the Creative Commons Attribution 4.0 License.
the Creative Commons Attribution 4.0 License.
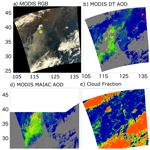
A Dark Target research aerosol algorithm for MODIS observations over eastern China: increasing coverage while maintaining accuracy at high aerosol loading
Robert C. Levy
Lorraine A. Remer
Shana Mattoo
Oleg Dubovik
Satellite aerosol products such as the Dark Target (DT) produced from the MODerate resolution Imaging Spectroradiometer (MODIS) are useful for monitoring the progress of air pollution. Unfortunately, the DT often fails to retrieve during the heaviest aerosol events as well as the more moderate events in winter. Some of the literature attributes this lack of retrieval to the cloud mask. However, we found this lack of retrieval is mainly traced to thresholds used for masking of inland water and snow. Modifications to these two masks greatly increase 50 % of the retrievals of aerosol optical depth at 0.55 µm (AOD) greater than 1.0. The “extra”-high-AOD retrievals tend to be biased when compared with a ground-based sun photometer (AErosol RObotic NETwork, AERONET). Reducing bias in new retrievals requires two additional steps. One is an update to the assumed aerosol optical properties (aerosol model); the haze in this region is both less absorbing and lower in altitude than what is assumed in the global algorithm. The second is accounting for the scale height of the aerosol, specifically that the heavy-aerosol events in the region are much closer to the surface than what is assumed by the global DT algorithm. The resulting combination of modified masking thresholds, new aerosol model, and lower aerosol layer scale height was applied to 3 months of MODIS observations (January–March 2013) over eastern China. After these two additional steps are implemented, the significant increase in new retrievals introduces no overall bias at a high-AOD regime but does degrade other overall validation statistics. We also find that the research algorithm is able to identify additional pollution events that AERONET instruments may not due to different spatial sampling. Mean AOD retrieved from the research algorithm increases from 0.11 to 0.18 compared to values calculated from the operational DT algorithm during January to March of 2013 over the study area. But near Beijing, where the severe pollution occurs, the new algorithm increases AOD by as much as 3.0 for each 0.5∘ grid box over the previous operational-algorithm values.
- Article
(8084 KB) - Full-text XML
- BibTeX
- EndNote
Because of rapid economic development and urbanization, eastern China, especially surrounding the Beijing area, has high large aerosol emissions and complex aerosol composition. The resulting large aerosol loading creates serious air quality and public health problems (Q. Zhang et al., 2012; Huang et al., 2014). Air quality issues in this region produce a significant socioeconomic impact due to the high density and continuous increase in the population and energy consumption. For example, Beijing alone had 12 million residents in 1998, with the number increasing rapidly to 21.51 million by the end of 2014 (Beijing Municipal Statistics Bureau). Studies show that the annual number of “haze days” over the North China Plain, defined as a day with visibility < 3 km (QX/T 113-2010; China Meteorological Administration, 2010), increased from 1980 and steeply increased since 2001 (Chen and Wang, 2015; Ding and Liu, 2014). In January 2013, eastern China experienced its worst ever severe haze or fog event. This event was marked by extremely high level of PM2.5 (particulate matter with an aerodynamic diameter less than 2.5 µm); long duration (70 % of the days in January exceeding the Chinese air quality standard of PM2.5 = 75 µg m−3); and extended spatial coverage (∼ 1.3 million km2), which affected ∼ 800 million people (Renhe et al., 2014; Huang et al., 2014). During this episode, Beijing's hourly PM2.5 exceeded 600 µg m−3, which is nearly 60 times higher than the World Health Organization's (WHO) “good” standard (WHO, 2006).
To try and mitigate this severe pollution, the Chinese government launched an air pollution control program in 1998. More drastic measures were taken during the 2008 Beijing Olympics (Chen et al., 2013) and also after the severe haze events of January 2013. Specifically, after the 2013 event, the Chinese State Council released the 5-year Clean Air Action Plan aiming to reduce annual PM2.5 to less than 60 µg m−3 by 2017 (Huang et al., 2014). To assess this achievement, the aerosol monitoring network was expanded quickly. While ground-based sites were added, there began a huge effort to utilize satellite aerosol products.
Even though satellite aerosol products represent a total-column optical measurement (aerosol optical depth; AOD) and not the PM2.5 measurements required for air quality concerns, the birds-eye view of the satellite has been shown to be useful. The Dark Target (DT) aerosol products on the MODerate resolution Imaging Spectroradiometer (MODIS) sensors onboard both Terra and Aqua satellites have become a popular data set used in studies of Chinese aerosol (Christopher and Zhang, 2002; Li et al., 2004; Yu et al., 2004; Quaas et al., 2008; K. Zhang et al., 2012; Costantino and Bréon, 2013; Luo et al., 2014; Bellouin et al., 2020). However, the DT products miss aerosol retrievals over eastern China, especially during wintertime. Many studies have discussed this issue (Yan et al., 2016; Bilal et al., 2014; Bilal and Nichol, 2015; Wei et al., 2019; Li et al., 2012; Zhang et al., 2020; Chen et al., 2017). Some attributed the problem of lack of retrieval to an overly aggressive cloud mask, which is not what we found in this study. Most of these studies tried to solve the missing data problem by using extra ancillary data or by developing a new algorithm (Yan et al., 2016; Li et al., 2012; Wei et al., 2019). Some of these methods are only applicable over a very small region, such as near the Beijing–Tianjing–Hebei area (Bilal and Nichol, 2015). We mention these studies here not as an endorsement but only to point out that identification of the problem of missing retrievals in China has received widespread notice in the published literature. None of these methods can provide real-time AOD consistent with the DT products. Near-real-time data are critical in terms of air quality forecasting and policy making, and the desire to be consistent with the DT product ties local characterization to a global standard. Due to the importance of satellite aerosol products for air quality and aerosol forecasting, our goal is to provide a more comprehensive near-real-time DT AOD map over China.
The main problem with the current DT product for China is a lack of retrievals. Even when the scene should be acceptable for an aerosol retrieval, the current operational algorithm fails to return a result. We show in this paper why there are so many missing retrievals, and we present a regionally specific research algorithm that remedies the problem. By bringing back these missing retrievals, new bias is introduced. We then fine-tune the algorithm to reduce the bias. There are always trade-offs in developing aerosol retrieval algorithms. In our case the priorities will be increased number of retrievals at high AOD and minimal overall bias against ground truth. However, to achieve these goals, root mean square error (RMSE) will increase. Thus, overall accuracy will be degraded, but product availability will increase, and no long-term biases will be introduced. The advantage of this regional research algorithm is that it is built within the structure of the DT algorithm so that implementation into the global operational system will be less onerous. However, implementation into the operational system is beyond the scope of this paper. In Sect. 2, we describe the data that are used in this study, and in Sect. 3 we illustrate the problem with the current operational algorithm with two case studies. This is followed by a presentation of a new research algorithm for China that (a) increases retrieval coverage in the region and (b) makes other adjustments so that high accuracy can be maintained as coverage is increased (Sect. 4). The new research algorithm is validated in Sect. 5, and in Sect. 6 we use the new algorithm to characterize an extreme pollution event. Section 7 summarizes and presents the conclusions.
2.1 MODIS Dark Target aerosol products and algorithm
The MODIS Dark Target algorithm, based on a lookup table (LUT) method, uses three wavelengths from 0.47 to 2.1 µm to retrieve aerosol properties over dark (vegetated and dark-soiled) land surfaces (Levy et al., 2007a, b, 2013; Remer et al., 2020). The basics of the overland algorithm are as follows: (1) consolidate higher-spatial-resolution (e.g., 500 m and 1 km) calibrated reflectance and radiance observations (known as level 1B “pixels”) into 10 km retrieval “boxes”; (2) apply various filters to remove (“mask”) clouds, cloud shadows, snow and ice, inland water, and any pixels that represent conditions that are not suitable for retrieval (Remer et al., 2005); (3) assign the aerosol model (optical and physical properties) that are most likely representative of a given season and location; (4) assume reflectance properties of the surface using an empirically derived equation; (5) search the pre-calculated LUTs simulating the observations of different loadings of the assumed aerosol type; (6) report the total aerosol loading (AOD) that, combined with the surface reflectance, provides the best match with the observed spectral reflectance; and finally (7) assign quality assurance and other diagnostics to the retrieval product. In a global sense, the generalized aerosol retrieval, along with strict quality assurance (e.g., QA confidence values = 3), has been shown to provide accurate retrievals and reasonable coverage over most conditions. For global retrievals over land, expected errors tend to be on the order of .
Although generally appropriate on a global scale, the thresholds for filtering or masking in step 2 can be too weak or too strong for particular regions. If too strong, they will falsely mask out legitimate aerosol retrievals. For land surfaces with vegetation, there is a strong absorption of radiation in the shortwave infrared (SWIR) by liquid water, and therefore the land surface parameterization is based on the assumption that liquid water in the leaves of vegetation is correlated with the pigments in those leaves that absorb radiation in the visible (blue and red wavelengths) during photosynthesis. However, liquid water not in vegetative structures (such as inland water) will also absorb SWIR wavelengths and confuse the algorithm and therefore must be masked. While designed to monitor the health of green vegetation (Tucker, 1979), the normalized difference vegetation index (NDVI) can also be used to detect inland water. It is defined as
where ρ is the reflectance at the top of the atmosphere (TOA) at 0.87 (near-infrared, NIR) and 0.66 µm (red) bands.
The aerosol algorithm uses NDVI in an inverse fashion to exclude nonvegetative scenes that might have a thin layer of water on the surface, such as melting snow or swamps. Scenes with very low values of NDVI (say < 0.1) include inland water, cloud edges, and arid regions such as deserts. Therefore, NDVI is an overall powerful tool that masks out many other conditions that are not optimal for applying the DT algorithm. The problem is that by increasing reflectance more in the red band than in the NIR band, heavy loadings of fine-dominated aerosol types (as are found in eastern China) can also depress the values of NDVI (Yang et al., 2020).
Analogously to the NDVI, we can define a difference index for detecting snow. A normalized difference snow index (NDSI) is similar to the NDVI used for the inland water mask, but as described in Li et al. (2005) it is based on different wavelengths. It is given as
where ρ is the reflectance at TOA at 0.87 (NIR) and 1.24 µm. This NDSI relies on the strong absorption and reflectance features of snow and ice, and in tandem with a brightness temperature threshold (e.g., 11 µm channel), it is used to mask out snow and ice. The operational DT algorithm considers pixels with NDSI > 0.01 and 11 µm channel brightness temperature less than 285 K to be snow, melting snow, or contaminated pixels near snow edges. Note that the temperature threshold is above the freezing level, which is a cautious approach to include regions where snow is in the process of melting and may be above freezing.
Overall, both the inland water (NDVI) and snow and ice (NDSI + temperature) filters are designed to optimize the balance between accuracy and availability of aerosol retrievals on a global basis but may not be ideal for a specific region such as China, where light- to heavy-pollution conditions occur year-round and particularly during the winter season.
