the Creative Commons Attribution 4.0 License.
the Creative Commons Attribution 4.0 License.
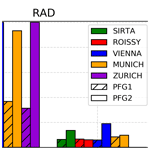
PARAFOG v2.0: a near-real-time decision tool to support nowcasting fog formation events at local scales
Jean-François Ribaud
Martial Haeffelin
Jean-Charles Dupont
Marc-Antoine Drouin
Felipe Toledo
Simone Kotthaus
An improved version of the near-real-time decision tool PARAFOG (PFG2) is presented to retrieve pre-fog alert levels and to discriminate between radiation (RAD) and stratus lowering (STL) fog situations. PFG2 has two distinct modules to monitor the physical processes involved in RAD and STL fog formation and is evaluated at European sites. The modules are based on innovative fuzzy logic algorithms to retrieve fog alert levels (low, moderate, high) specific to RAD/STL conditions, minutes to hours prior to fog onset. The PFG2-RAD module assesses also the thickness of the fog. Both the PFG2-RAD and PFG2-STL modules rely on the combination of visibility observations and automatic lidar and ceilometer (ALC) measurements. The overall performance of the PFG2-RAD and PFG2-STL modules is evaluated based on 9 years of measurements at the SIRTA (Instrumented Site for Atmospheric Remote Sensing Research) observatory near Paris and up to two fog seasons at the Paris-Roissy, Vienna, Munich, and Zurich airports. At all sites, pre-fog alert levels retrieved by PFG2 are found to be consistent with the local weather analysis. The advanced PFG2 algorithm performs with a hit rate of about 100 % for both considered fog types and presents a false alarm ratio on the order of 10 % (30 %) for RAD (STL) fog situations. Finally, the first high alerts that result in a subsequent fog event are found to occur for periods of time ranging from −120 min to fog onset, with the first high alerts occurring earlier for RAD than STL cases.
- Article
(4586 KB) - Full-text XML
- BibTeX
- EndNote
According to the World Meteorological Organization (2021), fog occurs when visibility at the Earth's surface is lower than 1 km due to the presence of suspended water droplets. The worldwide socioeconomic impact resulting from this “particular type of cloud” is just as significant as from other extreme events, such as storms (Gultepe et al., 2019). Indeed, fog may be responsible for severe disruptions at airports, including delays or even cancelations of flights (Gultepe et al., 2009), and causes frequent road accidents and may also impact rescue management, as well as military operations. In semi-arid and arid regions also, fog can constitute a fundamental resource as it can supply water to the local population (Gandhidasan and Abualhamayel, 2007). Therefore, accurate forecasting of fog events is essential.
The classical approach for fog forecasting used by national meteorological services relies on numerical weather prediction (NWP) models. Although the main physical processes leading to fog occurrences are well established (Gultepe et al., 2007), accurate fog formation (and dissipation) forecasting remains challenging for NWP models. According to Steeneveld et al. (2015), models generally struggle to accurately reproduce the timing of the fog onset, its spread, its depth, and its liquid water content. Statistical methods have been used to evaluate the possibility for an NWP model to accurately predict meteorological conditions favorable to fog formation (Menut et al., 2014; Román-Cascón et al., 2016). The difficulties of NWP fog forecasting can be explained by the fact that fog events are driven by complex surface–atmosphere interactions in the atmospheric boundary layer, where vertical resolution of NWP models is still not high enough (e.g., Philip et al., 2016). Specifically, components that yield difficulties for fog prediction for coarser-grid models are cloud microphysics parameterization, radiation parameterization, and potentially shallow convection parameterization, while for limited area models, boundary conditions also help determine advection, another potentially significant contribution to fog presence or absence. To simulate more detailed information, one-dimensional high-resolution numerical models have been used to complement the classical NWP setup, which allows specific local observations to be incorporated (e.g., Bergot et al., 2005). More recently, large-eddy simulations (LESs) have been used to explicitly resolve small-scale processes at play within the fog cloud (Bergot, 2016; Mazoyer et al., 2017; Wærsted et al., 2019). Still, LES modeling is computationally very expensive, and both microphysical and chemical parametrizations are still needed.
Another approach to forecast fog events is based upon ground- and/or space-based observations. From its top perspective, satellite imagery allows fog to be monitored by combining different bands with relatively good space–time resolutions. With this regard, Cermak and Bendix (2008) developed an operational fog/low stratus daytime scheme based on Meteosat data. Cermak and Bendix (2011) extended this approach to only discriminate ground radiation fog by introducing some microphysical hypotheses before being adapted by Egli et al. (2017) to make it suitable for several meteorological conditions encountered over Europe. Egli et al. (2018) proposed a hybrid diurnal fog product based on the combination of satellite images and ground-based observations. In addition, Kneringer et al. (2019) and Dietz et al. (2019) developed probabilistic fog nowcasting systems to forecast different low-visibility procedures from standard meteorological measurements available at Vienna international airport for lead times of +30 to +120 min.
While both the aforementioned satellite- and learn-based studies do not intend to track the evolution of particular physical processes driving fog formation, ground-based observations may provide valuable key information by monitoring their true values, complementing NWP models. Ground-based observations accurately allow key variables at play in a fog cloud at high temporal resolution (∼ every minute) to be measured. For instance, radiation fog formation results from an aerosol-particle hygroscopic growth process illustrating the vapor-to-liquid phase change before fog onset. Based on attenuated backscatter analysis, automatic lidar and ceilometer (ALC) data provide key information portraying this physical process. Haeffelin et al. (2016) developed the near-real-time fog analysis tool PARAFOG (hereafter referred to as PFG1), with the objective to predict radiation fog formation based on ALC measurements, together with classical meteorological observations. During the pre-fog condition (usually 1 to 3 h before fog), PFG1 determines a reference ALC-attenuated backscatter profile based on which the rate of change in aerosol-particle hygroscopic growth can be assessed. With this regard, PFG1 is able to retrieve pre-fog alert levels (low, moderate, or high alert) with a vertical resolution of about 15 m ranging from 0 to 400 m a.g.l. (above ground level) and time resolution of 1 min. PARAFOG is experimentally used at Paris international airports (Roissy-Charles de Gaulle and Orly) where it provides valuable information supporting the decision making of both weather forecasters and air traffic controllers that affects the scheduling of airplanes. Several years of experience with PFG1 have highlighted some limitations, such as the monitoring of stratus lowering fogs, its capabilities to monitor the entire fog life cycle, or even its anticipation for shallow radiation fog events near the surface.
