kα-Band Doppler Radar and a Microwave Radiometer, J. Atmos. Sci., 52, 2788–2799, https://doi.org/10.1175/1520-0469(1995)052<2788:MOSCAD>2.0.CO;2, 1995.
Frisch, S., Shupe, M., Djalalova, I., Feingold, G., and Poellot, M.: The retrieval of stratus cloud droplet effective radius with cloud radars, J. Atmos. Ocean. Tech., 19, 835–842, 2002.
Ghate, V. P. and Cadeddu, M. P.: Drizzle and Turbulence Below Closed Cellular Marine Stratocumulus Clouds, J. Geophys. Res. Atmos., 124, 5724–5737, https://doi.org/10.1029/2018JD030141, 2019.
Gossard, E. E., Strauch, R. G., and Rogers, R. R.: Evolution of dropsize distribution in liquid precipitation observed by ground-based doppler radar, J. Atmos. Oceanic Tech., 7, 815–828, https://doi.org/10.1175/1520-0426(1990)007<0815:EODDIL>2.0.CO;2, 1990.
Han, Y. and Westwater, E. R.: Analysis and Improvement of Tipping Calibration for Ground-Based Microwave Radiometers, IEEE Trans. Geosci. Remote Sens., 38, 1260–1276, 2000.
Hildebrand, P. H. and Sekhon, R. S.: Objective determination of the noise level in Doppler Spectra, J. Appl. Meteor. 13, 808–811, 1974.
Iacono, M. J., Mlawer, E. J., Clough, S. A., and Morcrette, J.-J.: Impact of an improved longwave radiation model, RRTM on the energy budget and thermodynamic properties of the NCAR Community Climate Model, CCM3, J. Geophys. Res., 105, 14873–14890, https://doi.org/10.1029/2000JD900091, 2000.
Jacob, M., Ament, F., Gutleben, M., Konow, H., Mech, M., Wirth, M., and Crewell, S.: Investigating the liquid water path over the tropical Atlantic with synergistic airborne measurements, Atmos. Meas. Tech., 12, 3237–3254, https://doi.org/10.5194/amt-12-3237-2019, 2019.
Kim, B.-G., Schwartz, S. E., Miller, M. A., and Min, Q.: Effective radius of cloud droplets by ground-based remote sensing: Relationship to aerosol, J. Geophys. Res., 108, 4740, https://doi.org/10.1029/2003JD003721, 2003.
Kim, B.-G., Miller, M. A., Schwartz, S. E., Liu, Y., and Min, Q.: The role of adiabaticity in the aerosol first indirect effect, J. Geophys. Res., 113, D05210, https://doi.org/10.1029/2007JD008961, 2008.
Kollias, P., Reímillard, J., Luke, E., and Szyrmer, W.: Cloud radar Doppler spectra in drizzling stratiform clouds: 1. Forward modeling and remote sensing applications, J. Geophys. Res., 116, D13201, https://doi.org/10.1029/2010JD015237, 2011a.
Kollias, P., Szyrmer, W., Reímillard, J., and Luke, E.: Cloud radar Doppler spectra in drizzling stratiform clouds: 2. Observations and microphysical modeling of drizzle evolution, J. Geophys. Res., 116, D13203, https://doi.org/10.1029/2010JD015238, 2011b.
Kotthaus, S., O'Connor, E., Münkel, C., Charlton-Perez, C., Haeffelin, M., Gabey, A. M., and Grimmond, C. S. B.: Recommendations for processing atmospheric attenuated backscatter profiles from Vaisala CL31 ceilometers, Atmos. Meas. Tech., 9, 3769–3791, https://doi.org/10.5194/amt-9-3769-2016, 2016.
Krueger, S. K., Morrison, H., and Fridlind, A. M.: Cloud-Resolving Modeling: ARM and the GCSS Story, AMS Meteorol. Monogr., 57, 25.1–25.16, https://doi.org/10.1175/AMSMONOGRAPHS-D-15-0047.1, 2016.
