the Creative Commons Attribution 4.0 License.
the Creative Commons Attribution 4.0 License.
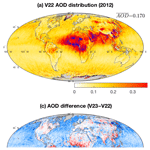
Introducing the 4.4 km spatial resolution Multi-Angle Imaging SpectroRadiometer (MISR) aerosol product
Michael J. Garay
Marcin L. Witek
Ralph A. Kahn
Felix C. Seidel
James A. Limbacher
Michael A. Bull
David J. Diner
Earl G. Hansen
Olga V. Kalashnikova
Huikyo Lee
Abigail M. Nastan
The Multi-angle Imaging SpectroRadiometer (MISR) instrument has been operational on the National Aeronautics and Space Administration (NASA) Earth Observing System (EOS) Terra satellite since early 2000, creating an extensive data set of global Earth observations. Here we introduce the latest version of the MISR aerosol products. The level 2 (swath) product, which is reported on a 4.4 km spatial grid, is designated as version 23 (V23) and contains retrieved aerosol optical depth (AOD) and aerosol particle property information derived from MISR's multi-angle observations over both land and water. The changes from the previous version of the algorithm (V22) have significant impacts on the data product and its interpretation. The V23 data set is created from two separate retrieval algorithms that are applied over dark water and land surfaces, respectively. Besides increasing the horizontal resolution to 4.4 km compared with the coarser 17.6 m resolution in V22 and streamlining the format and content, the V23 product has added geolocation information, pixel-level uncertainty estimates, and improved cloud screening. MISR data can be obtained from the NASA Langley Research Center Atmospheric Science Data Center at https://eosweb.larc.nasa.gov/project/misr/misr_table (last access: 11 October 2019). The version number for the V23 level 2 aerosol product is F13_0023. The level 3 (gridded) aerosol product is still reported at spatial resolution with results aggregated from the higher-resolution level 2 data. The format and content at level 3 have also been updated to reflect the changes made at level 2. The level 3 product associated with the V23 level 2 product version is designated as F15_0032. Both the level 2 and level 3 products are now provided in NetCDF format.
- Article
(11486 KB) - Full-text XML
- BibTeX
- EndNote
Atmospheric aerosols play important roles in the weather and climate of Earth. They affect the global and local energy budgets by scattering and absorbing solar radiation, impact the hydrological cycle through their influence on clouds and precipitation, and can have adverse effects on regional air quality and human health (e.g., IPCC, 2013; Kahn, 2012; Lelieveld et al., 2015). The National Aeronautics and Space Administration (NASA) launched the Terra satellite into a near-polar orbit in December 1999 as the flagship mission of the Earth Observing System (EOS) to measure key parameters – including aerosol amount and properties – that describe the state of the Earth system (Kaufman et al., 1998). As part of this enterprise, the Multi-angle Imaging SpectroRadiometer (MISR) instrument on Terra has now acquired more than 19 years of global observations using nine push broom cameras that image the Earth in four spectral bands (three visible and one near-infrared) across a common 380 km swath, with spatial resolutions ranging from 275 m to 1.1 km, depending on the band and camera (Diner et al., 1998).
Historically, the MISR investigation has provided opportunities for the development of new algorithms to retrieve aerosol properties over both land and ocean using multi-angle observations (Diner and Martonchik, 1985; Gordon, 1997; Kahn et al., 1997, 1998, 2001; Martonchik, 1997; Martonchik and Diner, 1992). These algorithms have been refined into an operational aerosol product that has been used in a variety of global and regional studies (e.g., Alfaro-Contreras et al., 2017; Dey and Di Girolamo, 2010; Guo et al., 2013; Li et al., 2013; Liu et al., 2007; Scollo et al., 2012; Tosca et al., 2017; Witek et al., 2016; Zhang and Reid, 2010; Zhao et al., 2017; a complete bibliography may be found at https://misr.jpl.nasa.gov/publications/peerReviewed/index.cfm, last access: 27 January 2020). Since launch, there have been over 800 MISR-related publications pertaining to aerosol studies from the scientific community, with more than 200 of these papers related to air quality and human health.
The operational retrievals developed by the MISR aerosol team have been through a number of iterations as the strengths and weaknesses of different approaches have become apparent (e.g., Diner et al., 2005; Kahn et al., 2005). Until recently, the last significant update to the MISR operational aerosol retrieval algorithms, designated as version 22 (V22), occurred in December 2007. Different aerosol retrieval algorithms are applied over land and over dark water. For V22, these two algorithms are described in detail in Martonchik et al. (2009) and Kalashnikova et al. (2013), respectively. Aerosol optical depth (AOD) and retrieved particle properties for V22 have been globally validated, to an extent that is practical, and a number of strengths, as well as shortcomings, have been identified (Kahn et al., 2010; Kahn and Gaitley, 2015; Shi et al., 2014; Witek et al., 2013). Comparisons have also been made with aerosol retrievals from other instruments, especially the Moderate Resolution Imaging Spectroradiometer (MODIS), which accompanies MISR on the Terra satellite (Kahn et al., 2007, 2009b; Shi et al., 2011; Zhang and Reid, 2010). Work with the independent MISR research algorithm (RA) has provided additional important insights (Kahn et al., 2001; Limbacher and Kahn, 2014, 2015, 2017, 2019). These studies, along with other more focused investigations described in greater detail below, as well as experience with the V22 aerosol data set, have motivated the development, testing, and release of an updated version of the MISR operational aerosol product, designated as version 23 (V23), which became publicly available in November 2017. The V23 aerosol retrieval algorithms have been applied to all past MISR data through reprocessing and are used in the current forward data processing as new data are acquired. This ensures that a consistent data set is available for the entire mission.
The MISR V23 aerosol product incorporates significant updates to the format, content, and underlying retrieval algorithms. These changes were made to simplify interpretation of the product and address several of the quality issues identified in the V22 products. The purpose of this paper is to introduce the user community to the MISR V23 aerosol algorithms and associated level 2 and level 3 products. We detail the changes made from V22 to V23, discuss the motivations for these changes, and show how they affect the behavior of the aerosol retrievals at regional and global scales. We focus primarily on highlighting key differences between the V23 product and its V22 predecessor. A preliminary validation against surface-based observations is also included.
The paper is organized as follows. Section 2 reviews the MISR aerosol retrieval approach and the performance of the V22 product. In Sect. 3 we discuss the approach the MISR aerosol team took toward the development of V23. Section 4 contains a description of the changes that affect the MISR level 2 (swath) aerosol product, including overall changes and changes that specifically affect retrievals over water and land. Key changes that affect the level 3 (globally gridded) product are described in Sect. 5. Section 6 evaluates V23 products relative to V22 retrievals, as well as observations from the AErosol RObotic NETwork (AERONET, Holben et al., 1998) and the associated Maritime Aerosol Network (MAN, Smirnov et al., 2011). Section 7 provides a summary and conclusions. Appendix A lists the parameter fields, also known as scientific data sets (SDSs), in V23 and contrasts them with those used in V22.
This section provides an overview of the MISR data products and introduces the terminology used to describe them. The basic concepts used in aerosol retrievals for both water and land surfaces are discussed, along with evaluations of the performance of the legacy V22 aerosol product.
2.1 MISR terminology
The MISR instrument flies aboard the NASA EOS Terra satellite at an orbital altitude of 705 km with an inclination of 98.2∘ and an orbital period of 99 min. The orbit of the satellite is sun-synchronous, crossing the Equator at 10:30 local time (LT) on its descending node (satellite motion from north to south on the sunlit side). Terra makes 14.56 orbits per day, and the ground-track pattern repeats every 16 d. As with data from the U.S. Geological Survey (USGS) and the NASA Landsat satellite, MISR data products are referenced to a set of 233 fixed ground tracks called “paths” defined by the second Worldwide Reference System (WRS-2) (Irons et al., 2012). The daylit portion of the Earth observed by MISR during each Terra orbit is assigned a single, unique, constantly incrementing “orbit” identifier and an associated WRS-2 path ranging from 1 to 233 (Diner et al., 1998).
The MISR instrument consists of nine push broom cameras oriented along the direction of satellite motion, with nominal view angles relative to the Earth's surface of , , , , and 0∘ (Diner et al., 1998). The forward-looking cameras are designated as Df, Cf, Bf, and Af, in order of decreasing view angle. The 0∘ (nadir) view is obtained with the An camera, and the aft-looking cameras are designated as Aa, Ba, Ca, and Da, in order of increasing view angle. This sequence from Df to Da is also the temporal order of image acquisition for any viewed location, and a point on the ground is imaged by all nine cameras over a time span of approximately 7 min. The MISR cameras make observations in four spectral bands: blue (446.6 nm), green (557.5 nm), red (671.7 nm), and near-infrared (866.4 nm) (Kahn et al., 2007). The An camera has the narrowest swath at 380 km, and imagery from this camera is reported at full resolution (275 m) in all four spectral bands. The red-band imagery in all the off-nadir cameras is also reported at 275 m, whereas the other 24 off-nadir bands are averaged onboard to 1.1 km resolution during standard, global-mode operation (Diner et al., 1998).
Raw data from the MISR instrument, which require detailed engineering information to interpret, are designated as level 0 and are not generally distributed except within the science data-processing stream (Bothwell et al., 2002). The level 0 files are reformatted into level 1A Hierarchical Data Format for the Earth Observing System (HDF-EOS) files that utilize the now legacy HDF4 data structure. Radiometric scaling and conditioning are applied to the L1A files to generate a set of nine L1B1 files, one for each MISR camera. Next, geometric rectification is performed and all nine cameras are registered to a common projection on a Space-Oblique Mercator (SOM) grid referenced to the World Geodetic System 1984 (WGS84) ellipsoid or the MISR digital elevation model (DEM) to produce L1B2 HDF-EOS data files (Diner et al., 1998; Jovanovic et al., 2007). The L1B2 files, one for each camera and nine per orbit, are designated as either “Ellipsoid” if projected to the WGS84 ellipsoid, or “Terrain” if projected to the DEM. MISR data products are made available to the public through the NASA Langley Research Center (LaRC) Atmospheric Science Data Center (ASDC) at https://eosweb.larc.nasa.gov/project/misr/misr_table (last access: 27 January 2020). The L1B2 data also serve as the primary input to the level 2 (L2) science algorithms, which report results on the MISR swath using the SOM projection. The L2 data are then statistically aggregated into global grids on time intervals of days, months, seasons, or years, to generate level 3 (L3) data products. In the case of the MISR aerosol products, the resolution of the L3 grid is 0.5∘ latitude by 0.5∘ longitude. The MISR L2 and L3 aerosol products, along with additional documentation that describes the MISR data products and processing stream in more detail, are available from the ASDC at the link provided above.
The L2 aerosol retrievals utilize a number of ancillary inputs in addition to the measured L1B2 radiances. The Ancillary Radiometric Product (ARP) preflight calibration (ARP_PRFLTCAL) file contains information on the standardized, response-weighted, extraterrestrial solar irradiances for each of the MISR spectral bands, as well as the spectral out-of-band correction matrix that is used to correct for out-of-band instrument response (Bruegge et al., 2004). The Ancillary Geographic Product (AGP) provides the latitude and longitude of each MISR pixel on a 1.1 km SOM grid. The AGP files also contain the MISR DEM surface elevations and surface feature identifiers used to discriminate land and water (Nelson et al., 2013). Camera-viewing zenith and azimuth angles are obtained from the geometric parameters (GP_GMP) product (Bothwell et al., 2002; Nelson et al., 2013). Finally, the Terrestrial Atmosphere and Surface Climatology (TASC) data set provides monthly values of surface pressure, ozone, water vapor, snow and ice cover, and near-surface wind speed on a global 1∘ by 1∘ grid (Kahn et al., 2007). Besides these ancillary data sets, the L2 aerosol retrievals depend on the output of three MISR clouds masks: the Radiometric Camera-by-camera Cloud Mask (RCCM, Yang et al., 2007; Zhao and Girolamo, 2004), the Stereoscopically Derived Cloud Mask (SDCM, Diner et al., 1998), and the Angular Signature Cloud Mask (ASCM, Di Girolamo and Wilson, 2003), which are all reported on a 1.1 km resolution SOM grid. Additional inputs to the aerosol retrievals include: (i) the aerosol configuration file, which sets the values of various thresholds; (ii) the Aerosol Climatology Product (ACP) Aerosol Physical and Optical Properties (APOP) that contains the pre-calculated scattering properties of the aerosol optical models used in the retrievals; (iii) the ACP mixture file that describes how these “pure” aerosol components are mixed; and (iv) the Simulated MISR Ancillary Radiative Transfer (SMART) file that contains pre-calculated results from a forward radiative transfer (RT) model run for each of the aerosol optical models for the MISR wavelengths and possible viewing geometries stored in a set of lookup tables (LUTs).
In order to accommodate the temporal dependence of the TASC and RCCM ancillary data sets, which depend on updated current monthly and seasonal climatologies, respectively, the MISR data-processing stream is split into “first look” and “final”. First look products are typically available to the public within 24 h of instrument acquisition. This is accomplished by making use of ancillary data for the same month or season from the previous year. Once the updated ancillary data sets become available, usually within 3 to 6 months, final processing is performed. The first look products have “first look” included in the filename – “MISR_AM1_AS_AEROSOL_FIRSTLOOK_∗” – whereas the final products do not have this designation and appear as “MISR_AM1_AS_AEROSOL_∗”. Within the NASA LaRC ASDC file system, the L2 MISR aerosol products are designated as MIL2ASAF for first look and MIL2ASAE for final. L3 aerosol products are only generated once the L2 final data products are available. These are designated as MIL3DAEN (daily), MIL3MAEN (monthly), MIL3QAEN (quarterly/seasonal), and MIL3YAEN (yearly). Information about the MISR data products can be found at https://eosweb.larc.nasa.gov/project/misr/misr_table (last access: 27 January 2020). The contents of the data files are explained in the data product specifications (DPS) document, available at https://eosweb.larc.nasa.gov/project/misr/dps (last access: 27 January 2020). Scientific users of MISR data are strongly encouraged to consult the Data Quality Statement (DQS) for each of the MISR data products, which can be found at: https://eosweb.larc.nasa.gov/project/misr/quality_summaries/misr_qual_stmts_current (last access: 27 January 2020).
2.2 Basic concepts of the MISR aerosol retrieval algorithms
The problem of retrieving aerosol properties from satellite remote sensing over surfaces ranging from dark oceans to bright deserts is extremely challenging. The operational MISR aerosol retrieval algorithms build upon many years of work done prior to and after the launch of the Terra satellite. Except where noted, the basic assumptions and design features underlying the MISR aerosol retrieval algorithms are largely unchanged in V23 compared to previous versions, and these can be enumerated as follows (Martonchik et al., 1998, 2009).
-
Aerosols are assumed to be horizontally homogenous over the spatial scale of the retrieval, meaning that the different atmospheric path lengths observed from different angles sample the same aerosol and that the aerosol amount along each path varies only due to differences in geometric path length.
-
Retrievals are performed by comparing the satellite observations with a set of pre-calculated RT model results generated for a range of naturally occurring aerosol types, which allows the retrievals to be computationally efficient; however, no geographic or seasonal constraints are applied to the types of aerosols considered.
-
RT calculations assume a plane-parallel atmosphere and neglect three-dimensional effects. A scalar RT is employed.
-
Aerosol types are assumed to be externally mixed.
-
A statistical formalism that explicitly accounts for estimated measurement uncertainty is used to assess the agreement between the observations and the models.
-
For dark water retrievals, the water-leaving radiance is assumed to be negligible in the red and near-infrared wavelengths, and the forward RT model calculations explicitly account for specular reflection and whitecaps due to near-surface winds. The water-leaving radiance assumption is updated for V23, as described in Sect. 4.2.6.
-
For land surfaces that are spatially heterogeneous, no prior assumptions are made about the surface bi-directional reflectance factors (BRFs) except that their normalized angular shapes are spectrally similar, and their contributions to the top-of-atmosphere (TOA) radiances can be parameterized as a sum of empirical orthogonal functions (EOFs) derived from the MISR observations themselves.
-
Retrievals are not performed for topographically complex surfaces (e.g., mountains), shallow (potentially turbid) water, cloudy, or sun-glint-contaminated regions.
Following this philosophy, the operational aerosol products are generated using two separate algorithms: dark water (DW), applied to regions identified in the MISR AGP as ocean or deep inland water, and heterogeneous surface (Het Surf), applied to regions containing any land. Internal to the Het Surf algorithm is the application of the angular spectral similarity constraint, referred to the as the homogeneous surface (Homog Surf) algorithm (Diner et al., 2005). The operational V22 algorithms are described in greater detail in Martonchik et al. (2009) for land and Kalashnikova et al. (2013) for water.
2.3 Evaluation of the V22 MISR aerosol product
An extensive history of validation studies predates the MISR V22 aerosol product, setting the stage for subsequent assessments (e.g., Abdou et al., 2005; Diner et al., 2001; Kahn et al., 2004, 2005b, 2007; Kinne et al., 2006; Liu et al., 2004; Martonchik et al., 2004; Myhre et al., 2005; Reidmiller et al., 2006; Russell et al., 2007; Schmid et al., 2003; Xiao et al., 2009). The MISR V22 aerosol product was analyzed in detail, mainly between 2008 and 2017, when it represented the operational version of the retrieval algorithm. Several groups performed AOD comparisons among MISR, MODIS, and other satellite instruments, as well as against AERONET and MAN surface-based sun photometer measurements (e.g., Cheng et al., 2012; Kahn et al., 2009, 2010, 2011; Li et al., 2009; Liu and Mishchenko, 2008; Mishchenko et al., 2010; Petrenko and Ichoku, 2013; Shi et al., 2011, 2014; Smirnov et al., 2011). Additional studies compared MISR AOD against coincident aircraft data obtained in field campaigns (e.g., Kahn et al., 2004, 2009a) or model simulations (e.g., Chin et al., 2014). Some studies compared the reported spectral AOD dependence, represented by the Ångström exponent (AE), and a few examined other MISR-retrieved particle properties (e.g., Eck et al., 2013; Kahn and Gaitley, 2015). A brief indication of the scope of these comparisons, the underlying principles applied, and the resulting assessment of the MISR V22 aerosol product strengths and limitations is presented in this section.