In this study, we present PARAFOG v2.0 (hereafter referred to as PFG2), which is an improved and extended version of PFG1 allowing the discrimination between radiation and stratus lowering fog formation.
-
Radiation (RAD) fog events refer to fog that forms during radiative cooling at the ground surface, usually at night, in the presence of anticyclonic, low-wind-speed, and clear-sky conditions (Gultepe et al., 2007). Due to the radiative cooling, the air just above the ground is affected by a progressive hygroscopic growth of fog condensation nuclei, turning water vapor into liquid after reaching supersaturation, thereby reducing the surface visibility.
-
When radiative cooling coincides with large-scale subsidence, the cloud base height of stratus clouds can gradually decrease down to the surface, defining stratus lowering (STL) fog events. Indeed, stratus cloud-top radiative cooling acts to transport larger cloud droplets downwards (while strengthening the cloud-top inversion) and permits the cloud base to subside until reaching the ground at times (Dupont et al., 2012).
This article is organized as follows: Sect. 2 introduces the measurements used as input to PFG2 and measurement sites where evaluation studies are conducted. Section 3 presents the new methodology developed in PFG2. Section 4 presents the PFG2 results obtained at different European sites. The quantitative assessment of PFG2 and its relative performance is presented in Sect. 5. Finally, a summary of both the main developments and results is given in Sect. 6, along with some thoughts for potential improvements.
2.1 SIRTA and European airports
The data used in this study are mainly based on observations collected at SIRTA (Instrumented Site for Atmospheric Remote Sensing Research; Haeffelin et al., 2005), which is a French multi-instrumental atmospheric observatory located on a plateau 20 km south of Paris (48.713∘ N, 2.208∘ E; 160 m a.s.l.). SIRTA aims to study both physical and chemical processes at play in the atmosphere with a particular emphasis on clouds, aerosols, atmospheric boundary layer processes, and solar energy. Today, most of the 150 state-of-the-art instruments (active and passive remote sensing, in situ sensors) at SIRTA are set up in an area of about 1 km2. The plateau is a fog-prone region in France where about 35 fog events occur every year (∼ 10 000 min per fog season), making it particularly suitable for fog studies (Haeffelin et al., 2010; Wærsted et al., 2019). PFG1 field experiments took place at SIRTA from 2006 to 2014, where a synergistic suite of instruments was designed to document the entire fog life cycle in correlation with dynamical, thermodynamical, optical, and microphysical properties (e.g., Elias et al., 2009; Dupont et al., 2012, 2016).
Complementing the SIRTA observations, data from major European airports regularly affected by fog are considered to test the robustness of PFG2 in a range of local environments and meteorological conditions. The present study considers the following four airports: Paris Roissy-Charles de Gaulle, Zurich, Munich, and Vienna (Fig. 1).
2.2 Instruments and fog event statistics
Among all the instruments deployed at SIRTA, the present study makes use of a Vaisala CL31 ceilometer (generation CLE321) providing attenuated backscatter profiles at ∼ 910 nm, as well as cloud base height, using the operating procedures recommended by Kotthaus et al. (2016). In addition, scatterometers (Degreane DF20/20+/320) provide horizontal visibility at 4 m a.g.l., whereas both temperature and relative humidity observations are recorded by an automatic weather station at 2 m a.g.l. All airports considered in this study are equipped with a Vaisala CL31, automatic weather station, and visibilimeters (Table 1). The present study considers 9 years of measurements at SIRTA from 2011 to 2019, and up to two fog seasons at the Paris-Roissy, Vienna, Munich, and Zurich airports between 2014 and 2017 (Table 1).
The number of fog events for each site is derived using the Tardif and Rasmussen (2007) analysis procedure. Fog events are defined by a visibility lower than 1000 m detected for at least 30 min within a 50 min period (three of five blocks of 10 min). Two fog events are merged if they are separated by less than 1 h. The events retrieved by the Tardif and Rasmussen (2007) algorithm are considered afterwards as the reference for the PFG2 analysis. In this study, we only focus on fog events that correspond to RAD or STL, which represent more than 90 % of cases regardless of the sites considered. Note that it may represent much less at other locations such as, for instance, coastal sites, where advection fog prevails and which PFG2 is not designed to monitor. Note also that fog events retrieved following the Tardif and Rasmussen (2007) algorithm are not considered when data are missing from either the CL31 or the meteorological station measurements as these are required input data to PFG2. The total number of fog events considered in this study is about 250 at SIRTA (2011–2019) and up to 40 at each airport site, unevenly distributed between 2014 and 2017 (Table 1).
3.1 Overview
PFG2 has been designed to operate with relatively standard instruments, which are commonly found at national meteorological service sites, airports, and/or research observatories. The rationale for this approach is to develop a fog decision tool widely and easily applicable that enables the evolution of key physical parameters to be tracked in near real time for fog formation. In the same way as PFG1, PFG2 makes use of ALC measurements, together with visibility and relative humidity from a meteorological station. The current version of PFG2 retrieves fog alerts at a vertical and temporal resolution of 15 m and 1 min, respectively. PFG2 has also been entirely upgraded to Python 3, and the main advances (compared to PFG1) are as follows:
- i.
a more efficient memory management
- ii.
the development of the specific radiation (PFG2-RAD) and stratus lowering (PFG2-STL) fog modules
- iii.
an assessment of the fog life cycle (discriminating between formation and mature stages)
- iv.
new output visualization options (operational and reanalysis mode; note that we only present PFG2 output visualizations in reanalysis mode in this study. The operational mode corresponds to a simplified version with the visibility, attenuated backscatter profiles between 0 and 400 m, alert levels, and fog type retrieved from PFG2, together with the status of the algorithm).
The methodology of the PFG2 algorithm (Fig. 2) is divided into four main steps:
- a.
PFG2 is “turned ON” when the relative humidity measured at ground level exceeds a value of 85 % for a period of at least 10 min.
- b.
The visibility allows for the discrimination between the formation and mature fog stages. If the visibility is greater than 1000 m for a period of at least 10 min, a fog formation module is activated.
- c.
The distinction between RAD and STL fog type during the formation stage is based on the cloud fraction analysis deduced from ALC measurements. If the 2 h averaged cloud fraction between 0 and 1000 m a.g.l. is greater (lower) than 50 %, the STL (RAD) formation calculation is activated. To reliably distinguish between RAD and STL fog situations, the cloud fraction calculation is updated every hour.
- d.
PFG2 retrieves pre-fog alerts (low, moderate, high) every minute indicating the risk of fog formation.