MWR3C: Atmospheric Radiation Measurement (ARM) user facility, updated hourly. Microwave Radiometer, 3 Channel (MWR3CTIP). 2015-10-01 to 2016-12-31, Eastern North Atlantic (ENA) Graciosa Island, Azores, Portugal (C1), compiled by: Cadeddu, M. and Ghate, V., ARM Data Center, https://doi.org/10.5439/1182058, 2019.
Lebsock, M. D., L'Ecuyer, T. S., and Stephens, G. L.: Detecting the Ratio of Rain and Cloud Water in Low-Latitude Shallow Marine Clouds, J. Appl. Meteor. Clim., 50, 419–432, 2011.
Löhnert, U. and Crewell, S.: Accuracy of cloud liquid water path from ground-based microwave radiometry 1. Dependency on cloud model statistics, Radio Sci., 38, 8041–8051, https://doi.org/10.1029/2002RS002654, 2003.
Luke, E. and Kollias, P.: Separating Cloud and Drizzle Radar Moments during Precipitation Onset Using Doppler Spectra, J. Atmos. Oceanic Tech., 30, 1656–1671, https://doi.org/10.1175/JTECH-D-11-00195.1, 2013.
McComiskey, A., Feingold, G., Frisch, A. S., Turner, D. D., Miller, M. A., Chiu, J. C., Min, Q., and Ogren, J. A.: An assessment of aerosol-cloud interactions in marine stratus clouds based on surface remote sensing, J. Geophys. Res., 114, D09203, https://doi.org/10.1029/2008JD011006, 2009.
McGibbon, J. and Bretheron C. S.: Skill of ship-following large-eddy simulations in reproducing MAGIC observations across the northeast Pacific stratocumulus to cumulus transition, J. Adv. Model. Earth Syst., 9, 810–831, https://doi.org/10.1002/2017MS000924, 2017.
Mech, M., Maahn, M., Kneifel, S., Davide, O., Crewell, S., and Kollias P.: Passive and Active Microwave Transfer (PAMTRA), 9th Workshop of the International Precipitation Working Group, Seoul, South Korea, 5–9 November, p. 2.18, 2018.
O'Connor, E. J., Hogan, R. J., and Illingworth, A. J.: Retrieving Stratocumulus Drizzle Parameters Using Doppler Radar and Lidar, J. Appl. Meteorol., 44, 14–27, 2005.
Painemal, D. and Zuidema, P.: Assessment of MODIS cloud effective radius and optical thickness retrievals over the Southeast Pacific with VOCALS_Rex in situ measurements, J. Geophys. Res., 116, D24206, https://doi.org/10.1029/2011JD016155, 2011.
Randall, D. A., Del Genio, A. D., Donner, L. J., Collins, W. D., and Klein, S. A.: The Impact of ARM on Climate Modeling, AMS Meteorol. Monogr., 57, 26.1–26.16, https://doi.org/10.1175/AMSMONOGRAPHS-D-15-0050.1, 2016.
Rémillard, J., Kollias, P., Luke, E., and Wood, R.: Marine Boundary Layer Cloud Observations in the Azores, J. Climate, 25, 7381–7398, https://doi.org/10.1175/JCLI-D-11-00610.1, 2012.
Rémillard, J., Fridlind, A. M., Ackerman, A. S., Tselioudis, G., Kollias, P., Mechem, D. B., Chandler, H. E., Luke, E., Wood, R., Witte, M. K., and Ayers, J. K.: Use of cloud radar Doppler spectra to evaluate stratocumulus drizzle size distributions in large-eddy simulations with size-resolved microphysics, J. Appl. Meteorol. Climatol., 56, 3263–3283, https://doi.org/10.1175/JAMC-D-17-0100.1, 2017.
Serpetzoglou, E., Albrecht, B. A., Kollias, P., and Fairall, C. W.: Boundary Layer, Cloud, and Drizzle Variability in the Southeast Pacific Stratocumulus Regime, J. Climate, 21, 6191–6214, https://doi.org/10.1175/2008JCLI2186.1, 2008.