Mid-visible AOD is by far the most commonly validated of the MISR-retrieved aerosol quantities, largely due to the simplicity of this single variable, its relevance to almost all climate- and air-quality-related questions, and the broad availability of independent data for comparison. The need was recognized early on for both an absolute comparison criterion, to adequately represent the lower bound on AOD measurement sensitivity, and a relative criterion, to capture the uncertainty at higher AOD that tends to scale with the magnitude of the AOD itself. As such, statistical assessments were performed in several studies comparing MISR and collocated AERONET sun photometer measurements using the percent of MISR observations falling within envelopes representing the larger of 0.05 or 20 % of the AOD and 0.03 or 10 % of the AOD, where the AERONET values were taken as “ground truth”. The latter envelope, the larger of 0.03 or 10 % of the AOD, represents the target requirement for AOD defined by the World Meteorological Organization's Global Climate Observing System. In some studies, the data were first stratified by expected aerosol type. For example, Kahn et al. (2010) concluded that for the MISR V22 product, using over 5000 coincidences, about 70 % to 75 % of the MISR AOD retrievals fell within the larger of 0.05 or 20 % of the AOD of the paired validation data from AERONET, and about 50 % to 55 % were within the stricter limits of 0.03 or 10 % of the AERONET AOD, except at sites where dust is commonly found. Based on the stratification by aerosol type for different AERONET sites, maritime-dominated sites offered the highest agreement between MISR and AERONET AODs, whereas sites where mixtures of smoke and dust occurred frequently had the poorest agreement, highlighting a lack of smoke–dust mixtures in the MISR aerosol climatology (Kahn et al., 2009b, 2010).
Most studies have concluded that the MISR-retrieved AODs are more accurate over brighter land surfaces than those from single-view satellite instruments (e.g., Petrenko and Ichoku, 2013). Other studies have identified the effects of cloud contamination, such as Li et al. (2009) and Shi et al. (2014), which emphasizes the importance of cloud masking in the aerosol retrieval process.
In making MISR–AERONET comparisons, AOD spatial and temporal variability is also a factor, due to the differences in sampling between the two measurement types. One approach to addressing this issue is to vary the MISR spatial averaging and/or the AERONET temporal averaging window so the two methods sample roughly similar aerosol air masses (e.g., Petrenko et al., 2012). In one study, MISR ∼20 km and ∼50 km windows were applied to near-coincident AERONET observations averaged over ±1 h (Kahn et al., 2010). For maritime, continental, biomass burning, and dusty sites, the larger spatial averaging produced ∼2 % to ∼7 % better agreement. However, for urban areas, where AOD varies on relatively short spatial scales, agreement was ∼5 % better with smaller averaging areas. As such, even with high-quality validation data, one must account for differences in sampling when assessing agreement. Further, regarding aerosol spatial variability, the 17.6 km resolution of the V22 retrievals lacks spatial detail, especially near aerosol source regions and wherever aerosol amount or types vary rapidly. In such regions, the coarse spatial resolution of the 17.6 km V22 product averages out the AOD peaks and valleys that appear when retrieval results are reported at higher resolution.
A summary of the issues with the V22 AOD product based on extensive validation work is given in Kahn et al. (2010). These include quantization effects apparent in the distribution of reported AODs, a gap in the retrieved AOD values between 0.00 and 0.02, a lack of several aerosol component optical analogues and mixtures in the algorithm climatology that are common in the atmosphere, and a systematic underestimation of the AOD for mid-visible AOD values above about 0.4 that is related at least in part to surface boundary conditions. Part of the motivation for the development of the MISR V23 aerosol product was to address several of these issues, while other issues are being explored with the MISR research algorithm (e.g., Limbacher and Kahn, 2019).
Obtaining validation data for aerosol information beyond AOD, such as aerosol particle properties, is more challenging, in part because both ground-based and aircraft validation data for MISR are very sparse and because remote sensing sensitivity to particle properties is much more dependent than AOD on retrieval conditions. Nevertheless, an analysis by Kahn and Gaitley (2015) of retrieval constraints on particle size, shape, and single scattering albedo (SSA) largely confirmed the results of pre- and post-launch theoretical sensitivity studies (e.g., Kahn et al., 1997, 1998, 2001; Kalashnikova and Kahn, 2006). A primary conclusion is that aerosol type discrimination increases greatly when the mid-visible AOD exceeds about 0.15 or 0.20. Particle property retrieval results are semiquantitative; under good retrieval conditions three to five size bins, two to four bins in SSA, and spherical vs. randomly oriented nonspherical particles can be identified. Where the AOD is sufficiently high and the expected particle types are included in the MISR algorithm climatology, MISR fine-mode fraction and AE match those of near-coincident AERONET retrievals. In a detailed study of smoke particles at a site in southern Africa, Eck et al. (2013) showed that the MISR-retrieved AOD matched the AERONET-observed seasonal trend, indicating that the MISR results correctly capture the seasonal change in SSA. Other individual case studies indicate good discrimination between nonspherical dust and spherical particles in field observations (e.g., Kahn et al., 2009a).
Production of the MISR V22 aerosol product at the NASA LaRC ASDC began on 1 December 2007 (see https://eosweb.larc.nasa.gov/project/misr/version/pge9, last access: 27 January 2020). The L2 swath-based product, designated as F12_0022, is provided in HDF-EOS format, based on the HDF4 data structure. The gridded L3 product, designated as F15_0031, derived from the V22 L2 data, is provided on a 0.5∘ latitude–longitude grid in HDF4 format. Discussions with the research community led to the subsequent development of an additional version of the L3 product in NetCDF-3 format, designated as F08_0031, with a .nc file extension, in contrast to the .hdf file extension used for the other MISR products.
As discussed in the previous section, evaluation of the V22 aerosol product has demonstrated that the reported AODs agree well with AERONET, and the aerosol particle properties show semiquantitative agreement with climatological expectations and available comparison data sets (Kahn et al., 2010; Kahn and Gaitley, 2015). The V22 aerosol product has been used in a variety of regional and global studies, as described in the Introduction, and has been assimilated in the NASA Modern-Era Retrospective analysis for Research and Applications, version 2 (MERRA-2), over bright surfaces (albedo >0.15) for the time period from January 2001 through June 2014 (Randles et al., 2017). The Naval Research Laboratory has also included MISR AOD information in their 11-year global aerosol reanalysis product (Lynch et al., 2016).
The primary motivations for the development of a new version of the MISR aerosol product were threefold: (i) to increase the product resolution from 17.6 to 4.4 km in order to satisfy a growing demand for higher-resolution AOD retrievals in the aerosol and public health scientific communities, (ii) to address a number of issues in MISR aerosol retrievals that were identified over the past several years, and (iii) to make the product easier to use. The first objective therefore was to deliver a product with higher spatial resolution and with quality and accuracy that was as good as or better than V22. Achieving this objective was not as straightforward as simply running the retrieval algorithm 16 times within a footprint of the V22 retrieval: a number of algorithmic issues with V22 retrievals become substantially magnified when retrievals are run at a higher resolution, and considerable effort was required to mitigate them. A notable example is cloud screening in DW retrievals: the original version proved less effective at 4.4 km resolution and had to be supported by additional screening methods not present in V22 (Witek et al., 2018b).
The second objective of the product upgrade was to address several shortcomings in MISR V22 retrievals, which were initially identified by Kahn et al. (2010) and later confirmed in a number of other studies. Most importantly, the efforts focused on mitigating the existence of a gap in retrieved AOD values below about 0.02. Tackling the quantization noise at low AOD mentioned in Kahn et al. (2010) was only part of the solution; addressing the gap problem required identification and development of a method for correcting stray light in MISR cameras (Witek et al., 2018a). In addition, substantial work was devoted to reengineering the retrieval process to make the utilization of goodness-of-fit functions in DW processing less threshold dependent. As demonstrated in Sect. 6, implementation of the revised algorithmic approaches has resulted in improved performance of the MISR retrievals.
The MISR aerosol team's third objective was to make the MISR aerosol products easier to use. The V23 aerosol product is distributed in NetCDF-4 format, as opposed to the HDF4 format used for previous product versions. A number of changes were also made to the individual fields in the product, including the introduction of latitude and longitude fields, the removal of the “stacked-block” format, and a shift of the reference AOD to the 550 nm wavelength from 558 nm. These changes are described in more detail in the next section and additional information can be found in Appendix A.
Some remaining issues pertaining to MISR aerosol retrievals were not addressed during development of the V23 product. Those include most aspects related to the suggested limitations of the set of mixtures currently included in the retrieval process (Kahn et al., 2010; Kahn and Gaitley, 2015). Over land, the retrieval processing is largely unchanged except for some simplifications and threshold adjustments (described in Sect. 4.3), and the observed performance improvements stem mainly from better characterization of surface-reflected signals in the retrieval process.
4.1 Changes affecting both ocean and land retrievals
4.1.1 Resolution change
MISR aerosol retrievals are reported on a regularly spaced 2-D grid aligned with the axes of the SOM map projection. The area of each grid cell is called a “region”, with spacing between grid centers defined by the resolution of the product. Regions are further divided into equally spaced 1.1 km subregions, corresponding to individual “pixels” in the radiance and cloud masking inputs. With the transition from V22 to V23, the regional resolution was increased from 17.6 to 4.4 km, thereby reducing the number of subregions per region from 256 (16×16) to 16 (4×4).
The change in resolution has consequences for the thresholds used to determine the regions suitable for aerosol retrievals. The algorithm performs a series of tests to identify subregions that are suitable for use in the retrieval. Since these are primarily focused on removing possible cloud contamination, subregions passing all the tests are designated as “clear”. Aerosol retrievals are only attempted where the number of clear subregions is greater than a configurable threshold. In V22, the threshold was 32 (of 256) for DW retrievals and 16 (of 256) for Het Surf retrievals. In V23, these thresholds have been modified to 2 (of 16) for both DW and Het Surf retrievals. Note that the percentage of required clear subregions is kept consistent for DW retrievals in both V22 and V23 at 12.5 %, but the percentage was doubled for Het Surf. Requiring a higher percentage of clear pixels for land retrievals decreases potential cloud contamination compared to V22.
The risk of cloud contamination is also increased for retrievals over water, where the DW algorithm always chooses the single darkest clear subregion to represent the aerosol properties for the region (Kahn et al., 2007; Kalashnikova et al., 2013). For 17.6 km resolution regions, the selected subregion can be physically farther from cloud fields than any subregion at 4.4 km resolution. More precisely, the single, darkest subregion selected from a 17.6 km region will always be equivalent to the darkest subregion for a single region out of the 16 coincident 4.4 km regions, while the other 15 subregions must be equivalent or brighter than the darkest subregion. During development, the effect of the resolution change on cloud contamination artifacts in the 4.4 km retrievals was immediately apparent and algorithm changes designed to mitigate the risk of cloud contamination are described in Sect. 4.1.3 and 4.2.4.
4.1.2 Veiling light correction
The MISR push broom cameras are subject to internal reflections and light scattering from optical elements that contribute to the emergence of structured and stray light in the recorded signals. These low-radiance-level effects are usually negligible and are typically well below the calibration requirements of the MISR instrument. However, artifacts become noticeable in contrast-enhanced images of dark regions adjacent to bright areas, e.g., when dark ocean is surrounded by bright clouds or sea ice. Structured, out of focus “ghosts” were first reported in MISR images by Bruegge et al. (2002, 2004). Later studies analyzing collocated radiances from the MISR and MODIS instruments further confirmed low-radiance biases in MISR data (Limbacher and Kahn, 2015; Witek et al., 2018a). Other studies revealed that MISR-retrieved AODs were systematically overestimated in pristine oceanic regions (e.g., Kahn et al., 2005a, 2010; Limbacher and Kahn, 2014; Witek et al., 2013) and stray light in the MISR cameras was identified as the main contributor to this bias (Limbacher and Kahn, 2015; Witek et al., 2018a).
To mitigate the ghosting and stray light effects in the MISR cameras, a simple correction model was developed and applied in the V23 processing of the MISR radiances (Witek et al., 2018a). The model assumes that the stray light is uniformly distributed across the field of view (referred to as veiling light), rather than taking the form of structured ghosts. As a result, the correction model depends on only two factors: (1) the average brightness of the scene and (2) an empirically determined set of coefficients for each MISR camera and spectral band. The empirical coefficients were first determined using various methods and then tested in prototype AOD retrievals. The set of coefficients that led to the best overall agreement between the prototype retrievals and the surface-based observations from MAN was chosen for implementation in the V23 operational retrieval algorithm. The simple linear model derived in this fashion has been shown to be highly effective in mitigating the high AOD biases in the MISR retrievals relative to MAN measurements on a statistical basis (Witek et al., 2018a).
It should be noted that the veiling light correction model implemented in the MISR V23 aerosol retrieval algorithm is only a first-order approximation to the complex processes that lead to the emergence of structured ghosts and other manifestations of stray light in the MISR cameras. For example, Limbacher and Kahn (2015) employed a multiple parameter approach to model these structured ghosts. A more sophisticated correction approach based on ray tracing within the MISR camera optics is currently being developed by the MISR team. This model will be used for reprocessing the MISR level 1B2 radiance data and will replace the current veiling light model that is only applied to the level 2 aerosol retrievals.
4.1.3 Cloud screening
The resolution increase from 17.6 km in V22 to 4.4 km in V23 guarantees that some retrievals are now performed closer to the edges of clouds, which in turn increases the probability of cloud contamination in those retrievals. Cloud-contaminated, high AOD retrievals in often pristine regions of the world were also apparent in the V22 aerosol product, highlighting existing deficiencies in the cloud clearing methods employed in MISR data-processing (e.g., Li et al., 2009; Shi et al., 2014). Detection of optically thin clouds (e.g., thin cirrus) is particularly challenging. Witek et al. (2013) examined the impact of cloud contamination on the accuracy of retrieved AODs in the MISR V22 aerosol product. They found that the agreement between MISR retrievals and ground-based observations from MAN and AERONET networks can be statistically improved by additionally screening MISR retrievals using a clear flag fraction (CFF) parameter. CFF is a measure of how many 1.1 km subregions and camera views within the retrieval region are designated by the algorithm as clear and likely to be suitable for an aerosol retrieval. The CFF parameter is a ratio of the number of clear observations to the total number of observations within the retrieval region ( in V22). The recommended CFF screening threshold to improve the quality of the V22 AOD retrievals was about 0.6, which reduced MISR biases over dark water by a factor of 0.02, as compared to surface-based observations.
In the V23 algorithm, an approach similar to the CFF-based screening is employed operationally for both DW and Het Surf regions to eliminate retrievals that could be contaminated by clouds. A few modifications were introduced relative to the original methodology described by Witek et al. (2013). First, the definition of the screening parameter was changed slightly to account for details in how subregions are tallied. For the 4.4 km V23 product, each retrieval region contains subregion and camera observations that are initially classified according to their suitability for use in an aerosol retrieval. These classifications include “cloudy”, “clear”, or “glitter contaminated”, among others. The V23 algorithm uses a “cloud screening parameter” (CSP), which is defined as the fraction of the 144 observations classified as clear relative to the number of observations considered available for aerosol processing. Observations in sun glint, for example, are not considered available for aerosol processing, which means that the denominator in the CSP can be less than 144. On average, the value of the CSP was found to be slightly greater than the value determined using the CFF parameter due to this change. A second adjustment modifies the value of the threshold to account for the change in the product resolution and a reduction in the maximum number of available observations. Third, in order to further improve the effectiveness of the cloud-screening in V23, another parameter derived from the CSP but which allows for an extended characterization of cloudiness around the retrieval region was developed. This new parameter is called the cloud screening parameter neighbor 3×3 (CSP_3×3) and is calculated as the average of the CSP over the box of 3×3 neighboring regions centered on the retrieval region. The two parameters, CSP and CSP_3×3, are used jointly to screen MISR V23 aerosol retrievals that are considered to have increased probability of being cloud-contaminated. Thresholds on these parameters were determined through statistical analysis of retrievals (results not shown) and are set to CSPthresh=0.7 and CSP_. When data from year 2007 were analyzed, the additional cloud screening using both parameters with the stated thresholds resulted in 19.5 % of retrievals being eliminated. Because the thresholds chosen for operational processing are somewhat arbitrary, a considerable number of potentially high-quality retrievals may be inadvertently lost. To address this, data without additional screening applied is reported in the product in the “AUXILIARY” group, designated by the “_raw” postfix. These data are provided to allow interested users to employ their own cloud screening approaches if they so desire.
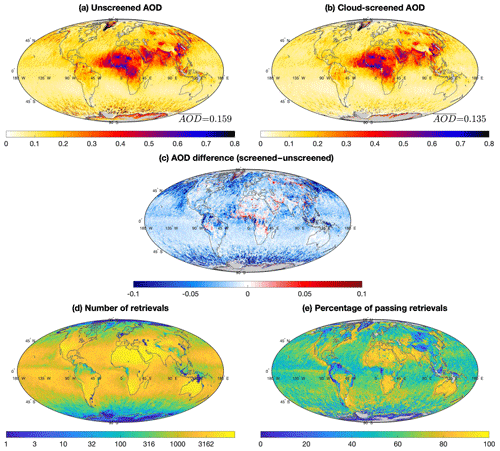
Figure 1Effects of additional cloud screening implemented operationally in V23 shown using 0.5∘ gridded retrievals from 2012: (a) global AOD distribution prior to additional cloud screening, (b) global AOD distribution with operational cloud screening, (c) AOD difference between the screened and unscreened data;, (d) number of retrievals (unscreened) in each grid box, and (d) percentage of retrievals remaining after cloud screening. Average AOD values in (a) and (b) are area-weighted.
Figure 1 shows the effects of operational cloud screening on the global AOD distribution and coverage using MISR V23 retrievals from 2012. The unscreened data (Fig. 1a) shows enhanced AOD values over the Southern Ocean and in high-latitude oceanic regions of the Northern Hemisphere. These likely cloud-contamination issues are substantially reduced after the additional cloud screening is employed (Fig. 1b). There are noticeable AOD reductions almost everywhere over oceans and largely over land, as seen in Fig. 1c. The global area-weighted average AOD decreases from 0.159 to 0.135, or by about 15 %. There are, however, some areas where the average AOD increases after cloud screening, a result that serves as a reminder that the screening procedure can also eliminate low AOD retrievals in addition to cloud-contaminated high AODs. The total number of AOD retrievals in 2012 data set is about 340 million, as compared to the about 227 million that pass the screening procedure, a removal rate of about 33 %. The percentage of passing retrievals (Fig. 1e) correlates well with the climatological distribution of cloudiness. Continents, especially Africa and Australia, generally have a higher retention of retrievals, whereas the screening rates over oceans are usually above 20 % due to higher cloudiness. Even though, on average, only 67 % of retrievals remain after the cloud screening procedure, the number of retrievals in V23 is substantially larger than in V22 due to increased resolution and other algorithmic changes. Differences in coverage and number of retrievals between V23 and V22 are analyzed in Sect. 6.2.1.