The LUT used in the DT retrieval algorithm is calculated from prescribed aerosol models. The prescribed models depend on season and region and are based on global categorization of AErosol RObotic NETwork (AERONET) inversion products (Dubovik and King, 2000) as described in Levy et al. (2007b). In operation, the aerosol model assumed for most of China and for most seasons is the “moderately absorbing” model, with a single-scattering albedo, ω0, at 0.55 µm around 0.90. During the summer and fall along China's coastal belt, as for a small region around Taiwan in all seasons, the “weakly absorbing” (ω0∼0.95) model is used instead. These models were assigned to China for the version of the algorithm that went into production in 2005 (Collection 5), and while re-evaluated for Collection 6, which began in 2013, they were not adjusted. However, with the continued development of China's industry and urbanization as well as their “clean air” movement after 2008, the aerosol composition may have changed significantly enough to warrant an update of aerosol model selection.
In addition to the aerosol models being prescribed, the LUTs are calculated assuming a vertical profile for the aerosol. Except for coarse dust aerosol, all fine-dominated aerosol types (including the moderately and weakly absorbing types used for China) are assumed to have a scale height (H) of 2.0 km. In fact, pollution aerosol in China, especially during the winter months, tends to form under extremely stable conditions. Studies (Tang et al., 2015; Li et al., 2015; Luan et al., 2018; Liu et al., 2015) indicate that scale heights for the haze can be significantly less than 1.0 km, which due to multiply scattering interactions with the molecular atmosphere lead to errors in the LUTs assumed to simulate satellite observations.
The combination of less-than-ideal filtering for inland water and snow–ice, changing aerosol composition in the last 2 decades, and wrong assumptions of aerosol scale height leads to systematic errors in both coverage and accuracy over China.
2.2 Other MODIS aerosol products
DT is not the only algorithm that makes use of MODIS observations to derive aerosol properties. There are two additional AOD algorithms, known as “Deep Blue” (DB) and “Multi-Angle Implementation of Atmospheric Correction” (MAIAC). Since each algorithm uses different criteria for filtering and masking, makes different assumptions regarding aerosol optical properties and surface reflectance, and uses different techniques for fitting spectral observations, we can examine them and their products to inform possible solutions to the China retrievals outside of AERONET's coverage.
The DB algorithm, as its name indicates, uses observations in the “Deep Blue” or near-ultraviolet (NUV) part of the spectrum (∼ 0.412 µm) in addition to observations in the visible blue (0.466 µm) and red wavelengths (Hsu et al., 2006). DB bands can capture the aerosol signals due to the fact that carbonaceous aerosol types have strong absorption in shorter wavelengths, and desert surfaces have weaker reflectance in these wavelengths. For MODIS, the DB product has the same spatial resolution (10 × 10 km) as the DT product and has reported uncertainties (for the highest quality assurance) defined by Eq. (3):
where μ0 and μ are the cosine of the solar and view zenith angles, respectively (Sayer et al., 2013). For heavy smoke, including pollution if very optically dense, DB developed a smoke detection scheme based on Lambertian equivalent reflectivity (Dave and Mateer, 1967) at 0.412, 0.488, and 0.672 µm as well as brightness temperature at 11 µm. Once the aerosol is classified as smoke, the cloud mask is relaxed to ensure good retrieval spatial coverage, and the spatial variability threshold is also relaxed when assigned the data quality (Hsu et al., 2019).
The MAIAC algorithm uses time series (up to 16 d) analysis to exploit multangular information of atmosphere and land surface in order to derive semi-empirical bidirectional reflectance functions of the surface (Lyapustin et al., 2014, 2018). The algorithm utilizes the fact that the surface is more static than the atmosphere components such as clouds or aerosols during a short time span. With a more accurate description of surface characteristics, MAIAC has better ability to retrieve very optically thick aerosol plumes (Mhawish et al., 2019). However, data gaps still exist in the cloud-free region due to terrain or high-surface-albedo issues (Bi et al., 2019). MAIAC produces a product with the much finer spatial resolution of 1 km and has a reported bulk uncertainty of 66 % of retrievals within AOD (Lyapustin et al., 2018). The MAIAC atmospheric product (MCD19A2) is not stored in a traditional format of MODIS granule (e.g., retrievals along the native swath) but uses the MODIS sinusoidal grid instead (Stackpole, 1994). All granules are regridded into a 1 km sinusoidal map, and the overpass time, based on the granule ID, is stored in the product. However, there are slight differences between the time stamp and the granule ID (Yujie Wang, personal communication, 2019), which becomes apparent when comparing the three MODIS aerosol products in case studies. The differences will not statistically affect the comparison in our region of interest.
2.3 AERONET sun and sky aerosol products
The AErosol RObotic NETwork (AERONET) is a global aerosol monitoring network that is commonly used as a benchmark for validating satellite-retrieved AOD and to study the aerosol properties globally (Holben et al., 1998; Levy et al., 2013; Remer et al., 2005; Sayer et al., 2013; Zhang and Reid, 2006; Shi et al., 2011, 2013; Giles et al., 2019). AERONET provides two aerosol products. One measures aerosol attenuation through direct sun measurements, which provides spectral aerosol optical depth every 3 or 15 min (Holben et al., 1998). The most current version of this product is the version 3 product (Giles et al., 2019), which changes the cloud screening procedures from the older version and includes more AOD observations that are higher than 1.0 (Eck et al., 2018, 2019). This change is critical to evaluate satellite performance over regions with high AOD loading. The new-version product also has better cirrus filters and more accurate quality control procedures. The uncertainty in AOD from version 3 remains the same as previous versions, which is ∼ 0.01 in the visible and near-infrared and ∼ 0.02 at ultraviolet (UV) wavelengths (Eck et al., 1999).
In addition, AERONET instruments measure sky radiance and provide inversion products that contain aerosol microphysical and optical properties, such as particle size distribution, complex refractive index, and phase function (Dubovik and King, 2000; Dubovik et al., 2002, 2006). The new version 3 inversion products contain both traditional almucantar-mode sky measurements and the new hybrid-mode sky measurements. The almucantar mode is a series of measurements of the sky with changing azimuthal angles from 0 to ±180∘ and a fixed solar elevation angle (Holben et al., 1998). Almucantar mode can only measure aerosol properties when the solar zenith angle (SZA; the complement of the solar elevation angle) is greater than 50∘. That is when there is a sufficient range of scattering angles for high retrieval accuracy (Holben et al., 2006). This angle limitation is associated with the aerosol diurnal cycle, which means that there will be no aerosol properties derived during the middle of the day. The hybrid scan, which changes in both azimuthal and zenith angle directions simultaneously, can provide robust retrievals for measurements up to 25∘ SZA (Sinyuk et al., 2020). Because the hybrid scan is only available with the new CIMEL Model T, the availability of hybrid measurements is limited, as compared with almucantar measurements. In our study, there are three sites that provide the hybrid scan: Beijing-CAMS, Beijing_PKU, and Yanqihu.
During this process, we used all available version 3 level 2 AOD and inversion products over China following QA procedures and recommendations found in Holben et al. (2006) to acquire a new aerosol model for the Beijing region as well as using AERONET data for validation purposes.
While the DT algorithm has been a proven success as a global product, there continue to be regions where the algorithm under performs, and one of those regions is China. Many studies find that the algorithm frequently fails to retrieve in situations. But we find there is no physical reason to prevent retrieval. These situations occur in both high- and low-AOD situations. We present two case studies to illustrate the problem.
3.1 An intense high-AOD pollution event on 9 October 2013
Figure 1 illustrates a heavily polluted condition over East Asia, which is common over this region. Figure 1a is the MODIS “true-color” reflectance image from 25 to 45∘ latitude and 105 to 145∘ longitude. Significant pollution aerosol plumes, appearing gray, cover Beijing (39.9∘ N latitude, 116.4∘ E longitude) and its surrounding regions and extend towards the southwest all the way to the edge of the image. Clear patterns of variation in pollution can be found within the plume. Figure 1b is the MODIS DT AOD for all available retrievals, including retrievals meant for only qualitative imagery (QA = 0 to 3). The AOD gradually increases from very low loading outside of the plume to close to 1.0 at the edge of the pollution. However, there is no AOD retrieved at the thickest part of the plume. Two nearby AERONET sites, Xianghe and Beijing, reported AOD at 0.5 µm from 2 to 3 and above 3, respectively. Figure 1c shows the MODIS DB AOD for all available DB retrievals (QA = 0 to 3). DB has retrieved similar AOD as compared with the DT product but has filled the DT data gap with mostly AOD of 3.0. This is the upper boundary for AOD in the DB retrieval as the AOD within the thickest part of the plume has very limited dynamic range and does not reflect the variation in AOD that is shown in Fig. 1a. Figure 1d shows the all available MODIS MAIAC AOD. The MAIAC product shows 1 km resolution AOD, and the majority of the region reports AOD around 1.5 to 2.0, which is lower than what DB reports. Over two small regions, AOD reaches 3.0 and above. We also checked the Ozone Monitoring Instrument (OMI) UV aerosol index (AI), which showed that the pollution plume was not very absorbing in the UV part of the spectrum. Figure 1 demonstrates that all of the MODIS retrieval algorithms have trouble detecting and/or retrieving the heavy pollution. However, they appear to fail for different reasons.
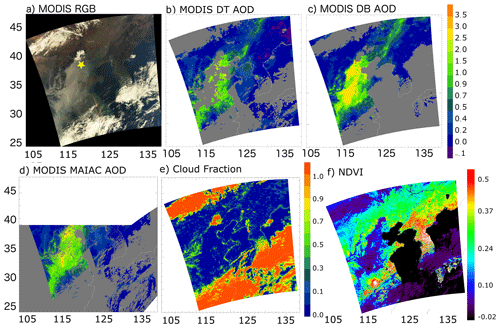
Figure 1A case study of a pollution event over eastern China on 9 October 2013. (a) MODIS Aqua RGB image; (b) MODIS Dark Target (DT) AOD at 0.55 µm for all available retrievals (QA = 0 to 3); (c) MODIS Deep Blue (DB) AOD at 0.55 µm for all available DB retrievals (QA = 0 to 3); (d) MODIS MAIAC AOD; (e) MODIS DT Cloud Fraction, a diagnostic of the MODIS aerosol product; (f) normalized difference vegetation index, used as the inland water mask by the DT algorithm. The yellow star represents the AERONET site Xianghe.
A natural hypothesis is that the aerosol retrieval fails because it confuses a heavy-aerosol plume with a cloud like many studies suggested (Mhawish et al., 2019; Bi et al., 2019; Tao et al., 2015; Yan et al., 2016). The DT algorithm, however, provides both a cloud fraction estimate and a quality assurance cascade to help determine the point in which the retrieval fails. Near the center of the plume, where the pollution is heaviest (Fig. 1d), the cloud fraction in the MODIS DT aerosol product is almost 0, which indicates that an overly aggressive cloud masking is not the major reason these aerosols are not retrieved. Instead, it is the NDVI map (Fig. 1e) which explains why the retrieval fails. Here the NDVI values are less than 0.1, which is used for an inland water mask, denoted by the dark blue to purple where the thickest pollution occurs. These values are below the threshold of the inland water mask, which triggers the mask in operational procedures and prohibits retrieval there. This case study illustrates the problem of not being able to retrieve AOD over high-optical-depth pollution scenarios and its impact.