SONDES: Atmospheric Radiation Measurement (ARM) user facility, updated hourly. Balloon-Borne Sounding System (SONDEWNPN). 2015-10-01 to 2016-12-31, Eastern North Atlantic (ENA) Graciosa Island, Azores, Portugal (C1), compiled by: Holdridge, D., Kyrouac, J., and Keeler, E., ARM Data Center, https://doi.org/10.5439/1021460, 2012.
Sorooshian, A., Feingold, G., Lebsock, M. D., Jiang, H., and Stephens G. L.: On the precipitation susceptibility of clouds to aerosol perturbations, Geophys. Res. Lett., 36, L13803, https://doi.org/10.1029/2009GL038993, 2009.
Turner, D. D., Clough, S. A., Liljegren, J. C., Clothiaux, E. E., Cady-Pereira, K., and Gaustad, K. L.: Retrieving liquid water path and precipitable water vapor from Atmospheric Radiation Measurement (ARM) microwave radiometers, IEEE Trans. Geosci. Remote Sens., 45, 3680–3690, https://doi.org/10.1109/TGRS.2007.903703, 2007.
Wall, C., Marchand, R., Zhao, W., and Cadeddu, M. P.: An Assessment of rain “contamination” in ARM two-channel microwave radiometer measurements, ASR PI meeting, Tysons, VA, 13–17 March, 2017.
Wang, H. and Feingold, G.: Modeling Mesoscale Cellular Structures and Drizzle in Marine Stratocumulus. Part I: Impact of Drizzle on the Formation and Evolution of Open Cells, J. Atmos. Sci., 66, 3237-3256, https://doi.org/10.1175/2009JAS3022.1, 2009.
Wood, R.: Drizzle in Stratiform Boundary Layer Clouds. Part I: Vertical and Horizontal Structure, J. Atmos. Sci., 62, 3011–3033, 2005.
Wood, R.: Stratocumulus Clouds, Mon. Weather. Rev., 40, 2373–2423, https://doi.org/10.1175/MWR-D-11-00121.1, 2012.
Wood, R., Comstock, K. K., Bretherton, C. S., Cornish, C., Tomlinson, J., Collins, D. R., and Fairall, C.: Open cellular structure in marine stratocumulus sheets, J. Geophys. Res., 113, D12207, https://doi.org/10.1029/2007JD009371, 2008.
Wood, R., Wyant, M., Bretherton, C. S., Rémillard, J., Kollias, P., Fletcher, J., Stemmler, J., De Szoeke, S., Yuter, S., Miller, M., Mechem, D., Tselioudis, G., Chiu, J. C., Mann, J. A. L., O'Connor, E. J., Hogan, R. J., Dong, X., Miller, M., Ghate, V., Jefferson, A., Min, Q., Minnis, P., Palikonda, R., Albrecht, B., Luke, E., Hannay, C., and Lin, Y.: Clouds, aerosols, and precipitation in the marine boundary layer. An ARM Mobile Facility Deployment, B. Am. Meteorol. Soc., 96, 419–440, 2015.
Yamaguchi, T., Feingold, G., and Kazil, J.: Stratocumulus to Cumulus Transition by Drizzle, J. Adv. Model. Earth Syst., 9, 2333–2349, https://doi.org/10.1002/2017MS001104, 2017.
Zheng, X., Klein, S. A., Ma, H.-Y., Caldwell, P., Larson, V.E., Gettelman, A., and Bogenschutz, P.: A cloudy planetary boundary layer oscillation arising from the coupling of turbulence with precipitation in climate simulations, J. Adv. Model. Earth Syst., 9, 1973–1993, https://doi.org/10.1002/2017MS000993, 2017.
Zhou, X., Kollias, P., and Lewis, E. R.: Clouds, Precipitation, and Marine Boundary Layer Structure during the MAGIC Field Campaign, J. Climate, 28, 2420–2442, https://doi.org/10.1175/JCLI-D-14-00320.1, 2015.
Zuidema, P., Westwater, E. R., Fairall, C., and Hazen, D.: Ship-based liquid water path estimates in marine stratocumulus, J. Geophys. Res., 110, D20206, https://doi.org/10.1029/2005JD005833, 2005.