The quantitative assessment of PFG2 algorithm performances at the five European sites is based on a contingency table analysis and the two following categorical statistics:
and
3.2 Radiation fog module
PFG1 was initially designed to monitor the early stages of RAD fog events by analyzing the rate of change in the aerosol-particle hygroscopic growth derived from ALC measurements in near real time. An in-depth analysis of the performance of PFG1 (see Sect. 5.1 for details about the methodology and Fig. 9) gave useful insights. Overall, PFG1 performance at SIRTA over 128 RAD fog events between 2011 and 2019 had a hit rate of 70 %. At the Paris-Roissy and Vienna airport sites, hit rates of about 90 % were achieved, while the performance was markedly lower than 50 % at the airport of Munich (37 %) and Zurich (31 %). This analysis reveals that a substantial number of RAD fog events were not detected at SIRTA and the Munich and Zurich airports using the PFG1 algorithm. Figure 3 shows histograms of visibility at both 4 and 20 m, together with attenuated backscatter profile statistics for the first 60 min of all RAD fog events that occurred at SIRTA during the observation period. Although observed values of horizontal visibility can fluctuate at the scale of a minute, the visibility measured at 4 m is lower than 1000 m for hit (missed) RAD fog events for ∼ 90 % (∼ 75 %) of the time. At 20 m, this situation is different since it represents 80 % (25 %) for hit (missed) RAD fog events. This discrepancy can be explained by the fact that missed RAD events correspond to shallow radiation fog layers, while RAD hit events are associated with thick radiation fog layers. The distinct distributions of the attenuated backscatter profiles related to hit/missed RAD fog events confirm this conclusion (Fig. 3c–d). Attenuated backscatter profiles associated with missed RAD events are on the order of 1 × 10−6 sr−1 m−1, except for the first range gate near the surface with values around 1 × 10−5 sr−1 m−1 (Fig. 3d). In contrast, the attenuated backscatter profiles associated with the thick RAD fog layers that resulted in PFG1 “hits” show high values ranging from 1 × 10−3 sr−1 m−1 at the surface to 1 × 10−6 sr−1 m−1 at 100 m and are around 1 × 10−7 sr−1 m−1 for higher altitudes (Fig. 3c). These two regimes are typical of thin and thick RAD fog events, respectively (Haeffelin et al., 2016). The thin RAD fog events occurred near the surface in a very shallow hydrated layer, where the ALC measurements are not able to monitor the aerosol hydration. According to Kotthaus et al. (2016), observation in the first range gate of the Vaisala CL31 measurement is of poor quality due to incomplete optical overlap. Therefore, PFG1 has difficulties to provide alerts for shallow radiation fog layers. Thin RAD fog events occur frequently at Munich and Zurich airports.
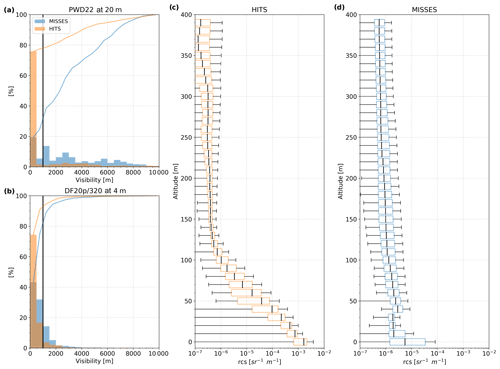
Figure 3Characterization of the first 60 min of radiation fog events recorded at SIRTA according to the performance of PFG1. Visibility distribution at 20 m (a) and 4 m (b), as well as boxplots of ALC-attenuated backscatter profiles associated to hits (c) and misses (d).
To incorporate also very shallow fog layers, a new approach based on a fuzzy logic algorithm has been implemented in PFG2. Here, the fuzzy logic algorithm (Mendel, 1995) transforms nonlinear data into scalar outputs referring to low, moderate, and high fog alerts (hereafter referred to as LOW, MOD, HIGH, respectively). The fuzzy logic algorithm has been selected due to its simple implementation and its low computational cost. Here it relies on a combination of visibility measurements and attenuated backscatter ratio gradient (RG in Haeffelin et al., 2016). RG allows the aerosol activation process (i.e., proxy to monitor for hygroscopic growth dynamics) to be monitored and is derived from a reference ALC-attenuated backscatter profile determined during pre-fog conditions. For each considered alert, a typical range of values is assigned to the visibility and RG variables. Each range of values is expressed as a membership function (MBF) and finally combined in a process called aggregation (A). The fuzzy logic method employed in the PFG2-RAD module uses one-dimensional trapezoidal MBFs (F) to calculate the aggregation score that describes how well the observations characterize the imminent fog formation. The general expression of the aggregation score Alevel(t) as a function of MBFs and alerts is given in Eq. (1):
where level refers to the considered alert, Fvisi represents the MBF associated with the visibility, and FRG is the MBF associated with the RG.
Table 2The trapezoid parameters for both RAD and STL modules and their associated variables used in PARAFOG v2.0.
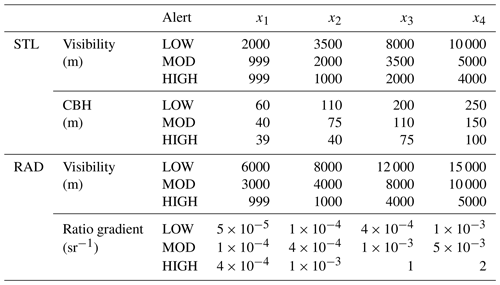
The MBFs are assumed to have trapezoidal shape and are described as follows:
where x is the considered variable, and x1 and x4 are the lower and x2 and x3 the upper corners of the trapezoid. Note that the complete parameters associated with the trapezoidal functions for fog formation in PFG2 are given in Table 2. The final score Alevel(t) in Eq. (1) is converted to a RAD fog alert level (LOW, MOD, or HIGH) by assigning the alert with the maximum score (i.e., maximum rule value).
Complementing the PFG2-RAD module, the analysis of RG values, together with its thickness, allows for discriminating if a thin or thick fog layer will occur. A thick RAD fog layer is characterized by RG values greater than 4 × 10−4 sr−1 m−1 over a layer of at least 30 m thickness. If these conditions are not met, then it is considered as a thin RAD fog layer situation. These values were empirically derived from about 10 RAD fog events analyzed at SIRTA. Discrimination between thin or thick RAD layers is only performed for RAD HIGH alerts.
