the Creative Commons Attribution 4.0 License.
the Creative Commons Attribution 4.0 License.
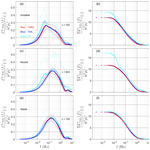
Water vapor density and turbulent fluxes from three generations of infrared gas analyzers
Seth Kutikoff
Xiaomao Lin
Steven R. Evett
Prasanna Gowda
David Brauer
Jerry Moorhead
Gary Marek
Paul Colaizzi
Robert Aiken
Liukang Xu
Clenton Owensby
Fast-response infrared gas analyzers (IRGAs) have been widely used over 3 decades in many ecosystems for long-term monitoring of water vapor fluxes in the surface layer of the atmosphere. While some of the early IRGA sensors are still used in these national and/or regional eco-flux networks, optically improved IRGA sensors are newly employed in the same networks. The purpose of this study was to evaluate the performance of water vapor density and flux data from three generations of IRGAs – LI-7500, LI-7500A, and LI-7500RS (LI-COR Bioscience, Inc., Nebraska, USA) – over the course of a growing season in Bushland, Texas, USA, in an irrigated maize canopy for 90 d. Water vapor density measurements were in generally good agreement, but temporal drift occurred in different directions and magnitudes. Means exhibited mostly shift changes that did not impact the flux magnitudes, while their variances of water vapor density fluctuations were occasionally in poor agreement, especially following rainfall events. LI-7500 cospectra were largest compared to LI-7500RS and LI-7500A, especially under unstable and neutral static stability. Agreement among the sensors was best under the typical irrigation-cooled boundary layer, with a 14 % interinstrument coefficient of variability under advective conditions. Generally, the smallest variances occurred with the LI-7500RS, and high-frequency spectral corrections were larger for these measurements, resulting in similar fluxes between the LI-7500A and LI-7500RS. Fluxes from the LI-7500 were best representative of growing season ET based on a world-class lysimeter reference measurement, but using the energy balance ratio as an estimate of systematic bias corrected most of the differences among measured fluxes.
- Article
(4298 KB) - Full-text XML
- BibTeX
- EndNote
The eddy covariance (EC) method is a standard way to monitor water vapor flux between the surface and atmosphere at most spatial scales and environments, including marine (Honkanen et al., 2018; Takahashi et al., 2005), forest (Novick et al., 2013; Zhang et al., 2012), grassland (Haslwanter et al., 2009; Hirschi et al., 2017), and cropland (Ding et al., 2013; Kochendorfer and Paw, 2011). In water-limited regions, the need to conserve a subsurface source, such as the US Ogallala Aquifer, serves as motivation for agricultural producers to estimate the crop water use for daily irrigation scheduling (Xue et al., 2017). Current crop production involves innovative water-saving measures, such as variable rate irrigation management, requiring high-quality evapotranspiration (ET) data to supplement efforts to calculate the correct amount of water to apply to crops (O'Shaughnessy et al., 2016). In ecosystem networks both large (FLUXNET; Baldocchi et al., 2001), and small (e.g., Delta-Flux; see Runkle et al., 2017), as well as at individual research fields in Texas (Evett et al., 2012a) and California (Oncley et al., 2007), the infrared gas analyzers (IRGAs) built by LI-COR Biosciences, Inc. (Lincoln, Nebraska, USA) have been widely used for over 2 decades to monitor water vapor fluxes.
The accuracy of ET measurements relative to a reference system can be assessed to investigate potential systematic problems with instrumentation (Mauder et al., 2006). Based on this analysis, an open-path, nondispersive infrared gas analyzer (IRGA) has long been selected as the standard fast-response hygrometer for decades after the era of Lyman-alpha and krypton hygrometer absorption sensors (absorption of ultraviolet radiation by water vapor; e.g., Kaimal and Finnigan, 1994). The optical sensor of the IRGA detects water vapor through differential or ratio measurement of infrared transmittance at two adjacent wavelengths with one located in a region of large water vapor absorption and the other where absorption is negligible (Kaimal and Finnigan, 1994). The transmitting path is typically 0.2–1.0 m long, and beams are usually modulated by a mechanical chopper to permit high-gain amplification of the detected signal. Generally, such an optical device is unreliable when air humidity reaches saturation (rainfall or dew) because of liquid water present in optical pathways. The ratio-detecting technique is used to improve the signal-to-noise ratio of water vapor signals and also removes the common noise in the absorption path length. For water vapor detected in all LI-COR 7500 models, the ratio of these measurements determines an estimate of vapor absorptance, which is converted to a concentration or density (absolute humidity), ρv, using a third-order calibration polynomial. Any biases occurring in this absorption, therefore, propagate to ρv measurement errors. Fratini et al. (2014) described contributing factors to this error, including the magnitude of absorptance fluctuations, and showed that drift in the calibration zero of ρv, i.e., the bias, tends to occur in steps rather than in a continuous fashion.
The IRGA specifications for water vapor density measurement ρv,m, including accuracy, precision, and drift, have been unchanged over three models of sensors: LI-7500, LI-7500A, and LI-7500RS. The LI-7500 was first introduced in 1999, followed by the LI-7500A in 2010 and LI-7500RS in 2016. The differences between the LI-7500 and LI-7500A reported by LI-COR primarily address electrical power requirements in cold climate conditions and ease of use. Progressing from the LI-7500A to the LI-7500RS, while no physical differences are evident, optical changes were made to improve the stability of measurements in the presence of window contamination which can cause systematic bias (Heusinkveld et al., 2008). LI-COR reported that ρv,m drift was more than an order of magnitude smaller in the LI-7500RS than the original LI7500A and was accompanied by reduced interinstrument variability (Burba et al., 2018). They also found that after rainfall LI-7500A and LI-7500RS measurements were similar, but agreement lessened after approximately 1 week. As the duration of IRGA deployment increases from weeks to months and years, calibration becomes more important to ensure accuracy for fast-response water vapor measurements since their measurement stability is relatively low (Iwata et al., 2012). The factory calibration procedure, resulting in span and zero coefficients, consists of measured water vapor density being compared to the absorption of water vapor from a dew point generator over a range of temperatures from 17 to 41 ∘C. Based on the manufacturer calibration and re-calibration sheets (after a certain period the IRGA is returned to the manufacturer for re-calibration), the span drift is primarily a function of temperature, whereas the zero drift is chiefly influenced by the measurement range of water vapor density.
In addition to the IRGA, a sonic anemometer is necessary to determine water vapor flux. It should be noted that transducer shadowing effects were heavily investigated during early sonic anemometer development in the 1980s and 1990s. The optimum geometry design minimizes shadowing effects (air flow distortion dynamics and line or path integration) for the sonic anemometer's geometry (e.g., a 120∘ orthogonal geometry), but this pair of instruments introduces systematic error due to their physical separation, which is a source of high-frequency turbulent signal loss (Massman, 2000). The magnitude of flux attenuation is enhanced by lighter wind speed and a greater ratio of horizontal separation to sensing height (Horst and Lenschow, 2009). The expected cospectra, or eddy flux in the spectral domain, can be estimated analytically with a series of transfer functions (Massman, 2000; Moncrieff et al., 1997) that account for signal loss at low and high frequencies. A spectral correction factor can often be determined based on how this modeled cospectrum departs from the measured cospectrum, indicating the degree of flux loss for a given observation period and EC system.