3.2 A low-AOD pollution event on 13 December 2018
In addition to not retrieving high aerosol loading, it is also common for DT to miss moderate- to low-AOD cases during winter. Figure 2 shows a typical moderate aerosol loading day (e.g AOD < 0.5) over East Asia on 13 December 2018. Figure 2a to c are similar to those from Fig. 1a to c, except this time DB only shows the best quality AOD (QA = 3). The reason that we show only the best quality DB AOD here is that there are sporadic, very high AODs scattered in the lower part of the granule, which is clearly contaminated AOD by clouds or other artifacts. Figure 2d and f are NDSI value and the brightness temperature of 11 µm, respectively. Both are used in the DT algorithm for masking out snow. Figure 2a shows a thick cloud deck covering the lower part of the granule; aerosol loading is low to moderate over East Asia, which is sufficiently diffuse to allow characterization of the surface cover. There are a couple tiny spots with visual evidence of snow or frozen ponds in eastern China, marked using arrows (yellow or red depending on the background image colors), as well as at the top of the image. From NASA Worldview (https://worldview.earthdata.nasa.gov, last access: 8 May 2021) we can see that 2 d before the case study, a snowstorm passed over the North China Plain, leaving snow on the ground that could be seen also on the day before the case study. The sequence of events suggests strongly that the day of the case study would continue to have patches of snow left on the ground, even if the snow patches were not explicitly discernible at MODIS resolution. The ground temperature on this day is above freezing but not too high; thus the snow melting is not very rapid. The nearby AERONET site Xuzhou-CUMT reported AOD at 0.5 µm of 0.3 to 0.6. Figure 2b shows that aerosol loading over this region is around 0.2 to 0.4 over land. Higher AOD, around 0.7, is retrieved over the coastal ocean; however, AOD above the adjacent land is not retrieved. Figure 2c and d show that the MODIS DB and MAIAC algorithm retrieved AOD over most of eastern China. However, the two products are not agreeing with each other in data coverage and AOD magnitude over some regions, and there is still missing data coverage from both products. Note that the visually identified snow patches are all removed from both products.
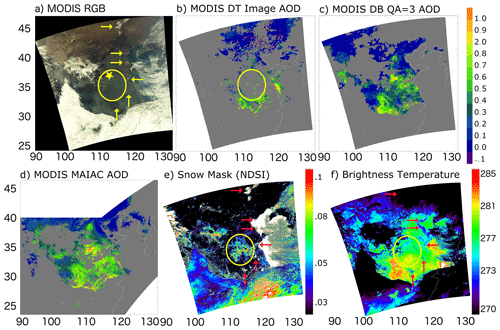
Figure 2A case study of a pollution event over eastern China on 13 December 2018. (a) RGB image, (b) MODIS DT for all available AOD (QA = 0 to 3), (c) MODIS DB AOD with QA = 3, (d) MODIS MAIAC for all available AOD, (e) MODIS DT NDSI used for snow masking, (f) 11 µm brightness temperature (BT). The yellow star represents the AERONET site XuZhou-CUMT. Arrows point at the snow patch locations in RGB, NDSI, and BT images, and the yellow circle encompasses the problem region.
We checked cloud and inland water masks, and these filters are not masking the pollution plumes in the DT product in this winter case study. Figure 2e shows the snow mask NDSI; the white color is when NDSI > 0.2, which is mostly snow over land or water surfaces. The algorithm uses NDSI > 0.01 (purple color) as the threshold, combined with temperature, to mask out snow. The snow features are shown in white at the center, but the snow edges are a combination of noisy color pixels from red to blue. Essentially any non-black color in Fig. 2e will be masked out given that the corresponding temperature is sufficiently cold. Note the relatively high values of NDSI where the arrows (red or yellow depending on the background) point to a snow feature, as defined by visual inspection in Fig. 2a. The problem arises in the large area identified as snow by the mask (within the yellow circle) that is not confirmed from visual inspection in Fig. 2a. This large area of misidentified snow differs from the identified snow features in that the NDSI ranges between 0.01 and 0.10, with no spatial connectivity to very snowy surfaces with NDSI > 0.10. Also, the region in the yellow circle is about 4 K warmer than the identified snow features that connect to the major snow fields. The yellow-circle temperature is about 277 K, while the identified features are closer to 273 K (Fig. 2f). Figure 2 illustrates how the snow mask can falsely mask out moderate to low aerosol loading over winter in China. This is a major reason that DT misses large areas of retrievals over this region during this season.
4.1 Increasing data coverage by the DT algorithm in China
Based on the case studies, we targeted two major causes of missing aerosol retrievals over wintertime China: inland water mask and snow mask. We then developed a method that can “rescue” the missing retrievals by altering these masks. Previous studies had shown that when NDVI values are between −0.02 and 0.1, the observed scenes can be coastal areas, surface with standing water, arid and desert surfaces, aerosols near cloud edges, and optically thick aerosol plumes (Shi et al., 2019). While we do not want to lose thick aerosol plumes, some of these situations are undesirable for an aerosol retrieval, and we use the NDVI test to mask those scenes. Thus, simply relaxing the NDVI threshold to below 0.1 will likely cause artifacts in retrieved AOD. We need another means to separate desirable from undesirable surface features other than a conservative threshold of NDVI. Reflectance at 2.13 µm is less affected by aerosol and strongly absorbed by water. According to this character, Yang et al. (2020) modify the inland water mask method for the haze conditions by simply adding an additional filter ρ2.13 < 0.08 but keeping the NDVI threshold unchanged. The haze aerosol has been successfully retrieved from a MODIS-like sensor, MERSI (Medium Resolution Spectral Imager), onboard the Chinese Fengyun-3D satellite.
The goal of this paper then is to develop new masking procedures also targeting coastal and semi-arid surfaces and then relax the NDVI thresholds. We start with reflectance at 2.13 µm and NDVIswir which uses the reflectance at TOA at 1.24 and 2.13 µm as shown in Eq. (4).
A pixel was determined to be inland water when NDVI < −0.02 or when −0.02 < NDVI < 0.1, and the conditions in Eq. (5) are met:
or
or
where ρ is the reflectance at TOA at 1.24 and 2.13 µm. Empirical investigation determined that when NDVI < −0.02, pixels are absolute water. When NDVI is between −0.02 and 0.1, ρ2.13<0.08 identifies undesirable coastal regions (Yang et al., 2020), and ρ2.13>0.25 or NDVIswir < 0.1 identifies semi-arid areas. Pixels within the −0.02 to 0.1 NDVI range and not caught by these additional filters are likely due to heavy aerosol loading and should be retained for retrieval.
Snow mask was modified as well to ensure low to moderate aerosol loading can be retrieved. From the case study in Sect. 3.2 we know that NDSI cannot be modified due to snow edge scenes; however, we can relax the BT11 from 285 to 278 K to exclude false snow detections when NDSI > 0.01. Again, the surprisingly warm temperature threshold was previously chosen to filter out tropical high-altitude snow. To avoid artifacts when temperature is relatively warm, and NDSI is very high, we exclude pixels with NDSI > 0.2 and BT11 < 285 K. We tested this change based on Li et al. (2005) and over global mid to high latitudes, and the results suggest that we can apply this new temperature threshold outside of our study region.
4.2 Maintaining accuracy for high-AOD retrievals
4.2.1 Research algorithm regional aerosol model
These modifications of the inland water and snow masks will increase the data coverage of the DT aerosol retrievals in both thick and thin pollution during winter over eastern China. There is potential for a large number of new retrievals, especially at the high-AOD end. Because poor assumptions in aerosol optical models are amplified in higher-aerosol-loading situations, we expect that adding new high-AOD retrievals will damage the overall accuracy of the product, even if no artifacts are introduced by the change in masking. As we mentioned in Sect. 2.1, over eastern China, the predetermined regional aerosol models are either moderately absorbing or non-absorbing depending on the region and season (Levy et al., 2007a). These analyses were done more than 10 years ago. Now that we may be introducing many additional high-AOD retrievals, it is important to revisit the aerosol model choice for our study region (Ichoku et al., 2003), especially since the aerosol environment is undergoing rapid change, and there are expanded data sets available to inform the analyses. We develop a local aerosol model by using version 3, level 2 AERONET-derived size distribution and complex refractive index from 24 sites, grouped into three clusters and then separated into summer and winter season. See Fig. 3. The grouping into geographical clusters fails to identify a sufficient reason to produce individual subarea aerosol models. Instead, in the end we will group all three clusters into one to construct a “regional” aerosol model, applicable to all of eastern China.
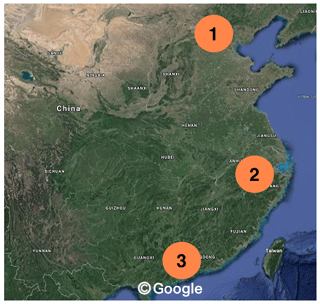
Figure 3Clusters of AERONET sites that are used in this study to generate the regional aerosol model. Cluster 1 includes Beijing, Beijing-CAMS, Beijing-RADI, Beijing_PKU, Lingshan_Mountain, Liangning, PKU_PEK, XiangHe, Xinglong, Yufa_PEK, and Yanqihu. Cluster 2 includes Hefei, NUIST, Shouxian, Hangzhou-ZFU, Qiandaohu, Hangzhou_City, and Taihu. Cluster 3 includes Hong_Kong_Hok_Tsui, Hong_ Kong_PolyU, Hong_Kong_Sheung, Kaiping, Zhongshan, and Zhongshan_Univ. The background map is from Google Maps.
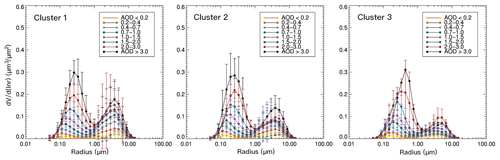
Figure 4Size distribution as a function of AERONET AOD at 0.675 µm, generated from the AERONET inversion products at three clusters illustrated in Fig. 3 using all available data records. Other than the last AOD bin, which is AOD > 3, the number of retrievals within each AOD bin is between hundreds and thousands. There are 263, 15, and 1 data points in the highest-AOD bin for cluster 1, 2, and 3, respectively. The error bars represent the standard deviation within each size bin.
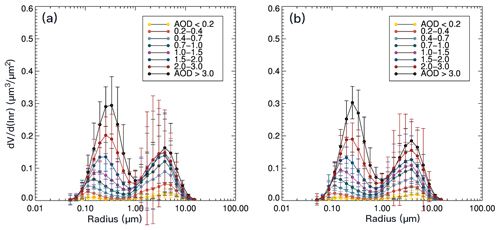
Figure 5Size distribution as a function of AERONET AOD at 0.675 µm, generated from the AERONET inversion products at cluster 1 illustrated in Fig. 3 using all available data records. Panel (a) is from April to September, panel (b) is from October to March. The error bars represent the standard deviation within each size bin.