4.1.4 Updates to product format and content
A number of file format changes were made to the V23 level 2 and level 3 products, with the overall goal of making MISR data-handling significantly easier for existing and new users. The most significant change is that the V23 level 2 aerosol products are now distributed in NetCDF-4 format. This change was made from the HDF4 format used in previous versions of the product to take advantage of the additional tools and packages available to work with NetCDF-4 files. NetCDF-4 is completely interoperable with HDF5, so any tool that can handle one format can handle the other. For example, the MISR V23 aerosol products can easily be read and visualized using the Panoply tool developed and maintained by Robert B. Schmunk at NASA Goddard Institute for Space Studies (GISS) (see https://www.giss.nasa.gov/tools/panoply/, last access: 27 January 2020).
A second major format change was the removal of the stacked-block format used in previous product versions and other MISR data products (Bothwell et al., 2002). The stacked-block format breaks up the spatial extent of each product into three dimensions: block (1–180), SOM x coordinate, and SOM y coordinate. This approach was originally developed to help fit the data from complete MISR orbits into the NASA HDF-EOS convention. However, the stacked-block format introduces additional complexity when working with the product, especially if the area of interest spans multiple blocks. The V23 products replace the three dimensions of the stacked-block format with a simpler, two-dimensional format: SOM x coordinate and SOM y coordinate. To further facilitate use, 2-D latitude, longitude, and time fields have been added to the product, so detailed knowledge of the SOM projection is no longer required (Jovanovic et al., 2002).
The structure of the V23 files has also been changed relative to the V22 files, with the goal of making the data sets easier to find and use. The V22 HDF4 files had eight top-level “directories”, with the relevant science data sets scattered across seven of these. In the V23 NetCDF-4 file, the number of top-level directories has been reduced to three, with all the science data contained in one. The most commonly used geophysical parameters can be found within the main science directory “4.4_KM_PRODUCTS”. Additional fields of interest for experienced users are located within the AUXILIARY group.
The field names have been changed to be more intuitive and to increase compatibility with other NASA satellite aerosol products. As a prime example, the AOD field in the MISR V22 product was named “RegBestEstimateSpectralOptDepth” and contained AODs retrieved in each of the four MISR spectral bands. However, most users are interested in the mid-visible MISR green band at 557.5 nm for comparisons with AERONET and other satellite data products like MODIS (e.g., Kahn et al., 2009b, 2010). In the V23 product, the primary quality-screened AOD field is named “Aerosol_Optical_Depth” and is reported at 550 nm to make it compatible with MODIS (Levy et al., 2013). AOD conversion between wavelengths is facilitated thanks to a reported field “Spectral_AOD_Scaling_Coeff”, which provides coefficients of a second-order polynomial fit to the spectral AODs. Overall, 88 fields present in V22 that were either redundant, confusing, or rarely used, have been eliminated in V23. Several new fields relevant to the V23 algorithm were added.
Additionally, the NetCDF attributes have been populated with information helpful to product users, including field descriptions, fill values, and units, hopefully reducing the need for users to consult the Data Product Specifications (DPS) document, which has also been updated and is available at https://eosweb.larc.nasa.gov/project/misr/dps/specific_products (last access: 27 January 2020). For the benefit of users transitioning from V22 to V23, a table mapping the fields from the old to the new version is provided in Appendix A.
4.2 Changes for dark water
4.2.1 AOD retrieval
A new approach to retrieving AODs over dark water was introduced in the V23 aerosol processing. The procedure is described in detail in Witek et al. (2018b). Key features of the new approach are summarized briefly here. Some important differences between the previous V22 and the new V23 version are highlighted.
The DW aerosol retrieval calculates a goodness-of-fit metric, , between the pre-calculated top-of-atmosphere (TOA) radiances and the MISR observations for a range of AODs (for 0.0 to 3.0 in the V23 LUT) and for each of the 74 aerosol mixtures in the LUT. , calculated as a function of MISR's green-band AOD (τ), is expressed as
In Eq. (1), ρMISR represents MISR equivalent reflectances, ρm represents modeled TOA equivalent reflectances for a given aerosol mixture, and σabs represents the absolute equivalent reflectance uncertainties in ρMISR, calculated as
where ΔρMISR is the minimum equivalent reflectance uncertainty, or the so-called “radiometric floor”. The summation index l is over the four MISR wavelengths and j is over the nine MISR cameras. The parameters ν(l,j) and wl are weights that depend on the wavelength and the availability of data (for details, see e.g., Kalashnikova et al., 2013). For example, wl are always equal to 1 for the MISR near-infrared (NIR) and red spectral channels but vary between 0 and 1 for the green and blue bands depending on AOD. Different AOD-dependent wl weights allow us to mitigate the non-negligible contribution from the water-leaving radiance to the TOA signal at shorter wavelengths.
In V22, the range of absolute equivalent reflectance uncertainty, σabs, was constrained by ΔρMISR, which was set to a value of 0.04, meant to represent the assumed accuracy of measured equivalent reflectances. In scenes where observed reflectances are very low, however, this limit was found to negatively affect the calculated values and limited the algorithm's sensitivity to retrieving low values of AOD. For that reason, in V23 the ΔρMISR is set to a very small value of 0.0001, which effectively eliminates the radiometric floor from σabs calculations. This modification improved the sensitivity of the algorithm to the angular and spectral information content of MISR observations.
In V22, the best-fitting value of τmix for each mixture, was taken to be the value that minimizes using a parabolic fitting approach applied to the values determined on a fine grid of optical depths (Diner et al., 2008). Furthermore, additional parameters were used to determine the goodness of fit of the particular aerosol mixture to the MISR data. Those parameters, , , and (for definitions, see e.g., Diner et al., 2008 of Kalashnikova et al., 2013), were calculated at the previously obtained value of τmix. An aerosol mixture was considered “successful” if all four metrics, , , , and , did not exceed certain empirically established thresholds (Witek et al., 2018b). The AODs of the successful mixtures were then averaged and reported as the “best estimate” AOD in the product.
In V23, a substantially different approach to determining AOD in DW retrievals was designed and implemented (Witek et al., 2018b). The new approach relies solely on the metric; the other three goodness-of-fit parameters are no longer used. Instead, the entire range of cost function values, for green-band AOD ranging from 0.0 to 3.0, is used for determining the retrieved AODs. The cost function values are first inverted to maximize the contribution of the best-fitting models, then averaged over all N=74 aerosol models, resulting in a combined goodness-of-fit distribution function that only depends on AOD, and is expressed as
The location of the peak of the resulting distribution f(τ) is reported in the V23 product as the retrieved AOD. Furthermore, the reported AOD is interpolated to 550 nm wavelength in order to standardize it and facilitate comparisons with other satellite products. The width of the combined distribution is proportional to the reported AOD uncertainty and calculated as the full width at half maximum divided by a scaling factor of , assuming a normal distribution of f(τ) (Witek et al., 2018b). Key benefits of this approach are that both the AOD and the AOD uncertainty are retrieved simultaneously from the same distribution, and all aerosol mixtures participate in the AOD determination so that no empirical thresholds are required to determine the “success” or “failure” of a particular mixture, as was done in the V22 algorithm (e.g., Kalashnikova et al., 2013).
The same ensemble-based approach is also applied to the characterization of AOD spectral dependence. For each of the MISR aerosol mixtures, the cost functions are initially derived with respect to the MISR green-band wavelength. The cost functions are then scaled to the three other MISR nominal wavelengths using the spectral dependence associated with individual aerosol models. The resulting cost functions are then inverted and averaged, similar to the procedure described above, at each of the MISR wavelengths, resulting in a total of four goodness-of-fit distribution functions. The peaks of these functions discretely characterize the retrieved spectral dependence of AOD. To further aid product users, a least-squares second-order polynomial fit is applied to the four spectral AODs and the resulting coefficients of the polynomial are reported in the product in the field “Spectral_AOD_Scaling_Coeff”. These can be used to calculate the retrieved AOD at any wavelength within the spectral range 400 to 900 nm. The scaling coefficients are also employed to calculate the Ångström exponent reported in the product, which is calculated using the AODs at 550 and 860 nm wavelengths.
4.2.2 Determination of particle properties
The ensemble-based approach in V23 DW retrievals has so far been applied to the derivation of AOD, AOD uncertainty, and the spectral AOD dependence, including the reported AE. However, other aerosol optical and microphysical properties, such as single scattering albedo or effective radius, are calculated in V23 using the same methodology employed in V22. In V23 the reported particle properties correspond to the best-fitting aerosol mixture, which is determined using two goodness-of-fit metrics, and . Furthermore, to maintain a level of consistency with V22, thresholds on these two metrics are preserved in order to assure that a particular mixture fits the observations sufficiently well. The particle properties corresponding to this mixture are reported in the product only if the and are below their respective thresholds, which are set to the same values as in V22. The particle properties are set to fill values if these conditions are not satisfied. This sometimes leads to a situation when a valid AOD retrieval does not have any associated particle properties; however, such scenarios are not very common, affecting less than 7 % of all 2012 DW AOD retrievals. Also, sensitivity to AOD is retained in the multi-angle data, particularly at mid-visible AOD values below about 0.15, even when sensitivity to particle microphysical properties is reduced (Kahn and Gaitley, 2015). The retrieved particle properties are converted to their corresponding fractional optical depths at 550 nm and reported in the product as absorption AOD; nonspherical AOD; and small-, medium-, and large-mode AOD. Reporting of these results as fractional AODs is done in order to facilitate aggregating and averaging of the data, which is often performed by the data users.
4.2.3 Per-pixel AOD uncertainty
As mentioned in the previous section, the V23 DW algorithm utilizes a combined goodness-of-fit distribution function f(τ) to determine the AOD, as well as the uncertainty associated with the retrieved AOD. In principle, the approach to calculating the AOD uncertainties combines elements of the optimal estimation technique and the ensemble technique for error estimation. The reported AOD uncertainty depends on a combination of factors, such as the absolute values of the cost functions for each aerosol mixture, the widths of the distribution of the cost functions as a function of AOD, and the spread of the cost function distributions among the ensemble of mixtures. The initial evaluation of the V23 AOD uncertainties showed a more reasonable statistical behavior compared to the uncertainties obtained in V22 (Witek et al., 2018b). The lack of independent metrics, however, makes it very difficult to assess retrieval uncertainties in a direct quantitative way (Povey and Grainger, 2015).
In a following study, Witek et al. (2019) performed a detailed statistical analysis of V23 AOD uncertainties using collocated MISR retrievals and ground-based AOD observations. They found that the reported AOD uncertainties exhibit characteristics similar to the standard error of a Gaussian distribution, suggesting that reported retrieval uncertainties approximate the standard retrieval error reasonably well. This feature is of great importance in data assimilation applications, where each geophysical retrieval needs to be accompanied by its associated uncertainty. In their study, they also examined possible dependencies between the AOD uncertainties and the aerosol properties reported in the product (Witek et al., 2019). For a given AOD, the AOD uncertainties are generally above average when absorbing or small-mode-dominated aerosols are retrieved and generally below average when nonspherical, medium-, or coarse-mode aerosols are reported. This result gives additional insight into the microphysical prescription of mixtures being considered in the MISR retrieval algorithm.
4.2.4 Aerosol retrieval confidence index (ARCI) for additional cloud screening
The combined goodness-of-fit distribution function f(τ) (Eq. 3), besides allowing a simultaneous determination of the AOD and its uncertainty, is further utilized to screen retrievals that may be of low quality. The value of the peak of the overall distribution function f(τ), which is called the “aerosol retrieval confidence index” or ARCI (see Fig. 1c in Witek et al., 2018b), represents the overall agreement between the MISR observations and the aerosol models in the LUT. A large ARCI indicates that some aerosol models had sufficiently small values that the confidence in the retrieval is high. Conversely, a low ARCI means that generally high values were obtained and that most aerosol models in the LUT fit the MISR observations poorly. Statistical analysis of the AODs and the ARCIs showed that an ARCI threshold of 0.15 is very effective at screening retrievals that are likely contaminated by clouds (Witek et al., 2018b). This is important, as most of the empirical thresholds used in the V22 DW algorithm to screen erroneous retrievals were eliminated in V23. Additionally, the higher-resolution V23 retrievals come much closer to cloud edges and therefore require more sensitive tests to eliminate the potential impacts of clouds.
4.2.5 Using glint pattern to retrieve wind speed
Specular reflection can produce artifacts in the aerosol retrievals over an otherwise dark ocean surface. As such, the MISR V23 DW aerosol retrieval algorithm excludes observations from cameras that have view angles within 40∘ of the direction of specular reflection, which is called the “glitter angle.” Camera views excluded by this glitter threshold are indicated at 17.6 km resolution in the Geometric Parameters File (GMP). Although studies using the MISR research algorithm have demonstrated that this 40∘ cutoff is overly conservative and that observations down to a glitter angle of 10∘ can be included by the addition of a (glitter-angle-dependent) weighting factor and glint uncertainty into the total TOA reflectance uncertainty budget (Limbacher and Kahn, 2017, 2019), in the operational V23 product the 40∘ glitter angle exclusion – which is also used in previous versions of the DW retrieval algorithm – is retained.
As described by Cox and Munk (1954), the peak surface reflectivity decreases, and the angular width of the glitter pattern increases systematically with wind speed. Given the range of view angles observed by the MISR instrument, it is possible to constrain the wind speed from the MISR data itself in some situations. For example, Fox et al. (2007) investigated in detail the degree to which the nine MISR view angles capture the wind-speed-related glitter pattern under a range of wind speeds and observing geometries. This work formed the basis of a simplified wind speed retrieval introduced in the new version of the aerosol product.
In V23, the modeled TOA reflectance, ρm, used for (Eq. 1) is pre-calculated at three discrete wind speeds: ws = 2, 5, and 7.5 m s−1. At locations where no MISR channels are within the 40∘ glitter angle range, the algorithm selects the wind speed nearest to the monthly wind speed climatology from the TASC data set. Otherwise, where at least one MISR channel is within the 40∘ glitter angle range, the algorithm selects the wind speed with the minimum equivalent reflectance difference, Δ(ws,τ), between the model (ρm) and the observation (ρMISR), expressed as
where v(l,j) is 1 for channels within 40∘ glitter angle range and 0 elsewhere. Note that the wind speed selected may be different for each aerosol optical model. The best estimate of wind speed reported in the V23 product is the wind speed selected for the aerosol optical model with the best fit according to and metrics.
4.2.6 Underlight correction
In the first order, the reflection of sunlight off the ocean surface can be accounted for by considering only the effects of sun glint and whitecaps. However, research initially performed by Kahn et al. (2005a) showed that ocean color (i.e., water body reflectance, or underlight) impacts could be non-negligible for aerosol retrievals using MISR radiances. These effects were first assessed in Limbacher and Kahn (2014). In that work, accounting for water body reflectance resulted in an AOD bias reduction of 0.005 relative to AERONET and MAN measurements, with about 5 % more over-ocean retrievals falling within the expected error envelope of the greater of 0.05 or 20 % of the AERONET or MAN AOD. Counterintuitively, the impact of underlight becomes more important as AOD increases, because only the red and NIR bands are used in the MISR DW retrievals if the AOD is less than 0.50. At AODs greater than 0.50 for the green band, green-band observations are included in the retrieval with a weight that increases linearly with AOD up to 1.0, where the weight becomes unity. Similarly, blue-band observations are included for AODs in that band greater than 0.75, weighted linearly up to an AOD of 1.50 where the weight becomes unity. Because the spectral water body reflectance decreases systematically with increasing wavelength, aerosol retrievals that include the green and blue bands are especially sensitive to ocean color. This sensitivity is the basis for the MISR research algorithm chlorophyll a retrievals described in Limbacher and Kahn (2017). The MISR DW V23 operational retrieval algorithm uses the underlight model introduced by Kahn et al. (2005a) and Limbacher and Kahn (2014). It is assumed that the ocean color can be adequately modeled as a Lambertian reflector with surface albedos of , , , and for the blue through the NIR bands, respectively.
4.3 Changes for land
4.3.1 Threshold changes
The MISR aerosol algorithm defines a minimum surface albedo contribution to be added to the modeled path radiance for establishing an AOD upper bound. The albedo contribution at a given location is set according to a configurable LUT indexed by seven distinct AGP surface types: deep ocean, deep inland water, shallow ocean, coastline, shallow inland water, ephemeral water, and land. For surface types corresponding to land or near land (including shallow water and coastlines), the albedo contribution in V22 is set to a constant value of 0.015. At locations with naturally dark surfaces (e.g., inland lakes, dark forests), the V22 algorithm was found to frequently fail due to the AOD upper bound being returned as negative, indicating a surface reflectance lower than that predicted by the constant albedo offset. To address this problem, this surface albedo offset was set to zero for all surface types.
The Het Surf algorithm requires observations from a prescribed minimum number of clear subregions viewed in common by at least five of the MISR cameras, including a mix of both near-nadir and oblique view angles. The V22 algorithm for deciding when this condition was satisfied was found to prematurely reject regions with substantial topographic obscuration, specifically due to a lack of commonly viewed 1.1 km subregions in the oblique views from opposing cameras. For example, in areas with mountainous terrain, locations viewed by the Da camera are more likely to be obscured in the Df camera view and vice versa. In such cases, simply dropping the problematic views may recover enough commonly viewed subregions to allow the retrieval to proceed. The V23 algorithm implements this latter approach and, instead of enforcing the logic used in V22, performs a comprehensive search over all possible valid camera combinations to meet the new requirement of only four valid camera views. Camera views beyond the nadir swath edge are excluded, but the An camera is not explicitly required for the V23 retrieval to proceed. Note, however, that the angular correlation test, which is used to ensure that the spatial distribution of radiance within a retrieval region is similar across view angles (Diner et al., 2008), requires at least one of the A cameras (Af, An, or Aa).
The Het Surf algorithm evaluates goodness-of-fit for each aerosol mixture based on the AOD uncertainty and the metric. Only those mixtures with both AOD uncertainty and at or below their respective thresholds are considered successful. The AOD uncertainty threshold is set to 0.1. The threshold is set to the smaller of two components: (1) an absolute threshold set in the configuration file or (2) a relative threshold dynamically calculated in the retrieval. The relative threshold is set to 1.5 times the minimum value of for any mixture. In V22, the absolute threshold was set to 4.0. In V23, the absolute threshold is disabled, leaving only the AOD uncertainty and the relative threshold to determine mixture success. The lack of an absolute threshold on substantially increases the number of retrievals over land by allowing successful retrievals even when the absolute fit calculated by may be poor.