To address offset errors of water vapor density from an IRGA, data are typically compared to another type of sensor. In a comparison to the enclosed-path EC155 system (Campbell Scientific, Logan, UT, USA), errors in water vapor density were generally between −3 and 3 g m−3 (Novick et al., 2013). Such errors were largest in early to mid-morning hours, coinciding with the likely formation of dew and fog, and after bias correction, the linear regression slope and offset were 1.01 and 1.68 g m−3, respectively. In a study involving an LI-7500 and Krypton hygrometer in a semi-arid climate where rainfall is irregular (34.6 mm in three events from approximately 3 weeks of data), flux comparisons were made using simple linear regression (Martínez-Cob and Suvočarev, 2015). With the Krypton hygrometer being unable to measure absolute concentration of water vapor, comparisons of ρv data were not made. In this case, ρv can be calibrated to a sensor explicitly designed to determine absolute humidity. This calibration should be stable (avoid short-timescale errors) and not drift (avoid long-timescale errors). In an environment prone to contamination, the measurement timeframe could be 1–2 weeks (Iwata et al., 2012). Accurate water vapor determination is also crucial in flux processing procedures, specifically to account for air density fluctuations which complicate the effect of error propagation into water vapor flux (Fratini et al., 2014).
Due to the high expense of infrared gas analyzers (IRGAs), there is little research intercomparing multiple instruments except by the manufacturer itself. Historically, instrumentation errors from EC systems average 10 %–20 %, with additional contributions from random errors and a smaller, non-negligible amount from systematic bias (Alfieri et al., 2011). Gas analyzers from the same manufacturer have been shown to differ in short-term drifts (Moncrieff et al., 2004). Here, we assess three generations of LI-7500 instruments in advective field conditions over 90 d by evaluating differences in water vapor density measurements and how those differences impact the estimation of the turbulent exchange of water vapor compared with that measured using a large weighing lysimeter. Flux characteristics and how they deviate over the course of the growing season are also analyzed to determine any advantages one set of water vapor analyzer may have over other models.
2.1 Site description and measurements
The field study was conducted between 16 June (day of the year (DOY) 168) and 13 September 2016 (DOY 257) on the lysimeter field at the USDA-ARS Conservation & Production Research Laboratory, Bushland, Texas (described by Morehead et al., 2019), located in the Texas panhandle (35.19∘ N, 102.09∘ W, 1170 m elevation above sea level). Corn (Zea mays L.) was planted on 10 May, with emergence 11 d later, and thereafter crop height grew steadily during the first part of the study period. From 20 June to 19 July, crop height hc increased nearly linearly from 0.85 m to its peak of 2.30 m. After this point, plants were in their reproductive stage with a decreasing leaf area index trend ensuing. The high ET demand of corn during its development is well known and necessitated irrigation to complement precipitation. In both intensity and frequency, precipitation was erratic (Evett et al., 2019) as typical for a semi-arid climate, which is mostly in the range of 250–350 mm (Gowda et al., 2009; Tolk et al., 2013) during the corn growing season at Bushland.
The EC experiment included three systems consisting of IRGA models LI-7500, LI-7500A, and LI-7500RS, with a sonic anemometer (CSAT3, Campbell Scientific, Inc., Logan, UT, USA), sampling at 20 Hz. Each IRGA outputs CO2, H2O, barometric pressure, and a diagnostic value indicating signal strength and statuses of optical wheel rotation rate, detector temperature, and chopper temperature. The gas analyzers were mounted at a height of 4.6 m above the ground (≥2hc), facing southward with the anemometers situated west of the gas analyzers perpendicular to the dominant (southerly) wind direction. Two systems (EC1 and EC2) were at a tower instrumented with an LI-7500RS, LI-7500A, and CSAT3. The horizontal separation between each gas analyzer and the sonic anemometer was approximately 10 and 20 cm, respectively. This spacing on the same tower is comparable to a recent intercomparison of fluxes from two open-path IRGAs (Polonik et al., 2019). The third system (EC3) affixed on a tower 26 m to the south, had an LI-7500 and CSAT3 separated by 10 cm horizontally. All gas analyzers were approximately 10 cm lower than the sonic anemometers and angled slightly downward in accordance with the manufacturer's recommendation to reduce collection of water droplets and contamination on the lens. Both towers had reference ρv data from an air temperature–humidity probe (HMP 155A, Vaisala, Helsinki, Finland) containing a capacitive-type humidity sensor (HUMICAP 180R, Vaisala, Helsinki, Finland). Ancillary data were taken of net radiation Rn (NR-LITE2, Kipp & Zonen, Delft, the Netherlands) at 2.6 m above ground, soil heat flux G (HFT-3.1, Radiation and Energy Balance Systems, Seattle, WA, USA) at 8 cm below ground, and soil heat storage at 2 and 6 cm below ground with thermistors and water-content reflectometers (CS655, Campbell Scientific, Inc., Logan, Utah, USA) (Kutikoff et al., 2019).
2.2 Data processing and statistical analysis
Water vapor density data among the three infrared open-path IRGAs were compared in a fashion similar to Mauder et al. (2006). The following characteristics of variance () and covariance () were of interest: regression intercept (a), slope (b), coefficient of determination (r2), root-mean-square deviation (rmsd), and bias (d). Comparability between LI-7500RS and the other two models was found using rmsd, defined as
where xA,i is the ith observation for the LI-7500/A and xREF,i is the ith observation for the reference. In one case, when evaluating sensor drift, the reference is the average of three IRGAs or three EC systems, and in another case, for instrument intercomparison, the reference is the latest sensor, LI7500RS. Interinstrument variability was also determined by rmsd except using the average of three IRGAs or three EC systems as a reference value. For fluxes, interinstrument variability was expressed relative to flux magnitude using the coefficient of variation (CVI-I). The data de-spiking process set all data beyond the upper (30 g m−3) and lower (2 g m−3) values by missing. Both upper and lower bounds were estimated by using ±3.5 standard deviations of a 5 min moving window with a half window overlapped in water vapor density time series in Bushland, Texas. Additionally, while the LI-7500A and LI-7500 were calibrated in 2014 and 2015, a correction to these data was made based on a factory calibration after data were collected. Otherwise, no additional conditioning was performed on the raw data. Given the interest in sensor sensitivity, comparisons were also made between collocated HMP155A and IRGA(s) at each tower, which were assumed to be sensing identical air parcels containing equal water vapor density.
To further ascertain the performance of IRGAs, (co)spectral density of ρv (wρv) measurements was calculated for each of three EC systems using Welch's periodogram method (Blanken et al., 2003). The distribution of power across frequencies, particularly signal loss at high frequencies, can indicate differences in flux characteristics with an expectation that latent heat would be underestimated. Of particular interest are results from an advective environment in which high-frequency variation is enhanced (Prueger et al., 2012). This condition was defined by finding half-hour observations between 10:00 and 18:00 LST in which latent heat exceeded available energy (a difference between net radiation and soil heat flux) or sensible heat flux was significantly negative (< = −10 W m−2) (Kutikoff et al., 2019). Such advection air, usually dry and warm, flowing from adjacent areas to irrigated crop fields is typically the driving force of enhanced daytime latent heat fluxes, especially during the afternoon, which may not be fully captured by EC systems and thereby cause reduced energy balance closure. Data were conditioned by linear detrending on half-hour (36 000 points) segments (Zhang et al., 2010). Spectral density () was calculated across these segments with a Hamming window length of 360 and overlap of 180 observations. Then the spectra were averaged into 100 evenly spaced bins on the logarithmic scale. The same procedure was repeated for the cospectra of vertical velocity and water vapor density, indicating the behavior of water vapor flux in the spectral domain. Finally, ogives were calculated to summarize differences in cospectra across wavelengths by integrating the cospectra from low-frequency energy to high-frequency energy on a scale from 0 to 1. The (co)spectra and ogives were multiplied by the frequency and normalized by mean (co)variance to make the data dimensionless.