Figure 4 shows three volume size distributions of 22 particle radii sorted as a function of AOD0.675 into bins of 0–0.2, 0.2–0.4, 0.4–0.7, 0.7–1.0, 1.0–1.5, 1.5–2.0, 2.0–3.0, and above 3.0, with the mean of each bin plotted. Figure 4 includes all seasons. Most bins have hundreds to thousands of data points. There is a systematic relationship between particle size distribution and AOD, with fine-particle median effective radius (rv) increasing with increasing AOD0.675. This relationship appears in all three clusters when ignoring the last AOD bin; Fig. 4b and c only have 15 data points and 1 data point within these two last bins. The size distribution from Fig. 4a and b are very similar, especially over the fine-mode aerosol regime. The fine-mode size distribution of cluster 3 is slightly larger than the other clusters, probably because cluster 3 is warmer and more humid, which leads to larger particles from swelling effects. Note that there is only one data point in cluster 3 within the highest-AOD bin. There are more coarse-mode particles in cluster 1 than cluster 2, which could be due to more dust particles in springtime or coagulation of soot particles in winter from public heating over the northern part of China. None of the differences between clusters is sufficiently robust to require maintaining separate aerosol models for the research algorithm. To further investigate differences in the aerosol model between winter and summer, we plotted volume size distributions from April to September (summer) and from October to March (winter) over cluster 1 (Fig. 5). Figure 5 shows that there is not much difference between the two time periods. The fine-mode size distribution is slightly skewed to the right in Fig. 5a than that from Fig. 5b, but this is hardly perceptible. As for coarse mode, Fig. 5a has a slightly different shape with a barely noticeable larger amount of coarse mode than Fig. 5b, perhaps due to transported dust in spring. Note that although in Fig 5b the size distribution of AOD > 3 (black line) is higher than Fig. 5a over the coarse-mode region, we refrain from drawing sweeping conclusions about the size distribution of these very-heavy-aerosol events because the number of data points in this AOD bin is 5 to 10 times smaller than the rest of the AOD bins. Based on the analysis of these plots, we decided to use one averaged size distribution to represent the bimodal pollution aerosol model over northern to eastern China, in summer and winter. Note that this fine-dominated pollution model will include the coarse mode, as seen in Figs. 4 and 5. During the retrieval, it will be mixed with another bimodal model representing an aerosol dominated by dust (Levy et al., 2007a, b).
Figure 6 shows the spectral dependence of the real and imaginary parts of the refractive index for all inversions, sorted as a function of AOD0.675. Because the AERONET inversion does not report a complex refractive index for each inverted size distribution, to increase the sample size in each AOD bin, we only use three AOD bins (0.4–1.0, 1.0–2.0, 2.0–4.0). Based on the AERONET teams' recommendation, only AERONET refractive index values with corresponding AOD0.44 larger than 0.4 are used to generate the model (Holben et al., 2006). Figure 6 shows there is no systematic relationship between the real part of the refractive index and AOD in this data set. The variability in each AOD bin exceeds the differences between the bins. There is a slight separation between low-AOD bin vs. high-AOD bin in the imaginary part of the refractive index. Thus, we use a single mean value for the real part of the refractive index and a parametric equation based on AOD for the imaginary part of the refractive index in our regional aerosol model. The real part of the refractive index is interpolated to 0.55 µm linearly, while the imaginary part of the refractive index is interpolated using logarithms from 0.44 and 0.675 µm (Lee et al., 2017).
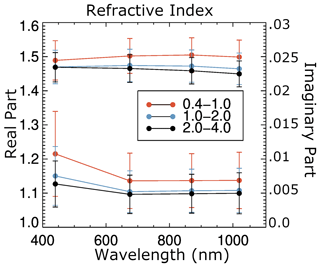
Figure 6The real (top set of curves and left axis) and imaginary (lower set of curves and right axis) parts of the refractive index as a function of AOD at 0.675 µm calculated from the AERONET inversion product at cluster 1. The error bars represent the standard deviation within each wavelength.
Table 1Optical properties of the aerosol model used by the operational DT algorithm over China and the regional model generated in this study using AERONET inversion products. The real and imaginary refractive index is a spectrally dependent quantity. Values in this table are for 0.55 µm.

Table 1 shows the comparison between the fine modes of the operational models that are used over the China region and the newly generated aerosol model. The coarse modes remain the same as the operational models (Levy et al., 2007b). The calculated natural logarithm of the standard deviation of the radius (σ) and the volume of particles per cross-section of the atmospheric column (V0) do not change much from the operational non-absorbing model. And our sensitivity studies show that changes in these two parameters are not the major factors in changing the output AOD. Thus, these two parameters remain the same. The new modal radius is very similar to what has been used operationally over part of coastal China during the fall and spring seasons, namely the “non-absorbing model” in Table 1. However, we are extending the same size distribution to a larger area of China over winter. The differences in the imaginary part of the refractive index show that when compared with the non-absorbing model, the new model is slightly more absorbing in the low-AOD range but less absorbing when AOD > ∼ 2. When compared with the operational moderately absorbing model, the regional model is more absorbing when AOD < 0.5 but is less absorbing when AOD is greater than this value. Overall, the new aerosol model is in between the operational non-absorbing aerosol model and moderately absorbing model when aerosol loading is moderate. Also notice that the moderately absorbing aerosol model shows increased absorption with increasing AOD, which is opposite to the non-absorbing model as well as to the regional model. This indicates that with an increase in aerosol loading, the absorption decreases in this region. These differences, especially due to the differences in absorption, can introduce a retrieval bias in AOD on the order of 1.
4.2.2 Aerosol layer scale height
Other than creating a new model, we also adjusted the aerosol layer height assumption when generating the LUT. In the operational algorithm, the LUT is calculated using an aerosol layer scale height of 2.0 km. Over China, when high loading of pollution accumulates, there is usually a high-pressure synoptic system, which suppress the aerosol layer vertical height (Zhao et al., 2013). Many field measurements reported the planetary boundary layer height during pollution episodes near the Beijing area to be only 800–1000 m (Tang et al., 2015; Li et al., 2015; Luan et al., 2018). A 4-year climatology of CALIOP aerosol layer height over wintertime China is also around 1.0–1.3 km over northeastern China (Liu et al., 2015). Thus, the scale height of the pollution layer in China is set to be around 0.5 km, which means that 80 % of the aerosols are within 1 km of the surface. This new assumed height is much lower than the operational value of 2 km, but we know that heavily polluted conditions increase the atmosphere stability and reduce the boundary layer height (Petäjä et al., 2016; Miao et al., 2017).
The DT algorithm has never changed aerosol scale height in the 2 decades of its operational history. The value is hardwired into the LUT calculation, as are other assumptions such as particle size distribution, refractive indices, and shape. Unlike aerosol retrieval algorithms that make use of measurements in the UV part of the spectrum (Torres et al., 2012), the DT algorithm relies only on visible and SWIR wavelengths, which are less sensitive to variations in scale height than the UV-dependent algorithms. Besides, aerosol layer height is variable on short temporal and spatial scales, making adjustments to the global constant value difficult to implement operationally. However, differences in aerosol layer height can impact retrieved AOD, especially for more absorbing aerosols. Figure 7 illustrates of how much change to expect in AOD if aerosol scale height changes from 2 to 0.5 km using the moderately absorbing model. The blue and red curves denote the calculated reflectance at TOA for a range of AODs, with blue representing aerosol at 0.5 km scale height and red for 2.0 km scale height. For a measured reflectance of 0.3, the AOD for the 0.5 km scale height would be 2.5, while for the 2.0 km scale height it would be 3.0. For a measured reflectance of 0.27, the AODs would be 1.8 and 2.0, respectively. Figure 7 shows for an aerosol model whose single-scattering albedo is 0.92 when AOD is around 2 to 3, the percentage differences in TOA reflectance between the two scale heights is ∼ 3 %–5 %, which leads to AOD changes of ∼ 8 %–15 %. Similarly, for the non-absorbing model (single-scattering albedo, SSA = 0.95), the changes in AOD are around 4 %–7 % when AOD is 2 to 3. Those are significant changes that require consideration and might be addressed for our specific region of interest as we develop a regional aerosol model for this research algorithm. We note that even adjusting the aerosol scale height for our specific region in certain conditions may improve retrievals in those conditions but make things worse at other times as aerosol layer height varies temporally. We choose to optimize for high-AOD conditions, accepting the possibility that biases may be introduced when AOD is low.
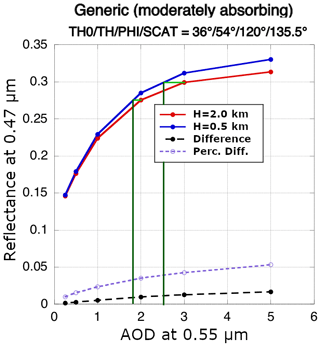
Figure 7Top-of-atmosphere reflectance at 0.47 µm corresponding to AOD at 0.55 µm using the moderately absorbing model for the specific geometry of solar zenith angle = 36∘, view zenith angle = 54∘, relative azimuth angle = 120∘, and scattering angle = 135.5∘. The red and blue lines are the calculated TOA reflectance at aerosol scale heights of 2.0 and 0.5 km, respectively. The dashed black and purple lines are the differences between the red and blue lines and the percentage differences. The horizontal light-green line segments indicate TOA reflectance when AOD is 2 and 3 using a scale height of 2.0 km. The corresponding vertical dark-green lines are corresponding AOD using a scale height of 0.5 km.
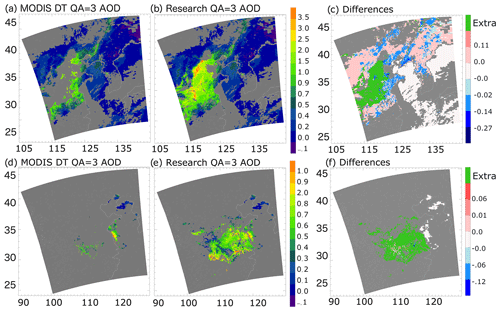
Figure 8(a–c) AOD images for the case study over eastern China on 9 October 2013: (a) operational DT AOD at 0.55 µm with QA = 3, (b) Research AOD at 0.55 µm with QA = 3 using altered thresholds on the NDVI test, snow test, and a new regional aerosol model with new aerosol scale height. (c) The differences between the research AOD (b) and the DT AOD (a). Panels (d) to (f) are for a case study of moderate pollution over eastern China on 13 December 2018. The increased research AOD data coverage is shown in green.
Using the regional pollution model with reduced aerosol scale height of 0.5 km and the algorithm with modified masking, we reproduce cases 3.1 and 3.2, as shown in Fig. 8. Figure 8a–c are for case study 3.1 (9 October 2013), where Fig. 8a shows the MODIS operational DT AOD with applied quality assurance (QA) equal to the highest value (= 3), Fig. 8b shows the AOD produced by the regional research algorithm with QA = 3, and Fig. 8c gives the differences in AOD (Fig. 8b minus Fig. 8a). The red–blue color scale is the AOD differences, with the extra data coverage in Fig. 8b highlighted in green. Comparing Fig. 8b with Fig. 8a, AOD is retrieved now at the center of the plume, where the operational DT has failed to retrieve. The AOD values change from 1.0 near the edge of the plume to ∼ 5 at the center. The pattern of the plume fits what we see from the RGB image (Fig. 1a). The difference plot shows that with the aerosol model and scale height change, the change in AOD is mostly less than 0.1, most of which is increasing AOD. The increase in AOD is mostly due to the aerosol model change, while the decreasing AOD is probably due to the aerosol scale height change. Figure 8d–f are similar to Fig. 8a–c but for case 3.2 (13 December 2018). Figure 8e has much increased data coverage over eastern China, with AOD values less than 1.0. Figure 2 shows these areas have no cloud or snow cover, and aerosol loading is generally less than 1.0, which fits what the new algorithm has retrieved. The difference plot mostly shows larger numbers of new pixels which are not retrieved in the operational algorithm. The change in AOD is mostly positive and less than 0.06. The reason for the large area of no data over the northern part of China is the surface being bright in the 2.1 µm reflectance, which causes the retrieved AOD to be assigned to a lower QA value, and thus it was not shown in Fig. 8d and e, where QA is required to be equal to 3.