3.3 Stratus lowering module
The PFG2-STL module is again based on a fuzzy logic algorithm. Since low stratus clouds can be close to the ground for hours before their cloud base height starts to descend, thereby causing a fog event, exploiting the attenuated backscatter ratio gradient as for PFG2-RAD does not provide useful insights. Hence, the PFG2-STL fuzzy logic algorithm relies on a combination of visibility and cloud base height (CBH) observations (Fig. 4). The PFG2-STL module uses one-dimensional trapezoidal MBFs (F), together with weights (w), to calculate the aggregation score as described in Eq. (2):
where level refers to the respective alert, Fvisi and FCBH represent the MBF associated with visibility and CBH, respectively, and wvisi and wCBH are related to the weight given to the visibility and CBH, respectively. As stratus clouds may oscillate a few tens of meters above the surface before lowering and becoming a fog, we define two aggregation equations to avoid discontinuity in the alerts delivered.
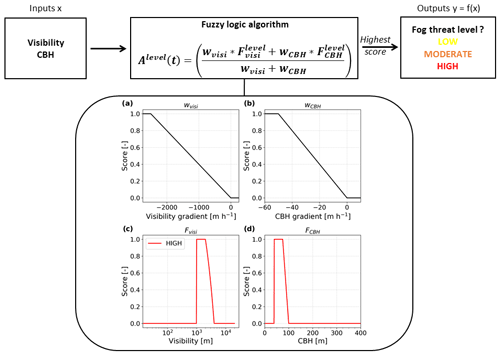
Figure 4Fuzzy logic scheme used in the PARAFOG v2.0 algorithm for the STL module. Weights associated with (a) visibility and (b) CBH gradients. HIGH alert membership functions for (c) the visibility and (d) the CBH values.
The weights are determined empirically from the temporal gradient of both visibility and CBH variables, considering the 60 min prior to STL fog events that occurred at SIRTA between 2011 and 2019. The weights are standardized with a linear scaling as follows:
where x is the original value of the considered variable (temporal evolution of visibility or CBH), and xmin (xmax) is the minimum (maximum) bound of x. The boundaries employed in this study are 0 to −2500 m h−1 for the visibility gradient and 0 to −50 m h−1 for the CBH gradient (Fig. 4a–b). Note that these thresholds may need to be adapted for sites with very different fog characteristics. The final score Alevel(t) in Eq. (3) is converted to an STL fog alert level (LOW, MOD, or HIGH) by assigning the alert with the highest score (i.e., maximum rule value).
Here we present the ability of the PFG2 algorithm to anticipate the alert level for three different meteorological situations prior to fog formation at Munich airport, SIRTA observatory, and Zurich airport.
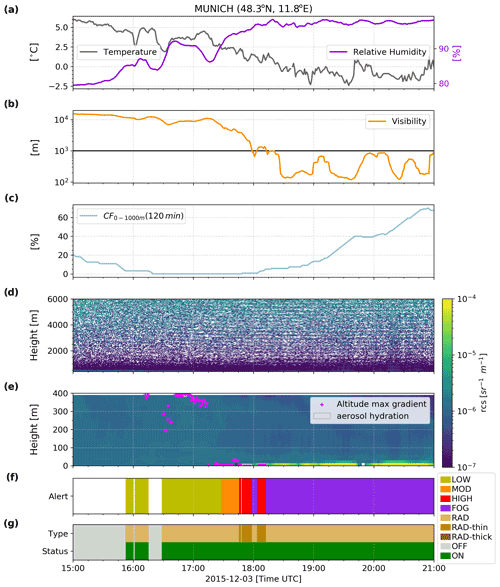
Figure 5Time series presenting measurements and the corresponding retrieved alert level outputs from PARAFOG v2.0 during a thin radiation fog formation on 3 January 2011 at Munich airport. (a) Temperature and relative humidity, (b) visibility at 4 m, (c) the cloud fraction (CF) between 0 and 1000 m over the last 2 h, (d) ALC-attenuated backscatter between 400 and 6000 m, (e) ALC-attenuated backscatter between 0 and 400 m (color contours), together with the altitude of the maximum gradient (fuchsia points) and the aerosol hydration (gray contours), (f) alert levels retrieved from PFG2, and (g) fog type and PFG2 status.
Figure 5 shows the time series measurements and the corresponding alert level outputs from PFG2 during a thin radiation fog formation on 3 March 2015 at Munich airport. Weather conditions are representative of a RAD fog event, with a decrease (increase) in temperature (relative humidity) at the surface level in response to a radiative cooling during this late winter afternoon. The reference attenuated backscatter profile is on the order of 1 × 10−7 sr−1 m−1, with a reference RHref = 71 %. The altitude of the maximum gradient (Hmax in Haeffelin et al., 2016 – fuchsia dots in Fig. 5e) marks the atmospheric level with the most rapid hygroscopic growth of aerosol particles. The aerosol hydration (gray contour in Fig. 5e) represents the entire layer which is hydrating before the attenuated backscatter exceeds 1 × 10−5 sr−1 m−1. In this case study, the first stages of the aerosol hydration occur above 300 m, reaching layers near the surface at about 45 min before the onset of the RAD fog event at 18:00 UTC. This thin fog layer would have been missed by PFG1, which is only based on the evolution of the rate of change in the aerosol-particle hygroscopic growth and only showed a few low to moderate alerts before 18:00 UTC. The combination of the visibility and the attenuated backscatter ratio gradient in the PFG2-RAD fuzzy logic algorithm, however, clearly improves the anticipation of such very shallow fog layers. With PFG2, the first low alerts occur 2h before the fog onset, while the high alerts appear about 15 min ahead of the fog event (Fig. 5f). Further, the automatic assessment of the fog layer thickness classifies the event as a thin fog layer (Fig. 5g).
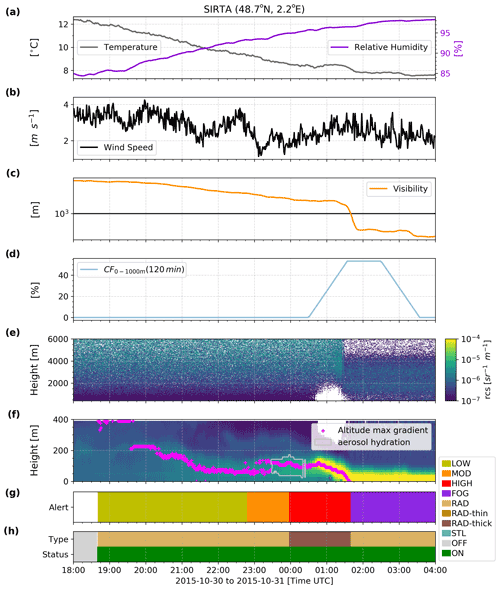
Figure 6Time series presenting measurements and the corresponding retrieved alert level outputs from PARAFOG v2.0 during a thick radiation fog formation on 30–31 October 2015 at SIRTA. (a) Temperature and relative humidity, (b) wind speed, (c) visibility, (d) the cloud fraction (CF) between 0 and 1000 m over the last 2 h, (e) ALC-attenuated backscatter between 400 and 6000 m, (f) ALC-attenuated backscatter between 0 and 400 m (color contours), together with the altitude of the maximum gradient (fuchsia points) and the aerosol hydration (gray contours), (g) alert levels retrieved from PFG2, and (h) fog type and PFG2 status.