After examining raw variances and covariances, water vapor fluxes (E) were processed using EddyPro (v6.2.0) software (LI-COR Bioscience, Lincoln, Nebraska, USA) for half-hour averaging periods when availability of data exceeded 90 % (w and ρv were recorded for at least 32 400 of 36 000 possible observations). Prior to computing fluxes, a statistical screening of time series data was implemented. Spikes were detected using the median absolute deviation for each half hour (Mauder et al., 2013) and replaced with the half-hour mean of non-outlier observations. Then data were detrended by block average, and corrections were made to account for sensor separation, tilt of the sonic anemometer via double rotation (Fratini and Mauder, 2014), and spectral energy loss in both low (Moncrieff et al., 2004) and high (Moncrieff et al., 1997) frequency ranges. The original water vapor flux was multiplied by the spectral correction factor of before adding Webb–Pearman–Leuning (WPL) density fluctuation terms (Kaimal and Finnigan, 1994). Sensible heat (H) was then corrected for humidity effects that arise from using sonic temperature in place of air temperature (Van Dijk et al., 2004). Finally, this corrected H was multiplied by its spectral correction factor, and the WPL term was added to the corrected water vapor flux to create a final E or λE. Approximately 13.5 % of available data were removed through the results of steady-state and fully developed turbulence tests (Mauder and Foken, 2004). The acquisition ratio of each half hour was obtained by dividing the count of non-filtered fluxes by the maximum number of observations (Kim et al., 2015).
Intercomparison of λE and its systematic error (δ) and random uncertainty (ε) components was conducted on half-hourly and daily timescales. The measured λE is assumed to be the difference between the actual flux and these errors. Systematic error can be evaluated in the context of surface energy balance, such that δ is zero when turbulent flux equals the available energy measured through solar radiation, ground heat flux, and heat storage during a given period (Mauder et al., 2013). The estimate of systematic error is then
and
where the terms in the numerator are independent (H is sensible heat flux, and λE is latent heat flux) for each EC system, and those in the denominator are shared among the EC systems. J was calculated as the sum of soil and photosynthesis heat storage since the other components of heat storage contribute negligibly to instantaneous energy balance in this ecosystem (Kutikoff et al., 2019). Random error associated with sampling was quantified with the method of Finkelstein and Sims (2001), which calculates the variance of the covariance using the raw time series data for each averaging period. Together, error quantification can indicate whether half-hour fluxes from the three EC systems statistically differ for half hours in which turbulent flux measurements are reliable.
Water vapor flux was compared using the equivalent total water depth ET for daily totals. Gap filling, following Reichstein et al. (2005), was done for half hours that were flagged for any of the three EC systems based on steady-state and developed turbulence tests (Mauder and Foken, 2004), occurrence of precipitation, and high relative humidity (RH > 95 %). Total gap-filled ET was close to the sum of the half-hour observations, with approximately a 3 % greater flux for each EC system. Flux accuracy of the three EC systems was assessed in relation to a large weighing lysimeter, which has an accuracy of 0.05 mm h−1 (Evett et al., 2012b, 2019). Located within 30 m of the EC system, lysimeter ET was computed using a soil water balance approach from a subsection of the same field. Briefly, the mass change of water measured by the weighing lysimeter was calculated and converted into a flux based on the surface area of the lysimeter and density of water. Description of the lysimeter data can be found in Moorhead et al. (2017, 2019).
The findings of the study are presented in three subsections, including water vapor density mean and fluctuations, spectra and cospectra, and fluxes. All were influenced by irrigation and precipitation events. Water added to the field included 498 mm from 33 separate subsurface drip irrigations (SDIs) (Evett et al., 2019) and 238 mm of precipitation (Evett et al., 2018), consistent with an average growing season (Gowda et al., 2009; Tolk et al., 2013). However, much of that rainfall (88 %) occurred after 1 August and, combined with crop maturity, eliminated the need for irrigation after 18 August.
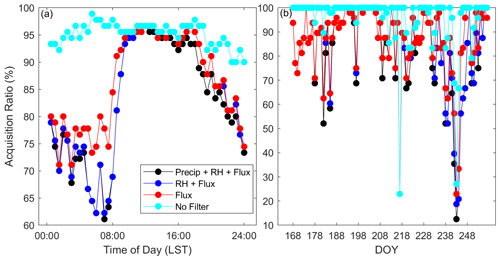
Figure 1Screening effects on the data acquisition ratio (AR) as a function of (a) diel cycle and (b) day of year. Precip + RH + Flux shows AR after all filtering has been completed, RH + Flux indicates AR after RH threshold and steady-state turbulence tests, and Flux denotes AR after only turbulent tests.
Data filtering also impacted all comparisons. After all threshold and precipitation screenings, 3577 out of a possible 4320 half-hour observations are available for analysis. The acquisition ratio was comparable to similar studies (Wu et al., 2015). Between 09:00 and 21:00 (LST), the ratio exceeded 92 %, whereas EC system issues reduced availability in the predawn hours to as low as 61 % for the half hour ending at 07:00 (Fig. 1).
3.1 Water vapor density validation
The long-term zero drift of water vapor density for the three IRGAs was evaluated as the 3-month change in bias Δρv. As the study period began, the reference value of water vapor density ρv,r ranged from 3 to 18 g m−3. Accordingly, the measured values ρv,m for the LI-7500 and LI-7500RS were biased low and the LI-7500A was biased high. After applying the post-correction to the LI-7500 and LI-7500A data, all ρv,m values were between 0.11 and 1.31 less than ρv,t (Fig. 2). At the end of the study period, all IRGAs clearly showed an increased bias relative to the HMP155. Interestingly, the LI-7500 and LI-7500A had moved towards larger values, whereas the LI-7500RS moved towards smaller values (Fig. 2). That resulted in the LI-7500 Δρv decreasing, whereas the other two newer analyzers ended with greater Δρv. The magnitude of bias was larger for the LI-7500 and LI-7500A than the LI-7500RS, and a similar degree of day–night variability (sensitivity to solar radiation) was apparent among the IRGAs regardless of ρv. These temporal patterns may indicate a low-frequency modulated signal hidden in the instruments.
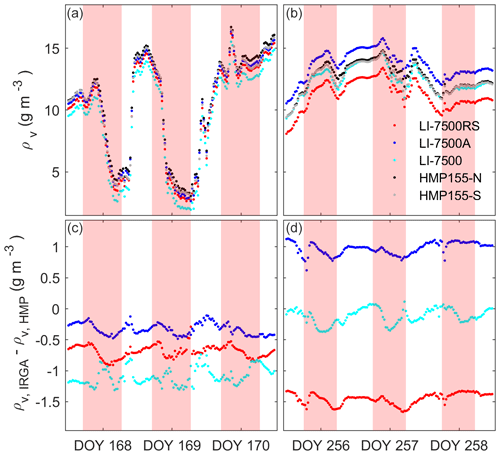
Figure 2Absolute humidity ρv (a, b) magnitude and (c, d) difference ρv between paired IRGA and HMP instruments (HMP155-N is paired with the LI-7500RS and LI-7500A; HMP155-S is paired with the LI-7500) during the first and last 3 d of the study. Shaded areas indicate daytime.
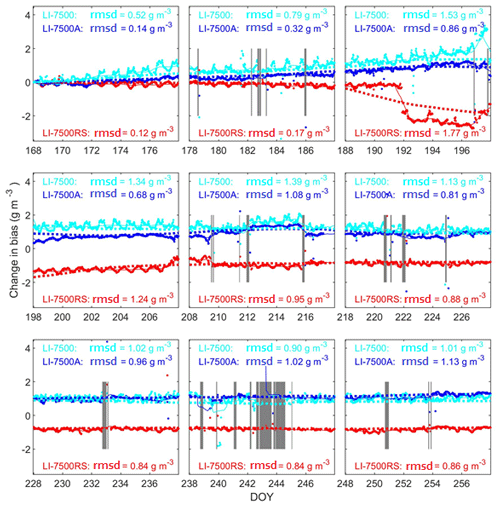
Figure 3Evolution of absolute humidity bias over nine 10 d periods, shown as half-hour bias ρv (points) and 1 d (thin solid lines) and 10 d (dotted lines) moving averages. Half hours with observed rainfall are indicated with vertical lines.