The research algorithm is applied to MODIS radiances in a region bounded by 20 to 42∘ N,100 to 130∘ E, during January to March 2013. The resulting AOD is evaluated against AERONET AODs and inter-compared with AODs from the operational DT product. Spatiotemporal collocations of MODIS retrievals within 0.3∘ latitude and longitude of the AERONET site location and AERONET observations within 30 min of the satellite overpass times are used to collocate the two data sets. Figure 9 shows the scatterplot of MODIS versus AERONET AODs for (1) the operational DT product and AOD retrieved using modified masks with the operational LUT as well as (2) the research version of the MODIS AOD using the new LUT and the modified masks (referred to as the research algorithm hereafter) and AOD retrieved using modified masks with the operational LUT. All error statistics are shown in Table 2. Figure 9a shows that the MODIS DT product correlates well with AERONET data, with the largest percentage of collocations falling within the global expected error (EE). However, we can see there is limited number of data greater than 2.0 that are reported when collocated with AERONET. Comparing the effect of the new masking (Fig. 9a red dots) with the operational data, the biggest change is that the number of AOD collocations at AOD > 1 increased almost 50 %, although these increases in high AOD also introduce an overall high bias, increase the root mean square error (RMSE), and reduce the percentage of data within the EE. The high bias drops to less than 0.10 for high AOD, and the overall RMSE is reduced in research AOD in Fig. 9b, which uses the new LUT. However, the model change also leads to additional low bias at low AOD when compared with the operational product, which is linked to optimizing the scale height change for heavy aerosol loading. The overall bias is very small for the research algorithm, partially because there are both high biases and low biases within the newly added high AOD, which leads to a mean bias of almost zero, and the RMSE remains higher than the operational DT algorithm even after the new LUT is implemented. The new research algorithm is not more accurate than the operational DT, but it is less biased at high AOD, and the data coverage has increased, especially over the high-AOD regime. The change in error statistics, especially the increased scattering, is a trade-off to the increase in data coverage, which is the main goal of this study.
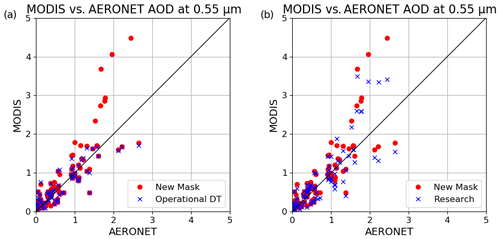
Figure 9Comparisons of the MODIS DT AOD at 0.55 µm against collocated AERONET observations during January, February, and March 2013 over China. Three data sets are used: operational DT AOD, an intermediate AOD retrieved using the same LUT as the operational DT but with modified masking (New Mask), and AOD retrieved with the full regional research algorithm (Research). (a) operational DT AOD overlay New Mask AOD; (b) Research AOD overlay New Mask AOD.
Table 2Statistics of validation between operational DT AOD, AOD using the operational LUT but with new masks (New Mask), and Research AOD against AERONET during January, February, and March 2013 over China. Numbers in parentheses are the statistics for AERONET AOD > 1.

We analyze the satellite AERONET bias of the DT and research AOD as a function of AERONET AOD and show the results in Fig. 10. In Fig. 10, AOD is binned every 47 pixels. When AERONET AOD is less than 1.0, there is a small bias between all MODIS products and the AERONET AOD. When AERONET AOD is greater than 1.0, the negative bias in the DT AOD grows to around −0.1 and then to −0.3 when AERONET is around 2.0. The mean negative bias in the operational AOD at AERONET AOD0.55 > 1.0 is partially due to the generic aerosol model used in the operational algorithm that is more absorbing than the heavy pollution generated in wintertime over eastern China. The AOD retrieved with the operational LUT but modified filters includes more collocations at high AOD, which leads to a larger positive bias. The research product maintains an absolute mean bias against AERONET of 0.01 or less across the entire range of AERONET AODs and shows very good agreement at the very highest AODs (AOD0.55 > 2). The very small mean bias is partially due to cancellation effects of overestimation and underestimation of research AODs as shown in Fig. 9b. The standard deviation of the bias can be large even when the mean bias is low. The regional aerosol model we use represents the fine-mode aerosol over the majority of China, except the west and north-central part of China, where other aerosol types, such as dust, can occur.
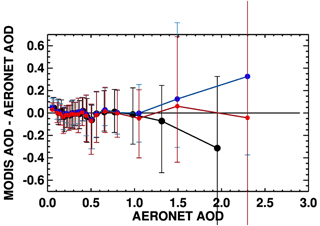
Figure 10Bias between MODIS and AERONET overland AOD at 0.55 µm as a function of AERONET AOD at 0.55 µm. Black represents the operational DT AOD, blue represents the AOD using the operational LUT but with new masks, and red represents the research AOD. The dots are the mean bias within each AERONET AOD bin, and the bars represent the standard deviation of the bias.
The new research algorithm increases data coverage temporally and spatially. A daily averaged AOD time series at the Xianghe AERONET site and the MODIS operational and research aerosol product are shown in Fig. 11. The Xianghe site is located near Beijing, where many heavy-pollution episodes occur. Thus, the AOD time series over this site show the most significant differences in data coverage between the DT AOD and the research AOD. The time series covers January to March 2013. When AERONET observes AOD < 0.5, both the operational DT and research products capture the AOD equally well. However, when AERONET observes AOD > 1, the operational product fails to retrieve the AOD, while the research product obtains a lot more AOD values over these days. Part of the discrepancies between the ground-based and satellite measurements are due to sampling differences as well as ground conditions such as snow cover or melting snow. For example, AERONET AOD around 2.7 at a Julian day of 15 is reduced to around 1.0 if we restrain the AERONET observation time to 30 min before or after the MODIS passing time. Similarly, the overestimation of research AOD above 3 between a Julian day of 75 and 80 is much smaller if we use the collocated data set instead of the daily average.
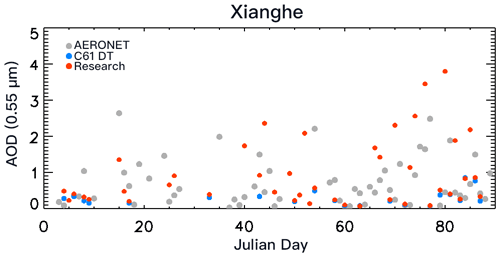
Figure 11Time series of daily averaged AERONET observations of AOD at 0.55 µm (in gray) as a function of Julian day in 2013 and the corresponding daily operational MODIS DT (in blue) and research (in red) AOD over the Xianghe AERONET site.
Table 3Identified days with pollution events from January to March 2013 over the Beijing area (37–40∘ N and 115–118∘ E) using both AERONET and DT research products.
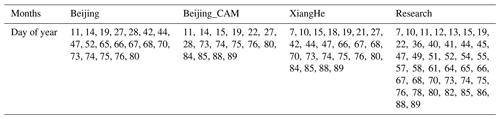
To further investigate the ability of using the DT research product to identify the pollution events, we calculated the AERONET-identified pollution day using three AERONET sites, Beijing, Beijing-CAMS, and XiangHe. As long as there are two observed AERONET AOD > 1.0 within 1 d, that day is considered a polluted day. The number of polluted days identified at these three sites between January and March 2013 is 19, 16, and 23 for Beijing, Beijing-CAMS, and XiangHe, respectively. There are also sampling differences between the three AERONET sites even though they are within 1∘ latitude and longitude of each other. Between Beijing and Beijing_CAM, there are 10 identified days in common. Between Beijing (Beijing_CAM) and XiangHe, there are 14 (12) identified days in common. Among all three sites, there are only 7 d that are commonly considered polluted days. Identified pollution event days are listed in Table 3.
The research product identified a total of 39 polluted days, of which 22 days were also identified as polluted by at least one of the three AERONET sites. There were 17 d when the research product identified a polluted day, but AERONET did not, and 7 d when AERONET observed AOD > 1.0, but the research algorithm did not capture the event. It is easy to understand when AERONET identified a polluted day, but the research retrieval did not because the AERONET observation time can be different from MODIS overpass time. The polluted scene can be cloud-covered at overpass but be captured by AERONET before or after, or the scene can significantly change between two observing times. It is more difficult to understand how the research algorithm could identify a pollution event on 17 d when all three AERONET stations do not report AOD > 1 at their quality-assured level (level 2). To begin we note that one of the three AERONET stations (Beijing-CAM5) was down for maintenance for more than a month during this time (Eck, 2021). Then, to confirm polluted days that the satellite identified but the operating AERONET stations did not, we visually compared each day using RGB images and MODIS DB and MAIAC AOD retrievals as well as nearby over-ocean AOD retrievals as a reference. Among these 17 d, 12 d have pollution present visually (with retrieval over cloud-free and snow-free land or ocean). Within these 12 d, analyses show two different scenarios lead to the discrepancies between AERONET and the research AOD. Scenario 1 includes the majority of the 12 d. In these days, AERONET level 2 (V3) reports AOD at 0.55 µm < 1 (0.50 to 0.90). Possible reasons for the differences can be (1) sampling differences, especially when an obvious gradient of AOD exists, or (2) the uncertainty within the research product (see Fig. 9). Scenario 2 consists of 5 d. These are days where there was no level 2 AERONET AOD with AOD550 > 1; however there were L1 data. Eck et al. (2018) found that for the Xianghe site, 15 % of high-AOD days (AOD500 > 1) never made it from L1 to L2. The 5 d that were identified by the satellite as pollution events but could not be confirmed by visual inspection were overcast with clouds (day 36, 41, 45, 49, and 61). In these five cases we expect that cloud effects in the MODIS product that do not appear in the AERONET data are causing the AOD to exceed the AOD = 1.0 threshold. We note that none of the 5 d in question have AOD over visually identified snow patches. Overall, we are happy with the ability of using the DT research product to identify pollution events, which can complement sparse ground observations.
With the research algorithm able to make many more additional retrievals and produce a better representation of the aerosol during winter, we examine the aerosol situation over China from January to March 2013 and investigate how the new results differ from the operational results in characterizing this situation. Figure 12 shows the AOD distribution change from operational DT AOD to research AOD in log-scale. The red is the research AOD, and the blue is the operational AOD. The histogram shows that when AOD is less than 1.0, the number of AOD retrievals increases by about 40 %. When AOD is greater than 1.0, and especially greater than 2.0, the increase in number of retrievals is much larger. The number between AOD 1.0 to 2.0 increased 130 %, while after that the number of data points tripled (342 %). In the negative AOD bin the number of data points is slightly increased due to the fact that the total number of retrievals increased 56 %.