4.3.2 AOD uncertainty calculation
The Het Surf algorithm retrieves up to four values of AOD at 550 nm per mixture, each obtained using measurements from different MISR spectral channels. These multiple per-band AOD estimates are then averaged to produce a retrieved AOD per mixture, with an uncertainty equal to the standard deviation of the set of AODs associated with that mixture. The AODs for successful mixtures are then averaged to produce the AOD for the retrieved region. In the V22 algorithm, the AOD uncertainty was calculated using one of two methods. First, where there were multiple successful mixtures, the reported AOD uncertainty was the standard deviation of the per-mixture AODs. Second, if there was only one successful mixture, the AOD uncertainty was the standard deviation of the per-band AODs for that mixture. This inconsistency in the method used to generate the reported AOD uncertainty could make it difficult to compare AOD uncertainties for different locations. To address this issue, the V23 algorithm calculates the AOD uncertainty as the standard deviation of the entire set of AODs associated with different spectral channels over all successful mixtures simultaneously. This provides a consistent approach, even in the case of a single successful mixture, because at least two per-band AOD estimates are always required for a mixture to be considered successful.
4.3.3 Greenland and Antarctica masking
The Het Surf retrieval algorithm does not perform well for homogeneous areas largely covered by snow or ice. To address this, all aerosol retrievals over Greenland and Antarctica are screened and set to a fill value (−9999.0). Locations may also be tagged with a “geographic_exclusion” flag in the Aerosol_Retrieval_Screening_Flags field. Unscreened results can be found in the Aerosol_Optical_Depth_Raw field in the AUXILIARY subgroup, but users are urged to treat these locations with caution.
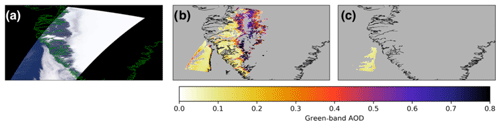
Figure 2MISR V23 aerosol retrievals over Greenland for 15 August 2017 (orbit 93 932, path 006): (a) MISR Ancillary camera RGB, (b) a map of unscreened Aerosol_Optical_Depth_Raw retrievals, and (c) a map of screened Aerosol_Optical_Depth retrievals showing geographic exclusion over land and filtering of lower-quality retrievals over the water.
Figure 2 is an example of the effects of Greenland and Antarctica masking on the reported retrievals. In this case the broad geographic exclusion adopted in V23 appears to have the unintended consequence of removing potentially valid retrievals over snow-free parts of Greenland. However, it also masks clearly erroneous high AOD retrievals over the ice and snow that is often present in these areas. Overall, the benefits of masking outweigh its drawbacks, offering an easy solution until a more sophisticated approach is developed.
5.1 Change inputs and field names to match L2
MISR's level 3 Component Global Aerosol (CGAS) Product provides a summary of the level 2 aerosol retrievals sampled with three different temporal frequencies (daily, monthly, and quarterly) on a latitude–longitude grid. The latest CGAS product (F15_0032) includes distributions of V23 aerosol product at each grid point. There are several changes in the new level 3 product with respect to the previous version (F08_0031) that are worth noting.
-
The latitudes are flipped and the range is from 89.75∘ N to 89.75∘ S.
-
Variable names have been changed to make them consistent with the naming conventions used in V23. For example, “Aerosol_Optical_Depth” in the new version represents “Optical_depth_average” in the previous version.
-
Averages and distributions of AOD, spectral AOD scaling coefficients, AE, and fractional AODs, such as absorbing AOD; small-, medium-, and large-mode AODs; and nonspherical AODs, are provided.
-
All optical depths are now reported at 550 nm wavelength.
-
SSA included in the previous version and calculated from averaged optical depths is now replaced with the absorbing optical depth, which is calculated as AOD × (1-SSA).
-
NetCDF-4 data format replaces the NetCDF-3 format used in the previous version.
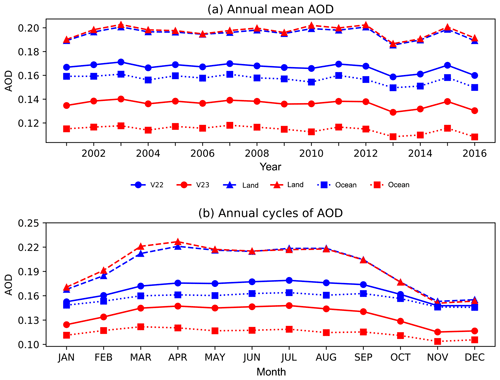
Figure 3(a) Time series of area-weighted annual mean AODs from V22 (blue) and V23 (red) between 2001 and 2016. Sold lines with circles, triangles with dashed lines, and squares with dotted lines represent global mean AOD, AOD averaged over land, and AOD averaged over ocean, respectively. (b) Annual cycles of AOD. AOD for each month is averaged for the 16 years.
5.2 L3 AOD time series comparison
Level 3 products are convenient for analyzing long time series, comparing AODs between different product versions and assuring temporal consistency of the retrieved parameters. In Fig. 3a, we compared 16-year time series of annual mean AOD at 550 nm obtained from V22 and V23 L3 aerosol products. Data from V22 and V23 are shown in blue and red colors, respectively; different markers represent the global average AOD (circles and solid lines), average AOD over land (triangles and dashed lines), and average AOD over oceans (squares and dotted lines). The AODs over land agree very well between V22 and V23 throughout the analyzed time period and the interannual variability is almost the same, confirming the temporal consistency of V23 retrievals. The AODs over ocean, however, are on average 27 % lower in V23 than in V22: the 16-year over-ocean mean AODs are 0.114 and 0.157 in V23 and V22, respectively. The lower AODs in MISR DW retrievals result from radiometric and algorithmic modifications introduced in V23 (Sect. 4.2 above), most notably the veiling light, cloud screening, and underlight corrections. The lower AODs over oceans in V23 are consistent with the level 2 product comparisons presented in Sect. 6.2. Despite the substantial reduction in global average AOD, the interannual variability exhibits similar behavior between the V22 and V23 versions of the aerosol product. The overall conclusions drawn from the time series analysis also hold true when the average seasonal cycle is analyzed (Fig. 3b). Note that the seasonal cycle in global average AOD in both V22 and V23 is mostly driven by AODs over land. The AODs over ocean remain relatively constant throughout the year, with only a small decrease in the winter season. The seasonal cycles in V22 and V23 are generally comparable; one small exception is slightly larger V23 AOD over land between January and May, with the largest difference of 0.009 in March.
As the primary purpose of this paper is to provide an introduction to the algorithmic and format changes contained in the V23 aerosol product, only an initial evaluation of the data has been performed to date. The main results are summarized in this section.
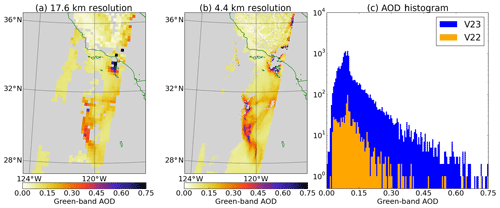
Figure 4Comparison of V22 and V23 AOD retrievals for 23 July 2016 (orbit 88 284): (a) V22 AOD map, (b) V23 AOD map, and (c) AOD histograms.
6.1 Scene comparisons
The impact of the change in retrieval region resolution from 17.6 to 4.4 km can be seen both qualitatively and quantitatively. The qualitative differences are most obvious when comparing scenes containing aerosol plumes or localized sources. Figure 4 gives an example, showing a transported smoke plume from the Soberanes Fire that spread over the Pacific Ocean west of Los Angeles. At 4.4 km resolution (Fig. 4b), the plume is better resolved and its distinct features are clearly visible, and the relationships between more and less optically thick elements along the plume dispersion route are evident. At 17.6 km resolution (Fig. 4a), the plume has a coarser structure and parts of it are absent. The histograms of AOD from V22 and V23 AOD retrievals (Fig. 4c) provide more quantitative insight into differences in the aerosol products. The higher horizontal resolution leads to a more than an order of magnitude larger number of retrievals, so the AOD histogram is smoother and closer to the expected lognormal distribution. In addition, the AOD gradients are better resolved in the V23 product, so the dynamic range of AOD values is greater in the V23 product, especially at the high-AOD end of the distribution.
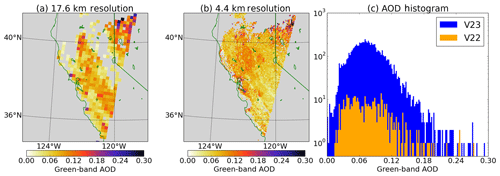
Figure 5Comparison of V22 and V23 AOD retrievals for 5 July 2016 (orbit 88 022): (a) V22 AOD map, (b) V23 AOD map, (c) AOD histograms.
As most of the retrievals shown in Fig. 4 are over dark water, Fig. 5 focuses on a region of northern and central California for which the MISR over-land algorithm was applied. The data shown in Fig. 5 coincides with a field campaign that was carried out in California's San Joaquin Valley in July 2016, with the primary goal of advancing the science needed to support speciated aerosol property retrievals for air quality applications (Kalashnikova et al., 2018). As an important feature needed for a particular matter (PM) characterization with a high-resolution MISR product, we see improvement in coverage in the V23 product for this example (Fig. 5b), especially in the upper-right part of the panel. The area where V23 regained coverage corresponds to the relatively dark Sierra Nevada. This example highlights the importance of algorithmic modifications described in Sect. 4.3.1 that were introduced to land retrievals in V23, specifically removing the minimum surface albedo constraint and improving the common-view camera combination logic. Further, enhanced aerosol optical depth around the San Francisco Bay Area can be easily discerned in Fig. 5b but not in the 17.6 km retrievals in Fig. 5a. Although the accuracy of fine-scale AOD features cannot be independently evaluated in this case, the aerosol spatial variability is consistent with field observations (Kalashnikova et al., 2018), and the V23 product provides the type of high-resolution, large-scale content that is needed for air quality applications. Garay et al. (2017) demonstrated that a prototype version of the V23 4.4 km retrievals agree substantially better with ground-based observations than the previous V22 17.6 km retrievals, in that study MISR retrievals were compared against observations carried out during several AERONET-DRAGON deployments around the globe. This would be expected, especially in places where aerosol amount varies on kilometer spatial scales. The histograms of AOD (Fig. 5c) show that the higher resolution and larger number of retrievals in V23 lead to a less noisy and more lognormally distributed AOD histogram and also better resolves the AOD maxima and minima, similar to the scene analyzed in Fig. 4. Prototype versions of the 4.4 km MISR product have already been used extensively over parts of southern and central California, and the current V23 product has been used over Mongolia to estimate PM with diameter less than 2.5 µm (PM2.5), less than 10 µm (PM10), and speciated PM2.5 concentrations (Franklin et al., 2017, 2018b; Meng et al., 2018). The 4.4 km MISR AOD product was proven, through cross-validation against surface monitoring stations, to capture PM2.5 variably on a 4.4 km scale and to separate PM2.5 and PM10 size modes (Franklin et al., 2018a). Ongoing studies are extending the MISR 4.4 km product application for predicting spatially resolved PM types to other highly polluted regions.
6.2 Global comparisons between V23 and V22
In this section we analyze the overall differences in aerosol loading and aerosol properties between the new V23 product and its V22 predecessor. In order to do that, MISR level 2 aerosol retrievals from 2012 are gridded at 0.5∘ resolution and annually averaged within each grid box for each of the MISR product versions. This procedure differs from using level 3 and averaging it over a period of 12 months in that only one temporal averaging is applied to the data. The choice of 2012 stems from the fact that in this year MISR instrument had relatively few missing orbits and that the Oceanic Niño Index was neutral. Unless stated otherwise, all retrieved aerosol properties are analyzed at the 550 nm wavelength.
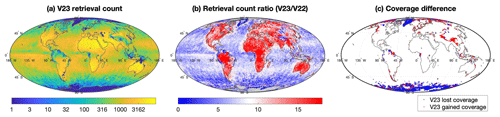
Figure 6(a) Number of MISR V23 retrievals from 2012, gridded at 0.5∘ resolution, the color scale is presented in decimal logarithm. (b) Ratio between the number of V23 retrievals and V22 retrievals; the average ratio, represented by white color in the color scale, is ∼9.0. (c) Locations of grid points where V23 loses (blue) or gains (red) coverage in comparison to V22.
6.2.1 Coverage comparison
Figure 6 analyzes the retrieval count from the 2012 gridded V23 product, the retrieval count ratio between V23 and V22, and the areas where V23 gains or loses coverage with respect to V22. The average ratio in the number of retrievals between V23 and V22 is about 9.0, but the ratio distribution is not uniform around the world (Fig. 6b). In an ideal scenario where both V22 and V23 have full coverage, the retrieval number ratio should be 16 because of the increased spatial resolution in V23. In practice, however, the ratio can be much larger than 16 (i.e., there is an increase in coverage) or, as is mostly the case over oceans, smaller than 16, depending on multiple factors such as cloudiness and retrieval success rates in the V22 and V23 algorithms. Although the color scale in Fig. 6b does not go beyond the value of 18, ∼6 % of grid points exceed this ratio and ∼0.2 % of grid points have ratios higher than 100. The ratios are generally higher over land (red colors), except in areas where the retrieval count in V23 is relatively low. The high ratios over land, indicating increased retrieval success rate in V23, are attributed to the algorithmic changes described in Sect. 4.3.1. The retrieval count ratios over oceans, on the other hand, are generally below 9 (blue colors in Fig. 6b). Part of this land–ocean difference is attributed to cloudiness, which is generally lower over land during the midmorning Terra overpasses. In addition, the underlying algorithmic difference in retrievals over land and ocean is a key contributor. Over oceans, a retrieval is always performed on an individual 1.1 km pixel regardless of the resolution. Setting radiometric and algorithmic differences between V22 and V23 aside, if there are 16 valid V23 retrievals in a 17.6 km region, the DW success criterion implies that the same region also has to have a valid V22 retrieval, setting the upper limit on the ratio to 16. The same constraint does not apply to retrievals over land, there could be multiple V23 but zero successful V22 retrievals within the same region. This example partially explains why the ratios over oceans are generally below 16, considerably smaller than those over land. Figure 6c highlights 0.5∘ grid points for which V23 gains (red) or loses (blue) coverage in comparison to V22. The coverage is mainly lost over Greenland and Antarctica due to the retrieval masking described in Sect. 4.3.3. The coverage gains are found in areas with dark vegetation and more complex topography due to modifications described in Sect. 4.3.1.
6.2.2 Aerosol optical depth (AOD) and AOD uncertainty (UNC) comparison
The global distribution of AOD from V22 and V23 for 2012 are shown in Fig. 7a and b, respectively. The V22 AODs are scaled to 550 nm wavelength using the reported V22 green-band (558 nm) AOD and Ångström exponent. The difference between the gridded AODs is shown in Fig. 7c, while the histograms of the gridded AODs are shown in Fig. 7d. The histograms are split into global, ocean only, and land only categories to allow for better characterization of differences between the versions. The overall AOD distribution patterns are, as expected, similar in V22 (Fig. 7a) and V23 (Fig. 7b). The most visible differences are the generally lower AODs over oceans and the lack of high-AOD artifacts over Greenland and Antarctica in V23. The difference plot (Fig. 7c) reveals more details in the AOD distributions, especially over land. V23 often has higher AODs in the tropics and subtropics over land (red hues), as well as over polluted areas of Southeast Asia, although there is considerable spatial variability in the gridded results, especially over the Amazon. Over oceans, on the other hand, the AOD difference is almost always negative (blue colors), clearly highlighting the impacts of the radiometric corrections, as well as the enhanced cloud screening implemented in V23.
Figure 7a, b also show the global area-weighted average AOD values in 2012 obtained with the two versions of the MISR aerosol product. The area-weighted average AOD is reduced by 0.039 between V22 and V23. For comparison, this difference is considerably larger than the reduction in AOD caused by the additional cloud screening introduced in V23 (Fig. 1 in Sect. 4.1.3). Furthermore, because Figs. 7b and 1b show the same data, except for the Antarctica and Greenland masking (Sect. 4.3.3) applied in Fig. 7b, we conclude that this masking reduces the global average AOD by 0.004, although this number might vary from year to year. These comparisons of globally averaged AOD provide useful insight into the relative importance of individual algorithmic changes in V23, though we caution that the mean values are sensitive to AOD outliers in the data. Additional insights into the general patterns in AOD difference over ocean and land are obtained from histograms of the gridded data (Fig. 7d). The overall V23 AOD histogram (dark gray shading) is shifted towards smaller AOD values in comparison to the V22 histogram, primarily driven by the AODs over oceans, as indicated by the difference between the solid blue (V23) and dashed blue (V22) lines. The AOD histograms over land, on the other hand, are very similar in both versions, suggesting the lack of a universal shift in retrieved AODs.
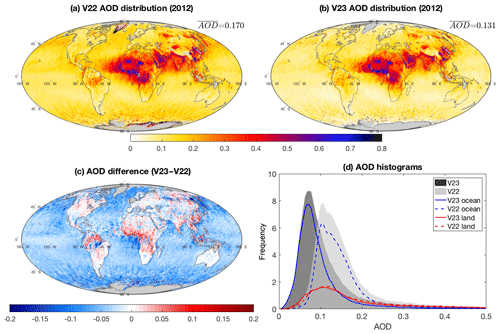
Figure 7(a) Global distribution of AOD in 2012 from V22 retrievals gridded at 0.5∘ resolution. Panel (b) is the same as (a) but using V23 retrievals. (c) AOD difference between V23 and V22. (d) Histograms of gridded AODs: the dark grey shading shows V23 data, the light grey shading shows V22 data, and the solid lines show V23 retrievals over ocean (solid blue) and land (solid red), whereas the dashed lines show V22 retrievals over ocean (dashed blue) and land (dashed red).