Figure 6 is the same as Fig. 5 for the case of 31 October 2015 at SIRTA. This is a classic thick RAD fog event. During the night of 30 to 31 October, radiative cooling at the surface occurred during strong anticyclonic conditions located over the Paris region. As shown in Fig. 6, the temperature (relative humidity) decreased (increased) from ∼ 13 to 8 ∘C (from ∼ 80 % to 99 %), in the presence of low-wind (< 4 m s−1) and clear sky conditions during the first part of the night. As a result of the radiative cooling, the visibility was reduced, leading to a fog onset at 01:40 UTC. Overall, the PFG2-RAD module performs well for this fog event since it has gradually delivered low to high alerts about 6 h before the fog onset. The reference attenuated backscatter profile is on the order of 1 × 10−7 sr−1 m−1, with a reference RHref of 68 %. The aerosol activation started in altitudes ranging from 50 to 200 m a.g.l. For this RAD fog case, the first HIGH alert occurred ∼ 100 min before fog onset, and the PFG2-RAD algorithm correctly identified the fog type as a thick radiation fog layer.
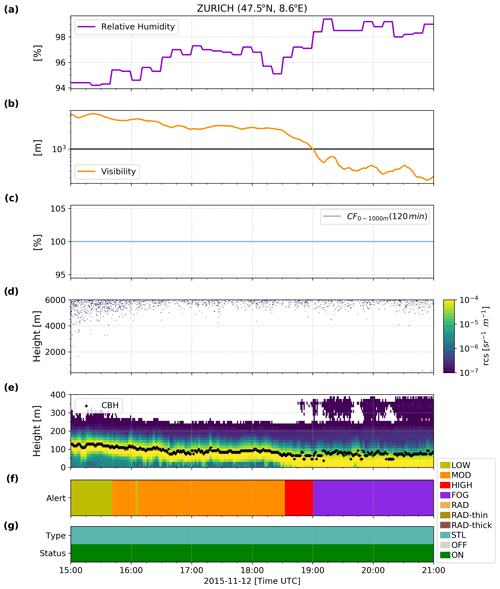
Figure 7Time series presenting measurements and the corresponding retrieved alert level outputs from PARAFOG v2.0 during stratus lowering fog formation on 12 November 2015 at Zurich airport. (a) Relative humidity, (b) visibility, (c) the cloud fraction (CF) between 0 and 1000 m over the last 2 h, (d) ALC-attenuated backscatter between 400 and 6000 m, (e) ALC-attenuated backscatter between 0 and 400 m (color contours), together with the altitude of the maximum gradient (fuchsia points) and the aerosol hydration (gray contours), (f) alert levels retrieved from PFG2, and (g) fog type and PFG2 status.
Figure 7 shows a classic STL fog event with a cloud base that fluctuates around 100 m a.g.l. 4 h before reaching the surface, causing a fog onset at 19:00 UTC on 12 November 2015 at Zurich international airport. This STL fog event is characterized by very high RH (> 95 %) at the surface (Fig. 7a) and a 100 % cloud fraction between 0 and 1000 m a.g.l. over the 2 h prior to the event (Fig. 7c, d, e). This STL fog event is characterized by a visibility which decreases from 4 × 103 to 2 × 103 m over the course of more than 3 h (15:00–18:30 UTC) before dropping rapidly (18:30–19:00 UTC) when the cloud base reached the surface (Fig. 7e). For such an STL fog formation, PFG2 alerts increase gradually from LOW to MOD (15:00–15:41 UTC) and then from MOD to HIGH (15:42–18:32 UTC), with HIGH alert reported more than 25 min prior to the fog onset.
The performance of the PFG2 algorithm is evaluated at five European sites, namely SIRTA, and the airports at Vienna, Munich, Zurich, and Paris-Roissy.
5.1 Assessment methodology
A specific assessment methodology has been designed to evaluate alerts provided by the PFG2 algorithm. The corresponding diagram of the PFG2 assessment methodology is shown in Fig. 8, while the overall assessment framework is described hereafter:
-
Since visibility measurements may fluctuate rapidly, only fog events retrieved by the Tardif and Rasmussen (2007) algorithm are evaluated.
-
There are two possibilities to define the total assessment period. Either it ends with a previous fog event or by reaching a set time limit of 3 (24) h to agree with the time involved in RAD (STL) fog formation physical processes (Dupont et al., 2012; Haeffelin et al., 2016). All other alerts occurring outside this period are not considered.
-
Although PFG2 is able to deliver an alert every minute, PFG2 performance is assessed on sub-periods of 45 min. The rationale for this approach is that PFG2 allows near-real-time tracking of physical key parameters at play within fog formation processes, which evolve over longer periods of time (longer than 1 min). During a 45 min sub-period, the PFG2 alerts are transformed into an alarm (LOW, MOD, HIGH) that aims to summarize the global behavior of alerts. Alarms are defined as follows:
-
A minimum of 10 alerts (N) in a 45 min period is required to trigger an alarm.
-
If one or more alert levels are present in a sub-period and exceed N, then the alert with the highest level defines the alarm reported.
-
If the first 45 min sub-period before fog onset ends with a HIGH alert and the gradient of the alert level over the last 15 min is null or positive (e.g., LOW to MOD, and MOD to HIGH), then a HIGH alarm is assigned.
-
After an episode of fog, a period of 90 min (two 45 min sub-periods) is removed from the performance analysis procedure if no new fog event occurs in that time. Given the visibility exceeds 1000 m during these 90 min after the event, PFG2 automatically calculates a formation risk (Fig. 2). However, both ALC and meteorological measurements may present the same signatures as for “real” fog formation (e.g., during fog dissipation by lifting) and may mislead the performance analysis.
-
The successive sub-periods presenting the same alarm levels are gathered in a single alarm (e.g., two consecutive HIGH alarms are counted as one).