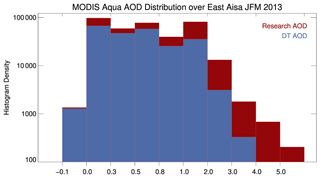
Figure 12The histogram of MODIS AOD over the study region from January to March 2013 in a logarithmic scale. The red is the research AOD; the blue is the operational AOD.
Monthly mean domain-averaged AOD statistics are shown in Table 4 for both MODIS aerosol retrievals over land. The operational DT product shows similar averaged AOD values around 0.58 and number of retrieved pixels around 18 000 in January and February. Both the DT AOD value and number of retrieved pixels increased in March. Compared to the DT AOD, the research AOD is higher, and the difference is largest in January (∼ 0.17 in AOD) and smallest in March (∼ 0.11). The same patterns can be found in the number of pixels; the increment is about 40 000 in January and 12 000 in March. This means that many more heavy-pollution episodes occur and possibly were missed by the operational DT algorithm in January than in the other 2 months. There is a reduction in research AOD in February, which could be caused by increasing the number of retrieved AOD smaller than 0.5 or by lowering the aerosol scale height without adding new retrievals. February is also associated with a decrease in the number of retrievals from the numbers seen in January, which could be caused by increased snow or cloud cover in February 2013.
Table 4Domain-averaged (25 to 40∘ N and 105 to 120∘ E) monthly mean MODIS-derived AOD with QA = 3 at 0.55 µm over land for the operational (DT) and research (Res) algorithms and the number of valid retrievals in 2013.
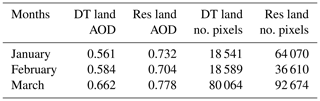
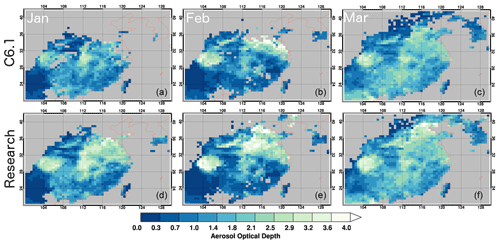
Figure 13Spatial distribution of averaged AOD from the operational product (a–c) and the research product (d–f) at 0.5∘ resolution over the study domain from January to March 2013.
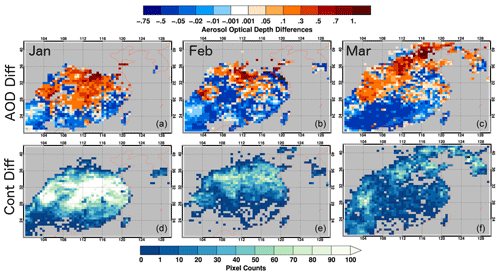
Figure 14Spatial distribution of AOD differences (a–c) and number of data point differences (d–f) between the operational product and the research product (research minus operational) at 0.5∘ resolution over the study domain from January to March 2013.
Figure 13 shows the spatial distribution of averaged AOD from the research product and the operational product at 0.5∘ resolution over the study domain from January to March 2013 with at least three data points per month per grid. The upper row is the operational DT AOD, and the lower row is the research AOD, with three columns representing January to March. The research product shows much more intense aerosol loading over eastern China, with more data coverage north of 35∘ N, than does the operational DT algorithm. The differences between the monthly DT and research AOD distribution are shown in Fig. 14 (upper panels) along with the number of pixel differences (lower panels). Grid boxes with AOD differences greater than 1.0 are found closer to Beijing and its surrounding area. Such large differences are found in 10 %, 17 %, and 5 % of the total land grid boxes in January, February, and March correspondingly within the domain. Near the Beijing area (∼ 40∘ N, ∼ 116.5∘ E), differences in gridded AOD can be above 3.0 in March. There is a large number of additional retrievals in the area south of Beijing, bounded by 30 to 35∘ N and 110 to 120∘ E, where for each grid the research algorithm produces more than 100 new data points in January and around 40 to 60 in February and March. Although not shown in this paper, we also see increments in the number of data points over northern India and the islands of Japan in January and February. So even though the research algorithm was developed and tested only for China, from the magnitude of increased AOD in these places, there is indication that heavily polluted cases also may be missed over these regions. Non-Chinese locations will require separate validation analysis and would likely benefit from an evaluation and adjustment to the aerosol model used in the LUT. For example, the strong decrease in AOD over southern China seen in Fig. 14 has not been validated and may indicate the local nature of the aerosol model or assumption of aerosol scale height in the research algorithm developed for the Beijing area. Another promising development seen in Figs. 13 and 14 is that there is no increase in the number of data points or significantly increased AOD over coastal regions or over arid and semi-arid areas in northern and western China (part of this area is shown here). This indicates that the changes we made to the masking algorithms have not allowed improper surface types to be retrieved.
The MODIS DT algorithm misses many retrievals over eastern China during the wintertime when compared with ground-based measurements. Two conditions can lead to missing retrievals: one is during heavy-pollution events, and another is in low to moderate aerosol loading when the snow mask is mistakenly invoked. Other than missing retrievals, there is also improvement that can be made to more accurately represent aerosols over this region. Other satellite aerosol products also have trouble representing the scale of the aerosol loading over the study period and domain.
To improve the data coverage without damaging the retrieval accuracy, we adjust the pixel selection routines, specifically the inland water mask and the snow mask. Then we use AERONET version 3 inversion products to first evaluate the aerosol models used operationally in China and then develop an aerosol model specifically for this region. The inland water mask is relaxed to allow for very high AOD but then used in combination with the reflectance at 2.13 µm to eliminate artifacts from coastal and brighter surfaces. The snow mask is also modified to include scenes currently misclassified as snow by lowering the threshold of surface temperature during snow cover and snow melting conditions. These measures increase the number of retrievals in our domain by 50 % and double the number of retrievals with AOD greater than 1.0. The higher the AOD, the more sensitive the retrieval will be to the aerosol model. After adding so many new high-AOD retrievals, we find that a new aerosol model is needed, which we develop from local AERONET inversion products. The new aerosol model has absorption in between the non-absorbing and moderately absorbing models that were used in the operational model. The assumed aerosol layer height was also lowered in the new LUT to match the aerosol vertical distribution over the study region. The combination of new aerosol model and lowered scale height reduces high bias for retrievals at high AOD but also introduces low bias at low AOD. That low bias may be accentuated as the algorithm is applied beyond the local Beijing area or when aerosol conditions change temporally in the local Beijing area. This is the first time that aerosol layer scale height has been adjusted in the DT retrieval since the at-launch algorithm 20 years ago and suggests there could be sensitivity to aerosol layer scale height in other regions with heavy aerosol loading.
We validated the research product from January to March 2013 using AERONET version 3 level 2 AOD. With the large number of new AOD retrievals, particularly new high AODs, the RMSE increased, and the percentage within the expected error (EE) was reduced; however, the overall bias at AOD > 1 was reduced to half (0.097). The research product captures a lot more high-aerosol-loading days that were observed by AERONET and can be used effectively in identifying pollution events. The new research algorithm is not more accurate than the operational DT, but the data coverage has increased without adding significant bias, especially over the high-AOD regime. The ability to now retrieve these optically thick pollution events alters our understanding of the aerosol system in this region. Statistical analyses illustrate the increase in the regional aerosol distribution during wintertime over eastern China, including a very large increment in AOD over Beijing. Using the new algorithm, the monthly regionally averaged overland AOD0.55 over the domain increases by 0.11 to 0.18 over values calculated from the operational DT products during January to March of 2013, with the largest increment happening in January. But near Beijing, where the severe pollution occurs, the new algorithm increases AOD0.55 by as much as 3.0 for each 0.5∘ grid box over the previous operational-algorithm values.
The large area of missing data and the magnitude of missing AOD will heavily alter our understanding of the severity of these pollution events, influence regional radiative balance, and impact the air quality community. Being able to bring back these missing data, especially in a near-real-time manner, can significantly influence the aerosol and air quality modeling and forecasting studies as well as any decision making that rely on instantaneous satellite aerosol data. Being able to include these rare but important heavy-aerosol events in the DT products is critical to preparing the DT product to be more suitable for a wider range of applications. There is also potential to apply the research algorithm globally, especially over regions where high-aerosol-loading events (e.g., large-scale wildfires or severe air pollution) frequently occur, such as the western US and India. The modification of inland water mask and snow mask has been lightly tested globally. Results show that inland water mask change introduced differences in retrieved AOD over coastal and arid and semi-arid regions, and snow mask works well on the selected scenes globally, including tropical high mountain regions. However, tests with longer time spans are needed before we can commit these changes globally.
The MODIS level 1B reflectance (https://doi.org/10.5067/MODIS/MYD021KM.061; MCST, 2017) and Dark Target level 2 aerosol data (https://doi.org/10.5067/MODIS/MOD04_L2.061; Levy et al., 2015) can be accessed via LAADS DAAC (https://ladsweb.modaps.eosdis.nasa.gov/, last access: 8 May 2021). The AERONET direct sun measurement data used in this study are available via the AERONET website (https://aeronet.gsfc.nasa.gov/new_web/download_all_v3_aod.html, AERONET team, 2021; Giles et al., 2019).
YRS, RCL, and LY initiated the research. YRS led this research and performed the research algorithm development and result analyses. RCL and LAR provided guidance throughout this research, especially on algorithm development and aerosol layer height. LY created the new inland water mask and helped with the case study. SM helped algorithm modification implementation. OD provided knowledge on creating a new aerosol model. All authors revised the manuscript.
The authors declare that they have no conflict of interest.
This article is part of the special issue “Satellite and ground-based remote sensing of aerosol optical, physical, and chemical properties over China”. It is not associated with a conference.
The authors thank the AERONET team for establishing and maintaining the AERONET sites and the data used in this investigation.
This research has been supported by the National Aeronautics and Space Administration (Terra/Aqua senior review; grant nos. NNH17ZDA001N-MEASURES and NNH19ZDA001N-PACESAT) and the National Natural Science Foundation of China (grant no. 41975036).
This paper was edited by Gerrit de Leeuw and reviewed by two anonymous referees.
AERONET team: AERONET direct sun measurements and inversion product, available at: https://aeronet.gsfc.nasa.gov/new_web/download_all_v3_aod.html, last access: 8 May 2021.
Bellouin, N., Quaas, J., Gryspeerdt, E., Kinne, S., Stier, P., Watson-Parris, D., Boucher, O., Carslaw, K. S., Christensen, M., Daniau, A. L., and Dufresne, J. L.: Bounding global aerosol radiative forcing of climate change, Rev. Geophys., 58, 2019RG000660, https://doi.org/10.1029/2019RG000660, 2020.
Bi, J., Belle, J. H., Wang, Y., Lyapustin, A. I., Wildani, A., and Liu, Y.: Impacts of snow and cloud covers on satellite-derived PM2.5 levels. Remote Sens. Environ., 221, 665–674, https://doi.org/10.1016/j.rse.2018.12.002, 2019.