In addition to AOD, the MISR aerosol product also reports retrieval-specific AOD uncertainties (UNC). These per-retrieval UNC estimates allow users to quantitatively evaluate the utility of the retrieval results to their research and are becoming increasingly employed in AOD data assimilation applications (Benedetti et al., 2018; Hyer et al., 2011; Shi et al., 2013). Historically, however, the quality of MISR-reported UNCs has not been routinely assessed. This is in large part due to the lack of an independent reference data, as in the case of AERONET for AOD and also the lack of a well-defined evaluation framework. The increasing accuracy of satellite AOD retrievals and the growing demand for retrieved AOD uncertainties motivate detailed evaluation of this product. Recently, Witek et al. (2019) assessed MISR UNC in DW retrievals by comparing them against actual retrieval errors using collocated observations from MAN and AERONET networks. They found that MISR-reported UNC realistically represent retrieval errors and the statistical behavior is similar to that of a Gaussian distribution. Sayer et al. (2020) comprehensively reviewed current prognostic and diagnostic methods used for quantifying uncertainty in satellite AOD retrievals, outlined a general framework for evaluating their accuracy, and used the proposed approach to compare products from several satellite instruments, including MISR. Using AOD observations from a set of 12 AERONET sites as reference, they concluded that no current technique uniformly performs best but that UNC values reported by MISR perform well at most sites. These recent studies by Sayer et al. (2020) and Witek et al. (2019) are the main motivations for comparing the reported UNC between V22 and V23 of the MISR aerosol product.
Figure 8 compares the distributions of average MISR V22 and V23 UNC using retrievals from 2012. There are considerable changes in UNC distributions between V22 and V23, especially considering the very similar distributions of AOD in these products. The highest UNC values in V22 are found over oceans, especially off the west coast of Africa. These high UNC values are substantially reduced in V23 and the highest UNC values are now found over land areas with high AOD. The difference map (Fig. 8c) shows that the UNC response depends on the type of algorithm used, with the DW algorithm generally leading to lower UNC values and the heterogenous land algorithm leading to higher UNC values. As described in Sect. 4.3.2, the over land UNC in V23 is calculated as the standard deviation of per-band AODs for all successful mixtures. This procedure utilizes up to 4 times more AOD values in the UNC derivation than the previous V22 approach. This algorithmic change explains the prevalence of higher UNC values over land in V23, also evident in the frequency distribution plot (Fig. 8d). A few exceptions to this rule are found in equatorial Africa and South America, which could be related to the decreased AODs in V23, as compared to V22 in these areas (Fig. 7c).
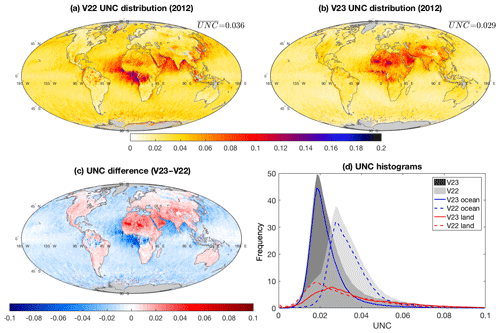
Figure 8As in Fig. 7 but instead using the MISR-reported pixel-level AOD uncertainties (UNC). Because of the uncertainties related to extrapolating V22 UNC values from MISR 558 nm band to 550 nm band, the values from V22 are analyzed at the 558 nm wavelength.
The changes in UNC values over ocean, on the other hand, have two general explanations. First, a very different approach to the calculation of AOD uncertainty was implemented in V23 (see Sect. 4.2.3 for details). The second reason is the overall decrease in oceanic AODs in V23 in comparison to V22, which on average should also lead to lower UNC values if we assume that UNC roughly scales with AOD (Witek et al., 2019).
6.2.3 Ångström exponent (AE) comparison
Comparisons of AE between the two product versions should take note of a small change in the way AE is calculated. In V22, AE is derived using a least-squares linear fitting using the natural logarithm of the best estimate AODs as a function of the natural logarithm of wavelength at all four MISR wavelengths. In V23, on the other hand, the reported AE is calculated using AODs at two wavelengths, 550 and 860 nm, and the AOD at 860 nm is derived using the spectral AOD scaling coefficients, also reported in the V23 product (Sect. 4.2.1). Nevertheless, these two ways of calculating AE are expected to lead to similar outcomes, and observed changes in AE most likely result from other algorithmic and radiometric modifications introduced between the two versions.
The differences in AE between V22 and V23 are visualized in Fig. 9. The gridded AE values shown in Fig. 9 are obtained by AOD-weighted averaging of individual AEs acquired over a year. Note that this averaging procedure is different to, for example, using daily values obtained from the L3 product and averaging them over the same period of time. AE maps from both V22 (Fig. 9a) and V23 (Fig. 9b) show similar global patterns of smaller AEs (larger particles) in the Saharan outflow area and where deserts are present and larger AEs (smaller particles) where biomass burning occurs and over continental midlatitudes. These expected patterns are indicative of MISR's ability to characterize aerosol AE over both land and ocean without prescribing aerosol type (Kahn and Gaitley, 2015). However, substantial differences between the two MISR product versions are apparent in Fig. 9. Over land, the AEs in V23 are larger in some areas (red colors in Fig. 9c) and smaller in others (blue colors in Fig. 9c), as compared to V22, without a clear positive or negative overall shift. As a result, the AE histograms over land are similar between the two versions, as indicated by solid and dashed red lines in Fig. 9d. This contrasts with the general AE shift seen over oceans, with the V23 AEs being considerably smaller than their V22 predecessors. A possible reason for the AE difference over oceans is the new way in which AEs are calculated in V23, using an ensemble-based approach described in the last paragraph of Sect. 4.2.1. Witek et al. (2019) performed preliminary validation of V23 DW AEs against AERONET and MAN observations and found generally good agreement between the data sets, with a small overall bias and a high correlation coefficient, although the range of MISR-retrieved AEs was narrower than that from surface-based observations. It is therefore likely that the narrower histogram of over-ocean AEs in V23 as compared to V22 (solid blue and dashed blue lines in Fig. 9d) is a result of the change in how AE is retrieved.
6.2.4 Single scattering albedo (SSA) comparison
In the V23 aerosol product SSA is not reported directly but can be easily calculated using the reported absorption AOD (AAOD). The formula gives the average AOD-weighted SSA in each grid box; AOD-weighting is also applied to SSA values reported in V22. SSA is based on the best-fitting aerosol model in both V23 and V22, although goodness-of-fit metrics are slightly different between the versions in DW retrievals (see Sect. 4.2.1 and 4.2.2 for details).
The SSA comparisons presented in Fig. 10 reveal some interesting differences between V23 and V22. The low SSA “hot spots” over Africa and South America that are clearly pronounced in V22 are diminished in V23. The SSAs over land are generally larger in V23 than in V22, as evidenced by mainly red hues over continents in Fig. 10c, indicating that V23 favors less-absorbing mixtures over land than V22. A reverse trend is observed over oceans, where V23 generally gives smaller average SSAs in comparison to V22, although results vary between regions. Furthermore, the V23 over-ocean SSAs exhibit higher spatial variability (or noise) than the more horizontally smooth SSAs in V22. This could be partially due to decreased ability of the MISR algorithm to distinguish between particle properties in low-AOD conditions.
The comparatively low V23 SSA values over remote and unpolluted areas such as the Southern Ocean or the Arctic Ocean, however, require further explanation. In V23 about 20 % of grid points over the Southern Ocean, defined by latitudes poleward of 45∘ S, have an average SSA<0.95. This contrasts with only ∼1.5 % of grid points satisfying this condition in V22. In the same area, the percentage of grid points with AOD>0.15 is 42 % in V22 and only 5 % in V23. This suggests that high-AOD outliers with low SSA are not responsible for the low average SSA values over the Southern Ocean seen in Fig. 10b. Further analysis (not shown) confirms that the dominant contribution to the low SSAs over the Southern Ocean comes from retrievals with AOD<0.2 and not cloud-contaminated high AOD retrievals. This suggests that in this area V23 tends to choose the lowest residual mixtures with low SSA more often than V22. This could be associated with the changes in radiometric calibration introduced in V23 with the veiling light correction. Indeed, the magnitude of veiling light correction varies between cameras, which has an impact on the relative agreement of observations with aerosol models and the choice of the lowest residual model. Furthermore, the amount of veiling light correction increases with increasing cloudiness, which is generally consistent with the fact that regions with climatologically higher cloud cover correlate well with the regions where differences in SSA between V22 and V23 are larger (Fig. 10c). Future upgrades to the current veiling light model are being developed by the MISR team (Witek et al., 2018a), which will likely improve SSA retrievals in remote oceanic areas. Presently, it is recommended to use SSA when AOD is above about 0.15, similar to the recommendation based on V22 retrievals (Kahn and Gaitley, 2015).
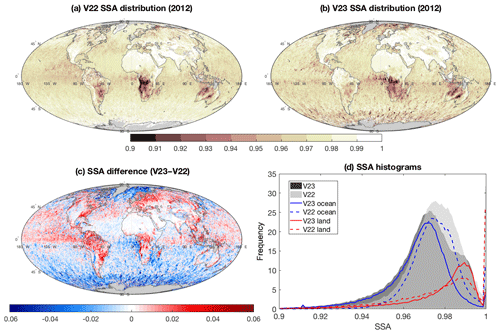
Figure 10As in Fig. 7 except for the MISR-retrieved SSA. AOD weighting is applied in SSA averaging at each grid box.
One other aspect in Fig. 10d that needs further explanation is the much higher frequency of SSA=1.0 in V22 than in V23. This is due to the Greenland and Antarctica land retrieval screening introduced in V23. The V22 retrievals over these areas, as evidenced in Fig. 10a, predominantly have SSA=1.0.
6.2.5 Aerosol nonsphericity comparison
The global distribution maps of aerosol nonspherical AOD fraction in 2012 from MISR V22 and V23 aerosol products are presented in Fig. 11. The average gridded values are AOD weighted. The nonspherical fractions are based on the best-fitting aerosol model. The overall geographical patterns are similar in V22 and V23. The Saharan dust outflow area and the Arabian Peninsula and its vicinity show elevated nonspherical AOD fractions, in agreement with climatological expectations. However, the values over land are smaller, which leads to considerable land–ocean contrasts in these areas, with nonspherical fractions being higher over oceans. The MISR heterogeneous land and DW retrieval algorithms are considerably different, and thus certain disagreement in terms of which mixture is chosen as the lowest residual is expected. An algorithm which uses an ensemble approach over all mixtures as opposed to the single lowest-residual model, as is done for V23 AOD retrievals over DW, will likely mitigate this land–ocean contrast issue in aerosol properties.
Another pronounced feature in Fig. 11a and b is the band of elevated nonspherical fractions over the Southern Ocean. This climatological artifact has been identified in MISR nonspherical AOD fraction retrievals by Kalashnikova et al. (2013). They tend to occur at view illumination geometries at which dust aerosols are indistinguishable from some types of cirrus particles. Such conditions are often found over the Southern Ocean, giving rise to the band of elevated nonspherical AOD fraction. In V23 retrievals these nonspherical fraction artifacts are further enhanced, which could be due to more frequent misclassification between dust–cirrus particles. Higher V23 nonspherical AOD fractions are also found over the southern subtropical Pacific, Atlantic, and Indian Ocean, as indicated by red colors in Fig. 11c. These could be again due to low AODs and unfavorable viewing geometries in those areas.
The overall nonspherical AOD fraction histograms (Fig. 11d) exhibit bi-modal behavior in both V22 and V23, each mode representing retrievals from the heterogeneous land and DW algorithms. The nonspherical AOD fractions peak at about 0.03 and 0.3 for land and DW retrievals, respectively. The magnitudes and widths of the modes differ between the product versions: V23 has a narrower mode over land and a wider mode over oceans in comparison to V22. The slightly wider DW mode in V23 is likely due to increased nonspherical AOD fractions over the Southern Ocean. The increased frequency of nonspherical AOD fraction equal to 0.0 in V22 is again due to retrievals over Antarctica and Greenland, which are masked in V23. The bi-modal behavior mentioned above and the histogram difference between land and DW retrievals are to a large extent caused by low AOD dust–cirrus misclassification in DW retrievals. Focusing the analysis on dust-dominated regions by limiting the geographic extent of the data to a latitude band between 0 and 45∘ N and excluding retrievals with AOD≤0.15 (to increase sensitivity to particle properties), the resulting histograms are closer to being lognormal, although nonspherical fractions over land are still lower than over oceans (results not shown). These findings suggest that caution is warranted when analyzing nonspherical AOD fractions in areas where AODs are low and where cirrus clouds might be present.
6.2.6 Dominant aerosol size comparison
The MISR aerosol retrieval algorithm distinguishes between three dominant aerosol size modes: small, medium, and large. These classifications are based on predefined particle types described by lognormal size distributions and characterized by their characteristic radius and width parameters (Kahn et al., 2010). This three-mode classification is different than a bi-modal description often used in aerosol studies that distinguish only between fine and coarse modes. Mapping of the MISR size bins to the fine and coarse modes is generally straightforward: the MISR small-size mode can be treated as the fine mode, whereas the medium- and large-size modes can be grouped together and considered the coarse mode (Kahn and Gaitley, 2015), although this mapping is approximate and might vary depending on the exact definition of the fine and coarse modes used by investigators. In this section we compare the global distributions of the MISR size mode fractions, gridded and averaged over 2012, obtained from V22 and V23 retrievals. The size mode classification in MISR retrievals is based on the properties of the best-fitting mixture that has the lowest overall residual, and as such it is sensitive to the choice of mixtures considered in the retrieval and to the changes in radiometric calibration of the instrument.
Figures 12, 13, and 14, show maps of small-, , and large-mode AOD fractions, respectively, along with the histograms of their distributions. Similar patterns emerge to those observed for other particle properties: (a) differences between V22 and V23 depend on the type of algorithm (land vs. DW) and (b) changes over land are generally less significant than changes over ocean. A few interesting shifts in size mode distributions between V22 and V23 can be observed. First, the DW small-mode AOD fraction in V23 is smaller than in V22, as evidenced by mostly blue colors over oceans in Fig. 12c and by a shifted histogram in Fig. 12d. This change is compensated for by the respective large-mode AOD fraction increase in V23 (Fig. 14c and d). This shows that the V23 DW algorithm chooses the lowest residual mixtures consisting of larger-sized aerosols more often than in V22. It is possible that radiometric changes applied in V23 (see Sect. 4.1.2 and 4.2.6 for details) allow for an improved detection of coarse-mode sea spray aerosol. The fact that the large-mode AOD fraction in V23 increases slightly over the windy Southern Ocean seems to confirm this notion (Fig. 14b). Another size mode distribution change worth noting is the largely opposite shift over land, with the V23 small-mode AOD fraction often being larger than in V22. Retrievals over Australia are a good example of this change: the small-mode AOD fraction increases substantially from V22 to V23 (Fig. 12c), and the large-mode AOD fraction sees a corresponding decrease between V22 and V23 (Fig. 14c). At the same time, the AODs over Australia remain mostly unchanged (Fig. 7c). Because the radiometric correction (Sect. 4.1.2) introduced in V23 is not a substantial factor for AOD retrievals over land, we conclude that the observed size mode shift between V22 and V23 is largely due to the resolution change and the resulting changes in the characterization of surface reflectance in the retrieval process.
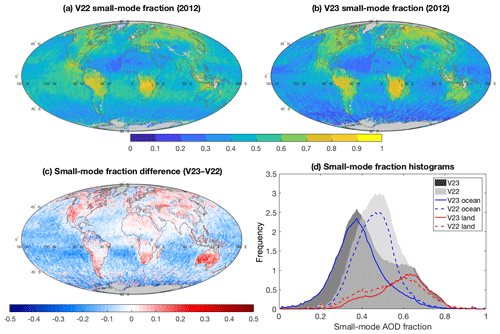
Figure 12As in Fig. 7 except for the MISR-retrieved small-mode AOD fraction. AOD weighting is applied in data averaging.
The medium-sized AOD fractions in MISR retrievals from both V22 and V23 are considerably smaller than the other two size modes, especially over land (Fig. 13). The changes in medium-sized fractions between the two versions are also relatively small. The medium-mode AOD fractions over land are generally below 0.1, with slightly larger values over northern Africa, the Middle East, and southern Asia, likely due to detection of mineral dust aerosols. The low values of medium-mode AOD fractions in MISR retrievals might result from a limited representation of this size mode in the current algorithm climatology (Kahn et al., 2010), given that medium-size mode and medium-sized particles have been retrieved from AERONET observations after fog or stratocumulus cloud dissipation events at several AERONET locations (Eck et al., 2012). A different explanation is that the bi-modal (fine and coarse) aerosol size characterization might be generally more suitable than the current three-mode classification.
6.3 Statistical comparisons with AERONET and MAN
The goal of this section is to present preliminary validation of the V23 retrievals against surface-based observations (OBS) from the AERONET and MAN networks. Furthermore, each MISR–OBS collocation has to include both V23 and V22 retrievals in order to also assess V23 performance against V22. The focus of these comparisons is on global retrievals of AOD and AE and supplements the analysis of V23 retrievals over dark water that has already been published (Witek et al., 2019).
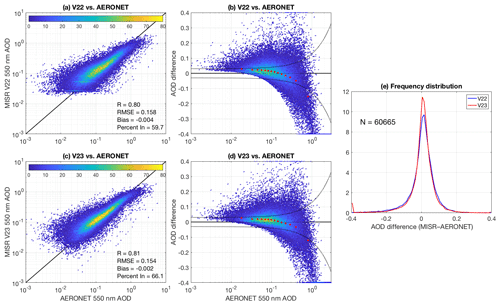
Figure 15MISR V22 and V23 retrieval comparisons against AERONET observations: (a) density plot of MISR V22–AERONET collocations including overall statistics, (b) density plot of AOD difference (MISR V22–AERONET) as a function of AERONET AOD, points outside of the y axis limits are grouped on the edges, red squares denote the median values for data split into 14 equally populated bins, and the dotted black lines mark the EE of %). (c) is similar to (a) but for MISR V23–AERONET collocations. Panel (d) is similar to (b) but for MISR V23 retrievals. (e) Histograms of the MISR–AERONET AOD difference for V22 (blue line) and V23 (red line) retrievals. The number of joint V22–V23–AERONET collocations is 60 665.