The divergence of ρv,m between early and late times in the study period is the result of many short-term changes in bias. To assess short-term drift Δρv, half-hour differences between LI-7500s and HMP155s were calculated, with each time series bias corrected to set the initial value to zero (Fig. 3). The magnitude of daily drift averaged 0.09 g m−3 for the LI-7500RS, 0.1 g m−3 for the LI-7500A, and 0.13 g m−3 for the LI-7500. Over 10 d periods, this drift increased to 0.36, 0.27, and 0.29, respectively. Rainfall contributed to the bulk of changes in drift. Rain-free periods as noted over the initial 10 d, gave the best insight into the stability of the sensors and suggested that the LI-7500RS performed best. However, the extended dry period between DOY 186 and DOY 196 suggested the opposite, when the LI-7500RS suffered from large short-term drift. After this time, the LI-7500RS appeared to be more stable, with a steady rmsd over the final 50 d compared to the other two instruments.
According to Figs. 2 and 3, analyzer performance differed between day and night. This diel cycle is indicative of a solar-radiation-induced error (Mauder et al., 2006; Miloshevich et al., 2009), and although amplitude varies, it appeared most prominently for the LI-7500 and least substantially for the LI-7500A. Periods with more instrument drift were coincident mainly with larger cycles, but the sudden performance change of the LI-7500RS on DOY 191 did not reflect this tendency. Accidental window contamination may explain this observation, with typical behavior of absolute humidity from the LI-7500RS resuming from DOY 192 onward.
To investigate the unexpected large drift exclusive to the LI-7500RS on DOY 191, biometeorological data were assessed. Light southerly winds and moderately humid conditions were observed when Δρv,RS increased from −0.96 to −2.45 between 20:30 and 21:30 LST. While nothing unusual occurred meteorologically, a 3 ∘C drop in temperature and 10 % increase in RH accompanying the loss of daytime heating were noted. It was instructive to look at the variation in RH as estimated using vapor and ambient pressure from the IRGAs and sonic temperature from the CSAT3. While the magnitude of RH did vary slightly among the sensors, the increase in RH was similar for the LI-7500 and LI-7500A while being less than half for the LI-7500RS. In the hours immediately prior to and after, the slopes of Δρv,RS among the IRGAs and HMPs were nearly in lockstep. Unlike other deviations that exist on a subdaily timescale, this new offset continued until DOY 197. Step changes are a dominant feature in the linear regression between and ρv,75RS.
Differences between the means and fluctuations of ρv are summarized in Fig. 4 as a function of day of year. Since variance of the ρv time series reflects the mean of squared fluctuations , greater variance in the half-hourly data reflects larger fluctuations . While the LI-7500 tended to have consistently greater values, the comparison between the LI-7500A and LI-7500RS was more complicated. For example, the LI-7500A initially had slightly larger or the same fluctuations as the LI-7500RS for most daytime observations. After noon on DOY 196, the LI-7500RS consistently began to have larger fluctuations. Then from midday on DOY 226 to DOY 232 noon, the pattern flipped again. Following DOY 232, agreement was consistently close until DOY 254, and greater fluctuations from the LI-7500A were again found through the remainder of the study period. Even when the LI-7500RS fluctuations tended to be relatively large, it did not have the large overestimation of fluctuations observed periodically with the LI-7500A, such as noted on DOY 184, 190, 193, 211, 216, and 253. While the stochastic nature of turbulence is partially responsible for the large scatter in shown in Fig. 4, the degree of variance in the older sensors exceeded that of the LI-7500RS.
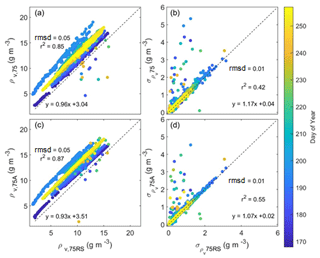
Figure 4Intercomparison of absolute humidity ρv means and standard deviations for (a, b) the LI-7500 and LI-7500RS and (c, d) the LI-7500A and LI-7500RS.
Agreement between ρv of the LI-7500RS and the older IRGAs was generally strong and stable despite occasional large errors. In the first week of the study, regardless of the absolute error, linear regression parameters indicated well-calibrated measurements for the purpose of eddy covariance, in which offset has no effect on the statistic. During the middle 30 d of the study, agreement was also high, reflected by r2 values of 0.94 and 0.97 and slopes of 0.98 and 0.93, respectively, for LI-7500 and LI-7500A. Little change from those parameters occurred across a wide range of ρv values during the final 30 d of the study, when lower temperature and higher relative humidity reduced evaporative demand. As expected, greater comparability in ρv was accompanied by a small error. However, while step changes in ρv occurred, did not change over time.
Variance of water vapor density was compared using the LI-7500RS as reference, for the entire dataset including daytime and advective periods only (Table 1). Nighttime estimates were particularly prone to overestimation by the LI-7500. Advective periods were prone to greater errors while having reduced interinstrument variability.
3.2 Spectra and cospectra
Since the three analyzers had the same specifications and were configured to measure turbulence in the same fashion, any deviations in spectral characteristics would be an indication of possible drift. Returning to the distinct LI-7500RS error on DOY 191, spectra were examined during the interval from 20:00–21:30 (LST), which consisted of three spectra corresponding to consecutive flux averaging periods. Overall, as evident from Fig. 5a–c, the shapes of spectra were in close agreement during the daytime, whereas the nighttime peak frequency was shifted to lower frequencies, indicating the predominance of large eddies after sunset. At 20:00, the three spectra were nearly identical and matched the predicted slope (Fig. 5d). In the following hour, the spectra of the LI-7500A and LI-7500 remained nearly identical, whereas the LI-7500RS spectra were greatly modified. Based on the 20 Hz time series, air humidity began to decrease suddenly at roughly 20:40 in concert with a doubling of fluctuation amplitude. As the other two IRGAs and HMPs continued to indicate increasing air humidity, Δρv,LI-7500RS steadily rose for nearly 1 h until ρv,LI-7500RS again agreed with the other instruments. Because only the averaging period between 21:00 and 21:30 is affected by increased variance water vapor, the spectrum corresponding to that half hour is the period with a shift towards higher frequencies.
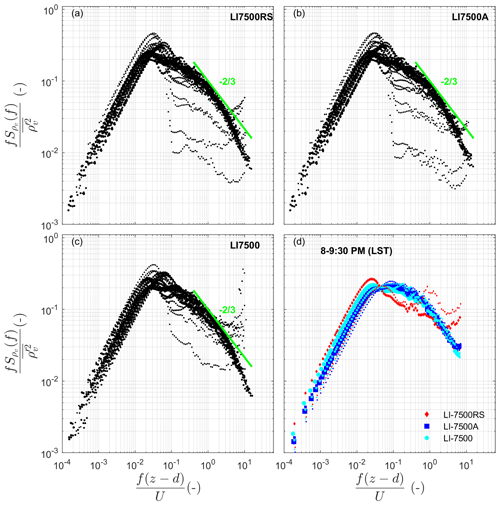
Figure 5Binned spectra of absolute humidity on DOY 191 are shown for 45 half-hour observations from (a) LI-7500RS, (b) LI-7500A, and (c) LI-7500 as a function of normalized frequency. A close-up comparison of the performance of the three gas analyzers is illustrated in (d) for three half hours.