Bilal, M. and Nichol, J. E.: Evaluation of MODIS aerosol retrieval algorithms over the Beijing-Tianjin-Hebei region during low to very high pollution events, J. Geophys. Res.-Atmos., 120, 7941–7957, https://doi.org/10.1002/2015JD023082, 2015.
Bilal, M., Nichol, J. E., and Chan, P. W.: Validation and accuracy assessment of a Simplified Aerosol Retrieval Algorithm (SARA) over Beijing under low and high aerosol loadings and dust storms, Remote Sens. Environ., 153, 50–60, https://doi.org/10.1016/j.rse.2014.07.015, 2014.
Chen, H. and Wang, H.: Haze days in North China and the associated atmospheric circulations based on daily visibility data from 1960 to 2012, J. Geophys. Res.-Atmos., 120, 5895–5909, https://doi.org/10.1002/2015JD023225, 2015.
Chen, Y., Jin, G. Z., Kumar, N., and Shi, G.: The promise of Beijing: Evaluating the impact of the 2008 Olympic Games on air quality, J. Environ. Econ. Manag., 66, 424–443, https://doi.org/10.1016/j.jeem.2013.06.005, 2013.
Chen, W., Fan, A., and Yan, L.: Performance of MODIS C6 aerosol product during frequent haze-fog events: A case study of Beijing, Remote Sensing, 9, 496, https://doi.org/10.3390/rs9050496, 2017.
China Meteorological Administration: Observation and forecasting levels of haze. The State Standard of the People's Republic of China QX/T 113-2010, China Meteorological Press, Beijing, China, 2010 (in Chinese).
Christopher, S. A. and Zhang, J.: Shortwave aerosol radiative forcing from MODIS and CERES observations over the oceans, Geophys. Res. Lett., 29, 6-1–6-4 https://doi.org/10.1029/2002GL014803, 2002.
Costantino, L. and Bréon, F.-M.: Aerosol indirect effect on warm clouds over South-East Atlantic, from co-located MODIS and CALIPSO observations, Atmos. Chem. Phys., 13, 69–88, https://doi.org/10.5194/acp-13-69-2013, 2013.
Dave, J. V. and Mateer, C.L.: A preliminary study on the possibility of estimating total atmospheric ozone from satellite measurements, J. Atmos. Sci., 24, 414–427, https://doi.org/10.1175/1520-0469(1967)024<0414:APSOTP>2.0.CO;2, 1967.
Ding, Y. and Liu, Y.: Analysis of long-term variations of fog and haze in China in recent 50 years and their relations with atmospheric humidity, Sci. China Earth Sci., 57, 36–46, https://doi.org/10.1007/s11430-013-4792-1, 2014.
Dubovik, O. and King, M. D.: A flexible inversion algorithm for retrieval of aerosol optical properties from Sun and sky radiance measurements, J. Geophys. Res.-Atmos., 105, 20673–20696, https://doi.org/10.1029/2000JD900282, 2000.
Dubovik, O., Holben, B., Eck, T. F., Smirnov, A., Kaufman, Y. J., King, M. D., Tanré, D., and Slutsker, I.: Variability of absorption and optical properties of key aerosol types observed in worldwide locations, J. Atmos. Sci., 59, 590–608, https://doi.org/10.1175/1520-0469(2002)059<0590:VOAAOP>2.0.CO;2, 2002.
Dubovik, O., Sinyuk, A., Lapyonok, T., Holben, B. N., Mishchenko, M., Yang, P., Eck, T. F., Volten, H., Munoz, O., Veihelmann, B., and Van der Zande, W. J.: Application of spheroid models to account for aerosol particle nonsphericity in remote sensing of desert dust, J. Geophys. Res.-Atmos., 111, D11208, https://doi.org/10.1029/2005JD006619, 2006.
Eck, T.: Interactive comment on “A Dark Target researchaerosol algorithm for MODIS observations overeastern China: Increasing coverage whilemaintaining accuracy at high aerosol loading” by Yingxi R. Shi et al., Atmos. Meas. Tech. Discuss., https://doi.org/10.5194/amt-2020-450-SC3, 2021.
Eck, T. F., Holben, B. N., Reid, J. S., Dubovik, O., Smirnov, A., O'neill, N. T., Slutsker, I., and Kinne, S.: Wavelength dependence of the optical depth of biomass burning, urban, and desert dust aerosols, J. Geophys. Res.-Atmos., 104, 31333–31349, https://doi.org/10.1029/1999JD900923, 1999.
Eck, T. F., Holben, B. N., Reid, J. S., Xian, P., Giles, D. M., Sinyuk, A., Smirnov, A., Schafer, J. S., Slutsker, I., Kim, J., and Koo, J. H.: Observations of the interaction and transport of fine mode aerosols with cloud and/or fog in Northeast Asia from Aerosol Robotic Network and satellite remote sensing, J. Geophys. Res.-Atmos., 123, 5560–5587, https://doi.org/10.1029/2018JD028313, 2018.
Eck, T. F., Holben, B. N., Giles, D. M., Slutsker, I., Sinyuk, A., Schafer, J. S., Smirnov, A., Sorokin, M., Reid, J. S., Sayer, A. M., and Hsu, N. C.: AERONET remotely sensed measurements and retrievals of biomass burning aerosol optical properties during the 2015 Indonesian burning season, J. Geophys. Res.-Atmos., 124, 4722–4740, https://doi.org/10.1029/2018JD030182, 2019.
Giles, D. M., Sinyuk, A., Sorokin, M. G., Schafer, J. S., Smirnov, A., Slutsker, I., Eck, T. F., Holben, B. N., Lewis, J. R., Campbell, J. R., Welton, E. J., Korkin, S. V., and Lyapustin, A. I.: Advancements in the Aerosol Robotic Network (AERONET) Version 3 database – automated near-real-time quality control algorithm with improved cloud screening for Sun photometer aerosol optical depth (AOD) measurements, Atmos. Meas. Tech., 12, 169–209, https://doi.org/10.5194/amt-12-169-2019, 2019.
Holben, B. N., Eck, T. F., Slutsker, I. A., Tanre, D., Buis, J. P., Setzer, A., Vermote, E., Reagan, J. A., Kaufman, Y. J., Nakajima, T., and Lavenu, F.: AERONET – A federated instrument network and data archive for aerosol characterization, Remote Sens. Environ., 66, 1–16, https://doi.org/10.1016/S0034-4257(98)00031-5, 1998.
Holben, B. N., Eck, T. F., Slutsker, I., Smirnov, A., Sinyuk, A., Schafer, J., Giles, D., and Dubovik, O.: December. AERONET's version 2.0 quality assurance criteria, in: Remote Sensing of the Atmosphere and Clouds, 6408, 64080Q, https://doi.org/10.1117/12.706524, 2006.
Hsu, N. C., Tsay, S. C., King, M. D., and Herman, J. R.: Deep blue retrievals of Asian aerosol properties during ACE-Asia, IEEE T. Geosci. Remote, 44, 3180–3195, https://doi.org/10.1109/TGRS.2006.879540, 2006.
Hsu, N. C., Lee, J., Sayer, A. M., Kim, W., Bettenhausen, C., and Tsay, S. C.: VIIRS Deep blue aerosol products over land: Extending the EOS long-term aerosol data records, J. Geophys. Res.-Atmos., 124, 4026–4053, https://doi.org/10.1029/2018JD029688, 2019.
Huang, R. J., Zhang, Y., Bozzetti, C., Ho, K. F., Cao, J. J., Han, Y., Daellenbach, K. R., Slowik, J. G., Platt, S. M., Canonaco, F., and Zotter, P.: High secondary aerosol contribution to particulate pollution during haze events in China, Nature, 514, 218–222, https://doi.org/10.1038/nature13774, 2014.
Ichoku, C., Remer, L. A., Kaufman, Y. J., Levy, R., Chu, D. A., Tanré, D., and Holben, B. N.: MODIS observation of aerosols and estimation of aerosol radiative forcing over southern Africa during SAFARI 2000, J. Geophys. Res.-Atmos., 108, 8499, https://doi.org/10.1029/2002JD002366, 2003.
Lee, J., Hsu, N. C., Sayer, A. M., Bettenhausen, C., and Yang, P.: AERONET-Based Nonspherical Dust Optical Models and Effects on the VIIRS Deep Blue/SOAR Over Water Aerosol Product, J. Geophys. Res.-Atmos., 122, 10384–10401, https://doi.org/10.1002/2017JD027258, 2017.
Levy, R. C. and Hsu, C.: MODIS Atmosphere L2 Aerosol Product, NASA MODIS Adaptive Processing System, Goddard Space Flight Center, USA [data set], https://doi.org/10.5067/MODIS/MOD04_L2.006, 2015.
Levy, R. C., Remer, L. A., Mattoo, S., Vermote, E. F., and Kaufman, Y. J.: Second-generation operational algorithm: Retrieval of aerosol properties over land from inversion of Moderate Resolution Imaging Spectroradiometer spectral reflectance, J. Geophys. Res.-Atmos., 112, D13211, https://doi.org/10.1029/2006JD007811, 2007a.
Levy, R. C., Remer, L. A., and Dubovik, O.: Global aerosol optical properties and application to Moderate Resolution Imaging Spectroradiometer aerosol retrieval over land, J. Geophys. Res.-Atmos., 112, D13210, https://doi.org/10.1029/2006JD007815, 2007b.
Levy, R. C., Mattoo, S., Munchak, L. A., Remer, L. A., Sayer, A. M., Patadia, F., and Hsu, N. C.: The Collection 6 MODIS aerosol products over land and ocean, Atmos. Meas. Tech., 6, 2989–3034, https://doi.org/10.5194/amt-6-2989-2013, 2013.
Li, F., Vogelmann, A. M., and Ramanathan, V.: Saharan dust aerosol radiative forcing measured from space, J. Climate, 17, 2558–2571, https://doi.org/10.1175/1520-0442(2004)017<2558:SDARFM>2.0.CO;2, 2004.
Li, M., Tang, G. Q., Huang, J., Liu, Z. R., An, J. L., and Wang, Y. S.: Characteristics of winter atmospheric mixing layer height in Beijing-Tianjin-Hebei region and their relationship with the atmospheric pollution, Huan jing ke xue = Huanjing kexue, 36, 1935–1943, https://doi.org/10.13227/j.hjkx.2015.06.004, 2015.
Li, R. R., Remer, L., Kaufman, Y. J., Mattoo, S., Gao, B. C., and Vermote, E.: Snow and ice mask for the MODIS aerosol products, IEEE Geosci. Remote S., 2, 306–310, https://doi.org/10.1109/LGRS.2005.847755, 2005.
Li, S., Chen, L., Xiong, X., Tao, J., Su, L., Han, D., and Liu, Y.: Retrieval of the haze optical thickness in North China Plain using MODIS data, IEEE T. Geosci. Remote, 51, 2528–2540, https://doi.org/10.1109/TGRS.2012.2214038, 2012.
Liu, J., Huang, J., Chen, B., Zhou, T., Yan, H., Jin, H., Huang, Z., and Zhang, B.: Comparisons of PBL heights derived from CALIPSO and ECMWF reanalysis data over China, J. Quant. Spectrosc. Rad., 153, 102–112, https://doi.org/10.1016/j.jqsrt.2014.10.011, 2015.