6.3.1 Comparisons against AERONET AOD observations
AERONET is a ground-based, global aerosol monitoring network of Cimel sun photometers that provides sub-hourly AOD and AE measurements for validating satellite aerosol retrievals (Holben et al., 1998, 2001). The robust calibration, data-processing, and screening procedures employed by AERONET ensure the high accuracy of AOD and AE, with an estimated uncertainty of ±0.01–0.02 for AODs at mid-visible bands (Eck et al., 1999). In order to validate MISR AOD and AE retrievals, the current study examines version 3 AERONET level 2.0 (cloud screened and quality assured) data (Giles et al., 2019) downloaded from https://aeronet.gsfc.nasa.gov/new_web/download_all_v3_aod.html (last access: 9 July 2018). This AERONET data set contains over 23.5 million individual observations from 1160 stations. Temporal and spatial collocations between MISR retrievals and AERONET observations are identified following a set of criteria. Temporally, AERONET observations within ±30 min of a MISR overpass are averaged to form a single reference observation. Spatially, only those MISR retrievals that fall within a circle of 25 km radius centered on the surface-based observations are averaged to form a corresponding comparison point. AERONET spectral AODs, measured at several wavelengths in the 340–1020 nm range, are interpolated to the MISR V23 550 nm wavelength using a second-order polynomial fit in ln(AOD) vs. ln(wavelength) space (Eck et al., 1999; Schuster et al., 2006). The MISR V22 AOD retrievals reported at 558 nm are extrapolated to 550 nm using the MISR-reported AE. This procedure results in 60 665 joint collocations among MISR V22, MISR V23, and AERONET. Over 85 % of those collocations involve MISR retrievals over land.
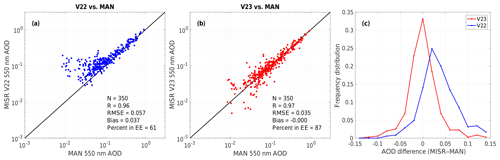
Figure 16Scatterplots and statistical comparison between collocated MISR V22, MISR V23, and MAN observations: (a) scatterplot of MISR V22–MAN collocations including overall statistics, (b) scatterplot of MISR V23–MAN collocations including overall statistics, and (c) histograms of the MISR–MAN AOD difference for V23 (red) and V22 (blue) retrievals.
According to the statistical comparison between collocated MISR retrievals and AERONET observations (Fig. 15), the accuracy of AOD retrievals in the MISR V23 data product improves upon (or is at least as good as) the previous V22 product. The correlation coefficient increases from 0.80 to 0.81, the root-mean-square error decreases from 0.158 to 0.154, the absolute value of the negative bias decreases from 0.004 to 0.002, and the percent of retrievals that fall within the error envelope (EE) of %) increases from 59.7 % to 66.1 %. The improvement is more substantial at low AOD ranges (AOD<0.1), where unrealistic quantization of MISR V22 AOD is largely eliminated in V23 (Fig. 15a, c). The histogram of AOD difference between V23 and AERONET (Fig. 15e) exhibits a narrower and steeper peak at zero, compared with V22, indicating better performance of the new product.
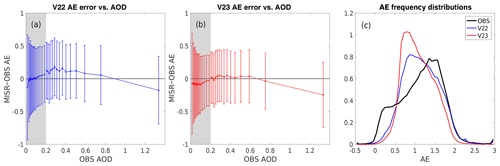
Figure 17V22 and V23 AE comparisons against AERONET + MAN observations (OBS). (a) AE difference (MISR V22 – OBS) as a function of OBS AOD; data are split into two AOD regimes, OBS AOD≤0.2 and OBS AOD>0.2, and divided into 15 equally populated bins within each of the AOD regimes. The shaded area marks the smaller AOD regime. The markers represent the median AE difference values within each AOD bin and the error bars are 1 standard deviation of AE difference within each bin. (b) is the same as (a) but for the MISR V23–OBS AE difference. (c) Normalized frequency distributions of AE obtained from the three collocated data sets. The total number of analyzed collocations is 61 015, and 17 027 of collocations have OBS AOD>0.2.
Although V23 resolves several issues in AOD retrieval relative to the previous version, it still tends to on average overestimate low AOD values and underestimated high AOD values (Fig. 15d), though to a lesser extent than V22. These biases have been extensively discussed in previous MISR product overview and validation studies (e.g., Kahn et al., 2010). Since the updates to the AOD retrieval in V23 are mainly associated with the DW algorithm, the improvement of AOD retrievals seen in collocated data set, which consists predominantly of land retrievals, is not as substantial as when the comparison focuses on ocean, as discussed below in Sect. 6.3.2. Witek et al. (2019) analyzed over 11 000 matchups between AERONET observations and MISR V23 DW retrievals and found very good agreement, as demonstrated by the correlation coefficient of 0.9, root-mean-squared error (RMSE) of 0.068, and 77 % of retrievals within the EE. Furthermore, the statistics improved even more when the collocation distance was reduced, indicating that aerosol spatial heterogeneity effects might be negatively impacting the comparison metrics and the performance assessment presented above.
6.3.2 Comparisons against MAN AOD observations
The Maritime Aerosol Network (MAN) (Smirnov et al., 2006, 2009, 2011), part of AERONET, has been collecting ship-based aerosol observations since 2006. Because the network employs handheld Microtops II sun photometers, the estimated uncertainty of the post-field-calibrated level 2 optical depth is approximately ±0.02, slightly larger than that of automated AERONET observations. In order to validate MISR AOD retrievals against MAN observations, the “series average” version 2 level 2.0 MAN data was used. The MAN spectral AOD was interpolated to 550 nm using a second-order polynomial fit in ln(AOD) vs. ln(wavelength) space (Eck et al., 1999). The MISR V22 AOD retrievals reported at 558 nm are extrapolated to the 550 nm wavelength using the MISR-reported AE. The collocation procedure is slightly different than the one used for finding MISR collocations with AERONET. The temporal collocation criterion is ±30 min between the time of MISR overpass and MAN observations. The spatial averaging is performed on MISR retrievals that fall within at least 25 km radius around the average MAN location; the radius is extended according to the distance traveled by a ship within the collocation time window (for details, see Witek et al., 2019). The procedure results in 350 matchups between MISR V22, MISR V23, and MAN.
Figure 16 shows statistical comparisons between collocated MISR retrievals and MAN observations. The red and blue colors represent MAN collocations with MISR V23 and V22 retrievals, respectively. There is substantial improvement in the accuracy of V23 retrievals as compared to the previous V22 product: the RMSE decreases from 0.057 to 0.035, the bias decreases from 0.037 to 0.0, and the percent of retrievals that fall within the EE of %) increases from 61 % to 87 %. The AOD difference histogram for V23 collocations (Fig. 16c) is centered at zero and narrower than that from V22. The collocations with MAN show very good performance of the V23 retrievals even when AOD levels are very low, indicating that the positive bias at low AODs present in V22 retrievals (Kahn et al., 2010) has been effectively removed.
The bias reduction between V23 and V22 retrievals with respect to MAN observations is 0.037. This number is close to 0.043, the difference in average AOD over ocean between V23 and V22 calculated using 16 years of L3 data (see Sect. 5.2). The close agreement between these assessments lends credence to the robustness and consistency of the improvements introduced in the V23 DW retrieval algorithm. Matching only the V23 product with MAN, Witek et al. (2019) found a larger number of collocations (406); the comparison statistics for this larger data set are in very close agreement with the statistics obtained in this paper.
6.3.3 Ångström exponent (AE) comparison against AERONET and MAN
In this section, the analyzed AE is defined as , where τ is the AOD and the reference wavelengths are λ1=440 nm and λ2=870 nm, except for the V22 data for which AE is calculated using a linear fit in the log–log space using AODs at all four MISR wavelengths (see Sect. 6.2.3 for additional details). Note that the reference wavelengths used for AE comparisons against surface-based observations are different than those used for the global AE analyses presented in Sect. 6.2.3. For AERONET and MAN data sets, if the AE between the reference wavelengths of 440 and 870 nm is missing in the data, it is independently calculated given that enough spectral AOD measurements are available. Depending on the available number of spectral channels, either a linear or a second-order polynomial fit in ln(AOD) vs. ln(wavelength) space is used to calculate AODs at the reference wavelengths, followed by AE calculation using the definition above. The numbers of MISR–AERONET and MISR–MAN AE matchups are 60 655 and 350, respectively.
Figure 17 presents comparisons between observed AE and AE retrieved by MISR for the combined MISR–OBS matchups. Figure 17a and b show the MISR–OBS AE difference as a function of OBS AOD for V22 and V23 retrievals, respectively, following a comparison method from Sayer et al. (2018, Fig. 6c). Data points are binned into equally populated intervals of AOD and analyzed separately for low AOD (≤0.2) and high AOD (>0.2) regimes. The error bars represent 1 standard deviation of AE differences within each AOD bin. A cursory look at Fig. 17a and b suggests that V22 tends to agree with OBS when AODs are low and overestimates AE at higher AODs. Conversely, V23 tends to underestimate AE in the low AOD regime but agrees well with OBS when AOD are higher. In both data sets, the largest AOD bin, which covers OBS AODs larger than about 0.9, shows that in this AOD range MISR tends to underestimate AE in comparison to OBS. The standard deviations of the AE errors are in general quite similar for V22 and V23, although they are on average about 7 % smaller in V23. Furthermore, the standard deviations decrease substantially, almost by 50 %, between the smallest AOD bin and the bins with AODs about 0.15–0.2. This suggests increased uncertainty in derived AE values when AOD levels are low, which applies to both surface-based observations (Wagner and Silva, 2008) and MISR retrievals alike.
Figure 17c shows histograms of AE from MISR V22, MISR V23, and OBS. MISR AE retrievals cover the bulk of observed values, but the shapes of the distributions are generally narrower than from OBS and peak at a lower value of AE. Especially, MISR AE retrievals tend to miss the low AE range of the distribution. Furthermore, V23 has a slightly narrower distribution than V22, which could be partially due to averaging of more retrievals within the collocation scene.
Selected AE comparison statistics are listed in Table 1. Only data points with OBS AOD>0.2 are included. Comparisons against AERONET, which mainly contain retrievals over land, show improvement in the overall statistics between V22 and V23. The matchups against MAN are substantially less numerous; the statistics are very similar between V22 and V23, except for the bias, which is considerably larger in V22. Overall, these comparisons suggest that the V23 AE retrievals have comparable accuracy to those in V22 when compared against reference AE observations from AERONET and MAN networks.
7.1 Product updates
Version 23 of the MISR aerosol product became publicly available in November 2017. Full reprocessing of the data was completed in June 2018, and production since then has made use of the new version. Currently, more than 19 years of consistent V23 aerosol data are available to the user community. Many changes and upgrades that enhance the overall quality and usability of the product were introduced in V23. These are summarized as follows.
For convenience, the data format was updated to NetCDF-4 and the content of the MISR aerosol product, including field names and general categories, was redefined to be more accessible and user-friendly. These updates were designed to encourage current and new MISR data users to better utilize the wealth of aerosol information provided by the MISR instrument.
The spatial resolution of MISR retrievals has been increased to 4.4 km (from to 17.6 km) without compromising the product quality. The higher resolution provides a much finer level of detail on aerosol spatial distribution and facilitates new applications of the data, for example, in studies of air pollution's impact on public health.
Radiometric corrections have been implemented to improve retrievals in low AOD conditions and to mitigate a low AOD gap present in the V22 product. Low-level stray light contamination in MISR cameras, which becomes relevant under clean conditions in high-contrast scenes was identified as the primary source of low AOD bias in MISR retrievals. A correction for veiling light is included the V23 algorithm. The incorporation of underlight has improved the representation of water-leaving radiances in the MISR DW algorithm and further improved AOD retrievals.
The MISR DW algorithm has been redesigned in order to utilize the full range of cost functions calculated in the retrieval processing. The new approach allows for accurate and consistent characterization of AOD and its uncertainty and, with the use of ARCI metric, provides an additional line of defense against cloud-contaminated retrievals. Furthermore, because all MISR mixtures take part in AOD derivation over ocean, it eliminates the need for mixture selection thresholds present in V22.
A number of upgrades to the Het Surf algorithm, such as threshold and logic changes, improvement in consistency of AOD uncertainties, and geographic masking, have been implemented to improve retrieval quality and coverage over land.
Additional cloud screening in the retrieval region and its vicinity has been implemented. This procedure eliminates potentially cloud-contaminated retrievals that have not been flagged by any of the previous cloud-clearing methods. Since thresholds on these additional metrics are not very strict, users can use pre-screened retrievals and experiment with their own screening approaches depending on application.
A number of other algorithmic changes, described in detail in Sect. 4, have been implemented in V23 to improve the quality, consistency, and usability of MISR retrievals. The overall impact of these updates is summarized below.
7.2 Initial evaluation
Initial evaluations of the data product contents are provided for the year 2012. This year was chosen because the MISR instrument had relatively few missing orbits and the Oceanic Niño Index was neutral. Furthermore, joint MISR V22, V23, and surface-based (AERONET and MAN) AOD collocation data obtained over a period of 18 years was used to assess MISR retrievals against reference observations. The principal findings are as follows.
Evaluation against surface-based observations indicates that V23 AOD retrievals over land have comparable accuracy to V22, but over oceans the V23 performance improved substantially. This demonstrates that numerous efforts directed towards improving AOD retrievals over oceans have been successful and that the quality of retrievals over land have been preserved in V23, despite the higher spatial resolution.
AE comparisons against AERONET and MAN indicate similar performance between V22 and V23 retrievals. This is an expected outcome, as the V23 release cycle did not focus on improving aerosol property determination in the MISR retrievals.
There is a systematic decrease in retrieved AODs over ocean between V23 and V22, which is mainly associated with the implementation of veiling light correction in V23 retrievals. AOD changes over land exhibit both positive and negative AOD differences, depending on the region.
Changes in reported AOD uncertainties are more substantial in comparison to AODs, reflecting a considerable algorithmic upgrade between V22 and V23, especially in DW retrievals. Two independent validation studies indicate that MISR-reported V23 AOD uncertainties represent retrieval error reasonably well, exhibiting behavior similar to the standard deviation of a Gaussian distribution. This suggests that V23 AOD uncertainties are more realistic than their V22 predecessors.
Differences in reported aerosol property distributions between V22 and V23 were analyzed. After examining AE, SSA, nonspherical AOD fraction, and size-mode AOD fractions, three general conclusions emerge: (a) differences between V22 and V23 depend on the type of algorithm (land vs. DW), (b) changes in aerosol properties over land are generally less significant than changes over ocean, and (c) aerosol property distributions over ocean are less homogenous in V23 than in V22. Some possible reasons for these differences were discussed, although more quantitative evaluation of retrieved particle properties is beyond the scope of this study.
All data analyzed in this study are publicly available. AERONET (Holben et al., 1998) and MAN (Smirnov et al., 2011) data are available at https://aeronet.gsfc.nasa.gov/new_web/data.html (last access: 27 January 2020). MISR V23 data are available at ftp://l5eil01.larc.nasa.gov/MISR/MIL2ASAE.003/ (last access: 27 January 2020).
MJG initiated the study and generated the initial draft of the manuscript. MLW led the manuscript to completion and provided key analyses and figures. RAK led the summary of the Version 22 product validation and the description of the sun glint approach. JAL and RAK led the description of the underlight approach and advised on many aspects of the Version 23 product validation effort overall. FCS contributed to the overall aerosol product improvements and evaluation with a focus on cloud screening. MAB contributed technical inputs to many sections and provided Figs. 4 and 5. HL drafted Sect. 5. DJD advised the study and contributed to the scientific merit of the manuscript, including proofreading for consistency with the algorithm structure. EGH supervised the study and managed the workflow. OVK helped in drafting Sect. 6.1. AMN prepared section 4.1.4 and, with assistance from MAB and MJG, Appendix A. YY drafted Sect. 6.3.1 and 6.3.2. All authors contributed to the writing of the manuscript.
The authors declare that they have no conflict of interest.
The research was carried out at the Jet Propulsion Laboratory, California Institute of Technology, under a contract from the National Aeronautics and Space Administration. Support from the MISR project is acknowledged. The work of Ralph Kahn and James Limbacher was performed at the NASA Goddard Space Flight Center and is supported in part by NASA’s Climate and Radiation Research and Analysis Program under Hal Maring, NASA’s Atmospheric Composition Mapping and Analysis Program under Richard Eckman, and the EOS MISR project. Special thanks to the two anonymous reviewers and Andrew Sayer for carefully reading the manuscript and providing valuable comments.
This research has been supported by the MISR project.
This paper was edited by Thomas Eck and reviewed by Andrew Sayer and two anonymous referees.
Abdou, W. A., Diner, D. J., Martonchik, J. V., Bruegge, C. J., Kahn, R. A., Gaitley, B. J., Crean, K. A., Remer, L. A., and Holben, B.: Comparison of coincident Multiangle Imaging Spectroradiometer and Moderate Resolution Imaging Spectroradiometer aerosol optical depths over land and ocean scenes containing Aerosol Robotic Network sites, J. Geophys. Res.-Atmos., 110, 1–12, https://doi.org/10.1029/2004JD004693, 2005.
Alfaro-Contreras, R., Zhang, J., Reid, J. S., and Christopher, S.: A study of 15-year aerosol optical thickness and direct shortwave aerosol radiative effect trends using MODIS, MISR, CALIOP and CERES, Atmos. Chem. Phys., 17, 13849–13868, https://doi.org/10.5194/acp-17-13849-2017, 2017.
Benedetti, A., Reid, J. S., Knippertz, P., Marsham, J. H., Di Giuseppe, F., Rémy, S., Basart, S., Boucher, O., Brooks, I. M., Menut, L., Mona, L., Laj, P., Pappalardo, G., Wiedensohler, A., Baklanov, A., Brooks, M., Colarco, P. R., Cuevas, E., da Silva, A., Escribano, J., Flemming, J., Huneeus, N., Jorba, O., Kazadzis, S., Kinne, S., Popp, T., Quinn, P. K., Sekiyama, T. T., Tanaka, T., and Terradellas, E.: Status and future of numerical atmospheric aerosol prediction with a focus on data requirements, Atmos. Chem. Phys., 18, 10615–10643, https://doi.org/10.5194/acp-18-10615-2018, 2018.
Bothwell, G. W., Hansen, E. G., Vargo, R. E., and Miller, K. C.: The multi-angle imaging spectroradiometer science data system, its products, tools, and performance, IEEE T. Geosci. Remote Sens., 40, 1467–1476, https://doi.org/10.1109/TGRS.2002.801152, 2002.
Bruegge, C. J., Chrien, N. L., Ando, R. R., Diner, D. J., Abdou, W. A., Helmlinger, M. C., Pilorz, S. H., and Thome, K. J.: Early validation of the Multi-angle Imaging SpectroRadiometer (MISR) radiometric scale, IEEE T. Geosci. Remote Sens., 40, 1477–1492, https://doi.org/10.1109/TGRS.2002.801583, 2002.