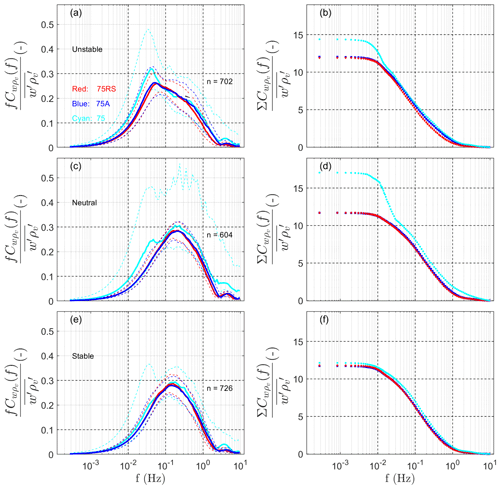
Figure 6Ensemble median daytime (a, c, e) cospectra and corresponding (b, d, f) ogives under unstable, neutral, and stable conditions. For cospectra, the area between dotted lines shows the interquartile range.
Cospectra were viewed through the lens of atmospheric stability because it predicts their shape according to Monin–Obukhov similarity theory (Kaimal and Finnigan, 1994). For all cospectra, the LI-7500 tends to have greater energy in the production and dissipation spectral regions while being nearly identical in the inertial subrange, and these differences translate into higher latent heat fluxes (Fig. 6). Lower-frequency components of flux were clearly greater, especially in unstable and neutral conditions, as observed by the LI-7500 (the oldest version), compared to the LI-7500A and LI-7500RS. While the two newer sensors exhibited similar behavior and relatively smaller fluxes than the LI-7500, under unstable conditions the LI-7500RS showed a difference in performance from the LI-7500A at high frequencies. For all three IRGAs, cospectra dipped at 2.5 Hz, which should not occur in any desired instruments (Kaimal and Finnigan, 1994). Strong turbulent motions were likely captured more by the LI-7500A within the surface layer. These cospectra were shifted towards a lower frequency compared to those in neutral and stable conditions, favoring larger eddy sizes with a smaller percentage of energy accumulated in the inertial subrange (Fig. 6b). This middle frequency range is where the IRGAs were most similar. Regardless of sensor, unstable conditions featured a flattened peak and more energy towards lower frequencies, as expected for various scalar fluxes measured with the same instrumentation (Wolf and Laca, 2007). However, in an irrigated cropland environment, the surface layer is prone to become stable more often than the surrounding area due to a temperature inversion forced by the relatively wetter, cooler canopy. A previous study demonstrated this effect by using simultaneous sensing over adjacent irrigated cotton and non-irrigated winter wheat fields, where energy production as depicted by was 2 orders of magnitude smaller for the irrigated field than the non-irrigated field (Prueger et al., 2012). Accordingly, in the present study, variability among cospectra was small under these conditions with relatively few large eddies (Fig. 6e). In contrast, under neutral and unstable conditions, the LI-7500 departed largely from the other two sensors with energy contribution from low-frequency eddies.
3.3 Water vapor fluxes
For much of the study period, λE values from the LI-7500RS and LI-7500A were similar with slightly larger magnitude than the LI-7500. Overall interinstrument variability CVI-I of λE was 20 %, about that of the underlying water vapor variance, and errors on average were less during daytime hours than nighttime (Table 2). For an average diel cycle, the largest CVI-I occurred during the middle of the night, rapidly declined after sunrise, reached its smallest value of 10 % at 16:00, and then increased at a relatively slow rate after sunset. On a seasonal basis, there was a slight, nonlinear increase in CVI-I over time, with mean values increasing from approximately 16 % to 24 %. Overall, the LI-7500 measured a 15 % greater flux than the LI-7500RS both on average and during only daytime hours. Meanwhile, LI-7500A and LI-7500RS fluxes were nearly identical, with 0.5 % less flux measured by the LI-7500A and an additional 0.2 % difference during the daytime. While the daily bias was as equally positive as negative, the LI-7500A tended to underestimate flux through the first and last thirds of the study period, although possible rainfall effects exist. Greater flux was observed on 27 of the 41 d from DOY 196–226, which coincided with greater accumulated ET (Fig. 7). Relative error varied little by time of day. An increase in variability during advective conditions was due to greater mean (co)variance. Under advective conditions, the coefficient of determination was particularly small (see Table 2), but this advection coincided with large turbulent fluxes including downward sensible heat that was also slightly biased towards increased magnitude.
Table 2Performance characteristics of LI-7500A and LI-7500 with reference to the LI-7500RS for corrected latent heat fluxes (λE). These include regression offset value (a), regression slope (b), coefficient of determination (r2), mean absolute bias (d), and comparability (rmsd).
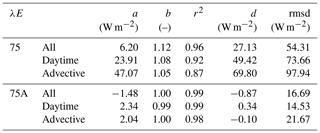
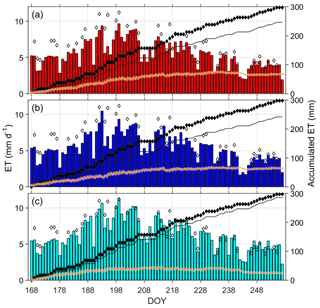
Figure 7Daily ET determined with (a) LI-7500RS (red), (b) LI-7500A (blue), and (c) LI-7500 (cyan). The daily lysimeter ET is displayed by open diamond markers. Accumulated lysimeter ET is shown with solid diamonds and accumulated eddy covariance ET measurements with solid lines. Accumulated daily residual energy is in orange circles. Final accumulated energy balance residuals, computed by subtracting eddy covariance ET from the other major energy balance terms () of each day, for these EC systems (mm) are 66.6, 63.3, and 20.0.
The 90 d ET (Fig. 7) was in good agreement among the three IRGAs, with slightly greater seasonal flux from the LI-7500, consistent with the larger variance in the time series of ρv. Systematic underestimation of ET for all IRGAs is consistent with advective conditions, especially in the earlier part of the growing season where the gap in daily ET is particularly large for a similar magnitude of ET (Fig. 7). Even if all spectral loss is corrected for, based on the conservation of water vapor and eddy covariance theory, the measured EC flux should be less than the true flux under advective conditions. Approximately 16 % of accumulated ET was underestimated from LI-7500A or LI-7500RS relative to the accumulated lysimeter ET at the end of the growing season (Fig. 7). However, only less than 5 % of accumulated ET was underestimated from the oldest LI-7000 analyzer (Fig. 7). Furthermore, the EC and lysimeter should differ more with increasing mean ET because the advective component of ET, not captured by EC systems, is more likely to be elevated (Alfieri et al., 2012).
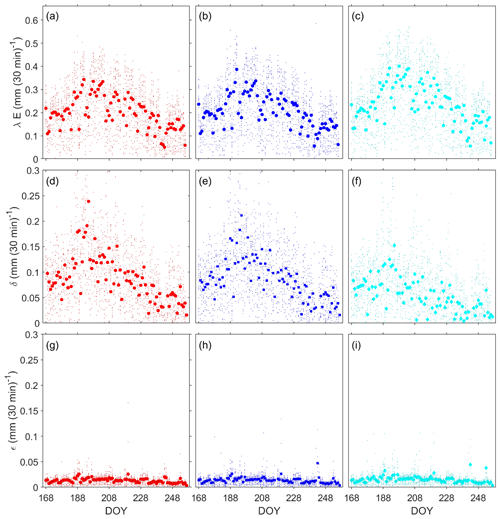
Figure 8Daytime (09:00–19:00 LST) ET fluxes for EC systems with (a) LI-7500RS, (b) LI-7500A, and (c) LI-7500 and the accompanying systematic errors (d–f) and random errors (g–i). Mean values are displayed as larger points.
The greater flux from the LI-7500 occurs nearly symmetrically on a diel basis, with relative differences being smallest during the day. The mean daytime error of measured flux λE between the LI-7500A and LI-7500RS systems was 4.5 %, with the LI-7500A estimating greater ET than the LI-7500RS on approximately 3 out of every 4 d. Systematic error δ averaged 0.08 mm for the LI-7500RS system, which is rather large considering the mean measuring flux of 0.2 mm. Larger systematic error is typically associated with greater flux underestimation due to failure to capture all low-frequency signals, consistent with the observed cospectra (Vickers and Mahrt, 1997). In contrast, daily λE differed by 18.6 % between LI-7500 and LI-7500RS systems, and the magnitude from the LI-7500RS only exceeded that of the LI-7500 on a single day. Comparing daily ET as a function of error, systematic error calculated as shown in Eq. (2) decreases during the study period consistent with declining ET (Fig. 8). Random error ε was overwhelmingly similar among the sensors, indicating that uncertainty due to sampling has little effect on differences in estimated ET.