-
-
Finally, PFG2 performance is assessed based on the alarm retrieved. If a HIGH (LOW, MOD, or NONE) alarm is encountered before a fog event, it is considered as a hit (miss). If a HIGH alarm is encountered at another period with no consecutive HIGH alarm up to the start of a fog event, it is considered as a false alarm. Note that the LOW and MOD alarms are not considered for the quantitative assessment of PFG2 performance. These alarms are intended as indicators of conditions favorable for fog formation but without specific lead times.
5.2 Application to SIRTA and European airport sites
The quantitative assessment of PFG2 algorithm performances at the European sites is presented in Fig. 9, whereas the contingency table is shown in Table 3.
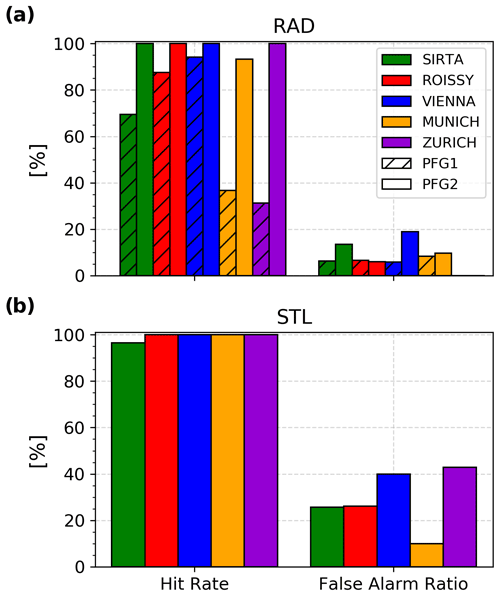
Figure 9PARAFOG scores for (a) radiation and (b) stratus lowering fog events for the SIRTA and EU sites. The hatched bars correspond to the scores obtained with PARAFOG v1.
Overall, one can note from Fig. 9a that the PFG2-RAD performance is greatly improved compared to PFG1, regardless of the site. The new fuzzy logic algorithm allows 100 % of RAD fog events at SIRTA and Paris-Roissy, Vienna, and Zurich airports to be detected, as well as 93 % at the Munich airport. RAD events with a shallow fog layer are well anticipated by the PFG2-RAD module, which allows us to correctly detect the 30 % missing at SIRTA (up to 60 % at Munich and Zurich airports) in comparison to PFG1. False alarm ratios are on the order of 10 %, with slightly higher values at SIRTA (14 %) and the Vienna airport (19 %).
Figure 9b presents the PFG2-STL module assessment at the test sites. Again, the statistics are mostly similar between locations. While PFG2-STL does not miss any STL events at the airport sites, at SIRTA the hit rate is very slightly reduced to 96 %. This demonstrates the efficiency of the fuzzy logic algorithm integrated into the PFG2-STL module. Overall, the false alarm rate associated with the PFG2-STL module is 26 % for the 114 STL fog events at SIRTA between 2011 and 2019. The statistics are similar for Paris-Roissy (26 %), whereas it becomes 10 % at Munich, 40 % at Vienna, and 43 % at Zurich. However, these results must be strengthened with a more substantial database for the different airports which only present a few cases of STL over one or two fog seasons (Table 1).
Note that this evaluation methodology has certain limitations. Arbitrary choices to consider only a 45 min alarm sub-period, or to have a minimum number of 10 alerts to trigger an alarm, may affect overall final performance. These parameters are optimized to provide a good compromise between hit, false alarm, or miss with the present evaluation scheme; however, a sensitivity study may optimize the results. In addition, this method only evaluates the performance of PFG2 when fog events occur. Outside of these evaluation periods (3 h for RAD and 24 h for STL), PFG2 may deliver high alerts/alarms in pre-fog conditions such as during a stratus lowering which has a cloud base height “stuck” a few tens of meters above the ground without leading necessarily to a subsequent fog event. This does not affect the PFG2 hit rate (number of hits or misses) but tends to underestimate the number of false alarms presented in this study.
5.3 First high-alert characterization at SIRTA
Another important parameter of the statistical PFG2 assessment relies on the characterization of the first HIGH alert that results in a subsequent fog event during periods of hits. Here, the first high alert in the longest block of high alerts since the start of a fog event is analyzed over a 180 min period. Figure 10 shows the distribution of these first HIGH alerts for both RAD and STL fog events at SIRTA. Each hour is characterized by a different regime. The probability that a first “true” high alert occurs more than 2 h before a fog event is relatively low, representing about 20 % (5 %) for RAD (STL) events. These probabilities are doubled between −120 and −60 min (RAD ∼ 40 %; STL ∼ 10 %), while it sharply increases (until reaching 100 %) over the last hour prior to a RAD/STL fog event. Here, the discrepancies between the first HIGH alerts for the PFG2-RAD and PFG2-STL modules highlight the difference in terms of dynamics between the two fog types. Radiative fog events occur most of the time during nighttime radiation cooling, characterized by low winds and high-pressure conditions. The hygroscopic growth of condensation nuclei is progressive and allows PFG2-RAD to anticipate well the related fog events by combining the visibility and the RG measurements. However, STL fog events may oscillate a few tens of meters above the surface before lowering and leading to a fog. This more “sudden” character is found in the first HIGH alerts of PFG2-STL which sometimes starts to retrieve them only a few minutes before the fog onset. As a result, PFG2 has already a 40 % (against 25 %) chance to have delivered the first HIGH alert for the RAD (STL) module 1 h prior to a fog formation.
A second version of PARAFOG (PFG2) has been developed to retrieve pre-fog alert levels and to discriminate between RAD and STL fog situations based on the analysis of ALC and meteorological station measurements in near real time. Two distinct modules have been developed to closely monitor the evolution of RAD and STL fog events which involve different physical processes. They rely on innovative fuzzy logic schemes that aim to combine both visibility and ALC measurements through one-dimensional trapezoidal membership functions (and weights for the STL module) to characterize the fog formation threat level. In addition to these two main modules, some important advances have been carried out within PFG2 such as the redesign of the code in Python 3 and a new memory management, together with new visualization outputs for both operational and research purposes.
Overall, the pre-fog alert levels retrieved by both the PFG2-RAD and PFG2-STL modules at SIRTA and both Munich and Zurich airports are found to be consistent with the local weather analysis. The pre-fog alert level gradually rises from LOW to MOD and then from MOD to HIGH as one gets closer to a fog event and the visibility decreases. The HIGH pre-fog alerts are found to occur between 30 and 60 min prior to fog formation regardless of the fog type considered, whereas the associated thin/thick discrimination matches well with RAD fog events.