Bruegge, C. J., Abdou, W., Diner, D. J., Gaitley, B. J., Helmlinger, M., Kahn, R. A., and Martonchik, J. V.: Validating the MISR radiometric scale for the ocean aerosol science communities, edited by: Morain, S. A. and Budge, A. M., Post-launch calibration of satellite sensors (103–115), Leiden, Netherlands: A.A. Balkema Publishers, 2004.
Cheng, T., Chen, H., Gu, X., Yu, T., Guo, J., and Guo, H.: The inter-comparison of MODIS, MISR and GOCART aerosol products against AERONET data over China, J. Quant. Spectrosc. Ra., 113, 2135–2145, https://doi.org/10.1016/j.jqsrt.2012.06.016, 2012.
Chin, M., Diehl, T., Tan, Q., Prospero, J. M., Kahn, R. A., Remer, L. A., Yu, H., Sayer, A. M., Bian, H., Geogdzhayev, I. V., Holben, B. N., Howell, S. G., Huebert, B. J., Hsu, N. C., Kim, D., Kucsera, T. L., Levy, R. C., Mishchenko, M. I., Pan, X., Quinn, P. K., Schuster, G. L., Streets, D. G., Strode, S. A., Torres, O., and Zhao, X.-P.: Multi-decadal aerosol variations from 1980 to 2009: a perspective from observations and a global model, Atmos. Chem. Phys., 14, 3657–3690, https://doi.org/10.5194/acp-14-3657-2014, 2014.
Cox, C. and Munk, W.: Measurement of the Roughness of the Sea Surface from Photographs of the Sun's Glitter, J. Opt. Soc. Am., 44, 838–850, https://doi.org/10.1364/JOSA.44.000838, 1954.
Dey, S. and Di Girolamo, L.: A climatology of aerosol optical and microphysical properties over the Indian subcontinent from 9 years (2000–2008) of Multiangle Imaging Spectroradiometer (MISR) data, J. Geophys. Res.-Atmos., 115, 1–22, https://doi.org/10.1029/2009JD013395, 2010.
Diner, D. J. and Martonchik, J. V.: Atmospheric transmittance from spacecraft using multiple view angle imagery, Appl. Opt., 24, 3503, https://doi.org/10.1364/ao.24.003503, 1985.
Diner, D. J., Beckert, J. C., Reilly, T. H., Bruegge, C. J., Conel, J. E., Kahn, R. A., Martonchik, J. V., Ackerman, T. P., Davies, R., Gerstl, S. A. W., Gordon, H. R., Muller, J. P., Myneni, R. B., Sellers, P. J., Pinty, B., and Verstraete, M. M.: Multiangle Image Spectroradiometer (MISR) instrument description and experiment overview, IEEE T. Geosci. Remote Sens., 36, 1072–1087, 1998.
Diner, D. J., Abdou, W. A., Bruegge, C. J., Conel, J. E., Crean, K. A., Gaitley, B. J., Helmlinger, M. C., Kahn, R. A., Martonchik, J. V., Pilorz, S. H., and Holben, B. N.: MISR aerosol optical depth retrieval over southern Africa during the SAFARI-2000 dry season campaign, Geophys. Res. Lett., 28, 3127–3130, https://doi.org/10.1029/2001GL013188, 2001.
Diner, D. J., Martonchik, J. V., Kahn, R. A., Pinty, B., Gobron, N., Nelson, D. L., and Holben, B. N.: Using angular and spectral shape similarity constraints to improve MISR aerosol and surface retrievals over land, Remote Sens. Environ., 94, 155–171, https://doi.org/10.1016/j.rse.2004.09.009, 2005.
Diner, D. J., Abdou, W. A., Ackerman, T. P., Crean, K., Gordon, H. R., Kahn, R. A., Martonchik, J. V., McMuldroch, S., Paradise, S. R., and Pinty, B.: Level 2 aerosol retrieval algorithm theoretical basis, Jet Propuls. Lab. Calif. Inst. Technol., 1–92, 2008.
Di Girolamo, L. and Wilson, M. J.: A first look at band-differenced angular signatures for cloud detection from MISR, IEEE T. Geosci. Remote Sens., 41, 1730–1734, https://doi.org/10.1109/TGRS.2003.815659, 2003.
Eck, T. F., Holben, B. N., Reid, J. S., Dubovik, O., Smirnov, A., O'Neill, N. T., Slutsker, I., and Kinne, S.: Wavelength dependence of the optical depth of biomass burning, urban, and desert dust aerosols, J. Geophys. Res.-Atmos., 104, 31333–31349, https://doi.org/10.1029/1999JD900923, 1999.
Eck, T. F., Holben, B. N., Reid, J. S., Giles, D. M., Rivas, M. A., Singh, R. P., Tripathi, S. N., Bruegge, C. J., Platnick, S., Arnold, G. T., Krotkov, N. A., Carn, S. A., Sinyuk, A., Dubovik, O., Arola, A., Schafer, J. S., Artaxo, P., Smirnov, A., Chen, H., and Goloub, P.: Fog- and cloud-induced aerosol modification observed by the aerosol robotic network (AERONET), J. Geophys. Res.-Atmos., 117, 1–18, https://doi.org/10.1029/2011JD016839, 2012.
Eck, T. F., Holben, B. N., Reid, J. S., Mukelabai, M. M., Piketh, S. J., Torres, O., Jethva, H. T., Hyer, E. J., Ward, D. E., Dubovik, O., Sinyuk, A., Schafer, J. S., Giles, D. M., Sorokin, M., Smirnov, A., and Slutsker, I.: A seasonal trend of single scattering albedo in southern African biomass-burning particles: Implications for satellite products and estimates of emissions for the world's largest biomass-burning source, J. Geophys. Res.-Atmos., 118(12), 6414–6432, https://doi.org/10.1002/jgrd.50500, 2013.
Fox, D., Gonzalez, E., Kahn, R., and Martonchik, J.: Near-surface wind speed retrieval from space-based, multi-angle imaging of ocean sun glint patterns, Remote Sens. Environ., 107, 223–231, https://doi.org/10.1016/j.rse.2006.10.021, 2007.
Franklin, M., Kalashnikova, O. V. and Garay, M. J.: Size-resolved particulate matter concentrations derived from 4.4 km-resolution size-fractionated Multi-angle Imaging SpectroRadiometer (MISR) aerosol optical depth over Southern California, Remote Sens. Environ., 196, 312–323, https://doi.org/10.1016/j.rse.2017.05.002, 2017.
Franklin, M., Kalashnikova, O. V., Garay, M. J., and Fruin, S.: Characterization of subgrid-scale variability in particulate matter with respect to satellite aerosol observations, Remote Sens., 10, 623, https://doi.org/10.3390/rs10040623, 2018a.
Franklin, M., Chau, K., Kalashnikova, O., Garay, M., Enebish, T., and Sorek-Hamer, M.: Using Multi-Angle Imaging SpectroRadiometer Aerosol Mixture Properties for Air Quality Assessment in Mongolia, Remote Sens., 10, 1317, https://doi.org/10.3390/rs10081317, 2018b.
Garay, M. J., Kalashnikova, O. V., and Bull, M. A.: Development and assessment of a higher-spatial-resolution (4.4 km) MISR aerosol optical depth product using AERONET-DRAGON data, Atmos. Chem. Phys., 17, 5095–5106, https://doi.org/10.5194/acp-17-5095-2017, 2017.
Giles, D. M., Sinyuk, A., Sorokin, M. G., Schafer, J. S., Smirnov, A., Slutsker, I., Eck, T. F., Holben, B. N., Lewis, J. R., Campbell, J. R., Welton, E. J., Korkin, S. V., and Lyapustin, A. I.: Advancements in the Aerosol Robotic Network (AERONET) Version 3 database – automated near-real-time quality control algorithm with improved cloud screening for Sun photometer aerosol optical depth (AOD) measurements, Atmos. Meas. Tech., 12, 169–209, https://doi.org/10.5194/amt-12-169-2019, 2019.
Gordon, R.: Atmospheric correction of ocean color imagery in the Earth Observing System era, J. Geophys. Res.-Atmos., 102, 17081–17106, https://doi.org/10.1029/96JD02443, 1997.
Guo, Y., Tian, B., Kahn, R. A., Kalashnikova, O., Wong, S., and Waliser, D. E.: Tropical Atlantic dust and smoke aerosol variations related to the Madden-Julian Oscillation in MODIS and MISR observations, J. Geophys. Res.-Atmos., 118, 4947–4963, https://doi.org/10.1002/jgrd.50409, 2013.
Holben, B. N., Eck, T. F., Slutsker, I., Tanré, D., Buis, J. P., Setzer, A., Vermote, E., Reagan, J. A., Kaufman, Y. J., Nakajima, T., Lavenu, F., Jankowiak, I., and Smirnov, A.: AERONET – Federated Instrument Network and Data Archive for Aerosol Characterization, Remote Sens. Environ., 66, 1–16, https://doi.org/10.1016/S0034-4257(98)00031-5, 1998.
Holben, B. N., Tanré, D., Smirnov, a., Eck, T. F., Slutsker, I., Abuhassan, N., Newcomb, W. W., Schafer, J. S., Chatenet, B., Lavenu, F., Kaufman, Y. J., Castle, J. Vande, Setzer, a., Markham, B., Clark, D., Frouin, R., Halthore, R., Karneli, A., O'Neill, N. T., Pietras, C., Pinker, R. T., Voss, K., and Zibordi, G.: An emerging ground-based aerosol climatology: Aerosol optical depth from AERONET, J. Geophys. Res., 106, 12067, https://doi.org/10.1029/2001JD900014, 2001.
Hyer, E. J., Reid, J. S., and Zhang, J.: An over-land aerosol optical depth data set for data assimilation by filtering, correction, and aggregation of MODIS Collection 5 optical depth retrievals, Atmos. Meas. Tech., 4, 379–408, https://doi.org/10.5194/amt-4-379-2011, 2011.
IPCC: Climate Change 2013: The Physical Science Basis. Contribution of Working Group I to the Fifth Assessment Report of the Intergovernmental Panel on Climate Change, edited by: Stocker, T. F., Qin, D., Plattner, G. K., Tignor, M. M. B., Allen, S. K., Boschung, J., Nauels, A., Xia, Y., Bex, V., and Midgley, P. M., Cambridge University Press, Cambridge, United Kingdom and New York, NY, USA, 1535 pp., 2013.
Irons, J. R., Dwyer, J. L., and Barsi, J. A.: The next Landsat satellite: The Landsat Data Continuity Mission, Remote Sens. Environ., 122, 11–21, https://doi.org/10.1016/j.rse.2011.08.026, 2012.
Jovanovic, V. M., Bull, M. A., Smyth, M. M., and Zong, J.: MISR in-flight camera geometric model calibration and georectification performance, IEEE T. Geosci. Remote Sens., 40, 1512–1519, https://doi.org/10.1109/TGRS.2002.801143, 2002.
Jovanovic, V. M., Moroney, C., and Nelson, D.: Multi-angle geometric processing for globally geo-located and co-registered MISR image data, Remote Sens. Environ., 107, 22–32, https://doi.org/10.1016/j.rse.2006.08.013, 2007.
Kahn, R. A., West, R., McDonald, D., Rheingans, B., and Mishchenko, M. I.: Sensitivity of multiangle remote sensing observations to aerosol sphericity, J. Geophys. Res.-Atmos., 102, 16861–16870, https://doi.org/10.1029/96JD01934, 1997.
Kahn, R. A., Banerjee, P., Mcdonald, D., and Diner, D. J.: Sensitivity of multiangle imaging to aerosol optical depth and to pure-particle size distribution and composition over ocean, J. Geophys. Res., 103, 32195–32213, https://doi.org/10.1029/98JD01752, 1998.
Kahn, R. A., Banerjee, P., and McDonald, D.: Sensitivity of multiangle imaging to natural mixtures of aerosols over ocean, J. Geophys. Res., 106, 18219, https://doi.org/10.1029/2000JD900497, 2001.
Kahn, R. A., Anderson, J., Anderson, T. L., Bates, T., Brechtel, F., Carrico, C. M., Clarke, A., Doherty, S. J., Dutton, E., Flagan, R., Frouin, R., Fukushima, H., Holben, B., Howell, S., Huebert, B., Jefferson, A., Jonsson, H., Kalashnikova, O., Kim, J., Kim, S. W., Kus, P., Li, W. H., Livingston, J. M., McNaughton, C., Merrill, J., Mukai, S., Murayama, T., Nakajima, T., Quinn, P., Redemann, J., Rood, M., Russell, P., Sano, I., Schmid, B., Seinfeld, J., Sugimoto, N., Wang, J., Welton, E. J., Won, J. G., and Yoon, S. C.: Environmental snapshots from ACE-Asia, J. Geophys. Res.-Atmos., 109, D19S14, https://doi.org/10.1029/2003JD004339, 2004.
Kahn, R. A.: Reducing the Uncertainties in Direct Aerosol Radiative Forcing, Surv. Geophys., 33, 701–721, https://doi.org/10.1007/s10712-011-9153-z, 2012.
Kahn, R. A. and Gaitley, B. J.: An analysis of global aerosol type as retrieved by MISR, J. Geophys. Res.-Atmos., 120, 4248–4281, https://doi.org/10.1002/2015JD023322, 2015.
Kahn, R. A., Li, W.-H., Martonchik, J. V., Bruegge, C. J., Diner, D. J., Gaitley, B. J., Abdou, W., Dubovik, O., Holben, B., Smirnov, A., Jin, Z., and Clark, D.: MISR Calibration and Implications for Low-Light-Level Aerosol Retrieval over Dark Water, J. Atmos. Sci., 62, 1032–1052, https://doi.org/10.1175/JAS3390.1, 2005a.
Kahn, R. A., Gaitley, B. J., Martonchik, J. V., Diner, D. J., Crean, K. A., and Holben, B.: Multiangle Imaging Spectroradiometer (MISR) global aerosol optical depth validation based on 2 years of coincident Aerosol Robotic Network (AERONET) observations, J. Geophys. Res.-Atmos., 110, 1–16, https://doi.org/10.1029/2004JD004706, 2005b.
Kahn, R. A., Garay, M. J., Nelson, D. L., Yau, K. K., Bull, M. A., Gaitley, B. J., Martonchik, J. V., and Levy, R. C.: Satellite-derived aerosol optical depth over dark water from MISR and MODIS: Comparisons with AERONET and implications for climatological studies, J. Geophys. Res.-Atmos., 112, D18205, https://doi.org/10.1029/2006JD008175, 2007.
Kahn, R. A., Petzold, A., Wendisch, M., Bierwirth, E., Dinter, T., Esselborn, M., Fiebig, M., Heese, B., Knippertz, P., Müller, D., Schladitz, A., and Von Hoyningen-Huene, W.: Desert dust aerosol air mass mapping in the western Sahara, using particle properties derived from space-based multi-angle imaging, Tellus B, 61, 239–251, https://doi.org/10.1111/j.1600-0889.2008.00398.x, 2009a.
Kahn, R. A., Nelson, D. L., Garay, M. J., Levy, R. C., Bull, M. A., Diner, D. J., Martonchik, J. V., Paradise, S. R., Hansen, E. G., and Remer, L. A.: MISR aerosol product attributes and statistical comparisons with MODIS, IEEE T. Geosci. Remote Sens., 47, 4095–4114, https://doi.org/10.1109/TGRS.2009.2023115, 2009b.
Kahn, R. A., Gaitley, B. J., Garay, M. J., Diner, D. J., Eck, T. F., Smirnov, A., and Holben, B. N.: Multiangle Imaging SpectroRadiometer global aerosol product assessment by comparison with the Aerosol Robotic Network, J. Geophys. Res.-Atmos., 115, D23209, https://doi.org/10.1029/2010JD014601, 2010.
Kahn, R. A., Garay, M. J., Nelson, D. L., Levy, R. C., Bull, M. A., Diner, D. J., Martonchik, J. V., Hansen, E. G., Remer, L. A., and Tanré, D.: Response to “Toward unified satellite climatology of aerosol properties, 3. MODIS versus MISR versus AERONET”, J. Quant. Spectrosc. Ra., 112, 901–909, https://doi.org/10.1016/j.jqsrt.2010.11.001, 2011.
Kalashnikova, O. V., Garay, M. J., Martonchik, J. V., and Diner, D. J.: MISR Dark Water aerosol retrievals: operational algorithm sensitivity to particle non-sphericity, Atmos. Meas. Tech., 6, 2131–2154, https://doi.org/10.5194/amt-6-2131-2013, 2013.
Kalashnikova, O. V., Garay, M. J., Bates, K. H., Kenseth, C. M., Kong, W., Cappa, C. D., Lyapustin, A. I., Jonsson, H. H., Seidel, F. C., Xu, F., Diner, D. J., and Seinfeld, J. H.: Photopolarimetric Sensitivity to Black Carbon Content of Wildfire Smoke: Results From the 2016 ImPACT-PM Field Campaign, J. Geophys. Res.-Atmos., 123, 5376–5396, https://doi.org/10.1029/2017JD028032, 2018.
Kalashnikova, O. V. and Kahn, R.: Ability of multiangle remote sensing observations to identify and distinguish mineral dust types: 2. Sensitivity over dark water, J. Geophys. Res.-Atmos., 111, D11207, https://doi.org/10.1029/2005JD006756, 2006.
Kaufman, Y. J., Herring, D. D., Jon Ranson, K., and James Collatz, G.: Earth observing system AMI mission to earth, IEEE T. Geosci. Remote Sens., 36, 1045–1055, https://doi.org/10.1109/36.700989, 1998.
Kinne, S., Schulz, M., Textor, C., Guibert, S., Balkanski, Y., Bauer, S. E., Berntsen, T., Berglen, T. F., Boucher, O., Chin, M., Collins, W., Dentener, F., Diehl, T., Easter, R., Feichter, J., Fillmore, D., Ghan, S., Ginoux, P., Gong, S., Grini, A., Hendricks, J., Herzog, M., Horowitz, L., Isaksen, I., Iversen, T., Kirkevåg, A., Kloster, S., Koch, D., Kristjansson, J. E., Krol, M., Lauer, A., Lamarque, J. F., Lesins, G., Liu, X., Lohmann, U., Montanaro, V., Myhre, G., Penner, J., Pitari, G., Reddy, S., Seland, O., Stier, P., Takemura, T., and Tie, X.: An AeroCom initial assessment – optical properties in aerosol component modules of global models, Atmos. Chem. Phys., 6, 1815–1834, https://doi.org/10.5194/acp-6-1815-2006, 2006.