4.1 Water vapor variance errors
Water vapor variance and flux were compared from three generations of IRGAs yielding similar results in rain-free periods. However, large ρv errors occurred under relatively small flux conditions, primarily with the LI-7500A systems. A pattern of increasing flux error corresponding with greater water vapor density error as observed by Fratini et al. (2014) was not found. These flux results are encouraging despite demonstrated substantial errors in the water vapor density measurements because the (co)variance of the water vapor density is more important for the flux quantity. Overestimation of water vapor variance could contribute to overestimated flux but is not necessarily the case (Mauder et al., 2006). For example,, the LI-7500 underestimated flux by approximately 20 W m−2 despite an overestimation of (3.5 and 0.84 g2 m−6 greater relative to LI-7500A and LI-7500RS) during the half-hour beginning at 15:00 LST on DOY 181.
In a vast majority of cases, large values were observed with both the LI-7500 and LI-7500A relative to the LI-7500RS and were associated with a recent rainfall event. A large discrepancy in among the three IRGAs occurred an hour after light rain on DOY 211, which suggests that thick water droplets may have been still evaporating from the mirror surface. Antecedent conditions were dry, and with the cessation of precipitation, a sudden increase in mean wind speed from under 3 to 5 m s−1 and a wind shift from east to south enabled sensible heat advection as clouds began to dissipate. Although air humidity decreased by the end of the half hour for all IRGAs, the magnitude measured by the LI-7500 was much smaller at the start of the averaging period than at the end, in contrast to observations by the LI-7500A and LI-7500RS. Further, we observed that the LI-7500A air humidity began decreasing within the first 15 min, suddenly increased by approximately 5 g m−3, and then began a rapid decrease. This pattern is different from what was observed by the LI-7500RS, which initially increased and then quickly decreased at an earlier time than for the LI-7500A (not shown).
A similar event occurred on DOY 196. However, for the half hour of interest, a relatively small difference in of the LI-7500 and LI-7500A resulted in a larger flux difference, in which a large, likely overestimated flux was measured by the LI-7500. Interestingly, 20 Hz fluctuations for all systems were dampened during roughly the first half of this averaging period, showing signs of low-frequency atmospheric motion. Once turbulence became more typical of a well-mixed boundary layer, the amplitude of then grew with a larger variance noted in the LI-7500A and LI-7500 compared to the LI-7500RS. This behavior resembles the observations on DOY 211 during its relevant averaging period.
4.2 Water vapor flux errors
The water vapor flux is generally not affected by the three IRGA's zero drifts (or biases) of water vapor density. The WPL corrections for water vapor flux are dependent upon on the covariance terms but not upon mean water vapor densities from three IRGAs because the mean water vapor density is determined by the slow response sensors of HMP155. However, notice that the CO2 flux is certainly affected by IRGA's CO2 zero drifts because WPL correction for CO2 flux requires the mean CO2 density from IRGA measurements. In the context of ET measurement, total daily magnitude is of prime importance for practical applications. Therefore, flux errors during the daytime, roughly between 09:00 and 17:00 LST, contribute to the vast majority of ET variation. The similarity between the LI-7500A and LI-7500RS fluxes is reflected by the lack of scatter in covariance data. As expected, errors were larger during advective periods than for other times, but overall correlation between and λE errors was weak. Highly advective conditions have been associated with large interinstrument variability (Alfieri et al., 2011).
Uncorrected fluxes were assessed to assure that the data processing steps did not appreciably affect our findings. Post-processing of turbulent fluxes could increment fluxes while causing greater error (Irmak et al., 2014). The magnitudes of a, d, and rmsd were slightly smaller for all comparisons, and b and r2 were nearly identical, indicating that the corrections contributed little to measurement uncertainty. For instance, the rmsd decreased by 6.8 % and 7.3 % for daytime fluxes against the LI-7500 and LI-7500A, respectively. Among the corrections, sensor separation and frequency response were of most interest for the LI-7500RS and LI-7500A pair since they are newer optical analyzers. These findings may be why, among the three generations of IRGAs, the LI-7500RS consistently had a larger spectral correction factor by approximately 2 % to 4 %, but again, this served to only slightly decrease flux error. Its midday mean value of 1.11, though slightly larger than for the LI-7500 and LI7500A, was still less than reported in a feedlot for an LI-7500 and CSAT-3 EC system (Prajapati and Santos, 2017). This suggests that high-frequency attenuation was relatively minor when turbulent intensity was large, and any missing flux was more attributable to low frequency. While the LI-7500 high-frequency energy compared more favorably to the LI-7500A than the LI-7500RS, a large departure from the LI-7500A and LI-7500RS pattern was clearly observed at low frequencies (Fig. 6).
It has previously been shown that turbulent flux error partitions primarily into random error, with daytime systematic error only as large as 0.018 mm (30 min−1) (Alfieri et al., 2011). In contrast, Sect. 3.3 demonstrated that the magnitudes of systematic error were generally large in response to daytime energy balance residuals. The different findings are based on different assumptions of what is true latent heat. In the prior study, the mean of multiple EC measurements was considered the true flux, and the systematic error was the variance of residuals between predicted and true flux. Following that approach, daytime error was comparable and ranged from 0.014 (LI-7500RS) to 0.024 mm (LI-7500) (30 min−1). Also, the prior study was conducted during the period of rapid leaf area index (LAI) increase in a cotton crop, while the present study was performed during both the period of rapid LAI increase and crop maturation.
The guidelines written by Fratini et al. (2014) can be used to avoid water vapor concentration errors. Even in the event that absorptances are not output via data logger code, and detection of contamination in real time is not done, the water vapor density errors will not adversely affect accuracy of eddy covariance on a growing season timescale. The averaged water vapor density from LI-7500RS was drifted in an opposite direction of both LI-7500A and LI-7500 analyzers' drifts. For the latent heat flux, larger fluxes were found from the older LI-7500 system evidenced not only from low-frequency energy components but also from high-frequency components under unstable conditions. Our study suggests that the LI-7500 outperformed newer LI7500A and 7500RS sensors in terms of accumulated ET comparison with lysimeter observations. While it was paired with a different sonic anemometer than the other two IRGAs, flux differences were attributed to differences in variance of turbulent fluctuations of water vapor rather than sonic anemometer error.
Differences in the response from the same model sensor measuring presumably the same air parcel were identified. In this study, the growth and maturation of the corn crop drove a change in turbulent flux partitioning. Increases in interinstrument variation for both water vapor variance and flux were observed when conditions were advective during the period of peak canopy development. Following precipitation, while performance characteristics were consistent in well-mixed turbulent air, larger interinstrument variation was observed under light winds that could cause variation in effects on the IRGA. Adjusting measured fluxes by the systematic error, which tended to be larger at one EC tower compared to the other, brought the water vapor fluxes into strong agreement.
MATLAB code is available upon request. Eddy-Pro eddy covariance data analysis software is available at https://www.licor.com/env/support/EddyPro/software.html (last access: 16 Feburay 2021).
Turbulent flux and observational data are available upon request.
SK and XL designed the experiments, analyzed the data, and wrote the manuscript. JM and GM handled in-season instrumentation. RA, PG, PC, SRE, DB, CO, LX, JM, and GM participated in data analysis and discussion and revised the manuscript.
The authors declare that they have no conflict of interest.
It is not our intention to favor any particular instrument and authors only present evidence-based scientific results. There were no financial implications for this study – LI-COR did not fund anything related to our findings.