Luan, T., Guo, X., Guo, L., and Zhang, T.: Quantifying the relationship between PM2.5 concentration, visibility and planetary boundary layer height for long-lasting haze and fog–haze mixed events in Beijing, Atmos. Chem. Phys., 18, 203–225, https://doi.org/10.5194/acp-18-203-2018, 2018.
Luo, Y., Zheng, X., Zhao, T., and Chen, J.: A climatology of aerosol optical depth over China from recent 10 years of MODIS remote sensing data, Int. J. Climatol., 34, 863–870, https://doi.org/10.1002/joc.3728, 2014.
Lyapustin, A., Wang, Y., Xiong, X., Meister, G., Platnick, S., Levy, R., Franz, B., Korkin, S., Hilker, T., Tucker, J., Hall, F., Sellers, P., Wu, A., and Angal, A.: Scientific impact of MODIS C5 calibration degradation and C6+ improvements, Atmos. Meas. Tech., 7, 4353–4365, https://doi.org/10.5194/amt-7-4353-2014, 2014.
Lyapustin, A., Wang, Y., Korkin, S., and Huang, D.: MODIS Collection 6 MAIAC algorithm, Atmos. Meas. Tech., 11, 5741–5765, https://doi.org/10.5194/amt-11-5741-2018, 2018.
Mhawish, A., Banerjee, T., Sorek-Hamer, M., Lyapustin, A., Broday, D. M., and Chatfield, R.: Comparison and evaluation of MODIS Multi-angle Implementation of Atmospheric Correction (MAIAC) aerosol product over South Asia, Remote Sens. Environ., 224, 12–28, https://doi.org/10.1016/j.rse.2019.01.033, 2019.
Miao, Y., Guo, J., Liu, S., Liu, H., Li, Z., Zhang, W., and Zhai, P.: Classification of summertime synoptic patterns in Beijing and their associations with boundary layer structure affecting aerosol pollution, Atmos. Chem. Phys., 17, 3097–3110, https://doi.org/10.5194/acp-17-3097-2017, 2017.
MODIS Characterization Support Team (MCST): MODIS 1km Calibrated Radiances Product, NASA MODIS Adaptive Processing System, Goddard Space Flight Center, USA [data set], https://doi.org/10.5067/MODIS/MYD021KM.061, 2017.
Petäjä, T., Järvi, L., Kerminen, V. M., Ding, A. J., Sun, J. N., Nie, W., Kujansuu, J., Virkkula, A., Yang, X., Fu, C. B., and Zilitinkevich, S.: Enhanced air pollution via aerosol-boundary layer feedback in China, Sci. Rep., 6, 18998, https://doi.org/10.1038/srep18998, 2016.
Quaas, J., Boucher, O., Bellouin, N., and Kinne, S.: Satellite-based estimate of the direct and indirect aerosol climate forcing, J. Geophys. Res.-Atmos., 113, D05204, https://doi.org/10.1029/2007JD008962, 2008.
Remer, L. A., Kaufman, Y. J., Tanré, D., Mattoo, S., Chu, D. A., Martins, J. V., Li, R. R., Ichoku, C., Levy, R. C., Kleidman, R. G., and Eck, T. F.: The MODIS aerosol algorithm, products, and validation, J. Atmos. Sci., 62, 947–973, https://doi.org/10.1175/JAS3385.1, 2005.
Remer, L. A., Levy, R. C., Mattoo, S., Tanré, D., Gupta, P., Shi, Y., Sawyer, V., Munchak, L. A., Zhou, Y., Kim, M., Ichoku, C., Patadia, F., Li, R.-R., Gassó, S., Kleidman, R. G., and Holben, B. N.: The Dark Target Algorithm for Observing the Global Aerosol System: Past, Present, and Future, Remote Sens., 12, 2900, https://doi.org/10.3390/rs12182900, 2020.
Renhe, Z., Li, Q., and Zhang, R.: Meteorological conditions for the persistent severe fog and haze event over eastern China in January 2013, Science China Earth Sciences, 57, 26–35, https://doi.org/10.1007/s11430-013-4774-3, 2014.
Sayer, A. M., Hsu, N. C., Bettenhausen, C., and Jeong, M. J.: Validation and uncertainty estimates for MODIS Collection 6 “Deep Blue” aerosol data, J. Geophys. Res.-Atmos., 118, 7864–7872, https://doi.org/10.1002/jgrd.50600, 2013.
Shi, Y., Zhang, J., Reid, J. S., Holben, B., Hyer, E. J., and Curtis, C.: An analysis of the collection 5 MODIS over-ocean aerosol optical depth product for its implication in aerosol assimilation, Atmos. Chem. Phys., 11, 557–565, https://doi.org/10.5194/acp-11-557-2011, 2011.
Shi, Y., Zhang, J., Reid, J. S., Hyer, E. J., and Hsu, N. C.: Critical evaluation of the MODIS Deep Blue aerosol optical depth product for data assimilation over North Africa, Atmos. Meas. Tech., 6, 949–969, https://doi.org/10.5194/amt-6-949-2013, 2013.
Shi, Y. R., Levy, R. C., Eck, T. F., Fisher, B., Mattoo, S., Remer, L. A., Slutsker, I., and Zhang, J.: Characterizing the 2015 Indonesia fire event using modified MODIS aerosol retrievals, Atmos. Chem. Phys., 19, 259–274, https://doi.org/10.5194/acp-19-259-2019, 2019.
Sinyuk, A., Holben, B. N., Eck, T. F., Giles, D. M., Slutsker, I., Korkin, S., Schafer, J. S., Smirnov, A., Sorokin, M., and Lyapustin, A.: The AERONET Version 3 aerosol retrieval algorithm, associated uncertainties and comparisons to Version 2, Atmos. Meas. Tech., 13, 3375–3411, https://doi.org/10.5194/amt-13-3375-2020, 2020.
Stackpole, J. D.: The WMO format for the storage of weather product information and the exchange of weather product messages in gridded binary form, US Department of Commerce, National Oceanic and Atmospheric Administration, National Weather Service, National Meteorological Center, Washington, D.C., USA, 1994.
Tang, G., Zhu, X., Hu, B., Xin, J., Wang, L., Münkel, C., Mao, G., and Wang, Y.: Impact of emission controls on air quality in Beijing during APEC 2014: lidar ceilometer observations, Atmos. Chem. Phys., 15, 12667–12680, https://doi.org/10.5194/acp-15-12667-2015, 2015.
Tao, M., Chen, L., Wang, Z., Tao, J., Che, H., Wang, X., and Wang, Y.: Comparison and evaluation of the MODIS Collection 6 aerosol data in China, J. Geophys. Res.-Atmos., 120, 6992–7005, https://doi.org/10.1002/2015JD023360, 2015.
Torres, O., Jethva, H., and Bhartia, P. K.: Retrieval of aerosol optical depth above clouds from OMI observations: Sensitivity analysis and case studies, J. Atmos. Sci., 69, 1037–1053, https://doi.org/10.1175/JAS-D-11-0130.1, 2012.
Tucker, C. J.: Red and Photographic Infrared Linear Combinations for Monitoring Vegetation, Rem. Sens. Environ, 8, 127–150, https://doi.org/10.1016/0034-4257(79)90013-0, 1979.
Wei, J., Li, Z., Peng, Y., and Sun, L.: MODIS Collection 6.1 aerosol optical depth products over land and ocean: validation and comparison, Atmos. Environ., 201, 428–440, https://doi.org/10.1016/j.atmosenv.2018.12.004, 2019.
World Health Organization: Air quality guidelines: global update 2005: particulate matter, ozone, nitrogen dioxide, and sulfur dioxide, World Health Organization, available at: https://apps.who.int/iris/handle/10665/69477 (last access: 8 May 2021), 2006.
Yan, X., Shi, W., Luo, N., and Zhao, W.: A new method of satellite-based haze aerosol monitoring over the North China Plain and a comparison with MODIS Collection 6 aerosol products, Atmos. Res., 171, 31–40, https://doi.org/10.1016/j.atmosres.2015.12.003, 2016.
Yang, L., Hu, X., Wang, Ha., He, X., Liu, P., Xu, Na., Yang, Z., and Zhang, P.: Preliminary test of quantitative capability in aerosol retrieval over land from MERSI-II onboard Fengyun-3D, National Remote Sensing Bulletin, Published Online, https://doi.org/10.11834/jrs.20200286, 2020.
Yu, H., Dickinson, R. E., Chin, M., Kaufman, Y. J., Zhou, M., Zhou, L., Tian, Y., Dubovik, O., and Holben, B. N.: Direct radiative effect of aerosols as determined from a combination of MODIS retrievals and GOCART simulations, J. Geophys. Res.-Atmos., 109, D03206, https://doi.org/10.1029/2003JD003914, 2004.
Zhang, J. and Reid, J. S.: MODIS aerosol product analysis for data assimilation: Assessment of over-ocean level 2 aerosol optical thickness retrievals, J. Geophys. Res.-Atmos., 111, D22207, https://doi.org/10.1029/2005JD006898, 2006.
Zhang, K., O'Donnell, D., Kazil, J., Stier, P., Kinne, S., Lohmann, U., Ferrachat, S., Croft, B., Quaas, J., Wan, H., Rast, S., and Feichter, J.: The global aerosol-climate model ECHAM-HAM, version 2: sensitivity to improvements in process representations, Atmos. Chem. Phys., 12, 8911–8949, https://doi.org/10.5194/acp-12-8911-2012, 2012.
Zhang, Q., He, K., and Huo, H.: Cleaning China's air, Nature, 484, 161–162, https://doi.org/10.1038/484161a, 2012.
Zhang, X., Wang, H., Che, H. Z., Tan, S. C., Shi, G. Y., Yao, X. P., and Zhao, H. J.: Improvement of snow/haze confusion data gaps in MODIS Dark Target aerosol retrievals in East China, Atmos. Res., 30, 105063, https://doi.org/10.1016/j.atmosres.2020.105063, 2020.
Zhao, X. J., Zhao, P. S., Xu, J., Meng,, W., Pu, W. W., Dong, F., He, D., and Shi, Q. F.: Analysis of a winter regional haze event and its formation mechanism in the North China Plain, Atmos. Chem. Phys., 13, 5685–5696, https://doi.org/10.5194/acp-13-5685-2013, 2013.
- Abstract
- Introduction
- Description of data products
- Case studies of high- and low-aerosol-loading scenarios over the Beijing area
- Research algorithm for eastern China and a new regional aerosol model
- Validation of the research AOD for January to March 2013
- Characterization of the 2013 winter China pollution situation
- Summary and conclusions
- Data availability
- Author contributions
- Competing interests
- Special issue statement
- Acknowledgements
- Financial support
- Review statement
- References
- Abstract
- Introduction
- Description of data products
- Case studies of high- and low-aerosol-loading scenarios over the Beijing area
- Research algorithm for eastern China and a new regional aerosol model
- Validation of the research AOD for January to March 2013
- Characterization of the 2013 winter China pollution situation
- Summary and conclusions
- Data availability
- Author contributions
- Competing interests
- Special issue statement
- Acknowledgements
- Financial support
- Review statement
- References