Lelieveld, J., Evans, J. S., Fnais, M., Giannadaki, D., and Pozzer, A.: The contribution of outdoor air pollution sources to premature mortality on a global scale, Nature, 525, 367–371, https://doi.org/10.1038/nature15371, 2015.
Levy, R. C., Mattoo, S., Munchak, L. A., Remer, L. A., Sayer, A. M., Patadia, F., and Hsu, N. C.: The Collection 6 MODIS aerosol products over land and ocean, Atmos. Meas. Tech., 6, 2989–3034, https://doi.org/10.5194/amt-6-2989-2013, 2013.
Li, S., Garay, M. J., Chen, L., Rees, E., and Liu, Y.: Comparison of GEOS-Chem aerosol optical depth with AERONET and MISR data over the contiguous United States, J. Geophys. Res.-Atmos., 118, 11228–11241, https://doi.org/10.1002/jgrd.50867, 2013.
Li, Z., Zhao, X., Kahn, R., Mishchenko, M., Remer, L., Lee, K. H., Wang, M., Laszlo, I., Nakajima, T., and Maring, H.: Uncertainties in satellite remote sensing of aerosols and impact on monitoring its long-term trend: A review and perspective, Ann. Geophys., 27, 2755–2770, https://doi.org/10.5194/angeo-27-2755-2009, 2009.
Limbacher, J. A. and Kahn, R. A.: MISR research-aerosol-algorithm refinements for dark water retrievals, Atmos. Meas. Tech., 7, 3989–4007, https://doi.org/10.5194/amt-7-3989-2014, 2014.
Limbacher, J. A. and Kahn, R. A.: MISR empirical stray light corrections in high-contrast scenes, Atmos. Meas. Tech., 8, 2927–2943, https://doi.org/10.5194/amt-8-2927-2015, 2015.
Limbacher, J. A. and Kahn, R. A.: Updated MISR dark water research aerosol retrieval algorithm – Part 1: Coupled 1.1 km ocean surface chlorophyll a retrievals with empirical calibration corrections, Atmos. Meas. Tech., 10, 1539–1555, https://doi.org/10.5194/amt-10-1539-2017, 2017.
Limbacher, J. A. and Kahn, R. A.: Updated MISR over-water research aerosol retrieval algorithm – Part 2: A multi-angle aerosol retrieval algorithm for shallow, turbid, oligotrophic, and eutrophic waters, Atmos. Meas. Tech., 12, 675–689, https://doi.org/10.5194/amt-12-675-2019, 2019.
Liu, L. and Mishchenko, M. I.: Toward unified satellite climatology of aerosol properties: Direct comparisons of advanced level 2 aerosol products, J. Quant. Spectrosc. Ra., 109, 2376–2385, https://doi.org/10.1016/j.jqsrt.2008.05.003, 2008.
Liu, Y., Park, R. J., Jacob, D. J., Li, Q., Kilaru, V., and Sarnat, J. A.: Mapping annual mean ground-level PM2.5 concentrations using Multiangle Imaging Spectroradiometer aerosol optical thickness over the contiguous United States, J. Geophys. Res.-Atmos., 109, 1–10, https://doi.org/10.1029/2004JD005025, 2004.
Liu, Y., Koutrakis, P., Kahn, R., Turquety, S., and Yantosca, R. M.: Estimating fine particulate matter component concentrations and size distributions using satellite-retrieved fractional aerosol optical depth: Part 2 – A case study, J. Air Waste Manage., 57, 1360–1369, https://doi.org/10.3155/1047-3289.57.11.1360, 2007.
Lynch, P., Reid, J. S., Westphal, D. L., Zhang, J., Hogan, T. F., Hyer, E. J., Curtis, C. A., Hegg, D. A., Shi, Y., Campbell, J. R., Rubin, J. I., Sessions, W. R., Turk, F. J., and Walker, A. L.: An 11-year global gridded aerosol optical thickness reanalysis (v1.0) for atmospheric and climate sciences, Geosci. Model Dev., 9, 1489–1522, https://doi.org/10.5194/gmd-9-1489-2016, 2016.
Martonchik, J. V.: Determination of aerosol optical depth and land surface directional reflectances using multiangle imagery, J. Geophys. Res.-Atmos., 102, 17015–17022, https://doi.org/10.1029/96JD02444, 1997.
Martonchik, J. V. and Diner, D.: Retrieval of Aerosol Optical Properties from Multi-Angle Satellite Imagery, IEEE T. Geosci. Remote Sens., 30, 223–230, 1992.
Martonchik, J. V., Diner, D. J., Kahn, R. A., Ackerman, T. P., Verstraete, M. M., Pinty, B., and Gordon, H. R.: Techniques for the Retrieval of aerosol properties over land and ocean using multiangle data, IEEE T. Geosci. Remote Sens., 36, 1212–1227, 1998.
Martonchik, J. V., Diner, D. J., Kahn, R., Gaitley, B., and Holben, B. N.: Comparison of MISR and AERONET aerosol optical depths over desert sites, Geophys. Res. Lett., 31, 1–4, https://doi.org/10.1029/2004GL019807, 2004.
Martonchik, J. V., Kahn, R. A., and Diner, D. J.: Retrieval of aerosol properties over land using MISR observations, in Satellite Aerosol Remote Sensing over Land, Springer Berlin Heidelberg, 267–293, 2009.
Meng, X., Garay, M. J., Diner, D. J., Kalashnikova, O. V., Xu, J., and Liu, Y.: Estimating PM2.5 speciation concentrations using prototype 4.4 km-resolution MISR aerosol properties over Southern California, Atmos. Environ., 181, 70–81, https://doi.org/10.1016/j.atmosenv.2018.03.019, 2018.
Mishchenko, M. I., Liu, L., Geogdzhayev, I. V., Travis, L. D., Cairns, B., and Lacis, A. A.: Toward unified satellite climatology of aerosol properties, J. Quant. Spectrosc. Ra., 111, 540–552, https://doi.org/10.1016/j.jqsrt.2009.11.003, 2010.
Myhre, G., Stordal, F., Johnsrud, M., Diner, D. J., Geogdzhayev, I. V., Haywood, J. M., Holben, B. N., Holzer-Popp, T., Ignatov, A., Kahn, R. A., Kaufman, Y. J., Loeb, N., Martonchik, J. V., Mishchenko, M. I., Nalli, N. R., Remer, L. A., Schroedter-Homscheidt, M., Tanré, D., Torres, O., and Wang, M.: Intercomparison of satellite retrieved aerosol optical depth over ocean during the period September 1997 to December 2000, Atmos. Chem. Phys., 5, 1697–1719, https://doi.org/10.5194/acp-5-1697-2005, 2005.
Nelson, D. L., Garay, M. J., Kahn, R. A., and Dunst, B. A.: Stereoscopic height and wind retrievals for aerosol plumes with the MISR INteractive eXplorer (MINX), Remote Sens., 5, 4593–4628, https://doi.org/10.3390/rs5094593, 2013.
Petrenko, M. and Ichoku, C.: Coherent uncertainty analysis of aerosol measurements from multiple satellite sensors, Atmos. Chem. Phys., 13, 6777–6805, https://doi.org/10.5194/acp-13-6777-2013, 2013.
Petrenko, M., Ichoku, C., and Leptoukh, G.: Multi-sensor Aerosol Products Sampling System (MAPSS), Atmos. Meas. Tech., 5, 913–926, https://doi.org/10.5194/amt-5-913-2012, 2012.
Povey, A. C. and Grainger, R. G.: Known and unknown unknowns: uncertainty estimation in satellite remote sensing, Atmos. Meas. Tech., 8, 4699–4718, https://doi.org/10.5194/amt-8-4699-2015, 2015.
Randles, C. A., da Silva, A. M., Buchard, V., Colarco, P. R., Darmenov, A., Govindaraju, R., Smirnov, A., Holben, B., Ferrare, R., Hair, J., Shinozuka, Y., and Flynn, C. J.: The MERRA-2 aerosol reanalysis, 1980 onward, Part I: System description and data assimilation evaluation, J. Climate, 30, 6823–6850, https://doi.org/10.1175/JCLI-D-16-0609.1, 2017.
Reidmiller, D. R., Hobbs, P. V., and Kahn, R.: Aerosol optical properties and particle size distributions on the East Coast of the United States derived from airborne in situ and remote sensing measurements, J. Atmos. Sci., 63, 785–814, https://doi.org/10.1175/JAS3674.1, 2006.
Russell, P. B., Livingston, J. M., Redemann, J., Schmid, B., Ramirez, S. A., Eilers, J., Kahn, R., Chu, D. A., Remer, L., Quinn, P. K., Rood, M. J., and Wang, W.: Multi-grid-cell validation of satellite aerosol property retrievals in INTEX/ITCT/ICARTT 2004, J. Geophys. Res.-Atmos., 112, 1–29, https://doi.org/10.1029/2006JD007606, 2007.
Sayer, A. M., Hsu, N. C., Lee, J., Kim, W. V., Dubovik, O., Dutcher, S. T., Huang, D., Litvinov, P., Lyapustin, A., Tackett, J. L., and Winker, D. M.: Validation of SOAR VIIRS Over-Water Aerosol Retrievals and Context Within the Global Satellite Aerosol Data Record, J. Geophys. Res.-Atmos., 123, 13496–13526, https://doi.org/10.1029/2018JD029465, 2018.
Sayer, A. M., Govaerts, Y., Kolmonen, P., Lipponen, A., Luffarelli, M., Mielonen, T., Patadia, F., Popp, T., Povey, A. C., Stebel, K., and Witek, M. L.: A review and framework for the evaluation of pixel-level uncertainty estimates in satellite aerosol remote sensing, Atmos. Meas. Tech., 13, 373–404, https://doi.org/10.5194/amt-13-373-2020, 2020.
Schmid, B., Redemann, J., Russell, P. B., Hobbs, P. V., Hlavka, D. L., McGill, M. J., Holben, B. N., Welton, E. J., Campbell, J. R., Torres, O., Kahn, R. A., Diner, D. J., Helmlinger, M. C., Chu, D. A., Robles-Gonzalez, C., and De Leeuw, G.: Coordinated airborne, spaceborne, and ground-based measurements of massive thick aerosol layers during the dry season in southern Africa, J. Geophys. Res.-Atmos., 108, 8496, https://doi.org/10.1029/2002JD002297, 2003.
Schuster, G. L., Dubovik, O., and Holben, B. N.: Angstrom exponent and bimodal aerosol size distributions, J. Geophys. Res.-Atmos., 111, 1–14, https://doi.org/10.1029/2005JD006328, 2006.
Scollo, S., Kahn, R. A., Nelson, D. L., Coltelli, M., Diner, D. J., Garay, M. J., and Realmuto, V. J.: MISR observations of Etna volcanic plumes, J. Geophys. Res.-Atmos., 117, 1–13, https://doi.org/10.1029/2011JD016625, 2012.
Shi, Y., Zhang, J., Reid, J. S., Hyer, E. J., Eck, T. F., Holben, B. N., and Kahn, R. A.: A critical examination of spatial biases between MODIS and MISR aerosol products – application for potential AERONET deployment, Atmos. Meas. Tech., 4, 2823–2836, https://doi.org/10.5194/amt-4-2823-2011, 2011.
Shi, Y., Zhang, J., Reid, J. S., Hyer, E. J., and Hsu, N. C.: Critical evaluation of the MODIS Deep Blue aerosol optical depth product for data assimilation over North Africa, Atmos. Meas. Tech., 6, 949–969, https://doi.org/10.5194/amt-6-949-2013, 2013.
Shi, Y., Zhang, J., Reid, J. S., Liu, B., and Hyer, E. J.: Critical evaluation of cloud contamination in the MISR aerosol products using MODIS cloud mask products, Atmos. Meas. Tech., 7, 1791–1801, https://doi.org/10.5194/amt-7-1791-2014, 2014.
Smirnov, A., Holben, B. N., Sakerin, S. M., Kabanov, D. M., Slutsker, I., Chin, M., Diehl, T. L., Remer, L. A., Kahn, R., Ignatov, A., Liu, L., Mishchenko, M., Eck, T. F., Kucsera, T. L., Giles, D., and Kopelevich, O. V.: Ship-based aerosol optical depth measurements in the Atlantic Ocean: Comparison with satellite retrievals and GOCART model, Geophys. Res. Lett., 33, 1–4, https://doi.org/10.1029/2006GL026051, 2006.
Smirnov, A., Holben, B. N., Slutsker, I., Giles, D. M., McClain, C. R., Eck, T. F., Sakerin, S. M., Macke, A., Croot, P., Zibordi, G., Quinn, P. K., Sciare, J., Kinne, S., Harvcy, M., Smyth, T. J., Piketh, S., Zielinski, T., Proshutinsky, A., Goes, J. I., Nelson, N. B., Larouche, P., Radionov, V. F., Goloub, P., Krishna Moorthy, K., Matarrese, R., Robertson, E. J., and Jourdin, F.: Maritime Aerosol Network as a component of Aerosol Robotic Network, J. Geophys. Res.-Atmos., 114, 1–10, https://doi.org/10.1029/2008JD011257, 2009.
Smirnov, A., Holben, B. N., Giles, D. M., Slutsker, I., O'Neill, N. T., Eck, T. F., Macke, A., Croot, P., Courcoux, Y., Sakerin, S. M., Smyth, T. J., Zielinski, T., Zibordi, G., Goes, J. I., Harvey, M. J., Quinn, P. K., Nelson, N. B., Radionov, V. F., Duarte, C. M., Losno, R., Sciare, J., Voss, K. J., Kinne, S., Nalli, N. R., Joseph, E., Krishna Moorthy, K., Covert, D. S., Gulev, S. K., Milinevsky, G., Larouche, P., Belanger, S., Horne, E., Chin, M., Remer, L. A., Kahn, R. A., Reid, J. S., Schulz, M., Heald, C. L., Zhang, J., Lapina, K., Kleidman, R. G., Griesfeller, J., Gaitley, B. J., Tan, Q., and Diehl, T. L.: Maritime aerosol network as a component of AERONET – first results and comparison with global aerosol models and satellite retrievals, Atmos. Meas. Tech., 4, 583–597, https://doi.org/10.5194/amt-4-583-2011, 2011.
Tosca, M. G., Campbell, J., Garay, M., Lolli, S., Seidel, F. C., Marquis, J., and Kalashnikova, O.: Attributing accelerated summertime warming in thesoutheast United States to recent reductions in aerosol burden: Indications from Vertically-resolved observations, Remote Sens., 9, 674, https://doi.org/10.3390/rs9070674, 2017.
Wagner, F. and Silva, A. M.: Some considerations about Ångström exponent distributions, Atmos. Chem. Phys., 8, 481–489, https://doi.org/10.5194/acp-8-481-2008, 2008.
Witek, M. L., Garay, M. J., Diner, D. J., and Smirnov, A.: Aerosol optical depths over oceans: A view from MISR retrievals and collocated MAN and AERONET in situ observations, J. Geophys. Res.-Atmos., 118, 12620–12633, https://doi.org/10.1002/2013JD020393, 2013.
Witek, M. L., Diner, D. J., and Garay, M. J.: Satellite assessment of sea spray aerosol productivity: Southern Ocean case study, J. Geophys. Res.-Atmos., 121, 872–894, https://doi.org/10.1002/2015JD023726, 2016.
Witek, M. L., Diner, D. J., Garay, M. J., Xu, F., Bull, M. A., and Seidel, F. C.: Improving MISR AOD Retrievals with Low-Light-Level Corrections for Veiling Light, IEEE T. Geosci. Remote Sens., 56, 1251–1268, https://doi.org/10.1109/TGRS.2017.2727342, 2018a.
Witek, M. L., Garay, M. J., Diner, D. J., Bull, M. A., and Seidel, F. C.: New approach to the retrieval of AOD and its uncertainty from MISR observations over dark water, Atmos. Meas. Tech., 11, 429–439, https://doi.org/10.5194/amt-11-429-2018, 2018b.
Witek, M. L., Garay, M. J., Diner, D. J., and Smirnov, A.: Oceanic Aerosol Loading Derived From MISR's 4.4 km (V23) Aerosol Product, J. Geophys. Res.-Atmos., 124, 10154–10174, https://doi.org/10.1029/2019JD031065, 2019.
Xiao, N., Shi, T., Calder, C. A., Munroe, D. K., Berrett, C., Wolfinbarger, S., and Li, D.: Spatial characteristics of the difference between MISR and MODIS aerosol optical depth retrievals over mainland Southeast Asia, Remote Sens. Environ., 113, 1–9, https://doi.org/10.1016/j.rse.2008.07.011, 2009.
Yang, Y., Di Girolamo, L., and Mazzoni, D.: Selection of the automated thresholding algorithm for the Multi-angle Imaging SpectroRadiometer Radiometric Camera-by-Camera Cloud Mask over land, Remote Sens. Environ., 107, 159–171, https://doi.org/10.1016/j.rse.2006.05.020, 2007.
Zhang, J. and Reid, J. S.: A decadal regional and global trend analysis of the aerosol optical depth using a data-assimilation grade over-water MODIS and Level 2 MISR aerosol products, Atmos. Chem. Phys., 10, 10949–10963, https://doi.org/10.5194/acp-10-10949-2010, 2010.
Zhao, B., Jiang, J. H., Gu, Y., Diner, D., Worden, J., Liou, K. N., Su, H., Xing, J., Garay, M., and Huang, L.: Decadal-scale trends in regional aerosol particle properties and their linkage to emission changes, Environ. Res. Lett., 12, 054021, https://doi.org/10.1088/1748-9326/aa6cb2, 2017.
Zhao, G. and Girolamo, L. D.: A cloud fraction versus view angle technique for automatic in-scene evaluation of the MISR cloud mask, J. Appl. Meteorol., 860–869, https://doi.org/10.1175/1520-0450(2004)043, 2004.
- Abstract
- Introduction
- MISR background
- Motivation for V23 aerosol product development
- Major algorithm changes
- Changes to level 3
- Initial evaluation
- Summary and conclusions
- Appendix A
- Data availability
- Author contributions
- Competing interests
- Acknowledgements
- Financial support
- Review statement
- References
- Abstract
- Introduction
- MISR background
- Motivation for V23 aerosol product development
- Major algorithm changes
- Changes to level 3
- Initial evaluation
- Summary and conclusions
- Appendix A
- Data availability
- Author contributions
- Competing interests
- Acknowledgements
- Financial support
- Review statement
- References