We thank the collaborative scientists and staff in Bushland for their courtesy in allowing access to the experimental site. The anonymous peer reviews provided feedback that improved the quality of this paper. We also appreciate the detailed editing by Dallas Staley on a previous version of the paper.
This research has been supported by the U.S. Department of Agriculture, Agricultural Marketing Service (grant no. 58-3090-5-009) and the U.S. Department of Agriculture, National Institute of Food and Agriculture (grant no. 2016-68007-25066).
This paper was edited by Jun Wang and reviewed by two anonymous referees.
Alfieri, J. G., Kustas, W. P., Prueger, J. H., Hipps, L. E., Chávez, J. L., French, A. N., and Evett, S. R.: Intercomparison of nine micrometeorological stations during the BEAREX08 field campaign, J. Atmos. Ocean. Tech., 28, 1390–1406, 2011.
Alfieri, J. G., Kustas, W. P., Prueger, J. H., Hipps, L. E., Evett, S. R., Basara, J. B., Neale, C. M. U., French, A. N., Colaizzi, P., Agam, N., Cosh, M. H., Chavez, J. L., and Howell, T. A.: On the discrepancy between eddy covariance and lysimetry-based surface flux measurements under strongly advective conditions, Adv. Water Resour., 50, 62–78, 2012.
Baldocchi, D., Falge, E., Gu, L., Olson, R., Hollinger, D., Running, S., Anthoni, P., Bernhofer, C., Davis, K., Evans, R., and Fuentes, J.: FLUXNET: A new tool to study the temporal and spatial variability of ecosystem-scale carbon dioxide, water vapor, and energy flux densities, B. Am. Meteorol. Soc., 82, 2415–2434, 2001.
Blanken, P. D., Rouse, W. R., and Schertzer, W. M.: Enhancement of evaporation from a large northern lake by the entrainment of warm, dry air, J. Hydrometeorol., 4, 680–693, 2003.
Burba, G. G., Begashaw, I., and Kathilankal, J.: New open-path low-power standardized automated COH2O flux measurement system, European Geosciences Union, Vienna, Austria, 2018.
Ding, R., Kang, S., Vargas, R., Zhang, Y., and Hao, X.: Multiscale spectral analysis of temporal variability in evapotranspiration over irrigated cropland in an arid region, Agr. Water Manage., 130, 79–89, 2013.
Evett, S. R., Agam, N., Kustas, W. P., Colaizzi, P. D., and Schwartz, R. C.: Soil profile method for soil thermal diffusivity, conductivity and heat flux: Comparison to soil heat flux plates, Adv. Water Resour., 50, 41–54, 2012a.
Evett, S. R., Schwartz, R. C., Casanova, J. J., and Heng, L. K.: Soil water sensing for water balance ET and WUE, Agr. Water Manage., 104, 1–9, 2012b.
Evett, S. R., Marek, G. W., Copeland, K. S., and Colaizzi, P. D.: Quality management for research weather data: USDA-ARS, Bushland, Texas, USA, Agrosystems, Geosciences & Environment, 1, 180036, https://doi.org/10.2134/age2018.09.0036, 2018.
Evett, S. R., Brauer, D. K., Colaizzi, P. D., Tolk, J. A., Marek, G. W., and O'Shaughnessy, S. A.: Corn and sorghum ET, E, Yield and CWP as affected by irrigation application method: SDI versus mid-elevation spray irrigation, T. ASABE, 62, 1377–1393, https://doi.org/10.13031/trans.13314, 2019.
Finkelstein, P. L. and Sims, P. F.: Sampling error in eddy correlation flux measurements, J. Geophys. Res.-Atmos., 106, 3503–3509, 2001.
Fratini, G. and Mauder, M.: Towards a consistent eddy-covariance processing: an intercomparison of EddyPro and TK3, Atmos. Meas. Tech., 7, 2273–2281, https://doi.org/10.5194/amt-7-2273-2014, 2014.
Fratini, G., McDermitt, D. K., and Papale, D.: Eddy-covariance flux errors due to biases in gas concentration measurements: origins, quantification and correction, Biogeosciences, 11, 1037–1051, https://doi.org/10.5194/bg-11-1037-2014, 2014.
Gowda, P. H., Senay, G. B., Howell, T. A., and Marek, T. H.: Lysimetric evaluation of simplified surface energy balance approach in the Texas High Plains, Appl. Eng. Agric., 25, 665–669, 2009.
Haslwanter, A., Hammerle, A., and Wohlfahrt, G.: Open- vs. closed-path eddy covariance measurements of the net ecosystem carbon dioxide and water vapour exchange: a long-term perspective, Agr. Forest Meteorol., 149, 291–302, 2009.
Heusinkveld, B., Jacob, A., and Hotslag, A.: Effect of open-path gas analyzer wetness on eddy covariance flux measurements: A proposed solution, Agr. Forest Meteorol., 148, 1563–1573, 2008.
Hirschi, M., Michel, D., Lehner, I., and Seneviratne, S. I.: A site-level comparison of lysimeter and eddy covariance flux measurements of evapotranspiration, Hydrol. Earth Syst. Sci., 21, 1809–1825, https://doi.org/10.5194/hess-21-1809-2017, 2017.
Honkanen, M., Tuovinen, J.-P., Laurila, T., Mäkelä, T., Hatakka, J., Kielosto, S., and Laakso, L.: Measuring turbulent CO2 fluxes with a closed-path gas analyzer in a marine environment, Atmos. Meas. Tech., 11, 5335–5350, https://doi.org/10.5194/amt-11-5335-2018, 2018.
Horst, T. W. and Lenschow, D. H.: Attenuation of scalar fluxes measured with spatially-displaced sensors, Bound.-Lay. Meteorol., 130, 275–300, 2009.
Irmak, S., Payero, J. O., Kilic, A., Odhiambo, L. O., Rudnick, D., Sharma, V., and Billesbach, D.: On the magnitude and dynamics of eddy covariance system residual energy (energy balance closure error) in subsurface drip-irrigated maize field during growing and non-growing (dormant) seasons, Irrigation Sci., 32, 471–483, 2014.
Iwata, H., Harazono, Y., and Ueyama, M.: Sensitivity and offset changes of a fast-response open-path infrared gas analyzer during long-term observations in an Arctic environment, J. Agric. Meteorol., 68, 175–181, 2012.
Kaimal, J. C. and Finnigan, J. J.: Atmospheric Boundary Layer Flows: Their Structure and Measurement, Oxford University Press, Oxford, UK, 1994.
Kim, W., Miyata, A., Ashraf, A., Maruyama, A., Chidthaisong, A., Jaikaeo, C., Komori, D., Ikoma, E., Sakurai, G., Seoh, H.-H., Son, I. C., Cho, J., Kim, J., Ono, K., Nusit, K., Moon, K. H., Mano, M., Yokozawa, M., Baten, M. A., Sanwangsri, M., Toda, M., Chaun, N., Polsan, P., Yonemura, S., Kim, S.-D., Miyazaki, S., Kanae, S., Phonkasi, S., Kammales, S., Takimoto, T., Nakai, T., Iizumi, T., Surapipith, V., Sonklin, W., Lee, Y., Inoue, Y., Kim, Y., and Oki, T.: FluxPro as a realtime monitoring and surveilling system for eddy covariance flux measurement, J. Agr. Meteorol., 71, 32–50, 2015.
Kochendorfer, J. and Paw, U.: Field estimates of scalar advection across a canopy edge, Agr. Forest Meteorol., 151, 585–594, 2011.
Kutikoff, S., Lin, X., Evett, S., Gowda, P., Moorhead, J., Marek, G., Colaizzi, P., Aiken, R., and Brauer, D.: Heat storage and its effect on the surface energy balance closure under advective conditions, Agr. Forest Meteorol., 265, 56–69, 2019.