An original approach to assess the performance of the pre-fog alert levels retrieved by both the PFG2-RAD and PFG2-STL algorithms has been subsequently proposed to support these results. This analysis is based upon comparisons of predicted and observed fog events over sub-periods of 45 min and the associated alarms deduced from the raw alert analysis. About 250 (up to 50) fog events that occurred at SIRTA (Munich, Zurich, Vienna, and Paris-Roissy airports) between 2011 and 2019 (2014–2017) have been processed to assess the performance of the PFG2 algorithm. The retrieved pre-fog alert levels by the new PFG2-RAD module greatly improve the performance in comparison to PFG1 that failed to detect shallow fog events due to poorly defined ALC measurements in the first range gates. The PFG2-RAD module presents a hit rate of about 100 % and a false alarm ratio on the order of 10 % regardless of the considered site. The retrieved pre-fog alert levels by the PFG2-STL algorithm are also defined by a hit rate of about 100 % and a false alarm ratio on the order of 30 %. Finally, the first HIGH alerts that result in a subsequent fog event are found to occur for periods of time ranging from −120 min to fog onset, with the first HIGH alerts occurring earlier for RAD than STL cases.
These encouraging results attest to a good performance of the PFG2 algorithm which warrants an extended application of the method at more locations. Implementing the PFG2 algorithm at the European scale, via the PROBE COST action (http://www.probe-cost.eu/, last access: 9 December 2021) and the E-PROFILE network, will help to determine whether the statistics obtained in this study are generally representative. In addition, it should be examined if and how other observations could improve the algorithm performance. For example, wind shear analysis could be used to support fog formation prediction by assessing the ambient horizontal wind speed and checking whether it may generate sufficient turbulence to prevent fog. Also, the combination of a cloud radar and a microwave radiometer in near real time to retrieve the minimum amount of liquid water path which is necessary for a fog to remain at the surface (Toledo et al., 2021) could be implemented to estimate the fog dissipation probability and enhance PFG2.
Data access can be requested for free following the conditions indicated in the SIRTA data policy (https://sirta.ipsl.fr/data_policy.html, last access: 9 December 2021; SIRTA observatory website: https://sirta.ipsl.fr/, last access: 9 December 2021; data request form: https://sirta.ipsl.fr/data_form.html, last access: 9 December 2021).
JFR, MH, and JCD developed the concept of the paper. JFR designed the methodology, performed the analysis, and interpreted the results. MH, JCD, FT, and SK, contributed to the design and discussion of the work. MAD and JFR performed the python 3 update and designed the main code structure of PFG2. JFR, with contribution from all authors, prepared the manuscript.
The contact author has declared that neither they nor their co-authors have any competing interests.
Publisher’s note: Copernicus Publications remains neutral with regard to jurisdictional claims in published maps and institutional affiliations.
The contribution of the first author and the PFG2 project were supported by the “Direction Générale de l'Armement” under grant DGA 2018 60 0074. Felipe Toledo thanks the French Association Nationale de la Recherche et de la Technologie (ANRT) and the company Meteomodem for their funding contribution. The authors would like to thank Rafael Eigenmann and Ulrich Goersdorf (DWD) for kindly providing the data for Munich airport, Maxime Hervo (Meteoswiss) for kindly providing the data for Zurich airport, Philipp Kneringer (Universität Innsbruck) for kindly providing the data for Vienna airport, and Météo-France for kindly providing the data for Paris-Roissy airport. Also, we would like to thank the PROBE COST action for setting up an EU-wide discussion framework regarding applications and methods for fog nowcasting methods based on remote sensing measurements.
This research has been supported by the Direction Générale de l'Armement (DGA, grant no. 2018 60 0074).
This paper was edited by Simone Lolli and reviewed by two anonymous referees.
Bergot, T.: Large-eddy simulation study of the dissipation of radiation fog, Q. J. Roy. Meteor. Soc., 142, 1029–1040, https://doi.org/10.1002/qj.2706, 2016.
Bergot, T., Carrer, D., Noilhan, J., and Bougeault, P.: Improved Site-Specific Numerical Prediction of Fog and Low Clouds: A Feasibility Study, Weather Forecast., 20, 627–646, https://doi.org/10.1175/WAF873.1, 2005.
Cermak, J. and Bendix, J.: A novel approach to fog/low stratus detection using Meteosat 8 data, Atmos. Res., 87, 279–292, https://doi.org/10.1016/j.atmosres.2007.11.009, 2008.
Cermak, J. and Bendix, J.: Detecting ground fog from space – A microphysics-based approach, Int. J. Remote Sens., 32, 3345–3371, https://doi.org/10.1080/01431161003747505, 2011.
Dietz, S. J., Kneringer, P., Mayr, G. J., and Zeileis, A.: Forecasting Low-Visibility Procedure States with Tree-Based Statistical Methods, Pure Appl. Geophys., 176, 2631–2644, https://doi.org/10.1007/s00024-018-1914-x, 2019.
Dupont, J., Haeffelin, M., Protat, A., Bouniol, D., Boyouk, N., and Morille, Y.: Stratus–Fog Formation and Dissipation: A 6-Day Case Study, Bound.-Lay. Meteorol., 143, 207–225, https://doi.org/10.1007/s10546-012-9699-4, 2012.
Dupont, J. C., Haeffelin, M., Stolaki, S., and Elias, T.: Analysis of dynamical and thermal processes driving fog and quasi-fog life cycles using the 2010–2013 ParisFog dataset, Pure Appl. Geophys., 173, 1337–1358, 2016.
Egli, S., Thies, B., Drönner, J., Cermak, J., and Bendix, J.: A 10 year fog and low stratus climatology for Europe based on Meteosat Second Generation data, Q. J. Roy. Meteor. Soc., 143, 530–541, https://doi.org/10.1002/qj.2941, 2017.
Egli, S., Thies, B., and Bendix, J. A: Hybrid Approach for Fog Retrieval Based on a Combination of Satellite and Ground Truth Data, Remote Sens., 10, 628, https://doi.org/10.3390/rs10040628, 2018.
Elias, T., Haeffelin, M., Drobinski, P., Gomes, L., Rangognio, J., Bergot, T., Chazette, P., Raut, J.-C., and Colomb, M.: Particulate contribution to extinction of visible radiation: pollution, haze, and fog, Atmos. Res., 92, 443–454, https://doi.org/10.1016/j.atmosres.2009.01.006, 2009.
Gandhidasan, P. and Abualhamayel, H. I.: Fog collection as a source of fresh water supply in the Kingdom of Saudi Arabia, Water Environ. J., 21, 19–25, https://doi.org/10.1111/j.1747-6593.2006.00041.x, 2007.