Martínez-Cob, A. and Suvočarev, K.: Uncertainty due to hygrometer sensor in eddy covariance latent heat flux measurements, Agr. Forest Meteorol., 200, 92–96, 2015.
Massman, W. J.: A simple method for estimating frequency response corrections for eddy covariance systems, Agr. Forest Meteorol., 104, 185–198, 2000.
Mauder, M. and Foken, T.: Documentation and instruction manual of the eddy covariance software package TK2, Work report vol. 26, Dept. of Micrometeorology, University of Bayreuth, Bayreuth, Germany, ISSN 1614-8926, 45 pp., 2004.
Mauder, M., Oncley, S. P., Vogt, R., Weidinger, T., Ribeiro, L., Bernhofer, C., Foken, T., Kohsiek, W., De Bruin, H. A. R., and Liu, H.: The energy balance experiment EBEX-2000, Part II: Intercomparison of eddy-covariance sensors and post-field data processing methods, Bound.-Lay. Meteorol., 123, 29–54, 2006.
Mauder, M., Cuntz, M., Drüe, C., Graf, A., Rebmann, C., Schmid, H. P., Schmidt, M., and Steinbrecher, R.: A strategy for quality and uncertainty assessment of long-term eddy-covariance measurements, Agr. Forest Meteorol., 169, 122–135, 2013.
Miloshevich, L. M., Vömel, H., Whiteman, D. N., and Leblanc, T.: Accuracy assessment and correction of Vaisala RS92 radiosonde water vapor measurements, J. Geophys. Res.-Atmos., 114, D11305, https://doi.org/10.1029/2008JD011565, 2009.
Moncrieff, J., Massheder, J. M., De Bruin, H. A. R., Elbers, J., Friborg, T., Heusinkveld, B. G., Kabat, P., Scott, S. L., Soegaard, H., and Verhoef, A.: A system to measure surface fluxes of momentum, sensible heat, water vapour and carbon dioxide, J. Hydrol., 188–189, 589–611, 1997.
Moncrieff, J., Clement, R., Finnigan, J. J., and Meyers, T.: Averaging, detrending and filtering of eddy covariance time series, in: Handbook of micrometeorology: a guide for surface flux measurements, edited by: Lee, X., Massman, W. J., and Law, B. E., Kluwer Academic, Dordrecht, the Netherlands, 2004.
Moorhead, J. E., Marek, G. W., Colaizzi, P. D., Gowda, P. H., Evett, S. R., Brauer, D. K., Marek, T. H., and Porter, D. O.: Evaluation of sensible heat flux and evapotranspiration estimates using a surface layer scintillometer and a large weighing lysimeter, Sensors-Basel, 17, 2316–2350, https://doi.org/10.3390/s17102350, 2017.
Moorhead, J. E., Marek, G. W., Gowda, P. H., Lin, X., Colaizzi, P. D., Evett, S. R., and Kutikoff, S: Evaluation of evapotranspiration from eddy covariance using large weighing lysimeters, Agronomy, 9, 99, https://doi.org/10.3390/agronomy9020099, 2019.
Novick, K. A., Walker, J., Chan, W. S., Schmidt, A., Sobek, C., and Vose, J. M.: Eddy covariance measurements with a new fast-response, enclosed-path analyzer: Spectral characteristics and cross-system comparisons, Agr. Forest Meteorol., 181, 17–32, 2013.
Oncley, S. P., Foken, T., Vogt, R., Kohsiek, W., De Bruin, H. A., Bernhofer, C., Christen, A., Van Gorsel, E., Grantz, D., Feigenwinter, C., and Lehner, I.: The energy balance experiment EBEX-2000, Part I: overview and energy balance, Bound.-Lay. Meteorol., 123, 1–28, 2007.
O'Shaughnessy, S. A., Evett, S. R., Andrade, M. A., Workneh, F., Price, J. A., and Rush, C. M.: Site-specific variable-rate irrigation as a means to enhance water use efficiency, T. ASABE, 59, 239–249, 2016.
Polonik, P., Chan, W. S., Billesbach, D. P., Burba, G., Li, J., Nottrott, A., Bogoev, I., Conrad, B., and Biraud, S. C.: Comparison of gas analyzers for eddy covariance: Effects of analyzer type and spectral corrections on fluxes, Agr. Forest Meteorol., 272–273, 128–142, 2019.
Prajapati, P. and Santos, E. A.: Measurements of methane emissions from a beef cattle feedlot using the eddy covariance technique, Agr. Forest Meteorol., 232, 349–358, 2017.
Prueger, J. H., Alfieri, J. G., Hipps, L. E., Kustas, W. P., Chavez, J. L., Evett, S. R., Anderson, M. C., French, A. N., Neale, C. M. U., McKee, L. G., Hatfield, J. L., Howell, T. A., and Agam, N.: Patch scale turbulence over dryland and irrigated surfaces in a semi-arid landscape under advective conditions during BEAREX08, Adv. Water Resour., 50, 106–119, 2012.
Reichstein, M., Falge, E., Baldocchi, D., Papale, D., Aubinet, M., Berbigier, P., Bernhofer, C., Buchmann, N., Gilmanov, T., Granier, A., and Grünwald, T.: On the separation of net ecosystem exchange into assimilation and ecosystem respiration: review and improved algorithm, Global Change Biol., 11, 1424–1439, 2005.
Runkle, B. R., Rigby, J. R., Reba, M. L., Anapalli, S. S., Bhattacharjee, J., Krauss, K. W., Liang, L., Locke, M. A., Novick, K. A., Sui, R., and Suvočarev, K.: Delta-Flux: An eddy covariance network for a climate-smart lower Mississippi basin, Agricultural & Environmental Letters, 2, 1–5, 2017.
Takahashi, S., Kondo, F., Tsukamoto, O., Ito, Y., Hirayama, S., and Ishida, H.: On-board automated eddy flux measurement system over open ocean, Scientific Online Letters on the Atmosphere, 1, 37–40, 2005.
Tolk, J. A., Howell, T. A., and Miller, F. R.: Yield component analysis of grain sorghum grown under water stress, Field Crop. Res., 145, 44–51, 2013.
Van Dijk, A. I. J. M., Moene, A. F., and De Bruin, H. A. R.: The principles of surface flux physics: theory, practice and description of the ECPACK library, Wageningen University, Wageningen, the Netherlands, 99 pp., 2004.
Vickers, D. and Mahrt, L.: Quality control and flux sampling problems for tower and aircraft data, J. Atmos. Ocean. Tech., 14, 512–526, 1997.
Wolf, A. and Laca, E. A.: Cospectral analysis of high frequency signal loss in eddy covariance measurements, Atmos. Chem. Phys. Discuss., 7, 13151–13173, https://doi.org/10.5194/acpd-7-13151-2007, 2007.
Wu, J. B., Zhou, X. Y., Wang, A. Z., and Yuan, F. H.: Comparative measurements of water vapor fluxes over a tall forest using open- and closed-path eddy covariance system, Atmos. Meas. Tech., 8, 4123–4131, https://doi.org/10.5194/amt-8-4123-2015, 2015.
Xue, Q., Marek, T. H., Xu, W., and Bell, J.: Irrigated corn production and management in the Texas high plains, Journal of Contemporary Water Research & Education, 162, 31–41, 2017.
Zhang, X., Jin, C., Guan, D., Wang, A., Wu, J., and Yuan, F.: Long-term eddy covariance monitoring of evapotranspiration and Its environmental factors in a temperate mixed forest in northeast China, J. Hydrol. Eng., 17, 965–974, 2012.
Zhang, Y., Liu, H., Foken, T., Williams, Q. L., Liu, S., Mauder, M., and Liebethal, C.: Turbulence spectra and cospectra under the influence of large eddies in the Energy Balance EXperiment (EBEX), Bound.-Lay. Meteorol., 136, 235–251, 2010.