Gultepe, I., Tardif, R., Michaelides, S. C., Cermak, J., Bott, A., Bendix, J., Müller, M. D., Pagowski, M., Hansen, B., Ellrod, G., Jacobs, W., Toth, G., and Cober, S. G.: Fog research: a review of past achievements and future perspectives, Pure Appl. Geophys., 164, 1121–1159, https://doi.org/10.1007/s00024-007-0211-x, 2007.
Gultepe, I., Pearson, G., Milbrandt, J. A., Hansen, B., Platnick, S., Taylor, P., Gordon, M., Oakley, J. P., and Cober, S. G.: The Fog Remote Sensing and Modelling Field Project, Bull. Amer. Meteor. Soc., 90, 341–360, https://doi.org/10.1175/2008BAMS2354.1, 2009.
Gultepe, I., Sharman, R., Williams, P. D., Zhou, B., Ellrod, G., Minnis, P., Trier, S., Griffin, S., Yum, S. S., Gharabaghi, B., Feltz, W., Temimi, M., Zhaoxia, P., Storer, L. N., Kneringer, P., Weston, M. J., Chuang, H., Thobois, L., Dimri, A. P., Dietz, S. J., França, G. B., Almeida, M. V., and Albquerque Neto, F. L.: A Review of High Impact Weather for Aviation Meteorology, Pure Appl. Geophys., 176, 1869–1921, https://doi.org/10.1007/s00024-019-02168-6, 2019.
Haeffelin, M., Barthès, L., Bock, O., Boitel, C., Bony, S., Bouniol, D., Chepfer, H., Chiriaco, M., Cuesta, J., Delanoë, J., Drobinski, P., Dufresne, J.-L., Flamant, C., Grall, M., Hodzic, A., Hourdin, F., Lapouge, F., Lemaître, Y., Mathieu, A., Morille, Y., Naud, C., Noël, V., O'Hirok, W., Pelon, J., Pietras, C., Protat, A., Romand, B., Scialom, G., and Vautard, R.: SIRTA, a ground-based atmospheric observatory for cloud and aerosol research, Ann. Geophys., 23, 253–275, https://doi.org/10.5194/angeo-23-253-2005, 2005.
Haeffelin, M., Bergot, T., Elias, T., Tardif, R., Carrer, D., Chazette, P., Colomb, M., Drobinski, P., Dupont, E., Dupont, J.-C., Gomes, L., Musson-Genon, L., Pietras, C., Plana-Fattori, A., Protat, A., Rangognio, J., Raut, J.-C., Rémy, S., Richard, D., Sciare, J., and Zhang, X.: PARISFOG: Shedding New Light on Fog Physical Processes, B. Am. Meteorol. Soc., 91, 767–783, 2010.
Haeffelin, M., Laffineur, Q., Bravo-Aranda, J.-A., Drouin, M.-A., Casquero-Vera, J.-A., Dupont, J.-C., and De Backer, H.: Radiation fog formation alerts using attenuated backscatter power from automatic lidars and ceilometers, Atmos. Meas. Tech., 9, 5347–5365, https://doi.org/10.5194/amt-9-5347-2016, 2016.
Kneringer, P., Dietz, S. J., Mayr, G. J., and Zeileis, A.: Probabilistic Nowcasting of Low-Visibility Procedure States at Vienna International Airport During Cold Season, Pure Appl. Geophys., 176, 2165–2177, https://doi.org/10.1007/s00024-018-1863-4, 2019.
Kotthaus, S., O'Connor, E., Münkel, C., Charlton-Perez, C., Haeffelin, M., Gabey, A. M., and Grimmond, C. S. B.: Recommendations for processing atmospheric attenuated backscatter profiles from Vaisala CL31 ceilometers, Atmos. Meas. Tech., 9, 3769–3791, https://doi.org/10.5194/amt-9-3769-2016, 2016.
Mazoyer, M., Lac, C., Thouron, O., Bergot, T., Masson, V., and Musson-Genon, L.: Large eddy simulation of radiation fog: impact of dynamics on the fog life cycle, Atmos. Chem. Phys., 17, 13017–13035, https://doi.org/10.5194/acp-17-13017-2017, 2017.
Mendel, J.: Fuzzy logic systems for engineering: a tutorial, P. IEEE, 83, 345–377, https://doi.org/10.1109/5.364485, 1995.
Menut, L., Mailler, S., Dupont, J. C., Haeffelin, M., and Elias, T.: Predictability of the meteorological conditions favourable to radiative fog formation during the 2011 Parisfog campaign, Bound.-Lay. Meteorol., 150, 277–297, 2014.
Philip, A., Bergot, T., Bouteloup, Y., and Bouyssel, F.: The Impact of Vertical Resolution on Fog Forecasting in the Kilometric-Scale Model AROME: A Case Study and Statistics, Weather Forecast., 31, 1655–1671, https://doi.org/10.1175/WAF-D-16-0074.1, 2016.
Román-Cascón, C., Steeneveld, G., Yagüe, C., Sastre, M., Arrillaga, J., and Maqueda, G.: Forecasting radiation fog at climatologically contrasting sites: evaluation of statistical methods and WRF, Q. J. Roy. Meteor. Soc., 142, 1048–1063, https://doi.org/10.1002/qj.2708, 2016.
Steeneveld, G. J., Ronda, R. J., and Holtslag, A. A. M.: The challenge of forecasting the onset and development of radiation fog using mesoscale atmospheric models, Bound.-Lay. Meteorol., 154, 265–289, https://doi.org/10.1007/s10546-014-9973-8, 2015.
Tardif, R. and Rasmussen, R. M.: Event-based climatology and typology of fog in the New York City region, J. Appl. Meteorol. Clim., 46, 1141–1168, https://doi.org/10.1175/JAM2516.1, 2007.
Toledo, F., Haeffelin, M., Wærsted, E., and Dupont, J.-C.: A new conceptual model for adiabatic fog, Atmos. Chem. Phys., 21, 13099–13117, https://doi.org/10.5194/acp-21-13099-2021, 2021.
Wærsted, E. G., Haeffelin, M., Steeneveld, G.-J., and Dupont, J.-C.: Understanding the dissipation of continental fog by analysing the LWP budget using idealized LES and in situ observations, Q. J. Roy. Meteor. Soc., 145, 784–804, https://doi.org/10.1002/qj.3465, 2019.
World Meteorological Organization: Cloud Atlas, available at: https://cloudatlas.wmo.int/en/fog-compared-with-mist.html, last access: 9 December 2021.